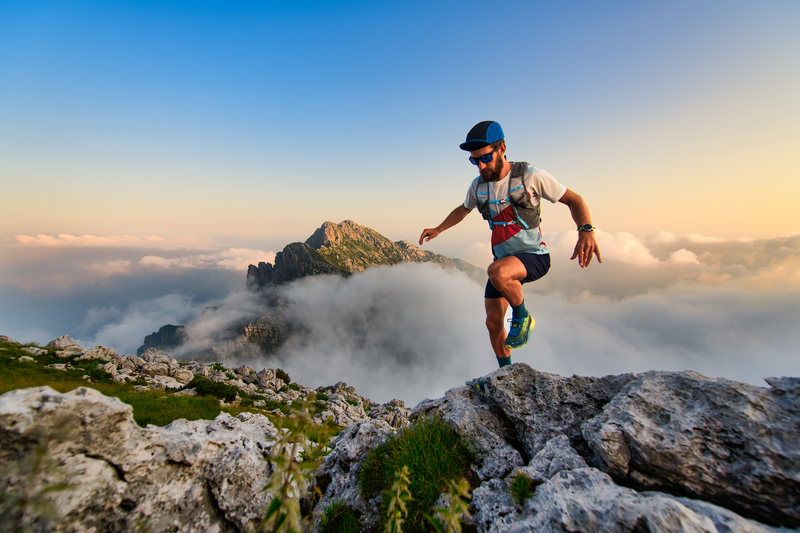
94% of researchers rate our articles as excellent or good
Learn more about the work of our research integrity team to safeguard the quality of each article we publish.
Find out more
ORIGINAL RESEARCH article
Front. Sustain. Food Syst. , 06 January 2025
Sec. Agricultural and Food Economics
Volume 8 - 2024 | https://doi.org/10.3389/fsufs.2024.1503951
This article is part of the Research Topic Environmental Resilience and Sustainable Agri-food System Management View all 11 articles
This study explores the impact of off-farm employment on the adoption of Integrated Pest Management (IPM) technology among farmers in rural China, with a focus on sustainable farm development. Utilizing micro-survey data and applying an endogenous switching Probit model within a counterfactual analysis framework, the research examines how off-farm employment influences the likelihood of adopting IPM technology. The findings reveal that 67.84% of farmers have adopted IPM technology, while 32.16% have not. The counterfactual analysis indicates that if farmers with off-farm employment did not engage in off-farm activities, their probability of adopting IPM technology would decrease by 71.57%. Conversely, if farmers without off-farm employment were to engage in off-farm activities, their probability of adopting IPM technology would increase by 76.95%. Key factors influencing off-farm employment include age, education level, number of elderly in the household, membership in a cooperative, number of land plots, village topography, and the number of family members and relatives working in government or public institutions. In addition, age, personal health status, number of household laborers, membership in a cooperative, number of land plots, village topography, and ecological cognition significantly affect the adoption of IPM technology. The study suggests that optimizing industrial structure, improving the rural employment market, enhancing the role of farmers’ professional cooperatives, strengthening education and training efforts, and increasing publicity efforts can collectively improve the adoption of IPM technology. These strategies contribute to sustainable farm development and better environmental outcomes.
Food security is a critical issue for governments and agricultural sectors worldwide. According to data from the Food and Agriculture Organization (FAO, 2020), approximately 820 million people globally face the challenge of undernutrition. Therefore, ensuring the stability and quality of food supply is essential for maintaining social stability and promoting the well-being of people. However, recurring pests and diseases have led to continuous crop yield reductions, posing a severe threat to food security (Savary et al., 2019). Each year, up to 40% of global crop yields are lost due to pest damage, amounting to losses exceeding $220 billion (Singh et al., 2023). In China, the area affected by pests is estimated to be 315 million acres, a 24% increase compared to the previous year, with an anticipated yield loss risk exceeding 36 billion pounds.
Chemical pesticides can effectively control the number of pests, pathogens, and weeds, reducing crop losses caused by pests and diseases and increasing crop yields (Hao and Zheng, 2017; Wang et al., 2015; Zhang et al., 2015a). Due to their convenience, specific efficacy, and economic benefits, chemical pesticides have become the primary method for controlling pests in agricultural production (Schreinemachers and Tipraqsa, 2012; Zhang et al., 2020).
To protect crops from pests and increase food production, a large amount of chemical pesticides has been used. According to FAO (2020) data, the global pesticide usage in 2020 was 2.66 million tons, with China’s agricultural pesticide usage at 258,000 tons, accounting for about 8% of global usage. However, long-term excessive use of chemical pesticides has led to environmental pollution, ecosystem degradation, and biodiversity loss (Zhang et al., 2015b; Nie et al., 2016; Rodrigues et al., 2018; Liu et al., 2018). Residues from chemical pesticides can enter soil, water bodies, and air, posing potential threats to ecosystems and possibly having adverse effects on human health (Mostafalou and Abdollahi, 2017; Zhang et al., 2018; Varjani et al., 2019; Wang et al., 2022). The long-term excessive use of the same type of chemical pesticides can lead to the development of resistance in pests or pathogens, causing them to rapidly reproduce in the environment and exacerbating pest problems (Bass and Jones, 2018). This not only increases the economic burden on farmers but also threatens food security. Hence, reducing the over-reliance on chemical pesticides and implementing sustainable agricultural development strategies has become a consensus among countries.
To reduce the adverse effects of chemical pesticides, many countries have adopted more sustainable and environmentally friendly innovative pest management technologies. Among these, Integrated Pest Management (IPM) technology has been widely utilized as an environmentally sustainable and socio-economically viable approach to address pests, diseases, and weeds (Sadique et al., 2018). IPM employs biological and physical methods for pest control, including strategies like biological pest control, ecological control, and the use of traps such as pheromone traps, yellow sticky traps, and infected site removal. It also includes the application of soil conditioners, pest-resistant crop varieties, biopesticides, and beneficial insects (Mian et al., 2016).
From an environmental perspective, IPM can protect soil and water resources, safeguard wildlife, enhance crop resilience, and promote ecosystem health (Fernandez-Cornejo, 1998; Pretty and Bharucha, 2015; Fahad et al., 2018). Economically, IPM is regarded as a cost-effective pest management strategy (Grasswitz, 2019), as it reduces pesticide application costs and improves the quality and marketability of agricultural products, enabling higher prices and monetary returns. It thus offers multiple advantages such as being environmentally friendly and economically beneficial (Yaguana et al., 2016; Gautam et al., 2017).
Consequently, IPM technology not only maintains stable crop yields while significantly reducing chemical pesticide usage but also minimizes implementation costs, reducing farmers’ agricultural production expenditures. Ultimately, it delivers substantial economic, social, and environmental benefits to farmers and provides a crucial material and technical foundation for sustainable agricultural development, environmental protection, and food safety.
To promote sustainable agricultural development and the adoption of IPM technology, the Chinese government has introduced a series of related policies. Government departments have established pest warning and control stations to monitor and forecast pest outbreaks and have set up demonstration sites for major pest control technologies in multiple regions. While the implementation of these policies has encouraged farmers to adopt pest control technologies, the actual adoption rate of IPM technology among farmers remains low (Otieno et al., 2023). In 2021, the coverage rate of green pest control for major crops in China was only 46%. Effectively optimizing the promotion and application of IPM technology in China is an urgent and important issue.
The successful implementation of Integrated Pest Management (IPM) technology cannot rely solely on government participation, as farmers are the primary decision-makers and implementers of this technology (Tian et al., 2022). Existing literature highlights several intrinsic factors influencing farmers’ adoption of IPM. From a policy perspective, Kaiser et al. (2010) conducted a case study in the grasslands of Brandenburg, Germany, demonstrating that subsidy policies can significantly improve farmers’ adoption of IPM practices. Similarly, Gautam et al. (2017) found through propensity score matching and inverse probability weighting that trained farmers gained better knowledge of pests and pesticides, adopted more IPM practices, and reduced the frequency of spraying and mixing different pesticides. From an individual characteristic perspective, Korir et al. (2015) observed that better-educated family farms tend to be more aware of the importance of land tenure security and the potential benefits of IPM. Risk-tolerant farmers are more likely to try IPM and other sustainable agricultural production technologies (Deguine et al., 2021). However, women, compared to men, often have less access to key resources such as land, cash, and labor, making it more challenging for them to adopt IPM (De Groote and Coulibaly, 1998; Shrestha et al., 2024). Yaguana et al. (2016) noted that many small-scale farmers, due to lower education levels, are reluctant to adopt IPM because they cannot comprehend the complex interactions between pests and the knowledge-intensive nature of IPM. From a household perspective, larger farm sizes and greater household sizes are associated with a higher likelihood of adopting IPM technology (Ma and Abdulai, 2018). Additionally, factors such as a lack of ecological literacy (Horgan, 2017; Murray et al., 2021), farming habits (Parsa et al., 2014), risk aversion (Deguine et al., 2021), and constraints on access to information resources (Yaguana et al., 2016) further influence farmers’ adoption decisions regarding IPM technology.
Currently, there is a trend of increasing off-farm employment among farmers in agricultural production, with the proportion of full-time farmers decreasing and the proportion of farmers with off-farm employment rising. Off-farm employment has become the main form of agricultural operation in China (Zhao, 1999; Chen, 2019; Bai et al., 2022). In academic circles, the impact of off-farm employment on technology adoption is debated: some argue that off-farm employment hinders technology adoption because it reduces farmers’ ability to adapt to new agricultural technologies (Xin and Anlu, 2017) and lowers the quality of agricultural labor input (Yang et al., 2020). This leads to farmers avoiding the risks associated with new agricultural technologies and preferring short-term investments in increased pesticide use (Jieling et al., 2018). On the other hand, some believe that off-farm employment promotes technology adoption. The primary reason is that risk aversion and agricultural technology investment are significantly negatively correlated (Liu and Huang, 2013; Rezaei et al., 2019). Off-farm employment can increase household income, thereby enhancing their ability to withstand risks and promoting the adoption of green production technology.
Therefore, exploring the relationship between farmers’ off-farm employment and their adoption of Integrated Pest Management (IPM) technology can provide valuable insights for addressing the disconnect between the promotion of IPM technology and the low adoption rate among farmers, as well as for controlling pollution at its source in agricultural production. Distinguishing from previous studies, the main contributions of this paper are as follows: Early research on reducing chemical pesticide use and promoting IPM primarily focused on economic incentives, technological awareness, and farmer characteristics (Moss, 2019; Lee et al., 2019; Lefebvre et al., 2014; Lucchi and Benelli, 2018). However, there is limited empirical research on how the growing trend of off-farm employment among farmers influences the adoption of IPM technology. The contributions of this study are twofold: First, it examines whether off-farm employment influences farmers’ adoption of IPM technology. Second, it addresses potential systematic biases in existing research methods, which often use econometric models like Logit and Probit. These models may fail to account for the simultaneous influence of observable variables on both off-farm employment and IPM adoption behaviors.
Farmers’ production behavior is rational. To mitigate the uncertainty risks in agricultural production and ensure survival, farmers choose production methods that align with their resources and objectives. The induced innovation theory suggests that resource scarcity, which affects the relative prices of factors, induces technological change (Hayami and Ruttan, 1985; Yujiro and Hang, 2004). In a market economy, farmers are influenced by changes in factor prices to seek technologies that can substitute increasingly scarce production factors and apply techniques that conserve relatively scarce resources (Liu and Shumway, 2006). When a particular labor endowment becomes scarcer compared to a capital endowment, the specific relative prices of these factors guide producers to adopt technologies that save labor and increase capital input. Producers, aiming for profit maximization, choose such biased technological changes to replace scarcer (and more expensive) factors with more abundant (and cheaper) ones to lower production costs.
Part-time farmers are defined as households where labor is engaged in both agricultural and non-agricultural work (Hao et al., 2013). As part-time farmers increasingly take up non-agricultural work, the allocation of production factors in agricultural production undergoes significant changes. On one hand, off-farm employment allows farmers to earn wage income. When farmers enter the non-agricultural sector, their income rises significantly, with wage income surpassing pure agricultural income and becoming the main source of income growth. This leads to an increase in the opportunity cost of farming and raises the relative price of agricultural labor. On the other hand, off-farm employment reduces the amount of agricultural labor input in farming households, making labor a scarce resource in agricultural production. Rising labor costs, in particular, encourage farmers to adopt technologically intensive production methods. As a technologically intensive agricultural technique (Kai et al., 2023), IPM technology integrates ecological regulation techniques, modern biotechnology, agricultural control, biological control, physical and chemical trapping techniques, and rational chemical control to build an “economical, simple, and effective” ecological engineering technology system. Through targeted interventions, the comprehensive control techniques can more effectively address specific pest problems, reduce reliance on external control measures, decrease indiscriminate pesticide application, and lower labor demands. Unlike traditional chemical pesticides, which often require frequent, large-scale spraying with little precision, IPM methods such as pheromone traps, biological agents, and precise spraying techniques minimize labor-intensive tasks associated with blanket chemical applications. Although the initial adoption of IPM may require higher upfront investment in training and tools, the overall costs are reduced through decreased reliance on chemical inputs and improved crop resilience.
Paudel et al. (2020) provided empirical evidence from Nepal, where they compared IPM and traditional farming techniques across four locations (Lalitpur, Banke, Surkhet, and Dadeldhura). Their study demonstrated that in crops such as tomatoes, cucumbers, and bitter gourds, adopting IPM technology not only reduced labor input and pest infestation but also required fewer pesticide applications, highlighting its efficiency in addressing labor and pest management challenges. In addition, off-farm employment increases household income, alleviating financial constraints in agricultural production and enabling the purchase of more agricultural inputs. Therefore, as farmers engage in off-farm work, they are inclined to adopt IPM technology. Based on the above analysis, the following hypothesis is proposed:
H1: Off-farm employment promotes farmers’ adoption of Integrated Pest Management technology.
Non-agricultural income increases household income, alleviating the financial limitations faced in agricultural production and providing funds for productive investment. However, the financial investment required for implementing Integrated Pest Management (IPM) technology is relatively low compared to the total agricultural expenditure. Thus, the effect of increased non-agricultural income is influenced by expected returns. Rational behavior theory suggests that farmers’ actions are influenced by expected returns, which in turn are affected by their cognitive level. Ecological cognition, an important part of farmers’ understanding, involves basic awareness of the ecological environment, the direct and indirect use values of ecosystem services, and understanding and grasping environmental protection policies and ecological scientific knowledge. It encompasses the perception and understanding of ecological phenomena and the quality of the ecological environment (Yuriev et al., 2020; Ren et al., 2022).
Cognitive behavior theory posits that cognition forms the basis of behavior, with individual preferences shaped by their understanding, ultimately influencing their decision-making (Yazdanpanah et al., 2015). The bounded rationality hypothesis in behavioral economics suggests that due to limited ecological cognition and incomplete information, farmers’ adoption of green production behavior is constrained (Li and Jin, 2022). When farmers perceive that the benefits of environmental protection outweigh the costs, they are more likely to prefer ecological behaviors. Constantino et al. (2021) and Rezaei et al. (2019) assert that there is a positive relationship between ecological cognition and behavior, significantly impacting decision-makers’ actions. Mouron et al. (2006) found that ecological cognition helps farmers choose optimal pest management methods and organic farming strategies.
Ecological cognition represents farmers’ subjective understanding of agricultural production and the ecological environment, derived from their knowledge related to the ecological environment (Zhu and Wang, 2019). Off-farm employment can effectively expand farmers’ social networks, breaking through traditional networks based on kinship and informal rules, enhancing their sources of knowledge acquisition and broadening their perspective and ability to acquire information. This improves their understanding and application of new technology. In addition, increased income from off-farm employment leads to greater attention to personal health concerns and a higher demand for a good ecological environment, thereby enhancing their ecological cognition. This, in turn, promotes the adoption of IPM technology. Based on this analysis, the following hypothesis is proposed:
H2: Off-farm employment enhances farmers’ ecological cognition, promoting the adoption of Integrated Pest Management technology.
Since it is impossible to simultaneously observe the adoption behavior of a single farmer under both off-farm employment and non-off-farm employment conditions, we cannot directly evaluate the impact of off-farm employment on the adoption of Integrated Pest Management (IPM) technology. Therefore, this study constructs an endogenous switching Probit model and uses a counterfactual framework based on regression results to estimate the treatment effect of off-farm employment on the probability of adopting IPM technology.
First, off-farm employment is considered as the treatment variable ( ). If the farmer is engaged in off-farm employment, ; otherwise, . The equation representing whether a farmer is engaged in off-farm employment is given in Equation 1:
Next, we define the outcome equations for farmers’ adoption behavior of IPM technology under different conditions, as shown in Equation 2:
In this equation, the latent variables and represent the adoption behavior of farmers toward IPM technology under off-farm employment and non-off-farm employment conditions, respectively. These latent variables determine the observed binary state variables and are other variables affecting the adoption behavior of IPM technology, and and are the coefficients to be estimated.
The probability that a farmer engaged in off-farm employment adopts IPM technology, estimated using the normal distribution function, is:
The probability that a farmer engaged in off-farm employment does not adopt IPM technology is:
The probability that a farmer not engaged in off-farm employment adopts IPM technology is:
The probability that a farmer not engaged in off-farm employment does not adopt IPM technology is:
Thus, the average treatment effect of off-farm employment on the adoption of IPM technology ( ) is represented by the difference between Equations 3, 4 and is given in Equation 7:
The average treatment effect of non-off-farm employment on the adoption of IPM technology (ATU) is represented by the difference between Equations 5 and 6, and is given in Equation 8:
In summary, this study uses the average values of ATT and ATU to examine the average treatment effect of off-farm employment on farmers’ adoption behavior of IPM technology.
Based on the previous analysis, off-farm employment mainly influences farmers’ adoption of IPM technology through their ecological cognition. This study draws on Heckman et al.’s (2013) approach to quantify the mechanism of influence and validate the proposed mechanism. The model is set as follows Equations 9–11:
Integrated Pest Management (IPM) refers to a crop management strategy aimed at preventing economic losses caused by pests, diseases, and weeds by using all applicable technical measures or combinations thereof in a manner suited to the local ecological environment and species dynamics (Zhu, 2015). There are various types of IPM technologies. Based on the research of Zhu (2015), this study considers any farmer who has adopted any form of IPM technology to have adopted IPM. Farmers who have adopted IPM technology are assigned a value of 1, while those who have not are assigned a value of 0.
The key independent variable is whether the farmer has an off-farm job. Farmers with off-farm jobs are assigned a value of 1, and those without are assigned a value of 0.
From Table 1, it is evident that 25.89% of the farmers have off-farm jobs, while 74.11% do not. The proportion of farmers who have adopted IPM technology is 54.28%, and those who have not adopted it is 45.72%. The survey results indicate that more than half of the farmers have adopted IPM technology. Furthermore, the adoption rate of IPM technology is higher among farmers with off-farm jobs compared to those without. Conversely, the rate of not adopting IPM technology is lower among farmers with off-farm jobs than those without. This indicates that farmers with off-farm jobs are significantly more likely to adopt IPM technology.
To apply the endogenous switching model, it is necessary to include an exclusion restriction variable, which serves as an instrumental variable that affects the selection equation but not the outcome equation (Mustafa et al., 2023). In this study, the number of family members or relatives working in government or public institutions is chosen as the instrumental variable. The primary reason for this choice is that farmers engaged in off-farm employment need to obtain certain information, and having relatives working in government or public institutions can increase their likelihood of off-farm employment. However, this variable does not directly influence farmers’ adoption of Integrated Pest Management (IPM) technology. The adoption of IPM depends on the farmers’ knowledge and the potential benefits they can obtain, which are not directly enhanced by having relatives working in government institutions.
Theoretically, an effective instrumental variable must satisfy two basic requirements: relevance and exogeneity. In this study, the adoption of IPM technology is the dependent variable, while off-farm employment, the instrumental variable (number of relatives working in government or public institutions), and other control variables are the independent variables. Using a probit model regression, it was found that the coefficient of the instrumental variable (number of relatives working in government or public institutions) is −0.018, with a corresponding p-value of 0.685. This indicates that the number of relatives working in government or public institutions does not directly affect the adoption of IPM technology by farmers, thus satisfying the exogeneity requirement.
Additionally, when off-farm employment is the dependent variable, with the instrumental variable and other control variables as independent variables, the probit model regression shows that the coefficient of the instrumental variable is 0.3385, with a corresponding p-value of 0.000. This demonstrates that the number of relatives working in government or public institutions influences off-farm employment, satisfying the relevance requirement.
In summary, based on these empirical tests, there is no evidence suggesting that the instrumental variable is inappropriate. Therefore, the selected instrumental variable (number of relatives working in government or public institutions) is considered effective for this study.
Ecological cognition refers to farmers’ subjective understanding of agricultural production and the ecological environment. Based on the realities of agricultural production, this study distinguishes ecological cognition into two dimensions: awareness of ecological benefits and awareness of economic benefits. Drawing on the research methods of Meng and Si (2022) and Duan and Luo (2024), and considering the practical context of agricultural production, this study measures awareness of ecological benefits using the question: “Do you think IPM and other green production technologies are beneficial for environmental protection and air pollution prevention?” Responses to this question are measured on a 5-point Likert scale: 1 = Strongly disagree, 2 = Disagree, 3 = Neutral, 4 = Agree, 5 = Strongly agree. Awareness of economic benefits is measured using the question: “Do you think IPM and other green production technologies are beneficial for increasing your household’s production and income?” Responses to this question are measured on a 5-point scale based on perceived financial impact: 1 = Very detrimental, 2 = Somewhat detrimental, 3 = No impact, 4 = Somewhat beneficial, 5 = Very beneficial. This approach provides a more nuanced measurement of ecological cognition, tailoring the scales to the specific nature of each dimension. It aligns with established methodologies in the literature, ensuring robustness and comparability with existing studies.
Based on the questionnaire data, this study selects the following personal characteristics of the sample: gender, age, years of education, and health status. It also includes household characteristics such as the number of household laborers, annual household income, farming area (mu), number of land plots, and membership in a cooperative, as control variables (Table 2).
The data for this study were obtained from household surveys conducted by the research team in August 2020 in selected areas of Hubei Province, Guangxi Zhuang Autonomous Region, Guizhou Province, Henan Province, and Anhui Province. These five provinces were chosen because Henan and Anhui have yields significantly higher than the national average, Hubei’s yield is slightly above average, and Guangxi and Guizhou have yields far below the national average. Hubei, Anhui, and Henan are major production bases for grain, cotton, and oil crops in China, with high total agricultural output. Guangxi and Guizhou are rich in agricultural resources and feature diverse crop varieties. The selected locations encompass plains, mountainous regions, and hilly terrains, making the statistical results broadly representative and generalizable.
Our survey employed a multi-stage sampling procedure to select households. First, socio-demographic data were collected for all counties in these provinces. Based on this information, 13 representative counties were chosen across the five provinces. Similarly, 55 villages were selected from these counties. Finally, households were randomly chosen from each village.
A standardized questionnaire was used to collect data on integrated pest management (IPM) technology, production practices, agricultural information sources, land conditions, transportation, and socio-demographic characteristics. Two questions were directly related to the adoption of IPM technology. Farmers were first asked whether they had adopted IPM technology in their agricultural production. After excluding farmers with missing values for key variables, the dataset comprised 842 farmers. Among these, 385 had not adopted IPM technology, while 457 had adopted it. Additionally, 624 farmers were not engaged in off-farm employment, whereas 218 had experience with off-farm employment.
This section examines the impact of off-farm employment on farmers’ adoption of IPM technology, using off-farm employment as the core variable and IPM adoption behavior as the outcome variable. Models (1) and (2) are estimated using full information maximum likelihood estimation (FIML). The regression results are presented in Table 3.
Table 3. Simultaneous estimation results of off-farm employment decision and farmers’ adoption of integrated pest management (IPM) using full information maximum likelihood estimation (FIML).
Using off-farm employment as the core variable and IPM adoption behavior as the outcome variable, Models (1) and (2) were estimated using full information maximum likelihood estimation (FIML). The regression results are shown in Table 3.
As indicated in Table 3, the Wald chi-square value is significant at the 1% level, indicating that the overall model is highly significant. Additionally, the value of rho1 is significant at the 10% statistical level, suggesting that there are unobservable factors that simultaneously affect whether farmers engage in off-farm employment and whether they adopt IPM technology. This implies that the baseline regression model may have selection bias (Mustafa et al., 2023; Leng et al., 2024), thus validating the use of the endogenous switching model.
The results for control variables, while informative, are not the primary focus of this study and are discussed briefly to confirm model robustness. Farmers’ off-farm employment decreases with higher education levels, which differs from the findings of Lu et al. (2022), who suggested that education promotes off-farm activities. One explanation could be that better-educated farmers are more likely to pursue full-time non-agricultural jobs or operate as new agricultural entities, focusing on providing agricultural services rather than combining these with off-farm work. Similarly, age negatively impacts off-farm employment, consistent with Brosig et al. (2009), indicating that younger individuals are more likely to engage in such activities.
Cooperative membership positively influences off-farm employment, as cooperatives may reduce farming labor requirements, allowing more time for off-farm activities (Brosig et al., 2009). Farm size also has a positive impact, likely because larger landholdings encourage the use of agricultural machinery, reducing manual labor and freeing up time for off-farm employment. In contrast, the number of land plots negatively affects off-farm employment, as managing multiple plots increases labor demands and reduces time available for non-agricultural activities (Weng et al., 2017).
Finally, having relatives employed in government or public institutions positively influences off-farm employment, likely due to better access to non-agricultural job information and opportunities. This finding aligns with the broader understanding of how social networks facilitate off-farm employment decisions.
Household income positively influences farmers’ adoption of IPM technology, consistent with the findings of Ma and Abdulai (2018). Farmers with higher household income are more likely to adopt IPM, as greater financial resources make it easier to afford the costs associated with implementing advanced pest management strategies. This relationship holds true for both off-farm and non-off-farm employment groups, highlighting the role of income in enabling access to sustainable agricultural practices.
In the non-off-farm employment group, male farmers are more inclined to adopt IPM technology than female farmers, likely due to differences in access to resources and decision-making authority. Additionally, farmers with larger cultivated land areas are more likely to adopt IPM practices. Larger-scale operations may have greater incentives and capacity to invest in technologies that improve pest management and productivity, aligning with the findings of Creissen et al. (2021).
While these variables provide useful context, the primary focus remains on how off-farm employment enhances household income, which in turn facilitates the adoption of labor-saving and environmentally sustainable IPM technology.
Using Equations 7, 8, we estimate the treatment effect of off-farm employment on farmers’ adoption of IPM technology. The results are shown in Table 4. In Table 4, columns (a) and (b) correspond to Equations 3, 5, respectively, representing the behavior of off-farm farmers who have and have not adopted IPM technology. Columns (c) and (d) represent the “counterfactual” results, corresponding to Equations 4, 6.
Overall, off-farm employment has a positive impact on farmers’ adoption of IPM technology, significant at the 1% level. The estimated results for the Average Treatment Effect on the Treated (ATT) indicate that if off-farm farmers were not engaged in off-farm activities, their probability of adopting IPM technology would decrease by 18.21%. The Average Treatment Effect on the Untreated (ATU) results suggest that if non-off-farm farmers were to engage in off-farm activities, their probability of adopting IPM technology would increase by 8.56%. This further demonstrates that off-farm employment positively influences farmers’ adoption of IPM technology.
The previous study shows that off-farm employment positively impacts farmers’ adoption of IPM technology. However, the mechanisms through which off-farm employment influences this adoption need further investigation. According to the previous analysis, off-farm employment enhances farmers’ ecological cognition, promoting the adoption of IPM technology. Ecological cognition refers to farmers’ subjective understanding of agricultural production and the ecological environment, divided into ecological benefit perception and economic benefit perception.
Table 5 presents the results of the mediating effect analysis using stepwise regression. Regression 1 in Table 5 shows that off-farm employment significantly promotes farmers’ adoption of IPM technology, further validating the previous analysis. Regression 2 indicates that off-farm employment significantly increases farmers’ expected ecological benefits. Regression 3 shows that an increase in expected ecological benefits significantly promotes farmers’ adoption behavior, consistent with the previous hypothesis. Comparing the results of models (1) and (3), when the mediating variable is included, the effect coefficient of off-farm employment on farmers’ adoption of IPM technology decreases from 0.518 to 0.431. This indicates that the influence of off-farm employment on farmers’ adoption behavior is partially mediated by expected ecological benefits, validating the proposed mechanism. The proportion of the mediating effect is 13.88%, indicating that approximately 13.88% of the influence of off-farm employment on farmers’ adoption behavior is mediated by expected ecological benefits.
Table 5. Path analysis of the impact of off-farm employment on farmers’ adoption of integrated pest management (IPM) technology.
Regression 5 indicates that off-farm employment significantly increases farmers’ expected economic benefits. Regression 6 shows that an increase in expected economic benefits significantly promotes farmers’ adoption behavior, consistent with the previous hypothesis. Comparing the results of models (4) and (6), when the mediating variable is included, the effect coefficient of off-farm employment on farmers’ adoption of IPM technology decreases from 0.518 to 0.41. This indicates that the influence of off-farm employment on farmers’ adoption behavior is partially mediated by expected economic benefits, validating the proposed mechanism. The proportion of the mediating effect is 15.64%, indicating that approximately 15.64% of the influence of off-farm employment on farmers’ adoption behavior is mediated by expected economic benefits.
Overall, approximately 29.52% of the influence of off-farm employment on farmers’ adoption behavior is mediated by expected ecological and economic benefits. Specifically, the role of expected economic benefits is higher than that of expected ecological benefits, indicating that when farmers believe that IPM technology can bring economic benefits, their willingness to adopt the technology is stronger.
Based on micro survey data, this study constructed an endogenous switching Probit model and used a counterfactual analysis framework to estimate the treatment effect of off-farm employment on farmers’ adoption of Integrated Pest Management (IPM) technology. The results indicate the following:
1. If off-farm farmers did not engage in off-farm activities, their probability of adopting IPM technology would decrease by 71.57%. Conversely, if non-off-farm farmers were to engage in off-farm activities, their probability of adopting IPM technology would increase by 76.95%.
2. Off-farm employment not only directly impacts farmers’ adoption of IPM technology but also indirectly influences adoption through the mediating effects of expected ecological and economic benefits. Approximately 29.52% of the total effect is achieved via these mediating pathways.
3. Factors such as age, personal health status, number of household laborers, membership in a cooperative, number of land plots, village topography, and cognition of environmental governance significantly affect farmers’ adoption of IPM technology.
1. Optimize industrial structure and improve rural employment market to increase farmers’ off-farm employment
The government should reasonably plan the industrial layout in rural areas, developing labor-intensive industries to promote off-farm employment and attract significant investment. This approach benefits households with elderly members by providing opportunities for off-farm employment while allowing them to care for their elders and contribute to agricultural production. Diversifying income sources and increasing household income levels enable farmers to invest in green production technologies, including IPM. The focus should be on developing the tertiary industry to create more jobs for rural laborers.
2. Enhance the role of farmers’ professional cooperatives and improve agricultural socialized service efficiency
Farmers’ professional cooperatives should collaborate with employment units and enterprises to increase job opportunities for rural laborers. They can also aggregate labor information and provide suitable employment opportunities to farmers. Cooperatives should verify job information to protect farmers’ rights and can sign long-term off-farm employment contracts to help farmers secure non-agricultural income.
3. Strengthen education and training for rural laborers to improve non-farm employment skills
The government should promote vocational and technical education to help farmers find suitable jobs. Enterprises can offer pre-job training based on market needs, determining training content and targets accordingly. Tailored educational programs should be provided for different types of farmers, ensuring the content is clear and practical. This approach will help farmers acquire relevant knowledge and skills, making them more adaptable to job requirements.
4. Increase publicity efforts to raise farmers’ cognition levels
The government can utilize local TV stations, radio, short video platforms, public accounts, and professional technical personnel to promote green production technologies, environmental protection policies, and agricultural machinery purchase subsidies. These efforts will enhance farmers’ awareness of farmland protection and new technologies. Farmers should also keep pace with the information age, actively learning about and responding to environmental governance policies, mastering green production techniques, and increasing their knowledge reserves to improve their cognition levels.
By following these recommendations, it is possible to enhance the adoption of IPM technology among farmers, contributing to sustainable agricultural practices and improved rural livelihoods.
While this study provides valuable insights into the relationship between off-farm employment and farmers’ adoption of Integrated Pest Management (IPM) technology, it is not without limitations. First, the data is cross-sectional, limiting the ability to infer causal relationships over time. A longitudinal approach would provide a more robust understanding of how these dynamics evolve. Second, while the survey covered diverse regions in China to ensure representativeness, local cultural and policy differences may still affect the generalizability of the findings. Finally, the measurement of ecological cognition, though carefully designed, relies on self-reported perceptions, which may introduce bias or limit the precision of the construct. Future research could address these limitations by incorporating panel data, exploring region-specific factors, and utilizing objective measures of ecological cognition.
The original contributions presented in the study are included in the article/supplementary material, further inquiries can be directed to the corresponding author/s.
Ethical review and approval was not required for the study on human participants in accordance with the local legislation and institutional requirements. Written informed consent from the patients/participants or patients/participants legal guardian/next of kin was not required to participate in this study in accordance with the national legislation and the institutional requirements.
JK: Conceptualization, Data curation, Formal analysis, Investigation, Methodology, Project administration, Software, Writing – original draft, Writing – review & editing. HD: Data curation, Formal analysis, Investigation, Methodology, Resources, Software, Writing – review & editing. QW: Funding acquisition, Investigation, Methodology, Resources, Supervision, Writing – review & editing. AR: Formal analysis, Investigation, Methodology, Resources, Software, Writing – review & editing.
The author(s) declare that financial support was received for the research, authorship, and/or publication of this article. This work was supported by Scientific Research Project of Education Department of Hubei (Grant no. B2022202).
The authors declare that the research was conducted in the absence of any commercial or financial relationships that could be construed as a potential conflict of interest.
The authors declare that no Gen AI was used in the creation of this manuscript.
All claims expressed in this article are solely those of the authors and do not necessarily represent those of their affiliated organizations, or those of the publisher, the editors and the reviewers. Any product that may be evaluated in this article, or claim that may be made by its manufacturer, is not guaranteed or endorsed by the publisher.
Bai, Y., Wang, W., and Zhang, L. (2022). Off-farm employment experience triggers heterogeneity of exiting part-time farming in rural China. China Agric. Econ. Rev. 14, 567–582. doi: 10.1108/CAER-04-2020-0069
Bass, C., and Jones, C. M. (2018). Editorial overview: pests and resistance: resistance to pesticides in arthropod crop pests and disease vectors: mechanisms, models and tools. Curr. Opin. Insect Sci. 27, iv–vii. doi: 10.1016/j.cois.2018.04.009
Brosig, S., Glauben, T., Herzfeld, T., and Wang, X. (2009). Persistence of full- and part-time farming in southern China. China Econ. Rev. 20, 360–371. doi: 10.1016/j.chieco.2008.10.005
Chen, Y. S. (2019). Time allocation of the rural labor from a perspective of farming season: farming and off-farm employment. Chin. J. Popul. Sci. 75–86, 127–128.
Constantino, S. M., Schlüter, M., Weber, E. U., and Wijermans, N. (2021). Cognition and behavior in context: a framework and theories to explain natural resource use decisions in social-ecological systems. Sustain. Sci. 16, 1651–1671. doi: 10.1007/s11625-021-00989-w
Creissen, H. E., Jones, P. J., and Tranter, R. B.others (2021). Identifying the drivers and constraints to adoption of IPM amongst arable farmers in the UK and Ireland. Pest Manag. Sci. 77, 4148–4158. doi: 10.1002/ps.6452
De Groote, H., and Coulibaly, N. (1998). Gender and generation: an intra-household analysis on access to resources in southern Mali. Afr. Crop. Sci. J. 6:827. doi: 10.4314/acsj.v6i1.27827
Deguine, J. P., Aubertot, J. N., Flor, R. J., Lescourret, F., Wyckhuys, K. A. G., and Ratnadass, A. (2021). Integrated pest management: good intentions, hard realities. A review. Agron. Sustain. Dev. 41:38. doi: 10.1007/s13593-021-00689-w
Duan, W., and Luo, G. (2024). Ecological cognition, digital agricultural technology adoption, and the sustainable development of family grain farms – an empirical study from China. Pol. J. Environ. Stud. 33, 3609–3623. doi: 10.15244/pjoes/178201
Fahad, S., Wang, J., Guangyin, H., Wang, H., Yang, X., Shah, A. A., et al. (2018). Empirical analysis of factors influencing farmers' crop insurance decisions in Pakistan: evidence from Khyber Pakhtunkhwa province. Land Use Policy 75, 459–467. doi: 10.1016/j.landusepol.2018.04.016
Fernandez-Cornejo, J. (1998). Environmental and economic consequences of technology adoption: IPM in viticulture. Agric. Econ. Agric. Econ. 18, 145–155. doi: 10.1016/S0169-5150(97)00054-6
Gautam, S., Schreinemachers, P., Uddin, M. N., and Srinivasan, R. (2017). Impact of training vegetable farmers in Bangladesh in integrated pest management (IPM). Crop Prot. 102, 161–169. doi: 10.1016/j.cropro.2017.08.022
Grasswitz, T. R. (2019). Integrated Pest management (IPM) for small-scale farms in developed economies: challenges and opportunities. Insects 10:179. doi: 10.3390/insects10060179
Hao, H., Li, X., and Zhang, J. (2013). Impacts of part-time farming on agricultural land use in ecologically-vulnerable areas in North China. J. Resour. Ecol. 40, 70–79. doi: 10.5814/j.issn.1674-764x.2013.01.010
Hao, Y., and Zheng, S. (2017). Would environmental pollution affect home prices? An empirical study based on China's key cities. Environ. Sci. Pollut. Res. 24, 24545–24561. doi: 10.1007/s11356-017-0073-4
Hayami, Y., and Ruttan, V. W. (1985). Agricultural development: An international perspective. Baltimore MD: Johns Hopkins University Press.
Heckman, J., Pinto, R., and Savelyev, P. (2013). Understanding the mechanisms through which an influential early childhood program boosted adult outcomes. Am. Econ. Rev. 103, 2052–2086. doi: 10.1257/aer.103.6.2052
Horgan, F. G. (2017). “Integrated pest management for sustainable rice cultivation: a holistic approach” in Achieving sustainable cultivation of Rice. ed. F. G. Horgan (Cambridge, UK: Burleigh Dodds), 271–292.
Jieling, Z., Zhengyi, D., and Yubin, W. (2018). “Same way, different destination”: the impact of migrant workers on farmers’ adoption of sustainable agricultural technology. China Rural Econ. 8, 83–98.
Kai, L., Yu, J., and Jie-hong, Z. (2023). Are vulnerable farmers more easily influenced? Heterogeneous effects of internet use on the adoption of integrated pest management. J. Integr. Agric. 22, 3220–3233. doi: 10.1016/j.jia.2023.08.005
Kaiser, T., Rohner, M., and Matzdorf, B. (2010). Validation of grassland indicator species selected for result-oriented Agri-environmental schemes. Biodivers. Conserv. 19, 1297–1314. doi: 10.1007/s10531-009-9762-8
Korir, J. K., Affognon, H. D., Ritho, C. N., Kingori, W. S., Irungu, P., Mohamed, S. A., et al. (2015). Grower adoption of an integrated pest management package for management of mango-infesting fruit flies (Diptera: Tephritidae) in Embu, Kenya. Int. J. Trop. Insect Sci. 35, 80–89. doi: 10.1017/S1742758415000077
Lee, R., Uyl, R., and Runhaar, H. (2019). Assessment of policy instruments for pesticide use reduction in Europe; Learning from a systematic literature review. Crop Prot. 126:104929. doi: 10.1016/J.CROPRO.2019.104929
Lefebvre, M., Langrell, S., and Gomez-y-Paloma, S. (2014). Incentives and policies for integrated pest management in Europe: a review. Agron. Sustain. Dev. 35, 27–45. doi: 10.1007/s13593-014-0237-2
Leng, X., Zhang, Y., and Cheng, R. (2024). Digital technology, labor allocation, and nutrition intake: Evidence from China. Technol Forecast Soc Change, 201:123228. doi: 10.1016/j.techfore.2024.123228
Li, C., and Jin, L. (2022). Multi-level determinants of acceptance in centralized pesticide delivery among farmers: Evidence from Huangshan city, China. Water 14:1566. doi: 10.3390/w14101566
Liu, E. M., and Huang, J. K. (2013). Risk preferences and pesticide use by cotton farmers in China. J. Dev. Econ. 103, 202–215. doi: 10.1016/j.jdeveco.2012.12.005
Liu, Y., Lonappan, L., Brar, S. K., and Yang, S. (2018). Impact of biochar amendment in agricultural soils on the sorption, desorption, and degradation of pesticides: a review. Sci. Total Environ. 645, 60–70. doi: 10.1016/j.scitotenv.2018.07.099
Liu, Q., and Shumway, C. R. (2006). Geographic aggregation and induced innovation in American agriculture. Appl. Econ. 38, 671–682. doi: 10.1080/00036840500397457
Lu, Y., Chen, M., and Weng, Z. (2022). Drivers of the peasant households’ part-time farming behavior in China. J Rural Stud, 93, 112–121. doi: 10.1016/j.jrurstud.2022.03.021
Lucchi, A., and Benelli, G. (2018). Towards pesticide-free farming? Sharing needs and knowledge promotes integrated Pest management. Environ. Sci. Pollut. Res. 25, 13439–13445. doi: 10.1007/s11356-018-1919-0
Ma, W., and Abdulai, A. (2018). IPM adoption, cooperative membership, and farm economic performance: insight from apple farmers in China. China Agric. Econ. Rev. 2018, 25. doi: 10.1108/CAER-12-2017-025
Meng, L., and Si, W. (2022). Pro-environmental behavior: examining the role of ecological value cognition, environmental attitude, and place attachment among rural farmers in China. Int. J. Environ. Res. Public Health 19:17011. doi: 10.3390/ijerph192417011
Mian, M. Y., Hossain, M. S., and Karim, A. N. M. (2016). “Integrated pest management of tropical vegetable crops” in Integrated pest management of vegetable crops in Bangladesh. eds. R. Muniappan and E. A. Heinrichs (Netherlands, Dordrecht: Springer), 235–249.
Moss, S. (2019). Integrated weed management (IWM): why are farmers reluctant to adopt non-chemical alternatives to herbicides? Pest Manag. Sci. 75, 1205–1211. doi: 10.1002/ps.5267
Mostafalou, S., and Abdollahi, M. (2017). Pesticides: an update of human exposure and toxicity. Arch. Toxicol. 91, 549–599. doi: 10.1007/s00204-016-1849-x
Mouron, P., Scholz, R. W., Nemecek, T., and Weber, O. (2006). Life cycle management on Swiss fruit farms: relating environmental and income indicators for apple-growing. Ecol. Econ. 58, 561–578. doi: 10.1016/j.ecolecon.2005.08.007
Murray, K., Jepson, P., Bouska, C., Scherr, M., Walenta, D., et al. (2021). Integrated Pest management summit reveals barriers, needs, and goals for agricultural extension. J. Ext. 58:24. doi: 10.34068/joe.58.03.24
Mustafa, N. M., Asfaw, F. F., Endris, E. A., and Bojago, E. (2023). Evaluating the impact of productive safety net program on rural household food security achievement: Endogenous switching regression modeling approach. J AGR FOOD RES, 14. doi: 10.1016/j.jafr.2023.100674
Nie, J. F., Li, B., Zhang, Y., Fan, J. L., Yi, Z. S., and Cai, Z. R. (2016). High-order calibration for the spectrofluorimetric determination of pesticides based on photochemical derivatization. A solution of the problems of inner-filter effects and matrix interferences in complex environmental water. Chemom. Intell. Lab. Syst. 156, 36–53. doi: 10.1016/j.chemolab.2016.05.004
Otieno, S. J., Ritho, C. N., Nzuma, J. M., and Muriithi, B. W. (2023). Determinants of adoption and dis-adoption of integrated Pest management practices in the suppression of mango fruit Fly infestation: evidence from Embu County, Kenya. Sustainability 15:1891. doi: 10.3390/su15031891
Parsa, S., Morse, S., Bonifacio, A., Chancellor, T. C. B., Condori, B., Crespo-Pérez, V., et al. (2014). Obstacles to integrated pest management adoption in developing countries. PNAS 111, 3889–3894. doi: 10.1073/pnas.1312693111
Paudel, S., Sah, L. P., Devkota, M., Poudyal, V., Vara Prasad, P.V., and Reyes, M. R. (2020). Conservation agriculture and integrated pest management practices improve yield and income while reducing labor, pests, diseases, and chemical pesticide use in smallholder vegetable farms in Nepal. Sustainability, 12. doi: 10.3390/su12166418
Pretty, J., and Bharucha, Z. P. (2015). Integrated pest management for sustainable intensification of agriculture in Asia and Africa. Insects 6, 152–182. doi: 10.3390/insects6010152
Ren, J., Lei, H., and Ren, H. (2022). Livelihood capital, ecological cognition, and farmers’ green production behavior. Sustain. For. 14:6671. doi: 10.3390/su142416671
Rezaei, R., Seidi, M., and Karbasioun, M. (2019). Pesticide exposure reduction: extending the theory of planned behavior to understand Iranian farmers’ intention to apply personal protective equipment. Saf. Sci. 120, 527–537. doi: 10.1016/j.ssci.2019.07.044
Rodrigues, E. T., Alpendurada, M. F., Ramos, F., and Pardal, M. Á. (2018). Environmental and human health risk indicators for agricultural pesticides in estuaries. Ecotoxicol. Environ. Saf. 150, 224–231. doi: 10.1016/j.ecoenv.2017.12.047
Sadique, R. M., Norton, G. W., and Harun-Ar, R. M. (2018). Economic impacts of integrated pest management on vegetable production in Bangladesh. Crop Prot. 113, 6–14. doi: 10.1016/j.cropro.2018.07.004
Savary, S., Willocquet, L., Pethybridge, S. J., Esker, P., McRoberts, N., and Nelson, A. (2019). The global burden of pathogens and pests on major food crops. Nat. Ecol. Evol. 3, 430–439. doi: 10.1038/s41559-018-0793-y
Schreinemachers, P., and Tipraqsa, P. (2012). Agricultural pesticides and land use intensification in high, middle and low income countries. Food Policy 37, 616–626. doi: 10.1016/j.foodpol.2012.06.003
Shrestha, S., Amgain, L. P., Pandey, P., Bhandari, T., and Khatiwada, S. (2024). Adoption status of integrated pest management (IPM) practices among vegetable growers of Lamjung district of Nepal. Heliyon 10:e37999. doi: 10.1016/j.heliyon.2024.e37999
Singh, B. K., Delgado-Baquerizo, M., Egidi, E., Guirado, E., Leach, J. E., Liu, H., et al. (2023). Climate change impacts on plant pathogens, food security and paths forward. Nat. Rev. Microbiol. 21, 640–656. doi: 10.1038/s41579-023-00900-7
Tian, M., Zheng, Y., Sun, X., and Zheng, H. (2022). A research on promoting chemical Fertiliser reduction for sustainable agriculture purposes: evolutionary game analyses involving ‘government, farmers, and consumers. Ecol. Indic. 144:109433. doi: 10.1016/j.ecolind.2022.109433
Varjani, S., Kumar, G., and Rene, E. R. (2019). Developments in biochar application for pesticide remediation: current knowledge and future research directions. J. Environ. Manag. 232, 505–513. doi: 10.1016/j.jenvman.2018.11.043
Wang, X., Han, Y., Chen, Y., Sui, P., Gao, W., Wu, X., et al. (2015). Efficiency and sustainability evaluation of a pollution-free vegetable production system based on emergy analysis. Acta Ecol. Sin. 35, 2136–2145. doi: 10.5846/stxb201306041318
Wang, X., Yu, D., Ma, L., Lu, X., Song, J., and Lei, M. (2022). Using big data searching and machine learning to predict human health risk probability from pesticide site soils in China. J. Environ. Manag. 320:115798. doi: 10.1016/j.jenvman.2022.115798
Weng, Z. L., Gao, X. P., and Tan, Z. P. (2017). Farmers endowment, regional environment, and food and agriculture concurrently: Based on the questionnaire survey of 1,647 grain growers in 9 provinces. J Agric Econ, 61–71.
Xin, G., and Anlu, Z. (2017). Farmland transfer, the relationship between the degree of concurrent business of farmers and production efficiency. China Popul. Resour. Environ. 27, 121–128.
Yaguana, V., Alwang, J., Norton, G., and Barrera, V. (2016). Does IPM have staying power? Revisiting a potato-producing area years after formal training ended. J. Agric. Econ. 67, 308–323. doi: 10.1111/1477-9552.12140
Yang, J., Wan, Q., and Bi, W. (2020). Off-farm employment and grain production change: new evidence from China. China Econ. Rev. 63:101519. ISSN 1043-951X. doi: 10.1016/j.chieco.2020.101519
Yazdanpanah, M., Feyzabad, F. R., Forouzani, M., Mohammadzadeh, S., and Burton, R. J. F. (2015). Predicting farmers’ water conservation goals and behavior in Iran: a test of social cognitive theory. Land Use Policy 47, 401–407. doi: 10.1016/j.landusepol.2015.04.022
Yuriev, A., Dahnem, M., Boiral, O., Paillé, P., and Guillaumie, L. (2020). Pro-environmental behaviors through the lens of the theory of planned behavior: A scoping review. Resour Conserv Recycl, 155:104660. doi: 10.1016/j.resconrec.2019.104660
Yujiro, S., and Hang, W. (2004). A new model of the relationship between rural and urban development from the perspective of globalization. J. Natl. Acad. Gov. 1, 92–95.
Zhang, C., Guanming, S., Shen, J., and Hu, R. F. (2015a). Productivity effect and overuse of pesticide in crop production in China. J. Integr. Agric. 14, 1903–1910. doi: 10.1016/S2095-3119(15)61056-5
Zhang, C., Hu, R., Shi, G., Jin, Y., Robson, M. G., and Huang, X. (2015b). Overuse or underuse? An observation of pesticide use in China. Sci. Total Environ. 538, 1–6. doi: 10.1016/j.scitotenv.2015.08.031
Zhang, L., Li, X., Yu, J., and Yao, X. (2018). Toward cleaner production: what drives farmers to adopt eco-friendly agricultural production? J. Clean. Prod. 184, 550–558. doi: 10.1016/j.jclepro.2018.02.272
Zhang, X. P., Zhang, Y. Y., Mai, L., Liu, L. Y., Bao, L. J., and Zeng, E. Y. (2020). Selected antibiotics and current-use pesticides in riverine runoff of an urbanized river system in association with anthropogenic stresses. Sci. Total Environ. 739:140004. doi: 10.1016/j.scitotenv.2020.140004
Zhao, Y. (1999). Leaving the countryside: rural-to-urban migration decisions in China. Am. Econ. Rev. 89, 281–286. doi: 10.1257/aer.89.2.281
Zhu, C. B. (2015). Adoption decision and adoption density of integrated pest control technology in rural households: An empirical analysis based on the double-hurdle model. J Agric Econ, 117–127.
Keywords: sustainable farm development, off-farm employment, integrated pest management technology, adoption behavior, agricultural policies, ecological cognition
Citation: Ke J, Dan H, Wang Q and Razzaq A (2025) The role of off-farm employment in promoting sustainable farm development through integrated pest management technology adoption. Front. Sustain. Food Syst. 8:1503951. doi: 10.3389/fsufs.2024.1503951
Received: 30 September 2024; Accepted: 11 December 2024;
Published: 06 January 2025.
Edited by:
Juan Lu, Nanjing Agricultural University, ChinaReviewed by:
He Li, Nanjing University of Posts and Telecommunications, ChinaCopyright © 2025 Ke, Dan, Wang and Razzaq. This is an open-access article distributed under the terms of the Creative Commons Attribution License (CC BY). The use, distribution or reproduction in other forums is permitted, provided the original author(s) and the copyright owner(s) are credited and that the original publication in this journal is cited, in accordance with accepted academic practice. No use, distribution or reproduction is permitted which does not comply with these terms.
*Correspondence: Qing Wang, aGFtMjU3MUAxNjMuY29t; Amar Razzaq, YW1hci5yYXp6YXFAaG90bWFpbC5jb20=
Disclaimer: All claims expressed in this article are solely those of the authors and do not necessarily represent those of their affiliated organizations, or those of the publisher, the editors and the reviewers. Any product that may be evaluated in this article or claim that may be made by its manufacturer is not guaranteed or endorsed by the publisher.
Research integrity at Frontiers
Learn more about the work of our research integrity team to safeguard the quality of each article we publish.