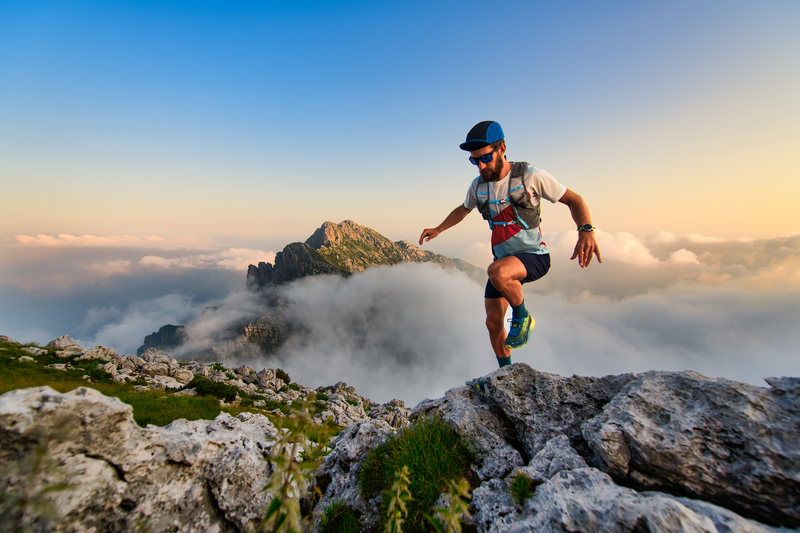
95% of researchers rate our articles as excellent or good
Learn more about the work of our research integrity team to safeguard the quality of each article we publish.
Find out more
ORIGINAL RESEARCH article
Front. Sustain. Food Syst. , 10 January 2025
Sec. Agricultural and Food Economics
Volume 8 - 2024 | https://doi.org/10.3389/fsufs.2024.1510328
This article is part of the Research Topic Harnessing Digital Innovation for Sustainable Agricultural Development View all 13 articles
Enhancing agricultural economic resilience is a critical component for ensuring sustainable agricultural development and promoting agricultural modernization. To explore the diverse influencing factors and effective pathways for enhancing agricultural economic resilience in the context of digital transformation in China, this study constructs a theoretical model of data elements empowering agricultural economic resilience based on the “Technology-Organization-Environment” framework. Using dynamic QCA methods, panel data from 30 provinces and municipalities in China from 2012 to 2022 are analyzed to configure the factors influencing agricultural economic resilience. The results indicate that no single variable constitutes a necessary condition for high agricultural economic resilience. With the introduction of time effects, it is found that digital inclusive finance and the necessity of agricultural industry digitalization have been increasing year by year. The patterns of enhancing agricultural economic resilience can be summarized as follows: the market-driven industrial technological innovation ecosystem optimization model, the TOE-enabled agricultural digital development model and the government-led cultural promotion of agricultural digital transformation model, all showing significant temporal and regional effects. Based on these findings, it is recommended that regions strengthen the synergistic interaction among elements in the technology, organization, and environment dimensions and formulate differentiated strategies for enhancing agricultural economic resilience tailored to local economic levels and the actual development of agricultural industries. These insights provide both theoretical and practical value for enhancing agricultural economic resilience in various regions worldwide.
Agriculture, as the cornerstone of global food security and rural development, plays an essential role in ensuring the supply of global food, reducing hunger, and alleviating poverty. In the face of intensifying global climate change and environmental challenges, enhancing the adaptability and resilience of agricultural systems is crucial for addressing extreme weather events, protecting the ecological environment, and promoting sustainable development (Hertel et al., 2021; Meuwissen et al., 2019). The United Nations Development Program (UNDP) has published the “UNDP Issues Brief on Resilient Food and Agriculture,” which discusses the impact of climate change on food security and emphasizes the importance of increasing the resilience of agricultural products to climate change. In the contemporary era, the world is undergoing the most significant transformations of the century, characterized by an escalation in geopolitical risks, frequent extreme weather disasters, the rise of trade protectionism and unilateralism, the Russia-Ukraine conflict, and heightened inflation, all of which contribute to the growing instability of the global economy. Many nations are confronting recession risks of varying degrees (Zhou et al., 2023). Against this backdrop, China’s economy has maintained a steady and forward trajectory, emerging as the major economy with the highest economic growth rate during the same period. Undoubtedly, China’s economic system possesses formidable resistance and adaptive capabilities when subjected to inevitable external shocks. Investigating the enhancement pathways of China’s agricultural economic resilience holds substantial significance for the high-quality development of global agriculture. As an ancient agricultural nation and a country with a large farming population, China, with a population of 1.4 billion and over 60% of its workforce engaged in farming, has seen its grain production reach 695.41 million tons in 2023, an increase of 8.88 million tons from the previous year (source: official website of Ministry of Agriculture of the People’s Republic of China for Rural Affairs). China has consistently played a pivotal role in stabilizing the global agricultural product market. The No. 1 central document of China in 2023 underscores the imperative to forge an agricultural powerhouse characterized by robust technological equipment, resilient management systems, and an industry endowed with formidable adaptability. Amidst the ongoing transition from traditional to modern agriculture in China, the intertwined natural and market risks have impeded the progress of agricultural modernization. Enhancing the economic resilience of agriculture to fulfill its role as a “stabilizer” and ballast for societal and economic development represents not only a pivotal pathway toward promoting high-quality agricultural development but also a crucial endeavor for China’s overall socio-economic prosperity.
A large number of scholars have conducted research on the issue of improving the resilience of agricultural economy. With the wide application of big data, cloud computing, artificial intelligence and other technologies in agriculture and rural areas, data elements have been fully integrated into every link of agricultural production and accelerated the process of agricultural modernization (Liu C. et al., 2023). As a basic resource and key factor of production in the era of digital economy, data factor is a new factor of production after traditional factors such as land, labor, capital and technology (Liu, 2024). It refers to data resources that participate in social production and operation activities and bring economic benefits to owners or users. It has the characteristics of non-competition, easy replication and element coordination (Chen and Xu, 2024). The optimal allocation of data elements can promote the transformation and upgrading of agricultural production modes, and play an important role in high-quality and sustainable agricultural development (Liu, 2024), thus enhancing the resilience of agricultural economy. Sun et al. (2024) measured data elements from three aspects: data foundation support, data transformation ability and data industry application, and proposed that there is a two-way positive promoting effect between data elements and rural revitalization (Sun et al., 2024). Liu (2024) measured data elements from four dimensions: data element configuration basis, data element application level, data element sharing level, and data element market size, and found that data elements can significantly promote high-quality agricultural development (Liu, 2024). It can be seen that the role of digital infrastructure, digital technology innovation, digital industry development and digital financial level under the data elements on the high-quality development of agriculture and rural areas has received extensive attention from the academic community. However, as a special labor product, data factor has both technical and social attributes (Guan and Qin, 2021), so it can give full play to its amplification and superposition effect when integrated with other factors. Therefore, this paper integrates data factor into the “technology-organization-environment” (TOE) framework. Explore how synergies between data elements and other elements enhance the resilience of the agricultural economy.
In addition, in terms of research methods, scholars mainly explore the causal relationship between various factors and agricultural economic resilience based on traditional empirical and static QCA methods. For example, Chao and Li (2024) demonstrated that agricultural production agglomeration can enhance agricultural economic resilience. Hao and Tan (2022) pointed out that the construction of digital countryside can better improve the resilience of China’s food system in areas with higher circulation digitalization and industrial integration. What’s more, Bai (2024) used the case study method to extract the action framework of “technology-institution-subject” digital technology to empower rural resilient governance. Tang and Xie (2023) utilize static QCA methods to identify pathways such as quality-enhancing factors, comprehensive optimization, technology-driven enhancement, and intelligence-driven promotion that elevate rural economic resilience.
To sum up, although the role of digital economy is increasingly recognized, several research gaps persist: firstly, scholars often focus on influencing factors, with limited exploration of diverse pathways; secondly, traditional indicators are predominantly used, with fewer specific measures related to digitalization; thirdly, the emphasis tends to be on the net effects of single factors on agricultural economic resilience, overlooking the comprehensive effects of multiple interactive factors.
The agricultural economic system operates as a coupled system that generates value through multiparty interactions, characterized by complexity, nonlinearity, and dynamics. The infiltration of data elements intensifies the density of interrelations among participants within the system, demanding higher levels of dependency and independence among its components. Coherent activities in the value chain, complementary industrial models, and alternative core technologies constitute crucial resilient factors for the agricultural economic system when confronted with shocks or pressures. Enhancing the resilience of agricultural economics poses a complex governance challenge, marked by asymmetric causal relationships. Merely considering a simplistic symmetrical relationship between single factors and resilience is unscientific; thus, a comprehensive exploration from a configurational perspective is necessary to fully understand the synergistic effects among condition variables on agricultural economic resilience. Traditional empirical studies focus solely on causal relationships, with disputed generalizability of single-case studies, whereas qualitative comparative analysis (QCA) integrates qualitative and quantitative analyses, incorporating diverse dimensions of influencing factors into a research framework to uncover configurational effects. This approach emerges as a critical direction for future studies on agricultural economic resilience. Furthermore, agriculture faces unforeseen challenges, necessitating a dynamic and developmental approach to examine its resilience across different temporal and regional contexts.
Given the aforementioned realities and theoretical research gaps, this paper, based on the TOE framework and adopting a configurational perspective, applies dynamic QCA research methods to overcome the limitations of traditional QCA in analyzing panel data. Using panel data from 30 provincial-level regions in China from 2012 to 2022, the study explores the complex configurational relationships between effective combinations of technological factors, organizational factors, environmental factors, and agricultural economic resilience. By identifying the necessity and sufficiency of different condition variables in enhancing agricultural economic resilience and exploring the interactive coupling of technological, organizational, and environmental factors within the agricultural economic system, this research enriches the study of agricultural economic resilience. Moreover, it provides diverse and tailored pathways for enhancing agricultural economic resilience globally, depending on regional conditions.
“Resilience” refers to an object’s ability to return to its original state after being impacted by external forces, a concept later introduced into economics (Reggiani et al., 2002). Economic resilience refers to an economic system’s capability to recover to its original economic state and achieve sustainable development by relying on its own inherent resistance to external adverse factors (Yu et al., 2023). Agricultural economic resilience can be defined as the ability of agricultural systems to maintain their original characteristics and sustain critical functions when facing external disturbances such as natural disasters, policy adjustments, and market fluctuations (Jiang et al., 2022). Scholars both domestically and internationally have identified numerous factors influencing agricultural economic resilience, including technological advancements (Tang et al., 2022) and agricultural infrastructure levels (Zhang and Hui, 2022) at the technological level; at the organizational level, rural industry integration, human capital accumulation (Hao and Tan, 2023), government support intensity (Qun et al., 2024), and environmental regulatory intensity (Zhang and Hui, 2022); cultural characteristics (Tang et al., 2022), digital inclusive finance (Li and Yan, 2024), market scale (Reggiani et al., 2002), and aging rural populations (Jiang et al., 2024) at the environmental level. The measurement methods for agricultural economic resilience mainly employ an indicator system approach, evolving from early models such as the “Pressure-State-Response (PSR)” model (Jiang et al., 2022) to encompass capabilities including resistance recovery, adaptive adjustment, and transformative innovation (Zhao and Xu, 2023; Zhou et al., 2023), which have gained widespread recognition and application among scholars globally. This paper adopts this measurement approach, decomposing agricultural economic resilience into resistance recovery capability, adaptive adjustment capability, and transformative innovation capability. Among these, resistance recovery capability refers to the agricultural economic system’s ability to resist external shocks and quickly return to normal after being impacted; adaptive adjustment capability emphasizes the system’s ability to effectively manage new challenges and achieve sustainable stable operation by adjusting industrial structures or improving technologies in response to changing environments; transformative innovation capability is defined as the ability of the agricultural economic system to transition from traditional models to sustainable and efficient production methods through innovation and transformation in long-term development.
Tornatzky and Fleischer (1990) introduced the TOE theoretical framework, originally aimed at exploring factors influencing organizational adoption and implementation of new technologies, which has since been widely applied across multiple domains such as digital transformation, innovation outcomes commercialization, and digital rural development. This framework encompasses factors at three levels: technological factors involve an organization’s technological state, including technological infrastructure and characteristics; organizational factors emphasize internal organizational characteristics such as resource allocation and organizational scale; environmental factors refer to external surroundings such as economic conditions, degree of marketization, and cultural environment (Feng et al., 2024). Within the TOE framework, the synergies and interdependencies among technological, organizational, and environmental conditions significantly influence organizational decisions and ultimate outcomes.
Agricultural economic resilience is influenced by multiple factors, requiring a multidimensional and comprehensive theoretical framework. The TOE framework fundamentally serves as an analytical framework based on technological application contexts (Feng and Li, 2020). It holds strong explanatory power and adaptability in the field of agricultural economic resilience research, aligning with this study’s focus on data elements and technological innovation in analyzing agricultural economic resilience issues. Currently, several scholars have applied the TOE framework to research agricultural or rural resilience in China, yielding beneficial outcomes (Tang and Xie, 2023). Therefore, based on the TOE framework and tailored to the practical context of China’s agricultural economic resilience, this study constructs a model of influencing factors suited to the national conditions (Figure 1).
Innovations in digital technology encompass advancements in nascent information technology, data analytics, and intelligent agricultural techniques, whereas digital infrastructure pertains to the network of foundational structures that underpin the application of digital technologies and data transmission. The evolution of both digital technological innovations and digital infrastructure can catalyze the upgrading of agricultural production methodologies, augmenting efficiency and quality while enhancing the informatization level and risk resilience of agricultural systems. Consequently, these factors exert a positive influence on the economic resilience of agriculture. Hence, this paper analyzes “digital technological innovations” and “digital infrastructure” as technical conditions for bolstering the economic resilience of agriculture.
The innovation of digital technologies provides multifaceted support for enhancing agricultural economic resilience, serving as a crucial driver for advancing agricultural modernization and high-quality development. Specifically, digital technology innovation and application first improve the efficiency and quality of agricultural production, facilitating precision management of agricultural processes. This reduces environmental pollution, promotes green agricultural development, and enhances agricultural systems’ resilience to natural disasters, thereby bolstering agricultural economic recovery. Secondly, digital technology makes agricultural market information more transparent. Through digital tools such as ecommerce platforms and social media, farmers can access market information more accurately, adjust production and sales strategies promptly, and enhance their ability to withstand market risks. Consequently, this improves the competitiveness of agricultural products and enhances the resilience of agricultural economies (Zhang H. et al., 2024).
Digital infrastructure, as a crucial material foundation for achieving agricultural economic development, exerts significant impacts on enhancing agricultural economic resilience. On one hand, it directly enhances the resilience and recovery capabilities of agricultural economies. Improved digital infrastructure in agricultural production and management equipment effectively ensures the conduct of agricultural activities, enhances the capability to withstand natural disasters in modern agriculture, and strengthens agricultural economic resilience (Wang et al., 2023). On the other hand, it indirectly enhances agricultural economic resilience by promoting agricultural industry integration and improving rural human capital and agricultural technological levels. The construction of digital infrastructure contributes to narrowing the urban–rural digital divide (Huo and Ying, 2022), facilitating the application of advanced urban technologies and equipment in rural agricultural industries, accelerating the integration of agricultural industries, and providing diversified industrial support to enhance agricultural economic resilience. It enables farmers to quickly access advanced technologies, improves their knowledge structure, enhances overall rural awareness (Lin et al., 2023), and strengthens the endogenous dynamics of agricultural economic development. Furthermore, it enhances agricultural technological levels (Wang and Fang, 2023), accelerates the digital transformation of agricultural economies, and increases agricultural economic resilience.
Digitalization of the agricultural industry refers to the degree of digital transformation in agricultural production, management, and marketing, which renders agricultural production modes more efficient, precise, and sustainable. The intensity of policy support for agriculture pertains to the extent of governmental policy backing and resource allocation aimed at fostering agricultural development. It provides a stable policy environment and resource support, thereby reducing costs and risks for agricultural enterprises and enhancing the resilience of the agricultural economy. Consequently, this paper analyzes “digitalization of agricultural entrepreneurship” and “policies supporting for agriculture” as organizational conditions for bolstering the resilience of the agricultural economy.
The digitization of agricultural industry enhances agricultural economic resilience through improving the ability to withstand economic risks and promoting technological innovation (Guo and Zhang, 2023). The digitization of agricultural industry involves the sharing of data elements throughout the entire value chain, optimizing the allocation of various resource elements, thereby strengthening the ability to withstand economic risks in agriculture. By leveraging new digital technologies, resources can flow more efficiently between the agricultural industry chain and the innovation chain, facilitating precise matching of supply and demand in the innovation chain and achieving the integration of the agricultural industry chain and the innovation chain (Huang and Li, 2022). Additionally, the digitization of agricultural industry indirectly impacts agricultural economic resilience through green technological innovation. The application of green technological innovations can promote the formation of intensive and scaled management models in the agricultural industry, extending the agricultural value chain and enhancing agricultural economic resilience (Meng and Ren, 2023).
The role of policy support in enhancing regional economic resilience is widely acknowledged by scholars both domestically and internationally. Governments can bolster the resilience of the agricultural economy by strengthening agricultural infrastructure through fiscal support, promoting the integration of rural industries, enhancing agricultural disaster prevention and mitigation, improving rural governance, and perfecting the agricultural safeguard system. As some scholars have proposed, government intervention can positively affect the resilience of the agricultural industry chain (Guo and Zhang, 2023). In provinces with ample fiscal support, the introduction of high-tech agricultural machinery and the cultivation of high-quality crops have led to increased agricultural production efficiency, thereby enhancing the risk resistance of the region’s agricultural economy (Zhang and Hui, 2022). Strengthening the resilience of the agricultural economy is thus achieved.
Digital inclusive finance refers to the utilization of digital technologies to achieve the universalization and deepening of financial services, thereby reducing the costs of financial services and enabling a greater number of farmers and enterprises to access convenient and efficient financial services. The degree of marketization emphasizes the role of market mechanisms in resource allocation, reflecting the level of economic freedom and competition. It exerts a positive influence on agricultural economic resilience by improving the agricultural production environment, optimizing resource allocation, and enhancing market competitiveness. The level of rural cultural modernization indicates the extent of modern transformation in rural areas in terms of ideologies, lifestyles, education, and technology. It strengthens agricultural economic resilience by enhancing the cultural literacy and innovative capabilities of agricultural entities. In summary, digital inclusive finance, the degree of marketization, and the level of rural cultural modernization, through their unique mechanisms, collectively impact agricultural economic resilience, propelling agricultural economic development toward a higher and more sustainable level. Therefore, this paper analyzes “digital inclusive finance,” “level of marketization,” and “modernization of rural culture” as environmental conditions for enhancing agricultural economic resilience.
Digital inclusive finance, supported by digital technologies, can boost agricultural technology research and development, enhance consumption power, and inject new momentum into the resilience enhancement of agricultural economy from both the demand and research perspectives. It also has the potential to overcome traditional financial constraints of time and geography, thereby enhancing agricultural production and distribution capabilities from the supply side. Firstly, in terms of agricultural research and development, digital inclusive finance can leverage digital technologies to broaden the coverage of financial services, reduce financing barriers and costs for small and medium enterprises, and provide exogenous impetus for agricultural technology research and innovation, thus strengthening the transformative innovation capacity of agricultural economy (Kang and Li, 2022). Secondly, from the perspective of agricultural production, it can mitigate negative impacts of various shocks on production entities, guide financial resources toward green and smart agriculture, and effectively ensure sustainable agricultural development (Li and Liu, 2021). Additionally, in terms of agricultural product circulation, digital inclusive finance can utilize financial infrastructure to extend financial resources, alleviate financing difficulties and high costs for circulation enterprises, and optimize the circulation of agricultural products (Lei and Jin, 2021). Finally, in the consumption phase, digital inclusive finance can promote the upgrade of residents’ consumption structure, making green and high-quality agricultural products mainstream, thereby supporting the resilience building of agricultural economy from the demand side (Zhang and Wen, 2022).
Industrial resilience, as a composite element, encompasses various aspects such as market stability and resource conservation (Yu et al., 2023). Enhancing the resilience of agricultural economies necessitates a higher degree of marketization. This can be achieved through several means: firstly, promoting optimized resource allocation. Heightened levels of marketization facilitate the efficient allocation of agricultural resources across broader scopes, thereby improving resource utilization efficiency and enhancing agricultural adaptability to market fluctuations. Secondly, stimulating innovation and application of new technologies. Market-driven incentives encourage agricultural enterprises and farmers to adopt new technologies and methods, thereby enhancing agricultural productivity and product quality, thus bolstering the resilience of agricultural products against risks. Thirdly, strengthening the competitiveness of agricultural product markets. Marketization can foster the branding and standardized production of agricultural products, thereby increasing their market competitiveness and fortifying agricultural economies against the impacts of market fluctuations.
The construction of rural culture, as an essential dimension of resilient rural development, plays a pivotal role in enhancing agricultural economic resilience. A modernized rural culture provides the spiritual impetus necessary for rural revitalization (Shang and Xie, 2024). A higher level of rural cultural modernization can significantly strengthen agricultural economic resilience, as evidenced by several key factors: firstly, the modernization of rural culture facilitates the transformation of farmers’ value systems, increasing their acceptance of new technologies and modern management practices, thereby enhancing the adaptability and innovative capacity of the agricultural economy. Secondly, through education and training, the technological and cultural competencies of farmers can be improved, equipping them with the skills necessary to master modern agricultural production techniques, which in turn bolsters agricultural productivity and competitiveness. Thirdly, the establishment of a governance system that integrates self-governance, rule of law, and moral governance enhances the stability of rural societies, thereby providing a conducive social environment for the stable development of agricultural economies. Lastly, a modernized rural culture encourages the development of diverse industries related to agriculture, facilitating the diversification of agricultural economies, reducing reliance on single agricultural product markets, and ultimately enhancing agricultural economic resilience.
The QCA method has been applied across various management fields such as digital economy to investigate systematic and complex managerial issues (YoungKi and Sunil, 2020). Compared with traditional empirical methods, QCA method has the following advantages: First, it has the advantages of both qualitative analysis and quantitative analysis, and is suitable for large, medium and small samples (Fang et al., 2024); Second, by focusing on the logical relation of the set rather than the simple correlation relation, the consistency assumption of causal effect is broken, the equivalence between different configurations and the substitution between anthems can be identified, and the endogeneity problem can be effectively dealt with Furnari et al. (2020). Third, different driving mechanisms leading to the occurrence and non-occurrence of results can be identified, which broadens the explanatory dimension of research problems (Ragin, 2008). This paper aims to comprehensively discuss the concrete path of enhancing the resilience of agricultural economy formed by the mutual cooperation between various elements under the TOE framework, so the QCA method is selected to carry out research.
However, constrained by theoretical framework and tools, traditional QCA methods ignore the “longitudinal time effect,” that is, the influence of condition variables on outcome variables may change over time. It also ignores the “horizontal case effect,” that is, the influence of condition variables on outcome variables may differ between different cases (Quan and Chen, 2024). To overcome these shortcomings, Roberto and Miguel (2016) proposed a dynamic QCA method, which completely processed panel data from three aspects: pooled, between and within, enabling researchers to observe the changes of a certain configuration in terms of time and individual (cluster) dimensions. In addition, using consistency adjustment distance to describe the degree of consistency change in time dimension and individual dimension is helpful to reflect the characteristics of causality change over time more accurately. Data elements enabling agricultural economic resilience is a continuous process occurring on the time axis. Research on the configuration based on cross-section data cannot fully demonstrate the interaction of causality in time dimension. Therefore, dynamic QCA method is adopted in this paper. RStudio software was used to break through the barrier between panel data and QCA, and the dynamic influence process of data elements on agricultural economic resilience was explained from the perspective of spatiotemporal combination.
Given China’s vast territory and uneven regional development, there are significant differences among provinces in terms of natural resources and economic conditions (see Table 1). Analyzing and comparing the factors influencing the agricultural economic resilience of different regions more accurately requires provincial-level data, which in turn provides a basis for formulating regional policies. Considering the availability, authority, and timeliness of data, balanced panel data from 30 provinces (autonomous regions, municipalities directly under the central government) from 2012 to 2022 (excluding Tibet, Hong Kong, Macao, and Taiwan) have been collected from multiple sources. The specific measurement methods and data sources for each variable are detailed below.
The outcome variable is agricultural economic resilience (hereinafter referred to as “Y”), for which a measurement framework is constructed based on existing literature (Zhao and Xu, 2023; Zhou et al., 2023; Tang and Xie, 2023). Entropy method is employed for measurement, and missing data are supplemented using moving average interpolation. Data are sourced from various publications including “China Statistical Yearbook,” “China Rural Statistical Yearbook,” “China Finance Yearbook,” “China Environmental Statistical Yearbook,” “China Social Statistical Yearbook,” “China Energy Statistical Yearbook,” “China Business Management Statistical Yearbook,” “China Rural Cooperative Economy Statistical Yearbook,” “China Rural Policy and Reform Statistical Yearbook,” National Bureau of Statistics website, Peking University Digital Finance Research Center, and provincial statistical yearbooks. Specific measurement indicators are detailed in Table 2.
This article, grounded in the TOE framework, introduces seven conditioning variables. Specific measurement methods, data sources, and references are detailed in Table 3. Notably, the metrics for digital infrastructure, agricultural digitalization, and rural cultural modernization employ the entropy method, supplemented by moving average interpolation to address missing data.
Drawing on existing research, combined with the specific conditions and data of the case itself, we directly calibrate antecedent conditions and resultant variables. The quantiles for anchoring the resultant and antecedent variables are set at 95, 50, and 5%, and 75, 50, and 25%, respectively, representing full membership, crossover points, and complete non-membership (Fiss, 2011). To avoid loss of truth table entries due to identical values with the anchoring points, a preprocessing step adds 0.001 to relevant variable values. The calibration results are presented in Table 4.
In qualitative comparative analysis based on cross-sectional data, a consistency score greater than 0.9 and coverage greater than 0.5 serve as universal criteria for assessing the existence of necessary conditions, a standard also applicable in dynamic QCA analysis. However, dynamic qualitative comparative analysis introduces the concept of adjusted distance when examining necessary conditions, distinguishing between between-group and within-group consistency adjusted distances. A consistency adjusted distance exceeding 0.2 for a particular antecedent condition suggests potential temporal or regional effects on its necessity, necessitating further investigation into its necessity across each year or case (Roberto and Miguel, 2016). Table 5 presents the results of necessity analysis for high agricultural economic resilience versus non-high agricultural economic resilience. While the overall consistency scores for the seven antecedent conditions are all below 0.9, some exhibit between-group and within-group consistency adjusted distances exceeding 0.2, indicating the need for further analysis of potential temporal and regional effects. Overall, a greater within-group consistency adjusted distance compared to between-group consistency adjusted distance suggests that the necessary relationships between antecedent conditions and outcomes are more influenced by regional effects.
Analysis was conducted on causal relationships where the between-group adjusted distance exceeded 0.2. The findings are presented in Table 6, illustrating the necessity levels of various factors for enhancing agricultural economic resilience over time, as depicted in Figure 2. From the analysis, several insights emerged: firstly, among the 12 scenarios in Table 6, none of the antecedent conditions constituted necessary requirements for agricultural economic resilience across the overall temporal dimension. Secondly, while high digital inclusive finance exhibited consistency above 0.9 during 2018–2022, it did not prove to be a necessary condition as indicated by scatter plot diagnostics (see Figure 3) (Wen and Jiang, 2023; Schneider and Wagemann, 2012). Thirdly, examination of the temporal evolution of between-group antecedent condition consistency (Figure 2) revealed a general increasing trend in the necessity levels of these factors for enhancing agricultural economic resilience, with the exception of policy intensity in agricultural support remaining relatively stable. This suggests that the importance of each factor in enhancing resilience gradually intensified over the study period (2012–2022), aligning with the Chinese context and emphasizing the need for agricultural economic resilience to adapt to marketization and high-quality agricultural development requirements. Fourthly, high digital inclusive finance, digitalization of agricultural industries, robust digital infrastructure, and high levels of marketization are gradually emerging as necessary conditions for agricultural economic resilience, underscoring their role as decisive factors in this regard. This validates the strategic shift from a predominantly supportive government policy model to one emphasizing the stimulation of endogenous agricultural dynamics. Ying and Cui (2022) also pointed out that the logic of blood transfusion can inhibit the generation of hematopoietic mechanisms, and government departments should establish an endogenous development mechanism with subject autonomy and long-term sustainability. Fifthly, the necessity of digital inclusive finance for agricultural economic resilience has shown the most pronounced increase, from nearly zero in 2012 to 1 in 2021, Li and Yan (2024) also proposed that digital inclusive finance has a promoting effect on the resilience of agricultural economy, which is highly consistent with the conclusion of this article. In contrast to previous studies, this paper reveals the evolving trends of these factors over time, providing a theoretical basis for enhancing agricultural economic resilience.
The intra-group consistency adjustment distances are generally greater than 0.2, indicating significant regional effects among the relationships between seven conditions and outcomes, which aligns with the substantial variations in natural environments and agricultural development levels across different regions of China. This is consistent with the conclusion drawn by Shi (2024) that there are significant regional differences in the resilience level of China’s agricultural economy. Building upon Garcia-Castro & Ari’s recommendation to further explore regional characteristics influencing necessary causal relationships (Roberto and Miguel, 2016), this study proceeds to analyze intra-group consistency coefficients across 30 provinces and municipalities. The analysis reveals notable regional disparities in the necessity of seven antecedent conditions: digital technology innovation, digital infrastructure, marketization level, and cultural modernization are essential conditions for robust agricultural resilience in Beijing, Fujian, Guangdong, and other provinces and municipalities with advanced agricultural economies in the eastern region. Agricultural industry digitalization emerges as a necessary condition for robust agricultural resilience in Hebei, Heilongjiang, Henan, Hubei, and other provinces, primarily affecting provinces and municipalities in the eastern and central regions. Policy support intensity for agriculture is identified as a necessary condition for robust agricultural resilience in provinces like Hainan, Gansu, Guangxi, and Ningxia, primarily influencing provinces in the western region. Inclusive digital finance is a necessary condition for robust agricultural resilience in provinces and municipalities such as Chongqing, Qinghai, and Hainan, affecting provinces in both the eastern and western regions, as detailed in Table 7. In summary, the causal necessity relationships between data elements and agricultural economic resilience are influenced by regional characteristics. Enhancing agricultural economic resilience should therefore be tailored to local conditions, emphasizing a scientifically informed deployment strategy.
Configuration analysis is a distinctive feature and core strength of QCA, capable of revealing multi-causal patterns leading to observed outcomes. The analysis involves setting three key parameters: consistency, PRI consistency, and frequency threshold. Referencing literature on consistency thresholds and based on a sample of 30 provincial cases (YoungKi and Sunil, 2020), this study sets the consistency threshold at 0.8, PRI threshold at 0.75, and frequency threshold at 3. Due to antecedent conditions not constituting necessary conditions across all temporal dimensions, they are not treated in the standardization analysis. Subsequently, identifying core and auxiliary conditions based on the nested relationships between intermediate and parsimonious solutions. The configuration analysis yields five configurations of high agricultural economic resilience, as presented in Table 8, with consistency coefficients all exceeding 0.9. The overall consistency coefficient is 0.918, and the overall coverage is 0.625, both surpassing acceptable minimum standards. Given that configurations H1a, H1b, and H1c share essentially the same antecedent conditions, they are considered second-order equivalent configurations (Li et al., 2024). In summary, three resilience-enhancing patterns for agricultural economies are identified: the market-driven industrial technological innovation ecosystem optimization model (M1), the TOE-enabled agricultural digital development model (M2), and the government-led cultural promotion of agricultural digital transformation model(M3). Finally, following the general steps of panel data QCA analysis, the aggregated results are first analyzed, followed by examining between-group and within-group results with adjustments for consistency distances (Table 7).
① Market-driven industrial technological innovation ecosystem optimization model.
The model corresponds to configurations H1a, H1b, and H1c. Configurations H1a and H1b suggest that high digital technology innovation and high digitalization in agricultural industry as core conditions, supplemented by advanced digital infrastructure and high marketization levels, can lead to enhanced agricultural economic resilience. Configuration H1c indicates that high digital technology innovation as core conditions, supported by high marketization levels and high digitalization in agricultural industry, can also contribute to agricultural economic resilience. This model covers 88% of cases with the highest applicability. The underlying mechanisms are as follows: an open and competitive market environment facilitates efficient allocation of agricultural resources and promotes deep integration of production and research, thereby enhancing adaptability and flexibility of the agricultural industry. Coupled with digital technology and digital management, the agricultural sector is better equipped for risk assessment and early warning, enabling swift responses to market fluctuations and natural risks, thereby reducing losses and bolstering resilience. Tang and Xie (2023) proposed that under the conditions of high digital technology, professional service level, and hardware facilities, the shortcomings of insufficient local market size can be overcome. The research results of this article point out that the level of marketization as an auxiliary condition has a positive effect on improving the resilience of agricultural economy.
The provinces corresponding to M1 are Shandong (2012–2022), Jiangsu (2012–2022), Guangdong (2012–2022), Zhejiang (2013–2022), Anhui (2014–2022), Hubei (2014–2022), Shanghai (2016–2022), Fujian (2017–2022), Beijing (2017–2022), and Sichuan (2017–2022). Among these, Guangdong Province has demonstrated significant progress in enhancing the resilience of its agricultural economy by constructing a robust ecosystem for industrial technological innovation under market guidance. Firstly, it has promoted deep integration of science and technology with agriculture. By 2023, the province achieved a contribution rate of agricultural scientific and technological progress exceeding 75%, with coverage rates of superior varieties for major crops such as rice exceeding 98%, and an implementation rate of key technologies reaching 98.5%. These achievements have positioned Guangdong at the forefront nationally across various indicators. Secondly, industrialization of agriculture has been actively pursued, with over 5,000 leading agricultural enterprises at all levels, including 1,183 at the provincial level and above. These enterprises have facilitated agricultural industrialization and market-oriented processes through methods such as listing and financing, injecting new vitality into Guangdong’s agricultural economy and strengthening its resilience.
② TOE empowers agricultural digital development model.
The pattern corresponding to configuration 2 emphasizes that high-level digital technological innovation and the digitization of the agricultural industry are core conditions, supported by high-level digital infrastructure, strong agricultural policy intensity, widespread digital financial inclusion, and modernization of rural culture, all contributing to enhanced agricultural economic resilience. The mechanisms at play are as follows: innovative digital technologies provide precision and automated solutions for agricultural production, thereby increasing efficiency, enhancing resilience against pests, diseases, and climate change, thereby improving the stability and risk mitigation capabilities of agricultural systems. Agricultural organizations adopting digital technologies optimize internal management processes, im-proving decision-making efficiency and responsiveness to market changes. Additionally, internal technical training and knowledge sharing within these organizations enhance agricultural practitioners’ mastery and application of new technologies, providing human capital support for the sustainable development and innovation of agricultural economies. The application of digital technologies also enhances the external environment of the agricultural industry, including market information transparency, supply chain efficiency, and traceability of agricultural products. Together with high levels of digital financial inclusion and modernization of rural culture, these factors constitute crucial environmental elements that enhance agricultural economic resilience. Guo et al. (2024) proposed that agricultural digitalization is the driving force to enhance the resilience of agricultural economy, while Nie et al. (2024) pointed out that new infrastructure can significantly improve the resilience of agricultural economy, while model 2 of this paper indicates that infrastructure needs to cooperate with organizational and environmental conditions to better enable the digital development of agriculture and enhance the resilience of agricultural economy.
The case provinces corresponding to M2 include Hunan (2018–2021), Shaanxi (2019–2021), and Hebei (2018–2019). Among these, Hunan province has demonstrated notable advancements in agricultural digitization empowered by the TOE. This initiative actively promotes agricultural science and technology innovation, exemplified by the establishment of the nation’s first unmanned dual-season rice farm in Wangcheng District. Utilizing unmanned combined rotary tillers and other smart agricultural machinery has significantly enhanced agricultural production efficiency and yield while reducing production costs. Furthermore, Hunan has integrated and optimized the agricultural industry chain through the construction of a modern agricultural industry system based on concepts such as cross-county clusters, one park per county, one industry per town, and one product per village. This integration has strengthened the organizational structure and market competitiveness of the agricultural industry. Hunan province’s implementation plan, titled “Financial Support for Comprehensive Rural Revitalization and Accelerating the Construction of an Agricultural Powerhouse,” outlines specific measures. These include accelerating the issuance of grain and oil credit, optimizing financial services for “basket of vegetables” products, and ensuring grain security and stable production of key agricultural products. Presently, the province has established 2,209 town-level comprehensive cultural stations and 35,109 village-level comprehensive cultural service centers, forming a pattern of “one station per township, one cultural room per village.” This layout provides robust cultural support for enhancing agricultural economic resilience.
③ Cultural-driven agricultural digital transformation model guided by government.
This model corresponds to configuration 3. This configuration indicates that high levels of digital technology innovation and advanced rural cultural modernization serve as core conditions, while strong policy support for agriculture acts as an auxiliary condition, collectively fostering high agricultural economic resilience. The underlying mechanism operates as follows: through governmental policy support and cultural guidance, the innovation vitality and intrinsic motivation within the agricultural sector are stimulated. Specifically, the government establishes supportive policies, provides financial subsidies, and optimizes the regulatory environment to create essential external conditions and incentive mechanisms for the digital transformation of agriculture. Concurrently, cultural factors function as a form of soft power, enhancing farmers’ digital literacy, strengthening the dissemination and application of agricultural technology, and cultivating an innovative culture conducive to digital transformation, thereby increasing agricultural practitioners’ acceptance and application capabilities for new technologies. Within this model, the interplay between governmental policy guidance and cultural promotion synergistically advances the modernization of agricultural production methods, improves agricultural productivity and the added value of agricultural products, and enhances the overall competitiveness and economic resilience of the agricultural sector, ultimately achieving sustainable agricultural development. Deng (2024) pointed out that the modernization of rural culture can promote the high-quality development of rural economy. Zhang and Li (2024) believe that enriching rural cultural life and improving the allocation of rural educational resources can promote agricultural modernization. This paper jointly verifies the results from different perspectives.
The corresponding cases to M3 are Heilongjiang Province (2013–2022) and Guangxi Province (2017). In Heilongjiang, the government encourages agribusiness enterprises to intensify their research and development (R&D) investments. Enterprises investing over 1 million RMB in R&D are granted subsidies of varying proportions to enhance their capacity to absorb technological achievements. Furthermore, substantial efforts have been made to implement cultural programs benefiting the populace, continually advancing standardized and equitable public cultural services throughout the province. This initiative aims to establish a modern public cultural and tourism service system characterized by government leadership, social participation, comprehensive coverage, and shared development and benefits. Over the past decade, Heilongjiang has established 1,752 digital cultural service points and digital cultural stations, alongside 8,233 rural comprehensive cultural service centers. All public cultural service facilities are freely accessible to the public, fostering a robust cultural foundation to cultivate high-quality farmers and thereby providing significant endogenous impetus for enhancing agricultural economic resilience.
Inter-group consistency measures whether each configurational condition group during each year of the sample period is a sufficient condition for the outcome, reflecting the level of cross-sectional consistency within each year of the panel data and addressing the time-blindness issue in traditional QCA configurations. As shown in Figure 4, the inter-group consistencies of the generated five configurations all exceed 0.90, surpassing the consistency criterion of 0.75. Additionally, the inter-group adjusted distances are all less than 0.2, indicating no significant temporal effects in the aforementioned configurations.
Further investigation into each configuration reveals the following findings: Firstly, the explanatory power of configuration H1a has shown a declining trend annually since 2016, particularly experiencing a substantial decline in 2021–2022. This decline may be attributed to sudden market or natural factors significantly impacting agricultural economic resilience, which cannot be adequately addressed solely through internal adjustments within the agricultural industry. Moreover, this configuration lacks the condition of governmental support for agriculture, thereby diminishing its explanatory power. This implies that during periods of significant agricultural challenges, governmental support serves as a necessary safeguard, akin to a health factor. Secondly, configuration 2 exhibited its lowest consistency levels during 2019–2020, possibly due to the sudden impact of a pandemic. During this time, policy and research resources were directed toward pandemic control, resulting in a halt in technological innovation, stagnation in financial markets, and obstacles in rural cultural modernization. Thirdly, the consistency level of configuration H1c has remained stable around 1, indicating that the integration of digital technology innovation guided by the market with agricultural digitalization can partially compensate for the deficiencies in policy and financial resources, as well as the lower levels of rural cultural modernization. Fourthly, except for configuration H1c, the consistencies of all configurations collectively decreased in 2018, likely due to the impact of Sino-US trade frictions, which reduced China’s agricultural exports and farmers’ income. This study underscores the importance of examining configurational changes over time to under-stand the evolving dynamics of agricultural systems under various external shocks and policy conditions.
The intra-group consistency was analyzed at the provincial level to assess whether each province’s configuration across conditions during the sample period serves as a sufficient condition for outcomes. The majority of provinces exhibited strong consistency among the five configurations. Overall findings indicate that from 2012 to 2022, provinces did not adhere uniformly to consistent condition configurations; specific provinces may be suited to more than one pathway for enhancing agricultural economic resilience. For instance, Fujian exhibited low consistency below 0.75 in configuration H1a, and Shaanxi showed consistency below 0.75 in configuration 2, whereas both Fujian and Shaanxi demonstrated consistency of 1 and above 0.8, respectively, in other configurations. Therefore, further in-depth analysis is war-ranted to elucidate suitable pathways for different regions across various years.
Based on the average regional coverage values in Table 9, the market-driven industrial technological innovation ecosystem optimization model appears more suitable for the central and eastern regions. This preference may stem from the comparatively more developed market mechanisms in these areas than in the western region. The digital innovation level and agricultural digital industry maturity are higher, facilitating research-industry integration and enhancing agricultural economic resilience. The eastern region is primarily represented by Shandong, Zhejiang, and Guangdong, while the central region typifies Henan, Anhui, and Hubei. Conversely, the TOE empowered agricultural digital development model seems more appropriate for the central and western regions. This suitability could be attributed to the less robust agricultural economic development in these regions compared to the more developed eastern regions. Multiple empowering factors—technological, organizational, and environmental—are required to promote agricultural industry digitalization, thereby enhancing agricultural economic resilience. Typical representative provinces include Hunan and Shaanxi. The government-guided cultural transformation model promoting agricultural digitalization exhibits a relatively even distribution across suitable cases, with a larger proportion in the central and western regions. This distribution may reflect a greater reliance in these areas on government policy resources to enhance agricultural economic resilience, with Guangxi being a typical example.
This paper further examines the configurations that produce non-high agricultural economic resilience, and divides the six configurations into three categories. One is the infrastructure deficiency and inadequate digital technology innovation (including con-figurations N1a and N1b), which takes digital technology innovation, digital infra-structure and digitalization of agricultural industry as the missing core conditions. The biggest feature of this mode is the lack of hardware required for agricultural economic development. To some extent, it has affected the level of technological innovation and the digital transformation of the agricultural industry, resulting in lower agricultural economic resilience; The second type is the lack of innovation vitality mode (including configuration N2a and N2b), with digital technology innovation and agricultural industry digitalization as the missing core conditions, characterized by the low level of technological innovation, resulting in the lack of innovation and transformation ability of agricultural economy, resulting in low agricultural economic resilience; The third type is the infrastructure deficiency combined with environmental barrenness (including configuration N3). This configuration takes digital infrastructure and digitalization of agricultural industry as the core missing conditions, and digital financial inclusion, marketization level and rural cultural modernization as the marginal missing conditions. It is characterized by backward infrastructure, poor financial, market and rural cultural environment, which is not conducive to improving the resilience of agricultural economy.
This study conducted robustness tests by altering the PRI consistency, case threshold, and original consistency threshold as follows: First, with reference to Zeng et al. (2024), the original consistency threshold was increased from 0.8 to 0.85, while other values remained unchanged; configuration results, overall consistency, and overall coverage remained fully consistent with previous findings. Second, refer to the practice of Zhang J. et al. (2024), the case threshold was raised from 3 to 4, with other values unchanged; configuration results differed only slightly from the original configuration and exhibited clear subset relationships; overall consistency and coverage were largely consistent (Table 10), This suggests that the results are robust (Schneider and Wagemann, 2012). Third, refer to the practice of Zhang and Jia (2024), PRI consistency was increased from 0.75 to 0.8, while other values remained unchanged; configuration results, overall consistency, and coverage were entirely consistent with previous results.
This study utilizes panel data from 30 provincial-level regions in China spanning from 2012 to 2022, introducing the dynamic QCA method into the field of agricultural economic resilience. It explores diverse pathways through which factors under the TOE framework contribute to the formation of agricultural economic resilience. The main findings are as follows: firstly, the necessity analysis reveals that technological, organizational, and environmental factors individually do not constitute necessary conditions for achieving high agricultural economic resilience. Secondly, the increasing importance over time of digital inclusive finance and agricultural industry digitization emerges as pivotal elements for achieving high agricultural economic resilience. Thirdly, the study summarizes three high resilience models: the market-driven industrial technological innovation ecosystem optimization model, the TOE-enabled agricultural digitization development model, and the government-led cultural transformation for agricultural digitization model. Additionally, it identifies three low resilience models: infrastructure deficiency and inadequate digital technology innovation, lack of innovation vitality, and infrastructure deficiency combined with environmental barrenness. Fourthly, the pathways to achieving high agricultural economic resilience exhibit diversity across provinces, with significant regional disparities in configuration coverage within each group. Specifically, the market-driven industrial technology innovation ecosystem model predominates in the central and eastern regions, while the TOE-enabled agricultural digitization development model is more prevalent in the central and western regions. Cases explained by cultural-driven agricultural digital transformation model guided by government pathway are distributed relatively evenly, primarily concentrated in the central and western regions.
Identification of key conditions and novel factors driving agricultural economic resilience. Our study identifies critical conditions for enhancing the resilience of agricultural economies and novel factors that drive resilience in agricultural economic development. With the widespread adoption of data-driven approaches, digital inclusive finance, agricultural industry digitization, digital infrastructure, and marketization are emerging as new decisive factors for enhancing agricultural economic resilience, surpassing traditional policy support mechanisms. This shift implies that innovation, human capital, and data are becoming pivotal elements in driving the resilience of agricultural economies.
Enrichment of theoretical research on agricultural economic resilience ecosystems. We investigate three ecological models that lead to high agricultural economic resilience, providing a nuanced perspective from the standpoint of data elements on mechanisms that enhance agricultural economic resilience. Specifically, the “market-driven industrial technological innovation ecosystem optimization model,” the “TOE-empowered agricultural digitization development model,” and the “culturally-driven agricultural digitization transformation model under governmental guidance” contribute to enhancing agricultural economic resilience. Conversely, “inadequate infrastructure - insufficient digital technology innovation,” “lack of innovation vitality,” and “lagging infrastructure - impoverished environment” lead to low levels of agricultural economic resilience. The introduction of these models holds significant reference value for developing countries aiming to enhance their agricultural economic resilience.
Emphasis on spatiotemporal and dynamic analysis in agricultural economic resilience research. This study underscores the necessity and feasibility of spatiotemporal and dynamic analysis in research on agricultural economic resilience. Traditional QCA studies with static configurational concepts may lead to theoretical stagnation and unsaturation, potentially causing sample time selection biases and non-robust configurational findings (Meng and Wei, 2023). This research pioneers the use of dynamic QCA methods to study issues of agricultural economic resilience, exploring configurational effects across time and space. It captures configurational structures that remain stable over time and space, thereby enhancing theoretical saturation and conclusion robustness.
First of all, the government should strengthen the cooperation between industry, university and research, promote the industrialization of agricultural scientific and technological achievements, build a collaborative innovation system based on market demand, and accelerate the upgrading of the technical level of the agricultural industry chain. Secondly, agricultural enterprises should accurately connect with market demand and optimize the direction of technological innovation, identify the potential demand and trend of agricultural market by using market segmentation and demand forecasting models, and ensure the effective allocation and market adaptability of technological innovation resources. Finally, agricultural enterprises should also promote the construction of agricultural brands, enhance the competitiveness of the industrial chain and the ability to resist risks.
First, the government should establish a more forward-looking, extensive coverage and stable support policy system by strengthening the top-level design of agricultural science and technology policy. It is also necessary to strengthen the construction of agricultural science and technology personnel, improve the quality of farmers and rural practical personnel, and promote science and technology to promote agricultural development. Second, governments should optimize the structure of agricultural organizations to improve management and operational efficiency, and promote knowledge sharing and technology diffusion. Finally, a good environment is essential to enhance the resilience of the agricultural economy, and the government should improve the market environment by making fair competition laws and regulations and strengthening market supervision. At the same time, provide agricultural credit support, develop agricultural insurance, optimize the financial environment; we will strengthen rural education, promote the modernization of rural culture, and enhance the resilience and sustainable development of the agricultural economy.
First, the government should strengthen the publicity, education and training of agricultural digital transformation to enhance farmers’ awareness and acceptance of digital technology. It is also necessary to optimize the layout of infrastructure according to local conditions based on the economic development level and resource endowment of different regions, so as to provide a strong guarantee for the digital transformation of agriculture. Secondly, public welfare organizations can participate in the promotion and application of agricultural digital technologies by mobilizing the whole society; third, the community can build a communication platform for agricultural digital transformation, organize on-site observation and demonstration activities of agricultural digital technology, so that agricultural enterprises and farmers can intuitively feel the advantages of digital technology.
The original contributions presented in the study are included in the article/supplementary material, further inquiries can be directed to the corresponding author/s.
WL: Conceptualization, Funding acquisition, Supervision, Validation, Visualization, Writing – review & editing. SZ: Conceptualization, Data curation, Formal analysis, Investigation, Methodology, Project administration, Resources, Software, Validation, Writing – original draft. YS: Investigation, Validation, Writing – review & editing. ST: Resources, Software, Writing – review & editing.
The author(s) declare that financial support was received for the research, authorship, and/or publication of this article. This work was carried out with support from National Natural Science Foundation of China and Guangxi Natural Science Foundation (nos. 72064008 and 2020GXNSFAA159166).
The authors declare that the research was conducted in the absence of any commercial or financial relationships that could be construed as a potential conflict of interest.
The authors declare that no Gen AI was used in the creation of this manuscript.
All claims expressed in this article are solely those of the authors and do not necessarily represent those of their affiliated organizations, or those of the publisher, the editors and the reviewers. Any product that may be evaluated in this article, or claim that may be made by its manufacturer, is not guaranteed or endorsed by the publisher.
Bai, Q. (2024). Digital technologies empowering rural resilient governance and its framework for action. J. Fujian Normal Univ. 3, 35–43+169.
Chao, R., and Li, J. (2024). The impact of agricultural production agglomeration on agricultural economic resilience: based on spatial spillover and threshold effect test. Front. Sustain. Food Syst. 8:8. doi: 10.3389/fsufs.2024.1464732
Chen, X., and Xu, W. (2024). Data elements, transportation infrastructure and industrial structure upgrading: based on quantitative spatial general equilibrium model analysis. Manage. World 40, 78–98. doi: 10.19744/j.cnki.11-1235/f.2024.0046
Deng, N. (2024). The role of digital economy in promoting high-quality rural development and its practical path. Agric. Econ. 4, 33–36.
Fang, F., Zhang, L., and Zhao, J. (2024). Analysis of multiple paths to improve agricultural green total factor productivity from the perspective of institutional configuration: panel data analysis based on dynamic QCA. Chin. Rural Econ. 2, 44–66.
Feng, Z., and Li, H. (2020). The influencing factors and practical path of poverty alleviation in poor counties: qualitative comparative analysis based on fuzzy sets of 60 cases in Southwest China. J. Yunnan Univ. Finance Econ. 36, 46–56. doi: 10.16537/j.cnki.jynufe.000643
Feng, L., Xu, M., Feng, Y., and Zhang, K. (2024). Research on the driving paths of business model innovation for incumbent enterprises in the digital context- configuration analysis based on the TOE framework. Soft Sci., 1–14.
Fiss, C. (2011). Building better causal theories: a fuzzy set approach to typologies in organization research. Acad. Manag. J. 54, 393–420. doi: 10.5465/amj.2011.60263120
Furnari, S., Crilly, D., Misangyi, F., Greckhamer, T., and Fiss, P. (2020). Capturing causal complexity: heuristics for configurational theorizing. Acad. Manag. Rev. 46, 778–799. doi: 10.5465/amr.2019.0298
Guan, X., and Qin, X. (2021). The dual attributes of data elements and their interactive effects. Teach. Res. 8, 68–76.
Guo, N., Lv, T., and Zong, H. (2024). The impact of agricultural digitization on agricultural economic resilience. Chin. J. Eco-Agric., 1–12.
Guo, F., Wang, J., Wang, F., and Zhang, X. (2020). Measuring the development of digital financial inclusion in China: index compilation and spatial characteristics. China Econ. Q. 19, 1401–1418. doi: 10.13821/j.cnki.ceq.2020.03.12
Guo, Y., and Zhang, C. (2023). Industrial digitalization, green technology innovation and agricultural industry chain resilience. J. Tech. Econ. Manage. 10, 117–122.
Hao, A., and Tan, J. (2022). The impact of digital village construction on the resilience of China's food system. J. S. China Agric. Univ. 21, 10–24.
Hao, A., and Tan, J. (2023). The mechanism and effect measurement of rural industry convergence enabling agricultural toughness. J. Agrotech. Econ. 07, 88–107. doi: 10.13246/j.cnki.jae.20220418.002
Hertel, T., Elouafi, I., and Ewert, F. (2021). Tanticharoen, building resilience to vulnerabilities, shocks and stresses: a paper on action track 5. Bonn: Center for Development Research (ZEF) in cooperation with the scientific group for the UN food system summit 2021.
Huang, Y., and Li, J. (2022). Construction of agricultural industry innovation chain: essence, support and model. Econ. Rev. J. 10, 89–95. doi: 10.16528/j.cnki.22-1054/f.202210089
Huo, P., and Ying, H. (2022). The theoretical basis, action logic and practical path of the urban-rural digital divide: an analysis based on the "network poverty alleviation action plan". J. China Agric. Univ. 39, 183–196. doi: 10.13240/j.cnki.caujsse.2022.05.007
Jiang, J., Wu, H., Li, S., and Liu, Q. (2024). The impact of rural population aging on the resilience of agricultural economy: an analysis based on the perspective of production factors. East China Econ. Manag. 38, 94–105. doi: 10.19629/j.cnki.34-1014/f.240406003
Jiang, H., Zhang, C., and Jiang, H. (2022). Study on the effect and mechanism of agricultural economic resilience on high-quality agricultural development in China. Agric. Econ. Manag. 1, 20–32.
Kang, W., and Li, Z. (2022). Digital financial inclusion and technological innovation: a new perspective from the enterprise life cycle. Macroeconomics 12, 21–42. doi: 10.16304/j.cnki.11-3952/f.2022.12.005
Lei, H., and Jin, M. (2021). Bank digital inclusive finance, bank competition and corporate financing constraints. Theory Pract. Finance Econ. 42, 2–9. doi: 10.16339/j.cnki.hdxbcjb.2021.06.001
Li, X., and Liu, Y. (2021). How can digital financial inclusion promote rural entrepreneurship? Bus. Manag. J. 43, 24–40. doi: 10.19616/j.cnki.bmj.2021.12.002
Li, J., Wang, C., and Yuan, Z. (2024). How can green finance improve food security? Based on the perspective of rural human capital and agricultural industry agglomeration. J. Univ. Jinan 34, 52–68.
Li, Z., Xiang, Y., and Liao, Y. (2024). Spatio-temporal evolution and spatial characteristics of high-quality development of agricultural economy in China from multi-scale perspective. J. China Agric. Resour. Reg. Plan. 1, 1–19. doi: 10.20004/j.cnki.ujn.2024.01.007
Li, Y., and Yan, F. (2024). Spatial spillover and threshold effect of digital financial inclusion on agricultural economic resilience: a case study of prefecture-level cities in the middle and lower reaches of the Yangtze River. J. China Agric. Univ. 29, 282–295.
Lin, H., Zhao, L., and Hu, Y. (2023). Whether digital village construction can promote common prosperity of old revolutionary base areas. Chin. Rural Econ. 05, 81–102. doi: 10.20077/j.cnki.11-1262/f.2023.05.005
Liu, C. (2023). Theoretical thinking on smart agriculture: development modes, potential problems, and promotion measures. Econ. Rev. J. 8, 63–70. doi: 10.16528/j.cnki.22-1054/f.202308063
Liu, Z. (2023). Study on the development level, regional differences and spatio-temporal evolution characteristics of digital agriculture. Stat. Decis. 39, 94–99.
Liu, H. (2024). Data element allocation, agricultural new quality productivity and agricultural high quality development. Stat. Decis. 40, 11–16. doi: 10.13546/j.cnki.tjyjc.2024.21.002
Liu, W., Zheng, X., and Ma, H. (2024). Mechanism and effect of agricultural digitization on food production security. J. China Agric. Univ. 29, 307–320.
Meng, W., and Ren, B. (2023). The influence mechanism and spatial effect of digital finance on rural industrial integration. J. Southwest Univ. Natl. 44, 96–106.
Meng, K., and Wei, B. (2023). Rethinking the "time blind spot" of QCA method: finding "time" for public management research. Chin. Public Adm. 1, 96–104. doi: 10.19735/j.issn.1006-0863.2023.01.11
Meuwissen, M., Feindt, P., Spiegel, A., Termeer, C., Mathijs, E., De Mey, Y., et al. (2019). A framework to assess the resilience of farming systems. Agric. Syst. 176:102656. doi: 10.1016/j.agsy.2019.102656
Nie, S., Wang, G., and Zhong, F. (2024). New infrastructure drives the improvement of agricultural economic resilience: theoretical mechanism and empirical test. J. Agrofor. Econ. Manag. 1, 1–11.
Quan, Y., and Chen, Y. (2024). Influencing factors and promoting path of digital government construction: dynamic analysis based on national municipal panel data. E-Government 03, 54–67. doi: 10.16582/j.cnki.dzzw.2024.03.005
Qun, W., Ranran, C., Jingsuo, L., and Khan, N. (2024). Toward a sustainable agricultural system in China: exploring the nexus between agricultural science and technology innovation, agricultural resilience and fiscal policies supporting agriculture. Front. Sustain. Food Syst 8:14. doi: 10.3389/fsufs.2024.1390014
Ragin, C. (2008). Redesigning social inquiry: Fuzzy sets and beyond. Chicago: University of Chicago Press, 12–14.
Reggiani, A., Graaff, D., and Nijkamp, P. (2002). Resilience: an evolutionary approach to spatial economic systems. Netw. Spat. Econ. 2, 211–229. doi: 10.1023/A:1015377515690
Roberto, C., and Miguel, A. (2016). A general approach to panel data set-theoretic research. J. Adv. Manag. Sci. Inf. Syst. 1, 263–276. doi: 10.6000/2371-1647.2016.02.06
Schneider, C., and Wagemann, C. (2012). Set theoretic methods for the social sciences: A guide to qualitative comparative analysis.Cambridge. Cambridge, UK: Cambridge University Press.
Shang, Z., and Xie, J. (2024). The construction of resilient villages in poverty-stricken ethnic areas: logic, measurement and approach. J. South China Agric. Univ. 23, 1–11.
Shi, Y. (2024). The level measurement and regional difference analysis of China's agricultural economy resilience. Stat. Decis. 40, 122–126. doi: 10.13546/j.cnki.tjyjc.2024.21.021
Shi, X., and Song, P. (2023). The theoretical mechanism and realization path of digital inclusive finance supporting high-quality agricultural development. Financ. Theory Pract. 9, 74–85.
Silvia, R., Gianluca, B., Manlio, B., and Ivano, S. (2021). The digitalization of agriculture and rural areas: towards a taxonomy of the impacts. Sustainability 13, –5172. doi: 10.3390/su13095172
Sun, Z., Tian, Y., Lu, Y., Yao, G., and Xu, J. (2024). Study on the spatial-temporal relationship between data factor development and rural revitalization in China. Res. Agric. Mod. 1, 1–14.
Sun, Y., Zhang, S., Zhao, T., and Zhang, Y. (2022). The impact of digital technology innovation on industrial structure upgrading and its spatial effect: a case study of the Yangtze River Economic Belt. Soft Sci. 36, 9–16. doi: 10.13956/j.ss.1001-8409.2022.10.02
Tang, J., Gong, J., and Song, Q. (2022). Digital financial inclusion and agricultural total factor productivity: a perspective based on factor flow and technology diffusion. Chin. Rural Econ. 7, 81–102.
Tang, X., and Xie, S. (2023). Spatial and temporal distribution and configuration influencing factors of rural economic resilience. J. Shenzhen Univ. 40, 78–87.
Tornatzky, L., and Fleischer, M. (1990). The processes of technological innovation. Lexington, MA: Lexington Books.
Wang, H., and Fang, L. (2023). Spatio-temporal coupling coordination and driving force analysis of digitization and greening of agriculture in China. Resour. Environ. Yangtze Basin 32, 868–882.
Wang, F., Wang, H., and Kong, F. (2024). Enhancing effect of rural digital development on agricultural total factor carbon productivity. China Popul. Resour. Environ. 34, 79–90.
Wang, Z., Zeng, H., and Luo, R. (2023). Does digital infrastructure make agricultural economies more resilient? Study Pract. 44, 33–44. doi: 10.1097/01.EEM.0000855788.18128.8c
Wen, Y., and Jiang, C. (2023). Attention redistribution, external resource dependence and digital rural governance performance: a configuration analysis based on TOE framework. Chin. Public Adm. 7, 58–67. doi: 10.19735/j.issn.1006-863.2023.07.07
Xiang, Y., Li, Z., and Lu, Q. (2022). The spatial disequilibrium and convergence of high-quality development of China's agricultural economy. J. China Agric. Univ. 27, 305–316.
Ying, L., and Cui, C. (2022). How does blood transfusion inhibit hematopoietic production -- the operation logic and governance dilemma of Y County's e-commerce poverty alleviation project. Sociol. Rev. China 10, 186–203.
YoungKi, P., and Sunil, M. (2020). Organized complexity of digital business strategy: a configurational perspective. MIS Q. 44, 85–127. doi: 10.25300/MISQ/2020/14477
Yu, Y., Shi, C., Yang, X., and Mu, Y. (2023). The Impact Mechanism of Rural Digitalization on Agricultural Resilience in China: Based on Coupling Coordination Degree and Intermediary Effect Model. JSCAU 7, 308–320.
Zeng, F., Chen, Y., Wei, B., Wang, P., and Yang, S. (2024). Exploring the multiple paths of digital government development: a dynamic QCA analysis based on organizational transformation. IEEE Access 12, 18451–18472. doi: 10.1109/ACCESS.2024.3358375
Zhang, W., and Bai, Y. (2022). Regional differences, dynamic evolution of distribution and spatial correlation of rural revitalization level in China. J. Quant. Tech. Econ. 39, 84–102. doi: 10.13653/j.cnki.jqte.2022.02.006
Zhang, Y., and Han, C. (2024). Digital economy driving high-quality development path in Yellow River Basin: dynamic QCA analysis based on TOE framework. Reform Econ. Syst. 2, 25–32.
Zhang, M., and Hui, L. (2022). Spatial differences and influencing factors identification of agricultural economic resilience in China. World Agric. 1, 36–50. doi: 10.13856/j.cn11-1097/s.2022.01.004
Zhang, Y., and Jia, H. (2024). The driving factors and path selection for the development level of China’s Mariculture- a dynamic analysis based on the TOE framework. Sustain. For. 16:9272. doi: 10.3390/su16219272
Zhang, J., and Li, Y. (2024). The logical relation, dilemma review and advancing path of agricultural and rural modernization in the process of high-quality development. Agric. Econ. 1, 3–6.
Zhang, J., Long, J., and Wang, Z. (2024). What institutional environment is conducive to generating high entrepreneurial activity: dynamic QCA analysis based on provincial panel data. Sci. Technol. Prog. Policy 1, 1–13. doi: 10.13872/j.1000-0275.2024.0008
Zhang, H., Lu, B., and Quan, T. (2024). Study on the influence and mechanism of rural digitization on agricultural development resilience. Res. Agric. Mod. 45, 124–136.
Zhang, L., and Wen, T. (2022). How does digital financial inclusion affect the integrated development of rural industries. Chin. Rural Econ. 7, 59–80.
Zhao, W., and Xu, X. (2023). The effect and mechanism of digital economy on the resilience of agricultural economy. J. South China Agric. 22, 87–96.
Keywords: agricultural economic resilience, data elements, TOE framework, agricultural industry digitization, dynamic QCA
Citation: Luo W, Zuo S, Song Y and Tang S (2025) The impact of data elements on agricultural economic resilience: a dynamic QCA analysis. Front. Sustain. Food Syst. 8:1510328. doi: 10.3389/fsufs.2024.1510328
Received: 12 October 2024; Accepted: 26 December 2024;
Published: 10 January 2025.
Edited by:
Siphe Zantsi, Agricultural Research Council of South Africa, South AfricaReviewed by:
Mhlangabezi Slayi, University of Fort Hare, South AfricaCopyright © 2025 Luo, Zuo, Song and Tang. This is an open-access article distributed under the terms of the Creative Commons Attribution License (CC BY). The use, distribution or reproduction in other forums is permitted, provided the original author(s) and the copyright owner(s) are credited and that the original publication in this journal is cited, in accordance with accepted academic practice. No use, distribution or reproduction is permitted which does not comply with these terms.
*Correspondence: Shanxiang Zuo, MTAyMDIyMTMwMkBnbHV0LmVkdS5jbg==
Disclaimer: All claims expressed in this article are solely those of the authors and do not necessarily represent those of their affiliated organizations, or those of the publisher, the editors and the reviewers. Any product that may be evaluated in this article or claim that may be made by its manufacturer is not guaranteed or endorsed by the publisher.
Research integrity at Frontiers
Learn more about the work of our research integrity team to safeguard the quality of each article we publish.