- 1Department of Public Management, Ethiopian Civil Service University, Addis Ababa, Ethiopia
- 2Department of Development Management, Ethiopian Civil Service University, Addis Ababa, Ethiopia
- 3Department of Policy Studies, Ethiopian Civil Service University, Addis Ababa, Ethiopia
It’s challenging to predict the potential impacts of mining extraction on household food security. On the one hand, mining projects can create job opportunities, improve market access, and enhance infrastructure. On the other hand, these projects can have adverse effects on livelihood assets, such as farmland, livestock, and grazing areas, which are crucial for household food security. The purpose of this article is to bridge the gap by studying impacts of mining projects on household food security in Ethiopia, using empirical evidence from the Benishangul-Gumuz Region (BGR). To achieve this goal, we employed a cross-sectional and quasi-experimental research designs. Using systematic random sampling, we collected primary data from a sample of 333 households, comprising 162 from a community located around the mining projects (treated households) and 171 from a community without mining projects in the area (control households). We analyzed the data using descriptive statistics and Propensity Score Matching (PSM) techniques. We measured food availability by assessing food energy intake (FEI). We evaluated food utilization by using the food consumption score (FCS), and we determined food stability through the coping strategy index (CSI). The Average Treatment Effect on the Treated (ATT) results show that treated households, on average, consume 1,407.34 kcal per adult per day and 8.54 more FCS per week compared to control households, respectively. The ATT results also showed that treated households had a 3.24 lower CSI than control households. This is due to the wage employment created by small-scale and the infrastructure built by large-scale mining projects in the study area. Our research indicates that expanding mining investments brings benefits, as long as local sustainable development is taken into account.
1 Introduction
In Ethiopia, recent developments in mineral investments have resulted in a growth of both domestic and foreign mining investors (Djigsa, 2021). In recent years, mining investments have increasingly become a vital component of Ethiopia’s economy. Similarly, the Benishangul-Gumuz Region (BGR), which is the specific focus of this study, has significant potential for precious construction and industrial minerals (Bullock and Morgan, 2018; Ministry of Mines, 2014). The BGR government has initiated efforts to facilitate the transition from informal ASM to legal small-scale mining investment projects (Hassen, 2022). As a result, currently, more than 396 mining projects are working in gold, marble, and coal production (Benishangul Gumuz Region Mining Agency (BGRMA), 2022).
Mining projects can significantly affect impact local communities in both positive and negative ways (Aristizabal-H et al., 2023). The positive effects include the creation of employment opportunities, which can enhance household incomes (Moritz et al., 2017). Conversely, the negative effects may involve soil degradation (Kinda and Thiombiano, 2021), water pollution (Ofosu and Sarpong, 2022), displacement of local communities (Arhin et al., 2022). These mixed impacts can directly or indirectly influence food security at the household level. Therefore, understanding the complex relationship between mining activities and food security is essential for evaluating their overall impact on households.
FAO et al. (2012) define food security as the availability, utilization, and stability of food to meet dietary needs for a healthy and active life. It is a multifaceted issue influenced by various socio-economic and environmental factors (Mekuria, 2019). Ethiopia, in particular, faces significant challenges in achieving food security because of the adverse effects of both human-induced and climate-related changes on agricultural productivity (Abegaz, 2017). The BGR state, characterized by its agrarian economy and diverse nature, is particularly vulnerable to these challenges (Aysheshim et al., 2022). In addition, the recent introduction of large-scale mining investments in the region adds another layer of complexity to the region’s food security dynamics.
Despite the increasing of mining, the impact of mining projects on the food security of host communities in developing countries is a topic of ongoing debate. However, there is limited research on this subject. The existing literature provides some valuable insights. For example, a study by Yadav et al. (2019) sheds light on this issue that industrial mining has improved food availability of affected households than non-affected households. In their study, they compared villages affected by industrial mining with those that were not. However, they did not control for covariates, such as livelihood assets, which significantly impact household food security. Consequently, it is difficult to conclude whether the improvement in food security was because of the presence of industrial mining or not.
Moreover, Wegenast and Beck (2020) examined the effects of mining on food security in sub-Saharan African countries. Their study found that mining operations tend to decrease food availability for women, while increasing access for men. Additionally, the presence of foreign companies reduces food availability, whereas domestic companies increase the availability of food for households. However, their study did not explain why domestic companies contribute to increased food availability, while foreign mining companies do not. Additionally, they did not clarify why the impacts on food security were gender specific.
In the context of Ethiopia, there is a lack of research examining the impacts of mining projects on food security of the host community. However, studies have been conducted on the impacts of large-scale agricultural investments, which can offer valuable insights. For example, Guyalo et al. (2021) discovered that large-scale agricultural investments in the Gambela region resulted in decreased food security for households due to land displacements and reduced access to natural resources. Similarly, Bekele et al. (2021) reported that large-scale agricultural investments also reduce food security for households in agro-pastoral areas of Ethiopia. These findings emphasize the potential risks associated with significant changes in land use in rural areas of Ethiopia. Despite this, both studies focus on the large-scale agricultural investments, not on the mining investments.
The BGR offers a unique opportunity to examine the impacts of mining projects on household food security. With an abundance of mineral resources, the region attracts both domestic and foreign investors who are keen on extracting coal, gold, and marble minerals. Most of the mining investors in the area are small scale and owned by domestic investors (Benishangul Gumuz Region Mining Agency (BGRMA), 2022). These small-scale mining companies lack advanced technology and heavily rely on labor. These projects created local employment for the host community and increased the average income of households (Haile et al., 2024a). This sets the BGR mining sector apart from the large-scale mining sector, which requires less labor and relies on more advanced technologies. Surprisingly, researchers have conducted no studies to investigate the impact of mining on household food security in the BGR. Understanding these dynamics is crucial for developing policies that can effectively balance economic development with the food security needs of the local population.
Therefore, the objective of this study is to evaluate the impact of mining projects on food security of households in the BGR state of Ethiopia. The study aims to answer specific research questions, including:
I. How do mining projects impact the food availability of households in the Benishangul-Gumuz Regional state of Ethiopia?
II. How do mining projects impact the food utilization of households in the study area?
III. How do mining projects impact the food stability of households for a short time in the study area?
This study aims to investigate the impacts of mining projects on food availability, utilization and stability of host community in the BGR state of Ethiopia. Achieving food security is a significant challenge for communities in this region (Aysheshim et al., 2022). Factors such as household land size, non-farm income, and irrigation land play crucial roles in determining food security in the BGR (Mohammed and Mohammed, 2021), and these factors directly or indirectly can be influenced by mining project operations. Thus, it is evident that the relationship between mining projects and food security is complex and multifaceted. This study aims to untangle these complexities by providing a comprehensive analysis of how mining projects impact household food security in the region. Following this introduction section, we have organized this paper as follows: We provide an overview of our research methods and materials. Section 3 demonstrates the results obtained. Section 4 shows the discussion of the result, and finally Section 5 a synthetic conclusion highlighting the main findings and policy implications of the study.
2 Methods
2.1 Description of the study area
We conducted this study in the Benishangul-Gumuz Regional (BGR) state of Ethiopia, as shown in Figure 1. This state is located in the northwest part of Ethiopia and is 1 of 12 regional states in the country. The region is divided into three administrative zones; 22 woredas; one special woreda; three city administrations; and 535 Kebeles (Benishangul-Gumuz Region Bureau of Finance and economic Development (BGRBoFED), 2020). The region is estimated to have a total area of 50,380 km2 and a population density of 22 people per km2 in 2022 CSA projection (CSA, 2013). It has a varied vegetation cover and ranges in elevation from 558 to 2,729 m above sea level. The average daily temperature is between 20 and 25°C. Nonetheless, 75% of its terrain is referred to as lowland, and its highest daily temperature can reach 34°C in the hottest months (Benishangul-Gumuz Region Bureau of Finance and economic Development (BGRBoFED), 2020). The region shares a common administrative border with Amhara regional state in the north and northeast, with Oromiya regional state in the south, southeast, and southwest, and with Sudan in the west.
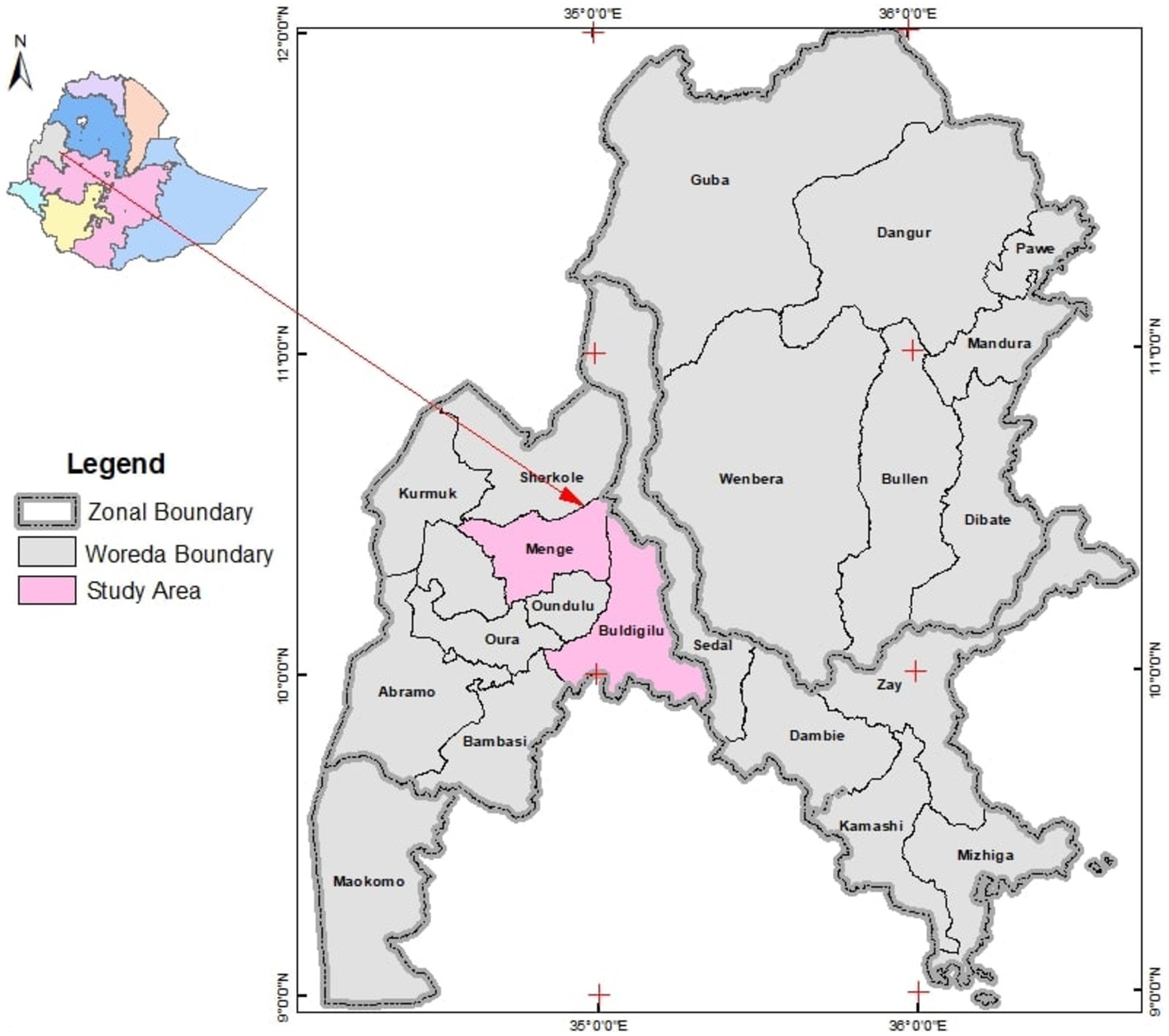
Figure 1. Map of Benishangul Gumuz, with selected districts. Source: Benishangul-Gumuz Region Bureau of Finance and economic Development (BGRBoFED) (2022).
According to CSA (2013) projections in 2022, the population of the region was estimated at 1,219,000, of which 619,000 (50.78%) were males and 600,000 (49.22%) were females. The CSA projection shows that 77% of the region’s population will live in rural areas in 2022 and the remaining 23% will live in urban areas (CSA, 2013). The regional economy is largely dependent on the agriculture sector, including crops, livestock, and forestry. As well, in the region, traditional gold mining has been an additional source of livelihood for most of the rural community. Many farmers still rely on plowing by hand or oxen. In recent years, foreign and local investors have acquired large amounts of land for agricultural and mining activities (Moreda, 2017). However, the transfer of large amounts of land for investors affects local people’s natural resource-based livelihood strategies that require large tracks of land and related resources for crop production, free grazing of livestock, forest collection, and various non-timber forest products such as forest honey, forest fruits, and root crops (Aysheshim et al., 2023).
The data collection specifically took place in the Asosa Zone, focusing on the Menge and Buldigilu districts. Menge district consists of 22 Kebeles, while the Buldigilu district has 30 Kebeles. Although there has not been a recent population census conducted in the country, the Federal Central Statistical Authority (CSA) projects a total population of 55,900 (49.5% female) and 78,383 (47% female) in Menge and Buldigilu districts, respectively, for 2022 (CSA, 2013). The household economies in this area heavily rely on the mixed agriculture sector, which includes forests, cattle, and crops (Teklemariam et al., 2016). Moreover, traditional gold mining has served as an additional source of livelihood for most rural communities in these districts (Hassen, 2022).
2.2 Research design
We used both cross-sectional and quasi-experimental research designs, which are non-experimental research designs. There is no need for a true control group in quasi-experiments, but a control group may be included if a comparison group is chosen (Rogers and Révész, 2020). Demographic and socio-economic characteristics of the control (comparison) group should be as similar as possible to those of the treatment group (Béné et al., 2018). Therefore, by considering the spillover effects of mining investment practices and different empirical evidence (Assan and Muhammed, 2018; Yamarak and Parton, 2021), we classified the treatment and control groups based on the distances of Kebeles in the sample districts from the mine sites. By considering empirical evidence and the effects of the mining project, the treatment households are households that are living within a 10 km radius of mining projects. In comparison, control households are households that are living within more than a 30 km radius of mining projects in the area.
2.3 Sampling technique and sample size
We conducted multi-stage sampling procedures to select sample respondents. First, Buldigilu and Menge districts from the Asosa Zone of BGR were selected purposefully for the reason that they have had a concentration of small-scale and large-scale mining projects for more than 10 years of operation (Benishangul Gumuz Region Mining Agency (BGRMA), 2022). In the second stage, from each selected study district, two Kebeles that are within a 10 km radius of the mining project practices were selected as a treatment group, and two other Kebeles that have more than a 30 km radius from any type of mining project practices were selected as control groups. In both the control and treatment Kebeles, the majority of the households are indigenous people (Benishangul Gumuz Region Bureau of Finance and economic Development (BGRBoFED), 2022). Based on this, the sample population was drawn proportionately from eight selected Kebeles in the two sample districts. Thus, Shegol and Bane Kebeles in Menge district and Beletseri and Campodisha Kebeles in Buldigilu district were selected as treatment Kebeles. In addition, Alimetema and Abora Kebeles from Menge district and Billanjaro and Bellaganda Kebeles from Buldigilu district are selected as comparison (control) Kebeles (Table 1).
Finally, the lists of households in eight selected Kebeles were used as the sampling frame. In this study, we employed the Cochran (1977) sample size determination technique because of the importance of probability sampling techniques in selecting households from a sampling frame. The study population for the study in the eight Kebeles that are purposefully selected are 3,500 (Benishangul Gumuz Region Bureau of Finance and economic Development (BGRBoFED), 2020).
Assuming the maximum unpredictability is equal to 50% (p = 0.5), and following a 95% confidence level with 5% precision, we calculate the required sample size as follows: p = 0.5, q = 1–0.5 = 0.5; e = 0.05; and z = 1.96.
The formula is given as . Where an attribute’s estimated proportion in the population, q = 1 − p, and e is the desired level of precision, with n0 = sample size, z = selected critical value, p = proportion in the population, and e = level of precision selected.
According Cochran (1977), a sample size reduction is necessary if the population is finite and the sample size exceeds 5% of the sample population, or 175 in this case. He suggested using the following adjustment method to get the ultimate sample size in this scenario.
Where, n is the corrected sample size no is the calculated sample size in the above equation, and N is the total sample population. Using this formula, the sample size is 351 households. This 351 study households was distributed to the eight Kebeles using the proportional to size formula. We drew the sample from the aforementioned sample areas through systematic random sampling with the formula where N is the total population, n is the required sample size, and k is the size of the interval for selection. Thus, 351 sample households were selected from sampling frame, and the selection process is using systematic random sampling, which proceeds at every 10th interval.
2.4 Types and sources of data
We collected both quantitative and qualitative types of data from both primary and secondary data sources. The primary data were collected by administering a survey questionnaire and conducting focus group discussions (FDGs). We also collected secondary data through document review from different published and unpublished official government reports. We pre-tested the questionnaire to adjust the original questionnaire thanks to the feedback of pilot participants. The data collection activity was undertaken with a time interval of January to May 2023. Stata version 14.0 was used to analyze the collected questionnaires. Both closed- and open-ended types of questions were included in the questionnaire that was utilized for this research. Almost 95.0% (333 households) of the survey questionnaires were completed and appropriate for analysis.
2.5 Measuring household food security
There are several ways to assess a household’s level of food security, and no single, ideal technique exists measuring food security (Bekele et al., 2021). At the household and individual level, indicators such as household consumption and expenditure survey (HCES), coping strategy index (CSI), household dietary diversity score (HDDS), household food insecurity access scale (HFIAS), food energy intake (FEI), and anthropometric weight and height measurements are commonly used techniques for measuring food security (Yohannes and Hoddinott, 2002). Thus, for this study, we used three indicators that are related to the impacts of mining on households’ food security: food energy intake (FEI), food consumption score (FCS), and coping strategy index (CSI). Each indicator is discussed hereunder.
The Food Energy Intake (FEI): The FEI measures the number of calories consumed by an adult attains over a day (Hoddinott, 1999). This is a measure of food availability in the households. To determine the FEI, we took daily calorie consumption as the measure of food security. The steps used for measurement are discussed hereunder.
The first step was listing all food items consumed by households in kilograms and liters. This data was crucial for understanding the quantity of food available for energy calculation. The second step involved converting all reported quantities from kilograms and liters into grams. To ensure consistency in measurement units for further calculation, we multiplied the amounts by 1,000. The third step was calculating the energy intake from the food consumed. We utilized the Ethiopian Health and Nutritional Research Institute’s (EHNRI) conversion and determination system (1997), which provides specific energy values for various foods based on their composition. 100 g typically expressed these values in kilocalories. Dercon and Krishnan adjust finally, the total kilo calories consume (1998) for Ethiopia (Dercon and Krishnan, 1998). This allowed the researcher to calculate the average kilocalories per adult equivalent per day, providing a clear indication of energy intake relative to the household consumption pattern (example: Equation 1).
Where, FEIi = Household food energy intake of the ith household, and i = 1, 2, 3,…. 333, which is the sample size of the study.
As per MoFED (2002), a household in Ethiopia is considered food insecure if the daily calorie intake per adult equivalent is less than 2,200. Conversely, a household is classified as food secure if the daily calorie intake per adult equivalent exceeds 2,200. For this study, households were divided into two categories: food insecure (those who did not meet the minimum requirement of 2,200 kcal per day per adult) and food secures (those who consumed at least 2,200 kcal per day per adult).
The Food Consumption Score (FCS): The FCS measures the diversity of diet, frequency of food intake, and the relative nutritional value of food groups (FAO et al., 2014). This is a measure of food utilization in the household. According to FAO et al. (2014), FCS scores of 28, 28.5–42, and 42.5 fall into three consumption groups: poor, borderline, and acceptable for sugar and oil frequently consumed areas. The BGR is one of the sugar consumption areas in Ethiopia (Mohammed and Mohammed, 2021). We collected data on food consumption from 333 households in the study area and captured the variety and frequency of foods consumed during the 7-day recall period. We calculated the frequency of consumption of food groups based on the seven recall period. We obtained the weight from the WFP (2008) weight allocation. If a household consumes all types of food for all 7 days, the maximum FCS will be 112 (example: Equation 2).
The Coping Strategy Index (CSI): The CSI is a household food insecurity measurement used in emergency situations (Maxwell, 2008). This is a measure of food vulnerability or stability of households. It provides insight into the capabilities and vulnerabilities of households over a short period of time (Hoddinott, 1999). Maxwell’s (2008) coping strategy index was changed and adapted to the local situation, and 11 commonly used coping strategies were identified through FGD (see Appendix Table A1). The main coping mechanisms included: relying on less preferred and less expensive foods; borrowing food or relying on help from a friend or relative; limiting portion size at mealtimes; reducing the number of meals eaten in a day; going without food the whole day; sending household members to eat elsewhere; gathering wild food, hunting, or harvesting immature crops and fruits; selling firewood and charcoal; selling productive livestock; engaging in traditional gold mining practices; and working as a daily laborer. To calculate the CSI, we multiplied the frequency and severity weight of each coping technique for each household. A higher CSI score indicates a greater severity of food insecurity (Maxwell, 2008). Respondents were asked about their actions when they do not have enough money to buy meals in the past 7 days. Coping mechanisms are applicable in the short term to address food shortages (example: Equation 3).
Where (f_i) represents the relative frequency of the coping strategy and (s_i) indicates the severity level (weight) of that coping strategy.
2.6 Methods of data analysis
As a method of analysis, we employed descriptive, inferential statistics, and econometric models. Graphs, percentages, frequencies, and means are used for description. The T-test and Chi-Square tests helped us ponder the existence of the mean difference between the treatment and control samples. The PSM model was used to examine the impact of mining projects on food security of households.
2.7 Analytical approach
We used the PSM technique to determine the impacts of mining projects on the food security of households in the study area. The lack of reliable baseline data for various variables of interest, such as demographics, socio-economic factors, livelihood assets, and food security makes the matching technique the preferred method for this study. In recent years, PSM has been applied to a wide range of topics to identify the causal effects of policies, development programs, and investments in developing countries like Ethiopia. Therefore, the propensity score matching procedure is primarily used in this study because of data availability.
To assess the impact of mining projects on food security, households were classified as treated (if located in a mining area) or controlled (if located in a non-mining area). Because there is no randomization between the treated and comparison groups, the PSM method is used to calculate the impact. Propensity scores are generally calculated using two methods: logistic regression and classification and regression tree analysis (Thavaneswaran and Lix, 2008). Logistic regression is the most common method for estimating PSM. Additionally, to analyze the impact of mining on households’ livelihood outcomes, the average impact of treatment on the treated (ATT) is estimated using the PSM technique. The detailed steps and procedures for implementing PSM are discussed below.
According to Caliendo and Kopeing (2005), in using PSM, there are five commonly used steps. The first step in implementing PSM is to estimate the propensity score. This process entails selecting an appropriate estimation model and identifying the relevant variables to include. In this study, we employed the logit model to estimate the propensity score while analyzing the impacts of mining projects on food security. The theoretical framework for using a logit model in the context of PSM to assess the impacts of mining investments on household livelihoods is grounded in the principles of causal inference. The logit model can be expressed as follows (example: Equation 4):
Where, is the probability that a household livelihood improves (Y = 1) given the vector of covariates X. is the intercept term. s are the coefficients corresponding to each covariate
The second step is to choose among different matching estimators. There are various approaches to implementing PSM, including nearest neighbor matching (NNM), radius matching (RM), stratification or interval matching, kernel matching (KM), and the weighting method. It’s important to note that there is no perfect algorithm without weaknesses. For this study, all matching methods were employed to analyze and compare the impact of mining on livelihood assets and food security of households. The best method was selected by comparing the standards discussed below. The third step is to check for overlap or common support. This ensures that individuals or groups with the same values for characteristic X have a positive probability of being both treated and controlled in a program or treatment. It enables the comparison of comparable units.
The fourth important step is to check for matching quality and conduct an effect analysis. Several techniques are available to check balancing, including mean comparisons between treatment and comparison groups (before and after matching), standardized bias, and overall measures of covariate imbalance. It’s also important to compare the pseudo-R2 before and after matching, as this provides a method for testing the matching quality (Thavaneswaran and Lix, 2008). A low pseudo-R2 after matching indicates the success of the matching process (Caliendo and Kopeing, 2005). Finally, the average treatment effect on the treated (ATT) can be estimated using the following steps (example: Equation 5):
Li represents the livelihood impact of mining on household “i.” The outcome for a household with and without treatment is denoted as “i,” and , respectively. Let us consider a binary indicator where 1 represents being assigned to treatment and 0 represents not being assigned treatment. The Average Treatment Effects (ATE) can be estimated using the following equation (example: Equation 6):
The Average Treatment Effect (ATE) refers to the average difference between households in mining areas (treated households) and households in non-mining areas (control households). However, if the selection of treatment and other factors are correlated with both the treatment itself and an omitted variable that influences the outcome variable, a simple comparison may not accurately represent the true impact of the treatment. The main issue arises from the fact that each household is either under treatment or control, but never both simultaneously. In such cases, a more preferred parameter to use instead of ATE is the average treatment effect on the treated (ATT). The ATT is defined as (example: Equation 7):
Where failing to observe the value of, and substituting it with the expected value of , which is observable in ATE, will result in an inaccurate estimate as long as for the treated and comparison group systematically differs. The true parameter is only identified if (example: Equation 8):
However, it is unlikely that this will remain valid in non-experimental studies. Therefore, this study employs a matching approach to create a counterfactual scenario, allowing treated households to be paired with non-treated households that share similar characteristics. This approach helps minimize bias that may arise from self-selection. The matching process is based on a measure called the propensity score, which captures the pre-treatment characteristics of each household. The propensity score indicates the probability of being assigned to treatment, denoted as P(x), based on a set of pre-treatment characteristics, denoted as X. This can be represented as (example: Equation 9):
Finally, we conducted the sensitivity analyses as a crucial step. Sensitivity analysis is important in order to identify any unobserved variables that may introduce hidden bias to the treatment and outcome variables (Caliendo and Kopeing, 2005). Therefore, Rosenbaum-bound sensitivity analysis employed to assess the presence of hidden bias.
2.8 Variable definition
We collected the data using survey questionnaires and FGDs in both Menge and Buldigilu districts of BGR. Detailed information on household demographics, socio-economic characteristics, livelihood assets and food security data was collected. Variable names, descriptions, and expected signs are presented in Table 2.
3 Results
3.1 Demographic and socio-economic characteristics of household groups
The results of Table 3 indicated that the sex of household heads, education level of household heads, irrigation, access to extension services, membership in farmers’ organizations, and non- or off-farm activity between treatment and control groups were statistically significant. On the other hand, there was no statistically significant difference between the two groups in access to credit service, and access to information. Table 3 shows that of the total 331 households in the study, 261 (78.5%) were male households. The result also shows that the majority, 185 (58.2%) of households, have no formal education. Of these illiterate households, 100 (58.5%) were control households, and 94 (58%) were treated households. In addition, the results of the study illustrated that about 42.7% of control households and 33.4% of treated households had access to irrigation, respectively. Regarding the accessibility of credit, 53.7% of respondents from the treatment group and 53.2% of the control households had access to credit services, respectively. Regarding access to agricultural extension, the treatment and control groups’ percentage distributions were 40 and 61%, respectively.
Moreover, Table 3 revealed that 74.9% of control households and 56.2% of treated households were members of farmers’ organizations. The result of access to information (having a personal mobile) shows that 58.5% of control households and 66% of treated households had access to information. Furthermore, 37.7% of treated households and 14.7% of control households had non- or off-farm activity. The results of the chi-square test indicated that there was a significant difference between groups in sex of household head, access to extension, membership in farmers’ organizations, and non- or /off-farm activity at less than 1%, and the education level of household head and irrigation access at a 10% significance level, respectively.
Furthermore, Table 4 illustrated that there was a significant difference between the treatment and control groups in their household size, dependency ratio, farmland size, and tropical livestock unit (TLU). On the other hand, the result indicated that there was no significant difference between the two groups in age of respondents. The total sample household age ranges from 19 to 58, meaning that the respondents were between the ages of 19 and 58; the mean age is 39. This shows that the majority of the respondents are in productive age groups. The mean ages of the treated and control households were 38.80 and 40.07, respectively. This implies that younger people are in the treated groups. The average family size of treated and control sample households is 7.57 and 8.22, respectively, implying that relatively fewer families are in the treated households. In addition, the mean dependency ratio shows that for treated households it is 1.945 with a standard deviation of 1.30 and for control households it is 2.25 with a standard deviation of 1.42, respectively. The mean farmland size of treated households is 2.34 with a standard deviation of 1.34, and the mean farmland size of control households is 3.07 with a standard deviation of 0.96, respectively. The mean livestock holdings of the treatment and control households were 5.12 and 6.58 total livestock units (TLU), respectively. The t-test showed that there was a significant difference between the treatment and control groups in farmland size, and TLU at a less than 1% significance level, whereas the dependency ratio was at 5% and household size at a 10% significance level, respectively.
3.2 Descriptive and summary statistics of food security status
The mean calorie consumption of treated and control households was 3,633.8 and 2,129.5 kcal/AE/day, respectively. The mean calorie consumption per day of treatment households is statistically greater than that of the control groups at a 1% significance level. The mean FCS of treatment and control households was 45.3 and 35.6, respectively. The mean FCS of treatment households is statistically greater than the FCS of control groups at a 1% statistically significance level. Table 5 also shows that the mean result of CSI is 11.7 and 16.57 for treated and control households, respectively. The mean CSI difference of treated households and control households is statistically significance at less than 1% level.
According to the FEI method of measuring food security, out of the total sample of households in the study area (n = 333), 172 (51.65%) were food secure, and the remaining 161 (48.34%) were food insecure (Table 6). Thus, of the 162 treated households, 95 (58.6%) were found to be food-secure, whereas 67 (41.4%) were found to be food-insecure. On the other hand, out of 171 control households, 77 (45%) were found to be food secure, whereas 94 (55%) of the households were found to be food insecure. Moreover, Table 6 shows the results of households’ food security status using FCS as the treatment and control groups. The FCS cutoff given showed 95 (58.6%) of treated households were food secure because their FCS exceeded the 42 acceptable cutoffs, whereas 63 (38.9%) of the households had FCSs between 28.5 and 42, which made them borderline food secure, whereas 4 (2.5%) had FCSs below 28 and were food insecure. On the other hand, 59 (34.5%) of the control households had FCS of 42.5 and above, which are food secure; 79 (46.2%) of them had FCS between the range of 28.5 and 42, and hence, they were borderline food secure; and 33 (19.3%) of the control households have been poorly food secure.
3.3 Propensity score matching estimates
We have used binary logit model to examine the propensity scores. For this model, we included about 13 variables as potential confounders. The model output shows that seven variables were significant (see Table 7). The positively significant variables were access to access to information at 10% significance, and on- non-farm activity at 1% significance level. The negatively related significant variables were sex of respondents at 1% significance level, the dependency ratio at 5% significance level, farmland size at 1% significance level, tropical livestock unit at 1% significance, access to extension service at 1% significance level, and membership in farmers organizations at 1% significance level.
3.3.1 Matching treated and control households
This section of the study describes the distribution of propensity scores (Figure 2), the estimate of common support ratings (Figure 3) for the treatment and control households, and the performance requirements of the matching algorithms (see Appendix Table A2). In this model, the radius (0.25) confirms the best and most appropriate matching algorithm for the data, which yields a lower pseudo-R2 and a large matched sample size. Prior to matching being conducted, seven out of the 13 chosen variables showed a statistically significant difference, while after matching; all covariates showed a statistically insignificant difference (see Appendix Table A2). This supports the finding that the matching procedure significantly contributes to balancing the covariate distribution in the matched sample.
After the models are estimated, it is crucial to check if the model assumptions actually hold. The performance criteria of the matching algorithms are evaluated using the distribution of the propensity scores and the score estimation of the common support of the treatment and control households in Figures 2, 3. Figure 2 shows that the treated sample (blue) and the control sample (red) are matching in the middle of the graph, so that the final sample that is used in the matching is very similar on average to the original sample. Additionally, the figure shows a high concentration of propensity scores for control households below 0.2, while there are a significant number of observations in the treated groups with propensity scores above 0.8.
Figure 3 demonstrates a high likelihood of obtaining good matches, with the propensity score distribution skewed to the left for treated households and to the right for controlled households. Out of the 333 households in the sample, 154 were treated households and 164 were control households that fell within the common support region (Figure 3). This means that 318 households fell within this range. To ensure adequate overlap in the characteristics of the treated and control groups, we removed seven observations from the control group (indicated in blue in Figure 3) and eight observations from the treated group (indicated in yellow in Figure 3). This adjustment was made to ensure sufficient matches for accurately estimating the effects on treated households while still maintaining the integrity of the common support region.
3.3.2 The average treatment effect on the treated (ATT)
The results of the ATT of the FEI, FCS, and CSI food measurement indicators are displayed in Table 8.
3.4 Sensitivity analysis
We conducted the Rosenbaum bounding approach to analyze the sensitivity. The Rosenbaum bounding approach indicates a low likelihood of hidden bias, in the PSM (see Appendix Table A3). The Rosenbaum bound sensitivity analysis of the outcome showed that the impact of mining on food security of untreated households is not sensitive to hidden bias at p < 0.001.
4 Discussion
The literature and empirical evidence suggest that predicting the effects of mining projects on household food security is challenging (Wegenast and Beck, 2020). On the one hand, mining creates job opportunities; which can enhance the food security of households. However, on the other hand, the operations of mining projects can lead to the marginalization of farmers and also adverse effects on the health of affected communities, potentially impacting food security. Therefore, it is important to study the impacts of mining projects on household food security. This study examined the impact of mining projects on the food security of households in the Benishangul-Gumuz Regional state of Ethiopia. We used villages unaffected by mining projects as comparison (control) households because we did not have baseline data available for treated households before the investments. Both descriptive and econometric models were used to analyze the impacts of mining projects on households’ food security. The study’s results indicated that living near mining projects positively impacted household food security in terms of availability, utilization, and stability, as evidenced by multiple food security indicators (FEI, FCS, and CSI).
The first measurement used to assess food security in this study was the Food Energy Intake (FEI) approach, which evaluates the availability of food within households. The measurement revealed a lower percentage of food shortages in areas affected by mining projects (treatment) compared to unaffected areas (control households). According to the FEI method, 58.6% of treated households were food-secure, while only 45% of control households were. Thus, treated households displayed higher levels of food security according to the FEI indicator. However, the study found that approximately half of the total sample respondents experienced food insecurity. Treated households consumed an average of 3,607.20 kcal/AE/day, while control households consumed only 2,199.86 kcal/AE/day. The average ATT differences indicated that treated households consumed approximately 1,407.34 kcal more per adult per day than control households. The ATT findings validated that mining projects had a statistically significant positive impact on the food security of the host community, as assessed by the FEI indicator, with a significance level below 1%.
This study demonstrated that the presence of these projects led to an increase in household food availability in the BGR. This enhancement can be attributed to the development of essential infrastructures, including, roads, markets, and other physical amenities. Haile et al. (2024b) contend that mining projects have significantly improved the basic physical needs and amenities of host community in the region. This aligns with Yadav et al. (2019), who found that the presence of industrial mining operations increased food availability for impacted households compared to non-impacted households. However, the effects of mining projects on food availability may vary by gender and ownership type, as indicated by Wegenast and Beck (2020). Their research suggests that while mining projects can boost food availability for male households, they may have a negative impact on female-headed ones. Additionally, domestic mining projects tend to have a more positive effect on food availability than those foreign companies.
Furthermore, the study by Bekele et al. (2021) emphasizes how large-scale investments, such as agricultural projects can contribute to improved food availability by improving road infrastructure and increasing market accessibility. These findings collectively suggest that the presences of these projects enhance food availability of households due to the improved infrastructures and improved market accessibility. However, it is crucial to consider the potential gender based disparities and the influence of ownership type when assessing the impacts of mining on food availability.
The food consumption score (FCS) was the second food security measurement used in this study to assess household food utilization. The FCS measurements revealed that treated households had higher levels of food security than control households. Of the treated households, 58.6% were food-secure, while only 34.5% of control households achieved food security. Additionally, only 2.5% of treated households and 19.5% of control households were categorized as poor regarding their food security status. These results indicate a significant difference in food security between treated and control households when using FCS measures. Furthermore, the mean FCS for treated households was 45.16 per week, whereas control households had an average FCS of 36.62 per week. The average ATT differences revealed that treated households had 8.54 more FCS per week than control households did. Regarding the FCS food security indicator, the findings show that treated households had better food security compared to control households. The ATT results confirm that mining projects have a statistically significant positive influence on the food security of the host community through FCS measurements, with a significance level of less than 1%. Therefore, the study suggests that mining projects improve household nutrition quality.
The study demonstrates that mining projects significantly enhance the food utilization of households in the BGR. This improvement can be attributed to the employment opportunities by small-scale mining project initiatives, which provide households with additional income to purchase food. Furthermore, the establishments of market driven by these projects have facilitated greater access to food, thereby increasing overall consumption levels. Hlatshwayo et al. (2023) supports this finding, noting that improved market access and participation positively influence food consumption score. Similarly, a research by Fitawek and Hendriks (2021) found that employment opportunities created by large-scale agricultural investments lead to increased household food consumption score of the host community. However, this findings contradict with the study conducted by Guyalo et al., 2022, which found that large-scale agricultural investments negatively affected the food consumption score in some contexts. This discrepancy highlights the complex and context dependent nature of relationships between investment type and consumptions scores.
Finally, the third food security measurement used for this study was the coping strategy index (CSI). The CSI serves as a measure of food vulnerability or stability within households. The study revealed that treated households had a lower CSI compared to control households, based on the CSI food measurement method. The mean CSI of treated households was 11.77 CSI/month, while control households had 15.02 CSI/month. The differences indicated that treated households had a CSI up to 3.24 units lower than control households. This indicates that treated households relied less on coping mechanisms for food shortages, indicating a higher level of food security. In both mining and non-mining areas, the most commonly adopted strategy for dealing with food shortages was to rely on less preferred and cheaper food options. The ATT findings support the notion that mining investments have a statistically significant positive impact on the food security of the host community, as assessed by the CSI indicator at a significance level of less than 1%. Therefore, the study conclusively demonstrates that treated households exhibit greater food stability compared to control households.
The study shows that mining projects significantly enhance food stability and lower the household coping index in the BGR. This improvement results from increased employment opportunities and livelihood diversification linked to mining activities. Haile et al. (2024a) note that wage employment in small-scale mining and entry-level positions in large-scale mining lead to higher average household incomes in the region. Furthermore, Haile et al. (2024b) argue that these projects improve both the financial and physical capital of households by creating wage employment opportunities and developing physical infrastructure. Consequently, households gain better access to resources, positively impacting food stability and overall wellbeing.
This study suggests that living proximity to mining projects leads to a decrease in the coping index of households. This may be due to the fact that working for mining companies lowers the CSI of households. These results align with the findings of Fitawek and Hendriks (2021), who also observed that employment in large-scale investments reduces the coping index of households. However, Bekele et al. (2021) conducted a study and concluded that the proximity to large-scale agricultural investments in rural areas has no impact on the coping strategies of households.
Overall, the study found that mining projects positively affect the food security of surrounding households, as evidenced by the three food security measurements: food availability, utilization and stability of households increase with the presence of mining investments in the study area. The empirical findings suggest that the presence of mining projects contribute to household food security, supporting the notion that such investments contribute significantly to the wellbeing of local communities. This aligns with a research by Yamarak and Parton (2021), which found that mining projects in Papua New Guinea improved the food quality and security of indigenous communities. Similarly, Yadav et al. (2019) highlighted that households in non-affected villages faced a 40% higher risk of food shortage compared to households in mine-affected villages in the coal mining industry. This phenomenon is largely attributed to the increased employment opportunities in mining regions, which enhances household disposable income at the expense of local agriculture production.
5 Conclusions and policy implications
This article examines the impact of mining projects on food security of households in the Benishangul-Gumuz Regional State of Ethiopia. Existing empirical evidence suggests that the impact of mining projects on household food security is a subject of debate. Therefore, studying the impacts of mining projects on food security is important. To mitigate potential bias from unobserved factors that affect both the treatment and outcome variables, such as livelihood and food security indicators, we used a propensity score-matching (PSM) econometric model.
From this background, we estimated the impact of mining projects on households’ food security in the BGR. We used a total sample of 333 households, including 162 from communities with mining projects (treated households) and 171 from communities without mining projects (control households). We conducted PSM to protect against selection bias and misleading results caused by unobservable factors that affect the treatment and outcome variables, such as food security indicators.
The descriptive result indicates that, compared to control households, treated households are better off in terms of mean total FEI and FCS and lower CSI. As well, the ATT result indicates that treated households had 1,404.45 kcal more per adult per day and 8.52 more FCS per week than control households did. Furthermore, the ATT result shows that treated households have a CSI that is up to 3.22 lower than the CSI that control households had. The study result shows that in all food security indicators, the mean ATT difference between treated and control groups is statistically significant at a level of less than 1% significance. This shows that, in all measurements of food security, mining projects affected food security positively.
These results can be attributed to the contributions of mining investments to economic development through the generation of employment opportunities, both directly and indirectly. The influx of mining-related income enhances the purchasing power of households, enabling them to acquire a more diverse array of foodstuffs. Moreover, the economic stimulus provided by these investments can lead to broader improvements in infrastructure services, facilitating greater access to markets and food distribution networks.
The positive impacts on food security are further amplified by the multiplier effects of mining investments. As mining-related income is spent and circulated within the local economy, it generates additional employment and income opportunities, known as indirect and induced effects. This economic ripple effect contributes to the overall improvement in household food security in the study area.
In conclusion, the findings underscore the potential of mining investments to positively impact food security by increasing income, diversifying food sources, and improving access to markets. However, it is crucial to note that these benefits are not automatically guaranteed and may vary depending on the specific context and implementation of mining projects. Policymakers and stakeholders should strive to maximize the positive impacts of mining investments on food security while mitigating any potential negative consequences through comprehensive and inclusive development strategies.
The outcomes of our study provide two recommendations concerning policy implications. First, the positive effects of mining projects on livelihood outcomes shows that the government’s mining investment is desirable and needs to expand and attract potential investors in BGR and Ethiopia. The second policy recommendation is that Ethiopia needs to establish appropriate institutional, legal, and policy frameworks to mitigate the adverse effects of extractive mining investments that should align with local sustainable development.
6 Limitations and future research
This study has several limitations. One significant limitation is the absence of baseline data on key household livelihoods and the nonrandom selection of respondents. These issues affect both the results and the application of the experimental research design. To address these limitations, the researcher could have utilized a more robust methodology, such as an experimental research design, which would have yielded more accurate results for this dissertation. Future research should incorporate baseline data, employ a longitudinal research design, and adopt experimental approaches to enhance the validity of the findings. Additionally, another limitation of this study was the small sample size used for the survey and focus group discussions (FGDs). This constraint may influence the study’s results. Future researchers should consider using a larger sample size and broader geographic area to mitigate such limitations.
Data availability statement
The raw data supporting the conclusions of this article will be made available by the authors, without undue reservation.
Ethics statement
Ethical review and approval was not required for the study on human participants in accordance with the local legislation and institutional requirements. Written informed consent from the [patients/participants OR patients/participants legal guardian/next of kin] was not required to participate in this study in accordance with the national legislation and the institutional requirements.
Author contributions
NH: Conceptualization, Data curation, Formal analysis, Funding acquisition, Investigation, Methodology, Project administration, Resources, Software, Supervision, Validation, Visualization, Writing – original draft, Writing – review & editing. AH: Conceptualization, Data curation, Formal analysis, Investigation, Methodology, Software, Supervision, Writing – original draft, Writing – review & editing. AB: Conceptualization, Investigation, Software, Writing – original draft, Writing – review & editing.
Funding
The author(s) declare that no financial support was received for the research, authorship, and/or publication of this article.
Acknowledgments
The authors extend their heartfelt gratitude to reviewers for their careful reading of the manuscript and their helpful comments that improved the presentation of this work. The authors also thank the households for the data source access. We also thank Ethiopian Civil Service University (ECSU) for their kind help with internet and other material access.
Conflict of interest
The authors declare that the research was conducted in the absence of any commercial or financial relationships that could be construed as a potential conflict of interest.
Publisher’s note
All claims expressed in this article are solely those of the authors and do not necessarily represent those of their affiliated organizations, or those of the publisher, the editors and the reviewers. Any product that may be evaluated in this article, or claim that may be made by its manufacturer, is not guaranteed or endorsed by the publisher.
References
Abegaz, K. H. (2017). Determinants of food security: evidence from Ethiopian rural household survey (ERHS) using pooled cross-sectional study. Agric. Food Secur. 6, 1–7. doi: 10.1186/s40066-017-0153-1
Arhin, P., Erdiaw-Kwasie, M. O., and Abunyewah, M. (2022). Displacements and livelihood resilience in Ghana’s mining sector: the moderating role of coping behaviour. Resour. Policy 78:102820. doi: 10.1016/j.resourpol.2022.102820
Aristizabal-H, G., Goerke-Mallet, P., Kretschmann, J., and Restrepo-Baena, O. J. (2023). Sustainability of coal mining. Is Germany a post-mining model for Colombia? Resour. Policy 81:103358. doi: 10.1016/j.resourpol.2023.103358
Assan, J. K., and Muhammed, A.-R. (2018). The impact of mining on farming as a livelihood strategy and its implications for poverty reduction and household well-being in Ghana. Int. J. Dev. Sustain. 7, 1–20.
Aysheshim, A., Ayal, D. Y., and Mulugeta, M. (2023). Assessing the impact of villagization program on land use land cover dynamics in Benishangul-Gumuz, Western Ethiopia. J. Degrad. Min. Lands Manag. 11, 4837–4845. doi: 10.15243/jdmlm.2023.111.4837
Aysheshim, A., Yayeh, D., and Mulugeta, M. (2022). Determinants of household food security in the Benishnagul-Gumuz region, Western Ethiopia. Ethiopian J. Dev. Res. 14, 174–188. doi: 10.3329/sja.v14i2.31257
Bekele, A. E., Dries, L., Heijman, W., and Drabik, D. (2021). Large scale land investments and food security in agropastoral areas of Ethiopia. Food Secur. 13, 309–327. doi: 10.1007/s12571-020-01131-x
Béné, C., Riba, A., and Wilson, D. (2018). Measuring changes in resilience as a result of the SUR1M project in Niger. 1–47. Available at: https://itad.com/wp-content/uploads/2018/05/DFID-BRACED_EA3-Impact-Evaluation_Niger_Final-for-DFID.pdf (Accessed June 11, 2022).
Benishangul Gumuz Region Bureau of Finance and Economic Developemnt (BGRBoFED). (2020). The population projection of the Benishangul Gumuz region for budget allocation.
Benishangul Gumuz Region Bureau of Finance and Economic Development (BGRBoFED) (2022). Benishangul Gumuz regional state physical and socio-economic profile. May, 1–130.
Bullock, L. A., and Morgan, O. (2018). The Asosa region of western Ethiopia: a golden exploration opportunity. Geol. Today 34, 31–34. doi: 10.1111/gto.12217
Caliendo, M., and Kopeing, S. (2005). Some practical guidance for the implementation of propensity score matching. J. Agric. Sci. 158, 313–325. doi: 10.1017/S0021859620000593
CSA (2013). Population projections for Ethiopia 2007–2037. Ethiopia: Central Statistical Agency Population, 188.
Dercon, S., and Krishnan, P. (1998). Changes in poverty in rural Ethiopia 1988–1995: measurement, robustness, test and decomposition : Center for the Study of African Economies, Institute of Economics and Statistics, University of Oxford.
Djigsa, W. K. (2021). Towards socially responsible Mining Investment in Ethiopia: imagining a new moral economy. Rev. Public Admin. Manag. 9, 1–6.
FAO, IFAD, & WFP. (2014). Food and Agriculture Organization of the United Nations. International Fund for Agricultural Development. World food Programme. The state of food insecurity in the world. Strengthening the enabling environment for food security and nutrition. Available at: https://www.fao.org/3/i4030e/i4030e.pdf (Accessed October 7, 2022).
FAO, WFP, & IFAD. (2012). The state of food insecurity in the world - executive summary. 4. Available at: https://www.fao.org/3/i2845e/i2845e00.pdf (Accessed December 3, 2023).
Fitawek, W., and Hendriks, S. L. (2021). Evaluating the impact of large-scale agricultural investments on household food security using an endogenous switching regression model. Land 10:323. doi: 10.3390/land10030323
Guyalo, A. K., Alemu, E. A., and Degaga, D. T. (2021). Impact of large-scale agricultural investment on the livelihood assets of local community in Gambella region, Ethiopia. Int. J. Soc. Econ. 48, 363–383. doi: 10.1108/IJSE-09-2020-0610
Guyalo, A. K., Alemu, E. A., and Degaga, D. T. (2022). Impact of large - scale agricultural investments on the food security status of local community in Gambella region, Ethiopia. Agric. Food Secur. 11:43. doi: 10.1186/s40066-022-00381-6
Haile, N. Z., Huluka, A. T., and Beyene, A. B. (2024a). Analysis of the factors influencing local employment in the mining sector in the Benishangul-Gumuz Regional state of Ethiopia. Scientific African. e02364. doi: 10.1016/j.sciaf.2024.e02364
Haile, N. Z., Huluka, A. T., and Beyene, A. B. (2024b). The impact of mining projects on household livelihoods in the Benishangul-Gumuz region, Ethiopia. Miner. Econ. doi: 10.1007/s13563-024-00471-w
Hassen, A. E. (2022). Competency oriented management for sustainable artisanal and small-scale mining sector development in Ethiopia. Nat. Res. Forum 46, 116–135. doi: 10.1111/1477-8947.12246
Hlatshwayo, S. I., Ojo, T. O., and Ngidi, M. S. C. (2023). Effect of market participation on the food and nutrition security status of the rural smallholder farmers: the case of Limpopo and Mpumalanga provinces, South Africa. Front. Sustain. Food Syst. 7, 1–13. doi: 10.3389/fsufs.2023.1097465
Hoddinott, J. (1999). Choosing outcome indicators of household food security! Technical guide #7! vol. 55. 2033 K Street, N.W. Washington, D.C. 20006 U.S.A: International Food Policy Research Institute, 815–844.
Kinda, H., and Thiombiano, N. (2021). The effects of extractive industries rent on deforestation in developing countries. Resour. Policy 73:102203. doi: 10.1016/j.resourpol.2021.102203
Maxwell, D. (2008). The coping strategies Ingix guideline a tool for measurement of household food security and the impact of aid programes in humaniterian emeregency; field method manual. Educ. Psychol. Meas. 73, 412–439. doi: 10.1177/0013164412465875
Mekuria, D. G. (2019). Food security in Ethiopia: review. Int. J. Res. Stud. Agric. Sci. 5, 1–7. doi: 10.20431/2454-6224.0501001
Ministry of Mines. (2014). Strategic assessment of the Ethiopian mineral sector, final report. Available at: https://openknowledge.worldbank.org/handle/10986/20585 (Accessed February 10, 2022).
MoFED. (2002). Ethiopian sustainable poverty reduction program 2002-2005. Ministry of Finance and Economic Development, July. Available at: https://www.imf.org/external/np/prsp/2002/eth/01/073102.pdf (Accessed May 22, 2023).
Mohammed, S., and Mohammed, A. (2021). Determinants of food security: the case of Kurmuk District in Benishangul Gumuz regional state, Western Ethiopia. Int. J. Nat. Resour. Ecol. Manag. 6:16. doi: 10.11648/j.ijnrem.20210601.14
Moreda, T. (2017). Large-scale land acquisitions, state authority and indigenous local communities: insights from Ethiopia. Third World Q. 38, 698–716. doi: 10.1080/01436597.2016.1191941
Moritz, T., Ejdemo, T., Söderholm, P., and Wårell, L. (2017). The local employment impacts of mining: an econometric analysis of job multipliers in northern Sweden. Miner. Econ. 30, 53–65. doi: 10.1007/s13563-017-0103-1
Ofosu, G., and Sarpong, D. (2022). Beyond the doom: sustainable water management practices of small-scale mining operations. Resour. Policy 77:102649. doi: 10.1016/j.resourpol.2022.102649
Rogers, J., and Révész, A. (2020). Experimental and quasi-experimental designs. Phys. Rev. B 111, 133–143.
Teklemariam, D., Azadi, H., Nyssen, J., Haile, M., and Witlox, F. (2016). How sustainable is transnational farmland acquisition in Ethiopia? Lessons learned from the Benishangul-Gumuz region. Sustainability (Switzerland) 8, 1–27. doi: 10.3390/su8030213
Thavaneswaran, A., and Lix, L. (2008). Propensity score matching in observational studies. Canada, Manitoba: Centre for Manitoba Health Policy.
Wegenast, T., and Beck, J. (2020). Mining, rural livelihoods and food security: a disaggregated analysis of sub-Saharan Africa. World Dev. 130:104921. doi: 10.1016/j.worlddev.2020.104921
WFP. (2008). Food consumption analysis calculation and use of the food consumption score in food security analysis Version 1, VA M Unit, Rome. Available at: https://resources.vam.wfp.org/data-analysis/quantitative/food-security/food-consumption-score
Yadav, A. K., Bhagat, R. B., and Yadav, V. (2019). Does coal mining affect household food security? An empirical study of Dhanbad, India. Agric. Food Secur. 8, 1–12. doi: 10.1186/s40066-018-0244-7
Yamarak, L., and Parton, K. A. (2021). Impacts of mining projects in Papua New Guinea on livelihoods and poverty in indigenous mining communities. Miner. Econ. 36, 13–27. doi: 10.1007/s13563-021-00284-1
Yohannes, Y., and Hoddinott, J. (2002). Dietary diversity as a household food security indicator. 136, 1–43. Available at: http://www.fantaproject.org/research/dietary-diversity-household-food-security (Accessed June 19, 2022).
Appendix A
Keywords: impact evaluation, mining projects, food security, propensity score matching, Benishangul-Gumuz Region
Citation: Haile NZ, Huluka AT and Beyene AB (2024) Impacts of mining projects on food security of households in Ethiopia: empirical evidence from Benishangul-Gumuz Region. Front. Sustain. Food Syst. 8:1481827. doi: 10.3389/fsufs.2024.1481827
Edited by:
F. E. Jalal, King Fahd University of Petroleum and Minerals, Saudi ArabiaReviewed by:
Ahsan Naseem, Ghent University, BelgiumOmer Altaf, Otago Polytechnic Auckland, New Zealand
Copyright © 2024 Haile, Huluka and Beyene. This is an open-access article distributed under the terms of the Creative Commons Attribution License (CC BY). The use, distribution or reproduction in other forums is permitted, provided the original author(s) and the copyright owner(s) are credited and that the original publication in this journal is cited, in accordance with accepted academic practice. No use, distribution or reproduction is permitted which does not comply with these terms.
*Correspondence: Netsanet Zeleke Haile, emVsZWtlbjM5QGdtYWlsLmNvbQ==
†ORCID: Netsanet Zeleke Haile, orcid.org/0009-0006-5831-2762
Admassu Tesso Huluka, orcid.org/0000-0002-1946-0977
Amsalu Bedemo Beyene, orcid.org/0000-0002-0317-0844