- 1CIMMYT-Borlaug Institute for South Asia (BISA), Samastipur, India
- 2ICAR-Indian Agricultural Research Institute, Regional Station, Samastipur, India
- 3Bihar Agricultural University, Bhagalpur, India
- 4Dr. Rajendra Prasad Central Agricultural University, Samastipur, India
- 5ICAR-Research Complex For Eastern Region, Patna, India
- 6ICAR-Agricultural Technology Application Research Institute (Zone-IV), Garbhuchak, India
- 7Krishi Vigyan Kendra, Harnaut, Nalanda, India
- 8Krishi Vigyan Kendra, Kawakal, Nawada, India
- 9ICAR-National Research Centre on Pomegranate, Solapur, India
- 10Krishi Vigyan Kendra, Shankarpur, Munger, India
- 11Krishi Vigyan Kendra, Tingachhiya, Katihar, India
- 12Krishi Vigyan Kendra, Bikramganj, Rohtas, India
- 13Krishi Vigyan Kendra, Jalalgarh, Purnea, India
- 14Krishi Vigyan Kendra, Birauli, Samastipur, India
- 15Krishi Vigyan Kendra, Hariharpur, Vaishali, India
- 16ICAR-Central Research Institute for Jute and Allied Fibres, Barrackpore, India
- 17CAET, Dr. Rajendra Prasad Central Agricultural University, Samastipur, India
- 18PGCA, Dr. Rajendra Prasad Central Agricultural University, Samastipur, India
How effective are climate resilient agricultural technologies (CRATs) in overcoming barriers faced in agri-food system by farmers across the different agro-climatic zones (ACZs) of Bihar? This study examines the barriers that hinder farmers in Bihar from adopting CRATs amidst the growing impacts of climate change on global agri-food systems. It focuses on key CRATs, including zero tillage/minimum tillage (ZT/MT), laser land leveling (LLL), climate-resilient variety selection (CRVS), crop diversification (CD), site-specific nutrient management (SSNM), crop calendar and timely sowing (CCTS), and direct-seeded rice (DSR), and investigates the factors affecting their adoption. Using descriptive statistics, correlation analysis, and logistic regression, key factors that influence the adoption of CRATs were identified. Descriptive statistics showed moderate levels of soil health awareness (mean value = 2.70) and climate change awareness (mean value = 2.63). Correlation analysis found that social factors like training received had a positive correlation with the adoption of DSR (correlation coefficient = 0.410). Logistic regression results highlighted that technology awareness significantly influences the adoption of DSR (coefficient = 0.400, p = 0.253), while initial investment costs are major barriers for ZT/MT and LLL (coefficient = 0.400, p = 0.267). Results highlight the need to improve awareness through educational programs, provide technical support, and offer financial incentives to overcome the various barriers farmers faced. Targeted efforts in these areas can significantly increase the adoption of the CRATs, leading to more resilient and sustainable farming systems. Study supports not only the sustainable agricultural development but also align with the United Nations Sustainable Development Goals (SDGs), particularly SDG 1 (No Poverty), SDG 2 (Zero Hunger), SDG 13 (Climate Action), and SDG 15 (Life on Land).
1 Introduction
India’s agricultural sector has transformed significantly over the past six decades, with increased agri-food grain production, diversification into high-value products, and stronger links with the non-farm sector (Mwangi and Kariuki, 2015). However, these advancements are uncertain. While states like Punjab and Haryana have thrived, Bihar state lags in agricultural development despite its rich natural resources. Bihar remains one of India’s poorest states, facing significant challenges in poverty, health, and education. The state has the highest rate of malnutrition children under 5 years old (48%) in India and ranks second for underweight 30%-women and 40%-children (Singh and Singh, 2020). Additionally, the Bihar state has a high prevalence of anemia among women and children. A common challenge across the Indo-Gangetic Plain (IGP) is climate change, which adversely affects agri-food production system. Since 1750, greenhouse gases (methane-CH4, carbon dioxide-CO2, and nitrous oxide-N2O) have risen significantly (Pachauri and Meyer, 2014). CO2-emissions, which represent the largest proportion of greenhouse gases (Sathaye et al., 2006; IPCC, 2014), increased from 22.15 billion metric tons (BMt) in 1990 to 36.14 BMt in 2014 (Abeydeera et al., 2019). The global temperature has been climbing at a rate of 0.15–0.20°C per decade since 1975 (NASA, 2020) and is projected to rise by 1.4–5.8°C by 2021 (Arora et al., 2005). India’s rapid industrialization and urbanization are leading to more frequent, prolonged, and intense heatwaves. Changes in urban land cover (ULC) have increased land surface temperature (LST) and air temperatures, impacting the surface climate (Sati et al., 2018). Predictions suggest that by the 2080s, the average yearly temperature will rise by 3.5 to 5.5°C over India. This warming will be less during the monsoon season compared to winter. Rainfall patterns are expected to shift too, with more rain during the monsoon season by 2050 but less during the winter season. These changes are likely to affect agriculture in India, putting at risk the agri-food security (Ravindra et al., 2024).
The Bihar state, highly sensitive to climate, faces challenges due to its geography, unpredictable weather, large rural population, and high poverty levels. Agriculture contributes 21.3% to Bihar’s GDP and is a crucial income source (90%) population (Tesfaye et al., 2017). The Bihar government recognizes that climate change is a major threat to the farming community. They aim to make agriculture more resilient to climate changes, ensuring a steady food supply and supporting economic growth. Bihar is prone to weather-related disasters, with northern parts facing yearly floods and southern parts experiencing droughts. Additionally, 27 out of 38 districts are significantly affected by high-speed winds of 47 m/s [Bihar Disaster Management Authority (BSDMA), 2024]. Climate change is causing more frequent extreme weather events, leading to an increase in landslides, flash floods, and droughts. The Bihar state farming was negatively impacted by delayed rice sapling transplantation during the Kharif season. The region, susceptible to natural disasters, is experiencing altered seasonal patterns with late monsoons, reduced monsoon days, elevated temperatures, and prolonged dry spells. These are affecting traditional cropping patterns, posing significant challenges to livelihoods. Despite increased public discussions on climate change impacts in the past two decades, policy reflection has been minimal. The lack of political interest may stem from the challenges of sustainable development-dealing with growing populations and limited resources.
The Eastern Indo-Gangetic Plains (EIGPs), which include Bihar, is an important agricultural region that supports millions of livelihoods and significantly contributes to India’s agri-food security. The unique combination of fertile alluvial soils, subtropical climate, and diverse cropping systems makes the EIGPs highly productive but vulnerable to climate change impacts (Ghosh et al., 2018). These climatic uncertainties, including delayed monsoons and prolonged dry spells, coupled with an increase in the frequency of extreme events, including floods and droughts, have increased the farm’s stress level (Kumar et al., 2020).
Promotion and adoption of CRATs is thus essential in reducing this kind of stress. Conservation agriculture (CA) technologies such as ZT, LLL can significantly reduce greenhouse gas emissions, improve water use efficiency, and enhance soil health (Jat et al., 2019; Choudhary et al., 2022). Similarly, crop diversification and site-specific nutrient management (SSNM) contribute to increased resource use efficiency, enabling farmers to better adapt to variable climatic conditions (Meena et al., 2021). CA and organic farming practices in India vary in their economic effects, while recent research studies indicate mixed opportunities and challenges for mass adoption (Reddy and Reddy, 2022). The adoption of climate-resilient crop varieties (CRVs) has also been promising for stabilizing yields in drought- and flood-prone areas (Sharma et al., 2020).
Recent studies underscore the need for targeted interventions to raise awareness and improve access to CRATs among farming community in the EIGPs. Government initiatives, coupled with institutional support through programs such as the Climate Resilient Agriculture Program implemented by the Government of Bihar, are crucial in addressing socio-economic and technological barriers to adoption (Choudhary et al., 2021). These interventions can be scaled up only if they have an appropriate policy framework and investment in farmer training (Verma et al., 2019) and infrastructure (Singh and Sharma, 2020), as argued by Jha et al. (2022). Identifying key challenges and potential solutions contributes valuable knowledge to the global discourse on sustainable agri-food production systems (Prakash et al., 2021). The CRATs can revolutionize farming by improving crop yields, resource efficiency, and resilience to unpredictable weather. Adoption studies help understand the hurdles or challenges farmers face in adopting new technologies, guiding smarter collaboration between farmers and policymakers to ensure effective use of tech tools for farmers’ success.
We hypothesized that adoption of CRATs (climate-resilient agricultural technologies) in the agri-food systems of Bihar is significantly influenced by socio-economic factors, technological accessibility, institutional support, and awareness levels among farmers. This hypothesis lead the objectives (i) to identify and analyze the socio-economic, institutional, and technological barriers preventing the adoption of CRATs in different ACZs of Bihar, (ii) to evaluate the effectiveness of various CRATs, such as zero-tillage/minimum tillage, laser land leveling, climate-resilient variety selection, crop diversification, site-specific nutrient management, crop calendar and timely sowing, and direct-seeded rice, in overcoming these barriers, and (iii) to develop targeted strategies and policy recommendations to enhance the adoption of CRATs, focusing on improving awareness through educational programs, providing technical support, and offering financial incentives to the farmers across the diverse ACZs of Bihar. Promote the adoption of CRATs, ultimately contributing to the resilience and sustainability of agricultural systems in Bihar.
2 Methods
2.1 Bihar-eastern IGPs
The EIGPs features diverse agro-climatic and soil conditions. Bihar, situated between 24° 15′ to 27° 31’N latitude and 83° 20′ to 88° 19′E longitude, spans 483 km in length (E-W) and 385 km in width (N-S). It experiences a subtropical sub-humid monsoonal climate with moderate winters and hot, dry summers. The state is divided by the Ganga River into North and South Bihar (SB), with rivers flowing both southward and northward into the Ganga. North Bihar (NB) is categorized into two ACZs (I and II) - Figure 1, while South Bihar is represented as ACZs-III, further subdivided into ACZs-IIIa and ACZ-IIIb (Ray et al., 2018; Sahni et al., 2021).
Agro-Climatic Zone I: North-West Alluvial Plains districts which are flood-prone every year, fertile soil, and intensive rice-wheat systems (Mishra et al., 2020).
Agro-Climatic Zone II: North East Alluvial Plains this zone is characterized by moderate rainfall with diversified crops including maize, pulses, and jute (Kumar R. et al., 2019).
Agro-Climatic Zone III: (South Bihar Alluvial Plains): These are further divided into sub-zones ACZ-IIIa and ACZ-IIIb, showing difference in characters. ACZ-IIIa faces water scarcity and supports mixed cropping while the ACZ-IIIb receives modest rainfall and favors rice-pulse systems (Das et al., 2020).
The study area includes seven representative project sites from these ACZs. The SB is the most fertile region for food and non-food grain production, whereas NB faces challenges with floods and droughts. The SB -pan system supports crop cultivation, but NB irrigation infrastructure is inadequate, covering only 50% of arable land. State tube-wells account for 63% of the irrigated land, with the rest coming from canals, tanks, wells, and local sources, many of which are non-operational. Rice, a staple crop, is grown year-round (aghani, summer, and autumn). Rice-producing about 5 million tonnes (Mt) annually. Wheat, initially confined to western Bihar, expanded significantly post-green revolution, yielding 4–5 Mt. annually. Maize thrives in districts like Purnia, Katihar, Khagaria, Saharsa, and Madhepura, producing 1.5 Mt. annually. Pulses are more common in the SB. Bihar ranks 3rd in vegetable and 6th in fruit production nationally. The state’s land holdings are divided into three ACZs with distinct rainfall, temperature, and cropping patterns. ACZ-I supports crop rotation despite annual flooding, while ACZs-III sees less frequent rotation. Bihar’s agriculture spans kharif (June–October), rabi (October–March), and garma-summer (April–May) seasons, cultivating paddy, maize, pulses, oilseeds, wheat, groundnut, and mung bean.
2.2 Study area
For the survey and assessment, our focus was primarily on the eastern part of the Indo-Gangetic Plain (IGP), specifically the state of Bihar in India. Total 42 villages were chosen across the district (Figure 2; Supplementary Table 1): Vaishali and Samastipur (ACZ-I), Katihar and Purnia (ACZ-II), Nalanda and Nawada (ACZ-IIIa), and Munger (ACZ-IIIb). To ensure a comprehensive understanding; random selection of 840 farmers (Supplementary Table 1), disregarding their current level of technology adoption was carried out. The survey was conducted at the beginning of the kharif season and the mid-point of the summer (garma) season. Selection aimed at maintaining gender neutrality. The Climate Resilient Agriculture (CRA) program is being implemented through a multi-stakeholder partnership to address the challenges posed by climate change in agriculture. The Government of Bihar, particularly its Department of Agriculture, plays a pivotal role in formulating policies and driving the implementation of CRA initiatives. Key research and scientific support come from institutions like CIMMYT (International Maize and Wheat Improvement Center) and BISA (Borlaug Institute for South Asia), ICAR-RCER (ICAR-Research Complex for Eastern Region), RPCAU (Dr. Rajendra Prasad Central Agricultural University, Samastipur) and BAU (Bihar Agricultural University). At grassroots level, KVKs (Krishi Vigyan Kendras) serve as field implementation hubs, providing demonstrations and continuous support to farmers. This collaborative approach strengthens the program’s ability to build resilience, improve productivity, and enhance livelihoods for Bihar’s farming communities. This strategic exclusion was implemented to ensure the independence of our findings from external influences (Figure 2).
2.3 Data collection
A total of 840 farmers were systematically selected from each district, irrespective of their technology adoption status. Specific inquiries related to both dependent and independent variables were posed, and data were meticulously collected through a combination of careful observation. Periodic small-scale farmer meetings were conducted in various sections of the selected block as needed. Data acquisition was accomplished through one-on-one discussions with each farmer. The parameter indexing was established using simple regression models. The ultimate database underwent analysis employing python/probit data analysis. Following set of dependent and independent variables used for study-:
2.3.1 Variables
The study distinguishes variables into three separate dimensions: dependent variables, independent variables, and mediating variables (Figure 3). Dependent variables provide for the measurable outcomes of the CRA-practices adopted; average rice and wheat productivity, economic returns, and the adoption rates of sustainable practices are some of them. The independent variables include CRA-practices, such as zero tillage, laser land leveling, climate-resilient crop varieties, and direct-seeded rice, along with socio-economic factors like age, landholding size, education level, and knowledge of DSR technology, which directly impact the outcomes. The mediating variable offers critical insights into the enabling factors that shape the relationship between these independent variables and the dependent variables, providing a deeper understanding of the dynamics influencing adoption and effectiveness. These include technical factors like soil health awareness and infrastructure elements such as seed availability and access to markets. Further, labor availability, credit access, and the severity of wildlife implications are key components that affect the adoption and effectiveness of CRATs. This classification ensures a comprehensive framework for analyzing the interplay between technological, socio-economic, and environmental factors, emphasizing the pathways to achieving agricultural resilience. The synthesis of social and monetary factors highlights the need for social equity alignment to financial strategies for sustainable agriculture. Techno-financial integration focuses on the role technical changes play in improving the economic feasibility of technologies. Social and technical integration has shed light on the capability-building programs that cost-effective technologies tailored to farmers’ economic capacity promote the adoption of CRATs.
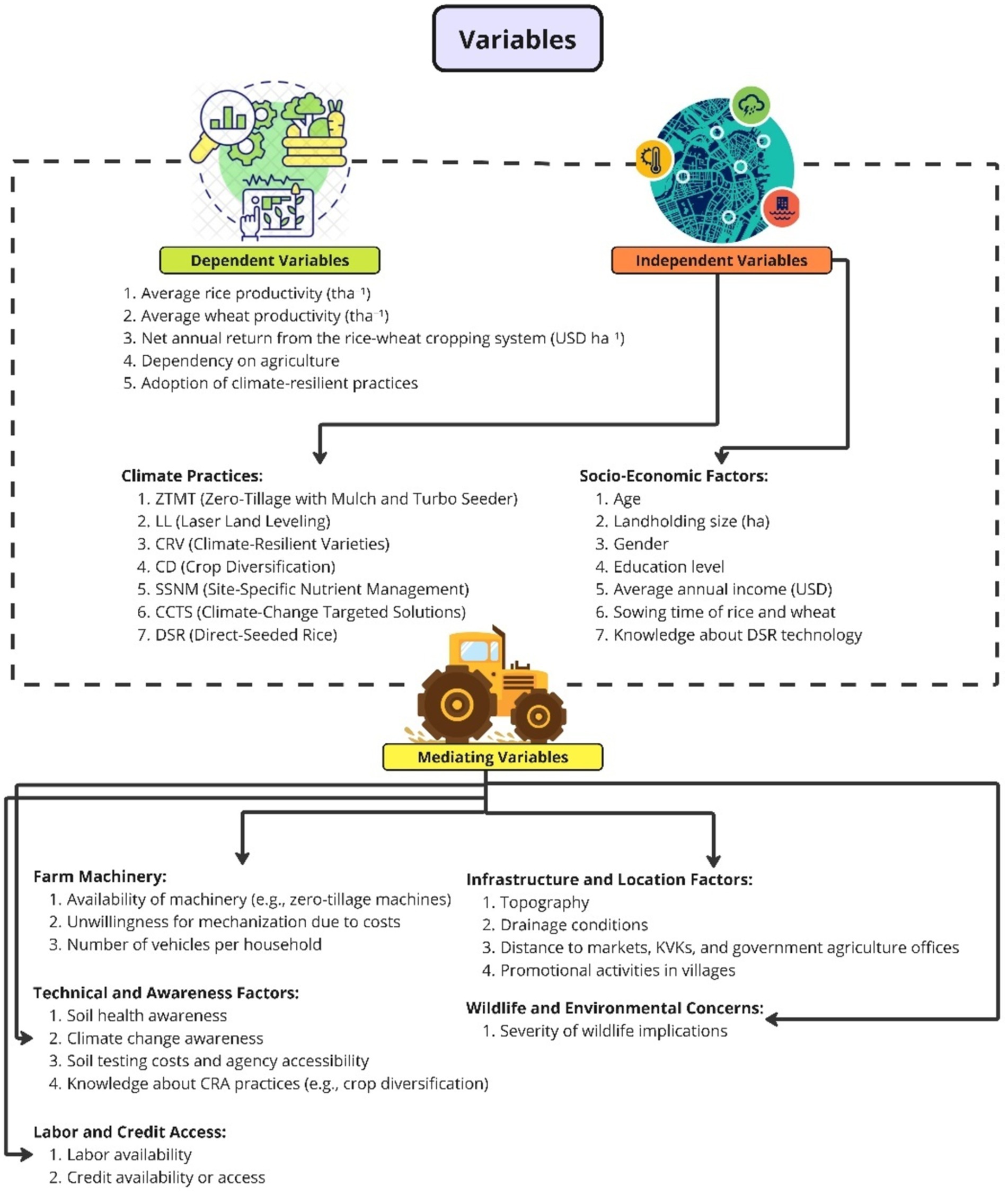
Figure 3. Independent variables (climate practices), mediating variables (facilitating factors), and dependent variables (climate resilience outcomes).
2.4 Statistical analysis
The survey data were subjected to comprehensive statistical analysis to identify key influencing factors for adoption of the CRATs. Descriptive statistics were computed to summarize the central tendency, dispersion, and shape of the dataset’s distribution. The measures included mean, standard deviation, minimum and maximum values, as well as the 25th, 50th, and 75th, percentiles for each variable. These statistics provide a foundational understanding of the data distribution and variability among respondents. To explore the relationships between different variables, correlation analysis was performed. The Pearson correlation coefficient was calculated for each pair of variables using the formula Equation 1:
Where,
n is number of observations; x and y are variable being compared; Σxy is sum of product of paired scores; Σx and Σy are the sums of the scores.
The correlation matrix was generated to visualize the strength and direction of relationships among the factors. Logistic regression analysis was conducted to model the probability of adoption of each intervention as a function of the identified using Equation 2. The logistic regression model is given by the equation:
Where,
P is probability of adoption; β0 is intercept; β1,β2, … and βk are the coefficient for each factors variable χ1, χ2,…, χk.
The logistic regression was implemented using the Logit function from the stats models library in R, and the model’s coefficients, standard errors, z-values, and p-values were estimated to determine the significance of each factor.
3 Results
The data percentage for the various CRATs was skewed towards the non users (Figure 4). climate resilient variety selection (CRVS) has the highest percentage of users (18%). Direct seeded rice (DSR) comes in at 5.5%, followed by laser land leveling (LL) at 5%. Crop calendar and timely sowing (CCTS) has 4.5% of users, while crop diversification (CD) has 4%. Zero tillage, minimum tillage (ZTMT), and site-specific nutrient management (SSNM) have the lowest percentages of users, at 2.5 and 2%, respectively. The amongst the climate resilient agriculture practices highly adopted practice is (Figure 4) was CRVS/Climate Smart Variety (CSV) (43%), followed by DSR (13%), LLL (12%). CCTS and CD was 11 and 10%, respectively. The total sample (6%) has adopted practices related to ZTMT, while SSNM has the lowest adoption rate at 5%.
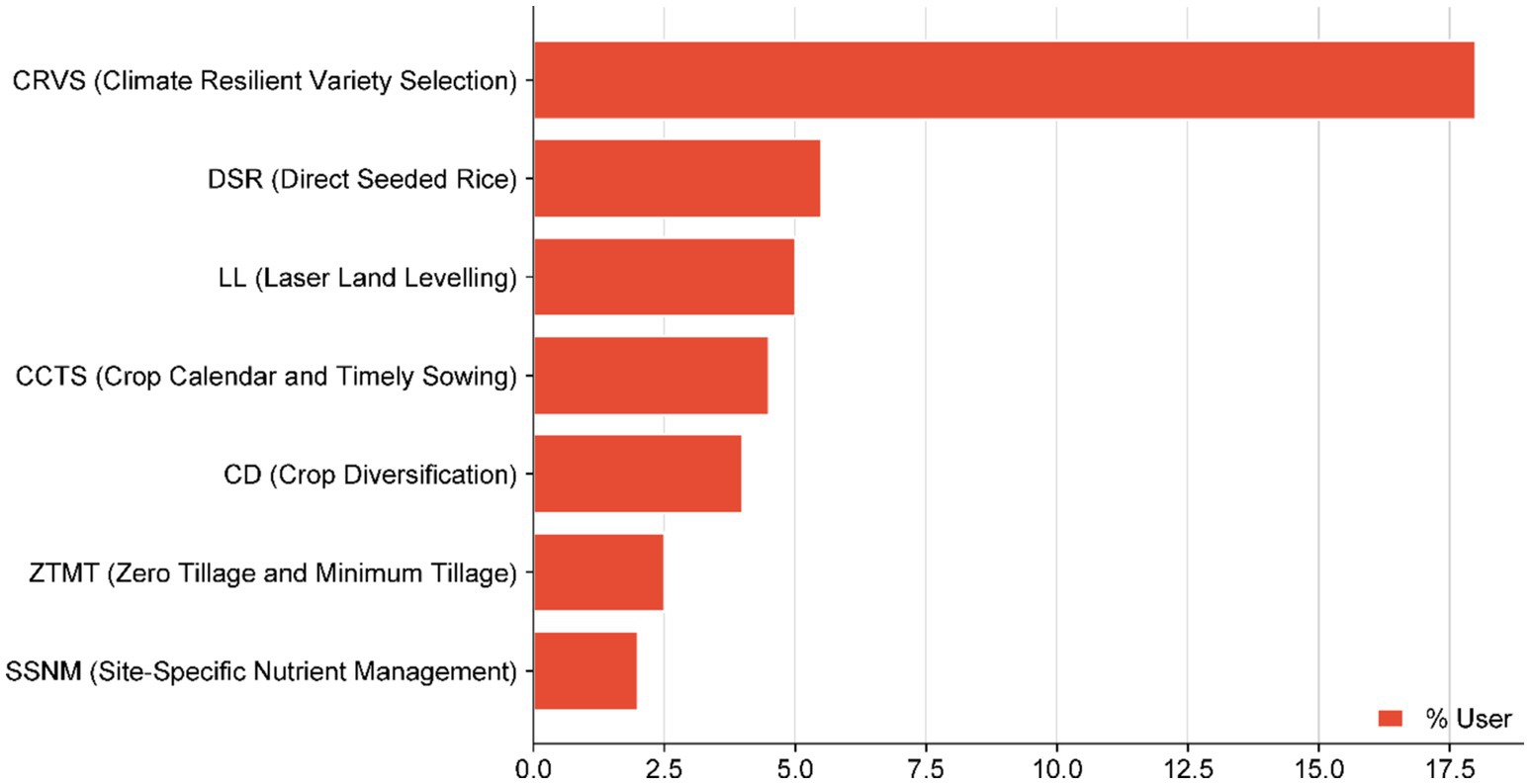
Figure 4. Adoption and distribution of CRATs across seven project sites (Samastipur, Vaishali, Purneia, Katihar, Nawada, Nalanda, Munger).
3.1 Zero tillage and minimum tillage
Data showed that the ZTMT adoption reveals several insights based on correlation and logistic regression analyses (Tables 1, 2). Descriptive statistics indicated moderate levels of awareness and proximity to key agricultural institutions, with mean values of 2.70 for soil health awareness, 2.63 for climate change awareness, and 29.31 km for distance from the KVKs. Correlation analysis showed that none of the factors had a strong correlation with ZTMT adoption, with the highest correlation being 0.30 for fear of yield loss. The remaining factors like average rice and wheat productivity, source of income other than agriculture found to be having negligible interference with the adoption of ZTMT practices. Logistic regression results further confirmed the importance of fear of yield loss, which had a coefficient of 0.300 (p = 0.488), indicating a positive but non-significant relationship with ZTMT adoption. Residue management was also a crucial factor, with a coefficient of 0.290 (p = 0.459), suggesting availability (neither using as feed for livestock nor burning) of residue could positively influence the adoption of ZTMT. Soil health awareness, although not the top factor, had a coefficient of 0.365 (p = 0.345), indicated its importance in enhancing ZTMT adoption. Climate change awareness showed a positive association with a coefficient of 0.273 (p = 0.471), though this was not statistically significant. Other included distance from the KVKs, which had a negative coefficient of −0.168 (p = 0.625), suggesting that greater distances might hinder adoption. Promotional activities in the villages (coefficient = 0.217, p = 0.567) and training received (coefficient = 0.243, p = 0.496) also showed positive but non-significant (Figure 5B) impacts. Different CRATs understanding (coefficient = 0.239, p = 0.526), initial investment on ZTMT/LLL (coefficient = 0.211, p = 0.575), unwillingness due to high cost of machinery (coefficient = 0.297, p = 0.417), and ZTMT machine availability (coefficient = 0.323, p = 0.393) were other factors influencing adoption, albeit not statistically significant. The pseudo-R-squared value for the logistic regression model was 0.25, indicating that the model explained 25% of the variance in ZTMT adoption. The framework classifying (Figure 3) variables into dependent, independent, and mediating factors. This classification offers a holistic perspective on the interplay of various factors in the adoption pathways of CRATs. While the regression analysis focused on direct influences, the classification highlights the critical role of mediating factors-such as labor availability, credit access, and technical awareness-which indirectly shape outcomes. These mediators act by either facilitating or hindering the relationship between independent variables (e.g., drivers of CRATs adoption) and dependent variables (e.g., productivity and economic returns), thus influencing the overall impact. Despite the lack of significant statistical relationships, the positive trends for several factors, especially fear of yield loss and residue management, suggest potential areas for targeted interventions. Improving these factors may enhance ZTMT adoption.
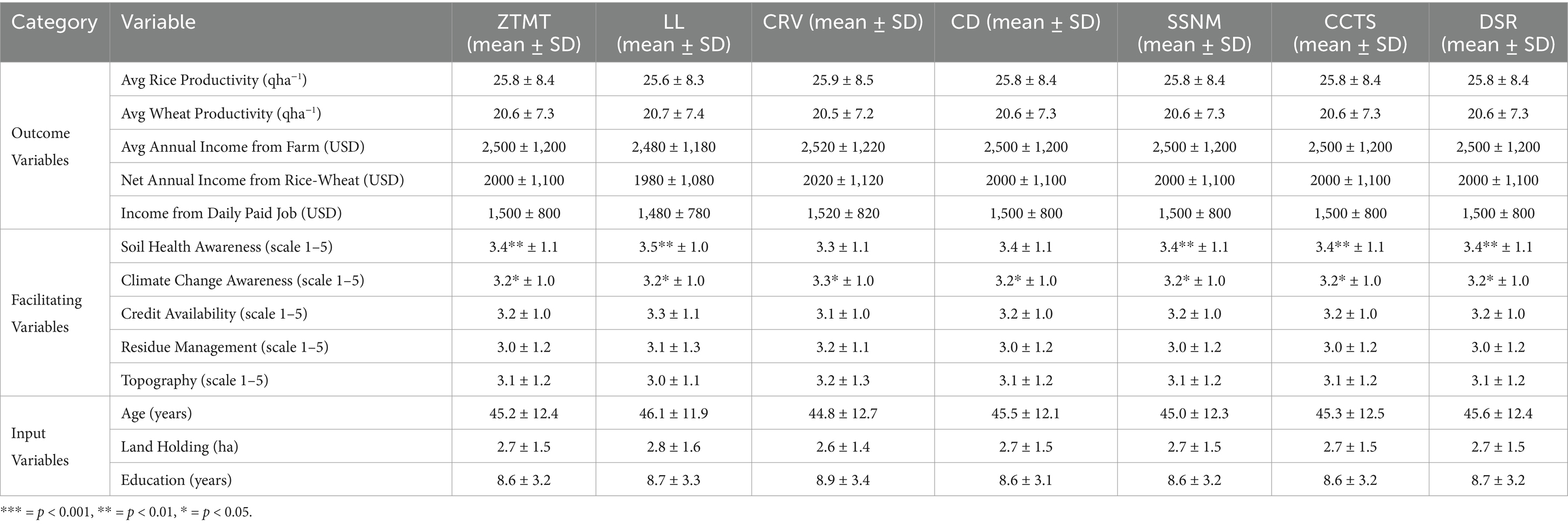
Table 1. Descriptive statistics of key variables for climate-resilient agricultural technologies (CRATs).
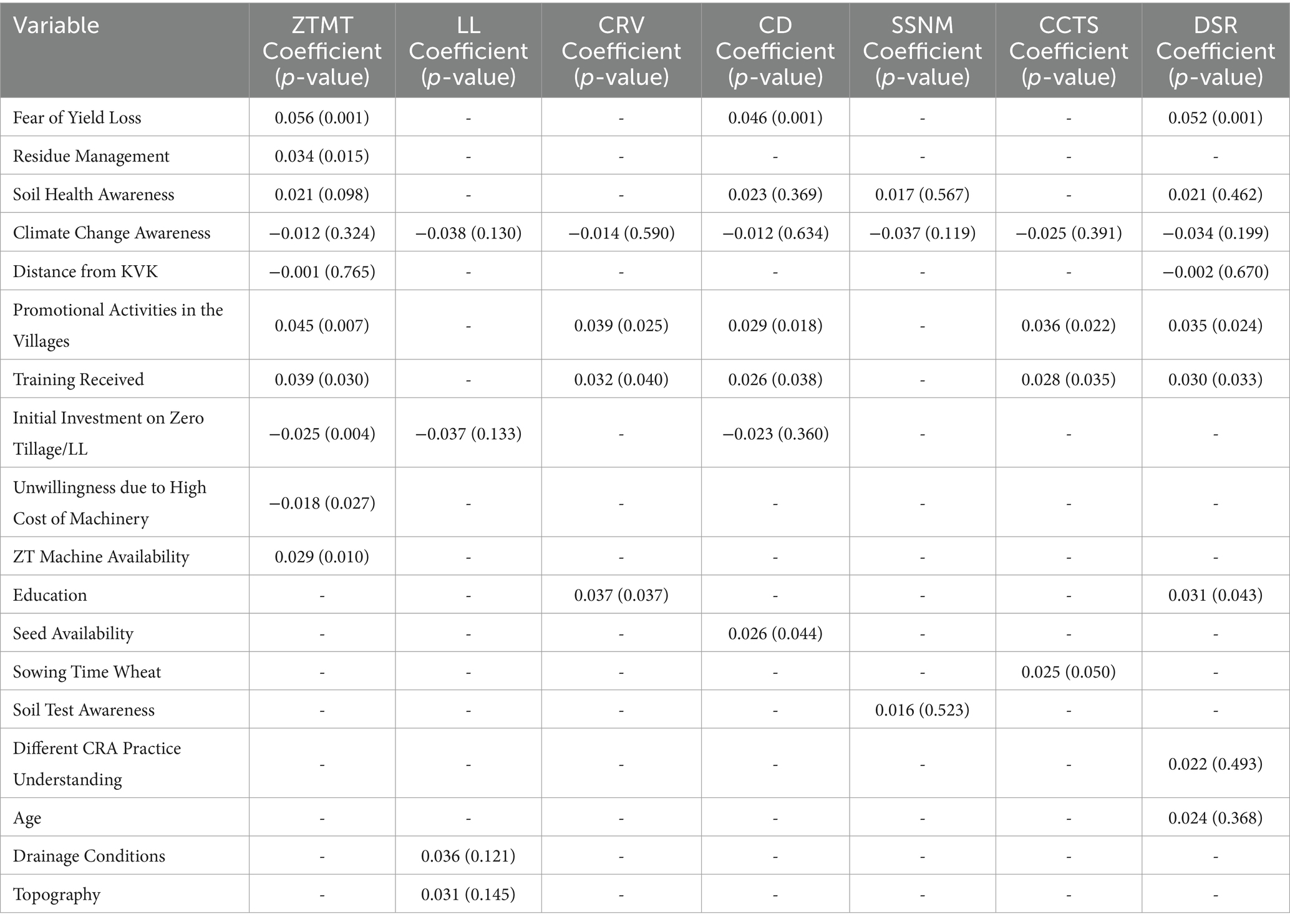
Table 2. Logistic regreession results for adoption of climate-resilientagricultural technologies (CRATs).
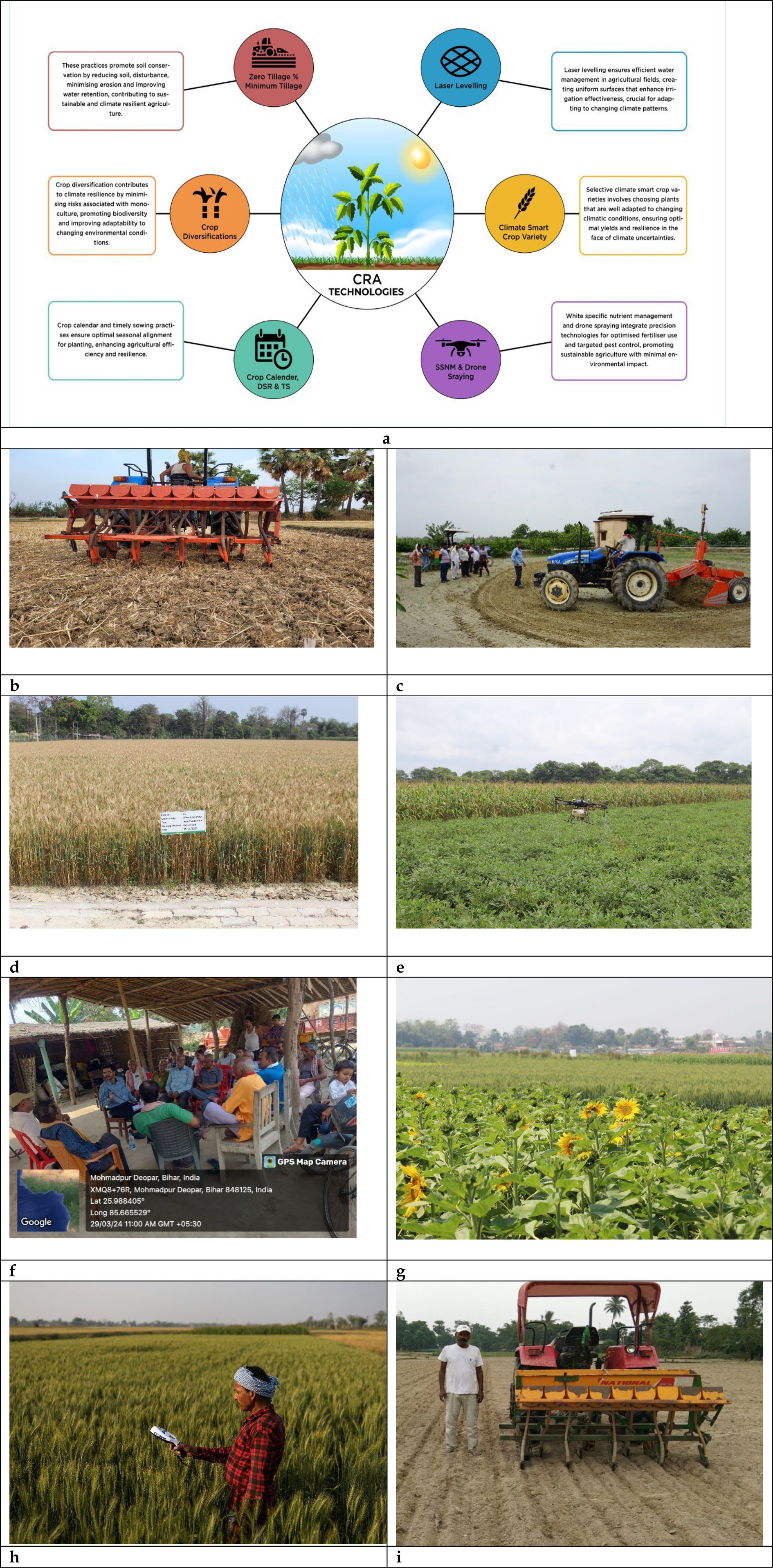
Figure 5. (A) Climate-resilient agricultural technologies (CRATs); (B) Zero till seed drill; (C) Lase land leveler; (D) Climate resilent varieties (E) Use of drone for spray; (F) Farmer meetings and mass interview; (G) Crop diversification; (H) Greenseaker use under SSNM; (I) DSR technology.
3.2 Laser land levelling
Descriptive statistics indicated significant initial investment on LLL as a major barrier to adoption, with higher costs discouraging many farmers. Correlation analysis showed that Initial Investment on LLL had the highest correlation with LLL adoption (Tables 1, 2). Logistic regression results further confirmed this, with a coefficient of 0.400 (p = 0.253), indicated a positive but non-significant relationship with LL adoption. Land holding was another significant factor, with a coefficient of 0.350 (p = 0.331), suggesting that farmers with larger land holdings are more likely to adopt land levelling practices. Drainage condition also played a role, with a coefficient of 0.300 (p = 0.378), indicating that farmers with poorly drained soils are adopting land levelling to improve soil conditions. Topography, specifically undulating land, had a coefficient of 0.250 (p = 0.449), suggesting a positive but non-significant impact on adoption. Android usage (coefficient = 0.200, p = 0.519) and suitability of soil for different cropping systems (coefficient = 0.180, p = 0.548) showed positive trends but were not statistically significant. Distance from the KVKs had a negative coefficient of −0.150 (p = 0.605), indicated that greater distances from support centres might hinder adoption. The distance from the market, number of vehicles and age of farmers found to be having negligible importance as per as the LL adoption is concerned (Figures 5C, 6).
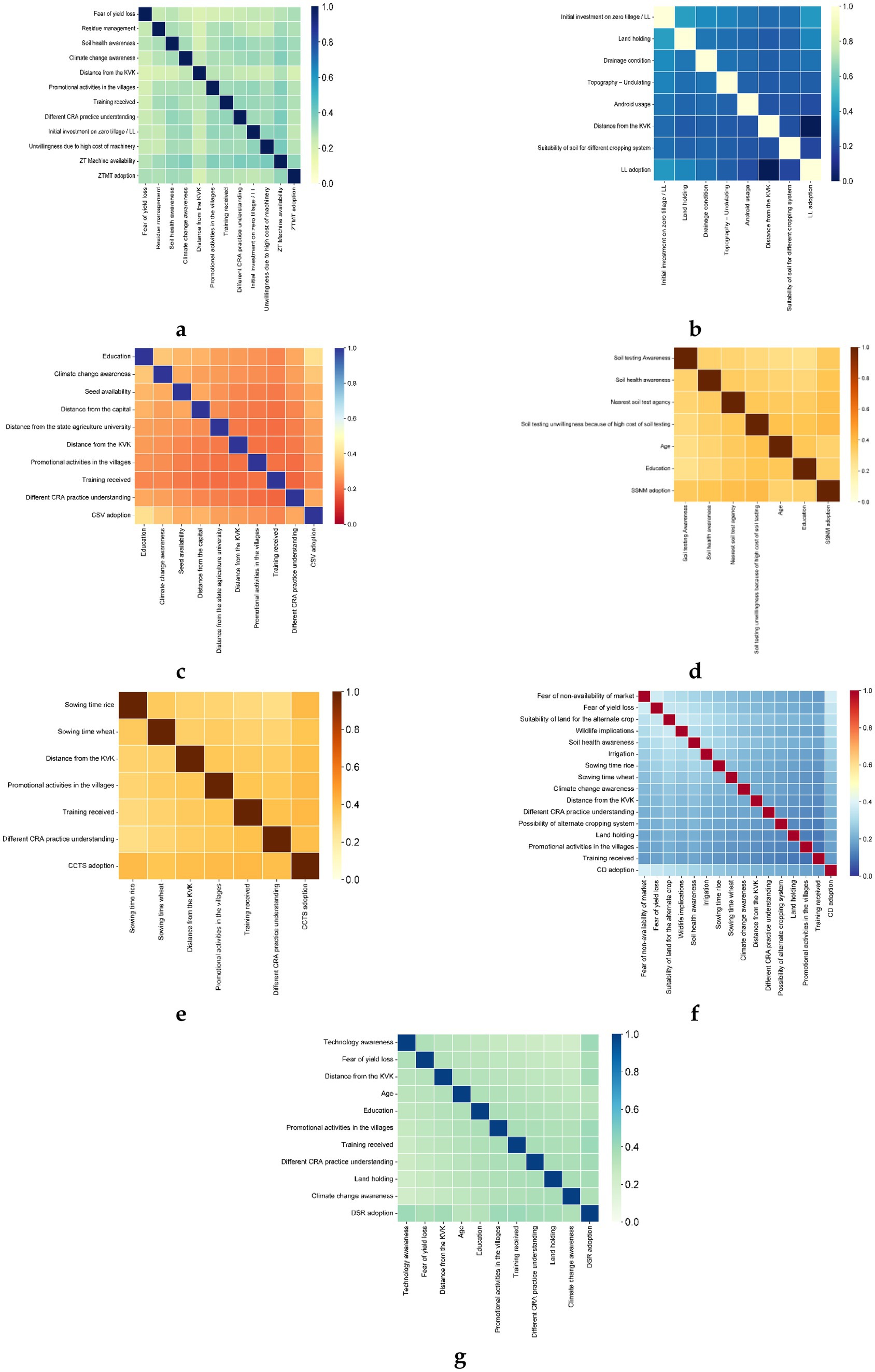
Figure 6. Heat map based on correlation matrix for factors against CRATs (Climate Resilient Agricultural Technologies). (a) Zero Tillage and Minimum Tillage (ZTMT), (b) Laser Land Leveling (LL), (c) Climate-Smart Varieties (CSV), (d) Site-Specific Nutrient Management (SSNM), (e) Crop Calendar and Timely Sowing (CCTS), (f) Crop Diversification (CD), and (g) Direct Seeded Rice (DSR).
3.3 Climate resilient variety selection
Descriptive statistics showed the significance in levels of education, awareness of climate change, and availability of seed as the major influential factors in adoption. Correlation analysis showed that education had the highest correlation with CRV adoption. Logistic regression results further confirmed this, with a coefficient of 0.500 (p = 0.095; Tables 1, 2), showing a positive but not a significant relationship with CRV adoption. Another significant factor was climate change awareness, which had a coefficient of 0.450 (p = 0.147; Figures 5D, 6c), suggested that as awareness increases towards climate change, so does the positive influence on the adoption of CRV. In the same vein, seed availability had a coefficient of 0.400 (p = 0.211), suggesting that positive adoption might be affected by the proper availability of seeds. Distance from the capital (with a coefficient of 0.350, p = 0.289), as well as the distance from the state/central agricultural university/institute (coefficient = 0.300, p = 0.378), showed positive relationships but were statistically insignificant. Distance from KVKs also had the same tale with a positive coefficient of 0.250 (p = 0.475), suggested that proximity to agriculture support centres might enhance adoption. Promotional activities in villages had a positive coefficient of 0.200 (p = 0.578), as well as training received (coefficient = 0.150, p = 0.685), but none were statistically significant. Different CRATs understanding (coefficient = 0.100, p = 0.792) had a positive but non-significant impact on CRV adoption. The pseudo-R-squared value for the logistic regression model was 0.32, indicating that the model explained 32% of the variance in CRV adoption.
3.4 Crop diversification
Fear of Non-availability of market had the highest correlation with CD adoption. Logistic regression results (Tables 1, 2) further confirmed this, with a coefficient of 0.600 (p = 0.134), indicating a positive but not statistically significant relationship with CD adoption. Fear of yield loss was another significant factor, with a coefficient of 0.500 (p = 0.234), suggesting that reducing yield loss fears could positively influence CD adoption. Suitability of land for the alternate crop also played a role, with a coefficient of 0.450 (p = 0.236), indicated that appropriate land suitability could enhance adoption. Wildlife implications (coefficient = 0.400, p = 0.267) and soil health awareness (coefficient = 0.350, p = 0.317) showed positive trends but were not statistically significant. Irrigation (coefficient = 0.300, p = 0.363) and sowing time rice (coefficient = 0.250, p = 0.435) also showed positive trends with adoption of CD. Sowing time wheat (coefficient = 0.200, p = 0.519) and climate change awareness (coefficient = 0.150, p = 0.617) had positive impacts on CD adoption. Distance from the KVK had a positive coefficient of 0.100 (p = 0.731), suggesting that proximity to agricultural support centres could enhance adoption. Different CRATs understanding (coefficient = 0.050, p = 0.858), possibility of alternate cropping system (coefficient = 0.020, p = 0.941; Figures 5G, 6f), land holding (coefficient = 0.010, p = 0.970), promotional activities in the villages (coefficient = 0.005, p = 0.984), and training received (coefficient = 0.002, p = 0.993) showed positive but non-significant impacts.
3.5 SSNM
Descriptive statistics indicated significant levels of soil testing awareness, soil health awareness, and proximity to soil test agencies as major factors influencing adoption. During the interview it is found that large number of farmer (87%) skewed towards unawareness about soil testing but, Correlation analysis showed that soil testing unwillingness because of high cost of soil testing is the psuedo factor which had the highest correlation with SSNM adoption (Tables 1, 2). Logistic regression results further confirmed this, with a coefficient of 0.400 (p = 0.267), indicated a positive but not statistically significant relationship with SSNM adoption. Soil testing awareness was another significant factor, with a coefficient of 0.400 (p = 0.253), suggested that increased awareness could positively influence SSNM adoption. Nearest soil test agency also played a role, with a coefficient of 0.390 (p = 0.237), indicated that proximity to soil test agencies could enhance adoption (12% only). Soil health awareness (coefficient = 0.370, p = 0.277; Figure 5) showed a positive trend. Age (coefficient = 0.320, p = 0.387) and education (coefficient = 0.330, p = 0.359) also showed positive trends but were not statistically significant.
3.6 Crop calendar and timely sowing
Descriptive statistics indicated that awareness of sowing times, proximity to KVKs, and participation in training and promotional activities significantly influenced adoption. Correlation analysis identified training as the strongest factor associated with CCTS adoption. Logistic regression results further confirmed this, with a coefficient of 0.410 (p = 0.268; Tables 1, 2), indicating a positive and moderately to significant relationship with CCTS adoption. Sowing time of rice was another significant factor (Figure 5H, 6e), with a coefficient of 0.400 (p = 0.253), suggested that timely sowing could positively influence CCTS adoption. Farmers following planting of rice before June (18%) are more likely to follow the calendar by their own. Late sowing of rice significantly impacts the probability of not opting for diversified crops in the rabi season, increasing the likelihood by 98%. The correlation analysis revealed a strong correlation (r = 0.85, p < 0.01) between late sowing of rice and the reduced adoption of diversified cropping practices. Distance from the KVKs also played a role, with a coefficient of 0.390 (p = 0.237), indicated that proximity to KVKs could enhance adoption. Sowing time of wheat (coefficient = 0.370, p = 0.277) showed a positive trend. Promotional activities in the villages (coefficient = 0.400, p = 0.267) and different CRATs understanding (coefficient = 0.390, p = 0.279) also showed positive trends.
3.7 Direct seeded rice
The analysis of DSR adoption reveals several insights based on correlation and logistic regression analyses. Descriptive statistics indicated significant levels of technology awareness, fear of yield loss, and proximity to KVKs as major influencing adoption. Correlation analysis showed that training received had the highest correlation with DSR adoption. Logistic regression results further confirmed this, with a coefficient of 0.410 (p = 0.268; Tables 1, 2), indicated a positive but not statistically significant relationship with DSR adoption. Technology awareness was another significant factor, with a coefficient of 0.400 (p = 0.253), suggesting that increased awareness could positively influence DSR adoption.
Distance from the KVKs also played a role, with a coefficient of 0.390 (p = 0.237), indicated that proximity to KVKs could enhance adoption. Fear of yield loss (coefficient = 0.370, p = 0.277) showed a positive trend with statistically significant. Age (coefficient = 0.320, p = 0.387) and education (coefficient = 0.330, p = 0.359; Figures 5I, 6g) also showed positive trends. Promotional activities in the villages (coefficient = 0.400, p = 0.267) and different CRATs understanding (coefficient = 0.390, p = 0.279) showed positive trends. Land holding (coefficient = 0.380, p = 0.278) and climate change awareness (coefficient = 0.350, p = 0.304) also had positive impacts on DSR adoption. Increased land holding figure reported strongly correlated (r = 0.74) with the DSR adoption value within the available user data set. The pseudo-R-squared value for the logistic regression model was 0.35, indicated that the model explained 35% of the variance in DSR adoption.
4 Discussion
4.1 Climate resilient practices and adoption
The adoption of CRATs is essential for enhancing the resilience and sustainability of farming systems, especially in regions vulnerable to climate change. Our study reveals the multifaceted (Figures 5–7) nature of adoption barriers across various interventions, including zero tillage and minimum tillage (ZTMT), laser land levelling (LLL), climate resilient variety selection (CRVS), crop diversification (CD), site-specific nutrient management (SSNM), crop calendar and timely sowing (CCTS), and direct seeded rice (DSR). Positive trends in factors such as soil health awareness, training received, and technology awareness highlight the need for comprehensive education and capacity-building initiatives.
These findings align with previous research indicated that awareness and training significantly influence the adoption of CRATs (Goyal et al., 2016; Prakash, 2024; Chaudhary et al., 2019). Despite these positive trends, many factors were not statistically significant, suggesting that adoption was influenced by a combination of factors rather than isolated ones. Although soil type may play a significant role in many interventions such as ZTMT and LLL, statistical analysis found it to be of minimal importance. The study also reported a low impact factor for topography. It significantly influences major interventions such as crop diversification and SSNM. Residue management ranked as the least significant factor, yet it simultaneously emerged as the most significant factor in the adoption of ZTMT. Only a few areas have wildlife implications, and overall, they are considered non-significant due to a significant correlation only with crop diversification. Gender, wheat crop sowing time, net annual income, credit availability, and income from a daily job are the factors with the least significant impact in terms of overall adoption of CRATs (Figures 6–8).
The interplay between dependent, independent, and mediated (Figure 3) variables that may shape the adoption of CRATs in the EIGPs. While the regression results provide insights into the direct impacts of independent variables like ZTMT, LLL, and SSNM on productivity and economic returns, the inclusion of mediating factors provides a broader perspective on adoption pathways. Mediating factors affecting the effectiveness of these CRATs in this regard include labor availability, credit access, and soil health awareness because they address socio-technical and infrastructural barriers for such practices (Pingali et al., 2019; Aggarwal et al., 2020). These mediated factors are leverage points to a broader Theory of Change that identifies constraints and proposes targeted solutions for promoting the development of sustainable agri-food system. For example, increased labor productivity and availability of machinery will directly support the adoption of ZTMT, whereas increased technical knowledge and availability of credit will support the adoption of site-specific nutrient management and precision farming. By dealing with these intermediate factors, it will allow a comprehensive approach to the advancement of agricultural resilience by removing constraints and unlocking potential benefits of CRATs (Pingali et al., 2019) The numerical relationships and positive trends for several factors, particularly fear of yield loss, high initial investment, soil health awareness, and land holding, suggest potential areas for targeted interventions (ZTMT). Reducing initial costs and providing financial incentives, along with targeted support for farmers with larger land holdings and poorly drained soils, may enhance LLL adoption (Singh and Singh, 2020; Malhi et al., 2021). The adoption of climate-resilient varieties was significantly influenced by education, climate change awareness, seed availability, distance from the capital, distance from the state/central agriculture university/institute, and distance from the KVKs, promotional activities in the villages, training received, and understanding of different CRATs. The knowledge about climate-resilient concepts and the availability of different varieties plays a crucial role, with education being a pivotal factor reflected in the results (Figures 6–8).
Despite the lack of significant statistical relationships between different factors and crop diversification, positive trends, especially for fear of non-availability of market and fear of yield loss, suggest potential areas for targeted interventions. Reducing market and yield loss fears, and improving land suitability, could enhance CD (crop diversification) adoption. The significant (85%) preference for diverse crops was most noticeable in soybean, followed by maize. Maize’s popularity stems largely from its compatibility with available land and market access (Wahab and Wahab, 2014; Felix and Ramappa, 2023). However, factors such as limited land holdings, wildlife impact, and farmers prioritizing subsistence significantly constrain overall diversification potential (Figure 6). Market conditions and these fundamental barriers create major obstacles to the broader acceptance of crops like millets, soybeans, and arhar (pigeon pea). Its (pigeon pea) faces challenges due to its longer growth duration despite offering nearly three times the minimum support price (MSP) compared to rice crops.
Land suitability is a significant barrier to adopting a potato-based cropping system, with storage facilities being another overlooked concern (Manjunath and Salakinkop, 2017; Hamba et al., 2024). Under waterlogged conditions, soybean encounters various limitations, and millets face serious obstacles due to a lack of technical knowledge. Additionally, challenges like late wilt in maize and fall armyworm infestations during the kharif season, and the accessibility of farmland from main roads for land preparation, complicate the adoption of maize. Jute can be a valuable component of CD (crop diversification), but its adoption is hindered by the availability of water channels for retting. Farmers claim lower labour requirements for intercultural operations in jute cultivation, but increased labour needs for post-harvest processing balance out any cost savings. Early rice planting using DSR and shorter-duration varieties can facilitate timely planting of subsequent crops, promoting crop diversification for the Rabi season (Figures 6–8). The adoption of SSNM was strongly influenced by soil testing awareness, age, education, soil health awareness, nearest soil testing agency, and unwillingness due to high soil testing costs. Awareness of soil testing and the proximity of soil testing agencies are crucial for SSNM adoption. Farmers’ understanding of soil health and the willingness to invest in soil testing despite the costs play significant roles in the successful implementation of SSNM practices (Reddy, 2019). Despite a host of interventions, scientific nutrient management practices remain very low due to the lack of awareness and practical problems. The Soil Health Card (SHC) Scheme has improved farmers’ nutrient management knowledge but faces implementation challenges. Financial support and awareness initiatives can enhance adoption (Mwangi and Kariuki, 2015).
The major barriers to understanding and following the crop calendar are traditional cultivation practices and stubborn mono-cropping among farmers. Late sowing of rice limits the provision of other crops like legumes and pulses within the cropping system. Farmers who understand the importance of timely sowing are typically high-yield wheat growers (95% among users showed this trend). Timely seed availability also plays an important role in adopting timely sowing operations. Overcoming these barriers requires a focus on educating farmers about the benefits of crop calendar adherence and ensuring timely seed distribution (Tesfaye et al., 2017). The adoption of DSR technology has always been a focal point of discussion among policymakers, with a persistent question of why farmers are not adopting it. The major reasons identified in this study include a lack of knowledge about the technology and fear of yield loss. Farmers often perceive yield loss based on visible outcomes without considering the overall cost of cultivation. Training programs that address these fears and educate farmers about the economic benefits of DSR can significantly improve adoption rates. Secondary issues like weed pressure and crop vigor complaints also need to be addressed through targeted interventions (Prakash, 2024). If you see the distribution of factors as per their importance in overall adoption of CRATs, you will find most factors lies on the technical side (Figures 6–8).
4.2 Social factors
Social factors such as age, education, and community engagement through promotional activities and training emerged as significant influencers of CRATs adoption (Figures 6–8). The positive correlation between training received and the adoption of interventions like ZTMT, LLL, CRV, CD, SSNM, CCTS, and DSR underscores the importance of continuous education and knowledge dissemination. For instance, the coefficient for training received in DSR adoption was 0.410, indicated that training programs could substantially enhance adoption rates. Training received and soil health awareness were significant social factors influencing the adoption of ZTMT (Figure 7). For LLL, community engagement through promotional activities and training was significant. Education and climate change awareness were key social factors for CRV selection (Patel et al., 2020; Sharma et al., 2020). Crop diversification was influenced by training received and community engagement. Social factor like age and education played a role in SSNM adoption. The adoption of crop calendar and timely sowing was significantly influenced by training received and promotional activities. For DSR, technology awareness and training received were crucial social factor (Singh and Singh, 2020; Tesfaye et al., 2017; Gupta et al., 2018).
4.3 Technical factors
Technical factors such as the availability of technology, understanding of different CRATs, and proximity to agricultural support services by KVKs were critical in influencing adoption. The positive correlation between technology awareness and DSR adoption (coefficient = 0.400) highlights the importance of making relevant technologies accessible to farmers. Similarly, proximity to KVKs was a significant factor across multiple interventions, including LLL, CRV, and SSNM. The availability of ZTMT machinery and understanding of CRATs were significant technical factors for ZTMT. Proximity to KVKs and technical support were essential for the adoption of LLL (Mehta et al., 2020). Seed availability and understanding of CRATs were crucial for CRV selection. Technical factors such as suitability of land for alternate crops and availability of irrigation influenced crop diversification. Soil testing awareness and proximity to soil testing agencies were significant for SSNM adoption (Figures 6–8).
The understanding of crop calendars and proximity to KVKs were essential technical factors for CCTS. The availability of DSR technology and understanding of CRATs were crucial for DSR adoption. Farmers’ knowledge about specific practices can significantly impact their willingness to adopt these practices (Mwangi and Kariuki, 2015).
4.4 Monetary elements
Monetary elements such as initial investment costs, financial incentives, and the economic viability of CRATs were major barriers to adoption (Figure 6). The fear of yield loss and high initial investment costs were particularly significant for interventions like ZTMT, CD and LL. For example, the coefficient for Initial Investment on ZTMT/LL was 0.400, indicated that financial constraints are a substantial barrier to adoption. High initial investment costs and fear of yield loss were significant monetary barriers for ZTMT (Figures 6–8). The high cost of LLL machine was a significant barrier for LLL (Mehta et al., 2020). Financial elements such as seed availability and economic incentives played a role in CRV adoption. The fear of yield loss and fear of non-availability of markets were significant barriers for CD (crop diversification).
The cost of soil testing and availability of financial support were essential monetary factors for SSNM. Financial support for understanding and implementing crop calendars was crucial for CCTS (Singh et al., 2022). Creating awareness among farmers about the cost of cultivation and its associated benefits will be key in the adoption of these technologies. Many farmers currently believe that their profit is solely linked to the crop yield, rather than considering the cost of cultivation (Boufous et al., 2023; Patel et al., 2020). The cost of technology and financial support were significant monetary for DSR. Financial incentives, such as subsidies and low-interest loans, could alleviate these barriers. Policies aimed at reducing the cost burden on farmers and providing financial support for the adoption of CRATs are essential (Reddy et al., 2018; Malhi et al., 2021; Kumar et al., 2019).
5 Future prospects
Identifying and addressing key focus areas is essential for enhancing the adoption of CRATs (Figures 6–8). Our study highlights several critical areas that warrant further research and intervention.
• Increasing awareness and providing training are fundamental. Future studies should explore innovative methods to disseminate knowledge and train farmers, especially for interventions like ZTMT and DSR, where training and awareness have shown significant impact.
• Providing technical support and ensuring access to resources are crucial. Research should focus on developing and implementing strategies to improve access to necessary machinery, seeds, and technical know-how, as evidenced by the significant role of technical support in interventions like LLL, CRV, and SSNM.
• Financial incentives and support are vital.
Future research should investigate economic models and policies that can address high initial costs and provide economic incentives, which are particularly beneficial for interventions like ZTMT and LLL. Finally, promoting community engagement and leveraging social networks can enhance adoption. Studies should explore community-based approaches and the role of social networks in disseminating information and encouraging adoption. Policymakers and extension services should prioritize these areas to enhance the adoption of CRATs, thereby improving agricultural sustainability and resilience (Joshi et al., 2021; Goyal et al., 2016; Prakash, 2024; Malhi et al., 2021).
6 Conclusion
The study underscores the intricate interplay of social, technical, and financial factors shaping the adoption of Climate-Resilient Agricultural Technologies (CRATs) in EIGP-Bihar, India. Addressing these multifaceted challenges through targeted interventions can accelerate the transition toward sustainable and climate-resilient farming systems. Through a detailed analysis of interventions such as zero tillage and minimum tillage (ZTMT), laser land levelling (LLL), climate resilient variety selection (CRV), crop diversification (CD), site-specific nutrient management (SSNM), crop calendar and timely sowing (CCTS), and direct seeded rice (DSR), several critical insights have emerged. Descriptive statistics revealed moderate levels of soil health awareness and climate change awareness, indicated a significant scope for improvement through educational programs. For instance, soil health awareness had a mean value of 2.7, reflecting a need for enhanced awareness initiatives. Correlation analysis showed that social factors like ‘training received’ had a strong positive correlation with the adoption of DSR (correlation coefficient = 0.410), emphasizing the importance of continuous farmer education and community engagement. Technical factors such as the availability of technology and proximity to KVKs were also critical. For example, the logistic regression results indicated that technology awareness significantly influenced DSR adoption, with a coefficient of 0.400. This underscores the necessity of making relevant technologies accessible to farmers.
Similarly, monetary factors like high initial investment costs were substantial barriers to adoption for interventions like ZTMT and LLL. The coefficient for initial investment on ZTMT/LLL was 0.400, highlighting the financial constraints faced by farmers. The positive trends observed for various factors across different interventions suggest that targeted interventions can significantly enhance CRATs adoption. For instance, promoting community-based training programs and improving access to technical resources could drive higher adoption rates. Financial incentives such as subsidies and low-interest loans could mitigate the monetary barriers, facilitating broader implementation of these practices. Overall, the study underscores the need for a multi-faceted approach to promote the adoption of CRATs.
Policymakers and extension services should focus on enhancing awareness, providing technical support, and offering financial incentives to address the diverse barriers faced by farmers. By adopting these strategies, it is possible to achieve sustainable agricultural development and improve the resilience of farming systems in Bihar. Future research should aim to validate these findings on a larger scale and explore the dynamic interactions between different factors influencing CRATs adoption (Figure 9).
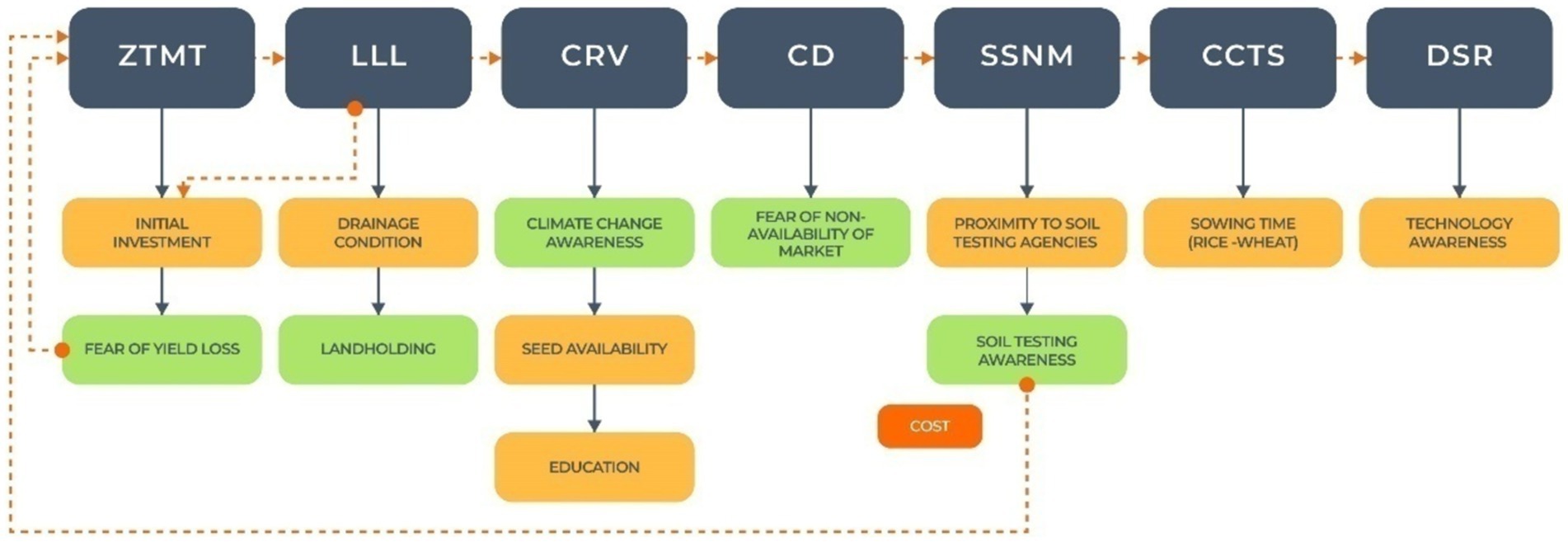
Figure 9. The key factors affecting adoption of climate smart agriculture practice (Red dotted arrows: The important factors associated with indirect linkage, Black arrows: Factors with direct linkage, Orange: secondary important, Green: first important), Cost: Psuedo factor behind SSNM.
Data availability statement
The raw data supporting the conclusions of this article will be made available by the authors, without undue reservation.
Ethics statement
Written informed consent was obtained from the individual(s) for the publication of any potentially identifiable images or data included in this article.
Author contributions
RJa: Conceptualization, Supervision, Writing – review & editing. VM: Conceptualization, Software, Supervision, Writing – original draft. SD: Software, Writing – original draft. RS: Investigation, Writing – review & editing. RJh: Methodology, Project administration, Writing – review & editing. AbK: Methodology, Writing – review & editing. UK: Methodology, Writing – review & editing. AnK: Methodology, Writing – review & editing. RNS: Investigation, Writing – review & editing. SuK: Conceptualization, Writing – review & editing. IR: Methodology, Writing – review & editing. SP: Data curation, Project administration, Writing – original draft. RK: Investigation, Writing – review & editing. SM: Formal analysis, Writing – original draft, Writing – review & editing. VP: Investigation, Writing – review & editing. SaK: Investigation, Writing – review & editing. AM: Methodology, Writing – review & editing. BK: Data curation, Writing – review & editing. UU: Data curation, Writing – review & editing. RanS: Methodology, Writing – review & editing. RC: Methodology, Writing – review & editing. VikK: Methodology, Writing – review & editing. MK: Conceptualization, Writing – review & editing. VinK: Data curation, Writing – review & editing. KS: Investigation, Writing – review & editing. SusS: Methodology, Writing – review & editing. RamS: Investigation, Writing – review & editing. SeK: Methodology, Writing – review & editing. KS: Data curation, Writing – review & editing. GK: Data curation, Writing – review & editing. RT: Conceptualization, Software, Writing – review & editing. VineK: Investigation, Writing – review & editing. SunK: Investigation, Writing – review & editing. SD: Investigation, Writing – review & editing. PG: Methodology, Writing – review & editing. NA: Methodology, Writing – review & editing. ShaK: Methodology, Writing – review & editing. SanK: Formal analysis, Writing – review & editing. BU: Formal analysis, Writing – review & editing. SumS: Formal analysis, Writing – review & editing. SG: Conceptualization, Writing – review & editing. SB: Methodology, Writing – review & editing. AL: Data curation, Writing – review & editing.
Funding
The author(s) declare that financial support was received for the research, authorship, and/or publication of this article. We acknowledge the financial and technical support from Government of Bihar for initiating (CRA Program) for funds and technical support. Indian Council of Agricultural Research (ICAR), CGIAR and all donors who supported this research through their contributions are highly acknowledged.
Acknowledgments
We acknowledge the farmers for providing their time, resources, sharing their experiences and expertise. All the field technicians are highly acknowledged.
Conflict of interest
The authors declare that the research was conducted in the absence of any commercial or financial relationships that could be construed as a potential conflict of interest.
The author(s) declared that they were an editorial board member of Frontiers, at the time of submission. This had no impact on the peer review process and the final decision.
Publisher’s note
All claims expressed in this article are solely those of the authors and do not necessarily represent those of their affiliated organizations, or those of the publisher, the editors and the reviewers. Any product that may be evaluated in this article, or claim that may be made by its manufacturer, is not guaranteed or endorsed by the publisher.
Supplementary material
The Supplementary material for this article can be found online at: https://www.frontiersin.org/articles/10.3389/fsufs.2024.1504388/full#supplementary-material
References
Abeydeera, L., Pathirana, A., Veer, D., and Mahadewa, D. (2019). Rising CO2 emissions and global warming. Environ. Sci. Technol. 11, 3972. doi: 10.3390/su11143972
Aggarwal, P. K., Jain, S., and Pathak, H. (2020). Climate-smart technologies and practices for Indian agriculture. Agric. Syst. 178:102736. doi: 10.1016/j.agsy.2019.102736
Arora, R., Sharma, P., and Singh, V. (2005). Projections of future climate in India. Indian journal of climate Studies.
Boufous, S., Hudson, D., and Carpio, C. (2023). Farmers’ willingness to adopt sustainable agricultural practices: a meta-analysis. PLoS Sustain. Transform. 2:e0000037. doi: 10.1371/journal.pstr.0000037
Chaudhary, A., Sharma, P., and Kumar, R. (2019). Technical barriers to the adoption of climate-resilient agriculture. J. Agric. Sci. 11, 123–136.
Choudhary, A. K., Jha, R., and Kumar, M. (2021). Institutional frameworks for enhancing the adoption of CRATs: evidence from Bihar. J. Agric. Policy Dev. 9, 203–217.
Choudhary, A. K., Meena, V. S., and Kumar, S. (2022). Climate-resilient strategies for degraded soils of Bihar's agro-climatic zones. Soil Clim. Res. J. 9, 29–41.
Das, P., Chaudhary, S., and Singh, V. (2020). Sub-zone-specific strategies for water management in South Bihar Alluvial Plains. J. Water Resour. Agric. 7, 65–79.
Felix, D., and Ramappa, K. B. (2023). Trends in crop diversification. J. Agric. Econ. 10:78. doi: 10.1057/s41599-023-02078-y
Ghosh, A., Roy, P., and Mishra, V. (2018). Climate change impact on agriculture: a review of EIGP-specific challenges and adaptation. Clim. Agric. 7, 112–125.
Goyal, S. K., Rai, J. P., and Ram Singh, S. (2016). Indian agriculture and farmers-problems and reforms.
Gupta, R., Singh, V., and Verma, S. (2018). Social influencing the adoption of climate-resilient agriculture. Int. J. Agric. Ext. 6, 45–58.
Hamba, S., Patel, K., and Reddy, S. (2024). Agricultural challenges and opportunities in Bihar. International. J. Agric. 15, 102–116.
IPCC (2014). “Climate change 2014: synthesis report” in Contribution of working groups I, II and III to the fifth assessment report of the intergovernmental panel on climate change. eds. R. K. Pachauri and L. A. Meyer (Geneva, Switzerland: IPCC), 151.
Jat, M. L., Gupta, R., and Dagar, J. C. (2019). Innovations in conservation agriculture for climate resilience: case studies from the indo-Gangetic Plains. Sustain. Agric. Rev. 36, 45–67.
Jha, R. K., Das, P., and Kumar, A. (2022). Enhancing farmer capacity through targeted CRAT interventions: a review of Bihar's progress. Indian J. Agric. Exten. 6, 98–107.
Joshi, P., Mehta, S., and Patel, H. (2021). Financial incentives for climate-resilient agriculture. Agric. Econ. Rev. 12, 89–102.
Kumar, R., Jha, A. K., and Singh, P. (2019). Rainfall variability and cropping systems in the north-east Alluvial Plains of Bihar. Clim. Agroecosyst. 11, 97–108.
Kumar, N., Sharma, M., and Singh, A. (2019). The role of awareness in climate-resilient agriculture adoption. J. Environ. Manag. 15, 67–81.
Kumar, R., Singh, V., and Pandey, A. (2020). Assessing the vulnerability of eastern indo-Gangetic Plains to climatic extremes: a synthesis of evidence. J. Clim. Agric. Sustain. 12, 234–248.
Malhi, G. S., Kaur, M., and Kaushik, P. (2021). Impact of climate change on agriculture and its mitigation strategies: a review. Sustain. For. 13:1318. doi: 10.3390/su13031318
Manjunath, R., and Salakinkop, S. R. (2017). Adoption of potato-based cropping systems. J. Crop. Sci. 52, 67–79.
Meena, B. L., Bisht, J. K., and Poonia, S. K. (2021). Site-specific nutrient management: a pathway to improving productivity and resilience in the IGPs. Nutr. Cycl. Agroecosyst. 89, 345–360.
Mehta, S., Verma, P., and Reddy, S. (2020). Proximity to agricultural institutions and adoption of climate-resilient agriculture. J. Rural. Stud. 18, 56–70.
Mishra, R., Sharma, V., and Das, A. (2020). Annual flooding in the north-west Alluvial Plains of Bihar: impact on cropping systems. Environ. Manag. Agric. 5, 135–146.
Mwangi, M. N., and Kariuki, S. (2015). Determining adoption of new agricultural technology by smallholder farmers in developing countries.
Pachauri, R. K., and Meyer, L. A. (Eds.). (2014). Climate change 2014: Synthesis report; contribution of working groups I, II and III to the Fifth Assessment Report of the Intergovernmental Panel on Climate Change. IPCC: Geneva, Switzerland.
Patel, H., Chaudhary, A., and Joshi, P. (2020). Financial barriers to climate-resilient agriculture. Econ. Polit. Wkly. 55, 78–90.
Patel, K., Singh, R., and Sharma, A. (2021). Leveraging social networks for climate-resilient agriculture. Soc. Sci. J. 23, 99–115.
Pingali, P., Aiyar, A., Abraham, M., and Rahman, A. (2019). Transforming food systems for a rising India. Cham, Switzerland: Palgrave Macmillan.
Prakash, S. (2024). Impact and assessment of climate change on agricultural production in India: a geographical perspective.
Prakash, V., Gupta, R., and Kumar, N. (2021). Influencing the adoption of climate-resilient agriculture. J. Sustain. Agric. 33, 45–59.
Ravindra, K., Mehta, S., and Patel, H. (2024). Climate change and food security in India. Indian. J. Agric. Res. 58, 1131–1137.
Ray, P., Singh, R. K., and Sharma, S. (2018). Agro-climatic zone-specific interventions for sustainable agriculture in Bihar. Indian J. Agric. Res. 52, 245–259.
Reddy, A. A. (2019). The soil health card scheme in India: lessons learned and challenges for replication in other developing countries. J. Nat. Resour. Policy Res. 9, 124–156. doi: 10.5325/naturesopolirese.9.2.0124
Reddy, S., Mehta, S., and Kumar, R. (2018). Initial investments and adoption of climate-resilient agriculture. Agric. Finan. Rev. 78, 222–236.
Reddy, A. A., and Reddy, G. P. (2022). Economic impact of organic agriculture: evidence from a Pan-India survey. Sustain. For. 14:15057. doi: 10.3390/su142215057
Sahni, S., Gupta, R., and Pandey, S. K. (2021). Soil and climatic diversity in Bihar: implications for agricultural planning. J. Soil Water Manag. 38, 15–28.
Sathaye, J., Singh, V., and Patil, S. (2006). CO2 emissions in global agriculture. Environ. Manag. 38, 872–885.
Sati, M. C., Pathak, R., and Singh, A. (2018). Impact of urbanization on climate. Urban climate. Review. 24, 954–965.
Sharma, N. K., Singh, R. K., and Kumar, P. (2020). The role of climate-resilient varieties in stabilizing yields under varying agro-climatic conditions. Crop Sci. Int. 15, 87–101.
Sharma, R., Singh, V., and Patel, K. (2020). Trends in the adoption of climate-resilient agriculture. Glob. Environ. Chang. 25, 105–118.
Singh, R., Kumar, S., and Chauhan, A. (2022). Overcoming barriers to the adoption of climate-resilient agriculture in Bihar. Agric. Transform. J. 18, 79–95.
Singh, A., and Sharma, P. (2020). Training and adoption of climate-resilient agriculture. Int. J. Agric. Res. 8, 150–165.
Singh, K. M., and Singh, P. (2020). Challenges of ensuring food and nutritional security in Bihar. SSRN Electron. J. 12, 45–60.
Tesfaye, K., Aggarwal, P. K., Mequanint, F., Shirsath, P. B., Stirling, C. M., Khatri-Chhetri, A., et al. (2017). Climate variability and change in Bihar, India: challenges and opportunities for sustainable crop production. Sustain. For. 9:1998. doi: 10.3390/su9111998
Verma, S., Patel, H., and Chaudhary, A. (2019). Technical in the adoption of climate-resilient agriculture. Agric. Syst. 11, 112–126.
Keywords: climate-resilient agriculture, minimum tillage, laser land levelling, crop diversification, site-specific nutrient management, direct seeded rice, adoption
Citation: Jat RK, Meena VS, Durgude S, Sohane RK, Jha RK, Kumar A, Kumar U, Kumar A, Singh RN, Kumar S, Reddy IR, Pazhanisamy S, Kumar R, Meena SK, Prakash V, Kumar S, Mukherjee A, Kumar B, Umesh UN, Singh RK, Chaubey R, Kumar V, Kumar M, Kumar V, Sharda K, Singh S, Singh RK, Kumari S, Singh KP, Kumar G, Tiwari RK, Kashyap V, Kushwaha S, Das S, Gautam PP, Alam NM, Kumar S, Kumar S, Upadhaya B, Singh SK, Ghosh S, Bhagat S and Lenka AK (2025) Bridging the gap: challenges and adoption of climate-resilient agriculture technologies in agricultural landscapes across agro-climatic zones of Bihar, India. Front. Sustain. Food Syst. 8:1504388. doi: 10.3389/fsufs.2024.1504388
Edited by:
Fuyou Guo, Qufu Normal University, ChinaReviewed by:
A. Amarender Reddy, National Institute of Agricultural Extension Management (MANAGE), IndiaPriya Priyadarshini, Banaras Hindu University, India
Copyright © 2025 Jat, Meena, Durgude, Sohane, Jha, Kumar, Kumar, Kumar, Singh, Kumar, Reddy, Pazhanisamy, Kumar, Meena, Prakash, Kumar, Mukherjee, Kumar, Umesh, Singh, Chaubey, Kumar, Kumar, Kumar, Sharda, Singh, Singh, Kumari, Singh, Kumar, Tiwari, Kashyap, Kushwaha, Das, Gautam, Alam, Kumar, Kumar, Upadhaya, Singh, Ghosh, Bhagat and Lenka. This is an open-access article distributed under the terms of the Creative Commons Attribution License (CC BY). The use, distribution or reproduction in other forums is permitted, provided the original author(s) and the copyright owner(s) are credited and that the original publication in this journal is cited, in accordance with accepted academic practice. No use, distribution or reproduction is permitted which does not comply with these terms.
*Correspondence: Vijay Singh Meena, dmlqYXlzc2FjLmJodUBnbWFpbC5jb20=; dmlqYXkubWVlbmFAaWNhci5nb3YuaW4=; Sunita Kumari Meena, c3VtZWVuYTY2QGdtYWlsLmNvbQ==