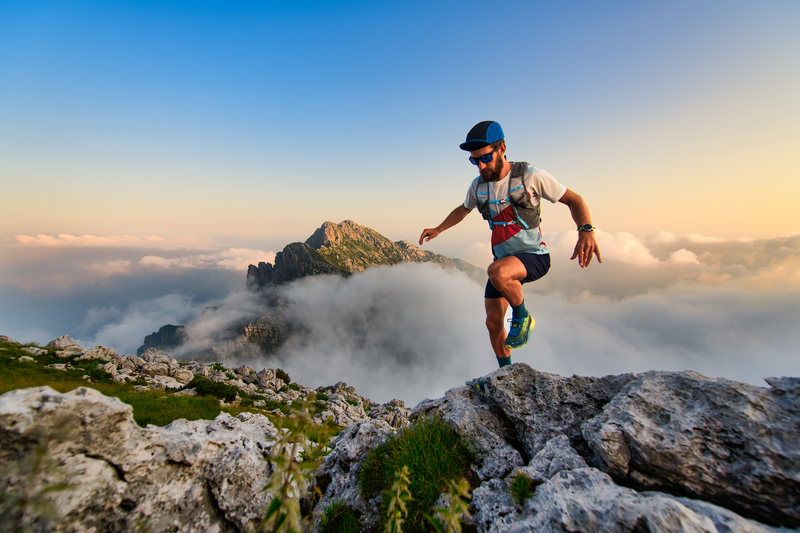
95% of researchers rate our articles as excellent or good
Learn more about the work of our research integrity team to safeguard the quality of each article we publish.
Find out more
OPINION article
Front. Sustain. Food Syst. , 06 January 2025
Sec. Crop Biology and Sustainability
Volume 8 - 2024 | https://doi.org/10.3389/fsufs.2024.1499973
This article is part of the Research Topic Multi-Scale Systems: Ecological Approaches to Investigate the Role of the Microbiota in Different Niches View all 6 articles
The global demand for food is driving the need for high-performance, sustainable agricultural systems that incorporate advanced technologies for monitoring, control, and decision-making. With the population expected to reach 9.7 billion by 2050, agriculture must boost productivity while maintaining sustainability. Precision agriculture (PA) addresses this challenge by using advanced technologies to increase yields, reduce resource waste, and minimize environmental impacts (Gebbers and Adamchuk, 2010; Delgado et al., 2020; El-Kader and El-Basioni, 2020). This “fourth agricultural revolution” is reshaping farming through innovations in data analytics, communication, and technology (Mohindru et al., 2021; Abdel-Basset et al., 2024).
A key aspect of sustainable agriculture is the soil microbiome, especially the rhizosphere, which promotes soil health and crop resilience while reducing environmental harm. Next-generation sequencing (NGS) techniques, such as amplicon sequencing and shotgun metagenomics, provide deep insights into microbial communities, their diversity, and functional roles. These tools are vital for monitoring agricultural interventions, identifying beneficial microbes, and detecting pathogens early to prevent crop diseases (Elnahal et al., 2022).
Understanding the physical, biological, and chemical characteristics of soil is crucial for optimizing crop management practices such as irrigation, drainage, and nutrient management—key components of PA. The integration of advanced technologies like artificial intelligence (AI), remote sensing, unmanned aerial vehicles (UAVs), big data analytics, the Internet of Things (IoT), Global Positioning system (GPS), and Geographic Information Systems (GIS) enables the precise management of spatial variability in fields. UAVs, with their high spatial resolution and flexibility, have revolutionized soil and crop monitoring, offering real-time data collection from difficult-to-reach areas (Boursianis et al., 2022).
Integrating UAV-based remote sensing with soil metagenomics represents a transformative step forward for PA and ecosystem restoration. The fusion of these advanced tools not only enhances farming by improving resource efficiency but also aligns with the broader objectives of sustainable agriculture, reducing the environmental impact of farming by minimizing chemical inputs and fostering healthier, more resilient ecosystems. As these technologies evolve and become more cost-effective and accessible, their integration will likely become a standard practice in modern agriculture, driving widespread adoption and promoting a sustainable future for global food production. We review the current advancements in both fields, propose methods for integrating remote sensing data with soil microbiome profiles, and present a framework for implementing this integrated approach to optimize precision farming.
Soil is a diverse environment, home to billions of microorganisms. Enhancing soil health can boost crop productivity by 10–50%, and with plant growth-promoting microbes, productivity can rise by 50–60% (Abram, 2015; O'Callaghan et al., 2022). This reduces reliance on chemical fertilizers, supporting sustainable agriculture. Metagenomics, which sequences and analyzes environmental DNA, reveals microbial diversity and aids in discovering therapeutic molecules, biotechnological innovations, and sustainable practices (Abram, 2015; Garrido-Oter et al., 2018). It offers insights into microbial community structures, including bacteria, archaea, and eukaryotes, based on functional gene composition (Philippot et al., 2013; Martínez-Porchas and Vargas-Albores, 2017). The workflow of soil metagenomics will be discussed in detail further.
Metagenomic studies involve collecting soil samples, particularly from the rhizosphere, where soil microbes and root secretions interact (Weaver, 1994; Brooks, 2015). Total DNA is extracted from the samples using kits like Genejet Soil DNA Kit (Thermo Fisher) or Fast DNA SPIN Kit (MP Biochemicals). DNA is then enzymatically fragmented using library preparation kits such as Nextera Tagmentation (Illumina) or Fragmentase (New England Biolabs), with alternative methods including acoustic shearing, sonication, and others (Sabale et al., 2020). DNA concentration and purity are measured using Qubit and Nanodrop, while integrity is assessed via agarose gel electrophoresis or Agilent TapeStation. The DNA fragments are cloned into bacterial plasmids, featuring elements like an origin of replication, restriction sites, selective markers, and cloning sites (Granjou and Phillips, 2019). Fragments are analyzed using a fragment analyzer for quality and quantity. Sequencing is conducted on platforms like Illumina, Pyrosequencing, Nanopore, and PacBio. Post-sequencing, data are de-multiplexed and analyzed (Martin, 2011; Oulas et al., 2015; Mahmoud et al., 2019; Zhang et al., 2021).
Pre-processing of soil metagenomics data begins with quality control to filter out low-quality reads and remove adapter sequences. Tools such as UCHIME, MG-RAST, RDP tools (Bolger et al., 2014), KTrim, Trim Galore, and Trimmomatic (Sun, 2020) are utilized for these tasks, ensuring sequences are trimmed for uniform length and quality. Following trimming, reads are further filtered to exclude sequences below specified length thresholds, and errors are corrected while polymerase chain reaction (PCR) duplicates are removed to enhance accuracy. Denoising of metagenomic data is achieved using platforms like MOTHUR and QIIME 2, with UCHIME used for detecting and eliminating chimeric sequences (Santamaria et al., 2018). Post-processing involves grouping reads by unique barcodes, removing primers, and employing tools such as Taxator-tk (Dröge et al., 2015) and MEGAHIT (Liu et al., 2015) for further taxonomic and functional classification. Recent advancements in de novo assemblers like Meta-IDBA, metaSPAdes, Ray Meta, and Contig Extender allow for assembly of metagenomic reads into contigs, particularly beneficial for sequencing novel microbial genomes without prior reference sequences (Peng et al., 2011; Boisvert et al., 2012; Kumar et al., 2018; Deng and Delwart, 2021). Subsequently, reads are aligned to reference databases or assembled into contigs for comprehensive taxonomic and functional analysis, facilitating robust interpretation of soil microbial community data.
Metagenomic data processing forms the basis for taxonomic and functional profiling, essential for understanding microbial communities in soil. Tools like Krona, MEGAN, and phyloseq in R visualize taxonomic diversity and abundance (Huson et al., 2007; Ondov et al., 2011; McMurdie and Holmes, 2013). Functional analysis begins with gene prediction using tools such as Prodigal and MetaGeneMark, followed by annotation via KEGG, COG, and Pfam databases using eggNOG-mapper and InterProScan (Hyatt et al., 2010; Zhu et al., 2010). Pathway reconstruction is achieved using KEGG and MetaCyc databases with HUMAnN2 (Franzosa et al., 2018) and PathoScope (Hong et al., 2014), while functional capabilities are predicted by tools like PICRUSt, and Tax4Fun (Langille et al., 2013; Sun et al., 2020). Statistical analyses, including DESeq2, edgeR, PCA, and NMDS, identify and visualize differential taxa or functions. Integration of taxonomic and functional data, along with network and ecological models, further explores microbial interactions and their ecological roles (Robinson et al., 2010).
Common microbial species isolated from rhizosphere soil using metagenomic approaches include Firmicutes, Bacteroidetes, Proteobacteria, Actinobacteria, and others (Babalola, 2010; Santos et al., 2019; Prasad and Zhang, 2022). Table 1 highlights microbial species and their impact on soil quality. By promoting beneficial microbes that fix nitrogen or solubilize phosphorus, farmers can reduce reliance on synthetic fertilizers, enhancing crop productivity, profits, and sustainability (Mendes et al., 2013). Enzymes like sulfatases, dehydrogenases, and phosphatases improve soil fertility, crop growth, and yield, reducing pesticide use (Peng et al., 2018). Metagenomics also supports the development of biofertilizers and microbial inoculants for agriculture and offers cost-effective alternatives to traditional soil remediation methods (Philippot et al., 2013, 2024). Several studies have demonstrated the economic advantages of microbial inoculants in agriculture. For instance, recurrent pre-sowing applications of Pseudomonas fluorescens have significantly boosted maize growth, reducing reliance on costly chemical fertilizers (Papin et al., 2024). Investigations into microbiota responses to nitric oxide regulation in Arabidopsis thaliana highlight the potential for enhancing crop productivity through optimized plant-microbe interactions (Berger et al., 2024). Moreover, trials using affordable microbial nutrient solutions have underscored their role in improving food security while offering cost-effective alternatives to conventional agricultural practices (van der Velde et al., 2013).
The appropriate spatio-temporal resolution required for PA depends on various factors, including management objectives, field size, and the capability of farm equipment to vary input (irrigation, fertilizer, pesticide, etc.) application rates. While PA can use a variety of sensors, this paper limits itself to those studies that primarily used UAV image data (Shafi et al., 2019). UAVs are transforming agriculture by offering precise, efficient, and sustainable solutions for various farming practices. They help in enhancing productivity, conserving resources, and promoting eco-friendly practices. Recent studies predict that by 2025, the global UAV industry for agriculture would increase at a compound annual growth rate of 35.9% and reach $5.7 billion (Agriculture Drones Market).
Aerial platforms such as UAVs or drones generally provide higher spatial resolution (< 5 meters) images compared to satellites (Bochtis et al., 2023). Thus, UAVs and other ground-based platforms offer greater flexibility in providing images at fine spatial and temporal resolutions (more frequently) or as needed. The hydrologic and climatic parameters—such as soil organic carbon, soil moisture, soil characteristics, the normalized Difference Vegetation Index (NDVI), leaf area index (LAI), groundwater, and rainfall—as well as the health of the vegetation and soil are monitored using unmanned aerial vehicles (UAVs) (Zhang et al., 2022).
Equipped with various sensors, UAVs perform specialized tasks: multispectral sensors capture plant health data in specific light wavelengths, thermal cameras detect temperature changes to identify irrigation or pest issues, and LIDAR creates detailed topographic maps for land and water management (Maddikunta et al., 2021; Tahir et al., 2023). However, global adoption of drone technology varies due to differing legal, financial, and physical conditions across countries. Supplementary Table S1 lists the various drones that are recognized for their capabilities in precision farming and improved crop management, ensuring compliance with safety and operational standards of respective aviation authorities.
Data collection and preprocessing in precision agriculture (PA) involve drones and IoT sensors, creating a comprehensive dataset. IoT sensors, such as Decagon EC-5 for soil, Davis Vantage Pro2 for weather, and GreenSeeker for crops, gather key data on moisture, temperature, pH, nutrients, climate, and crop health (García et al., 2020; Fuentes and Chang, 2022). Supplementary Table S2 highlights IoT sensors employed in precision agriculture, emphasizing their importance in enabling real-time, data-driven farming solutions. Connectivity networks like LoRaWAN, NB-IoT, and 5G transmit this data through gateways like Kerlink Wirnet Station. Flight planning software like DJI Ground Station Pro and drones like DJI Phantom 4 RTK capture aerial images (KriŽanović et al., 2023), followed by georeferencing and image stitching using tools like Agisoft Metashape. Noise reduction in software like Pix4Dmapper enhances image quality, while cloud computing supports secure data storage and real-time analysis (Debauche et al., 2022).
Precision farming is widely adopted due to the power of AI, driven by machine learning (ML) and deep learning (DL) (Khan et al., 2022; Ojo and Zahid, 2022; Hashmi and Kesakr, 2023). ML models analyze UAV-captured images, and AI-enabled farm management systems use sensor data to provide real-time recommendations for farmers. Machine learning (ML) approaches like Support Vector Machines (SVM) and Random Forests were applied for soil management to analyze soil temperature, moisture, and drying patterns (Liakos et al., 2018; Sharma et al., 2021). Models utilizing Decision Trees and Neural Networks were created to predict soil pH and fertility (Suchithra and Pai, 2020), while Multiple Linear Regression (MLR) and Support Vector Regression (SVR) were used to estimate pH and Soil Organic Matter (SOM) in paddy soils (Yang et al., 2019). Partial Least Squares Regression (PLSR) was used to predict moisture content (MC), total nitrogen (TN), and soil organic carbon (SOC) (Morellos et al., 2016). Soil moisture was estimated by combining the Auto-Regressive Error Function (AREF) with Gradient Boosting and k-Nearest Neighbors (k-NN) (Johann et al., 2016). Extreme Learning Machine (ELM) integrated with a Self-Adaptive Evolutionary agent (SaE) was used to assess soil temperature (Nahvi et al., 2016), while ELM was employed to forecast surface humidity (Acar et al., 2019). Lastly, Random Forests and SVM were used to estimate SOC and TN in Moroccan soils (Reda et al., 2019). Several studies also advanced ML and image recognition techniques for seed sorting and counting (Li et al., 2021; Nehoshtan et al., 2021; Laudari et al., 2022; Ekramirad et al., 2024). Deep Learning, especially Convolutional Neural Networks (CNNs), revolutionized plant disease and pest detection, allowing rapid and accurate diagnosis, which is crucial for minimizing crop loss (Mohanty et al., 2016; Ramcharan et al., 2017; Too et al., 2019; Argüeso et al., 2020). These methods optimize agricultural processes like planting, irrigation, and fertilization, enhancing productivity. Table 2 highlights key soil parameters detected using UAV technologies across various crops, showcasing advancements in PA research. AI models further refine resource use by recommending precise amounts of water, fertilizer, and pesticides, reducing waste and minimizing environmental impact. These models also simulate different farming scenarios, helping farmers assess and select the most effective strategies (Marvuglia et al., 2022).
Complex data is simplified into actionable insights for farmers, delivered to farmers via mobile apps and dashboards, providing real-time updates, visualizations, and alerts. For instance, farmers receive notifications about pest outbreaks with suggested treatments or alerts about sudden soil moisture drops with actionable irrigation advice. Farmers implement these recommendations and provide feedback, which helps refine the system's accuracy and relevance over time. This feedback loop, combined with adaptive learning, enhances the system's predictive capabilities for future growing seasons. The benefits include optimized operations through precise irrigation, fertilization, and pest control, increased yields from timely interventions, and sustainable practices by reducing chemical use and promoting efficient resource allocation. This practice empowers farmers to make informed decisions, boosting productivity, profitability, and sustainability in agriculture.
Various studies highlight the power of integrating advanced technologies for sustainable agriculture. Remote sensing and metagenomics have been used to monitor biodiversity and microbial diversity in agricultural landscapes (Herzog and Franklin, 2016; Lewin et al., 2024). The combination of exascale computing, AI, and multi-omics data supports plant biology research and the UN's Sustainable Development Goals (Streich et al., 2020; Cembrowska-Lech et al., 2023). Integrating microbiome analysis, metagenomics, and imaging links microbial dynamics to broader ecological processes and plant root health (Beatty et al., 2021; Singer et al., 2021). Soil-plant-microbiota interactions, crucial for ecosystem health, are emphasized for improved sustainability (Giovannetti et al., 2022; Dlamini et al., 2023). UAVs, metagenomics, and environmental sensors optimize real-time soil health management (Meena et al., 2024; Zeng et al., 2024), while AI-driven studies advance forest management, nutrient cycling, and drought tolerance in crops (Chaudhury et al., 2024; Jamil et al., 2024). Additionally, omics and AI applications enhance phytoremediation and environmental outcomes (Mohan et al., 2024). As demonstrated by these studies and illustrated in Figure 1, it is clear that these integrated techniques can significantly promote sustainable farming practices. While integrating diverse datasets within an interdisciplinary framework offers a promising pathway forward, it poses significant challenges due to the complexity and variability of harmonizing data with differing scales, structures, and complexities, requiring solutions such as multivariate statistical and network-based methods (Streich et al., 2020; Cembrowska-Lech et al., 2023). Ensuring model interpretability is another hurdle, as many AI/ML models function as “black boxes,” complicating biological interpretation and limiting trust in predictions. Overfitting, a common issue in ML, further undermines model generalizability and predictive accuracy. Additionally, automating analysis, uncovering non-linear interactions, and fostering interdisciplinary collaboration are essential but demanding tasks that require significant expertise and innovation. Addressing these challenges is crucial for leveraging the full potential of UAV and metagenomics data integration in sustainable agriculture.
Figure 1. Integrated approach to smart farming. Field sensors, data from unmanned aerial vehicles (UAVs), and artificial intelligence (AI) work together to provide real-time insights into key agricultural parameters, such as irrigation needs, soil pH, temperature, weather patterns, pest activity, and nutrient levels. These technologies allow continuous monitoring and real-time adjustments to optimize soil fertility, crop health and productivity. Simultaneously, soil sampling and metagenomic sequencing offer detailed taxonomic and functional profiling of soil microorganisms, revealing the microbial communities involved in nutrient cycling, disease resistance, and soil health. By integrating these datasets, precision agriculture can be enhanced, enabling smarter decision-making and more efficient resource management. This combined approach allows farmers to make timely adjustments in water, fertilizer, and pesticide application while promoting sustainability through reduced resource waste and minimized environmental impact.
The future of sustainable agriculture hinges on an interdisciplinary approach that integrates remote sensing data with Omics data, all grounded in the One Health concept, which emphasizes the interconnectedness of human, plant, and environmental health. Collaboration among experts in molecular biology, microbiology, ecology, bioinformatics, and computer science is key to managing complex datasets, enhancing resource efficiency, and improving precision farming. In-field technologies like drones, sensors, and real-time sequencing (e.g., using Oxford Nanopore's MinION) enable immediate analysis of microbial communities, soil health, and crop conditions, delivering actionable insights directly to farmers through mobile-friendly, easy-to-understand reports. These advances promise a low-intervention, automated agricultural system where sophisticated algorithms process data instantly, enabling farmers to optimize resource use, respond to challenges in real time, and improve yields. Despite challenges around data complexity, technical expertise, integration, privacy, security, and cost limitations, this integrated technology-driven approach holds the potential to boost soil health, crop productivity, and sustainability, transforming modern farming into a more resilient and efficient system.
BA: Conceptualization, Data curation, Writing – original draft, Writing – review & editing. RMan: Data curation, Writing – original draft. RMad: Writing – review & editing.
The author(s) declare that no financial support was received for the research, authorship, and/or publication of this article.
We acknowledge the management, Mr. G. V. Ranga Reddy, Honorable Secretary cum Correspondent and Principal and Dr. N. Hemalatha of Sardar Patel College, Secunderabad for providing facilities and institutional support.
The authors declare that the research was conducted in the absence of any commercial or financial relationships that could be construed as a potential conflict of interest.
All claims expressed in this article are solely those of the authors and do not necessarily represent those of their affiliated organizations, or those of the publisher, the editors and the reviewers. Any product that may be evaluated in this article, or claim that may be made by its manufacturer, is not guaranteed or endorsed by the publisher.
The Supplementary Material for this article can be found online at: https://www.frontiersin.org/articles/10.3389/fsufs.2024.1499973/full#supplementary-material
AI, Artificial Intelligence; AREF, Auto-Regressive Error Function; CNN, Convolutional Neural Networks; DESeq2, Differential gene expression analysis based on the negative binomial distribution; DL, Deep learning; DNA, Deoxyribonucleic Acid; ELM, Extreme Learning Machine; GIS, Geographic Information Systems; GPS, Global Positioning System; IoT, Internet of Things; KEGG, Kyoto Encyclopedia of Genes and Genomes; k-NN, k-Nearest Neighbors; LAI, Leaf Area Index; LIDAR, Light Detection and Ranging; LoRaWAN, Long Range Wide Area Network; MC, Moisture Content; MG-RAST, Metagenomic Rapid Annotations using Subsystems Technology; ML, Machine Learning; MLR, Multiple linear Regression; NB-IoT, Narrowband Internet of Things; NDVI, Normalized Difference Vegetation Index; NGS, Next-generation sequencing; NMDS, Non-metric multidimensional scaling; PA, Precision Agriculture; PCA, Principal Component Analysis; PCR, Polymerase Chain Reaction; PICRUSt, Phylogenetic Investigation of Communities by Reconstruction of Unobserved States; PLSR, Partial Least Squares Regression; QIIME, Quantitative Insights Into Microbial Ecology; RDP, Ribosomal Database Project; SaE, Self-Adaptive Evolutionary Agents; SOC, Soil Organic carbon; SOM, Soil Organic Matter; SVM, Support Vector Machines; SVR, Support Vector Regression; TN, Total Nitrogen; UAV, Unmanned Aerial Vehicle; UN, United Nations; 5G, Fifth generation of wireless cellular technology.
Abdel-Basset, M., Hawash, H., and Abdel-Fatah, L. (2024). Artificial Intelligence and Internet of Things in Smart Farming. Boca Raton, FL: CRC Press.
Abram, F. (2015). Systems-based approaches to unravel multi-species microbial community functioning. Comput. Struct. Biotechnol. J. 13, 24–32. doi: 10.1016/j.csbj.2014.11.009
Acar, E., Ozerdem, M. S., and Ustundag, B. B. (2019). “Machine learning based regression model for prediction of soil surface humidity over moderately vegetated fields,” in 2019 8th International Conference on Agro-Geoinformatics (Agro-Geoinformatics) (Istanbul: IEEE).
Alabi, T. R., Abebe, A., Chigeza, C., and Fowobaje, K. (2022). Estimation of soybean grain yield from multispectral high-resolution UAV data with machine learning models in West Africa. Remote Sens. Appl. Soc. Environm. 27:100782. doi: 10.1016/j.rsase.2022.100782
Argüeso, D., Picon, A., Irusta, U., Medela, A., San-Emeterio, M. G., Bereciartua, A., et al. (2020). Few-Shot Learning approach for plant disease classification using images taken in the field. Comp. Elect. Agricult. 175:105542. doi: 10.1016/j.compag.2020.105542
Ashrafi-Saiedlou, S., Rasouli-Sadaghiani, M., Samadi, A., Barin, M., and Sepehr, E. (2024). Aspergillus niger as an eco-friendly agent for potassium release from K-bearing minerals: Isolation, screening and culture medium optimization using Plackett-Burman design and response surface methodology. Heliyon 10:e29117. doi: 10.1016/j.heliyon.2024.e29117
Babalola, O. O. (2010). Beneficial bacteria of agricultural importance. Biotechnol. Lett. 32, 1559–1570. doi: 10.1007/s10529-010-0347-0
Bashan, Y., Holguin, G., and de-Bashan, L. E. (2004). Azospirillum-plant relationships: physiological, molecular, agricultural, and environmental advances (1997-2003). Can. J. Microbiol. 50, 521–577. doi: 10.1139/w04-035
Basurto-Lozada, D., Hillier, A., Medina, D., Pulido, D., Karaman, S., and Salas, J. (2020). Dynamics of soil surface temperature with unmanned aerial systems. Pattern Recognit. Lett. 138, 68–74. doi: 10.1016/j.patrec.2020.07.003
Beatty, D. S., Aoki, L. R., Graham, O. J., and Yang, B. (2021). The future is big-and small: remote sensing enables cross-scale comparisons of microbiome dynamics and ecological consequences. mSystems 6:e0110621. doi: 10.1128/mSystems.01106-21
Berger, A., Pérez-Valera, E., Blouin, M., Breuil, M. C., Butterbach-Bahl, K., Dannenmann, M., et al. (2024). Microbiota responses to mutations affecting NO homeostasis in Arabidopsis thaliana. New Phytol. 244, 2008–2023. doi: 10.1111/nph.20159
Bochtis, D., Tagarakis, A. C., and Kateris, D. (2023). Unmanned Aerial Systems in Agriculture: Eyes Above Fields. London: Elsevier.
Boisvert, S., Raymond, F., Godzaridis, E., Laviolette, F., and Corbeil, J. (2012). Ray Meta: scalable de novo metagenome assembly and profiling. Genome Biol. 13:R122. doi: 10.1186/gb-2012-13-12-r122
Bolger, A. M., Lohse, M., and Usadel, B. (2014). Trimmomatic: a flexible trimmer for Illumina sequence data. Bioinformatics 30, 2114–2120. doi: 10.1093/bioinformatics/btu170
Boursianis, A. D., Papadopoulou, M. S., Diamantoulakis, P., Liopa-Tsakalidi, A., Barouchas, P., Salahas, G., et al. (2022). Internet of Things (IoT) and Agricultural Unmanned Aerial Vehicles (UAVs) in smart farming: A comprehensive review. Internet of Things 18:100187. doi: 10.1016/j.iot.2020.100187
Brooks, J. (2015). “Soil sampling for microbial analyses,” in Manual of Environmental Microbiology. Washington, DC, USA: ASM Press.
Cembrowska-Lech, D., Krzemińska, A., Miller, T., Nowakowska, A., Adamski, C., Radaczyńska, M., et al. (2023). An integrated multi-omics and artificial intelligence framework for advance plant phenotyping in horticulture. Biology 12:1298. doi: 10.3390/biology12101298
Chain, P., Lamerdin, J., Larimer, F., Regala, W., Lao, V., Land, M., et al. (2003). Complete genome sequence of the ammonia-oxidizing bacterium and obligate chemolithoautotroph Nitrosomonas europaea. J. Bacteriol. 185, 2759–2773. doi: 10.1128/JB.185.9.2759-2773.2003
Chaudhury, R., Chakraborty, A., Rahaman, F., Sarkar, T., Dey, S., and Das, M. (2024). Mycorrhization in trees: ecology, physiology, emerging technologies and beyond. Plant Biol. 26, 145–156. doi: 10.1111/plb.13613
Chen, M., Yang, G., Sheng, Y., Li, P., Qiu, H., Zhou, X., et al. (2017). Inoculation improves the root system architecture, photosynthetic efficiency and flavonoids accumulation of liquorice under nutrient stress. Front. Plant Sci. 8:931. doi: 10.3389/fpls.2017.00931
Chen, Y., Zhou, D., Qi, D., Gao, Z., Xie, J., and Luo, Y. (2017). Growth promotion and disease suppression ability of a sp. CB-75 from banana rhizosphere soil. Front. Microbiol. 8:2704. doi: 10.3389/fmicb.2017.02704
Debauche, O., Mahmoudi, S., Manneback, P., and Lebeau, F. (2022). Cloud and distributed architectures for data management in agriculture 4.0: Review and future trends. J. King Saud Univer. 34, 7494–7514. doi: 10.1016/j.jksuci.2021.09.015
Delgado, J. A., Sassenrath, G. F., and Mueller, T. (2020). Precision Conservation: Goespatial Techniques for Agricultural and Natural Resources Conservation. Hoboken, NJ: John Wiley and Sons.
Deng, Z., and Delwart, E. (2021). ContigExtender: a new approach to improving de novo sequence assembly for viral metagenomics data. BMC Bioinformat. 22:119. doi: 10.1186/s12859-021-04038-2
Dlamini, P., Sekhohola-Dlamini, L. M., and Cowan, A. K. (2023). Editorial: Soil-microbial interactions. Front. Microbiol. 14:1213834. doi: 10.3389/fmicb.2023.1213834
Dröge, J., Gregor, I., and McHardy, A. C. (2015). Taxator-tk: precise taxonomic assignment of metagenomes by fast approximation of evolutionary neighborhoods. Bioinformatics 31, 817–824. doi: 10.1093/bioinformatics/btu745
Earl, A. M., Losick, R., and Kolter, R. (2008). Ecology and genomics of Bacillus subtilis. Trends Microbiol. 16, 269–275. doi: 10.1016/j.tim.2008.03.004
Ekramirad, N., Doyle, L., Loeb, J., Santra, D., and Adedeji, A. A. (2024). Hyperspectral imaging and machine learning as a nondestructive method for proso millet seed detection and classification. Foods. 13:1330. doi: 10.3390/foods13091330
El-Kader, S. M. A., and El-Basioni, B. M. M. (2020). “Precision agriculture technologies for food security and sustainability,” in Engineering Science Reference.
Elnahal, A. S., El-Saadony, M. T., Saad, A. M., Desoky, E. S. M., El-Tahan, A. M., Rady, M. M., et al. (2022). The use of microbial inoculants for biological control, plant growth promotion, and sustainable agriculture: a review. Eur. J. Plant Pathol. 162, 759–792. doi: 10.1007/s10658-021-02393-7
El-Sawah, A., El-Keblawy, A., Ali, D., Ibrahim, H., El-Sheik, M., Sharma, A., et al. (2021). Arbuscular mycorrhizal fungi and plant growth-promoting rhizobacteria enhance soil key enzymes, plant growth, seed yield, and qualitative attributes of guar. Collection FAO: Agriculture 11:194. doi: 10.3390/agriculture11030194
Fan, Y., Feng, H., Yue, J., Jin, X., Liu, Y., Chen, R., et al. (2023). Using an optimized texture index to monitor the nitrogen content of potato plants over multiple growth stages. Comp. Electr. Agricult. 212:108147. doi: 10.1016/j.compag.2023.108147
Franzosa, E. A., McIver, L. J., Rahnavard, G., Thompson, L. R., Schirmer, M., Weingart, G., et al. (2018). Species-level functional profiling of metagenomes and metatranscriptomes. Nat. Methods 15, 962–968. doi: 10.1038/s41592-018-0176-y
Fuentes, S., and Chang, J. (2022). Methodologies used in remote sensing data analysis and remote sensors for precision agriculture. Sensors 22:7898. doi: 10.3390/s22207898
Galeano, R. M. S., Silva, S. M., Yonekawa, M. K. A., de Alencar Guimarães, N. C., Giannesi, G. C., Masui, D. C., et al. (2023). Penicillium chrysogenum strain 34-P promotes plant growth and improves initial development of maize under saline conditions. Rhizosphere 26:100710. doi: 10.1016/j.rhisph.2023.100710
García, L., Parra, L., Jimenez, J. M., Lloret, J., and Lorenz, P. (2020). IoT-based smart irrigation systems: an overview on the recent trends on sensors and iot systems for irrigation in precision agriculture. Sensors 20:1042. doi: 10.3390/s20041042
Garrido-Oter, R., Nakano, R. T., Dombrowski, N., Ma, K.-W., Team, A., McHardy, A. C., et al. (2018). Modular traits of the rhizobiales root microbiota and their evolutionary relationship with symbiotic rhizobia. Cell Host Microbe 24, 155–167.e5. doi: 10.1016/j.chom.2018.06.006
Gebbers, R., and Adamchuk, V. I. (2010). Precision agriculture and food security. Science 327, 828–831. doi: 10.1126/science.1183899
Giovannetti, M., di Fossalunga, A. S., Stringlis, I. A., Proietti, S., and Fiorilli, V. (2022). Unearthing soil-plant-microbiota crosstalk: looking back to move forward. Front. Plant Sci. 13:1082752. doi: 10.3389/fpls.2022.1082752
Granjou, C., and Phillips, C. (2019). Living and labouring soils: metagenomic ecology and a new agricultural revolution? Biosocieties 14, 393–415. doi: 10.1057/s41292-018-0133-0
Greening, C., Berney, M., Hards, K., Cook, G. M., and Conrad, R. (2014). A soil actinobacterium scavenges atmospheric H2 using two membrane-associated, oxygen-dependent [NiFe] hydrogenases. Proc. Natl. Acad. Sci. USA. 111, 4257–4261. doi: 10.1073/pnas.1320586111
Guan, Y., Grote, A., Schott, J., and Leverett, K. (2022). Prediction of soil water content and electrical conductivity using random forest methods with UAV multispectral and ground-coupled geophysical data. Remote Sensing 14:1023. doi: 10.3390/rs14041023
Guo, L., Shi, T., Chen, Y., Zhang, H., Meng, R., and Wang, S. (2020). Mapping field-scale soil organic carbon with unmanned aircraft system-acquired time series multispectral images. Soil and Tillage Res. 196:104477. doi: 10.1016/j.still.2019.104477
Hariprasad, P., Chandrashekar, S., Singh, S. B., and Niranjana, S. R. (2014). Mechanisms of plant growth promotion and disease suppression by Pseudomonas aeruginosa strain 2apa. J. Basic Microbiol. 54, 792–801. doi: 10.1002/jobm.201200491
Harman, G. E., Howell, C. R., Viterbo, A., Chet, I., and Lorito, M. (2004). Trichoderma species–opportunistic, avirulent plant symbionts. Nat. Rev. Microbiol. 2, 43–56. doi: 10.1038/nrmicro797
Hashmi, M. F., and Kesakr, A. G. (2023). Machine Learning and Deep Learning for Smart Agriculture and Applications. Pennsylvania: IGI Global.
Herzog, F., and Franklin, J. (2016). State-of-the-art practices in farmland biodiversity monitoring for North America and Europe. Ambio 45, 857–871. doi: 10.1007/s13280-016-0799-0
Hong, C., Manimaran, S., Shen, Y., Perez-Rogers, J. F., Byrd, A. L., Castro-Nallar, E., et al. (2014). PathoScope 2.0: a complete computational framework for strain identification in environmental or clinical sequencing samples. Microbiome 2:33. doi: 10.1186/2049-2618-2-33
Hong, S. W., Kim, D.-R., Kwon, Y. S., and Kwak, Y.-S. (2019). Genome-wide screening antifungal genes in Streptomyces griseus S4-7, a Fusarium wilt disease suppressive microbial agent. FEMS Microbiol. Letters 366:12. doi: 10.1093/femsle/fnz133
Hunt, M. L., Blackburn, G. A., Carrasco, L., Redhead, J. W., and Rowland, C. S. (2019). High resolution wheat yield mapping using Sentinel-2. Remote Sens. Environ. 233:111410. doi: 10.1016/j.rse.2019.111410
Huson, D. H., Auch, A. F., Qi, J., and Schuster, S. C. (2007). MEGAN analysis of metagenomic data. Genome Res. 17, 377–386. doi: 10.1101/gr.5969107
Hyatt, D., Chen, G. L., Locascio, P. F., Land, M. L., Larimer, F. W., and Hauser, L. J. (2010). Prodigal: prokaryotic gene recognition and translation initiation site identification. BMC Bioinformatics 11:119. doi: 10.1186/1471-2105-11-119
Jamil, A. (2021). Antifungal and plant growth promoting activity of Trichoderma spp. against Fusarium oxysporum f. sp. lycopersici colonizing tomato. J. Plant Protect. Res. 2021, 243–253. doi: 10.24425/jppr.2021.137950
Jamil, S., Ahmad, S., Shahzad, R., Umer, N., Kanwal, S., Rehman, H. M., et al. (2024). Leveraging multiomics insights and exploiting wild relatives' potential for drought and heat tolerance in maize. J. Agric. Food Chem. 72, 16048–16075. doi: 10.1021/acs.jafc.4c01375
Johann, A. L., de Araújo, A. G., Delalibera, H. C., and Hirakawa, A. R. (2016). Soil moisture modeling based on stochastic behavior of forces on a no-till chisel opener. Comp. Electr. Agricult. 121, 420–428. doi: 10.1016/j.compag.2015.12.020
Kang, Y., Walitang, D. I., Seshadri, S., Shin, W. S., and Sa, T. (2022). Methane oxidation potentials of rice-associated plant growth promoting Methylobacterium species. Korean J. Environm. Agricult. 41, 115–124. doi: 10.5338/KJEA.2022.41.2.15
Kefauver, S. C., Vicente, R., Vergara-Díaz, O., Fernandez-Gallego, J. A., Kerfal, S., Lopez, A., et al. (2017). Comparative UAV and field phenotyping to assess yield and nitrogen use efficiency in hybrid and conventional barley. Front. Plant Sci. 8:1733. doi: 10.3389/fpls.2017.01733
Khan, M. A., Khan, R., and Ansari, M. A. (2022). Application of Machine Learning in Agriculture. New York: Academic Press.
Killeen, P., Kiringa, I., Yeap, T., and Branco, P. (2024). Corn grain yield prediction using UAV-based high spatiotemporal resolution imagery, machine learning, and spatial cross-validation. Remote Sens. 16:683. doi: 10.3390/rs16040683
KriŽanović, V., Grgić, K., Spišić, J., and Žagar, D. (2023). An advanced energy-efficient environmental monitoring in precision agriculture using LoRa-based wireless sensor networks. Sensors 23:6332. doi: 10.3390/s23146332
Kumar, S., Stecher, G., Li, M., Knyaz, C., and Tamura, K. (2018). MEGA X: molecular evolutionary genetics analysis across computing platforms. Mol. Biol. Evol. 35, 1547–1549. doi: 10.1093/molbev/msy096
Langille, M. G. I., Zaneveld, J., Caporaso, J. G., McDonald, D., Knights, D., Reyes, J. A., et al. (2013). Predictive functional profiling of microbial communities using 16S rRNA marker gene sequences. Nat. Biotechnol. 31, 814–821. doi: 10.1038/nbt.2676
Laudari, S., Marks, B., and Rognon (2022). Classifying grains using behaviour-informed machine learning. Sci. Rep. 12:13915. doi: 10.1038/s41598-022-18250-4
Lewin, S., Wende, S., Wehrhan, M., Verch, G., Ganugi, P., Sommer, M., et al. (2024). Cereals rhizosphere microbiome undergoes host selection of nitrogen cycle guilds correlated to crop productivity. Sci. Total Environ. 911:168794. doi: 10.1016/j.scitotenv.2023.168794
Li, C., Li, H., Liu, Z., Li, B., and Huang, Y. (2021). SeedSortNet: a rapid and highly effificient lightweight CNN based on visual attention for seed sorting. PeerJ. Comp. Sci. 7:e639. doi: 10.7717/peerj-cs.639
Liakos, K. G., Busato, P., Moshou, D., Pearson, S., and Bochtis, D. (2018). Machine learning in agriculture: a review. Sensors 18:2674. doi: 10.3390/s18082674
Lindenstruth, F. (2020). “Spatio-temporal patterns of crop signals: is UAV-based multispectral imagery a suitable tool to detect soil compaction at field scale?,” in EGU General Assembly Conference Abstracts, p. 19619.
Liu, J., Zhu, Y., Tao, X., Chen, X., and Li, X. (2022). Rapid prediction of winter wheat yield and nitrogen use efficiency using consumer-grade unmanned aerial vehicles multispectral imagery. Front. Plant Sci. 13:1032170. doi: 10.3389/fpls.2022.1032170
Liu, P., Wang, X.-H., Li, J.-G., Qin, W., Xiao, C.-Z., Zhao, X., et al. (2015). Pyrosequencing reveals fungal communities in the rhizosphere of Xinjiang Jujube. Biomed Res. Int. 2015:972481. doi: 10.1155/2015/972481
Lynd, L. R., Weimer, P. J., van Zyl, W. H., and Pretorius, I. S. (2002). Microbial cellulose utilization: fundamentals and biotechnology. MMBR 66, 506–77. doi: 10.1128/MMBR.66.3.506-577.2002
Ma, Y., Chen, Z., Fan, Y., Bian, M., Yang, G., Chen, R., et al. (2023). Estimating potassium in potato plants based on multispectral images acquired from unmanned aerial vehicles. Front. Plant Sci. 14:1265132. doi: 10.3389/fpls.2023.1265132
Maddikunta, P. K. R., Hakak, S., Alazab, M., Bhattacharya, S., Gadekallu, T. R., Khan, W. Z., et al. (2021). Unmanned aerial vehicles in smart agriculture: applications, requirements, and challenges. IEEE Sens. J. 21, 17608–17619. doi: 10.1109/JSEN.2021.3049471
Mahapatra, S., Yadav, R., and Ramakrishna, W. (2022). Bacillus subtilis impact on plant growth, soil health and environment: Dr. Jekyll and Mr. Hyde. J. Appl. Microbiol. 132, 3543–3562. doi: 10.1111/jam.15480
Mahmoud, M., Zywicki, M., Twardowski, T., and Karlowski, W. M. (2019). Efficiency of PacBio long read correction by 2nd generation Illumina sequencing. Genomics 111, 43–49. doi: 10.1016/j.ygeno.2017.12.011
Martin, M. (2011). Cutadapt removes adapter sequences from high-throughput sequencing reads. EMBnet. J 17:10. doi: 10.14806/ej.17.1.200
Martínez-Porchas, M., and Vargas-Albores, F. (2017). Microbial metagenomics in aquaculture: a potential tool for a deeper insight into the activity. Rev. Aquacult. 9, 42–56. doi: 10.1111/raq.12102
Marvuglia, A., Bayram, A., Baustert, P., Gutiérrez, T. N., and Igos, E. (2022). Agent-based modelling to simulate farmers' sustainable decisions: farmers' interaction and resulting green consciousness evolution. J. Clean. Prod. 332:129847. doi: 10.1016/j.jclepro.2021.129847
Mazur, P., Gozdowski, D., Stepień, W., and Wójcik-Gront, E. (2023). Does drone data allow the assessment of phosphorus and potassium in soil based on field experiments with winter rye?. Agronomy 13:446. doi: 10.3390/agronomy13020446
McMurdie, P. J., and Holmes, S. (2013). phyloseq: an R package for reproducible interactive analysis and graphics of microbiome census data. PLoS ONE 8:e61217. doi: 10.1371/journal.pone.0061217
Meena, S. K., Kumar, A., Sinha, S., and Rana, L. (2024). “Advanced and emerging techniques in soil health management,” in Microorganisms for Sustainability. Singapore: Springer Nature Singapore, 343–362.
Mendes, R., Garbeva, A., and Raaijmakers, J. M. (2013). The rhizosphere microbiome: significance of plant beneficial, plant pathogenic, and human pathogenic microorganisms. FEMS Microbiol. Rev. 37, 634–663. doi: 10.1111/1574-6976.12028
Mohan, I., Joshi, B., Pathania, D., Dhar, S., and Bhau, B. S. (2024). Phytobial remediation advances and application of omics and artificial intelligence: a review. Environ. Sci. Pollut. Res. Int. 31, 37988–38021. doi: 10.1007/s11356-024-33690-3
Mohanty, S. P., Hughes, D. P., and Salathé, M. (2016). Using Deep Learning for Image-Based Plant Disease Detection. Front. Plant Sci. 7:1419. doi: 10.3389/fpls.2016.01419
Mohindru, V., Singh, Y., Bhatt, R., and Gupta, AK (2021). Unmanned Aerial Vehicles for Internet of Things (IoT): Concepts, Techniques, and Applications. Hoboken, NJ: John Wiley and Sons.
Morellos, A., Pantazi, X. E., Moshou, D., Alexandridis, T., Whetton, R., Tziotzios, G., et al. (2016). Machine learning based prediction of soil total nitrogen, organic carbon and moisture content by using VIS-NIR spectroscopy. Biosyst. Eng. 152, 104–116. doi: 10.1016/j.biosystemseng.2016.04.018
Nahvi, B., Habibi, J., Mohammadi, K., Shamshirband, S., and Al Razgan, O. S. (2016). Using self-adaptive evolutionary algorithm to improve the performance of an extreme learning machine for estimating soil temperature. Comp. Electr. Agricult. 124, 150–160. doi: 10.1016/j.compag.2016.03.025
Nehoshtan, Y., Carmon, E., Yaniv, O., Ayal, S., and Rotem, O. (2021). Robust seed germination prediction using deep learning and RGB image data. Sci. Rep. 11:22030. doi: 10.1038/s41598-021-01712-6
O'Callaghan, M., Ballard, R. A., and Wright, D. (2022). Soil microbial inoculants for sustainable agriculture: limitations and opportunities. Soil Use Managem. 38, 1340–1369. doi: 10.1111/sum.12811
Ojo, M. O., and Zahid, A. (2022). Deep learning in controlled environment agriculture: a review of recent advancements, challenges and prospects. Sensors 22:7965. doi: 10.3390/s22207965
Ondov, B. D., Bergman, N. H., and Phillippy, A. M. (2011). Interactive metagenomic visualization in a Web browser. BMC Bioinform. 12:385. doi: 10.1186/1471-2105-12-385
Oulas, A., Pavloudi, C., Polymenakou, P., Pavlopoulos, G. A., Papanikolaou, N., Kotoulas, G., et al. (2015). Metagenomics: tools and insights for analyzing next-generation sequencing data derived from biodiversity studies. Bioinform. Biol. Insights 9, 75–88. doi: 10.4137/BBI.S12462
Papin, M., Polrot, A., Breuil, M. C., Czarnes, S., Zigha, A., Roux, X., et al. (2024). Pre-sowing recurrent inoculation with Pseudomonas fluorescens promotes maize growth. Biol. Fertility Soils 1–16. doi: 10.1007/s00374-024-01873-2
Peng, W., Li, X., Song, J., Jiang, W., Liu, Y., and Fan, W. (2018). Bioremediation of cadmium- and zinc-contaminated soil using Rhodobacter sphaeroides. Chemosphere 197, 33–41. doi: 10.1016/j.chemosphere.2018.01.017
Peng, Y., Leung, H. C. M., Yiu, S. M., and Chin, F. Y. L. (2011). Meta-IDBA: a de Novo assembler for metagenomic data. Bioinformatics 27, i94–101. doi: 10.1093/bioinformatics/btr216
Philippot, L., Chenu, C., Kappler, A., Rillig, M. C., and Fierer, N. (2024). The interplay between microbial communities and soil properties. Nat. Rev. Microbiol. 22, 226–239. doi: 10.1038/s41579-023-00980-5
Philippot, L., Raaijmakers, J. M., Lemanceau, P., and van der Putten, W. H. (2013). Going back to the roots: the microbial ecology of the rhizosphere. Nat. Rev. Microbiol. 11, 789–799. doi: 10.1038/nrmicro3109
Prasad, R., and Zhang, S.-H. (2022). Beneficial Microorganisms in Agriculture. 1st edn. Singapore, Singapore: Springer (Environmental and Microbial Biotechnology).
Ramcharan, A., Baranowski, K., McCloskey, P., Ahmed, B., Legg, J., and Hughes, D. P. (2017). Deep learning for image-based cassava disease detection. Front. Plant Sci. 8:293051. doi: 10.3389/fpls.2017.01852
Reda, R., Saffaj, T., Ilham, B., Saidi, O., Issam, K., and Brahim, L. (2019). A comparative study between a new method and other machine learning algorithms for soil organic carbon and total nitrogen prediction using near infrared spectroscopy. Chemomet. Intellig. Laborat. Syst. 195:103873. doi: 10.1016/j.chemolab.2019.103873
Robinson, M. D., McCarthy, D. J., and Smyth, G. K. (2010). edgeR: a Bioconductor package for differential expression analysis of digital gene expression data. Bioinformatics 26, 139–140. doi: 10.1093/bioinformatics/btp616
Sabale, S. N., Suryawanshi, P. P., and Krishnaraj, P. U. (2020). “Soil metagenomics: concepts and applications,” in Metagenomics - Basics, Methods and Applications. London: IntechOpen.
Santamaria, M., Fosso, B., Licciulli, F., Balech, B., Larini, I., Grillo, G., et al. (2018). ITSoneDB: a comprehensive collection of eukaryotic ribosomal RNA internal transcribed spacer 1 (ITS1) sequences. Nucleic Acids Res. 46, D127–D132. doi: 10.1093/nar/gkx855
Santos, M. S., Nogueira, M. A., and Hungria, M. (2019). Microbial inoculants: reviewing the past, discussing the present and previewing an outstanding future for the use of beneficial bacteria in agriculture. AMB Express 9:205. doi: 10.1186/s13568-019-0932-0
Sedlacek, C. J., Nielsen, S., Greis, K. D., Haffey, W. D., Revsbech, N. P., Ticak, T., et al. (2016). Effects of bacterial community members on the proteome of the ammonia-oxidizing bacterium nitrosomonas sp. strain is79′, Appl. Environm. Microbiol. 82, 4776–4788. doi: 10.1128/AEM.01171-16
Shafi, U., Mumtaz, R., García-Nieto, J., Hassan, S. A., Zaidi, S. A. R., and Iqbal, N. (2019). Precision agriculture techniques and practices: from considerations to applications. Sensors 19:3796. doi: 10.3390/s19173796
Shameem, M., Sonali, J., Kumar, P. S., Rangasamy, G., Gayathri, K. V., and Parthasarathy, V. (2023). Rhizobium mayense sp. Nov., an efficient plant growth-promoting nitrogen-fixing bacteria isolated from rhizosphere soil. Environm. Res. 220:115200. doi: 10.1016/j.envres.2022.115200
Sharma, A., Jain, A., Gupta, P., and Chowdry, V. (2021). Machine learning applications for precision agriculture: A comprehensive review. IEEE Access 9, 4843–4873. doi: 10.1109/ACCESS.2020.3048415
Singer, E., Vogel, J. P., Northen, P., Mungail, C. J., and Juenger, T. (2021). Novel and emerging capabilities that can provide a holistic understanding of the plant root microbiome. Phytobiomes J 5, 122–132. doi: 10.1094/PBIOMES-05-20-0042-RVW
Song, Q., Gao, X., Song, Y., Li, Q., Chen, Z., Li, R., et al. (2023). Estimation and mapping of soil texture content based on unmanned aerial vehicle hyperspectral imaging. Sci. Rep. 13, 14097. doi: 10.1038/s41598-023-40384-2
Starkenburg, S. R., Chain, P. S., Sayavedra-Soto, L. A., Hauser, L., Land, M. L., Larimer, F. W., et al. (2006). Genome sequence of the chemolithoautotrophic nitrite-oxidizing bacterium Nitrobacter winogradskyi Nb-255. Appl. Environm. Microbiol. 72, 2050–2063. doi: 10.1128/AEM.72.3.2050-2063.2006
Streich, J., Romero, J., Gazolla, J. G. F. M., Kainer, D., Cliff, A., Prates, E. T., et al. (2020). Can exascale computing and explainable artificial intelligence applied to plant biology deliver on the United Nations sustainable development goals? Curr. Opin. Biotechnol. 61, 217–225. doi: 10.1016/j.copbio.2020.01.010
Suchithra, M. S., and Pai, M. L. (2020). Improving the prediction accuracy of soil nutrient classification by optimizing extreme learning machine parameters. Inform. Proc. Agricult. 7, 72–82. doi: 10.1016/j.inpa.2019.05.003
Sun, K. (2020). Ktrim: an extra-fast and accurate adapter- and quality-trimmer for sequencing data. Bioinformatics 36, 3561–3562. doi: 10.1093/bioinformatics/btaa171
Sun, S., Jones, R. B., and Fodor, A. A. (2020). Inference-based accuracy of metagenome prediction tools varies across sample types and functional categories. Microbiome 8:46. doi: 10.1186/s40168-020-00815-y
Tahir, M. N., Lan, Y., Zhang, Y., Wenjiang, H., Wang, Y., and Naqvi, S. M. Z. A. (2023). “Application of unmanned aerial vehicles in precision agriculture,” in Precision Agriculture (New York: Academic Press), 55–70.
Too, E. C., Yujian, L., Njuki, S., and Yingchun, L. (2019). A comparative study of fine-tuning deep learning models for plant disease identification. Comp. Electr. Agricult. 161, 272–279. doi: 10.1016/j.compag.2018.03.032
van Bruggen, A. H. C., Sharma, K., Kaku, E., Karfopoulus, S., Zelenev, V., and Blok, W. (2015). Soil health indicators and Fusarium wilt suppression in organically and conventionally managed greenhouse soils. Appl. Soil Ecol. 86, 192–201. doi: 10.1016/j.apsoil.2014.10.014
van der Velde, M., See, L., You, L., Balkovi,č, J., Fritz, S., Khabarov, N., et al. (2013). Affordable nutrient solutions for improved food security as evidenced by crop trials. PLoS ONE 8:e60075. doi: 10.1371/journal.pone.0060075
Walsh, C. M., Gebert, M. J., Delgado-Baquerizo, M., Maestre, F. T., and Fierer, N. (2019). A global survey of mycobacterial diversity in soil. Appl. Soil Ecol. 85:17. doi: 10.1128/AEM.01180-19
Weaver, R. W. (1994). Methods of Soil Analysis, Part 2: Microbiological and Biochemical Properties. ACSESS. Madison: Soil Science Society of America, 985–1017.
Weller, D. M., Raaijmakers, J. M., Gardener, B. B. M., and Thomashow, L. S. (2002). Microbial populations responsible for specific soil suppressiveness to plant pathogens. Annu. Rev. Phytopathol. 40, 309–348. doi: 10.1146/annurev.phyto.40.030402.110010
Yang, C.-Y., Yang, M. D., Tseng, W. C., Hsu, Y. C., Li, G. S., Lai, M. H., et al. (2020). Assessment of rice developmental stage using time series UAV imagery for variable irrigation management. Sensors 20:5354. doi: 10.3390/s20185354
Yang, M., Xu, D., Chen, S., Li, H., and Shi, Z. (2019). Evaluation of machine learning approaches to predict soil organic matter and pH using vis-NIR spectra. Sensors 19:263. doi: 10.3390/s19020263
Zeng, Y., Verhoef, A., Vereecken, H., Ben-Dor, E., Veldkamp, T., Shaw, L., et al. (2024). Tracking soil health: monitoring and modeling the soil-plant system. ESS Open Archive (preprint). doi: 10.22541/essoar.171804479.91646868/v1
Zhang, L., Chen, F., Zeng, Z., Xu, M., Sun, F., Yang, L., Bi, X., et al. (2021). Advances in metagenomics and its application in environmental microorganisms. Front. Microbiol. 12:766364. doi: 10.3389/fmicb.2021.766364
Zhang, Y., Han, W., Zhang, H., Niu, X., and Shao, G. (2023). Evaluating soil moisture content under maize coverage using UAV multimodal data by machine learning algorithms. J. Hydrol. 617:129086. doi: 10.1016/j.jhydrol.2023.129086
Zhang, Z., Liu, H., Yang, C., Ampatzidis, Y., Zhou, J., and Jiang, Z. (2022). Unmanned Aerial Systems in Precision Agriculture: Technological Progresses and Applications. Cham: Springer Nature.
Keywords: soil metagenomics, remote sensing, unmanned aerial vehicle, artificial intelligence, precision agriculture
Citation: Ambaru B, Manvitha R and Madas R (2025) Synergistic integration of remote sensing and soil metagenomics data: advancing precision agriculture through interdisciplinary approaches. Front. Sustain. Food Syst. 8:1499973. doi: 10.3389/fsufs.2024.1499973
Received: 23 September 2024; Accepted: 12 December 2024;
Published: 06 January 2025.
Edited by:
Kaline Arnauts, KU Leuven, BelgiumReviewed by:
Marika Pellegrini, University of L'Aquila, ItalyCopyright © 2025 Ambaru, Manvitha and Madas. This is an open-access article distributed under the terms of the Creative Commons Attribution License (CC BY). The use, distribution or reproduction in other forums is permitted, provided the original author(s) and the copyright owner(s) are credited and that the original publication in this journal is cited, in accordance with accepted academic practice. No use, distribution or reproduction is permitted which does not comply with these terms.
*Correspondence: Bindu Ambaru, YmluZHUuYW1iYXJ1QGdtYWlsLmNvbQ==
Disclaimer: All claims expressed in this article are solely those of the authors and do not necessarily represent those of their affiliated organizations, or those of the publisher, the editors and the reviewers. Any product that may be evaluated in this article or claim that may be made by its manufacturer is not guaranteed or endorsed by the publisher.
Research integrity at Frontiers
Learn more about the work of our research integrity team to safeguard the quality of each article we publish.