- College of Economics and Management, Qingdao Agricultural University, Qingdao, China
This study focuses on the role of agricultural production agglomeration in strengthening agricultural economic resilience, exploring the threshold effect of agricultural technological innovation level and the spatial spillover effect of agricultural production agglomeration on agricultural economic resilience. We conducted research across 31 provinces (including autonomous regions and municipalities) in China from 2007 to 2022. By constructing the evaluation index system of agricultural economic resilience, the entropy value method is used to measure the value of agricultural economic resilience, and then kernel density estimation and spatial econometrics model, threshold regression model are used to analyze the relationship between agricultural production agglomeration, agricultural technological innovation and agricultural economic resilience. (1) The analysis of the spatiotemporal evolution trend shows that the overall level of China’s agricultural economic resilience continued to rise, and presented a spatial development pattern of “high in the east and low in the west.” The overall level of agricultural production agglomeration in China shows a trend of first rising and then falling, among which the level of agricultural production agglomeration in the central region is significantly higher than that in the northwest and southeast regions. (2) The spatial Durbin model shows that agricultural production agglomeration can not only effectively improve the level of local agricultural economic resilience, but also have a positive impact on neighboring agriculture economic resilience produces positive spatial spillover effects. (3) Agricultural production agglomeration can improve the level of agricultural economic resilience by promoting agricultural social service. (4) The impact of agricultural production agglomeration on agricultural economic resilience shows great differences in different geographical regions. Among them, agricultural production agglomeration in the central region has a significant positive impact on the agricultural economic resilience of both local and adjacent areas. (5) The threshold effect model shows that the impact of agricultural production agglomeration on agricultural economic resilience has significant nonlinear characteristics, and its impact shows an increasing marginal effect as the level of agricultural technological innovation increases. To address this, policymakers should reinforce agricultural cluster construction, boost innovation capacity and treasure spillover effects between regions. These insights provide valuable direction for policymakers in crafting effective measures to enhance agricultural economic resilience.
1 Introduction
As a basic industry for national economic construction and development, the healthy development of agriculture is of great significance to ensuring national food security and maintaining social stability (Berry et al., 2015; Calicioglu et al., 2019). Since the reform and opening up, China’s comprehensive agricultural production capacity has been continuously improved, and the agricultural economy has developed rapidly (Lai et al., 2020). However, China’s agriculture is also facing many severe challenges, which seriously restrict the sustainable development of the agricultural economy. On the one hand, with the decreasing marginal effect of factor input and the tightening constraints of resource and environmental, the damage to public interest caused by extensive land use exceeds the benefits of producing additional food on new land, and it endangers environmental sustainability (Burney et al., 2010; Tilman et al., 2011). On the other hand, due to the unreasonable food consumption structure and the significant change in food demand structure, the pressure on the effective food supply and transformation of the agricultural food system has continued to increase (Elechi et al., 2022). At the same time, with the increase in the frequency and intensity of uncertainty events such as extreme weather and pandemics, the uncertainty and instability risks faced by the agricultural modernization process are constantly superimposed (Mishra et al., 2021; Li and Liu, 2023).
Maintaining the stable and sustainable development of the agricultural economy is a practical step in promoting agricultural modernization in China. The 20th National Congress of the Communist Party of China outlined a visionary roadmap, emphasizing the acceleration of constructing a robust agricultural country, with a foundational guarantee of enhancing economic resilience. Due to the natural attributes of agricultural production, such as long cycles and regionality, as well as the inherent weaknesses in circulation and consumption, agriculture is very vulnerable to uncertain shocks (Lu and Zhu, 2011; Atzberger, 2013; Han and Yang, 2024). How to enhance agriculture’s ability to resist and adapt to shocks and improve the agricultural economic resilience has become a practical problem that needs to be solved in the process of achieving sustainable agricultural development (Bennett et al., 2014; Meuwissen et al., 2019; Hertel et al., 2021). For this reason, exploring the driving mechanism and policy optimization path for strengthening the resilience of the agricultural economy is undoubtedly of great practical significance.
Agricultural production agglomeration, a crucial driver of agricultural modernization, impacts the agricultural system through factor resource reallocation and numerous spillover effects. This agglomeration is anticipated to serve as a novel institutional arrangement to bolster agricultural economic resilience (Yao et al., 2024). Due to differences in resource endowments and natural conditions, as well as effective government guidance and support, various regions have formed advantageous agricultural product production agglomeration areas, such as the vegetable agglomeration area in Shouguang, Shandong, and the cotton agglomeration area in Xinjiang, which are considered by many scholars to be effective choices for promoting agricultural modernization (Doronina et al., 2016; Han and Yang, 2023). The report of the 20th National Congress of the Communist Party of China clearly pointed out that it is necessary to develop various forms of moderate-scale agricultural operations. As an important part of agricultural scale and intensive management, agricultural production agglomeration has become an important path to solve a series of problems facing China’s agriculture (Du et al., 2017).
Based on this, unlike previous literature that focuses on the impact of the digital platform (Singh et al., 2023), CAP direct payments (Žičkienė et al., 2022) on agricultural economic resilience, this paper focuses on the role of agricultural production agglomeration in strengthening agricultural economic resilience, including spatial spillover effects and threshold effects. As agricultural production agglomeration itself has the characteristics of spatial correlation and dynamic change, few studies have deeply examined the spatial spillover mechanism of agricultural production agglomeration on agricultural economic resilience. Therefore, this paper uses a spatial econometric model to test its spatial effect. In addition, the effect of agglomeration externalities is limited by the level of local technological innovation. When the technological innovation capability meets certain threshold conditions, a greater agglomeration effect can be generated. Therefore, this paper uses threshold regression to determine the threshold conditions for exerting agglomeration effects. Accordingly, this paper attempts to answer the following questions: Can agricultural production agglomeration enhance agricultural economic resilience, and what is the effect? How can the impact of agricultural production agglomeration be more conducive to improving the agricultural economic resilience? In particular, does its impact have a spatial spillover effect? The answers to these questions are of great practical significance for giving full play to the role of agricultural production agglomeration and strengthening the resilience of the agricultural economy, in order to provide theoretical support and decision-making reference for improving the agricultural economic resilience with agricultural production agglomeration as the carrier.
2 Literature review
The concept of “resilience” originated from the field of physics, and later Holling (1973) introduced it into the field of ecology. With the continuous deepening of research, the concept of resilience has gradually been introduced into the discipline of agricultural economics, and the concept of “agricultural economic resilience” has been derived. At present, research on economic resilience mainly focuses on regional economic resilience, while research on agricultural economic resilience is relatively less and more concentrated in time. At first, scholars mainly carried out theoretical analysis from the forging path of agricultural system resilience (Tittonell, 2020; van der Lee et al., 2022), and then measured the resilience of the agricultural economy and conducted empirical analysis. The methods used included the counterfactual index method (Ming et al., 2024) and the indicator system method (Volkov et al., 2021). In recent years, the indicator system method has become mainstream, and the measurement dimensions have become increasingly clear, mainly including three dimensions: resistance, recovery, and reformation. Among them, resistance refers to the ability to reduce the impact when encountering a disaster, recovery refers to the ability to restore to the original state after encountering a disaster, and reformation refers to the ability to change and adjust oneself after encountering a disaster. In terms of research content, some literature has measured agricultural economic resilience or food production resilience and analyzed its spatiotemporal differentiation characteristics (Berry et al., 2022; Ye et al., 2022; Jiang et al., 2023). In the research on influencing factors, the focus is mainly on digital inclusive finance (Zhao et al., 2023), digital platform (Singh et al., 2023), agricultural insurance (Kramer et al., 2019), etc. The studies have shown that these factors have a positive role in improving agricultural resilience.
As an important means to improve agricultural production efficiency, will agricultural production agglomeration affect agricultural economic resilience? At present, there is a lack of targeted research on this issue in the academic community. Existing literatures have underscored the importance of agglomeration effects on economic resilience in manufacturing and financial industries. Hill et al. (2012) found that the impact of agglomeration of durable goods manufacturing in the United States on economic resilience in different periods of economic development was heterogeneous. Martin et al. (2017) took enterprises as the research object and found that enterprises in agglomeration areas have better resilience, and their survival rate and growth rate in crises are higher. Li (2023) examined the driving effect of financial agglomeration on urban economic resilience in multiple dimensions.
Recent studies on agricultural production agglomeration mainly focus on areas such as high-quality agricultural development (Zhao and Zhao, 2020) and environmental efficiency (Yan et al., 2022). The research on the improvement of economic resilience by agglomeration of different industries in the existing literature can provide a reference for studying the impact of agricultural production agglomeration on agricultural economic resilience.
In summary, in terms of research content, existing research mainly focuses on the economic effects of industrial and financial agglomeration, but has not yet focused on agricultural production, ignoring the role of agricultural production agglomeration in strengthening agricultural resilience, which provides room for expansion for this article’s research. In terms of research methods, the traditional linear econometric model has limited fitting ability for nonlinear relationships, and its spatial homogeneity assumption will lead to biased estimation results, making it difficult to explain the complex relationship between agricultural production agglomeration and agricultural economic resilience. Therefore, this paper establishes a spatial econometric model to examine the spatial impact of agricultural production agglomeration on agricultural economic resilience, correcting the estimation bias caused by ignoring spatial spillover effects; and constructs a threshold model to reveal the nonlinear impact characteristics of agricultural production agglomeration on agricultural economic resilience, enriching existing research results.
3 Theoretical analysis and research hypothesis
According to the theory of agglomeration economies, agglomeration can generate externalities and economies of scale (Marshall, 1890), promoting changes in traditional agricultural production methods and management systems, and thus affecting agricultural economic resilience. Specifically, the impact mechanism of agricultural production agglomeration on agricultural economic resilience is: (1) Scale economy effect. Agricultural production agglomeration absorbs factors such as land, labor, and capital and produces large-scale production effects. This can not only improve the utilization efficiency of agricultural production materials and reduce agricultural production costs (Deng et al., 2020), but also stimulate the supply of production service outsourcing, promote the deepening of vertical division of labor in agricultural production, and improve agricultural production efficiency (Li et al., 2019). In addition, agricultural production agglomeration can also effectively alleviate farmers’ market transaction difficulties and reduce the difficulty of information search and transaction costs (Pamphile, 2012), further strengthening the resilience of the agricultural economy. (2) Knowledge spillover effect. Agricultural production agglomeration attracts similar production technologies and helps to exert the knowledge spillover effect (Verspagen and Schoenmakers, 2004). The geographical proximity and transparency of agricultural production make in-depth communication between farmers possible. On the basis of promoting the rapid dissemination of agricultural information and production technology, it also reduces the farmers’ information search costs and technology promotion costs. At the same time, the diffusion and application of advanced production technologies promote the development of agricultural production in the direction of automation and intensification, further improve agricultural production efficiency and optimize the quality of agricultural products, and enhance the resilience of the agricultural economy. In short, agricultural production agglomeration mainly promotes the improvement of the level of agricultural economic resilience through economies of scale and knowledge spillover effects. Based on this, the following research hypothesis is proposed:
H1: Agricultural production agglomeration can enhance the agricultural economic resilience.
Agricultural production agglomeration can not only directly affect the agricultural economic resilience, but also indirectly affect the agricultural economic resilience through agricultural social service. On the one hand, from the perspective of technological demand, with the improvement of agglomeration, the profit motive gradually replaces the livelihood motive, and farmers are eager to obtain the division of labor economy by improving production efficiency (Jabbour, 2015), which leads to an increase in the demand for effective technology (Cai and Cai, 2014). The increased technological demand needs to be met through market division of labor in the production process, thus giving rise to agricultural social service organizations (Li et al., 2019). On the other hand, from the perspective of transaction costs, when the level of agricultural production agglomeration is low, the dispersion of farmers means that there are high organizational costs for the alliance. On the contrary, as the level of agglomeration increases, the concentration of farmers makes reductions to the cost of organization and significantly improves organizational efficiency, which is conducive to the construction of agricultural social service organizations. Agricultural social service will provide farmers with production materials, technical support, market information and other services by replacing the original factor inputs, enabling farmers to enjoy economies of scale brought about by division of labor, cooperation and externalities, which results in the improvement of the agricultural production efficiency and the agricultural economic resilience. Therefore, the following research hypothesis is proposed:
H2: Agricultural production agglomeration can indirectly improve the level of agricultural economic resilience by promoting agricultural social service.
Agglomeration often presents obvious spatial externality characteristics (Zhang et al., 2022). On the one hand, the positive effects of agricultural production agglomeration on regional agricultural economic development, technological progress, etc. can play a leading and exemplary role in neighboring regions. In addition, agricultural production agglomeration can promote inter-regional division of labor and cooperation, promote the optimal allocation of agricultural production factors in a larger area, and enhance the role of production agglomeration in promoting the agricultural economic resilience of neighboring regions. On the other hand, in the process of agricultural production agglomeration, with the flow of capital, technology and other resource factors to the core area, obvious siphon effects and polarization effects appear, leading to uneven agricultural economic development among regions, thus forming a negative impact on the agricultural economic resilience of neighboring areas. Therefore, the impact of agricultural production agglomeration on the agricultural economic resilience of neighboring regions depends on the positive and negative externality effects of production agglomeration. If the positive externality is greater than the negative externality, it is a positive impact, otherwise it is a negative impact. Therefore, the following research hypothesis is proposed:
H3: Agricultural production agglomeration has a spatial spillover effect on agricultural economic resilience.
In theory, agricultural production agglomeration can improve the level of agricultural economic resilience due to economies of scale and knowledge spillovers, but in reality, there are large differences in the ability of agricultural production agglomeration regions to cope with uncertainty risks. The possible reason lies in the level of local agricultural technology innovation. Agricultural technology innovation breaks the limitations of resource scarcity and backward traditional technology, greatly improving factor utilization efficiency and agricultural production efficiency, thereby promoting agricultural economic growth and alleviating the vulnerability of agricultural production when facing uncertain shocks (Li et al., 2021; He and Yang, 2021). Accelerating the improvement of agricultural technology innovation level and leading the high-quality development of agriculture can make full use of the positive externalities generated by agricultural production agglomeration, promote the diffusion and application of advanced agricultural production technology and the exchange and dissemination of tacit knowledge among farmers, promote the improvement of agricultural production efficiency, and thus strengthen the resilience of the agricultural economy. If agricultural technology innovation is insufficient and agricultural production technology is relatively backward, the agglomeration effect of agricultural production agglomeration on agricultural economic resilience will be greatly limited. At the same time, agricultural production agglomeration can expand farmers’ effective demand for agricultural technology innovation results, generate feedback on agricultural technology innovation, and form a virtuous circle. Therefore, agricultural technology innovation can strengthen the positive externalities of agricultural production agglomeration and enhance agricultural economic resilience. Based on the above analysis, the following research hypotheses are proposed:
H4: Agricultural technological innovation has a threshold effect in the relationship between agricultural production agglomeration and agricultural economic resilience. The higher the level of technological innovation, the greater the strengthening effect of agricultural production agglomeration on agricultural economic resilience.
4 Model setting and index selection
4.1 Model setting
4.1.1 Spatial metrology model
The Spatial Durbin model (SDM) is used to explore the spatial spillover effect of agricultural production agglomeration on agricultural economic resilience. The model is set as Equation 1:
Among them, represents the spatial autoregression coefficient, represents the spatial weight matrix, and represent the spatial spillover coefficient of agricultural production agglomeration and other control variables. We construct a geographic distance weight matrix for model estimation.
4.1.2 Threshold effect model
Agricultural technological innovation is an important factor affecting agricultural economic resilience. With the enhancement of agricultural technological innovation effect, the impact of agricultural production agglomeration on agricultural economic resilience may have a nonlinear growth effect. Therefore, we construct the following model based on the nonlinear panel threshold model proposed by Hansen (1999) (see Equation 2):
Among them, the threshold variable is agricultural technological innovation, which is measured by the number of authorized agricultural effective invention patents. The is a specific threshold value, and is an indicator function. , and represent the threshold effect, which is the estimated coefficient of the impact of agricultural production agglomeration on agricultural economic resilience when the threshold variable is less than or greater than the threshold value.
4.2 Variables setting
4.2.1 Dependent variable
The explained variable in this paper is agricultural economic resilience (Res). Considering the limitations of single index method and economic model in measuring scope, this paper adopts comprehensive index system method to measure agricultural economic resilience.In the setting of guideline layers, Jiang et al. (2024) and Chen et al. (2024) constructs a comprehensive evaluation index system for agricultural economic resilience and agricultural resilience based on the three dimensions of resistance, recovery and regeneration. In terms of the selection of target layers, Chen et al. (2024) measures recovery from the perspective of infrastructure, policy and financial support, and measures regeneration from the perspective of agricultural human capital and investment in agricultural fixed asset, while Berry et al. (2022) defines agricultural economic resilience from the perspective of financial stability, economic performance, income and crop diversification. Therefore, referring to the practices of Berry et al. (2022), Chen et al. (2024), and Jiang et al. (2024), this paper systematically constructs an agricultural economic resilience evaluation index system from three dimensions of resistance, recovery and reformation, and selects six target layers from economic foundation, financial support, sustainability, restoration, human capital, and infrastructure (see Table 1).
Specifically, (1) resistance refers to the ability of the economic system to maintain normal operation of its internal functions after being subjected to external shocks, that is, the ability of the agricultural system to resist uncertain shocks, which is the basis for subsequent dynamic adaptation, adjustment and reorganization of development paths. Resistance is mainly measured from two dimensions: economic foundation and financial support. (2) Recovery refers to the ability to return to a stable equilibrium state while maintaining normal internal functions, that is, the ability of the agricultural system to dynamically adapt to resource and environmental constraints. Recovery is mainly measured from two aspects: sustainability and restoration. (3) Reformation refers to the ability to build a new development model through scientific and technological innovation, that is, the ability of the agricultural system to reorganize the development path to achieve a change in production methods. Reformation is mainly measured from two aspects: human capital and infrastructure. The entropy method is used to assign weights to each indicator to obtain the agricultural economic resilience index.
4.2.2 Independent variable
Current methods for measuring the level of agricultural production agglomeration include industry concentration, Herfindahl index, location entropy, spatial Gini coefficient, etc. In comparison, the location entropy index is widely used because it can better eliminate factors such as regional scale differences. Therefore, this paper uses location entropy to measure the degree of agricultural production agglomeration (Agg), and its expression is shown in Equation 3:
Among them, and represent the regional grain crop sowing area and agricultural crop sowing area, respectively. and represent the national grain crop sowing area and agricultural crop sowing area, respectively.
4.2.3 Threshold variable
This study employs agriculture technology innovation as the threshold variable, specifically gauged by the number of authorized valid agricultural invention patents.
4.2.4 Control variables
The following variables are selected as control variables in this paper: (1) Effective Irrigation Rate (Water): Measured by the proportion of effective irrigation area to cultivated land area. (2) Fiscal Support (Policy): Represented by local agricultural, forestry and water affairs expenditure. (3) Green Production (Green): Represented by the application of regional agricultural fertilizers. (4) Industrial Structure (Struc): Measured by the proportion of the sum of the added value of the secondary and tertiary industries to GDP. A detailed overview of the related variables and their descriptions is presented in Table 2.
5 Analysis of empirical results
5.1 Spatiotemporal evolution characteristics of agricultural production agglomeration and agricultural economic resilience
5.1.1 Spatiotemporal evolution characteristics of agricultural production agglomeration
Considering the approach of Zhao and Zhang (2022) and based on the heterogeneity of the Hu Huanyong Line regional segmentation, the 31 provinces are divided into three regions: the northwest region, the southeast region, and the central region (provinces not represented by the northwest and southeast regions). The location entropy index of agricultural production agglomeration was calculated using Excel, and the estimated values for the country and three regions from 2007 to 2022 were obtained. The results are shown in Figure 1. It can be seen that the level of agricultural production agglomeration in the central region is the highest, and the northwest region was higher than the southeast region before 2016, and lower than the southeast region after 2016, but both of them are lower than the national average. Compared with the northwest region, the central region has a flatter terrain, with advantages in natural conditions and geographical location, which creates good conditions for large-scale agricultural operations and a high level of agricultural agglomeration. The reason why the level of agricultural production agglomeration in the southeast region is low is that the economic development in the southeast region is relatively high, which will occupy arable land resources while expanding urban area, and surrounding cities may choose to plant cash crops with higher economic benefits or choose to work outside and withdraw from agricultural production.
In addition, the overall level of agricultural production agglomeration in the country and in various regions shows a trend of first rising and then falling, showing a decreasing trend overall. The possible reason is that after 2014, in response to the contradiction between supply and demand of grain varieties, local governments took the initiative to optimize the agricultural production structure and regional layout, appropriately reduced the amount of land devoted to growing corn in non-advantageous areas, and adopted strategies such as replacing corn with soybeans cultivation, replacing grain crop with feed crop or oil crop cultivation to adjust the agricultural planting structure.
5.1.2 Spatiotemporal evolution characteristics of agricultural economic resilience
Based on the measurement result and quantile classification method, agricultural economic resilience is divided into four levels: low resilience level, slightly low resilience level, slightly high resilience level and high resilience level to show its spatial evolution pattern. We also characterized the dynamic evolution trend of agricultural economic resilience, which is portrayed with the kernel density estimation method.
5.1.2.1 Temporal characteristics of agricultural economic resilience
The projection of the kernel density curve of agricultural economic resilience from 2007 to 2022 on the horizontal axis continues to extend to the right, indicating that the overall level of agricultural economic resilience across the country continues to rise (see Figure 2). Specifically: (1) From the perspective of the center of gravity and change range of the curve, the center of gravity and change range of the curve continue to move to the right, indicating that the level of national agricultural economic resilience tends to increase significantly. (2) From the perspective of the height and width of the main peak of the curve, the height of the main peak continues to decrease. The width continues to expand, indicating that regional differences in agricultural economic resilience tend to expand. (3) From the left and right tails of the curve, the left tail of the curve is significantly shortened and the right tail is significantly extended, indicating that the low-value areas of agricultural economic resilience have obvious catching-up potential trend, and the growth rate of high-value areas has also accelerated significantly. In general, although the national agricultural economic resilience level has been significantly improved with the rapid economic and social development in recent years, there are obvious heterogeneity of factors such as the intensity of financial support and economic development levels affect the agricultural economic resilience level and growth rate in different regions, which making it difficult for areas with low agricultural economic resilience to quickly catch up with areas with high resilience in the short term. The gap between the low resilience and high resilience areas continues to widen for a certain period of time.
5.1.2.2 Spatial characteristics of agricultural economic resilience
From 2007 to 2022, the level of agricultural economic resilience in China has increased year by year, and it presents a spatial development pattern of “high in the east and low in the west” (see Figure 3). Specifically: (1) In 2007, the agricultural economic resilience in most areas was at a low level of resilience, and only Jiangsu Province was at a high level of resilience. (2) From 2007 to 2012, the number of areas with slightly high level of agricultural economic resilience increased to 3, all distributed in the eastern coastal areas, and Jiangsu Province jumped to a high level of resilience. (3) From 2012 to 2017, the agricultural economic resilience of Heilongjiang Province, Sichuan Province, Henan Province and Hebei Province jumped to a slightly high level of resilience, and the number of areas with slightly high level increased to 7. Jiangsu Province is still the only high level area. (4) From 2017 to 2022, the number of regions with slightly high level of agricultural economic resilience expanded significantly in the east and central regions of China, and the proportion increased to 32%. At the same time, the number of high level areas increased to 8. (5) In 2022, the agricultural economic resilience of most areas in China was at a slightly high level of resilience or above. In general, the resilience of China’s agricultural economy continues to improve, and the areas of high resilience are gradually expanding from the eastern coastal region to the central and northeastern regions, presenting an increasingly balanced spatial evolution pattern as a whole.
5.2 Spatial spillover effects of agricultural production agglomeration on agricultural economic resilience
Before discussing the spatial effects of each variable, it is necessary to test whether there is spatial correlation. Therefore, the Moran’s I index was calculated (Modica et al., 2024) and the relevant results are shown in Table 3. It can be seen that the Moran’s I index of agricultural economic resilience and agricultural production agglomeration from 2007 to 2022 are positive, and both have passed the significance test, indicating that there are obvious spatial positive correlation characteristics in both provincial agricultural economic resilience and agricultural production agglomeration in China. Notably, compared with other years, the Moran’s I index of agricultural economic resilience shows stronger significance in 2010–2021, which means agricultural economic resilience has a more obvious spatial correlation during these years.
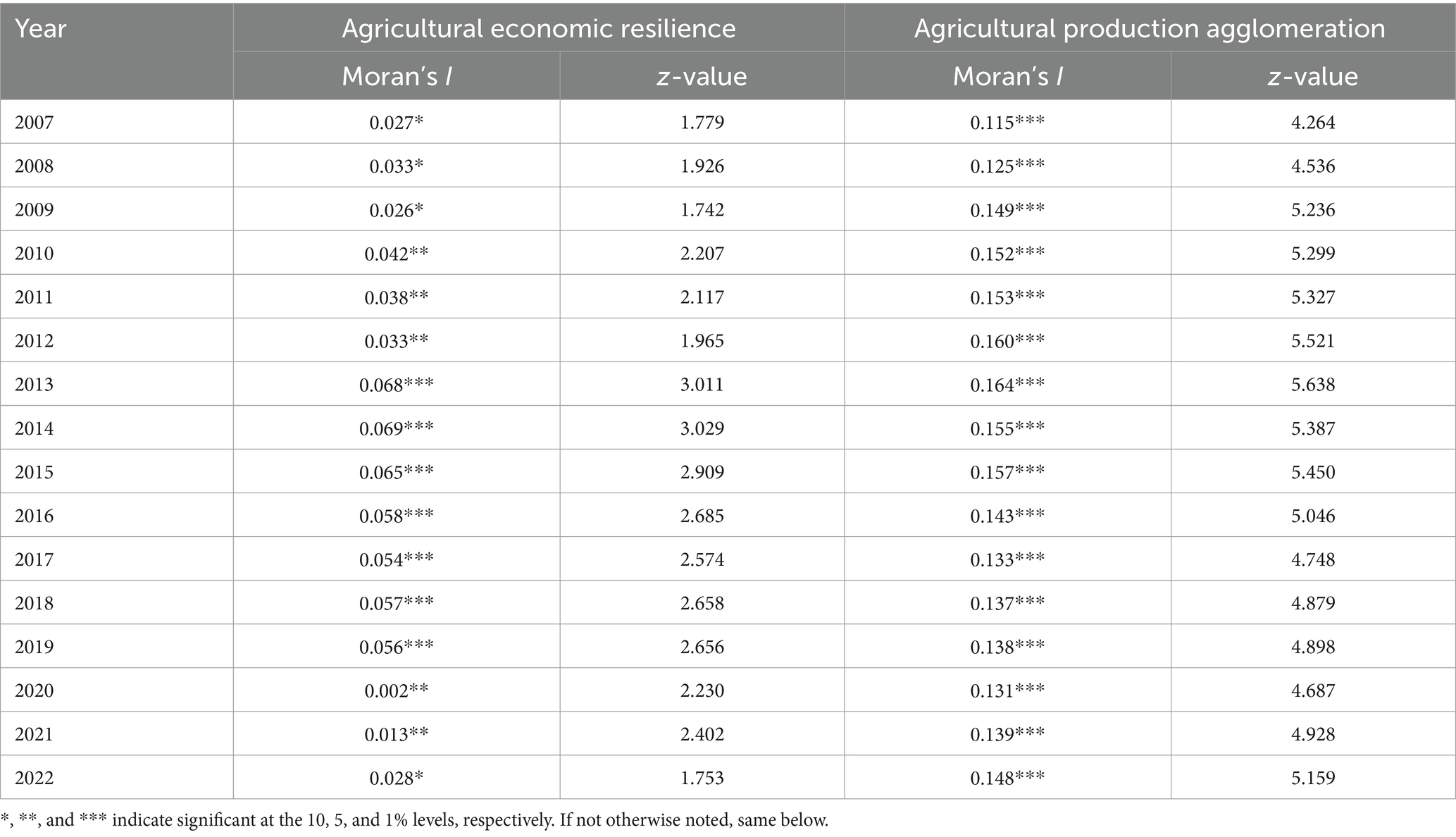
Table 3. Moran’s I index of agricultural economic resilience and agricultural production agglomeration from 2007 to 2022.
In order to test the robustness of the spatial correlation results and determine the specific form of the model, we conducted LM test, LR test, Wald test, Hausman test and joint significance test in sequence. The results are shown in Table 4. It can be seen that LM and Robust - LM both pass the significance test, and both LR and Wald tests reject the null hypothesis that the model degenerated into SAR and SEM at the 1% significance level, so SDM for the best choice. In addition, the Hausman and joint significance tests are significantly positive, so we choose the double fixed effects spatial Durbin model to test the direct effects and spillover effects.
Lesage and Pace (2009) pointed out that when the spatial lag coefficient of the explained variable is significantly non-zero, the regression coefficient obtained by using the SDM model to measure the spillover effect will have a systematic bias. In order to measure the spatial spillover effect of agricultural production agglomeration on agricultural economic resilience objectively, we use the partial differential method to decompose the explained spatial spillover effect of explanatory variables into direct effects, indirect effects and total effects. The results are shown in Table 5. It can be seen that whether it is a two-way fixed effect or a spatial direct effect, agricultural production agglomeration has a positive impact on agricultural economic resilience and has passed the significance test. In addition, the spatial autoregressive coefficient of agricultural economic resilience is significantly positive under the weight of the geographical matrix, indicating that the agricultural economic resilience of provinces with geographically adjacent locations exhibits “spatial strategic complementarity,” that is, an increase in the level of agricultural economic resilience in a certain place will promote the enhancement of agricultural economic resilience in its neighboring areas.
From the perspective of the spatial direct effect, the estimated coefficient of agricultural production agglomeration is 0.119 and passed the 1% significance level test, indicating that agricultural production agglomeration has a significant positive effect on agricultural economic resilience. The hypothesis 1 is established. As the level of agricultural production agglomeration continues to improve, the degree of specialization of agricultural production has increased, and the sharing of production technology has generated positive externalities of agglomeration, which effectively improves resource utilization efficiency and reduces agricultural production costs, promotes agricultural production efficiency, and thereby strengthens agricultural economic resilience. From the perspective of the spatial spillover effect of agricultural production agglomeration on agricultural economic resilience, the estimated coefficient of agricultural production agglomeration is significantly positive at the 5% level, with an estimated value of 0.373, indicating that agricultural production agglomeration has significant room for agricultural economic resilience. The spillover effect is greater than the siphon effect, and the hypothesis 3 is established. Agricultural agglomeration in neighboring areas can not only promote the supply of agricultural production factors, attract advanced production technology, pre- and post-production and other related industries to gather in the area, forming an industrial development center, but also enable the sharing of equipment and facilities, thereby promoting the development of local agricultural economy.
5.3 Robustness and endogeneity
Robustness testing is conducted by replacing the core explanatory variable and weight matrix. Considering that there is an obvious positive correlation between grain yield and grain sown area, and referring to the research of Zhao and Zhou (2020), grain crop yield is selected as an alternative indicator of sown area to calculate the location entropy index to measure the level of agricultural production agglomeration in each region. The estimation results are shown in column (1) of Table 6. Regarding the replacement of the weight matrix, the adjacency matrix (WL), economic distance matrix (WE) and economic geography nested matrix (WQ) are selected to replace the original matrix and the model is re-estimated. The results are shown in columns (2)–(4) of Table 6. It can be seen that the coefficient signs and significance levels of the decomposition effect and the total effect under different variable measurement methods and weight matrices are basically consistent with the benchmark regression.
In order to alleviate possible endogeneity, the lagged variable of the endogenous variable agricultural production agglomeration ( ) is selected as an instrumental variable, and the 2 SLS method is used for estimation. The results are shown in Table 7. In the first-stage regression results, the RKF test and the unidentifiable test show that the null hypothesis of “insufficient identification of instrumental variables” is rejected when there is no problem of weak instrumental variables, that is, the instrumental variables selected in this study is relatively reasonable. In the second-stage regression results, the impact coefficient of agricultural production agglomeration on agricultural economic resilience is 0.143, which is still statistically significant. Therefore, when the instrumental variable method is effective, the above regression results still hold.
5.4 Mechanism identification and regional difference
5.4.1 Mechanism test
In order to further analyze the mechanism of agricultural production agglomeration on agricultural economic resilience, this paper takes agricultural social service as the mediating variable and uses the stepwise regression method to test its transmission mechanism. The development level of regional agricultural social service is represented by the logarithmic value of output of specialized and auxiliary activities in agriculture, forestry, animal husbandry and fishery in each province, and the regression results are shown in Table 8. Column (2) shows that agricultural production agglomeration has a significant positive impact on agricultural socialization service, indicating that agricultural production agglomeration can promote the development of agricultural socialization service. After including the intermediary variable of agricultural socialization service, column (3) shows that the coefficient of agricultural production agglomeration on agricultural economic resilience drops to 0.097, and is still significant at the 1% level. Therefore, the results show that agricultural social service have a partial mediating effect between agricultural production agglomeration and agricultural economic resilience. The hypothesis 2 is established. Agricultural production agglomeration optimizes resource allocation and improves production efficiency by building a large-scale, specialized and organized agricultural social service system, thereby realizing the agricultural economic resilience effect brought about by external economy of scale.
5.4.2 Heterogeneity analysis based on geographical location
Considering the approach of Zhao and Zhang (2022) and based on the heterogeneity of the Hu Huanyong Line regional segmentation, the 31 provinces are divided into three regions: the northwest region, the southeast region, and the central region (provinces not represented by the northwest and southeast regions). The regression results are shown in Table 9.
It can be seen that the impact of agricultural production agglomeration on agricultural economic resilience varies greatly in the three regions. Specifically, the direct effect and indirect effect of the central region are significantly positive. The reason is that the middle region is an important grain production base in China and has historical and natural advantages in developing agricultural clusters. Therefore, the agricultural agglomeration effect and its spillover effect is more obvious. The agglomeration of agricultural production in the northwest region has a significant negative impact on the agricultural economic resilience of adjacent areas, and has no obvious effect on the area itself. The possible reason is that the northwest region has a poor geographical environment and relatively backward economic development conditions, which restricts the supporting and improvement of agricultural infrastructure, resulting in a greater competitive effect than cooperative effect with neighboring areas, making it impossible to generate positive spillover effects on neighboring areas, and there may even be a siphon effect. The agricultural production agglomeration in the southeast region has a significant positive impact on the agricultural economic resilience of adjacent areas, but its effect on the local agricultural economic resilience is not significant. The possible reason is that the southeast region has a high level of economic development and relatively complete agricultural infrastructure construction, while the room for growth in agricultural production agglomeration is relatively limited. At this time, the effect of agricultural production agglomeration in promoting regional agricultural economic resilience is relatively weak.
5.5 Threshold effect of agricultural production agglomeration on agricultural economic resilience
Through the above analysis, it can be concluded that agricultural production agglomeration helps to improve the level of agricultural economic resilience. However, whether this promotion effect has nonlinear characteristics needs further research. This study uses agricultural technological innovation as a threshold variable to measure the nonlinear impact of agricultural production agglomeration on agricultural economic resilience. Before estimating the threshold model, a panel threshold existence test was conducted based on the method of Hansen (1999). After 300 repeated samplings using the “bootstrap method”, the results are shown in Table 10. It can be seen that in the impact of agricultural production agglomeration on agricultural economic resilience, there is a double threshold effect with agricultural technological innovation as a threshold variable, and the threshold values are 4.771 and 6.172, respectively, (see Table 10).
The regression results of the double threshold model are shown in Table 11. It can be seen that at different stages of agricultural technology innovation, the impact of agricultural production agglomeration on agricultural economic resilience has obvious nonlinear characteristics. When , the coefficient of agricultural production agglomeration is 0.096, and pass the 5% significance test. When , the coefficient of agricultural production agglomeration is 0.121, and passe the 1% significance test. When , the coefficient of agricultural production agglomeration increases to 0.161, and it also pass the 1% significance test. It indicating that as the level of agricultural technological innovation increases, the incentive effect of agricultural production agglomeration on agricultural economic resilience has a marginal increasing trend. It shows that agricultural production agglomeration in areas with higher levels of agricultural technological innovation has a greater effect on improving agricultural economic resilience, and Hypothesis 4 has been verified. When the level of agricultural technological innovation is low, it is difficult to fully exert the positive externality effects of agricultural production agglomeration due to resource and environmental constraints. When the level of agricultural technological innovation is high, factor utilization efficiency and agricultural production efficiency are greatly improved by breaking the constraints of resource scarcity and backward traditional technology, thereby amplifying the strengthening effect of agricultural production agglomeration on agricultural economic resilience.
6 Conclusions and policy implications
6.1 Conclusion
This study uses panel data from 31 provinces (cities) in China from 2007 to 2022, and on the basis of explaining the influencing mechanism of agricultural production agglomeration on agricultural economic resilience, analyzes the spatiotemporal evolution characteristics of agricultural economic resilience, and examines the agricultural production agglomeration Spatial spillover effects and threshold characteristics on agricultural economic resilience. The results found that: (1) The overall level of China’s agricultural economic resilience is constantly rising, and presents a spatial development pattern of “high in the east and low in the west.” The overall level of agricultural production agglomeration in China has shown a trend of first rising and then falling, among which the level of agricultural production agglomeration in the central region is significantly higher than that in the western and eastern regions. (2) Agricultural production agglomeration can not only effectively improve the level of local agricultural economic resilience, but also produce positive spatial spillover effects on the agricultural economic resilience of neighboring areas. For example, under the modern agricultural industrial cluster development model of Shouguang city, Shandong province, its vegetable production has formed a large-scale industrial cluster and built a standardized vegetable industry chain, which not only greatly promoted the regional agricultural economic growth, but also effectively improved the level of agricultural economic resilience. (3) Mechanism analysis shows that agricultural production agglomeration can improve the level of agricultural economic resilience by promoting agricultural social services. (4) Heterogeneity analysis shows that agricultural production agglomeration in the middle zone has a significant positive impact on local agricultural economic resilience, while the effects of the northwest and southeast areas are not significant; agricultural production agglomeration in the middle zone and southeastern area has a significant positive impact on the agricultural economic resilience of adjacent areas, while the northwest area has a significant negative impact. (5) The influence of agricultural production agglomeration on agricultural economic resilience has significant nonlinear characteristics, and its impact shows an increasing trend in marginal effects as the level of agricultural technological innovation increases. Specifically, although agricultural production agglomeration shows a positive effect on agricultural economic resilience when the level of agricultural technological innovation is lower or higher than the threshold value, it is obvious that when the level of agricultural technological innovation is higher than the threshold value, the positive nonlinear effect of agricultural production agglomeration on agricultural economic resilience will be enhanced.
6.2 Policy implications
Drawing from the conclusions, several policy recommendations emerge. Firstly, there is a need to improve the level of agricultural production agglomeration and strengthen the resilience-enhancing effect. Research shows that agricultural production agglomeration has a significant impact on agricultural economic resilience. Promote large-scale and intensive agricultural operations, improve the efficiency of land resource allocation, and achieve a significant increase in agricultural production efficiency. At the same time, we need to build a multi-industry cooperation platform and improve the construction of agricultural production agglomeration areas to expand the scale of agricultural production agglomeration and improve the quality of agglomeration. Promote the improvement of agricultural economic resilience.
Secondly, strengthen inter-regional exchanges and cooperation to promote the coordinated improvement of agricultural economic resilience. Research shows that agricultural production agglomeration has significant spatial spillover effects, that is, agricultural economic resilience will be affected by local and foreign agricultural production agglomeration. Promote exchanges and cooperation in the upstream and downstream regions of the agricultural industry chain, build an inter-regional collaboration platform for related industries, strengthen information exchange and sharing, give full play to the positive external effects of agricultural production agglomeration, resist potential agricultural risks, and enhance the overall agricultural economic resilience.
Thirdly, improve the level of agricultural technological innovation and give full play to the effect of agricultural agglomeration. Research shows that with the improvement of agricultural technological innovation level, the incentive effect of agricultural production agglomeration on agricultural economic resilience has a marginal increasing trend. Therefore, we should further increase support for technological innovation, strengthen the introduction of agricultural innovation technologies, and increase the enthusiasm of local governments to develop agricultural production agglomeration. On the one hand, we should increase R&D investment, attract and cultivate high-level agricultural science and technology talents, and strengthen cutting-edge technological innovation. On the other hand, we should formulate preferential policies for agricultural production agglomeration, scientifically adjust the layout of agricultural industries, optimize the allocation of agricultural resources, and guide agricultural production agglomeration within the carrying capacity of regional resources and the environment.
Data availability statement
The datasets presented in this study can be found in online repositories. The names of the repository/repositories and accession number(s) can be found in the article/supplementary material.
Author contributions
CR: Writing – original draft, Visualization, Software, Methodology, Investigation, Formal analysis, Data curation, Conceptualization. LJ: Writing – review & editing, Validation, Supervision, Resources, Project administration, Funding acquisition.
Funding
The author(s) declare that financial support was received for the research, authorship, and/or publication of this article. National Social Science Foundation General Project ‘Research on the Agricultural Science and Technology Innovation System and Talent Strategy of Major Countries in the World’ (23BGL209).
Conflict of interest
The authors declare that the research was conducted in the absence of any commercial or financial relationships that could be construed as a potential conflict of interest.
Publisher’s note
All claims expressed in this article are solely those of the authors and do not necessarily represent those of their affiliated organizations, or those of the publisher, the editors and the reviewers. Any product that may be evaluated in this article, or claim that may be made by its manufacturer, is not guaranteed or endorsed by the publisher.
References
Atzberger, C. (2013). Advances in remote sensing of agriculture: context description, existing operational monitoring systems and major information needs. Remote Sens. 5, 949–981. doi: 10.3390/rs5020949
Bennett, E., Carpenter, S., Gordon, L., Ramankutty, N., Balvanera, P., Campbell, B., et al. (2014). Toward a more resilient agriculture. Solutions 5, 65–75.
Berry, E. M., Dernini, S., Burlingame, B., Meybeck, A., and Conforti, P. (2015). Food security and sustainability: can one exist without the other? Public Health Nutr. 18, 2293–2302. doi: 10.1017/S136898001500021X
Berry, R., Vigani, M., and Urquhart, J. (2022). Economic resilience of agriculture in England and Wales: a spatial analysis. J. Maps 18, 70–78. doi: 10.1080/17445647.2022.2072242
Burney, J. A., Davis, S. J., and Lobell, D. B. (2010). Greenhouse gas mitigation by agricultural intensification. Proc. Natl. Acad. Sci. 107, 12052–12057. doi: 10.1073/pnas.0914216107
Cai, R., and Cai, S. (2014). An empirical study on outsourcing of agricultural production links: a survey based on the main rice producing areas in Anhui Province. J. Agrotech. Econ. 4, 34–42. doi: 10.13246/j.cnki.jae.2014.04.004
Calicioglu, O., Flammini, A., Bracco, S., Bellù, L., and Sims, R. (2019). The future challenges of food and agriculture: An integrated analysis of trends and solutions. Sustain. For. 11:222. doi: 10.3390/su11010222
Chen, X., An, Y., Pan, W., Wang, Y., Chen, L., Gu, Y., et al. (2024). Dynamic transfer and driving mechanisms of the coupling and coordination of agricultural resilience and rural land use efficiency in China. J. Geogr. Sci. 34, 1589–1614. doi: 10.1007/s11442-024-2262-7
Deng, Q., Li, E., and Ren, S. (2020). Impact of agricultural agglomeration on agricultural non-point source pollution: An analysis of threshold effect based on panel data of prefecture-level cities in China. Geogr. Res. 39, 970–989. doi: 10.11821/dlyj020181090
Doronina, I. I., Borobov, V. N., Ivanova, E. A., Gorynya, E. V., and Zhukov, B. M. (2016). Agro-industrial clusters as a factor of increasing competitiveness of the region. Int. J. Econ. Financ. Issues 6, 295–299.
Du, J., Zhang, J., and Shao, S. (2017). Research on the formation and evolution of China's agricultural industry agglomeration under the background of supply-side reform. Finance Trade Res. 28, 33–46. doi: 10.19337/j.cnki.34-1093/f.2017.05.004
Elechi, J. O. G., Nwiyi, I. U., and Adamu, C. S. (2022). “Global food system transformation for resilience” in Sustainable development. eds. A. I. Ribeiro-Barros, D. S. Tevera, L. F. Goulao and L. D. Tivana, vol. 1 (IntechOpen). Available at: https://www.intechopen.com/chapters/81235
Han, H., and Yang, D. (2023). Study on the spatial spillover effect of agricultural industrial agglomeration on the growth of agricultural green total factor productivity. Arid Land Resour. Environ. 37, 29–37. doi: 10.13448/j.cnki.jalre.2023.134
Han, D., and Yang, Q. (2024). Can the digitalization of agricultural product circulation improve the resilience of agricultural economy. J. Northeast Agric. Univ. 22, 19–32. doi: 10.3969/j.issn.1672-3805.2024.01.004
Hansen, B. E. (1999). Threshold effects in non-dynamic panels: estimation, testing and inference. J. Econ. 93, 345–368. doi: 10.1016/S0304-4076(99)00025-1
He, Y., and Yang, S. (2021). Research on the resilience forging of agricultural industrial chain under the "dual circulation" scenario. Agric. Econ. Issues 10, 78–89. doi: 10.13246/j.cnki.iae.20210714.001
Hertel, T. W., and Elouafi, I., Ewert, F. , Tanticharoen, M. (2021) Building resilience to vulnerabilities, shocks and stresses: A paper on action track 5. Bonn: Center for Development Research (ZEF) in cooperation with the scientific group for the UN food system summit 2021. Online-Ausgabe in bonndoc.
Hill, E., Clair, T., Wial, H., Wolman, H., Atkins, P., Blumenthal, P., et al. (2012). “Economic Shocks and Regional Economic Resilience” in Urban and regional policy and its effects: Building resilient regions. eds. M. Weir, N. Pimdus, and H. Wial (Washington: Brookings Institution Press), 193–274.
Holling, C. S. (1973). Resilience and stability of ecological systems. Annu. Rev. Ecol. Syst. 4, 1–23. doi: 10.1146/annurev.es.04.110173.000245
Jabbour, L. (2015). Determinants of international vertical specialization and implications on technology spillovers. Geophys. Prospect. Petrol. 2, 188–196. doi: 10.3969/j.issn.1000-1441.2015.02.010
Jiang, H., Chen, Y., and Liu, Z. (2023). Spatiotemporal pattern of China's grain production resilience and its influencing factors. Econ. Geogr. 6, 126–134. doi: 10.15957/j.cnki.jjdl.2023.06.013
Jiang, J., Wu, H., Li, S., and Liu, Q. (2024). Impact of rural population aging on the resilience of the agricultural economy: analysis based on the perspective of production factors. East China Econ. Manag. 8, 94–105. doi: 10.19629/j.cnki.34-1014/f.240406003
Kramer, B., Hellin, J., Hansen, J., Rose, A., and Braun, M. (2019). Building resilience through climate risk insurance: insights from agricultural research for development (CCAFS Working Paper No. 287). Wageningen, the Netherlands: CGIAR Research Program on Climate Change, Agriculture and Food Security (CCAFS). Available at: https://hdl.handle.net/10568/106171
Lai, Z., Chen, M., and Liu, T. (2020). Changes in and prospects for cultivated land use since the reform and opening up in China. Land Use Policy 97:104781. doi: 10.1016/j.landusepol.2020.104781
Li, S. (2023). Financial agglomeration, innovation and entrepreneurship vitality and urban economic resilience. Econ. Survey 40, 26–36. doi: 10.15931/j.cnki.1006-1096.2023.04.007
Li, Y., and Liu, Y. (2023). Farmers' education empowers rural industry resilience: internal mechanism and action path. J. Hunan Agric. Univ. 24, 54–64. doi: 10.13331/j.cnki.jhau(ss).2023.03.007
Li, B., Xing, L., and Xu, X. (2019). Can agricultural production agglomeration promote farmers' income growth? Empirical evidence from freshwater aquaculture. Agric. Technol. Econ. 5, 39–51. doi: 10.13246/j.cnki.jae.2019.05.004
Li, H., Zhang, J., Si, L., and He, K. (2021). The impact and mechanism of agricultural technological innovation on agricultural development quality: An empirical analysis based on spatial perspective. Res. Dev. Manag. 33, 1–15. doi: 10.13581/j.cnki.rdm.20201077
Lu, K., and Zhu, Q. (2011). Rerecognizing the characters and functions of agriculture. J. South China Agric. Univ. 10, 19–24. doi: 10.3969/j.issn.1672-0202.2011.01.003
Martin, P., Mayer, T., and Mayneris, F. (2017). Are clusters more resilient in crises? Evidence from French exporters in 2008—2009 the factory-free economy: Outsourcing, Servitization, and the future of industry. Oxford: Oxford University Press.
Meuwissen, M. P., Feindt, P. H., Spiegel, A., Termeer, C. J., Mathijs, E., De Mey, Y., et al. (2019). A framework to assess the resilience of farming systems. Agric. Syst. 176:102656. doi: 10.1016/j.agsy.2019.102656
Ming, H., Zhu, Z., and Li, X. (2024). Can e-commerce in rural areas enhance the resilience of the agricultural economy? An empirical study on the comprehensive demonstration policy of e-commerce in rural areas. World Agric. 2, 85–98. doi: 10.13856/j.cn11-1097/s.2024.02.008
Mishra, A., Bruno, E., and Zilberman, D. (2021). Compound natural and human disasters: managing drought and COVID-19 to sustain global agriculture and food sectors. Sci. Total Environ. 754:142210. doi: 10.1016/j.scitotenv.2020.142210
Modica, G., Pulvirenti, A., Spina, D., Bracco, S., D'Amico, M., and Di Vita, G. (2024). Clustering olive oil Mills through a spatial and economic GIS-based approach. Clean. Environ. Syst. 14:100207. doi: 10.1016/j.cesys.2024.100207
Pamphile, K. D. (2012). Transaction costs in the trading system of cashew nuts in the north of Benin: a field study. Am. J. Econ. Sociol. 2, 277–296. doi: 10.1111/j.1536-7150.2012.00830.x
Singh, N., Kumar, A., and Dey, K. (2023). Unlocking the potential of knowledge economy for rural resilience: the role of digital platforms. J. Rural. Stud. 104:103164. doi: 10.1016/j.jrurstud.2023.103164
Tilman, D., Balzer, C., Hill, J., and Befort, B. L. (2011). Global food demand and the sustainable intensification of agriculture. Proc. Natl. Acad. Sci. 108, 20260–20264. doi: 10.1073/pnas.1116437108
Tittonell, P. (2020). Assessing resilience and adaptability in agroecological transitions. Agric. Syst. 184:102862. doi: 10.1016/j.agsy.2020.102862
van der Lee, J., Kangogo, D., Gülzari, Ş. Ö., Dentoni, D., Oosting, S., Bijman, J., et al. (2022). Theoretical positions and approaches to resilience assessment in farming systems: a review. Agron. Sustain. Dev. 42:27. doi: 10.1007/s13593-022-00755-x
Verspagen, B., and Schoenmakers, W. (2004). The spatial dimension of patenting by multinational firms in Europe. J. Econ. Geogr. 4, 23–42. doi: 10.1093/jeg/4.1.23
Volkov, A., Žičkienė, A., Morkunas, M., Baležentis, T., Ribašauskienė, E., and Streimikiene, D. (2021). A multi-criteria approach for assessing the economic resilience of agriculture: the case of Lithuania. Sustain. For. 13:2370. doi: 10.3390/su13042370
Yan, H., Wei, X., Qi, Y., and Zhang, M. (2022). Does agricultural production agglomeration improve environmental efficiency? An analysis based on the regulatory effect of environmental regulation. Agric. Econ. Manag. 6, 26–37.
Yao, R., Ma, Z., Wu, H., and Xie, Y. (2024). Mechanism and measurement of the effects of industrial agglomeration on agricultural economic resilience. Agric. Basel 14:337. doi: 10.3390/agriculture14030337
Ye, Y., Zou, P., Zhang, W., Liu, X., Liu, B., and Kang, X. (2022). Spatial-temporal evolution characteristics of agricultural economic resilience: evidence from Jiangxi Province, China. Agronomy Basel 12:3144. doi: 10.3390/agronomy12123144
Zhang, H., Li, J., and Li, Y. (2022). Agglomeration of productive services and urban green economic efficiency: An empirical test based on the dynamic spatial Durbin model and threshold model. East China Econ. Manag. 36, 75–86. doi: 10.19629/j.cnki.34-1014/f.220517004
Zhao, G., and Zhang, X. (2022). Can urbanization break through the “HuHuanyong line”? Empirical test based on panel data of provinces in China from 2005 to 2020. China Soft Sci. 12, 89–101.
Zhao, Y., and Zhao, J. (2020). Study on the geographical agglomeration trend of grain planting industry in my country: measurement analysis, spatiotemporal characteristics and development strategies. Rural Econ. 7, 86–93.
Zhao, W., Zhao, T., and Ma, J. (2023). Digital inclusive finance, rural industrial integration and agricultural economic resilience. J. Agric. Forest. Econ. Manag. 22, 555–565. doi: 10.16195/j.cnki.cn36-1328/f.2023.05.58
Zhao, D., and Zhou, H. (2020). Agricultural production agglomeration: how to improve food production efficiency? A re-examination based on different development paths. Agric. Technol. Econ. 8, 13–28. doi: 10.13246/j.cnki.jae.2020.08.002
Keywords: agricultural economic resilience, agricultural production agglomeration, agricultural technological innovation, spatial spillover, threshold effect
Citation: Ranran C and Jingsuo L (2024) The impact of agricultural production agglomeration on agricultural economic resilience: based on spatial spillover and threshold effect test. Front. Sustain. Food Syst. 8:1464732. doi: 10.3389/fsufs.2024.1464732
Edited by:
Juan Lu, Nanjing Agricultural University, ChinaReviewed by:
Daniela Spina, University of Catania, ItalyZongyao Yang, Nanjing Agricultural University, China
Copyright © 2024 Ranran and Jingsuo. This is an open-access article distributed under the terms of the Creative Commons Attribution License (CC BY). The use, distribution or reproduction in other forums is permitted, provided the original author(s) and the copyright owner(s) are credited and that the original publication in this journal is cited, in accordance with accepted academic practice. No use, distribution or reproduction is permitted which does not comply with these terms.
*Correspondence: Li Jingsuo, bGlqaW5nc3VvMjAwMUAxNjMuY29t