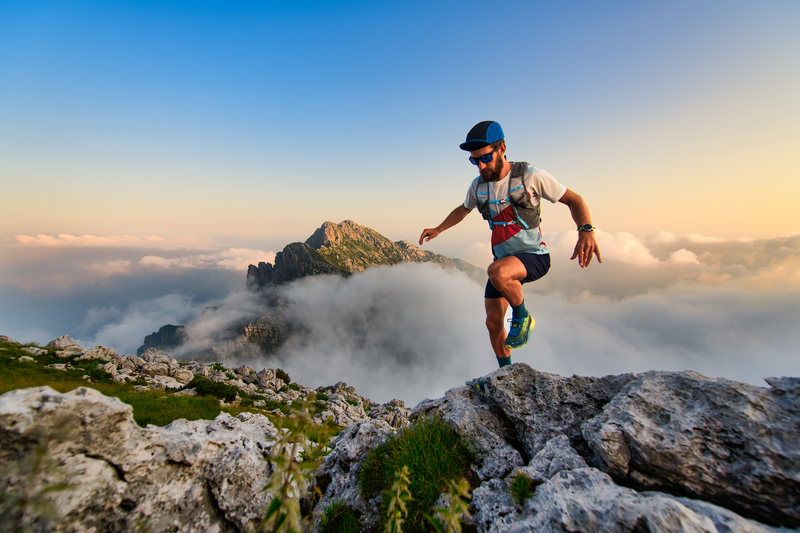
95% of researchers rate our articles as excellent or good
Learn more about the work of our research integrity team to safeguard the quality of each article we publish.
Find out more
METHODS article
Front. Sustain. Food Syst. , 13 November 2024
Sec. Social Movements, Institutions and Governance
Volume 8 - 2024 | https://doi.org/10.3389/fsufs.2024.1470442
The agricultural innovation system (AIS) is an approach that considers agricultural innovation from a multidisciplinary perspective. The Rapid Appraisal of Agricultural Innovation Systems is one of the commonly used operational methods. It involves a series of structured and participatory activities, including interviews, focus group discussions, and stakeholder workshops, to identify the actors, institutions, and relationships involved in the AIS, as well as the strengths and weaknesses of the system. This approach has been structured in such a manner that it adapts easily to different contexts where different stakeholders, such as developers, academicians, and policymakers are working with community members on innovation. A reflection on the concept -what to know (the requirements) and what to get (the outputs) is necessary for coming up with practical, reflective analysis and applicable procedures for a deeper understanding of the context where the innovations are introduced. Following this will enable us to fully understand the bottlenecks in transitioning from conventional systems to an agroecological model thus pinpointing potential entry points for promoting these systems’ dissemination and scaling.
Commonly, innovation is widely recognized as a key driver of economic development, resource efficiency, and societal progress (Jorgenson, 2011). It plays a crucial role in enhancing economic competitiveness and addressing global challenges (Santiago, 2014). Innovation measurement has expanded to capture a broader range of activities beyond technological product and process innovation (Bloch, 2007). This has led to a broader framework whereby innovation may be classified; for instance, product, process, and auxiliary types of innovation (Walker, 2006). In agriculture, innovation is focused on increasing output prices (both yield and income improvements), adapting to changes in the environment, handling social and economic problems, ensuring long-term sustainable land use and value chain development (Schut et al., 2014).
As a perspective, the innovation system has developed since the 1980s, when it was initiated with the ideas by Freeman (1991) and Lundvall et al. (2002). This approach focuses prominently on how different actors and institutions contribute to the development of innovation (Varblane and Tamm, 2012). Despite its widespread adoption, the concept faces challenges in setting system boundaries and providing a dynamic view. To address these issues, researchers have proposed focusing on the functional aspects of innovation systems (Varblane and Tamm, 2012), as seen for the agricultural innovation system introduced by Klerkx et al. (2012) highlighting the importance of knowledge brokering and innovation facilitation in fostering technical, social, and institutional change.
Currently, even though the linear view of innovation remains significant, innovation policy has started to shift from a supply driven approach, which focuses on R&D and specific technologies, towards a network structure. Emphasis is placed on fostering more comprehensive innovation, taking into consideration various ideas other than just being research based, as well as involving more actors so that they contribute to solutions for the most urgent problems surrounding agricultural systems. The changing paradigm of the agricultural innovation systems (AIS) recognizes these networks as a complex series of organizations or institutions and actors involved in the development, distribution, and scaling of agricultural innovations, such as farmers, researchers, policymakers, extension services, private sector agents as well as other actors (Nain et al., 2024). The process of innovating surpasses the mere technological changes to inclusive reforms in law, regulation, norms, and system dynamics that enlarge viability in innovation initiatives (Spielman et al., 2009).
The Rapid Appraisal of Agricultural Innovation Systems (RAAIS) is an evolution of earlier methodologies aimed at understanding and improving agricultural systems. It builds on concepts from Rapid Rural Appraisal (RRA) and Rapid Appraisal of Agricultural Knowledge Systems (RAAKS). These approaches share core principles: a systems perspective, triangulation of data collection, and iterative analysis (Beebe, 2008). RAAIS draws on and extends other concepts and theoretical pillars of agricultural innovation systems and uses a combination of different types of data. Collection offering a set of detailed entry points to innovation that help to overcome multifold agricultural challenges. RAAIS gives the possibility to find out who the main actors are as well as the institutions and relationships formulating an AIS. It will show its merits and demerits since its process involves activities like surveying, focus group discussions and meetings. Besides, motivation and hurdles towards innovation are revealed by the same process while showing prospects that can enhance the efficiency of AIS. RAAIS leads to a more proactive way of inclusive working in agricultural innovation that supports stakeholder driven processes of policy formulation, program development, and project implementation aiming at increasing agricultural productivity and ensuring sustainable agricultural development (Schut et al., 2015a).
Similarly, agroecology is a set of emerging transformative agricultural innovations, characterized by a holistic framework with a bundle of 13 principles that transcends mere techniques (Wezel et al., 2020). It embodies a system-level approach, acknowledging the interconnectedness of ecological, social, and economic factors within agricultural systems unlike conventional approaches (Migliorini and Wezel, 2017; Palomo-Campesino et al., 2018). At its core, agroecology recognizes the importance of studying, understanding and acting on various actors’ engagement and motivations (Gliessman, 2020). This will offer promising pathways towards more sustainable and inclusive agricultural development. This resemblance reflects that it becomes imperative to refine and adapt RAAIS to accommodate the specific challenges, opportunities, and dynamics inherent in agroecology transition, which can ensure that the assessment process accurately reflects the complexities of the transition process. These complexities are due to the structure of the various agricultural components and processes along the value chains, which are marked by several actors and stakeholders from agricultural and other related sectors. Additionally, agroecological innovations must account for different scales of operation, ranging from plot-level and farm-level to broader landscape and food system scales. An adapted RAAIS requiring different methodologies and guidelines becomes a necessity and forms the main objective of this research.
Based on Schut et al. (2015a) and Schut et al. (2015b) the conceptual framework of the Rapid Appraisal of Agricultural Innovation Systems (RAAIS) begins with the crucial step of stakeholders’ identification, ensuring that all relevant perspectives from sector actors are considered. This will ensure a comprehensive understanding of the complexity of agricultural problems from different dimensions. Then it aims to evaluate the innovation capacity through the assessment of existing capabilities and potential for innovation. Afterwards, it focuses on agricultural innovation support system identification, including all policies, institutions and networks that contribute positively or negatively to the innovation process. Finally, uncovering the interactions between the complexities of problems and the innovative capacity will define how these system components are interconnecting and influencing each other.
By definition, actors can be anybody who is directly or indirectly involved or connected to an issue or its possible solution (Voronov and Weber, 2020). The participants in the exploration of potential solutions to complex agricultural problems are known as stakeholders, and their involvement is seen as a crucial success element (Giller et al., 2011). In AIS, these stakeholders can be divided into 5 major groups (as cited in Table 1). This classification aims to involve an exhaustive multileveled list of actors to fulfill key innovation functions and cover different levels (farm, landscape, national) as suggested by (Lamers et al., 2017). This elementary step is constantly evident and crucial for any kind of network assessment (Schut et al., 2014).
Problems in agricultural systems that are complicated are usually not of one aspect and involve various parties (Schut et al., 2014). Problems of this nature have more than one dimension as they stem from a convergence of biophysical, technical, sociocultural, economic, institutional, and political factors. Soil quality and water availability might, for instance, represent the biophysical parts. Agricultural advances and technology would, however qualify under the technical dimensions. Social-cultural factors relate to community practices and tradition, economic elements relate to market access and financial resources, institutional aspect relates to policies and regulations governing agriculture and political element involves the influence of governmental and non-governmental organizations. These issues are deeply rooted in multi-level interactions from local farms and communities up to regional, national and even global systems (Schut et al., 2015a).
The capacity for innovation in the agricultural system refers to the capability of actors and organizations to leverage new and existing skills, including knowledge, aptitudes, and expertise, for continuous enhancement and adaptation (Nin-Pratt and Stads, 2023). This capacity involves not only the creation of new solutions but also the effective mobilization of existing resources to recognize and prioritize barriers to innovation and seize opportunities in a context of dynamic systems. Essentially, innovation capacity involves taking both anticipatory and responsive measures, always in motion, and responding to new possibilities and obstacles (Van Mierlo et al., 2010; Wieczorek and Hekkert, 2012).
The agricultural innovation support system provides the structural circumstances that can promote innovation within the agricultural system and its subsystems if they are available or inhibit them if they are absent or non-functional (Wieczorek and Hekkert, 2012). In order to promote innovation, there are four crucial structural conditions that need to be fulfilled. These are infrastructure and assets; institutions; interaction and collaboration; capabilities and resources. Infrastructure and assets are basic facets essential to innovation. These assets could contain the base of knowledge and institutions of learning associated to material infrastructure like roads, dams, and suitable climate. In terms of institutions innovations require effective institutions, which consist of agricultural policies, laws and regulations, and informal institutions such as social and cultural norms and values. Innovation is at its best in environments where stakeholders engage and work together; this includes multi-stakeholder encounters for joint learning and problem solving, knowledge and information dissemination, public-private collaborations, as well as collective bodies such as cooperatives or farmer associations. The availability and quality of human resources, including qualifications, education, and literacy levels, as well as easily accessible financial resources, are essential to creating an enabling environment for innovation (Schut et al., 2015b).
Analyzing deeply interconnected problems in agriculture, the capacity of the system to generate new ideas and innovations, facilitates the identification of particularized approaches or windows of innovations. Understanding the capabilities, gaps, and effectiveness of support mechanisms can assist in the co-identification of context-relevant and viable solutions. This approach aims that interventions will be both contextualized and specific enough to address certain requirements and generic enough to foster general system enhancements. Under a holistic view, it is possible to come up with innovative designs that are both technically feasible and socially just as well as economically viable and sustainably enhancing resilient agricultural sector (Schut et al., 2014).
In general, the term agroecology has been given varied interpretations and meanings and has been used as a description for social movements and a set of agricultural practices (Wezel et al., 2009, 2020). Agroecology, being defined in different ways and languages by different populations, shows how complex and dynamic it is given the influence of global cultural and linguistic differences on it (Wezel et al., 2009). As a scientific discipline, agroecology is dedicated to study how plants, animals, and humans all interact within their environment. It involves such disciplines as agronomy, soil science, economics, as well as rural sociology. Scientific agroecology intends to comprehend thoroughly how agricultural undertakings affect natural systems and communities, otherwise socio-ecosystems (Wezel et al., 2009; Gliessman, 2020). In the recent literature, the list of agroecology principles includes 13 elements, which are recycling, input reduction, soil health, animal health, biodiversity, synergy, economic diversification, co-creation of knowledge, social values and diets, fairness, connectivity, land and natural resource governance, and participation (Wezel et al., 2020).
Agroecology’s journey started in the first half of the twentieth century concentrating on plot or field levels. From the mid-1930s to the 1960s, it focused on the environment’s interior of these plots and how different species interacted with each other, specifically looking at soil, crops and surrounding wildlife. This provided the groundwork for understanding simple ecological principles in agricultural contexts, but with time it grew bigger. By the seventies, agroecology began taking into account complete farming units and later on far more complex units, such as landscapes, and eventually whole food systems. The need for a full understanding as well as the achievement of agricultural sustainability explained this shift. Instead of looking at individual parts or elements in isolation, it now demands a synthesis that incorporates social, economic, and environmental aspects within which interactions among them would be considered (Wezel et al., 2009; Sevilla Guzmán and Woodgate, 2013).Contemporary agroecology with its multiple’s dimensions necessitates a multitiered and inter-disciplinary approach in order to keep pace. Food production processes, processing and marketing dynamics, economic policies, political decisions, and consumer behaviors all need to be studied due to the complicated nature of agroecosystems. The interconnections between these facets are inextricable across scales, temporalities, and complexities. Nevertheless, despite the breadth of agroecology’s scope, clear ideas or analytical approaches capable of combining all these dimensions are still lacking. This vagueness and ambiguity can be seen as a weakness since it makes planning and implementing agroecological research and practice strategies difficult. Nevertheless, it shows how this area is dynamic and changeable, hence aiding in coming up with alternatives to the various bottlenecks of sustainable agriculture (Méndez et al., 2013).
As a movement, agroecology includes initiatives from various perspectives designed to boost food security, including food sovereignty and self-sufficiency. This includes farmers’ groups involved in rural renewal advocacy, activists’ movements for sustainable and equal farming policies, and also strategic alliances that undertake environmental action. It focuses also on the significance of community engagements and indigenous information for successful agriculture systems. The practitioners recommend practices that would help enhancing biodiversity, improving soil health while reducing external input use. Their main aim is creating efficient food systems through the reinforcement of economic efficiency and sharing the common responsibilities (Rosset and Martínez-Torres, 2012; Zaremba et al., 2021).
As practices, agroecology is diverse and adaptable. It includes innovative, modified or traditional techniques that foster sustainable and environmentally-friendly agriculture such as organic farming, integrated pest management, agroforestry, and permaculture. The aim of these practices is to boost ecological processes and minimize adverse environmental consequences while keeping up farm productivity at a certain level. Which require a profound knowledge about ecological basics (Wezel et al., 2014; Bezner Kerr et al., 2021).
What Agroecology does is that it fits right into the point where agriculture, environment, and sociology intersect with the intention of creating sustainable food systems able to cope under exogenous and indigenous pressures. In other words, when it comes to Agroecology, one of its principal dilemmas involves making sense amid all these different fields and dimensions (as presented in Figure 1). An integrative approach must be taken that goes beyond traditional considerations. It must reflect an interlinkage between agriculture on the one hand and environment and social studies on the other. Such an approach provides an indication that community-based agroecology can be used as a tool to promote not only sustainable food production but also sustainable development. This means considering ecological, health and socio-economic aspects of agriculture and food production to advance agroecology as a pathway for system transformation toward sustainability (Méndez et al., 2013; Cremilleux et al., 2023). Agroecology is considered a policy instrumental approach that strives to transform the food system (Tittonell et al., 2020). It advocates for policies that support sustainable agricultural practices, promote food sovereignty, and increase food systems’ resilience. It involves promoting actions that support crop diversification, enhance soil fertility, and reduce chemicals’ use by aligning regulations with ecological concepts and agroecological principles (Gliessman, 2020).
Figure 1. Agroecology as a perspective (own elaboration based on Wezel et al., 2009, 2014, 2020; Méndez et al., 2013; Migliorini and Wezel, 2017; Bezner Kerr et al., 2021; Cremilleux et al., 2023).
The concept of agroecology has shifted from its basic description as the practical application of ecology for agricultural purposes and having a multidisciplinary and transdisciplinary approach where different knowledge systems are melded together and applied for common objectives. Generally, this approach addresses the food systems comprehensively, encompassing aspects of ecology, sociality and economy (Tress et al., 2005; Méndez et al., 2015). Transdisciplinary agroecology connects academic and non-academic insights associated with local knowledge under the participatory action research (PAR) approach to come up with co-created and contextually relevant solutions (Méndez et al., 2013; Méndez et al., 2015).
In the context of agricultural innovation, it’s fundamental that agroecology is rooted deeply in it. Concretely, any new farming method may lead to significant changes both in terms of costs and profits, as well as in relation to natural resources use. A set of examples, such as agroforestry, cover cropping, or integrated pest management, can improve productivity, foster food security for communities, and contribute to natural resources conservation while promoting social justice simultaneously (Aguilera et al., 2020). These advancements usually result from a process of knowledge co-creation among various sectors’ actors. With local participation, agroecological innovations will suit local specificities, needs, and challenges. Combining traditional knowledge and heritage to agroecological concepts will create a well contextualized technology (Tomich et al., 2011).
In agroecology networks’ studies have a crucial role to play, especially in analyzing cropping systems’ resilience and biodiversity reserve networks. It can also assess vulnerability and adaptive capacity under fast societal or environmental alterations (Labeyrie et al., 2021). Analyzing innovation networks offers important insights about agroecosystem dynamics as well as practical pathways to foster adaptability and enhance food system resilience (Dapilah et al., 2019). Also, studying the interconnectedness between various components or actors is crucial. As within agricultural innovation systems (AIS), diverse paradigms of agroecology could be advocated by various stakeholders, each with distinct priorities and interpretations. These paradigmatic differences can generate competition or complementarities, influenced by prevailing power dynamics and stakeholder interactions that must be taken into consideration (Gasselin et al., 2020; Schnebelin et al., 2021). Additionally, improving the innovative potential of these networks will reinforce the core stakeholders with an inestimable adaptative capacity facing environmental and socio-economic pressures (Castella et al., 2022).
Understanding the capabilities, gaps, and effectiveness of support mechanisms can assist in the co-identification of context-relevant and viable solutions. This approach aims that interventions and innovations will be both contextualized, responding to existing reasons behind, in a timely manner and specific enough to address certain requirements, as well as generic enough to foster wider systematic enhancements. Under a holistic view, it is possible to come up with innovative designs that are both technically feasible and socially acceptable, as well as economically viable and sustainably enhancing resilient agricultural sector.
The protocol suggested (Table 2) describes functional analysis for each level of AIS in the form of segmental questions to be addressed. Within each functional analysis, a recommended analysis can be found, and other possible analyses are supplied in case the recommended one is impractical. Each group of recommended analyses is designed to support one of the five main objectives, each objective accompanied by tailored data collection protocols. Then, each of these objectives is related to certain sets of agroecological principles. For example, to address the first objective, which is to illustrate production systems, measure resilience and evaluate differences between farmer households, specific methods are suggested, which are, respectively, PCA, RIMA and PLS. These analyses directly correspond to principles 1, 2, 4, 6, 7, and 9, assuming data collection through effective survey protocols.
The following sections contain the operational steps required to achieve the desired outcomes for each specific objective (cited in Table 1). The required data for each objective is defined, ensuring that all necessary specifications are provided. As well as the software tools to be utilized for analysis of data are indicated. Otherwise, a detailed roadmap is provided for meeting the aim by systematically explaining how data will be gathered, analyzed, and discussed.
Before starting, the research domain’s size must be outlined geographically. The survey must comprise a representative sample of farmers who actually reflect the varied information that is requisite for upscaling, verifying any assumptions, and producing evidence. The sample ought to be conceived in its entirety, including underrepresented households and farmers, so it could serve as an all-encompassing picture of agroecosystems. If the survey has to be accurate, the persons to be interviewed must be individuals who have broad knowledge about the household and farming practices. In order to achieve maximum understanding and get high-quality responses, the questionnaire should take place in the local dialect. It begins with seeking permission and meeting the requirements of ethics. Also, it carries on logically through different parts for fear of repetition. The families’ demographic attributes are explored in the first section with a focus on their size, learning qualifications, years, and exposure to farming activity. Thereafter, questions are raised about the size of land and its features, such as whether it is irrigated or rain-fed, the level of fertility as well as who owns it. Furthermore, the questionnaire asks whether or not there are any financial resources, where they come from and how much they amount to. Afterwards, the farming is defined by questions focusing on the main crops, productivity, yield, animal raising practices as well as other related activities namely; grazing and vaccination. The next set of questions addresses the use of inputs, including fertilizers and animal feed, followed by those seeking to find out more about production targets: where products are going after production, are they for self-consumption only, or is there any marketing involved in their distribution. In the next section, questions about diets and patterns of consumption are raised. Also, among our foci, it will be access to basic services such as schools, water provision, and, lastly, how households experience shocks and what strategies they use in response.
Once collected, the data must be uniform and standardized. Two main sets must be distinguished: a typology of households and farming profiles as well as a measure of resilience. The data can be used for analysis using a number of software programs like IBM SPSS, STATA or R that can handle PCA and the calculations. This is then followed by exporting the data into CSV format which is compatible with smart PLS software for modeling after identifying the theoretical model.
Thereafter, the RIMA framework (Mondal et al., 2023) is used to generate the index calculation, after which a Multi-Group Analysis (MGA) is performed to compare the different model versions among groups. The model should match certain conditions so that it would explain an acceptable proportion of variance. The resulting outcomes are assembled within one single Excel document, which should be scrutinized in order to yield some figures in a digital format. Thus, a more elaborate comprehension of farming systems is gained through the operational steps explained herein that outline different attributes for each category of farmers. This allows for sampling based specifically on the group’s characteristics with regard to undertaking possible surveys or carrying out demonstrations. The study can be used to show the types of agriculture through spider diagrams or profile sections, commonly used in typology studies and geography. The level of holistic resilience displayed by individual farmers is captured by the agricultural resilience index, concurring with the fundamental values of agroecology. Mapping this indicator provides critical leverage points for advancing agroecology by illustrating how some factors can be more relevant for resilience than others within different contexts.
The first step is to identify the geographic reach of the study to make sure it encompasses a clearly delineated landscape. Picked grids or limits should be meaningful, for example, altitude or rainfall, or any other strict selection criterion. Besides, one needs to define the evaluation period, i.e., 10 years ago. The most accurate and publishable information must be retrieved from certified global servers such as ESRI and MODIS during data collection or processed from Sentinel-2 Imagery. Raster format will be chosen when downloading Land use/Land cover (LULC) data with the most reliable resolution being 30×30 meters and 10×10 meters for MODIS and ESRI, respectively.
Two methods would be used to verify this data; in-person GPS points that are gathered during another survey and also Google Earth images. For every stage, one hundred points per LULC class will be selected as a set validating different types of land covers. The accuracy level needs to be checked (a value of 80% is considered good, 90% and exceeding is considered perfect, with only 10% margins for errors). After that – as soon as field data has been approved in this form – we’ll employ proper analysis for its processing.
FRAGSTATS or LecoS and Semi-Automatic Classification plugins with QGIS can be used to generate land use maps, landscape metrics, and land cover change data. This involves defining the change matrix similar to those used in Trends.Earth frame and aligning with best practice guide document. The software automatically generates output results; nonetheless, time consumed in this process may vary from 1 min to as much as a few hours due to the RAM capacity of a given computer. This means that one can export these results into other tabular software such as SPSS, Excel among others, for further analysis.
The results presented here enable a better comprehension of the state of different land use and land cover at one point in time in terms of which ones are degraded or improving. Moreover, they show whether there have been similar shifts in scale or percentage throughout history. In addition, the research assesses natural habitat fragmentation and ecosystem services by means of different metrics or indicators like Patch Density (PD), Contagion Index (CONTAG), and Shannon’s Diversity Index (SHDI). This step uncovers vital information about the dynamics of agroecosystems at the landscape level, which assists in the formulation of specific conservation and management strategies.
To begin this process, the actors at three levels—local, intermediate, and national levels- should be identified first. The identification must focus on powerful actors whose influence on the studied area is strong, including those that have direct contact with innovations’ final users. A specific survey about social networks is going to take place within the nearby community and between primary actors who collaborate around four main areas: relationships, service provision, technical know-how, and information. This survey includes determining how often people collaborate together and why, as well as rating Likert scale scores to evaluate the level of satisfaction by these collaborations.
Once all necessary information is gathered, the next step involves splitting the data obtained into two different CSV files: one that contains nodes (the actors) while the other comprises edges (the collaboration). Thereafter, this data can now be analyzed using network analysis tools such as Gephi. In order to produce clear illustrations, some formatting and editing steps are required. Indeed, social network indicators can be generated following certain steps and commands in the software. In conformity with what most publishers demand, these pictures may also be saved and exported in JPEG format at 300 dots per inch (dpi) resolutions. The tables can be directly exported in Excel format.
The generated results, including illustrations and centrality analysis, provide deeper insights into the institutional landscape and how it functions. It also helps in identifying the strongest, most powerful actors within the network. Otherwise, it also helps in assessing how effective the key actors in the Agricultural Innovation System (AIS) are. As, for better strategy formulation aiming for AIS efficiency and impact improvement, actors’ roles and interactions should be mapped and understood by stakeholders.
This process commences by recognizing significant national strategic interventions that serve in deliberations on the foremost policy issues impacting upon agroecology principles, after which well-known actors on specific policy dimensions undergo identification from a review of gray literature. Afterwards, a specialized, semi-structured interview will be necessary for every single actor in question. The person being interviewed has to know how policy unfolded over time and its most critical episodes. Thereafter, the gathered information goes through an analytical process meant to highlight where power shifts took place and bring into focus existing institutional frameworks in place at that time.
After analysing these aspects, there is a need to organize a multi-policy actors’ workshop to chart out a national policy schematic that shows how authority, finance and information flow. Topic modelling methods can help improve analytic quality for grey literature in some cases. However, this is only possible with numerical versions of every document involved, including official reports that are available in hard copies only.
This step gives more insight on the policy actors’ landscape vis-à-vis agroecology, thereby targeting the future investment efforts through which innovation capacity can be strengthened, awareness increased, as well as support and advocacy done on behalf of agroecology. Additionally, it shows who supports, who opposes and who is neutral at different times about agroecology. This information is gathered by studying the positions of champions, supporters and opponents of agroecology to understand how they might change in the future.
To begin this process, the initial step would be identifying the key subjects of the review so that they are representative. Such is selecting commodities that mirror both national strategic options and priorities as well as the economic worth of the sub-sectors. Factors to consider incorporate import value, extent, exports, and the inputs with regard to the agroecology principles that may affect them detrimentally or favorably. All useful documents, numerical figures and datasets, research reports, notes of prospective value technical analysis, and legislative texts must be considered. These resources are afterwards categorized in order to select the most relevant information for conducting deeper analysis.
The economic context and its relationship to agroecology are clearly traced in narrative or illustrative sections where the outcomes can be presented. Such a method aids in revealing those macro factors that are not seen but support or inhibit transformative innovations such as agroecology. By understanding these factors, stakeholders can better strategize to support policies and incentives that enhance and foster the adoption of agroecology as a practice and as an approach for sustainable intensification.
RAAIS helps in identifying issues and challenges such as insufficient institutional support and guidance, scarcity of resources, backward economies, which directly affect agroecological transition (Schiller et al., 2020). It effectively assesses constraints across diverse system levels, highlighting techno-economic barriers such as capital and production costs (Seguin et al., 2021). However, RAAIS has some drawbacks in regard to relaying the multiplex interaction between constraints and their roles in the manifestation of general blocking factors more broadly, which might conceal the extent of difficulties within agricultural transformation processes (Seguin et al., 2021). A mission-oriented agricultural innovation systems (MAIS) approach can complement RAAIS to identify synergistic objectives and consider the systematic drivers of food system changes, and it brings out a clear course on the visionary goals toward agroecological transition (Klerkx and Begemann, 2020).
Similarly, the suggested methods contain advantages and disadvantages that must be considered in any application to ensure achieving the research objectives and reduce the error probability. Following the order of the suggested setup, starting from Principal Components Analysis is advantageous, as it facilitates handling with large datasets with numerous variables, identifies the most critical variables driving variation and visualizes complex relationships in a low-dimensional space (Lever et al., 2017) which is the case when studying agroecological transitions. However, PCA is sensitive to the scale of the variables and requires careful interpretation of the principal components. Additionally, it may lose some information when reducing dimensionality (Jolliffe and Cadima, 2016). Whereas measuring a broad concept such as resilience can be done by Resilience Index Measurement and Analysis Model (RIMA-II), which offers a standardized way of measurement that can be used for comparisons across different contexts and to establish which are the most important factors that underpin resilience (Alinovi et al., 2010; FAO, 2016). However, self-generated information is likely to give rise to some certain kind of bias in the results, which requires getting hold of a large number of participants to ensure that the sample is really representative of the whole population so that there will be no overlooking of certain important aspects associated with resilience (Egamberdiev et al., 2023). Additionally, it is crucial to note that if chosen as the fitting technique, Partial Least Squares Regression (PLS) has the advantage of dealing with multicollinearity in predictors, performing optimally with small sample sizes (Firinguetti et al., 2017). However, there are several drawbacks associated with PLS; it is affected by outliers, the number of latent variables needs to be selected with care, and the model tends to overfit (Rönkkö et al., 2016; Dhehibi et al., 2023). Likewise, Landscape Metrics and Intensity Analysis provide an objective measurement of landscape morphology, enable comparisons across landscapes, and delineate hotspots of change (Lausch et al., 2015; Shiri et al., 2024) However, these metrics depend on the spatial resolution and the extent of data, and are unable to capture all landscape functions and need specialized tools and expertise (Uuemaa et al., 2013; Lausch et al., 2015).Furthermore, Social Network Analysis (SNA) is beneficial in the aspect of identifying the key actors, mapping communities, and the detection of patterns of cooperation (Tabassum et al., 2018). The drawbacks include self-reported survey-based information, which could be inaccurate or incomplete, excluding some contextual variables important for analysis, and the requirement of specific software and competencies (Ouerghemmi et al., 2024; Borgatti et al., 2009). Also, the Kaleidoscope Model Application offers a systematic framework to analyze the political economy aspects of policy shifts, map relevant stakeholders and their motivations, and formulate hypotheses about future actions. Nevertheless, it entails significant data gathering and examination, can omit some details of the policy process, and is not immune to the predispositions and assumptions of the researcher (Resnick et al., 2018; Haggblade et al., 2019). Lastly, systematic reviews are supposed to systematically aggregate, evaluate, and summarize all available studies related to a particular question. Meta-analyses offer timely and balanced synthesis of the findings and apply transparent and replicable techniques while posing the least risk of bias. Nonetheless, there are several limitations when conducting systematic reviews, including that they are lengthy and costly processes, depend on the quality of the included studies, and there is the possibility of not identifying other related studies (Sánchez et al., 2014).
The goodness of the data retrieved and the inclusiveness and lack of bias in the process determine the quality of the respective outputs of each suggested methodology. This protocol implementation requires an interdisciplinary team considering the system from different angles. Both concepts and operations of agroecology and innovation are not simple interventions that can be inserted into a system to induce an instant transformation. The vague and broaden nature of these terms—agroecology on one hand and agricultural innovations on the other – create a degree of ambiguity. This work aims to provide a bundle of operational tools applicable in any context, system, or country. It presents an operational approach or toolbox that can help in conducting research into the feasibility of agroecology transitions. This approach helps to recognize and identify entry points for interventions to ensure effective innovation transfer (also called windows for innovations) and a transformative process (also called transition pathways) that benefits agroecosystems and food systems.
The original contributions presented in the study are included in the article/supplementary material, further inquiries can be directed to the corresponding author.
HO: Conceptualization, Formal analysis, Methodology, Writing – original draft, Writing – review & editing. AF: Funding acquisition, Project administration, Resources, Writing – original draft, Writing – review & editing. ZS: Formal analysis, Methodology, Writing – original draft. HR: Supervision, Validation, Writing – review & editing.
The author(s) declare that financial support was received for the research, authorship, and/or publication of this article. This work was supported by the One CGIAR initiative on “Transformational Agroecology across Food, Land and Water Systems” under a grant agreement (#200302) with the International Center for Agricultural Research in the Dry Areas (ICARDA - https://www.icarda.org/).
The authors declare that the research was conducted in the absence of any commercial or financial relationships that could be construed as a potential conflict of interest.
All claims expressed in this article are solely those of the authors and do not necessarily represent those of their affiliated organizations, or those of the publisher, the editors and the reviewers. Any product that may be evaluated in this article, or claim that may be made by its manufacturer, is not guaranteed or endorsed by the publisher.
The opinions expressed here belong to the authors and do not necessarily reflect those of ICARDA or CGIAR.
Adachi, K. (2019). Factor analysis: latent variable, matrix decomposition, and constrained uniqueness formulations. WIREs Comput. Stat. 11:e1458. doi: 10.1002/wics.1458
Aguilera, E., Díaz-Gaona, C., García-Laureano, R., Reyes-Palomo, C., Guzmán, G. I., Ortolani, L., et al. (2020). Agroecology for adaptation to climate change and resource depletion in the Mediterranean region. A review. Agric. Syst. 181:102809. doi: 10.1016/j.agsy.2020.102809
Akinyemi, F. O., Pontius, R. G., and Braimoh, A. K. (2017). Land change dynamics: insights from intensity analysis applied to an African emerging city. J. Spat. Sci. 62, 1–15. doi: 10.1080/14498596.2016.1196624
Alinovi, L., Mane, E., and Romano, D. (2010). Measuring household resilience to food insecurity: application to Palestinian households. Agric. Survey Methods, 341–368. doi: 10.1002/9780470665480.ch21
Altbach, P. G. (2013). Advancing the national and global knowledge economy: the role of research universities in developing countries. Stud. High. Educ. 38, 316–330. doi: 10.1080/03075079.2013.773222
Beebe, J. (2008). Basic concepts and techniques of rapid appraisal. Hum. Organ. 54, 42–51. doi: 10.17730/humo.54.1.k84tv883mr2756l3
Bezner Kerr, R., Madsen, S., Stüber, M., Liebert, J., Enloe, S., Borghino, N., et al. (2021). Can agroecology improve food security and nutrition? A review. Glob Food Sec 29:100540. doi: 10.1016/j.gfs.2021.100540
Bizikova, L., Nkonya, E., Minah, M., Hanisch, M., Turaga, R. M. R., Speranza, C. I., et al. (2020). A scoping review of the contributions of farmers’ organizations to smallholder agriculture. Nat. Food 1, 620–630. doi: 10.1038/s43016-020-00164-x
Bloch, C. (2007). Assessing recent developments in innovation measurement: the third edition of the Oslo manual. Sci. Public Policy 34, 23–34. doi: 10.3152/030234207X190487
Borgatti, S. P., Mehra, A., Brass, D. J., and Labianca, G. (2009). Network analysis in the social sciences. Science 323, 892–895. doi: 10.1126/science.1165821
Castella, J.-C., Lestrelin, G., Phimmasone, S., Tran Quoc, H., and Lienhard, P. (2022). The role of actor networks in enabling Agroecological innovation: lessons from Laos. Sustain. For. 14:550. doi: 10.3390/su14063550
Clark, J. (1995). The state, popular participation, and the voluntary sector. World Dev. 23, 593–601. doi: 10.1016/0305-750X(94)00147-Q
Cremilleux, M., Michaud, A., Cayre, P., Martin, B., Rigolot, C., and Michelin, Y. (2023). Combining systemic and pragmatic approaches for the holistic diagnosis of a farm in agroecological transition in a health context. Front. Sustain. Food Syst. 7:875820. doi: 10.3389/fsufs.2023.875820
Dapilah, F., Nielsen, J., and Friis, C. (2019). The role of social networks in building adaptive capacity and resilience to climate change: a case study from northern Ghana. Clim. Dev. 12, 42–56. doi: 10.1080/17565529.2019.1596063
Dhehibi, B., Dhraief, M. Z., Frija, A., Ouerghemmi, H., Rischkowsky, B., and Ruediger, U. (2023). A contextual ICT model to explain adoption of mobile applications in developing countries: a case study of Tunisia. PLoS One 18:e0287219. doi: 10.1371/journal.pone.0287219
Egamberdiev, B., Bobojonov, I., Kuhn, L., and Glauben, T. (2023). Household resilience capacity and food security: evidence from Kyrgyzstan. Food Secur. 15, 967–988. doi: 10.1007/s12571-023-01369-1
FAO (2016). Resilience index measurement and analysis—II. Food and Agriculture Organization of the United Nations Rome.
Firinguetti, L., Kibria, G., and Araya, R. (2017). Study of partial least squares and ridge regression methods. Commun. Stat. Simul. Comput. 46, 6631–6644. doi: 10.1080/03610918.2016.1210168
Freeman, C. (1991). Networks of innovators: a synthesis of research issues. Res. Policy 20, 499–514. doi: 10.1016/0048-7333(91)90072-X
Gasselin, P., Lardon, S., Cerdan, C., Loudiyi, S., and Sautier, D. (2020). The coexistence of agricultural and food models at the territorial scale: an analytical framework for a research agenda. Rev. Agric. Food Environ. Stud. 101, 339–361. doi: 10.1007/s41130-020-00119-7
Gewers, F. L., Ferreira, G. R., Arruda, H. F., Silva, F. N., Comin, C. H., Amancio, D. R., et al. (2021). Principal component analysis: a natural approach to data exploration. ACM Comput. Surv. 54, 1–34. doi: 10.1145/3447755
Giller, K. E., Tittonell, P., Rufino, M. C., van Wijk, M. T., Zingore, S., Mapfumo, P., et al. (2011). Communicating complexity: integrated assessment of trade-offs concerning soil fertility management within African farming systems to support innovation and development. Agric. Syst. 104, 191–203. doi: 10.1016/j.agsy.2010.07.002
Gliessman, S. R. (2020). Transforming food and agriculture systems with agroecology. Agric. Hum. Values 37, 547–548. doi: 10.1007/s10460-020-10058-0
Haggblade, S., Babu, S. C., Resnick, D., and Hendriks, S. L. (2019). “The policymaking process: introducing the kaleidoscope model for food security policy analysis” in Food security policy, evaluation and impact assessment (London: Routledge), 88–99.
Jolliffe, I. T., and Cadima, J. (2016). Principal component analysis: a review and recent developments. Philos. Trans. R. Soc. A Math. Phys. Eng. Sci. 374:20150202. doi: 10.1098/rsta.2015.0202
Jorgenson, D. W. (2011). Innovation and productivity growth: T.W Schultz Lecture. Am. J. Agric. Econ. 93, 276–296. doi: 10.1093/ajae/aaq191
Klerkx, L., and Begemann, S. (2020). Supporting food systems transformation: the what, why, who, where and how of mission-oriented agricultural innovation systems. Agric. Syst. 184:102901. doi: 10.1016/j.agsy.2020.102901
Klerkx, L., van Mierlo, B., and Leeuwis, C. (2012) in Evolution of systems approaches to agricultural innovation: Concepts, analysis and interventions BT - farming systems research into the 21st century: The new dynamic. eds. I. Darnhofer, D. Gibbon, and B. Dedieu (Dordrecht: Springer Netherlands), 457–483.
Labeyrie, V., Antona, M., Baudry, J., Bazile, D., Bodin, Ö., Caillon, S., et al. (2021). Networking agrobiodiversity management to foster biodiversity-based agriculture. A review. Agron. Sustain. Dev. 41:4. doi: 10.1007/s13593-020-00662-z
Lamers, D., Schut, M., Klerkx, L., and van Asten, P. (2017). Compositional dynamics of multilevel innovation platforms in agricultural research for development. Sci. Public Policy 44, 739–752. doi: 10.1093/scipol/scx0009
Lausch, A., Blaschke, T., Haase, D., Herzog, F., Syrbe, R.-U., Tischendorf, L., et al. (2015). Understanding and quantifying landscape structure – a review on relevant process characteristics, data models and landscape metrics. Ecol. Model. 295, 31–41. doi: 10.1016/j.ecolmodel.2014.08.018
Lever, J., Krzywinski, M., and Altman, N. (2017). Principal component analysis. Nat. Methods 14, 641–642. doi: 10.1038/nmeth.4346
Lundvall, B.-Å., Johnson, B., Andersen, E. S., and Dalum, B. (2002). National systems of production, innovation and competence building. Res. Policy 31, 213–231. doi: 10.1016/S0048-7333(01)00137-8
Méndez, V., Bacon, C., and Cohen, R. (2013). Agroecology as a transdisciplinary, participatory, and action-oriented approach. Agroecol. Sustain. Food Syst. 37, 3–18. doi: 10.1080/10440046.2012.736926
Méndez, V. E., Bacon, C. M., Cohen, R., and Gliessman, S. R. (2015). Agroecology: A transdisciplinary, participatory and action-oriented approach. Boca Raton: CRC press.
Migliorini, P., and Wezel, A. (2017). Converging and diverging principles and practices of organic agriculture regulations and agroecology. A review. Agron. Sustain. Dev. 37:63. doi: 10.1007/s13593-017-0472-4
Mondal, M., Biswas, A., Mandal, S., Bhattacharya, S., and Paul, S. (2023). Developing micro level resilience index for Indian Sundarban adopting resilience indicators for measurement and analysis (RIMA) methodology. Geosyst. Geoenviron. 2:100129. doi: 10.1016/j.geogeo.2022.100129
Nain, M. S., Rashmi, S., Mishra, J. R., and Singh, A. K. (2024). Developing model for diffusion of farmers’ innovations for maximizing farm income: Indian Agricultural Research Institute experiences. Indian J. Extens. Educ. 60, 105–110. doi: 10.48165/IJEE.2024.60120
Nair, P. (2011). Evolution of the relationship between the state and non-government organisations: a south asian perspective. Public Adm. Dev. 31, 252–261. doi: 10.1002/pad.610
Nie, F., Li, Z., Wang, R., and Li, X. (2023). An effective and efficient algorithm for K-means clustering with new formulation. IEEE Trans. Knowl. Data Eng. 35, 3433–3443. doi: 10.1109/TKDE.2022.3155450
Nin-Pratt, A., and Stads, G.-J. (2023). Innovation capacity, food system development, and the size of the agricultural research system. Front. Sustain. Food Syst. 7:1051356. doi: 10.3389/fsufs.2023.1051356
Ouerghemmi, H., Frija, A., Souissi, A., Carpentier, I., Shiri, Z., Dhehibi, B., et al. (2024). Are farmer organizations effective intermediaries and facilitators of agricultural innovations processes? Evidence from Tunisia. Front. Sustain. Food Syst. 8:1440086. doi: 10.3389/fsufs.2024.1440086
Palomo-Campesino, S., González, J. A., and García-Llorente, M. (2018). Exploring the connections between Agroecological practices and ecosystem services: a systematic literature review. Sustain. For. 10:339. doi: 10.3390/su10124339
Prautzsch, H., and Boehm, W. (2018). Geometric concepts for geometric design. New York: AK Peters/CRC Press.
Pray, C. E. (2001). Public-private sector linkages in Research and Development: biotechnology and the seed industry in Brazil, China and India. Am. J. Agric. Econ. 83, 742–747. doi: 10.1111/0002-9092.00201
Rashedi, E., Mirzaei, A., and Rahmati, M. (2015). An information theoretic approach to hierarchical clustering combination. Neurocomputing 148, 487–497. doi: 10.1016/j.neucom.2014.07.014
Resnick, D., Haggblade, S., Babu, S., Hendriks, S. L., and Mather, D. (2018). The kaleidoscope model of policy change: applications to food security policy in Zambia. World Dev. 109, 101–120. doi: 10.1016/j.worlddev.2018.04.004
Rönkkö, M., McIntosh, C. N., Antonakis, J., and Edwards, J. R. (2016). Partial least squares path modeling: time for some serious second thoughts. J. Oper. Manag. 48, 9–27. doi: 10.1016/j.jom.2016.05.002
Rosset, P. M., and Martínez-Torres, M. E. (2012). Rural social movements and agroecology. Ecol. Soc. 17:317. doi: 10.5751/ES-05000-170317
Sánchez, A. C., Estrada-Carmona, N., Juventia, S. D., and Jones, S. K. (2021). The impact of diversified farming practices on terrestrial biodiversity outcomes and agricultural yield worldwide: a systematic review protocol. Methods Protoc 4, 1–24. doi: 10.3390/mps4010008
Sánchez, B., Rasmussen, A., and Porter, J. R. (2014). Temperatures and the growth and development of maize and rice: a review. Glob. Chang. Biol. 20, 408–417. doi: 10.1111/gcb.12389
Santiago, F. (2014). Innovation for inclusive development. Innov. Dev. 4, 1–4. doi: 10.1080/2157930X.2014.890353
Schiller, K. J. F., Klerkx, L., Poortvliet, P. M., and Godek, W. (2020). Exploring barriers to the agroecological transition in Nicaragua: a technological innovation systems approach. Agroecol. Sustain. Food Syst. 44, 88–132. doi: 10.1080/21683565.2019.1602097
Schnebelin, É., Labarthe, P., and Touzard, J.-M. (2021). How digitalisation interacts with ecologisation? Perspectives from actors of the French agricultural innovation system. J. Rural. Stud. 86, 599–610. doi: 10.1016/j.jrurstud.2021.07.023
Schut, M., Klerkx, L., Rodenburg, J., Kayeke, J., Hinnou, L. C., Raboanarielina, C. M., et al. (2015a). RAAIS: rapid appraisal of agricultural innovation systems (part I). A diagnostic tool for integrated analysis of complex problems and innovation capacity. Agric. Syst. 132, 1–11. doi: 10.1016/j.agsy.2014.08.009
Schut, M., Rodenburg, J., Klerkx, L., Kayeke, J., van Ast, A., and Bastiaans, L. (2015b). RAAIS: rapid appraisal of agricultural innovation systems (part II). Integrated analysis of parasitic weed problems in rice in Tanzania. Agric. Syst. 132, 12–24. doi: 10.1016/j.agsy.2014.09.004
Schut, M., van Paassen, A., Leeuwis, C., and Klerkx, L. (2014). Towards dynamic research configurations: a framework for reflection on the contribution of research to policy and innovation processes. Sci. Public Policy 41, 207–218. doi: 10.1093/scipol/sct048
Seguin, R., Lefsrud, M. G., Delormier, T., and Adamowski, J. (2021). Assessing constraints to agricultural development in circumpolar Canada through an innovation systems lens. Agric. Syst. 194:103268. doi: 10.1016/j.agsy.2021.103268
Sevilla Guzmán, E., and Woodgate, G. (2013). Agroecology: foundations in agrarian social thought and sociological theory. Agroecol. Sustain. Food Syst. 37, 32–44. doi: 10.1080/10440046.2012.695763
Shiri, Z., Frija, A., Rejeb, H., Ouerghemmi, H., and Le, Q. B. (2024). Data on the land cover transition, subsequent landscape degradation, and improvement in semi-arid Rainfed agricultural land in north–West Tunisia. Data 9:96. doi: 10.3390/data9080096
Spielman, D., Ekboir, J., and Davis, K. (2009). The art and science of innovation systems inquiry: applications to sub-Saharan African agriculture. Technol. Soc. 31, 399–405. doi: 10.1016/j.techsoc.2009.10.004
Stephens, E., Jones, A., and Parsons, D. (2017). Agricultural systems research and global food security in the 21st century: an overview and roadmap for future opportunities. Agric. Syst. 163, 1–6. doi: 10.1016/j.agsy.2017.01.011
Tabassum, S., Pereira, F. S. F., Fernandes, S., and Gama, J. (2018). Social network analysis: an overview. WIREs Data Mining Knowl. Discov. 8:e1256. doi: 10.1002/widm.1256
Tittonell, P., Piñeiro, G., Garibaldi, L. A., Dogliotti, S., Olff, H., and Jobbagy, E. G. (2020). Agroecology in large scale farming—a research agenda. Front. Sustain. Food Syst. 4:584605. doi: 10.3389/fsufs.2020.584605
Tomich, T. P., Brodt, S., Ferris, H., Galt, R., Horwath, W. R., Kebreab, E., et al. (2011). Agroecology: a review from a global-change perspective. Annu. Rev. Environ. Resour. 36, 193–222. doi: 10.1146/annurev-environ-012110-121302
Tress, G., Tress, B., and Fry, G. (2005). Clarifying integrative research concepts in landscape ecology. Landsc. Ecol. 20, 479–493. doi: 10.1007/s10980-004-3290-4
Uuemaa, E., Mander, Ü., and Marja, R. (2013). Trends in the use of landscape spatial metrics as landscape indicators: a review. Ecol. Indic. 28, 100–106. doi: 10.1016/j.ecolind.2012.07.018
Van Mierlo, B., Leeuwis, C., Smits, R., and Klein Woolthuis, R. J. A. (2010). Learning towards system innovation: evaluating a systemic instrument. Technol. Forecast. Soc. Change 77, 318–334. doi: 10.1016/j.techfore.2009.08.004
Varblane, U., and Tamm, D. (2012) in The development of the systemic approach to innovation BT - innovation Systems in Small Catching-up Economies: New perspectives on practice and policy. eds. E. G. Carayannis, U. Varblane, and T. Roolaht (New York, NY: Springer New York), 3–19.
Voronov, M., and Weber, K. (2020). People, actors, and the humanizing of institutional theory. J. Manag. Stud. 57, 873–884. doi: 10.1111/joms.12559
Walker, R. M. (2006). Innovation type and diffusion: an empirical analysis of local government. Public Adm. 84, 311–335. doi: 10.1111/j.1467-9299.2006.00004.x
Wang, J., and Wang, X. (2019). Introduction to structural equation modeling. Struct. Equ. Model., 1–32. doi: 10.1002/9781119422730.ch1
Wezel, A., Bellon, S., Doré, T., Francis, C., Vallod, D., and David, C. (2009). Agroecology as a science, a movement and a practice. A review. Agron. Sustain. Dev. 29, 503–515. doi: 10.1051/agro/2009004
Wezel, A., Casagrande, M., Celette, F., Vian, J.-F., Ferrer, A., and Peigné, J. (2014). Agroecological practices for sustainable agriculture. A review. Agron. Sustain. Dev. 34, 1–20. doi: 10.1007/s13593-013-0180-7
Wezel, A., Herren, B. G., Kerr, R. B., Barrios, E., Gonçalves, A. L. R., and Sinclair, F. (2020). Agroecological principles and elements and their implications for transitioning to sustainable food systems. A review. Agron. Sustain. Dev. 40:40. doi: 10.1007/s13593-020-00646-z
Wieczorek, A. J., and Hekkert, M. P. (2012). Systemic instruments for systemic innovation problems: a framework for policy makers and innovation scholars. Sci. Public Policy 39, 74–87. doi: 10.1093/scipol/scr008
Zaremba, H., Elias, M., Rietveld, A., and Bergamini, N. (2021). Toward a feminist agroecology. Sustain. For. 13:244. doi: 10.3390/su132011244
Keywords: agricultural innovation system, RAAIS, agroecology, stakeholders, methods
Citation: Ouerghemmi H, Frija A, Shiri Z and Rejeb H (2024) Methodological guidelines for a rapid appraisal of agricultural innovation systems under agroecology (RAAIS-AE). Front. Sustain. Food Syst. 8:1470442. doi: 10.3389/fsufs.2024.1470442
Received: 25 July 2024; Accepted: 01 November 2024;
Published: 13 November 2024.
Edited by:
Manjeet Singh Nain, Indian Agricultural Research Institute (ICAR), IndiaReviewed by:
Edmore Gasura, University of Zimbabwe, ZimbabweCopyright © 2024 Ouerghemmi, Frija, Shiri and Rejeb. This is an open-access article distributed under the terms of the Creative Commons Attribution License (CC BY). The use, distribution or reproduction in other forums is permitted, provided the original author(s) and the copyright owner(s) are credited and that the original publication in this journal is cited, in accordance with accepted academic practice. No use, distribution or reproduction is permitted which does not comply with these terms.
*Correspondence: Hassen Ouerghemmi, aC5vdWVyZ2hlbW1pQGNnaWFyLm9yZw==
Disclaimer: All claims expressed in this article are solely those of the authors and do not necessarily represent those of their affiliated organizations, or those of the publisher, the editors and the reviewers. Any product that may be evaluated in this article or claim that may be made by its manufacturer is not guaranteed or endorsed by the publisher.
Research integrity at Frontiers
Learn more about the work of our research integrity team to safeguard the quality of each article we publish.