- 1Division of Agricultural Extension, ICAR-Indian Agricultural Research Institute, New Delhi, India
- 2Division of Agricultural Extension, Indian Council of Agricultural Research, New Delhi, India
- 3Division of Social Science, ICAR-National Rice Research Institute, Odisha, India
- 4Division of Agricultural Economics, ICAR-Indian Agricultural Research Institute, New Delhi, India
- 5Division of Statistics, ICAR-Indian Agricultural Research Institute, Assam, India
- 6ICAR-Central Soil Salinity Research Institute, Regional Research Station, West Bengal, India
- 7ICAR-Indian Institute of Millets Research, Telengana, India
Introduction: Research into various aspects of vulnerability and poverty has played a key role in shaping cash transfer systems. Cash-based direct benefit transfers (DBT) are frequently discussed, as they help reduce corruption and limit intermediary involvement, which often impedes policy implementation in countries like India. Small and marginal farmers (SMFs), constituting 86% of India’s farming population, are essential for sustainable agricultural growth and ensuring food and nutrition security. The Pradhan Mantri Kisan Samman Nidhi (PM-KISAN) scheme provides Rs.6000 annually to the farmer families via DBT mechanism. Despite reports and information from various government sources, significant concerns remain regarding the performance of PM-KISAN scheme in improving living conditions of SMFs.
Methods: For having the highest number of SMFs, a research study was conducted in Bihar and Uttar Pradesh. Two cluster of villages from each of these two states were chosen by simple random sampling (SRS) method. An ex post facto research design was used; data was gathered from 240 SMFs, with 120 beneficiaries and 120 non-beneficiaries, using a stratified disproportionate simple random sampling technique. The study used 18 indicators related to livelihood assets—human capital, social capital, natural capital, physical capital, and financial capital—to generate a Livelihood Index (LI) through Principal Component Analysis (PCA).
Results: The beneficiaries of the scheme had an average LI score of 0.396, while non-beneficiaries had a score of 0.366. Propensity Score Matching (PSM) was employed to compare the beneficiaries and non-beneficiaries in order to determine the impact of the scheme. In the Livelihood Index of beneficiaries, in comparison to non-beneficiaries, there was a significant improvement of 3.34 to 4.13 percentage points, according to the analysis, which utilized a variety of matching algorithms.
Discussion: Implications from the study suggests that PM-KISAN should be integrated into a broader rural development strategy to maximize multiplier effects. By combining cash transfers with complementary initiatives like modern technology adoption and high-yield seeds, farmers can make productive investments that could enhance their livelihood status.
1 Introduction
Cash transfers, a type of social protection, are non-contributory payments sent directly, regularly, and predictably to raise and stabilize earnings in order to minimize poverty and vulnerability. The assumption that unprivileged people are empowered and trusted to effectively utilize the resources available to improve their living conditions when they receive income transfers, whether conditional or unconditional, is the basis of such policy intervention. The Organization for Economic Cooperation and Development (OECD, 2009) defined social protection as policies and activities that directly improve poor people’s capacity to overcome poverty and effectively manage risks and shocks. In order to accomplish the Sustainable Development Goal 2 (SDG2), which aims to eliminate malnutrition and hunger by 2030 through the encouragement of sustainable agriculture, governments around the globe have been advancing a variety of policies. The food insecure and rural poor are primarily targeted by social security programmes in general and cash transfer policies in particular.
Cash transfers have a history that dates back to the last century, and in recent decades, they have become a widely adopted anti-poverty measure in developing countries (Bertrand, 2003; Boone et al., 2013; Manley et al., 2013; Paes-Sousa and Santos, 2009; Sadoulet et al., 2001; Olken, 2019). On average, these nations dedicate 1 to 2% of their GDP to these programs, which have become a vital source of income for many low-income households (Grosh et al., 2008). The primary objectives of these investments are to increase the real income and living standards of poor and vulnerable households by reducing poverty and improving human capital, such as children’s education and health. In situations where households experience limited and unstable income, cash transfers can help stabilize consumption by maintaining spending on essentials like food, education, and healthcare, thereby avoiding harmful coping strategies (Arnold et al., 2011). These interventions are designed to alleviate poverty, vulnerability, and risks, and can be carried out by the state, non-governmental organizations, or the private sector. Despite the global emphasis on social protection, 71% of the world’s population still has limited or no access to a comprehensive social protection system, hindered by factors such as demographic changes, slow economic growth, migration, conflict, and environmental challenges (ILO, 2017). Research indicates that cash transfer programs have a significant impact on reducing poverty and vulnerability, especially through social transfers that target the poorest households and help narrow inequality gaps (Leroy et al., 2009; Cooper et al., 2020).
Cash transfers started in Brazil in the mid-1980s. While cash transfer systems were initially implemented in different localities to address unique challenges, they were subsequently incorporated into the “Bolsa Familia” scheme in 2004. The program has succeeded in reducing inequality and poverty in Brazil over the years (Vyasulu, 2010). Many countries—the United States, Mexico, Latin America, Southeast Asia, and a few African republics—adopted the technique as Conditional Cash Transfers (CCT) following the program’s success. The largest and most effective conditional cash distribution system in the world is the Bolsa Familia Program. CCTs in Mexico, such as Bolsa Famlia and Oportunidades, covered about 12 and 5 million low-income families in 2010, respectively (Fajth and Vinay, 2010). Conditional cash transfer programmes aimed at increasing human capital investment and discovered that when households are provided unconditional cash, they would consume less conditioned commodities while consuming more non-conditioned goods (Das et al., 2005). For over 50 years, cash transfers have played a significant role in reducing poverty in developed nations; they were thought to be excessive or unfeasible in nations with lower incomes (Bryant, 2009). However, the success of such programs in countries like Brazil, where inequality has decreased through the Conditional Cash Transfer (CCT) program “Bolsa-Familia,” one of the largest CCT programs globally, has shown otherwise (Scorzafave and Lima, 2010). In order to improve livelihood standards, corruption, inequality, and poverty, a number of countries have adopted the notion of giving social benefits to their citizens.
Although there are notable differences within and between countries, quantitative impact evaluations of cash transfer programs in countries like Zambia (Daidone et al., 2014a, 2014b), Malawi (Boone et al., 2013; Covarrubias et al., 2012), Kenya (Asfaw et al., 2014), and Lesotho (Daidone et al., 2014a, 2014b) show that easing credit, liquidity, and savings constraints can boost agricultural and livestock production. In Ethiopia, too, it is more likely that beneficiaries adopt advanced agricultural technologies (Gilligan et al., 2008). Additionally, these studies emphasize how cash transfers give households greater freedom to make choices about how to allocate labor; the effects vary according to location, age, and gender. For example, studies indicate that cash transfers can cause a shift in labor from casual wage work to work on beneficiaries’ own small farms (Asfaw et al., 2014; Davis et al., 2016; Bastagli et al., 2019). This shift also includes a decrease in child labor in programs aimed at orphans and vulnerable children, such as those in Kenya and Lesotho (Bosworth et al., 2016; Pellerano et al., 2016), as well as in broader programs targeting the ultra-poor, like those in Malawi (Miller and Tsoka, 2012; Miller et al., 2010).
In addition to boosting farm production, cash transfers have been shown to increase investments in income-generating activities, particularly in Zambia (Daidone et al., 2014a, 2014b) and among women in Kenya (Asfaw et al., 2014), as well as in Malawi and Lesotho (Boone et al., 2013; Daidone et al., 2014a, 2014b). Cash transfers can also enhance employment opportunities for prime-aged adults by facilitating labor migration, as seen in South Africa (Ardington et al., 2009; Zizzamia, 2020). Cash transfers and migration, on the other hand, vary according to the region. For instance, even with cash transfers, beneficiaries in Malawi may not be able to afford migration (Fisher et al., 2017; Nnaeme, 2021). Problems such as the application of conditional transfers in comparison to unconditional transfers (e.g., the Child Support Grant in South Africa does not require that beneficiary children attend school, while Brazil’s Bolsa Familia does) and the supply of cash in comparison to food to households that are poorer (Farrington and Slater, 2006; van Daalen et al., 2022). In order to evaluate program efficacy, these studies often use large-scale household economic surveys. They compare the results of control groups with those who receive government cash transfers and of groups that do not (Paxson and Schady, 2007). However, they rarely investigate the extent of household dependence on cash transfers or how these transfers affect overall livelihoods.
Livelihood perspectives gained prominence in development discussions following the advocacy of Chambers and Conway in the early 1990s. Over time, the term “livelihood,” once considered neutral and descriptive of making a living, has evolved into a “boundary term” that unites diverse perspectives, disciplines, and institutions around a shared understanding (Scoones, 2009). Farm-based livelihoods remain crucial to rural development planning, especially in agrarian economies within developing countries. The Livelihood Framework developed by Robert Chambers provides a holistic approach to understanding how individuals and households sustain their means of living (Nunan, 2022; Natarajan et al., 2022). It emphasizes five core assets—human, social, natural, physical, and financial capitals—that are essential for maintaining livelihoods. A sustainable livelihood, according to this framework, not only provides for the present needs but also enhances the ability to withstand external shocks and stresses without compromising future options (Khan et al., 2020). The framework highlights the dynamic interaction between these assets, external influences such as policies and institutions, and the vulnerability context in which people live, including risks, shocks, and trends. Chambers’ approach is particularly focused on the rural poor, emphasizing that sustainable livelihoods must not only meet immediate needs but also withstand external stresses, thereby ensuring long-term security (Cornwall and Scoones, 2022).
Agriculture and allied sector are the mainstay of Indian economy supporting livelihood of about half of India’s population. Farming is the major source of income for rural families, dominated by small and marginal farmers (SMFs). The SMFs are distinguished on the basis of amount of land they own or operate and live on. The average size of small and marginal holdings in all India level is just 1.41 ha and 0.38 ha, respectively (Agricultural Census 2015–2016) (Agriculture Census Division, DAC, and FWN Government of India, 2019). These farmers alone form 86 percent of the total farmers in India. Without a doubt, the performance of SMFs is significantly dependent on sustainable agricultural growth as well as on the nation’s food and nutrition security. The government must, therefore, legitimately invest in programs that would increase farmers’ income in order to improve the condition of SMFs. Discussions about Direct Benefit Transfer (DBT) in terms of cash are common. The subsidy amount is directly paid to the beneficiaries’ bank accounts without using intermediaries. The Pradhan Mantri Kisan Samman Nidhi (PM-KISAN) scheme was launched by the Indian government in order to double farmers’ income by 2022. PM-KISAN is a central sector scheme that is run by the Indian government. It is administered by the Ministry of Agriculture and Farmer’s Welfare (MoAFW). On February 24, 2019, Prime Minister Narendra Modi unveiled it (Ahmad and Haneef, 2019). This income support scheme aims to help farmers meet their financial needs to maintain healthy crops and achieve good yields. Under the scheme, small and marginal landholding farmer families (SMFs) receive a direct annual cash transfer of ₹6,000 in three equal installments of ₹2,000 each, distributed every 4 months. On June 1, 2019, following a Union Cabinet decision, PM-KISAN benefits were extended to all farmers, regardless of land size. Sharma (2019) found that PM-KISAN has a greater positive impact on farmers’ income, agriculture growth, and macroeconomic indicators than fertilizer subsidies, suggesting income support programs better enhance farmers’ financial wellbeing. Mondal et al. (2020) highlight post-Amphan betel farming recovery in South Bengal, supported by cash transfer schemes like Krishak Bandhu and MGNREGS. Despite 68% loss in leaf sales, financial assistance from Krishak Bandhu scheme and organic farming offered export potential. Kavitha et al. (2020) identified improved financial access, infrastructure, and technology dissemination as key to boosting performance in underperforming states under PM-KISAN. They recommend digitizing land records and Aadhar-linked bank accounts. Varshney et al. (2020) found that PM-KISAN reached one-third of farmers in the first 3 months, showing no selection bias across social, economic, or agricultural factors. The scheme significantly aided farmers more reliant on agriculture and with poor credit access, promoting equitable agricultural support. Amitha et al. (2021) found that all respondents of the Rythu Bandhu Scheme were aware, satisfied with cash transfers, and invested in agricultural inputs. Statistically significant results showed that the scheme has offered debt prevention benefits. A sudy by Reddy et al. (2022) examined beneficiary targeting in an Indian village, revealed that significant exclusion errors in welfare schemes, which largely favor middle-income households over the poorest. The reach and extent of welfare programs, particularly Direct Benefit Transfers, expanded significantly during the COVID-19 pandemic. Another study by Reddy et al. (2024) in Emped village of India, analyzed 287 households and 29 welfare schemes. It was found that Public Distribution System, Direct benefit transfers, and employment schemes benefitted most, while large schemes like housing had limited reach. Welfare schemes often faced delays, leakages, and exclusion errors, with most benefits going to middle-and high-income households, excluding the poorest. A review of the evolution of cash transfer policy of India reveals that, like many countries. India has made significant strides in embracing cash transfers and basic income as key policy solutions for addressing development and poverty challenges (Mehta, 2019). This progression is evident from 2008 to 2019, as outlined in Table 1.
The Livelihood Framework has been widely applied in research related to poverty alleviation and rural development (Scoones, 2013). In India, it has been used to assess various development programs, including agricultural policies, microfinance initiatives, and natural resource management strategies (Patnaik and Prasad, 2014). In the context of cash transfer schemes like PM-KISAN, the livelihood framework is vital in assessing whether such initiatives have led to an improvement in the livelihoods of small and marginal farmers. The PM-KISAN scheme provides direct income support to farmers, which, in theory, should bolster their financial capital. However, the true impact of the scheme needs to be analyzed through the lens of Chambers’ livelihood components. Beyond financial stability, the scheme’s success needs to be measured by improvements in social networks (social capital), enhanced access to productive resources (natural capital), and better infrastructure or technology (physical capital). Evaluating these components helps determine whether the scheme has genuinely uplifted the livelihood status of SMFs in India.
The performance of PM-KISAN, therefore, hinges on whether it has enabled farmers to improve agricultural productivity, and sustain their livelihood amidst growing economic and climate challenges. The SMFs in India are vulnerable to a number of aspects in terms of socio-economic conditions, climate change impact, technology adoption and many more. The unconditional cash transfer under PM-KISAN allows the beneficiaries to spend their cash transfer anywhere they like. However the intended objective is to augment agricultural production and to ease liquidity constraints of farmers for a better livelihood. PM-KISAN remains crucial in addressing income disparities and supporting sustainable agricultural growth. In one hand, the unconditional income support under PM-KISAN scheme allows farmer to utilize the money as they like in order to ease the liquidity constraints in a farmers’ life and on the other hand, the question of ₹6,000 annually as income support for the sustenance of the farmers’ family is a matter of concern. Despite the facts and figures stated in various Govt. reports, the performance of the scheme in raising livelihood standards is of a serious concern. Several studies have evaluated the effects of PM-KISAN on farmers’ income and agricultural development but whether the cash transfers has led to any improvement in the farmers’ livelihood has rarely been studied. It is considered necessary to have a thorough discussion about the livelihood of the small and marginal farmers who are benefiting from the PM-KISAN scheme in this context. To fully explain the livelihood status of SMF households in India, this study uses an ex-post facto research design and implements an indicator-based composite measure. Based on the livelihood index created, the study aims to ascertain whether the performance of PM-KISAN scheme has substantially improved the livelihood of the beneficiaries in comparison to the non-beneficiaries. Figure 1 shows the conceptual framework of the study.
2 Materials and methods
2.1 Locale of the study
The Indo-Gangetic Plain (IGP) in India plays a crucial role in national food security and supports millions of rural poor. Spanning 57.66 Mha across Punjab, Haryana, Uttar Pradesh, Bihar, and West Bengal, (Aggarwal et al., 2000) it hosts 40% of India’s 12.6 crore small and marginal land holdings, with Bihar and Uttar Pradesh alone contributing 75.2% of the IGP’s small and marginal holdings and 30% nationwide (Agricultural Census 2015–16). For this reason, Uttar Pradesh and Bihar were chosen for the study because it will provide us with a comprehensive understanding of the impact of the PM-KISAN scheme on the SMFs that are vulnerable. A multistage random sampling procedure was followed. Bahraich and Bulandshahr districts of Uttar Pradesh and Bhojpur and Muzaffarpur districts of Bihar were selected. In the consecutive stage, from each selected district, two cluster of villages, i.e., 2 g panchayats were selected. Kataha and Ghasipur Panchayat were selected from Bahraich district. Chhapna and Machad Panchayat were selected from Bulandshshr district. Dhandiha and Bakri Panchayat were selected from Bhojpur district. Gavasara and Mohammadpur Khaje Panchayat were selected from Muzaffarpur district (Figure 2).
2.2 Sampling and data collection
A sampling frame was prepared for each of the selected Gram Panchayats, and from each Gram Panchayat 15 respondents was selected as beneficiaries and 15 respondents was selected as non-beneficiaries of the said scheme using stratified disproportionate simple random sampling procedure. Beneficiaries for the purpose included SMFs who received at least 7 installments under PM-KISAN. Non-beneficiaries for this purpose refers to the SMFs who has not received the benefits of the scheme due to some operational error in documents related to improper Aadhaar or Land records or are not eligible to receive benefits as per exclusion categories of the scheme. Thus, a total of 240 farmers (120 beneficiary farmers and 120 non-beneficiary farmers) were selected for the present study. In the year 2022, a structured personal interview schedule was used to conduct household surveys and personal interviews for this study.
2.3 Data analysis
The scheme’s performance was measured at the household level, and it was operationalized in terms of how well it has helped small and marginal farmers enhance their livelihoods. The capacities, assets (both social and material resources), and activities that are necessary for a means of subsistence constitute livelihood, according to Chambers and Conway (1991). Stress, shocks, and agricultural seasonal trends affect small and marginal farmers. The possibility of turning available assets into “living” assets is affected by these changes. As a result, after receiving financial assistance, the fundamental assets of livelihood, which include financial, physical, natural, and human capital, will be evaluated for any potential improvements. A composite index is a function of variables and weights that converts many qualities into a single real value. While development indicators are useful, a summary measure that integrates various indicators into a single figure is required. As a result of this technique, composite development indexes have been developed (Santos and Santos, 2013). Developing an index using Principal Component Analysis (PCA) assigns weights to the original variables based on their contributions to the principal components, offering a more balanced approach compared to arbitrary weighting schemes (Wang, 2015; Schlossarek et al., 2019). A PCA-led index is advantageous over individual variables as it reduces dimensionality, addressing multicollinearity issues (Tefera, 2013). It captures the most significant variations while minimizing redundancy, revealing latent structures and relationships that may not be apparent when examining variables separately (Demšar et al., 2013). By merging correlated variables into principal components, it improves interpretability, and ensures that the index accurately reflects the underlying data structure more effectively. Accordingly, five indices comprise the Livelihood Index (LI): Human Capital Index (HCI), Social Capital Index (SCI), Financial Capital Index (FCI), Natural Capital Index (NCI), and Physical Capital Index (PCI). Table 2 shows that SCI and FCI had three indicators, while HCI, NCI, and PCI had four indicators each.
The design of composite indices for measuring livelihood comprised two main steps:
i. Eliminating scale bias in indicators: Each indicator was normalized according to their relation to the composite index. By subtracting the indicator’s minimum value from its actual value and dividing the result by the range, i.e., the difference between the maximum and least value of the selected indicator—it was normalized for indicators that had a direct (positive) relationship with the composite index. By subtracting the indicator’s actual value from its maximum value and then dividing the result by the range, i.e., the difference between the maximum and least value of the selected indicator—it was normalized for indicators that had an indirect (negative) relationship with the composite index.
ii. Determining appropriate weights to be applied to distinct indicators: The next tough challenge was to assign suitable weights to the selected indicators once the scale bias was removed from the observations. Assignments of arbitrary weights that are based on independent judgment are subjective and should only be made as a last option. Consequently, using PCA, the weights of individual indicators were objectively assigned in this analysis. Statistical Package for Social Sciences (SPSS version 25 for Windows) was used to perform PCA.
To calculate Eigen values and Factor Loadings, PCA is performed using SPSS (version 25). Eigen values >1 are identified, and the same number of components are extracted for each variable from the rotational component matrix, based on the number of Eigen values above one. The extracted component matrix is then multiplied by the Eigen Values. This starts with the first Eigen Value multiplied by the first extracted component column, then the second Eigen Value multiplied by the second extracted component column, and continues in the same way. The weight of each indicator is calculated by summing the values for that indicator. By combining the weights of all indicators, we obtain the Grand Total Weight. The weight of each indicator is multiplied by its respective normalised value. The index is created by dividing the total weight of each multiplication by the Grand Total Weight. Thus, the formula that is employed to ascertain the index is:
Where, I is the index, Xi is the normalised value of i-th indicator, Lij is the factor loading value of the ith variable on the jth factor, Ej is the Eigen Value of the jth factor (NUEPA, 2009).
In order to have precise assessment about the performance of PM-KISAN scheme in enhancing livelihood of beneficiaries, it was necessary to avoid the selection bias on covariates. Selection bias can occur when farmers who are given treatment are not selected randomly. An instrumental variable (IV) method is often used to solve this issue (Abadie, 2003; Heckman and Salvador, 2003; Yen et al., 2008). However, it is difficult to find an instrument that is reliable (Imbens and Woolridge, 2009). Furthermore, as the IV technique does not rely on exogeneity assumptions, it violates the overlap assumption (Imbens, 2004). The current study utilized a propensity score matching (PSM) technique to aid in determining the causal relationships between the data (Dehejia and Wahba, 2002; Gautam et al., 2017; Schreinemachers et al., 2016; Gitonga et al., 2013; Khan et al., 2012; Abebaw et al., 2010). All those variables which have the tendency to influence outcome should be included in the model for estimating balancing score, i.e., propensity score (Rosenbaum and Rubin, 1983).
The probability of participation (Di) for a farm i given a set X = xi of farm attributes p(X) = Pr(Di = 1| X = xi) is the propensity score (Bryson et al., 2002). The Average Treatment Effect on the Treated (ATT) is given by:
ATT = E[Dᵢ = 1, p(Xᵢ)] − E[Dᵢ = 0, p(Xᵢ)].
Using a large amount of observable information, PSM pairs individuals in a treated group with those in an untreated group. According to Dehejia and Wahba (2002), this method can result in unbiased estimators of the treatment impact because the results are independent of assignment to treatment and conditional on pre-treatment covariates. Matching of beneficiary and non-beneficiary observations using the values of the propensity score was conducted using Stata 14 software for Windows (Leuven and Sianesi, 2012). The steps for PSM includes:
i. Establishing common support: A conditional independence assumption (CIA) and a substantial common support or overlap in propensity scores between beneficiaries and non-beneficiaries are two conditions that determine the validity of matching methods; however, these methods can help produce a counterfactual from the control group (Caliendo and Kopeinig, 2008).
ii. Choosing matching algorithm: The performance of the PM-KISAN scheme on farmers’ livelihood is estimated using two matching algorithms: Caliper matching and Nearest Neighbor matching.
iii. Assessing the matching quality: It is necessary to assess whether the matching procedure is capable of balancing the distribution of the relevant variables in both the treatment and control group, as we do not condition on all covariates but on the propensity score.
iv. Robustness check: A estimator called inverse probability weighted regression adjustment (IPWRA) was used for the purpose of evaluating the robustness of PSM estimates. IPWRA is consistent when the treatment or outcome model is misspecified (Wooldridge, 2010). The ATT is calculated in two stages by the IPWRA models (Imbens and Woolridge, 2009): first, propensity scores are calculated using multinomial probit regression.
v. Sensitivity analysis: The sensitivity parameter Γ (gamma), a measurement of the degree of deviation from random treatment assignment, is the basis of Rosenbaum’s sensitivity analysis method. To invalidate the PSM analysis results, we look at how significantly an unobserved variable may affect the selection process. We do this by using Rosenbaum’s bounding technique (Caliendo and Kopeinig, 2008).
3 Results
3.1 Weights of indicators and indices
As calculated through PCA, the constituent indicators of HCI, SCI, FCI, NCI, and PCI carried distinct component weights. Within the 18 rural livelihood indicators, there was a grand weight (W) of 44.261 (Table 3). The HCI contributed 21.35 percent to the LI’s total weightage. Among the four indicators (x4) Food consumption received highest weightage (3.788) followed by (x3) Health care (2.269), (x1) Ratio of active family members in working age group (1.737), and (x2) Education status of the Family (1.654). The SCI contributed to 11.27 percent. (x5) Market access received maximum weightage (1.847) followed by (x6) Give: Receive ratio (1.729) and (x7) Extension Agency Contact (1411). The FCI contributed 14.93 percent. Among three indicators, (x10) Household income was most important as evident from weightage (3.777) followed by (x8) Banking activities (1.773) and (x9) Rural indebtness (1.059). The NCI contributed to as much as 22.42 percent. Among three indicators, (x11) Per capita area under cultivation received maximum importance (3.934) followed by (x13) Cropping intensity (2.157) and (x12) Yield index (1.677). The PCI contributed to more than one-fourth (30.03 percent) of the total weightage of LI. Among the four indicators (x15) Household gadgets possessed received maximum weightage (4.133), followed by (x16) Small implements possessed (3.945), (x17) Herd size and (3.371), and (x18) Bird unit size (1.840).
3.2 Livelihood of SMF households
The cumulative cube root frequency method was used to divide the sample of households, which included both beneficiary and non-beneficiary, into three categories of livelihood based on the household level LI (Table 4). The households with LI score < 0.342 had a low status of livelihood. Households with a LI score ranging from 0.342 to 0.466 had a medium status of Livelihood and Households >0.466 had a High status of Livelihood. From the score of LI it was found that about 47.5 percent of the beneficiaries had medium status of livelihood followed by 32.5 percent of beneficiaries with low status of livelihood. And a less percentage of beneficiaries, i.e., 20 percent had high status of livelihood. Similarly among non-beneficiaries, about half of the non-beneficiaries, i.e., 50 percent had medium status of livelihood followed by 39.17 percent of non-beneficiaries had low status of livelihood. A lesser percentage, i.e., 10.83 percent of non-beneficiaries had high status of livelihood (Table 4). Based on individual indices, it was found that for Beneficiary household the HCI was highest (0.494) followed by NCI (0.477), SCI (0.434), FCI (0.303). Similarly for Beneficiary household the HCI was highest (0.464) followed by NCI (0.455), SCI (0.385), FCI (0.276) and PCI (0.268) (Table 5).
3.3 Performance of the PM-KISAN scheme
With the goal of determining whether the scheme PM-KISAN performed well in enhancing the livelihood of the beneficiaries as compared to the non-beneficiaries, the assessment by means of PSM focused on one outcome variable, i.e., the “Livelihood index” for the respondents calculated earlier. The explanatory variables include socio-economic and socio-psychological variables like age, education, family size, operational land holding, crops grown, occupation status, farming experience, annual income, social participation, extension orientation, mass media exposure, economic motivation, risk orientation and innovativeness.
3.3.1 Establishing common support
The region of overlap between the treated and control groups, i.e., beneficiaries and non-beneficiaries, respectively, shows the matching quality between the two groups based on region of common support. The region of common support is 0.15851574–0.91159513 (Figure 3). The propensity score’s balancing property was tested after the common support assumption has been met. Five blocks were identified and there was no difference between the mean of propensity scores in each block. Matching improved overlap between the marginal distributions of the covariates.
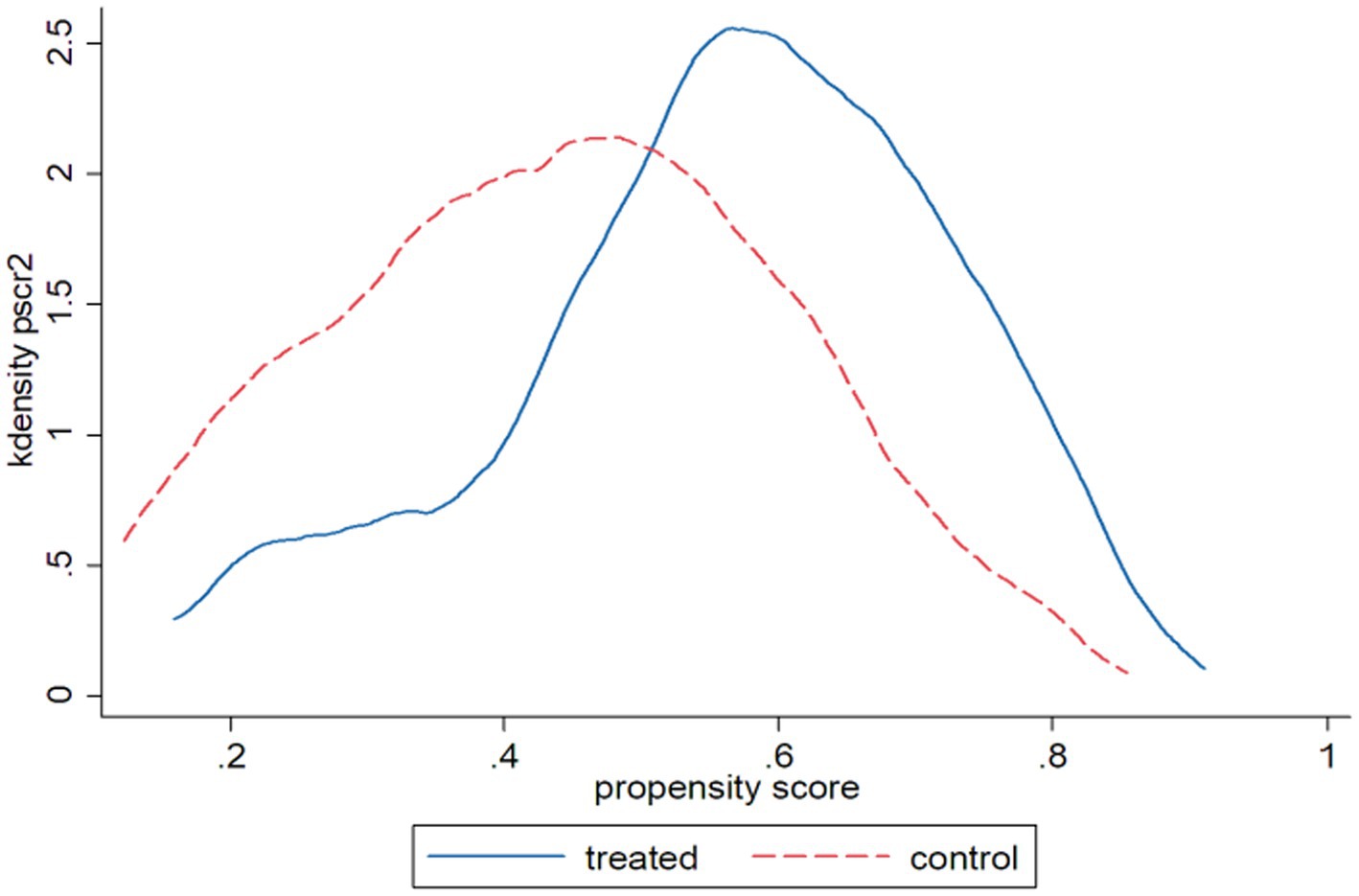
Figure 3. Common support for identification of comparable beneficiaries (treatment) and non-beneficiaries (control group).
3.3.2 Choosing matching algorithm
Although Caliper matching and Nearest Neighbor matching algorithms gave different quantitative results, their qualitative results were similar. In case of Nearest Neighbor Matching method, i.e., NN 1, NN 2, NN 3 matching, there were 120 matched pairs with no loss of in the sample size (Table 6). The average treatment effect on the treated (ATT) was found to be 0.0414 in case of NN-1 followed by 0.0349 in case of NN-5 and 0.0335 in case of NN-3 (Table 6). In case of Caliper Matching Method, in Caliper (0.03), there were 115 matched pairs. With Caliper (0.05), there were 117 matched pairs. And with Caliper (0.1), there were 118 matched pairs. The average treatment effect on the treated (ATT) in Caliper Matching was found to be 0.0386 in case of Caliper(0.1) followed by 0.0378 in case of Caliper (0.05) and 0.0367 in case of Caliper (0.03). The results of ATT in either type of matching was significant (p = 0.05) (Table 6). This study follows suit by calculating the standard error for the estimated scheme impact upon livelihood by using a bootstrapping methodology. Bootstrapping with 50 replications were used to test statistical significance of standard error which was established (Table 6).
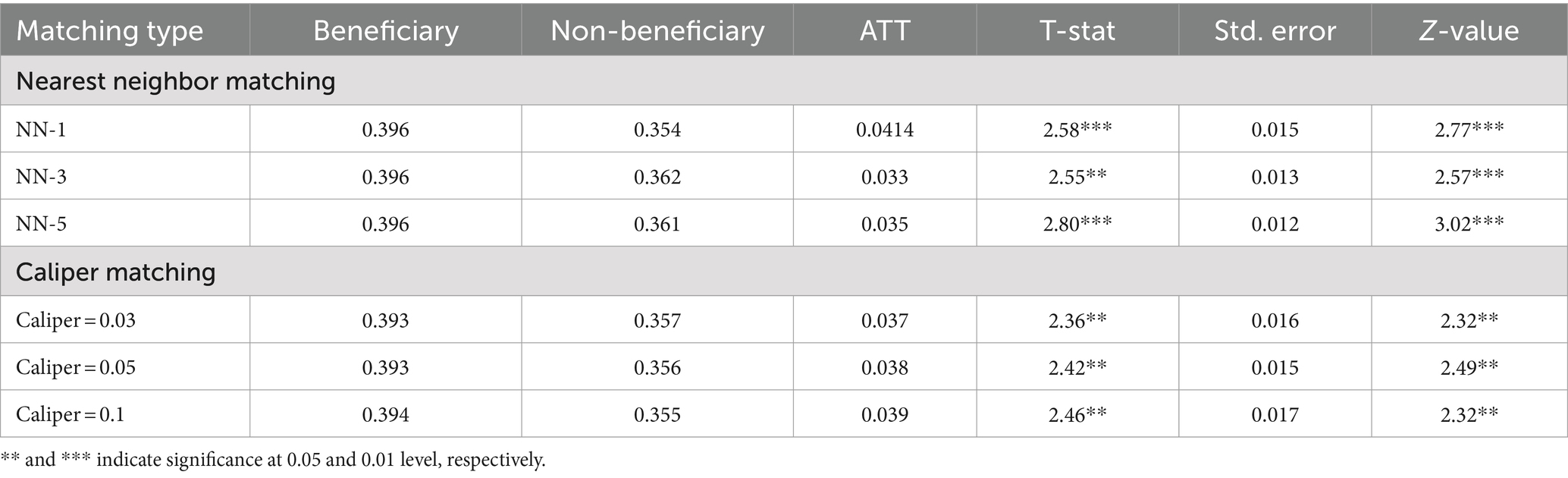
Table 6. Average treatment effect on livelihood index of beneficiaries of PM-KISAN scheme using propensity score matching techniques.
3.3.3 Assessing the matching quality
The balancing of covariates was evaluated by reduction in the mean absolute standardized bias between the matched and unmatched models. The matching procedures achieved better balance over time due to the decreasing trend (Table 7). The percent bias reduction in case of NN-5 was 40.82 percent followed by NN-3 with 31.29 percent, Caliper = 0.05 with 13.6 percent, both Caliper = 0.03 and Caliper = 0.1 with 12.9 percent and NN-1 with 8.84 percent. The Nearest Neighbor Matching (NN-5) approach has the best matching quality, while NN-1 has the worst, as shown by the average standardized bias measure. As a result, the PSM succeeded in decreasing selection bias as a result of the characteristics that were observed. The comparison of pseudo R2 values in Table 7 indicates that pseudo R2 is lower after matching than before for all matching algorithms. This suggests that, post-matching, there is no systematic difference in the distribution of independent variables between adopters and non-adopters (Izudi et al., 2019; Khan et al., 2022). Thus it ensures that covariates are randomly distributed among adopter and non-adopter groups (Table 7).
3.3.4 Robustness check
According to ATT results, the livelihood index of the PM-KISAN scheme has been enhanced by 0.025 (Table 8) with a standard error of 0.006. They are significant at 0.01 level and very similar to the PSM results, which supports our PSM results.

Table 8. Average treatment effect on livelihood index of beneficiaries of PM-KISAN scheme using IPWRA.
3.3.5 Sensitivity analysis
In this analysis, the maximum value for gamma (Γ) was set from 1 to 1.5 with increments of 0.01. When unobserved covariates were present at various critical levels of Γ, the treatment effect was quite strong. At Γ = 1.34, the findings were significant (p = 0.05). For the various treatment variables, the point at which we began to question the conclusion of a positive impact of the PM-KISAN scheme on the beneficiaries’ livelihood index was at Γ = 1.34. Beyond this threshold, the significance level exceeds the standard 0.05, rendering the results insignificant (Table 9).
4 Discussion
The current method for assessing livelihood sustainability is both reliable and beneficial, as it involves a micro-level evaluation using household surveys and the analysis of data based on a set of objectively weighted criteria. This approach provides a more accurate understanding of livelihood conditions in the study area compared to macro-level assessments, which generally rely on secondary data from broader regions such as blocks, districts, or states (Liu et al., 2018; Singh and Hiremath, 2010). Macro-level estimation techniques frequently rely on extrapolating data from all households within the same geographical area. This could ignore variations at the household level, including adaptive capacities, income, living standards, risks, assets, and livelihood strategies (Wang et al., 2016; Rahman and Akter, 2014). The livelihood strategy that a household chooses is significantly affected by the kind of livelihood capital that the household holds (Pour et al., 2018; Huang et al., 2022). For instance, households with more social, natural, and physical capital are more inclined to engage in farm-based livelihoods. On the other hand, households with more human and financial capital are more inclined to pursue livelihoods that are not farm-based (Hua et al., 2017; Habib et al., 2023). Moreover, not all of the indices were equally significant; for instance, the PCI contributed up to 30.02 percent of LI whereas the SCI contributed just 11.27% (Table 3). The natural, physical, and human capital indices worked together to contribute as much as 73.8 percent to the livelihood index, showing that households with better cropping diversity, higher crop intensities, and greater net sown areas, as well as possession of various physical implements and farm tools, as well as alternative sources of income from poultry units and cattle, and better educational status with a higher number of active family members, added to proper food consumption.
Several studies conducted throughout the world have found that diversifying one’s livelihood to include additional and alternative sources of income is a crucial step in ensuring the sustainability and wellbeing of one’s household (Pace et al., 2022; Pandey et al., 2017; Gautam and Andersen, 2016; Fang et al., 2014). Comparing beneficiaries and non-beneficiaries of various Livelihoods sub-indices, it was discovered that the average rise between beneficiaries and non-beneficiaries was greater for social capital and less so for natural capital (Table 5). In order to guarantee income and sustain a livelihood, the market is essential. A significant determining factor in the adoption of high-return livelihood choices is proximity to market centres (Khatiwada et al., 2017) or district centres (Negash et al., 2023). Choice of crops is greatly influenced by market access (Grogan et al., 2012). The importance of extension service providers has always been considered in emerging and underdeveloped countries. This is particularly true for the development of small and marginal farmers with limited resources (World Bank, 2010; FAO, 2005). In the end, agricultural production did not rise; rather, the ownership of agricultural equipment and livestock increased continuously; this indicates that farmers made a long-term investment in their farms (Varshney et al., 2020).
Large, one-time transfers focused on agriculture can significantly impact production, although this may partly result from the support and guidance provided alongside the transfers (Beaman et al., 2015; Ghosh et al., 2022). The results from the ATT value calculated through detailed proceedings of PSM confirmed that the livelihood index of the beneficiaries as compared to non-beneficiaries is greater by 3–4 percent which was statistically significant (p = 0.05). The quality of matching was validated using the standardized bias approach (Karim et al., 2022). Although most empirical studies consider a bias reduction below 3 to 5 percent as sufficient (Caliendo and Kopeinig, 2008), there is no definitive measure of the matching procedure’s success in this case. To further confirm the PSM results, a doubly robust estimator, IPWRA, was employed. The ATT is calculated in two stages by the IPWRA model (Imbens and Woolridge, 2009). Propensity scores were estimated first by means of a multinomial probit regression. Next, the inverse of these scores were used as weights in the linear outcome model. The ATT value of 2.5% significant at p = 0.01 implied that results of the PSM estimate are robust to overt bias that may arise from misspecification of the model. Rosenbaum’s sensitivity analysis implied that individuals differ in their odds of treatment by a factor of 34 %, in terms of unobserved covariates or hidden bias. This value or bound reflects “worst-case situations” and so does not reveal the presence of selection bias; rather, it tells us how significant the selection bias should be in order to invalidate our conclusions. Most social science studies are not completely immune to hidden bias, and Γ values between 1 and 2 are frequently utilised as a starting point for many social science data sets (Keele, 2010). Although statistically significant result was obtained, but the increase in amount of LI of beneficiaries compared to non-beneficiaries is very meagre. Additionally, policy measures to revise the amount based on inflation index or crop based income support based on cost of cultivation of particular crop can be provided that would further enhance the performance of the scheme. To effect a positive change in livelihood sustainability, long-term support and guidance will be necessary (Paul et al., 2019). In order to improve the livelihoods of small farmers, large cash transfers can be effective tools, as this study is among the first to suggest. In the long run, a steady increase in income could be achieved if farmers allocate a portion of the PM-KISAN cash transfer to investments that are productive (Sadoulet et al., 2001).
5 Conclusion
Cash transfer programmes like PM-KISAN is basically a development programme aimed at increasing the social status of the farmers especially the SMFs who faces challenges like credit constraints, poor infrastructure, untimeliness of input supply, poor market linkage, volatile market conditions, and climatic variability which plunges them into poverty and they cannot produce enough income to meet even their basic requirements of their families. In this regard, the holistic view of taking Livelihood of the SMFs in concern to probe into the performance of the scheme is beneficial for further considerations in the policy framework. Very little attention has been paid to the indirect effects of cash transfer programs. Our findings indicate that these effects can be highly significant and should be fully considered when designing such programs. Our study establishes evidence that the PM-KISAN scheme has significantly stimulated the cash transfers’ impact on the livelihood of small and marginal farmers. In particular, the study shows that PM-KISAN has performed well as it has enhanced the livelihood index of beneficiaries by 2.5 percentage points which is statistically significant, as compared with non-beneficiaries. However the increase is very meagre and the scheme should not be taken as a standalone. Lessons learned from this research suggest that the agricultural extension system can aid in realizing more benefits of PM-KISAN, as they can encourage farmers to make productive investments in agriculture that would enhance their livelihood as cash transfers have a multiplier effect thus augmenting the status of the five components of livelihood including human, social, natural, physical, and financial capitals. The policy implication from this analysis is that if multiplier effects are substantial and responsive to policy changes, then the PM-KISAN program would benefit from being integrated into a broader strategy aimed at maximizing these effects. The ultimate goal is to increase the income of the targeted households, which can be achieved by introducing complementary rural development initiatives that create opportunities to use the transfers more productively. PM-KISAN aids small and marginal farmers in enhancing their livelihoods through the promotion of superior high-yield variety (HYV) seeds and the encouragement of modern technology adoption. This, in turn, creates a pathway for farmers to make productive investments in agriculture. However, the scheme should not be viewed as a standalone solution. It must be integrated with other initiatives, such as the Public Distribution System and Procurement schemes, to offer comprehensive support that can help break the cycle of intergenerational poverty and low income among farmers through investment in modern technology.
Despite efforts to ensure impartiality and rigor, this study has its own limitations. Findings rely on respondents’ opinions, may be region-specific, and are confined to only two states of India. The need for a longitudinal approach also limit the study’s scope and objectivity. The study can be extended to other states of the country to get a comprehensive view regarding the performance of the scheme. The study helped in analysing the performance of the scheme at the beneficiary level, i.e., the extent to which, their livelihood is enhanced by providing income support. The methodology used for assessing the performance can also be used in evaluating other DBT schemes of India in the same light. Further research studies delineating facilitating and inhibiting factors of the cash transfers under PM-KISAN scheme may be taken by researchers in future. Apart from these, studies to measure vulnerability mitigation in light of impact from cash transfers may also be looked into in future.
Data availability statement
The raw data supporting the conclusions of this article will be made available by the authors, without undue reservation.
Ethics statement
The studies involving humans were approved by the Social Sciences Research Ethics Committee of the ICAR-Indian Agricultural Research Institute. The studies were conducted in accordance with the local legislation and institutional requirements. The ethics committee/institutional review board waived the requirement of written informed consent for participation from the participants or the participants’ legal guardians/next of kin because it was verbal and based on the questionnaire.
Author contributions
BG: Conceptualization, Formal analysis, Investigation, Methodology, Software, Writing – original draft, Writing – review & editing. RB: Conceptualization, Methodology, Supervision, Validation, Writing – review & editing. RP: Methodology, Supervision, Validation, Writing – review & editing. SP: Conceptualization, Methodology, Resources, Writing – review & editing. GM: Conceptualization, Resources, Supervision, Writing – review & editing. PK: Methodology, Resources, Software, Writing – review & editing. AB: Methodology, Software, Validation, Writing – review & editing. SM: Conceptualization, Formal analysis, Writing – review & editing. SS: Writing – review & editing. SG: Writing – review & editing. SM: Writing – review & editing. SQ: Writing – review & editing.
Funding
The author(s) declare that no financial support was received for the research, authorship, and/or publication of this article.
Acknowledgments
The authors are heartily thankful to all the sampled farmer families to respond to the personal interviews conducted with them.
Conflict of interest
The authors declare that the research was conducted in the absence of any commercial or financial relationships that could be construed as a potential conflict of interest.
Publisher’s note
All claims expressed in this article are solely those of the authors and do not necessarily represent those of their affiliated organizations, or those of the publisher, the editors and the reviewers. Any product that may be evaluated in this article, or claim that may be made by its manufacturer, is not guaranteed or endorsed by the publisher.
References
Abadie, A. (2003). Semi-parametric instrumental variable estimation of treatment response models. J. Econom. 113, 231–263. doi: 10.1016/S0304-4076(02)00201-4
Abebaw, D., Fentie, Y., and Kassa, B. (2010). The impact of food security program on household food consumption in northwestern Ethiopia: a matching estimator approach. Food Pol. 35, 286–293. doi: 10.1016/j.foodpol.2010.01.002
Aggarwal, P. K., Talukdar, K. K., and Mall, R. K. (2000). Potential yields of Rice-wheat system in the indo-gangetic plains of India. Rice-wheat consortium paper series 10. New Delhi: Rice-Wheat Consortium for Indo-Gangetic plans.
Agriculture Census Division, DAC, and FWN Government of India (2019). Agricultural census 2015–16. (phase-I). All India report on number and area of operational holdings. Available at: https://agcensus.nic.in/document/agcen1516/T1_ac_2015_16.pdf (Accessed May 20, 2024).
Ahmad, T., and Haneef, R. (2019). Pradhan Mantri Kisan Samman Nidhi (PM-KISAN) trial on farmers. Rashtriya Krishi 14, 95–96.
Amitha, C. D., Karthikeyan, C., and Devi, M. N. (2021). Assessing the impact of Rythu Bandhu scheme in Warangal District of Telangana. Asian J. Agric. Ext. Econ. Sociol. 39, 335–340. doi: 10.9734/ajaees/2021/v39i1130758
Ardington, C., Case, A., and Hosegood, V. (2009). Labor supply responses to large social transfers: longitudinal evidence from South Africa. Am. Econ. J. Appl. Econ. 1, 22–48. doi: 10.1257/app.1.1.22
Asfaw, S., Davis, B., Dewbre, J., Handa, S., and Winters, P. (2014). Cash transfer programme, productive activities and labour supply: evidence from a randomised experiment in Kenya. J. Dev. Stud. 50, 1172–1196. doi: 10.1080/00220388.2014.919383
Bastagli, F., Hagen-Zanker, J., Harman, L., Barca, V., Sturge, G., and Schmidt, T. (2019). The impact of cash transfers: a review of the evidence from low-and middle-income countries. J. Soc. Policy 48, 569–594. doi: 10.1017/S0047279418000715
Beaman, L., Karlan, D., Thuysbaert, B., and Udry, C. (2015). Selection into credit markets: Evidence from agriculture in Mali. Econometrica 91, 1595–1627. doi: 10.3982/ECTA18916
Bertrand, M. (2003). Public policy and extended families: evidence from pensions in South Africa. World Bank Econ. Rev. 17, 27–50. doi: 10.1093/wber/lhg014
Boone, R., Covarrubias, K., Davis, B., and Winters, P. (2013). Cash transfer programs and agricultural production: the case of Malawi. Agric. Econ. 44, 365–378. doi: 10.1111/agec.12017
Bosworth, J., Alvar, C., Corral, L., Davis, B., Musembi, D., Mwasiaji, W., et al. (2016). “The cash transfer programme for orphans and vulnerable children: the catalyst for cash transfers in Kenya” in From evidence to action: The story of cash transfers and impact evaluation in sub-Saharan Africa. eds. B. Davis, S. Handa, N. Hypher, N. Winder Rossi, P. Winters, and J. Yablonski (Oxford: FAO, UNICEF, Oxford University Press), 117–145.
Bryant, J. H. (2009). Health Kenya’s cash transfer program: protecting the health and human rights of orphans and vulnerable children. Health Hum. Rights 11, 65–76
Bryson, A., Dorsett, R., and Purdon, S. (2002). The use of propensity score matching in the evaluation of active labour market policies. Working Paper no: 4. London: Policy Studies Institute and National Cemtre for Social Research.
Caliendo, M., and Kopeinig, S. (2008). Some practical guidance for the implementation of propensity score matching. J. Econ. Surv. 22, 31–72. doi: 10.1111/j.1467-6419.2007.00527.x
Chambers, R., and Conway, G. (1991). Sustainable rural livelihoods: practical concepts for the 21st century. Falmer: Institute of Development Studies (UK).
Cooper, J. E., Benmarhnia, T., Koski, A., and King, N. B. (2020). Cash transfer programs have differential effects on health: a review of the literature from low and middle-income countries. Soc. Sci. Med. 247:112806. doi: 10.1016/j.socscimed.2020.112806
Cornwall, A., and Scoones, I. (2022). Revolutionizing development: Reflections on the work of Robert Chambers. London: Taylor & Francis.
Covarrubias, K., Davis, B., and Winters, P. (2012). From protection to production: productive impacts of the Malawi social cash transfer scheme. J. Dev. Eff. 4, 50–77. doi: 10.1080/19439342.2011.641995
Daidone, S., Davis, B., Dewbre, J., and Covarrubias, K. (2014a). Lesotho child Grants Programme: 24-month impact report on productive activities and labour allocation. Rome: FAO.
Daidone, S., Davis, B., Dewbre, J., Gonzalez-Flores, M., Handa, S., Seidenfeld, D., et al. (2014b). Zambia’s child Grant Programme: 24-month impact report on productive activities and labour allocation. Rome: FAO.
Das, J., Do, Q. T., and Özler, B. (2005). Reassessing conditional cash transfer programs. World Bank Res. Obs. 20, 57–80. doi: 10.1093/wbro/lki005
Davis, B., Handa, S., Hypher, N., Winder Rossi, N., Winters, P., and Yablonski, J. (2016). “Conclusions and policy implications for cash transfer programmes” in From evidence to action: The story of cash transfers and impact evaluation in sub-Saharan Africa. eds. B. Davis, S. Handa, N. Hypher, and N. W. Rossi (Oxford: FAO, UNICEF, Oxford University Press), 335–358.
Dehejia, R. H., and Wahba, S. (2002). Propensity score-matching methods for nonexperimental causal studies. Rev. Econ. Stat. 84, 151–161. doi: 10.1162/003465302317331982
Demšar, U., Harris, P., Brunsdon, C., Fotheringham, A. S., and McLoone, S. (2013). Principal component analysis on spatial data: an overview. Ann. Assoc. Am. Geogr. 103, 106–128. doi: 10.1080/00045608.2012.689236
Fajth, G., and Vinay, C. (2010). Conditional cash transfers: a global perspective. MDG Insights. 1, 1–6.
Fang, Y., Fan, J., Shen, M., and Song, M. (2014). Sensitivity of livelihood strategy to livelihood capital in mountain areas: empirical analysis based on different settlements in the upper reaches of the Minjiang river. China. Ecol. Indic. 38, 225–235. doi: 10.1016/j.ecolind.2013.11.007
FAO (2005). Modernizing National Agricultural Extension Systems-a Practical Guide for Policy Makers in developing countries. Rome: FAO.
Farrington, J., and Slater, R. (2006). Introduction: cash transfers: panacea for poverty reduction or money down the drain? Dev. Policy Rev. 24, 499–511. doi: 10.1111/j.1467-7679.2006.00344.x
Fisher, E., Pozarny, P., and Estruch, E. (2017). Qualitative research on decent rural employment: Malawi case study. Rome: FAO.
Gautam, Y., and Andersen, P. (2016). Rural livelihood diversification and household wellbeing: insights from Humla. Nepal. J. Rural Stud. 44, 239–249. doi: 10.1016/j.jrurstud.2016.02.001
Gautam, S., Schreinemachers, P., Uddin, M. N., and Srinivasan, R. (2017). Impact of training vegetable farmers in Bangladesh in integrated pest management (IPM). Crop Protect. 102, 161–169. doi: 10.1016/j.cropro.2017.08.022
Ghosh, B., Roy Burman, R., Padaria, R. N., and Paul, S. (2022). Global lens - agricultural development and poverty alleviation - cash transfer as policy instrument. Agriculture Today 25, 56–58.
Gilligan, D. O., Hoddinot, J., and Taffesse, A. S. (2008). The impact of Ethiopia’s productive safety net programme and its linkages DP00839. Washington, DC: IFPRI.
Gitonga, Z. M., De, G. H., Kassie, M., and Tefera, T. (2013). Impact of metal silos on households maize storage, storages losses and food security: an application of a propensity score matching. Food Pol. 43, 44–55. doi: 10.1016/j.foodpol.2013.08.005
Grogan, P., Lalnunmawia, F., and Tripathi, S. K. (2012). Shifting cultivation in steeply sloped regions: a review of management options and research priorities for Mizoram state, Northeast India. Agrofor. Syst. 84, 163–177. doi: 10.1007/s10457-011-9469-1
Grosh, M., del Ninno, C., Tesliuc, E., and Ouerghi, A. (2008). For protection and promotion: the design and implementation of effective safety nets. Washington, DC: World Bank.
Habib, N., Ariyawardana, A., and Aziz, A. A. (2023). The influence and impact of livelihood capitals on livelihood diversification strategies in developing countries: a systematic literature review. Environ. Sci. Pollut. Res. 30, 69882–69898. doi: 10.1007/s11356-023-27638-2
Heckman, J., and Salvador, N. L. (2003). Using matching, instrumental variables, and control functions to estimate economic choice models. Uppsala: IFAU.
Hua, X., Yan, J., and Zhang, Y. (2017). Evaluating the role of livelihood assets in suitable livelihood strategies: protocol for anti-poverty policy in the eastern Tibetan plateau, China. Ecol. Indic. 78, 62–74. doi: 10.1016/j.ecolind.2017.03.009
Huang, L., Yang, L., Tuyến, N. T., Colmekcioglu, N., and Liu, J. (2022). Factors influencing the livelihood strategy choices of rural households in tourist destinations. J. Sustain. Tour. 30, 875–896. doi: 10.1080/09669582.2021.1903015
ILO (2017). World social report. Available at: https://www.ilo.org/wcmsp5/groups/public/—dgreports/—dcomm/—publ/documents/publication/wcms_604882.pdf (Accessed November 24, 2020).
Imbens, G. (2004). Nonparametric estimation of average treatment effects under exo-geneity: a review. Rev. Econ. Stat. 86, 4–29. doi: 10.1162/003465304323023651
Imbens, G., and Woolridge, J. (2009). Recent developments in the econometrics of program evaluation. J. Econ. Lit. 47, 5–86. doi: 10.1257/jel.47.1.5
Izudi, J., Tamwesigire, I. K., and Bajunirwe, F. (2019). Does completion of sputum smear monitoring have an effect on treatment success and cure rate among adult tuberculosis patients in rural eastern Uganda? A propensity score-matched analysis. PloS ONE 14:e0226919. doi: 10.1371/journal.pone.0226919
Karim, M. E., Pellegrini, F., Platt, R. W., Simoneau, G., Rouette, J., and de Moor, C. (2022). The use and quality of reporting of propensity score methods in multiple sclerosis literature: a review. Mult. Scler. J. 28, 1317–1323. doi: 10.1177/1352458520972557
Kavitha, H. N., Kumar, P., Anbukkani, P., Burman, R. R., Venkatesh, P., Jha, G. K., et al. (2020). Performance of universal basic income programme in India: a case of PM-KISAN scheme. Indian J. Ext. Educ. 56, 1–8. doi: 10.5958/2454-552X.2020.00001.8
Keele, L. J. (2010). An overview of rbounds: an R package for Rosenbaum bounds sensitivity analysis with matched data. Washington, DC: Georgetown University.
Khan, M. A., Alam, M. F., and Khan, J. L. (2012). The impact of co-management on household income and expenditure: an empirical analysis of common property fishery resource management in Bangladesh. Food Pol. 65, 67–78. doi: 10.1016/j.ocecoaman.2012.04.014
Khan, N., Fahad, S., Naushad, M., and Faisal, S. (2020). Analysis of livelihood in the world and its impact on world economy. Available at: https://ssrn.com/abstract=3717265 (Accessed October 22, 2020).
Khan, N., Ray, R. L., Kassem, H. S., Khan, F. U., Ihtisham, M., and Zhang, S. (2022). Does the adoption of mobile internet technology promote wheat productivity? Evidence from rural farmers. Sustainability 14:7614. doi: 10.3390/su14137614
Khatiwada, S. P., Deng, W., Paudel, B., Khatiwada, J. R., Zhang, J., and Su, Y. (2017). Household livelihood strategies and implication for poverty reduction in rural areas of Central Nepal. Sustain. For. 9, 1–20. doi: 10.3390/su9040612
Leroy, J. L., Ruel, M., and Vershofstadt, E. (2009). The impact of conditional cash transfer programmes on child nutrition: a review of evidence using a programme theory framework. J. Dev. Eff. 1, 103–129. doi: 10.1080/19439340902924043
Leuven, E., and Sianesi, B. (2012). PSMATCH2: Stata module to perform full Mahalanobis and propensity score matching, common support graphing, and covariate imbalance testing. Boston, MA: Statistical Software Components.
Liu, Y., Huang, C., Wang, Q., Luan, J., and Ding, M. (2018). Assessment of sustainable livelihood and geographic detection of settlement sites in ethnically contiguous povertystricken areas in the aba prefecture. China. Int. J. Geo-Inf. 7, 1–18. doi: 10.3390/ijgi7010016
Manley, J., Gitter, S., and Slavchevska, V. (2013). How effective are cash transfers at improving nutritional status? World Dev. 48, 133–155. doi: 10.1016/j.worlddev.2013.03.010
Mehta, V. (2019). The great Indian basic income debate. Vadodara: India Network for Basic Income Foundation.
Miller, C., and Tsoka, M. (2012). Cash transfers and children’s education and labour among Malawi’s poor. Dev. Policy Rev. 30, 499–522. doi: 10.1111/j.1467-7679.2012.00586.x
Miller, C., Tsoka, M., Reichert, K., and Hussaini, A. (2010). Interrupting the intergenerational cycle of poverty with the Malawi social cash transfer. Vulnerable Children Youth Stu. 5, 108–121. doi: 10.1080/17450120903499452
Mondal, B., Saha, R., and Samanta, A. (2020). Post-Amphan management and rejuvenation of the ravaged betel (Piper betle) baroj in South Bengal. Farming Manage. 5, 91–100. doi: 10.31830/2456-8724.2020.009
Natarajan, N., Newsham, A., Rigg, J., and Suhardiman, D. (2022). A sustainable livelihoods framework for the 21st century. World Dev. 155:105898. doi: 10.1016/j.worlddev.2022.105898
Negash, T., Etsay, H., Aregay, M., Kidu, G., and Machine, Z. (2023). Livelihood options of landless rural households in Tigrai region, northern Ethiopia: evidence from selected districts. Agric. Food Secur. 12:6. doi: 10.1186/s40066-023-00413-9
Nnaeme, C. C. (2021). How cash transfers activate beneficiaries’ decision-making in livelihood activities: a case of Soweto, South Africa. Dev. Southern Africa 38, 282–296. doi: 10.1080/0376835X.2020.1834352
NUEPA (2009). Educational development index (EDI): A suggestive framework for computation. New Delhi: Department of Educational Management Information System.
Nunan, F. (2022). “Livelihoods: concepts and frameworks” in The Routledge handbook on livelihoods in the global south. eds. S. Parnell and S. Oldfield (London: Routledge), 10–19.
OECD (2009). The role of employment and social protection: Making economic growth more pro-poor, Policy Statement, DAC High-level meeting. Paris: OECD.
Olken, B. A. (2019). Designing anti-poverty programs in emerging economies in the 21st century: lessons from Indonesia for the world. Bull. Indones. Econ. Stud. 55, 319–339. doi: 10.1080/00074918.2019.1690411
Pace, N., Sebastian, A., Daidone, S., Campos, A. P. D. O., Prifti, E., and Davis, B. (2022). Cash transfers’ role in improving livelihood diversification strategies and well-being: short-and medium-term evidence from Zimbabwe. World Dev. 154:105874. doi: 10.1016/j.worlddev.2022.105874
Paes-Sousa, R., and Santos, L. M. P. (2009). Measuring the impact of Bolsa Familia program based on data from health and nutrition days (Brazil). United Nations food and agriculture organization (FAO) hunger-free Latin America and the Caribbean initiative, Working Paper. Paris: FAO.
Pandey, R., Jha, S. K., Alatalo, J. M., Archie, K. M., and Gupta, A. K. (2017). Sustainable livelihood framework-based indicators for assessing climate change vulnerability and adaptation for Himalayan communities. Ecol. Indic. 79, 338–346. doi: 10.1016/j.ecolind.2017.03.047
Patnaik, S., and Prasad, C. S. (2014). Revisiting sustainable livelihoods: insights from implementation studies in India. Vision 18, 353–358. doi: 10.1177/0972262914553258
Paul, S., Das, T. K., Pharung, R., Ray, S., Mridha, N., Kalita, N., et al. (2019). Development of an indicator based composite measure to assess livelihood sustainability of shifting cultivation dependent ethnic minorities in the disadvantageous northeastern region of India. Ecol. Indic. 110:105934. doi: 10.1016/j.ecolind.2019.105934
Paxson, C., and Schady, N. (2007). Does money matter? The effects of cash transfers on child health and development in rural. Ecuador: World Bank Policy Research Working Paper.
Pellerano, L., Daidone, S., Davis, B., Farooq, M., Homayoun, M., Kardan, A., et al. (2016). “Does evidence matter? Role of the child grant programme in the consolidation of social protection sector in Lesotho” in From evidence to action: The story of cash transfers and impact evaluation in sub-Saharan Africa. eds. B. Davis, S. Handa, N. Hypher, N. W. Rossi, and P. Winters (Oxford: FAO, UNICEF, Oxford University Press), 247–280.
Pour, M. D., Barati, A. A., Azadi, H., and Scheffran, J. (2018). Revealing the role of livelihood assets in livelihood strategies: towards enhancing conservation and livelihood development in the Hara biosphere reserve, Iran. Ecol. Indic. 94, 336–347. doi: 10.1016/j.ecolind.2018.05.074
Rahman, S., and Akter, S. (2014). Determinants of livelihood choices: an empirical analysis from rural Bangladesh. J. South Asian Dev. 9, 287–308. doi: 10.1177/0973174114549101
Reddy, A. A., Sarkar, A., and Onishi, Y. (2022). Assessing the outreach of targeted development programmes—a Case study from a South Indian Village. Land 11:1030. doi: 10.3390/land11071030
Reddy, A. A., Sarkar, A., Rani, C. R., Das, A., Reddy, C. P., and Sneha, A. V. (2024). Mapping welfare and development schemes to SDGs at village level in India. SAARC J. Soc. Sci. 2, 27–59. doi: 10.2139/ssrn.4931929
Rosenbaum, P. R., and Rubin, D. B. (1983). The central role of the propensity score in observational studies for causal effects. Biometrika 70, 41–55. doi: 10.1093/biomet/70.1.41
Sadoulet, E., de Janvry, A., and Davis, B. (2001). Cash transfer programs with income multipliers: PROCAMPO in Mexico. World Dev. 29, 1043–1056. doi: 10.1016/S0305-750X(01)00018-3
Santos, M., and Santos, G. (2013). Composite indices of development. IDRC Research results. Available at: http://hdl.handle.net/10625/51550 (Accessed December 19, 2013).
Schlossarek, M., Syrovátka, M., and Vencálek, O. (2019). The importance of variables in composite indices: a contribution to the methodology and application to development indices. Soc. Indic. Res. 145, 1125–1160. doi: 10.1007/s11205-019-02125-9
Schreinemachers, P., Wu, M., Uddin, M. N., Ahmed, S., and Hanson, P. (2016). Farmers training in off-season vegetables: effects on income and pesticide use in Bangladesh. Food Pol. 61, 132–140. doi: 10.1016/j.foodpol.2016.03.002
Scoones, I. (2009). Livelihoods perspectives and rural development. J. Peasant Stud. 36, 171–196. doi: 10.1080/03066150902820503
Scoones, I. (2013). “Livelihoods perspectives and rural development” in Critical perspectives in rural development studies. ed. S. M. Borras (London: Routledge), 159–184.
Scorzafave, L., and Lima, E. (2010). Inequality in evolution in Brazil: the role of cash transfer programs and other income sources. Res. Econ. Inequality 18, 107–129. doi: 10.1108/S1049-2585(2010)0000018008
Sharma, A. K. (2019). Macro-economic impact of income support programme for farmers in India. J. Income Wealth 41, 177–187.
Singh, P. K., and Hiremath, B. N. (2010). Sustainable livelihood security index in a developing country: a tool for development planning. Ecol. Indic. 10, 442–451. doi: 10.1016/j.ecolind.2009.07.015
Tefera, T. (2013). Landownership-the path towards rural women empowerment: a case from southern Ethiopia. Int. J. Sociol. Anthropol. 5, 330–338. doi: 10.5897/IJSA2013.0481
van Daalen, K. R., Dada, S., James, R., Ashworth, H. C., Khorsand, P., Lim, J., et al. (2022). Impact of conditional and unconditional cash transfers on health outcomes and use of health services review. BMJ Glob. Health 7:e007902. doi: 10.1136/bmjgh-2021-007902
Varshney, D., Joshi, P. K., Roy, D., and Kumar, A. (2020). Pradhan Mantri Kisan Samman Nidhi (PM-KISAN) and the adoption of modern agricultural technologies in Uttar Pradesh, India (Vol. 1907). Intl. Food Policy Res. Inst. Available at: https://ssrn.com/abstract=3529623
Vyasulu, V. (2010). Brazil’s’ Fome zero’ strategy: can India implement cash transfers? Econ. Polit. Wkly. 45, 89–95.
Wang, E. (2015). Benchmarking whole-building energy performance with multi-criteria technique for order preference by similarity to ideal solution using a selective objective-weighting approach. Appl. Energy 146, 92–103. doi: 10.1016/j.apenergy.2015.02.048
Wang, C. C., Zhang, Y. Q., Yang, Y. S., Yang, Q. C., Kush, J., Xu, Y. C., et al. (2016). Assessment of sustainable livelihoods of different farmers in hilly red soil erosion areas of southern China. Ecol. Indic. 64, 123–131. doi: 10.1016/j.ecolind.2015.12.036
Wooldridge, J. M. (2010). Econometric analysis of cross section and panel data. London: The MIT Press.
World Bank (2010). Strengthening agricultural extension and advisory systems: Procedure for assessing, transforming and evaluating extension systems. Agriculture and Rural Development discussion paper 45. Washington, DC: World Bank.
Yen, S. T., Andrews, M., Chen, Z., and Eastwood, D. B. (2008). Food stamp program participation and food insecurity: An instrumental variables approach. J. Agri. Econ. 90, 117–132.
Keywords: cash transfers, direct benefit transfer, PM-KISAN, livelihood, impact, propensity score matching
Citation: Ghosh B, Burman RR, Padaria RN, Paul S, Mahra GS, Kumar P, Bhowmik A, Mallick S, Saini S, Gorai SK, Mukherjee S and Quader SW (2024) Performance of cash transfer program on farmers’ livelihood: evidence from PM-KISAN scheme of India. Front. Sustain. Food Syst. 8:1478795. doi: 10.3389/fsufs.2024.1478795
Edited by:
Anas Tallou, University of Bari Aldo Moro, ItalyReviewed by:
Vengadeshvaran Sarma, University of Nottingham, United KingdomA. Amarender Reddy, National Institute of Agricultural Extension Management (MANAGE), India
Bharat Ram Dhungana, Pokhara University, Nepal
Copyright © 2024 Ghosh, Burman, Padaria, Paul, Mahra, Kumar, Bhowmik, Mallick, Saini, Gorai, Mukherjee and Quader. This is an open-access article distributed under the terms of the Creative Commons Attribution License (CC BY). The use, distribution or reproduction in other forums is permitted, provided the original author(s) and the copyright owner(s) are credited and that the original publication in this journal is cited, in accordance with accepted academic practice. No use, distribution or reproduction is permitted which does not comply with these terms.
*Correspondence: Bhaskar Ghosh, YnVtYmFkb25ib3NjbzQyMDFAZ21haWwuY29t; Rajarshi Roy Burman, cmFqYXJzaGkuYnVybWFuQGljYXIuZ292Lmlu; Rabindra Padaria, cmFiaW5kcmFAaWFyaS5yZXMuaW4=