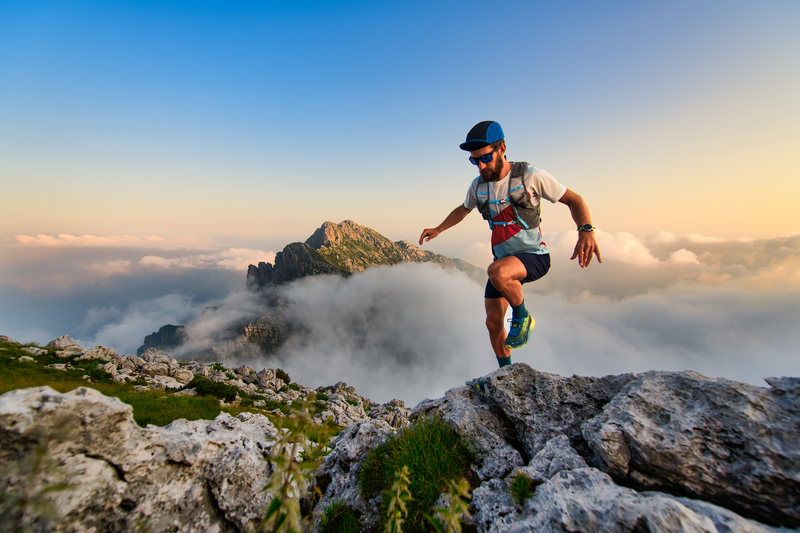
95% of researchers rate our articles as excellent or good
Learn more about the work of our research integrity team to safeguard the quality of each article we publish.
Find out more
ORIGINAL RESEARCH article
Front. Sustain. Food Syst. , 15 October 2024
Sec. Agricultural and Food Economics
Volume 8 - 2024 | https://doi.org/10.3389/fsufs.2024.1467162
Introduction: With the rapid development of digital agriculture, digitalization has gradually become a key factor affecting the resilience of China’s pig farming industry.
Methods: From both the test results and the theoretical point of view, the individual fixed-effect model is more suitable for this paper’s study. Therefore, based on the panel data of 31 provinces in China from 2011 to 2022, this study constructs an individual fixed-effect model to examine how digitization affects the resilience of China’s pig farming industry.
Results and discussion: The findings indicated that digitization significantly enhanced the resilience of China’s hog breeding industry, especially in potential growth areas. Improving breeding technology and scale is a crucial avenue for digitalization to bolster the industry’s resilience. Digitalization exerts a double threshold effect on the industry’s resilience, with its promotional impact only realized within a specific range. The article proposes policy suggestions, such as strengthening infrastructure construction and giving play to regional advantages.
As the saying goes, “Hogs and grain can stabilize the nation.” As a pillar of the agriculture industry, hog farming is essential in China’s rural economic development (Geng et al., 2022; Zhong et al., 2022). However, in recent years, due to the frequent occurrence of diseases, rising costs, and other factors, the supply and demand relationship of China’s hog market has not been stable, and the price of pork is frequently fluctuating, which seriously threatens the stable development of the hog industry. With the rapid diffusion and deep penetration of digital technologies such as 5G, big data, and artificial intelligence, the application degree of digitalization in agriculture and rural areas is increasing, and it has become a new driving force for the agricultural economy growth, transformation, and upgrading (Zhao and Zhao, 2024). It can be seen that the deep integration of digitalization and animal husbandry has become an essential trend of future agricultural innovation and development, and enabling the resilience of the hog breeding industry through digitalization will become an important direction of future farm development (Cao and Wang, 2024). The resilience of the hog breeding industry is directly related to the survival and development of the hog industry. If its resilience level is low, it may be challenging to resist market fluctuations or external shocks (Yang and Deng, 2023). Therefore, in the context of building a modern agricultural power, it is of great practical significance to deeply explore the impact of digitalization on the resilience of the hog breeding industry for ensuring China’s food safety, realizing sustainable agricultural development, and enhancing the comprehensive competitiveness of agriculture.
Holling (1973) first proposed “resilience” in ecology, believing it includes maintaining and repairing after suffering shocks. Later, the concept of resilience was introduced into economics and became an effective tool for scholars to analyze and explain various complex economic phenomena (Fujita and Thisse, 2002). Martin et al. (2015) also have a more comprehensive understanding of economic resilience, emphasizing resistance, adaptability, recoverability, and vulnerability in the face of shocks. At present, the research on economic resilience mainly focuses on resilience evaluation, spatiotemporal evolution, and influencing factors involving regional (Shang and Liu, 2024), urban (Zhang et al., 2023), and industrial levels (Zhang et al., 2024). With the deepening of research, the concept of resilience has gradually been applied to agriculture and has become an essential perspective in studying the stability and sustainability of agricultural systems. Current research shows that the overall development level of China’s agricultural resilience is on the rise, and the enhancement of agricultural resilience is conducive to the long-term stable operation of China’s economic system (Yu et al., 2023). In addition, factors such as digital villages (Zhao and Zhao, 2024), scientific and technological innovation (Wan et al., 2024), food prices (Yang et al., 2022), digital technologies (Chaudhuri and Kendall, 2020; Quan et al., 2024), and digital economy (Ma and Lv, 2024) are key factors affecting agricultural resilience. However, compared with the food system’s resilience, only a few scholars have studied the resilience of the pig breeding industry. For example, Shi and Hu (2023), from the perspective of the impact of African swine fever, measured the resilience of China’s pig industry and its spatial evolution characteristics from two dimensions of resistance and recoverability and used Geodetector to analyze the key factors affecting the resilience of the pig industry.
Digitalization is the application of digital technologies such as the Internet of Things, blockchain, artificial intelligence, and 5G (Li et al., 2018). As a technological innovation, its application in production activities can not only improve the traditional production mode but also help optimize the organizational structure and production system to improve resource allocation efficiency (Gao et al., 2022). Digitization is also a technological form that uses digital infrastructure such as the Internet and cloud platform as a transmission carrier for exchanging data, information, and knowledge and achieves the deep integration of digital technology and production and life on a global scale (Wen and Guo, 2024). At present, the research on the impact of digitalization on the resilience of China’s hog farming industry is mainly carried out from the following three aspects. Firstly, digitalization enables the green development of the hog farming industry. The wide application of information technology such as “Internet +” is conducive to improving the green total factor productivity of large-scale pig farming, thus accelerating the transformation and upgrading of the pig farming industry and promoting the green development of the pig farming industry (Geng et al., 2022). Secondly, digitalization enables the stable development of the hog breeding industry. The digital technology is conducive to expanding the pig breeding scale (Ma and Lv, 2024), while the industrial scale stabilizes pig price fluctuations (Yang et al., 2024), which is conducive to the stable development of the pig breeding industry. Thirdly, digitalization enables high-quality development of the hog breeding industry. The Internet can significantly improve breeding efficiency by adjusting the production mode of animal husbandry, thus promoting the high-quality development of animal husbandry (Chai et al., 2023). Although the above studies implicitly explain the impact of digitalization on the resilience of China’s hog farming industry in theoretical and empirical parts, there is no systematic demonstration.
The existing literature provides an essential reference for this study, but there are some deficiencies in the research perspective, content, and methods. For example, scholars mainly study agricultural resilience from the perspectives of the digital countryside, digital economy, and digital technology, ignoring the role of digitalization in the resilience of the hog farming industry. The direct impact and mechanism of digitalization on the resilience of the hog farming industry need to be further enriched.
The possible contributions of this paper are as follows. First, from a research perspective, it is the first time digitalization has been linked with the resilience of the hog breeding industry. Combined with China’s national conditions, the resilience evaluation system of the hog breeding industry is scientifically and reasonably constructed through the index system method, and the resilience comprehensive index of the hog breeding industry is calculated from three dimensions: resistant capability, recovery capability, and renewal capability. Second, in terms of research content, by combining the theoretical logic of digitalization enabling the resilience of the hog breeding industry and the mediating role played by the level of breeding technology and scale, the influencing mechanism of digitalization on the resilience of the hog breeding industry are revealed, to provide a new entry point for further enhancing the resilience of the hog breeding industry. Third, in terms of research methods, the individual fixed effect model is used to study the direct impact of digitalization on the resilience of the hog breeding industry. The relationship between the two is deeply explored through the mediating effect, threshold effect, and regional heterogeneity to provide empirical evidence for digitalization to boost the resilience of China’s hog breeding industry.
The rest of the study is structured as follows: Section 2 describes the theoretical mechanism and presents the corresponding research hypothesis. Section 3 outlines the research methods, defines the variables, and explains the data sources. Section 4 uses the individual fixed effect model, the intermediary effect model, and the threshold effect model to conduct empirical tests, providing data support for policy implementation. Section 5 summarizes the main conclusions and puts forward relevant suggestions according to China’s national conditions and the actual situation of the development of the pig breeding industry.
Digitalization can promote the organic integration of production factors and emerging technology, improve production and operation efficiency, and enhance the resilience of the hog farming industry. The dynamic capability theory emphasizes that the focus of enterprises should not be limited to how to own resources but, more importantly, how to develop the dynamic capability of integrating, constructing, and reconfiguring internal and external resources to adapt to environmental changes quickly. Driven by digital development, the hog breeding industry will take the initiative to introduce or independently develop new digital equipment adapted to the hog production process or reengineer the traditional breeding methods and production processes to realize the comprehensive technological upgrading of the hog industry. The integration of digitalization and production activities will promote the efficiency of the hog breeding industry through the optimization of factor allocation, the improvement of operation mode, and the improvement of production efficiency (Acemoglu and Restrepo, 2019), and promote the sustainable development of the hog breeding industry by improving animal welfare (Christos et al., 2021; Collins and Smith, 2022), further enhance the resilience of the hog breeding industry. For example, farmers can use extensive data analysis to predict the best breeding time, improve pregnancy rate and reproductive efficiency, and optimize the allocation of breeding hog resources; Real-time monitoring of hog health and breeding environment through sensors and monitoring equipment, timely detection and solution of problems; Automated feeding, cleaning, and waste disposal systems reduce manual labor and increase productivity; Assess and improve animal welfare by monitoring hog activity and social behavior.
Digitization can promote the connection of upstream and downstream of the industrial chain, ensure the efficient operation of business processes, and then enhance the resilience of the hog farming industry. According to the constraint theory, enterprises will be committed to identifying the constraints in the production process and take necessary measures to eliminate their adverse effects in time to improve the output capacity of enterprises and reduce inventory and production costs. During the COVID-19 pandemic, regional control policies and social distancing requirements have seriously threatened the regular operation of the hog farming industry. At this time, the emergence of digitalization provides a new way to solve this unfavorable situation. On the one hand, digital applications provide a new mode of work for the hog farming industry, and online communication can reduce work dependence on physical contact and on-site communication so that the workflow is no longer limited by distance. On the other hand, the “unmanned production line,” “unmanned workshop,” and “unmanned factory” built by digital technology ensure a safe, orderly, and qualified production process of the hog breeding industry and provide technical guarantees for the recovery and reorganization of the hog breeding industry after external shocks. This shows that the application of digitalization in the hog industry not only improves the flexibility of the work of breeding personnel but also promotes the intelligence of the production process, reduces the risk of disease transmission by reducing the direct contact between personnel, ensures the stable operation of the hog industry, and thus improves the resilience of the hog breeding industry in times of crisis (Hu et al., 2022). Digitization can enhance the resilience of the hog breeding industry in the face of market fluctuations and external shocks and provide a foundation for the resilience of the hog breeding industry to resist, recover, and renew. Hence, we propose the first hypothesis:
H1: Digitalization positively impacts the resilience of the hog farming industry.
On the one hand, the deep integration of the hog industry and digital technology can realize digital supervision in the breeding process, help farmers monitor hog health and welfare issues to improve the technical level of hog breeding, and form a management model of precision cultivation (Benjamin and Yik, 2019). The shift from fuzzy to refined management can improve the overall efficiency of hog farming, ensure the quality of pork products, and protect consumers’ health. For example, farmers can use sensors and data analysis technology to automatically adjust feed amounts according to the hog’s weight and growth stage, achieving precise feeding and improving feed conversion; Through digital monitoring equipment, farmers can monitor the environmental temperature, humidity, ventilation, and other conditions in real-time, to ensure the best environment for hog life, reduce the occurrence of diseases, improve breeding efficiency; The use of extensive data analysis and artificial intelligence technology to monitor the health status of each hog, timely detection and isolation of sick hogs, reduce disease transmission, reduce drug residues, and ensure the safety of pork products.
On the other hand, technological change is an essential driving force for upgrading industrial structures. The improvement of breeding technology can optimize the efficiency of resource allocation (Hua et al., 2024) and promote the transformation of the hog industry from traditional breeding to modern and intelligent to enhance the ability of the hog breeding industry to cope with various risks and shocks. For example, through data analysis and predictive models, optimize supply chain management for feed procurement and hog sales, avoid excess or shortage, and then improve resource turnover efficiency; Digital technology can manage the waste disposal of livestock farms, optimize the collection, treatment and reuse of waste through intelligent systems, reduce environmental pollution and improve resource efficiency. In China, many enterprises have actively applied digitalization to pig farming. For example, NetEase Weiyang1 through the organic combination of Internet technology and modern agriculture to enhance the traditional aquaculture industry and improve food safety standards; Alibaba Cloud ET Agricultural Brain2 uses artificial intelligence technology to enhance pig efficiency and pork quality; Jingdong Agriculture’s intelligent farming solution3 is a modern farming system that uses artificial intelligence, the Internet of Things, big data, and other technologies to improve farming efficiency and reduce costs. The improvement of the breeding technology level brought by digitalization has provided unlimited possibilities for transforming the pig breeding industry into a high-tech industry, and digital technology is also constantly stimulating the innovation of traditional industries and the real economy (Wan et al., 2023). Thus, we present the second hypothesis:
H2: Digitization enhances the resilience of the hog farming industry by improving breeding technology level.
Based on the theory of production process management, enterprises can effectively reduce breeding costs by managing and controlling the production process to avoid unnecessary losses and waste. Through digital means, hog farms can accurately manage the feeding process of each hog and improve its economic output. In addition, automation technology can reduce human labor input in repetitive physical labor, provide technical guarantees for cost reduction, and increase the potential of large-scale hog breeding. For example, farmers can not only introduce automation and intelligent equipment, such as automatic feeding systems and intelligent monitoring systems, reduce human input, and promote the efficiency of hog production but also realize the complete traceability of hog breeding products through digital technology, improve product transparency, and enhance consumer trust. The wide application of digitalization in the whole industrial chain, such as hog breeding, product promotion, and pork consumption, has further promoted the expansion of the scale of hog breeding (Zhou K. et al., 2023).
However, because the asset locking effect will increase the opportunity cost of enterprises to adjust the production mode (Foster and Rosenzweig, 2022), and large-scale investment quickly makes enterprises over-dependent on specific technology or production equipment, enterprises will be more inclined to maintain a stable production mode in the future development process (Richter, 2013). The Walson Law also holds that large-scale management’s information advantages may help farmers survive in the market for a long time and maintain production stability. Due to the low average production cost of scale operators, it is easy to play the advantages of technology, capital, standardized management, etc., in the face of market fluctuations and uncertainties, measures can be quickly taken to respond to reduce the possibility of passive exit from the market (Alvarez and Arias, 2003; Sumner, 2014), and play the role of “ballast stone” and “stabilizer” in the stable production of the hog industry. Therefore, digital development is conducive to realizing large-scale farming in the hog industry, thus enhancing its resilience. Consequently, we raise the third hypothesis:
H3: Digitization enhances the resilience of the hog farming industry by improving scale level.
The specific theoretical research framework is shown in Figure 1.
To investigate the impact of digitization level on the resilience of the hog farming industry, the following baseline regression model was constructed:
Where indicates the resilience of the hog farming industry; represents digitization level; and are province and year; refer to a series of control variables, including feed production capacity (), fiscal support for agriculture (), regional industrial structure (), residents’ consumption level () and logistics development level ; is individual fixation effect; is random disturbance term.
To further analyze the mechanism of digital level affecting the resilience of the hog breeding industry, that is, whether there is an intermediary effect of breeding technology level and scale level, this paper refers to the research method of Wen and Ye (2014) and builds the following regression model of mediating effect:
Where represents the breeding technology level or scale level, Equation 1–3 is the concrete step of the mediating effect test. Formula 1 examines the direct impact of digitalization on the resilience of the hog breeding industry; Formula 2 analyzes the impact of digitalization on the breeding technology level and scale level, respectively; Formula 3 incorporates digitalization level, breeding technology level, and scale level into the model, respectively. To examine its relationship with the resilience of the hog breeding industry. If is not significant, there is no intermediary effect. Under the premise that is significant, if is not significant and smaller than , it is an entirely mediating effect. If is significant and smaller than , it is partially mediated.
The resilience of the hog breeding industry. At present, the academic community has not formed a widely recognized standard framework or index system to comprehensively evaluate and quantify the resilience of the hog farming industry. Therefore, referring to the resilience evaluation system constructed by the existing research, the resilience evaluation system of the hog breeding industry is built from three aspects: resistant capability, recovery capability, and renewal capability with comprehensive indicators. The specific indicators are shown in Table 1. Among them, resistant capability refers to the ability to reduce the impact of the hog breeding industry in the face of emergencies such as epidemics and natural disasters; Recovery capability describes the ability of the hog farming industry to recover to its initial state after an adverse shock; Renewal capability refers to the hog breeding industry after the impact of self-adjustment to enhance the ability to resist risks in the future. The entropy method was used to analyze the weight of multiple indicators measuring the resilience of the hog breeding industry, and the final resilience index of the hog breeding industry was calculated.
Taking 2011 and 2022 as examples, this paper uses ArcGIS10.8 to draw the spatial distribution map of the resilience of the hog breeding industry in 31 provinces of China to study the temporal and spatial evolution characteristics of resilience in the pig breeding industry more intuitively. The results are shown in Figure 2. By comparing the two, it can be found that the resilience of China’s hog breeding industry is on the rise as a whole, and the provinces with solid resilience of the hog breeding industry are mainly concentrated in major grain-producing areas. The possible explanation is that provinces in major grain-producing regions can quickly and efficiently provide the feed resources required by the hog breeding industry to ensure a stable supply of material resources. Hence, the hog breeding industry in major grain-producing areas is more resilient than in non-major grain-producing regions.
Digitization level. Based on the studies of Gao et al. (2022), Ma and Lv (2024), and Zhao et al. (2020), this paper comprehensively measures the digitalization level of various provinces in China from the two aspects of the development of Internet and digital finance. Among them, the level of Internet development is measured from four aspects: mobile phone penetration rate, relevant employees, relevant output, and Internet penetration rate. The corresponding contents are as follows: the number of mobile phone users per 100 people, information transmission, software, and information technology service industry employees in urban units, the proportion of postal and telecommunications services in GDP, and the number of Internet broadband access users per 100. The development level of digital finance is represented by the digital financial inclusion index compiled by the Institute of Digital Finance Peking University. The above indexes were standardized and subjected to dimensionality reduction through the principal component analysis method, resulting in the final digital level index.
By comparing the spatial distribution map of the digitalization level in 2011 and 2022, it can be found that the digitalization level of various provinces in China shows an apparent upward trend. The digitalization level of the central and western regions has a faster growth rate, and the gap between them and the eastern areas has gradually narrowed, as shown in Figure 3. The specific reason may be that the central and western regions lag behind the east region in the level of digital development due to the relatively weak digital infrastructure and the relatively backward level of digital technology application and innovation. To promote coordinated development among regions, China has transformed its innate beauty advantages into development momentum through the “East data, west computing” project, created a digital development cooperation model of “Western computing network hub + Eastern computing resource demand,” explored and implemented ways to introduce high-level digital talents such as “open bidding for selecting the best candidates,” and accelerated the development of digital level in the central and western regions.
Breeding technology level and scale level. The hog output rate is a standard index reflecting the level of provincial breeding technology, which is measured by the ratio of hog output in t year and hog inventory at the end of t-1 year (Zhang et al., 2019). According to the China Pig Production Development Plan (2016-2020), scale level is measured by the proportion of farms with 500 or more to the total number of farms. According to the theoretical analysis above, the development of the digital level helps to realize digital supervision in the process of hog breeding, improve the technical level of hog breeding by enhancing the efficiency of resource allocation and forming a fine management mode, and then promote the transformation and upgrading of the hog industry and improve the resilience of the hog breeding industry. The wide application of digital technology can reduce the labor input in the hog breeding process and stimulate hog farmers to expand the production scale. At the same time, the locking effect brought by large-scale management can stabilize hog production, conducive to the sustainable development of the hog breeding industry, and enhance its resilience.
It is measured as the ratio of corn production to total grain production for the same period. As the most crucial feed source in the hog breeding process, corn reflects the advantages and disadvantages of a province’s resource endowment (Huang et al., 2021). The better the resource endowment conditions are, the lower the cost of hog breeding, the more beneficial it is to the development of the hog industry, and the stronger the resilience of the hog breeding industry.
The proportion of expenditures on agriculture, forestry, and water affairs in the general budget measures it. The greater the financial support to agriculture, the more conducive it is to technological innovation in the region, realizing the transformation of agricultural technology achievements (Wan et al., 2024) and then promoting the improvement of the resilience level of the pig breeding industry.
It is measured by the proportion of the added value of animal husbandry to the gross regional product. The higher proportion indicates that the development level of animal husbandry in the region is better. The government is more willing to introduce various policies and measures to support and protect the development of animal husbandry to promote the transformation and upgrading of industrial structure (Jin et al., 2023) and enhance the resilience of the hog farming industry.
It is measured by urban and rural residents’ per capita pork consumption. Market demand is an essential driving force that attracts industrial transfer. Driven by interest, farmers are more willing to transfer to areas with more significant potential for pork consumption (Zhang et al., 2019). Therefore, residents’ consumption level may have a particular guiding role in developing the hog breeding industry, impacting its resilience.
It is measured by road freight volume. The development of the hog breeding industry has the characteristics of path dependence. The opening of expressways will significantly reduce transportation and time costs (Li et al., 2019), which will help obtain inputs, expand the hog sales market, improve circulation efficiency, and thus enhance the resilience of the hog breeding industry.
This paper selects the provincial panel data of 31 provinces in China (except Hong Kong, Macao, and Taiwan) from 2011 to 2022 for research. The relevant data mainly come from the China Statistical Yearbook and China Rural Statistical Yearbook, the National Bureau of Statistics of China, the EPS database, the Brick Agricultural Products Database, and the Institute of Digital Finance Peking University. Referring to the practice of Shi and Hu (2023), the missing data on the number of workers employed in large, medium, and small farms are filled with the average data of China, while the missing data in other provinces are filled with the interpolation method. The descriptive statistics of each variable are shown in Table 2.
From the test results, the F-test results show that the p-value is 0.0000, which is less than 0.01. The original hypothesis of “no significant differences between individuals” is rejected, and there are believed to be significant differences between individuals. However, since the individual fixed effects may also exist in the form of random effects, after the Hausmann test, it is found that the p-value is 0.0040. The original hypothesis that “random effects are superior to fixed effects” is rejected at 1%, indicating that the individual fixed effects model is better. Theoretically speaking, based on the practice of Guo et al. (2016), considering that the samples are short panel data, there are pronounced regional differences among provinces, and the results of the individual fixed effect model are closer to the actual situation, this study chooses the individual fixed effect model for empirical research.
The multicollinearity test of all the variables in the regression shows that the vif value is less than 5, and there is no multicollinearity between the variables. According to the law of large numbers and the central limit theorem, when the sample size is more than 100, the large sample theory no longer restricts the perturbation term to obey the normal distribution (Chen, 2015). The sample size of this paper is 372, which is greater than 100. Therefore, only “cross-sectional correlation, heteroscedasticity and autocorrelation problems,” which are prone to occur in short panel data, are tested. It is found that there are no autocorrelation problems in this paper, but cross-sectional correlation and heteroscedasticity problems. Therefore, to improve the accuracy of the results, this paper selects the individual fixed effect model with cluster robust standard error for subsequent research.
Because the F test is ineffective when clustering robust standard error is used, this paper further investigates it using the LSDV method. The test results show that most individual dummy variables are significant at the 5% level, rejecting the null hypothesis that “all individual dummy variables are 0” and an individual fixed effect exists. Similarly, since the traditional Hausmann test is not applicable in the case of heteroscedasticity, this paper uses the overidentification test to determine which model to adopt. The results show that the p-value is 0.0005, which firmly rejects the null hypothesis of random effects, so the individual fixed effects model is adopted in this paper.
As seen from Table 3, in columns (3) and (4), no matter whether control variables are included, the influence coefficients of digitalization on the resilience of the pig breeding industry are all positive and have passed the significance test. Before the introduction of control variables, the coefficient of digitization is 0.0099, which is significant at the 1% level. After the inclusion of the control variables, the coefficient of digitization increases to 0.0109, which, despite a decrease in significance, still confirms the promotion effect at the 5% level. In column (4), the regional industrial structure and household consumption level pass the significance test at 5 and 1%, respectively. The coefficients are all positive, indicating that the higher the proportion of regional industrial structure, the better the development level of animal husbandry in the region, and the more conducive to promoting the resilience of the pig breeding industry. At the same time, areas with higher pork consumption levels can enhance the resilience of the pig breeding industry. Accordingly, hypothesis H1 is verified.
To ensure the reliability of the empirical results, the robustness test is carried out using the instrumental variable method, explanatory variable lag one stage, sample elimination, and variable replacement.
To alleviate the endogenous problem as much as possible, the number of landline telephones per 100 people in 1984 was selected as the instrumental variable and analyzed by the two-stage least square method. The year 1984 is far away from the starting year of the study and has no substantial influence on the samples. Moreover, the number of fixed telephones in each region can reflect the construction of local telecommunications infrastructure and has a specific correlation with the development of regional digitalization level. Drawing on the processing method of Hou et al. (2023), the interaction term between the number of Internet access ports in the previous year and the number of fixed telephones per 100 people in 1984 is introduced as the instrumental variable of the current digitalization level, expressed in IV. The analysis results of 2SLS are shown in Table 4. According to Kleibergen-Paaprk’s LM statistics and Wald F statistics, there is no problem of weak instrumental variables or insufficient or over-recognition of instrumental variables in this paper, and the digitization level still has a significant positive impact on the resilience of the pig breeding industry, indicating that the conclusions of the previous research are still valid.
Considering the long construction time of digital infrastructure, there may be a certain lag in the impact on the resilience of the pig farming industry. Therefore, this paper uses a new variable, L.lnDig, produced by a one-stage lag in digitization level, as a new explanatory variable for regression. The results are shown in Table 4. The digitization level has a significant positive impact on the resilience of the pig breeding industry at the level of 10%, indicating that the promotion effect of the digitization level does have a delayed effect.
Drawing on the practice of Wan et al. (2024), considering the severe impact of COVID-19 on various industries and economies worldwide in 2020, this paper conducted a regression analysis after excluding sample data from 2020 to 2022. The results in Table 4 show that digitization significantly positively affects the resilience of the pig farming industry at the 5% level, with a regression coefficient of 0.0125, which is consistent with the benchmark regression results.
Referring to the practice of Liu et al. (2021), the Internet penetration rate was incorporated into the model as an indicator to measure the level of digitalization, and the regression analysis was carried out, as shown in Table 4. The influence coefficient of the explanatory variable is 0.0286, which passes the significance test at the 1% level, indicating that the digitization level can still significantly improve the resilience of the hog breeding industry and verifies the authenticity of the baseline regression results.
The above four different robustness test results consistently show that the digitization level can significantly positively affect the resilience of the hog breeding industry. This confirms the strong robustness of the benchmark regression results in this paper, enhances the credibility of the research conclusion, and verifies hypothesis H1 again.
China has a vast geographical area, and different regions have apparent differences in natural resources, ecological environment, and economic development. According to the regional layout of hog production in provinces in China Hog Production Development Plan 2016–2020, the sample data were divided into four parts: key development area, constrained development area, potential growth area, and moderate development area, which were represented by dis_1, dis_2, dis_3, and dis_4, respectively. In addition, due to the small observations after segmentation by region, it is easy to have the problem of significant deviation of estimates, which makes it difficult to show substantial differences in the results after grouping regression. Referring to the research methods of existing literature (Tang and Yang, 2022), this paper further analyzed the heterogeneous impact of digitization level on the resilience of the hog breeding industry with the help of the interaction term coefficient of core explanatory variables and regional category dummy variables.
According to the heterogeneity analysis results in Table 5, the coefficient of digitization level in key development areas is positive and significant. In contrast, the coefficient of the interaction term is negative and significant, indicating that digitization in this area does not play a role in promoting the resilience of the hog breeding industry. The reason may be that provinces in crucial development areas have a unique strategic position in the hog breeding industry, and most are advantageous in hog breeding (Zhang et al., 2019). These provinces have advanced infrastructure and professional aquaculture technicians. At the same time, their scale and intensive degree are high; in terms of production management, farmers will pay more attention to innovation and technology by trying and adopting new technology to improve production efficiency and industrial competitiveness, resulting in the region’s digitalization promotion role reaching saturation. Compared with other areas, its elevating effect was not noticeable and even showed an inhibitory effect.
The coefficient of the interaction term in the potential growth area passed the significance test at the 5% level, indicating that the digitization of the area can significantly promote the improvement of the resilience of the hog breeding industry. Potential growth area usually refers to some areas because of rich feed resources, low labor costs, environmental carrying capacity, and other innate advantages that become the areas of hog breeding. Provinces located in potential growth areas are often more likely to receive policy and financial incentives from the Chinese government, which will promote the adoption and application of digital technologies. Moreover, these provinces are primarily located in major grain-producing areas, which provide convenience for feed supply and lower breeding costs, so they are more conducive to promoting digital levels. However, the coefficient of the interaction term of the constrained and moderate development area failed the significance test, and only the coefficient of the explanatory variable was significantly positive, indicating that the digitization level had no significant role in promoting the resilience of the hog breeding industry in these areas.
To explore whether digitalization can enhance the resilience of the pig breeding industry by improving the level of breeding technology and scale, this paper uses the intermediary effect model to analyze its mechanism of action, and the regression results are shown in Table 6. In column (1), the regression coefficient of the digitalization level is 0.0109, which is significant at the 5% level, indicating that the digitalization level can significantly positively affect the resilience of the pig breeding industry. In columns (2) and (4), the influence coefficients of digitalization on the breeding technology level and scale level are 0.0895 and 0.8399, respectively, which pass the significance test, indicating that digitalization can significantly promote the improvement of the breeding technology level and scale level. In columns (3) and (5), the regression coefficients of breeding technology level and scale level on the resilience of the pig breeding industry are still significantly positive. In contrast, the coefficients of digitization level are insignificant and smaller than those in column (1), indicating that breeding technology level and scale level play a complete mediating effect. Accordingly, hypothesis 2 and hypothesis 3 are tested.
The benchmark regression results show that the digitization level can significantly improve the resilience of the hog breeding industry. So, does it mean that as long as the Chinese government promotes digital construction, it will undoubtedly enhance the resilience of the hog farming industry? In other words, is there a tipping point or turning point in the effect of digitalization on the resilience of the hog farming industry? For example, once the level of digitization passes a critical point, the positive impact on the resilience of the hog farming industry will diminish or reach saturation. This issue is of great significance for the Chinese government to take targeted measures to effectively promote the application of digitalization in the hog farming industry. Therefore, to further study whether the digitization level has a threshold effect on the resilience of the hog breeding industry, this paper introduces the panel threshold model proposed by Hansen to test the nonlinear relationship between the digitization level and the resilience of the hog breeding industry. The specific formula is as follows:
In Formula (4), indicates the threshold variable, represents the indicator function, is the threshold estimate, and refers to the error term.
To verify the existence of the threshold effect, the Bootstrap method is used to sample 300 times and repeatedly obtain threshold test results. As can be seen from Table 7, under the triple threshold, the p-value fails the significance test. However, the p-value passed the significance test at 1% under the double and single threshold. Therefore, this paper uses a double threshold for analysis, and the threshold values are 0.8423 and 1.0076, respectively, indicating a threshold effect on the influence of digitalization level on the resilience of the hog breeding industry.
The model regression results under the double threshold effect are shown in Table 8. Specifically, the level of digitalization has a nonlinear impact on the resilience of the hog breeding industry. Only when 0.8423 < lnDig≤1.0076 did the positive effects of digitization level on the resilience of the pig breeding industry pass the significance test, and the regression coefficient was 0.0303. This shows that the level of digitalization can only effectively promote improving the resilience of the pig breeding industry within a specific range. When digitalization is low, it is weak for the pig breeding industry. With the continuous deepening of digital development, it will have a more prominent role in promoting the resilience of the pig breeding industry. However, when the digitization level has been elevated to a particular stage, and the digital development of the pig breeding industry has been relatively perfect, the positive effect will become less noticeable.
This paper takes 31 provinces in China (except Hong Kong, Macao, and Taiwan) as the research object. It uses principal component analysis and entropy methods to measure the digitization level and the resilience of the pig breeding industry in each province from 2011 to 2022. Based on theoretical analysis, the impact of digitization on the resilience of the pig breeding industry is studied through the individual fixed effect model. The following conclusions are drawn after robustness tests, heterogeneity analysis, mediation effect test, and threshold effect test. Firstly, the level of digitalization can significantly promote the resilience of the hog breeding industry, and this conclusion is still robust after a series of tests and eliminating the endogeneity problem. Secondly, the digitalization level of potential growth areas can significantly enhance the resilience of the hog breeding industry. The digitalization level of crucial development areas has an inhibitory effect on the resilience of the hog breeding industry. In contrast, the impact of the digitalization level of moderate and constrained development areas on the resilience of the hog breeding industry fails the significance test. Thirdly, the digital level enhances the resilience of the hog breeding industry by promoting the improvement of breeding technology level and scale level. The breeding technology level and the scale level play a full intermediary role. Fourthly, the results of the threshold effect test showed that the digitization level had a double threshold effect on the resilience of the pig breeding industry, with threshold values of 0.8423 and 1.0076, respectively, and the resilience of the pig breeding industry could be significantly improved when the digitization level was maintained in the range of 0.8423 to 1.0076.
This paper puts forward the following policy recommendations based on the above research conclusions.
The first is to improve the construction of digital infrastructure and accelerate the promotion of the digital level. First of all, the pig farming industry should pay attention to strengthening the construction of pig farm network facilities, ensure high-speed and stable data transmission, provide essential support for the operation of the digital management system, and increase research and development investment as much as possible, develop intelligent equipment and software systems suitable for pig farming, such as intelligent feeding, environmental monitoring, disease early warning system, etc., to promote the digital transformation of the pig farming industry. Help the pig breeding industry to improve resilience. Secondly, the government should give positive policy guidance and necessary financial support, encourage enterprises to apply intelligent farming equipment, guide upstream and downstream enterprises in the pig farming industry chain to pay attention to the necessity of infrastructure development and strive to form a development environment for the digital transformation of the pig farming industry. For example, the China Pig Big Data Center, jointly promoted by China Animal Husbandry Station and Chongqing Rongchang District People’s Government, realizes the information monitoring of the whole pig industry chain through the use of digital technologies such as the Internet of Things, big data and blockchain, which is an important measure to promote the digital transformation of the pig industry, reflecting the importance and support of the Chinese government to the pig farming industry. Finally, digital skills education and training should be carried out to improve the digital literacy of breeding subjects, strengthen talent cultivation and introduction, attract high-end talents and innovative teams in the digital field, and enhance the suitability of human capital and digital technology. For example, Wens Foodstuff Group Co., Ltd., a well-known breeding company in China, has provided its employees with specialized digital skills training and more than 300 courses and established an online training platform and training base. Regardless of the level and position, a graded, classified, hierarchical training management system must be provided. These measures not only enhance the digital literacy of employees but also offer strong talent support for the high-quality development of enterprises.
The second is to combine their development positioning and give full play to the advantages of regional resources. Careful consideration of resource endowment, consumption potential, and other conditions while ensuring the quality and safety of pork products and forming a diversified hog farming model to improve the overall resilience level. As the core area of the national pig industry, key development areas should speed up the digital transformation and upgrading of the industry, introduce and promote advanced breeding technology and management mode, improve production efficiency and product quality, and promote the development of the pig industry to modernization, standardization, and scale. At the same time, pay attention to optimizing the organizational structure and production system, stabilize the total output and transfer of pork, and strive to become an essential support for China’s pig breeding industry. Potential growth areas should effectively utilize their advantages, such as feed resources and environmental capacity, and continuously expand production scale to achieve increased production and efficiency while realizing the combination of planting and breeding. Pay attention to cultivating and promoting excellent breeding pigs and improve the pork cold chain logistics system. It is also necessary to further enhance digitalization, improve breeding efficiency, and drive the development of the pig breeding industry. Although the moderate development area is vast and rich in land resources, the pig breeding foundation is weak. It can encourage and support large enterprise groups to invest in the construction of pig breeding bases, promote farmers to develop moderate-scale breeding, introduce and popularize advanced pig breeding technology, promote ecological breeding, and highlight regional characteristics. Resources and environmental conditions restrict restricted development areas, and the development space of pig production is limited. It can be committed to stabilizing the total amount of breeding, rationally planning the breeding area, improving the quality of pig varieties by strengthening the construction of the breeding system, and implementing the comprehensive utilization mode of manure such as the integration of breeding and breeding, to achieve the sustainable development of the pig breeding industry.
The third is to continuously optimize hog breeding technology and encourage the development of large-scale hog breeding. With the help of modern scientific and technological means, it accurately analyzes the data information in the process of hog breeding, promotes the development of fine management mode, improves the overall efficiency of the hog industry, and forms a win-win situation of economic, social and environmental protection in the farming industry. At the same time, it should also increase the application of digital technology, promote the close cooperation and integration of the upstream and downstream of the industrial chain, accelerate the response speed of the hog industry to market changes, and enhance the resilience level of the hog breeding industry. For example, the pig intelligent breeding system developed by Beijing Xiaolong Stealth Technology Co., Ltd. uses advanced technologies such as deep learning and machine vision to achieve real-time accurate collection and analysis of production data. This not only improves the breeding efficiency and management level but also provides high-quality data services for upstream and downstream enterprises in the pig breeding industry chain, thereby promoting the sustainable development of the pig breeding industry and improving the efficiency and response speed of the entire industry chain. The system has been demonstrated in many pig farms and has achieved good application results. In addition, large-scale farming can not only increase the economic benefits of the hog industry, achieve cost-effectiveness, and optimize the allocation of resources but also better cope with market fluctuations and risk impacts after forming a stable large-scale operation system, reduce the uncertainty faced by the farming industry, thus promoting the improvement of the resilience of the hog farming industry. Large-scale farming plays an increasingly important role in modern animal husbandry, but large-scale agriculture also faces challenges, such as environmental pollution and animal disease prevention and control. Therefore, while developing large-scale farming, it is also necessary to pay attention to sustainable development and ecological protection to ensure the long-term healthy development of the pig farming industry.
However, this paper also has some limitations. First, more kinds of mechanisms, such as regulatory effects, can be developed to illustrate the role of digitization in improving the resilience of the hog farming industry from different perspectives, and other models and methods can be used to study the relationship between the two. Second, this paper selects the measurement indicators of digitization level and resilience of the hog breeding industry by studying relevant excellent literature. However, given the availability of sample data, the final selected indicators may still be insufficient. In the end, the research data in this paper mainly comes from China’s statistical yearbook and database, and the research objects are 31 provinces in China, so the research conclusions and suggestions may only apply to China and other countries with similar national conditions.
The raw data supporting the conclusions of this article will be made available by the authors, without undue reservation.
JH: Conceptualization, Formal analysis, Writing – original draft, Writing – review & editing, Methodology. CM: Data curation, Software, Writing – original draft. WL: Methodology, Software, Writing – review & editing. ZH: Conceptualization, Formal analysis, Writing – review & editing. PZ: Methodology, Validation, Writing – review & editing.
The author(s) declare that financial support was received for the research, authorship, and/or publication of this article. This research was funded by Project of the National Natural Science Foundation of China in 2023: Research on Uncertainty Shock, Farmer Behavior Response and Risk Formation Mechanism of Pig Price Fluctuation (72373036); 2023 Ministry of Education Humanities and Social Science Planning project “Mechanism and Path Research on Digital Transformation Driving the Resilience of Live Pig Industry Chain” (23YJA790029).
Thanks to all the students and teachers who helped with this article and the reviewers for their constructive comments.
The authors declare that the research was conducted in the absence of any commercial or financial relationships that could be construed as a potential conflict of interest.
All claims expressed in this article are solely those of the authors and do not necessarily represent those of their affiliated organizations, or those of the publisher, the editors and the reviewers. Any product that may be evaluated in this article, or claim that may be made by its manufacturer, is not guaranteed or endorsed by the publisher.
Acemoglu, D., and Restrepo, P. (2019). Automation and new tasks: how technology displaces and reinstates labor. J. Econ. Perspect. 33, 3–30. doi: 10.1257/jep.33.2.3
Alvarez, A., and Arias, C. (2003). Diseconomies of size with fixed managerial ability. Am. J. Agric. Econ. 85, 134–142. doi: 10.1111/1467-8276.00108
Benjamin, M., and Yik, S. (2019). Precision livestock farming in swine welfare: a review for swine practitioners. Animals 9:133. doi: 10.3390/ani9040133
Cao, L., and Wang, G. S. (2024). Impact of digital finance on agricultural output: from the perspective of digital development of agriculture. Financ. Res. Lett. 66:105698. doi: 10.1016/j.frl.2024.105698
Chai, Z. H., Tian, M. J., and Yao, F. T. (2023). The impact of internet use on production efficiency of animal husbandry: based on the evidence of 340 herdsmen in Inner Mongolia, China. Sustain. For. 15:7955. doi: 10.3390/SU15107955
Chaudhuri, B., and Kendall, L. (2020). Collaboration without consensus: building resilience in sustainable agriculture through ICTs. Inf. Soc. 37, 1–19. doi: 10.1080/01972243.2020.1844828
Chen, X. H., An, Y. Y., Pan, W., Wang, Y., Chen, L. T., Gu, Y., et al. (2024). Dynamic transfer and driving mechanisms of the coupling and coordination of agricultural resilience and rural land use efficiency in China. J. Geog. Sci. 34, 1589–1614. doi: 10.1007/S11442-024-2262-7
Christos, T., Panagiotis, S., Konstantinos, A., and Panagiotis, P. (2021). An overview of the current trends in precision pig farming technologies. Livest. Sci. 249:104530. doi: 10.1016/j.livsci.2021.104530
Collins, L. M., and Smith, L. M. (2022). Review: smart Agri-systems for the pig industry. Animal 16:100518. doi: 10.1016/j.animal.2022.100518
Foster, A. D., and Rosenzweig, M. R. (2022). Are there too many farms in the world? Labor market transaction costs, machine capacities, and optimal farm size. J. Polit. Econ. 130, 636–680. doi: 10.1086/717890
Fujita, M., and Thisse, J. F. (2002). Economics of agglomeration cities, industrial location, and regional growth. Cambridge: Cambridge University Press.
Gao, D., Li, G., and Yu, J. Y. (2022). Does digitization improve green total factor energy efficiency? Evidence from Chinese 213 cities. Energy 247:123395. doi: 10.1016/j.energy.2022.123395
Geng, N., Liu, Z. J., Wang, X. J., Meng, L., and Pan, J. Y. (2022). Measurement of green Total factor productivity and its spatial convergence test on the pig-breeding industry in China. Sustain. For. 14, –13902. doi: 10.3390/su142113902
Guo, G. C., Ding, C. X., and Wang, Y. R. (2016). The impact of new-type urbanization on industrial land use efficiency: a theoretical framework and empirical test. China Land Sci. 30, 81–89. doi: 10.11994/zgtdkx.20160921.144532
Hao, A. M., and Tan, J. Y. (2022). Impact of digital rural construction on food system resilience. J. S. China Agric. Univ 21, 10–24. doi: 10.7671/j.issn.1672-0202.2022.03.002
Holling, C. S. (1973). Resilience and stability of ecological systems. Annu. Rev. Ecol. Syst. 4, 1–23. doi: 10.1146/annurev.es.04.110173.000245
Hou, S. F., Zhang, W., and Zhu, D. G. (2023). Digital economy, tax enforcement and Enterprise tax behavior. J. Univ. Jinan 33:87-101+177. doi: 10.20004/j.cnki.ujn.2023.06.009
Hu, H. F., Song, X. X., and Dou, B. (2022). The value of digitalization in times of crisis: evidence of corporate resilience. Finance Trade Econ. 43, 134–148. doi: 10.19795/j.cnki.cn11-1166/f.20220706.008
Hua, J. G., Yu, J. J., Song, Y., Xue, Q., and Zhou, Y. J. (2024). The enabling effect of digital economy on high-quality agricultural development-evidence from China. Sustain. For. 16:3859. doi: 10.3390/su16093859
Huang, B. K., Geng, X. H., and Hu, H. (2021). Was the change of farm scale structure caused by hog industrial policy in China? An empirical analysis based on Markov chain. China Rural Surv. 4, 123–144.
Jin, G. C., Wang, G. L., and He, Y. R. (2023). Digitization level, industrial structure adjustment and regional carbon emission reduction. Stat. Decis. 39, 27–32. doi: 10.13546/j.cnki.tjyjc.2023.03.005
Li, L., Su, F., Zhang, W., and Mao, J. Y. (2018). Digital transformation by SME entrepreneurs: a capability perspective. Inf. Syst. J. 28, 1129–1157. doi: 10.1111/isj.12153
Li, L. B., Yan, L., and Huang, J. L. (2019). Transportation infrastructure connectivity and manufacturing Industries in Peripheral Cities in China: markup, productivity and allocation efficiency. Econ. Res. J. 54, 182–197.
Liu, X. L., Zhang, W. Y., Ge, S., and Yang, P. P. (2021). Does digitization help narrow the development gap between cities? Based on empirical research in 283 cities. Sci. Sci. Manag. 42, 102–113.
Ma, X. X., and Lv, L. Z. (2024). Facilitating the high-quality agricultural development with digital economy: a panel data study of Jiangsu Province from 2013 to 2021. Front. Sustain. Food Sci. 8:1424097. doi: 10.3389/FSUFS.2024.1424097
Martin, R., Sunley, P., and Tyler, P. (2015). Local growth evolutions: recession, resilience and recovery. Cambridge J. Reg. Econ. Soc. 8, 141–148. doi: 10.1093/cjres/rsv012
Quan, T. S., Zhang, H., Quan, T. L., and Yu, Y. (2024). Unveiling the impact and mechanism of digital technology on agricultural economic resilience. CJPRE 22, 136–145. doi: 10.1016/J.CJPRE.2024.06.004
Richter, M. (2013). Business model innovation for sustainable energy: German utilities and renewable energy. Energy Policy 62, 1226–1237. doi: 10.1016/j.enpol.2013.05.038
Shang, X. Y., and Liu, Q. (2024). Digital inclusive finance and regional economic resilience: evidence from China's 283 cities over 2011-2021. Financ. Res. Lett. 69:105953. doi: 10.1016/J.FRL.2024.105953
Shi, Z. Z., and Hu, X. D. (2023). African swine fever shock: China’s hog Industry’s resilience and its influencing factors. Animals 13:2817. doi: 10.3390/ani13182817
Sumner, D. A. (2014). American farms keep growing: size, productivity, and policy. J. Econ. Perspect. 28, 147–166. doi: 10.1257/jep.28.1.147
Tang, K. J., and Yang, G. Y. (2022). Does digital infrastructure cut carbon emissions in Chinese cities? Sustain. Prod. Consum. 35, 431–443. doi: 10.1016/j.spc.2022.11.022
Wan, Q., Chao, R. R., Li, J. S., and Khan, N. (2024). Toward a sustainable agricultural system in China: exploring the nexus between agricultural science and technology innovation, agricultural resilience and fiscal policies supporting agriculture. Front. Sustain. Food Sci. 8:1390014. doi: 10.3389/fsufs.2024.1390014
Wan, Q. C., Tang, S. Y., and Jiang, Z. W. (2023). Does the development of digital technology contribute to the innovation performance of China's high-tech industry? Technovation 124:102738. doi: 10.1016/J.TECHNOVATION.2023.102738
Wang, Y. F., Ye, J., and Cao, J. (2023). Study on the mechanism and effect of digital finance improving the resilience of grain system. Econ. Surv. 40, 48–60. doi: 10.15931/j.cnki.1006-1096.2023.05.003
Wen, L. D., and Guo, S. F. (2024). The reshaping effect of digitalization on regional innovation geography: a study based on the empirical data of cities in China. Sci. Technol. Prog. Policy 41, 46–56. doi: 10.6049/kjjbydc.Q202206024
Wen, Z., and Ye, B. (2014). Analyses of mediating effects: the development of methods and models. Adv. Psychol. Sci. 22:731. doi: 10.3724/sp.j.1042.2014.00731
Yang, G. G., and Deng, F. (2023). Can digitalization improve enterprise sustainability?–evidence from the resilience perspective of Chinese firms. Heliyon 9:e14607. doi: 10.1016/j.heliyon.2023.e14607
Yang, Q., Qiao, S. Y., and Ying, R. Y. (2024). Agricultural industrial scale, price random fluctuation, and profitability levels: evidence from China’s pig industry. Front. Sustain. Food Sci. 8:1291743. doi: 10.3389/FSUFS.2024.1291743
Yang, Q. F., Zhang, P. Y., Ma, Z. P., Liu, D. Q., and Guo, Y. J. (2022). Agricultural economic resilience in the context of international food Price fluctuation—An empirical analysis on the Main grain–producing areas in Northeast China. Sustain. For. 14:14102. doi: 10.3390/SU142114102
Yao, R. K., Ma, Z. S., Wu, H. T., and Xie, Y. F. (2024). Mechanism and measurement of the effects of industrial agglomeration on agricultural economic resilience. Agric. 14:337. doi: 10.3390/AGRICULTURE14030337
Ye, Y. M., Zou, P., Zhang, W. H., Liu, X. Q. H., Liu, B., and Kang, X. L. (2022). Spatial–temporal evolution characteristics of agricultural economic resilience: evidence from Jiangxi Province, China. Agron 12, –3144. doi: 10.3390/AGRONOMY12123144
Yu, L. Y., Shi, C. Y., Yang, X., and Mu, Y. Y. (2023). Impact mechanism of rural digitization on China's agricultural resilience: An empirical study based on the coupling coordination degree and mediating effect models. J. China Agric. Univ. 28, 308–320. doi: 10.11841/j.issn.1007-4333.2023.07.26
Zhang, Y. Y., Wu, Q., and Sun, S. M. (2019). The determinants of hog scale production and its spatial correlation: An analysis based on data from 13 Main provinces of hog production in China. Chinese Rural Econ. 1, 62–78.
Zhang, J. F., Yang, Z. R., and He, B. (2023). Does digital infrastructure improve urban economic resilience? Evidence from the Yangtze River Economic Belt in China. Sustain. For. 15:14289. doi: 10.3390/SU151914289
Zhang, J. F., Yang, Z. R., and He, B. (2024). Empowerment of digital Technology for the Resilience of the logistics industry: mechanisms and paths. Systems 12:278. doi: 10.3390/SYSTEMS12080278
Zhao, T., Zhang, Z., and Liang, S. K. (2020). Digital economy, entrepreneurship, and high-quality economic development: empirical evidence from urban China. J. Manag. World 36, 65–76. doi: 10.19744/j.cnki.11-1235/f.2020.0154
Zhao, X., and Zhao, R. (2024). The impact and mechanism of digital villages on agricultural resilience in ecologically fragile ethnic areas: evidence from China’s provinces. Agric. 14:221. doi: 10.3390/agriculture14020221
Zhong, S., Li, J. W., and Guo, X. Y. (2022). Analysis on the green total factor productivity of pig breeding in China: evidence from a meta-frontier approach. PLoS One 17, –e0270549. doi: 10.1371/journal.pone.0270549
Zhou, J., Chen, H. P., Bai, Q. Y., Liu, L. X., Li, G. H., and Shen, Q. L. (2023). Can the integration of rural industries help strengthen China’s agricultural economic resilience? Agriculture 13:1813. doi: 10.3390/AGRICULTURE13091813
Keywords: digitization, the pig breeding industry, industrial resilience, breeding technology level, scale level, sustainable development
Citation: Hua J, Ma C, Li W, He Z and Zhu P (2024) Effects and mechanisms of digitalization to enhance the resilience of the hog breeding industry: evidence from China. Front. Sustain. Food Syst. 8:1467162. doi: 10.3389/fsufs.2024.1467162
Received: 19 July 2024; Accepted: 02 October 2024;
Published: 15 October 2024.
Edited by:
Suresh Neethirajan, Dalhousie University, CanadaReviewed by:
Syahrul Junaini, University of Malaysia Sarawak, MalaysiaCopyright © 2024 Hua, Ma, Li, He and Zhu. This is an open-access article distributed under the terms of the Creative Commons Attribution License (CC BY). The use, distribution or reproduction in other forums is permitted, provided the original author(s) and the copyright owner(s) are credited and that the original publication in this journal is cited, in accordance with accepted academic practice. No use, distribution or reproduction is permitted which does not comply with these terms.
*Correspondence: Zejun He, a2VuaGV6akAxNjMuY29t; Panpan Zhu, enBwQGhlbmF1LmVkdS5jbg==
Disclaimer: All claims expressed in this article are solely those of the authors and do not necessarily represent those of their affiliated organizations, or those of the publisher, the editors and the reviewers. Any product that may be evaluated in this article or claim that may be made by its manufacturer is not guaranteed or endorsed by the publisher.
Research integrity at Frontiers
Learn more about the work of our research integrity team to safeguard the quality of each article we publish.