- 1Department of Management of Complex Systems, School of Engineering, University of California-Merced, Merced, CA, United States
- 2Department of Chemical and Materials Engineering, School of Engineering, University of California-Merced, Merced, CA, United States
Digital agriculture supports farmers’ decision making to improve productivity and profitability. However, adoption of digital technology is uneven. Through interviews with 21 medium acreage almond growers and crop consultants in California’s Central Valley, we examine barriers to adoption through the lens of technology acceptance models, Technology Acceptance Model (TAM-3) and Unified Theory of Acceptance and Use of Technology (UTAUT-2). Not surprisingly, farmers are willing to adopt technology when profitability and ease of use are shown, with economic returns (either anticipated or demonstrated) from the technology investment serving as the primary factor influencing adoption. Trust operates as a moderating factor to the desire for economic returns that influences adoption. There may be trust, or lack of trust, in technology performance or in the advisors who recommend it. Producer trust is affected by expectations of technology relevance and usefulness, and it is influenced by prior experience. Concerns about data management (e.g., governance, quality, privacy, security) take a back seat to more practical issues such as profitability, leaving producers in an imbalanced position with tech companies who have an interest in their agricultural data. We assert that producer acceptance of data management practices (despite their uncertainty in how to utilize the data being generated) implies that there is a basic level of trust in tech companies’ data management practices that is consistent with models of moralistic trust behaviors for precision ag adoption. Our findings contribute to the growing research on digital agriculture that debates the benefits and downsides of digital agriculture.
1 Introduction
Digital agriculture enabled by the Internet of Things (IoT) is transformative technology that empowers farmers to make data-informed decisions to optimize resource allocation, reduce waste, and enhance productivity. IoT technology refers to networks of sensors and digital devices that autonomously connect and share data, with minimal human prompting (Farooq et al., 2019; Kagan et al., 2022; Liu et al., 2021). As shown in Figure 1, IoT technologies include sensors, robotics, and unmanned aircraft that interface to detect water and nutrient content in row or orchard crops. Sensors attached to plants transmit data that is collected by unmanned aircraft and transmitted through communications networks, typically in rural areas. The development of these systems involves multiple points for data management which warrants closer examination whether agricultural producers are willing to give up management of data in exchange for improved nutrient detection that provides the potential for improved profitability.
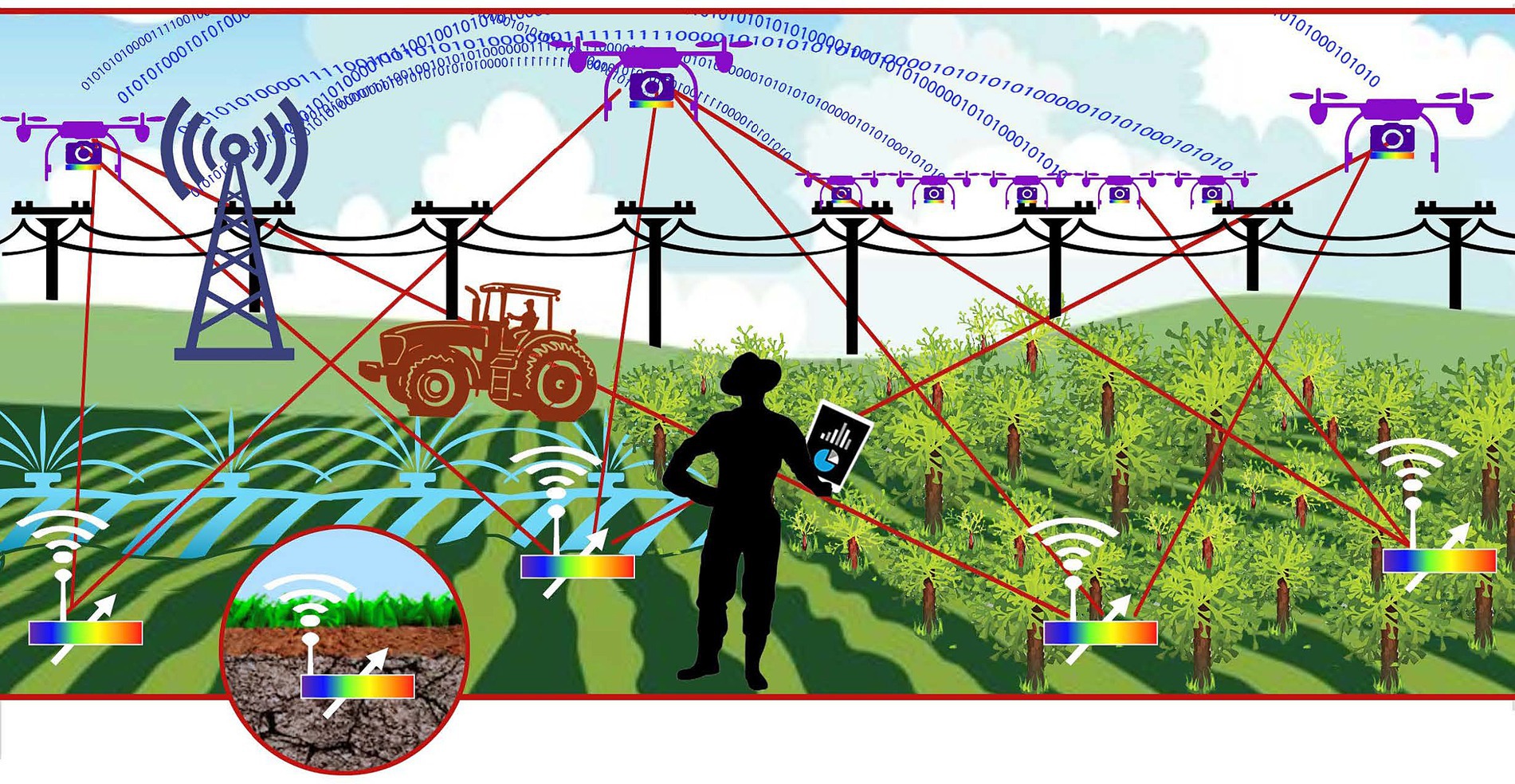
Figure 1. Internet of Things (IoT) agricultural system with sensors, robotics, and unmanned aircraft transmitting data viewable by a farmer on a tablet. Reprinted with permission from the IoT4Ag Gen-4 NSF ERC (https://iot4ag.us) © IoT4Ag ERC.
IoT technologies involve ubiquitous sensing, autonomous farming, and big data. These are extensions of digital agriculture, which utilizes tools that collect and analyze data from agricultural applications that may extend beyond the farmgate and throughout the entire agricultural value chain (Shepherd et al., 2018). Digital agriculture encompasses precision agriculture technology that enables farmers to modify input (e.g., water and nitrogen) applications (Osrof et al., 2023). In other examples of digital agriculture, Agribots, for example, have taken to seed mapping, weed mapping, and micro spraying throughout various countries (Revise as Reddy, 2016).
Despite its potential benefits, the adoption of digital agriculture is uneven (Osrof et al., 2023). Larger farms are most likely to realize economic benefits of automation through reduced labor costs and can spread the fixed costs of technology investment across a larger acreage (Basso and Antle, 2020). The economic advantage provided by digital agriculture may thereby result in large farms becoming larger and farm consolidation, a phenomenon that has been documented in the 2022 Census of Agriculture (USDA, 2024).
Data managed as a commodity is of interest to companies throughout the supply chain. Some farmers have begun to view data as an asset to be managed like other farm assets (Wiseman and Sanderson, 2019; Wysel et al., 2021) that can be shared or sold. As more technology solutions are developed and deployed in agriculture, farming dynamics continue to change, often placing farmers in a subordinate relationship to technology companies (Neubauer, 2021). Some studies have indicated that farmers using ag data software products and sharing data with outside consultants are significantly more likely to make data-informed management decisions, with these farmers also more likely to regard their decisions as yield-enhancing (DeLay et al., 2020). However, other authors (Gardezi and Stock, 2021) have expressed concern that farmers are adopting digital agriculture to be morally complicit, in other words to be viewed as “good farmers” that contribute to food security; meanwhile, agtech firms have successfully positioned their knowledge products as superior to farmers’ experiential knowledge, thereby perpetuating farmers’ sustained engagement with digital agriculture technologies for the purposes of data capture and corporate capital accumulation.
Without understanding barriers to adopting digital agriculture technology, there is strong potential to perpetuate imbalances between farmers and tech companies, and between large and small farmers, as digital agriculture and IoT technology expand (Soma and Nuckchady, 2021). Faik et al. (2024) note that poverty limits the affordability of digital technologies for segments of the population, and that small farming operations may have insufficient means to acquire digital technology. Furthermore, there is great importance to engage small farmers in technology adoption, due to the high prevalence of small farmers. According to Lowder et al. (2021), farms of less than two hectares comprise 84% of all farms, though they operate 12% of all agricultural land.
We contribute to the literature by assessing user adoption behavior and barriers to digital agriculture technology through 21 qualitative interviews with medium sized almond growers and technology consultants in California’s Central Valley, and by identifying potential strategies to increase adoption. Utilizing abductive reasoning (Dubois and Gadde, 2002, 2014) to cycle between theory and our data, we infer the best explanation for this reluctance to adopt new technologies. Through this iterative analysis of our interview data, we apply the Technology Acceptance Model (TAM-3) (Venkatesh and Bala, 2008) and the Unified Theory of Acceptance and Use of Technology (UTAUT-2) (Venkatesh et al., 2012) to frame our findings. Our results indicate that economic profitability is the foremost factor influencing producers’ decisions to adopt technology. Trust in technology performance, and in those who recommend it, is a moderating factor that either facilitates or hinders adoption. de Vries et al. (2023) identified a need for nuanced exploration of how trust influences the adoption of digital technologies in the agri-food value chain, and identified three themes. Our work contributes to the theme ‘trust and digitalization,’ largely through the lens of production-focused technologies. Interestingly, concerns about data management logistics are secondary to profitability, aligning with Gardezi and Stock’s (2021) notion of moralistic trust in corporate entities, who are perceived as inherently trustworthy in their data management practices. However, this finding contrasts with the work of other authors (Fielke et al., 2022; Jakku et al., 2019; Wiseman et al., 2019).
Next, we provide a literature review on the TAM-3 and UTAUT-2 models and the literature on digital data management practices. This is followed by a description of the methodology, discussion, and conclusions.
2 Literature review
TAM-3 and UTAUT-2 are based upon the widely accepted Technology Acceptance Model (TAM) for examining barriers to adopting computers, first introduced by Davis (1989), which is an adaptation of Ajzen’s (1991) Theory of Planned Behavior that behavior follows intention. Davis’s TAM found that perceived usefulness (PU) and perceived ease of use (PEOU) were both critical to technology adoption. The TAM has been used in other agricultural tech adoption studies to examine the willingness to adopt a nutrient management plan in Ireland (McCormack et al., 2021), precision agriculture adoption in Canada (Aubert et al., 2012), technology adoption in Indian agricultural industries (Kumari et al., 2018), unmanned aerial vehicles in Turkey (Parmaksiz and Cinar, 2023), agricultural e-commerce in Iran (Zarei et al., 2022) and larger technology systems or bundles such as integrated pest control and smart farming (Rezaei et al., 2020; Silva et al., 2017; Tubtiang and Pipatpanuvittaya, 2015). However, though there is no evidence of the use of TAM to evaluate barriers to adopting digital agriculture technology in the United States, it is often used to explain adoption and use of technology service bundles in general (Schilke and Wirtz, 2012). The TAM-3 and UTAUT-2 models advance the TAM model by incorporating new variables and by considering evolving technology and user behaviors like perceived enjoyment, social influence (from peers, social networks, and social media), and external variables such as trust, subjective norms, and facilitating conditions. The UTAUT-2 introduces several new constructs such as performance expectancy, effort expectancy, social influence, and facilitating conditions. These factors help in understanding the influence of external factors, social norms, and perceived usefulness on technology adoption. The UTAUT-2 also considers moderating variables like gender, age, experience, and voluntary use, which can influence the relationship between the determinants and actual technology usage. The UTAUT-2 also acknowledges the importance of context in technology adoption, considering organizational, cultural, and situational factors.
Shi et al. (2022) use the UTAUT-2 model to examine the contributing factors that make Bangladesh farmers willing to pay for and to adopt IoT. They find that a government developed IoT infrastructure, and subsidies are critical facilitating conditions to promulgate adoption and for creating an affordable system that will ensure commodity price competitiveness.
Using the TAM-3 and UTAUT-2 frameworks, we identify new themes to consider for targeted intervention and strategies to promote the adoption of digital agriculture and how the chasm between early adoption and late adoption can be bridged. Late adopters often cannot access new services or processes (Woodcock, 2014), which in the case of small farmers will leave them further economically disadvantaged, possibly leading to a downward spiral of being unable to catch up with technology adopters and large farms, becoming further marginalized and non-competitive. Not surprisingly, our study finds that the adoption of technology is highly dependent on whether it increases farm profitability. However, unlike earlier findings on trust in digital technology companies (e.g., Fielke et al., 2022; Jakku et al., 2019; Wiseman et al., 2019), concerns about data sharing with these companies seem to be of lesser importance to our participants, leaving farmers in a vulnerable position regarding data management.
Digital agriculture has the potential to increase productivity in a transformative way, and hence, to improve profitability, while reducing environmental impact. Weersink et al. (2018) note that agricultural practices have been slow to change, and although there is greater data generated, the cost savings and improvements to profitability are demonstratable on a case-by-case basis:
“But despite these obvious benefits, it must be noted that the value of the additional information provided from precision agriculture relative to its cost is another likely barrier hindering its widespread adoption. In particular, the history of how farmers use technologies such as yield monitors and variable application rate fertilizers suggests a relatively flat payoff function for these technologies that in many cases means there is no real financial incentive for farmers to invest. Hence, the existing evidence points to extremely varied uptake.” (Weersink et al., 2018, p. 23)
In a survey of European farmers, Barnes et al. (2019) found that high costs of purchase, along with small farm size are the top two reasons for non-adoption of precision ag technology. Lack of information, low return on investment, and farmer age rounded out the top reasons for non-adoption. Similarly, the authors found that decreased production costs were the top reason for adopting technology.
Lowenberg-DeBoer and Erickson (2019) note that some aspects of precision agriculture technologies like Global Navigation Satellite Systems have been adopted rapidly, while other technologies, such as variable rate technology (VRT) application have proven to be slower to being adopted, due to uncertainties in achieving financial payback. DeLay et al. (2022) found that profitability and technical efficiency both increase with technology bundling of complementary products. Bundling examples include yield monitors and grid soil sampling; and, aerial imagery, hand-held GPS devices, and soil survey maps.
In a copious literature review of digital agriculture from a social science lens, Klerkx et al. (2019) indicate a need to prioritize tech adoption research on data ownership, privacy and ethics in digitalizing agricultural production systems. As van der Burg et al. (2021) point out, there are several studies, implemented through surveys and interviews, that indicate farmers are hesitant to share their data through lack of trust. Among these, Wiseman et al. (2019) surveyed 1,000 agricultural operations in Australia, finding that a lack of understanding about how data will be used is among farmers’ chief hesitancies about sharing data, despite the widespread adoption of digital technology in animal agriculture contexts, like dairy milking stations. Wiseman et al. (2019) also indicate that farmers are uncomfortable with the sale of data extracted from digital ag technology for profit. In interviews with 26 grain farmers and agricultural industry stakeholders, Jakku et al. (2019) note that trust over how data will be used is a central concern, which creates skepticism of the value of digital technologies.
Trust has been cited as a key factor in technology adoption (McKnight et al., 2002, 2011; McKnight and Chervany, 2006). TAM and UTAUT, while useful models, do not capture the role of trust in predicting adoption behaviors (Gefen et al., 2003; McKnight et al., 2002). Strategic trust is characterized by beliefs in the trustworthiness of technology (or people) and beliefs based on experience (Gardezi and Stock, 2021). The traditional strategic trust concepts – general trust, context trust, and specific trust – apply equally to technologies as they do for personal and institutional trust environments (McKnight et al., 2002). Where technology is concerned, general trust reflects an individual’s personal propensity to trust technology. Context trust is tied to expectations of relevance or usefulness of a class of technology, and specific trust is anchored in experience with a specific technology (McKnight et al., 2011). Early adopters typically have high levels of general trust while late adopters and laggards come with low levels of general trust, leading to greater reliance on expectations and experience to build other forms of strategic trust.
Moralistic trust, unlike strategic trust, does not rely on rational assessment. Rather, it is rooted in a societal or community culture (Gardezi and Stock, 2021). To be perceived as responsible members of their community, farmers may choose to adopt tools and techniques used by their peers, trusting that all involved parties (vendors, government, customers, etc.) are collectively working for the common good. For instance, digital agriculture technologies have been used to enhance consumer-grower trust by allowing growers to provide more transparency and traceability in their operations, signaling good farming practices to their customers (Finlay-Smits et al., 2023).
Our study finds that farmers prioritize expected gains in profitability as the leading motivation for adopting digital agriculture. Trust in technology and in those who recommend it serves as a moderating factor to whether digital technology will be adopted. Our study is also the first to frame digital ag adoption with almond growers. Most technology studies have been conducted with row crops, though a handful of studies have been implemented with apple orchards and the olive sector. Gallardo and Sauer (2018) examined technology adoption to address the need for labor saving technologies in agriculture with apple orchards. Gallardo and Brady (2015) looked at barriers to adopting platforms during harvesting, in lieu of ladders, that would improve farm worker safety and labor productivity in apple orchards. In contrast to Gallardo and Brady (2015), our study focuses on digital technology, rather than mechanization. Parra-López et al. (2024) implemented a semi-quantitative multicriteria framework to assess the impact that technological innovation systems have in facilitating digital transformation in the olive sector in Andalusia, Spain. The authors note that, “As data becomes a valuable asset in agriculture, safeguarding the rights of all stakeholders to their data ensures not only ethical practice, but also trust in the digital transition.” (p. 12). Our results are consistent with the findings of Parra-López et al. (2024). Next, we discuss study methodology, findings, discussion, and conclusions.
3 Methodology
Our investigation was approved by the University of California Merced Institutional Review Board (Study # UCM 2021-115). We developed a semi-structured interview protocol to evaluate six main topics: cost, technological compatibility, IT Infrastructure, data management, data privacy, and cybersecurity with an average of three questions per topic.
Using cognitive framing as our analytical lens, our interview questions probed experiences with agriculture technology adoption. We included prompts related to data management given that prior studies have indicated data privacy may be an issue for growers (Jakku et al., 2019; Wiseman et al., 2019). We developed 3 background questions at the beginning and three closing questions at the end of interviews to ensure participants had the opportunity to share any experiences during our conversation.
Three focus groups with eight participants were conducted to validate and test the protocol. Employing snowball sampling (Parker et al., 2019), from January through August 2022, we conducted semi-structured interviews with eleven almond producers from small and mid-sized family farms1 and ten industry professionals representing a mix of ag technology companies and farm advisors, all of whom had experience assisting producers with the implementation of digital ag technology. All subjects were from Central California.
Our audio recorded interviews each lasted approximately one hour and were later transcribed. During the interviews, we focused on listening to what growers said about barriers with no pre-conceived framework for analysis, consistent with grounded theory methodology (Glaser and Strauss, 2017).
Applying abductive reasoning (Dubois and Gadde, 2002, 2014) to the generally held observation that there is low adoption of new technology in agriculture, we use our interview data to infer the best explanation for this reluctance. After familiarizing ourselves with the data, we determined that a framework based on TAM would be appropriate, leading to the development of our initial NVivo codebook based on TAM-3. While TAM-2 and TAM-3 are both extensions of the original TAM model, we selected TAM-3 because it can be used to identify adoption barriers as well as potential interventions (Venkatesh and Bala, 2008). Interviews were coded in NVivo software by the lead author and research assistant, who collaboratively drafted a coding scheme that was inclusive of initial insights and reconciled their results for internal consistency and reproducibility. Each coder separately coded the same five interviews, then discussed, revised, and added code themes, as appropriate. For the rest of the interviews, the research assistant coded the remaining interviews with the lead author suggesting changes to codes and coding. After this first round of coding, the lead author undertook repeated rounds of coding, categorizing, and comparing. In addition, both authors discussed the coded interview quotes to further refine code definitions and identify core themes —a process that led to the inclusion of price value from UTAUT-2 and the development of a more holistic, hierarchical framework for interpreting our results. Through this iterative process, trust emerged as an important factor in the adoption decision.
We now describe the in-depth qualitative insights that we theorized to develop our conceptual model. The letter-number pairs at the end of each interview quotes indicated the interviewee who made those comments, with G indicating a grower and C indicating a consultant; select demographic characteristics of the interviewees are found in Table 1.
4 Results
In our initial analysis, we identified a conceptual model that identified expectations, experience, and, most importantly, economics, as the factors influencing adoption decisions, just as earlier research would have us expect. However, while no one research subject specifically mentioned it, trust emerged as a construct worth deeper investigation. After another pass at the data, coding for trust, we saw evidence that trust could moderate the relationship between rational economic arguments to adopt and adoption – if a grower does not trust that a new technology will perform as promised, then they will be less willing to invest in that technology.
We engaged in an exploratory correlation cluster analysis using NVivo’s built-in functionality. As coding data are binary, we used the Jaccard coefficient to examine the relationship between codes. We found that a stable core of five clusters emerged, as presented in Figure 2A. When combined with our initial conceptual model, we interpreted the clusters with the lens of our initial conceptual model (Figure 2B). Using this interpretation, we refined our conceptual model, providing nuance to the important expectations, experiences, and economic factors that guide adoption decisions, as presented in Figure 3. Below we discuss these constructs with quotes from our data.
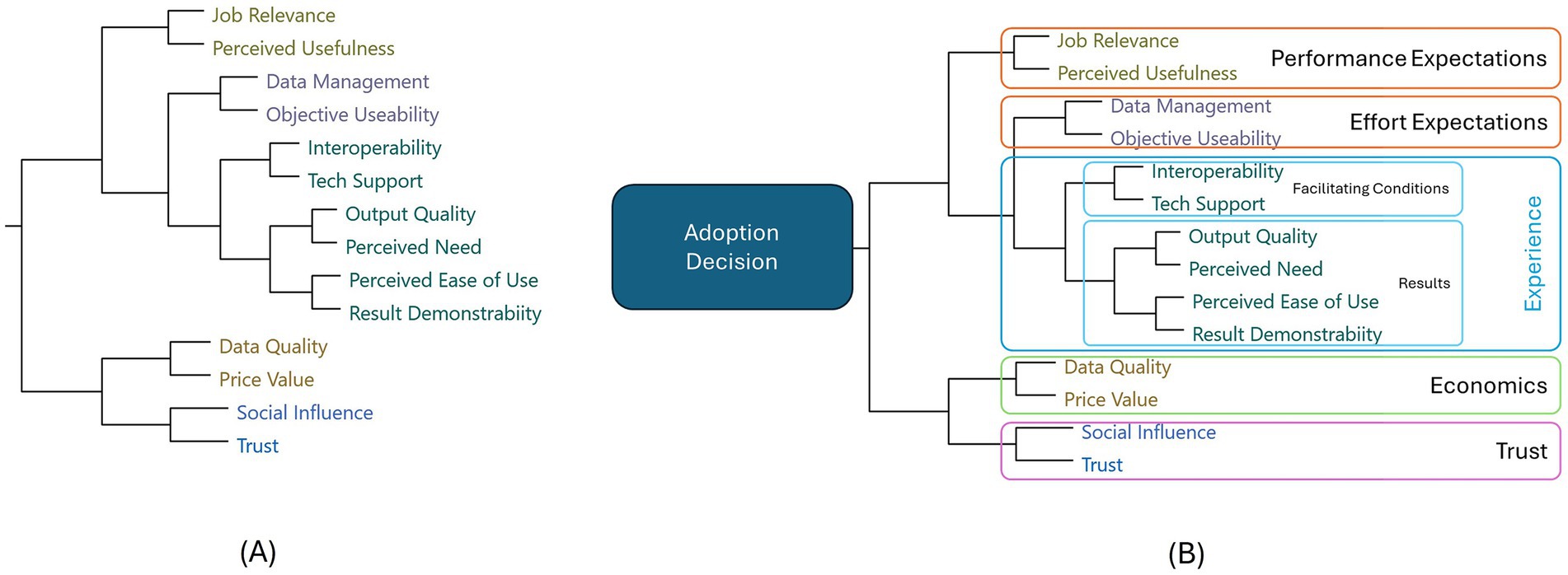
Figure 2. Code clusters determined by NVivo using Jaccard coefficient (A), and interpreted through the lens of our initial conceptual model (B).
Economics, expectations, and experience form the foundational concerns when making a new technology adoption decision. Expectations are set, which are then influenced by past experiences both directly and indirectly with agricultural technology implementations. Our conceptual model (Figure 3) depicts how these types of frames – economics, expectations, and experiences shape the adoption of new agriculture technology in tree crops. An important element that emerged from the data is the role of trust in adoption. We found comments in many interviews that speak to how much trust growers have, or can have, that the technology will deliver the promised value. We interpret trust as a moderator, facilitating or hindering the effect of economic arguments to adopt.
The model presented in Figure 3 shows the interactions between core concepts. However, the relationships between these concepts are dynamic. As growers gain experience with technology, they update both expectations and trust.
As discussed in the literature review and elsewhere (Marra et al., 2003), if the economic value of a technology is there, then growers are interested in adopting it. However, the economics of a technology have a heavy dependence on the size of the farming operation and the pricing model (subscription/lease versus ownership). Data quality directly impacts assessments of the economic value as well, although other data management concerns (e.g., governance, security, and privacy) do not appear to be directly linked to the economic dimension in technology adoption; rather, these aspects of data management appear to be more closely associated with effort and experience assessments (see Table 2).
4.1 Functionality
Broadly speaking, two groups of factors influence the beliefs about the functionality of a new technology: performance and effort expectations, and direct and indirect experiences with the technology.
[…] every technology that has succeeded on this farm has made someone's life easier, right? And more importantly, the active user right to his point, right. The ones I've tried to do that don't work, make it say better for me, but harder on the employees, right? And it's more work for them and those just never seem to stick. (G9)
4.1.1 Expectations
When considering new technology, its expected performance – its relevance and usefulness in addressing the problem – is critical; the economic argument cannot begin to be made until there is evidence that the technology will work for the operation. However, we often saw complaints about the relevance or usefulness of data that are provided by sensor systems.
When performance expectations are positive, the effort expected to achieve them is assessed.
Well. We try not to, we try not to invest in things that we think there's that steep learning curve (G10)
The effort required to understand the data is as critical to adoption decisions as the effort expended in collecting it. It’s not surprising to hear that if the data cannot be easily used to support decision making, then it is of little value to farmers. High quality data at low effort makes the economic argument for adoption stronger (see Table 3).
Our data demonstrate that data management and the objective useability are primary dimensions of effort considered by our participants. Our participants' current approaches to data management center around Excel (spreadsheets) and paper documents for farm management and decision making. a spreadsheet is probably as good as it gets. If you can, we will, we, we will help them put in this Excel spreadsheet. (C5) we track like yields and stuff like that … Just [in] Excel, on paper that type of stuff very basic just to get. Yeah. So Excel is a big one. (G4)
Issues with data input through other tools (e.g., integrating with an accounting system, gathering data for a [new] tool), increase the effort and decrease useability. It’s unsurprising that where farmers expect adoption to require high effort, they are less likely to move forward with adoption.
4.1.2 Experience
Experience breaks into two clusters—facilitating conditions and results. Facilitating conditions are situations that need to be in place to support successful implementation of a new technology.
I think there's not a problem in adopting precision ag as long as it's been proven to actually, yeah, well, I guess the problem is, can it be proven to make people money? (C9)
Past experiences with lack of interoperability and poor access to tech support have negatively affected farmers—without these critical facilitating conditions, the risks posed by a new technology are often assessed as too high. Tech support takes many forms – setting up new systems, updating hardware and software, facilitating self-repair options, and, critically, fast response to replace or repair equipment especially during critical growing operations. Given the remote settings for equipment deployment, response time is always an issue if something malfunctions in the field (see Table 4).
When it comes to results, past experiences—such as beta testing, personal adoption, or observing others’ adoption—play a key role in evaluating new technologies. These technologies are assessed based on the quality of output, necessity, ease of use, and demonstration of results, particularly regarding how well they have supported improved decisions or operational outcomes on the farm. This evaluation also includes comparing the performance of existing systems and technologies already in place to address the business problem (see Table 5).
4.2 Trust
McKnight et al. (2002) argue that trust is an essential element in adoption, and that the traditional strategic trust concepts – general trust, context trust, and specific trust – apply equally to technologies as they do for personal and institutional trust environments. Strategic trust is characterized by beliefs in the trustworthiness of technology (or people), and beliefs based on experience (Gardezi and Stock, 2021). We find evidence supporting the need for strategic trust in both people and technology.
…consistent, repeatable success is a big thing… (G4)
General trust in technology is characterized as a willingness to depend on it across a spectrum of situations, the assumption that technology is usually consistent, reliable, and functional, and that it provides better outcomes when it does meet these assumptions (McKnight et al., 2002). This general disposition to trust is considered an individual characteristic and, while we may be able to infer some participants’ general trusting beliefs from their comments, we did not directly examine this in our conversations. However, consultants seemed to indicate that growers do not have very high levels of general trust in technology. For emerging technology, promises have been broken in the past – results did not match expectations or companies disappeared – so there is greater proof needed to overcome low levels of general trust (see Table 6).
Unlike general trust, context and specific trust are rooted in expectations and experience with focal technologies. Context trust reflects the expectations of relevance and usefulness of a class of technology – for example, that irrigation technology provides a useful solution to a relevant challenge faced by growers. Our interviews uncovered many examples of technologies evaluated as having job relevance and reliable results; in these cases, there were favorable adoption attitudes.
On the other hand, we also found cases where the growers felt the results did not live up to the industry hype; instead, they could do as well with existing, established practices.
We already bought the weeder and [Partner] went to Italy and we got we sold it already. There you go. And it made so much sense, right? [Partner] went to Italy. You got to get the planter to match up with the weeder, so you get the auto planner and then you're going to get the weeder and everything's going to marry up and we're going to save a ton of money and labor's hard to find, you know, so let's do it and we did it and the weeder couldn't weed. (G5)
Relationships are at the heart of building specific trust. Where a grower may trust irrigation technology, their trust in a specific irrigation solution will depend on experience with the product, its vendor, and even a specific salesperson. Further, this type of trust can be influenced by other trusted sources of information – peers and extension agents, for example.
Our sales are because of neighbors talking to other neighbors, to other farmers, and letting they know “hey if you got a, a problem call these guys. They work weekends, they work nights, and they will get you going,” because farming, when it’s go time, it’s go time. There is no stopping. (C10)
Moralistic trust, unlike strategic trust, does not rely on rational assessment, but rather is rooted in a societal or community culture (Gardezi and Stock, 2021). Social influence is described as “…the extent to which consumers perceive that important others believe they should use a particular technology” (Venkatesh et al., 2012, p. 159). This influence can be critical in forming generalized moralistic trust. To be perceived as responsible members of their community, farmers may choose to adopt tools and techniques used by their peers, trusting that all involved parties (vendors, government, customers, etc.) are collectively working for the common good. For example, in California, being seen as a good water steward is socially important. This social pressure can strongly motivate growers to adopt irrigation technologies, even if they have concerns about data management (e.g., privacy, sovereignty) or the reliability of a specific product.
So whether it's, you know, our employees or our equipment or all the above, we, you know, our fertility and we, our water usage, we all, are under a microscope right to be able to do the right thing. And sustainability is doing the right thing so we can continue to pass it on to the next generation and continue to be profitable and all the things we've done. (G5)
This aspect of moralistic trust, where the adoption of technology is seen as an ethical commitment to sustainable practices, was particularly evident in discussions around the use of irrigation technology, which has seen widespread adoption among the growers in our sample.
Determining how to use our water and the most efficient way is becoming a real big, big issue. Aerial imaging. Various methods of measuring soil moisture and interpreting what they mean. That's becoming more and more critical, we’re, we're just short of water everywhere and in the West right now, but California particularly, so we're trying to learn how to farm with less water. (G3)
Moreover, when discussing the potential for chemical application technology, growers highlighted its value but also noted that current solutions are too costly for their operations. This contrast between the adoption of irrigation technology and the hesitation around chemical application technology further underscores the nuanced role that moralistic trust plays in technology adoption decisions.
As far as like the GPS driven variable rate application type stuff. That stuff is not applicable to me because I have too small of blocks and it wouldn’t make sense. Like it’s too small. Why fertilize this area different than this area? It’s, it’s small like, put it all the same. You know what I mean? So, I couldn’t justify getting a specialized piece of equipment that's gonna change the amount of fertilizer I put in one area of the field versus another one. (G1)
They came up with a machine called GUSS and it was there at the Almond Conference, GUSS. It is a completely robotic self-driving sprayer, but they built this sprayer in a way that it covers like, they know exactly what it takes to spray an orchard, right? … So a thousand acre guy could probably buy one of these GUSS sprayers and justify more easily. (G1)
4.3 Data governance, privacy, and security
Not represented in our model (Figure 3) are worries about data management (e.g., governance, privacy, security). While these issues did arise, they took a back seat to the more practical concerns, leaving producers in an imbalanced position with tech companies.
So again, with like the soil moisture sensors, I forgot to mention one part of the grant was that they would monitor how much water you're using per year for a year or two. So, the only issue with that that we talked about was if they use that data against us and say that we’re using too much water. Which we're like, we're really not using that much water that is needed. Like, that's the part that we worry about. We really don't care if someone knows how much you're doing about. (G7)
The general sentiment was that the types of on-farm data collected would be of little value to competitors.
No one's no one's going after [Product] because they know exactly what I know, which is nobody cares about your GPS maps. You know, there's no value in that to someone, you know, if they if they want to know, you know, anything of value, they want to know subscribers’ private information. I guess email addresses might be valuable, but even then, like. (G10)
This doesn’t interest me! I’m not interested. Like you know do I like the fact of people taking the data and doing stuff with it? No. But is it a big decision-making thing for me? No, it doesn’t drive my decision making. (G1)
Few growers seemed to have considered the question of data ownership. Among those who had given the issue some thought, they believed that the data belonged to them but assumed that the vendor would use the data solely to better serve their needs.
And you think even if they [Product Vendor] owned that data, it would probably be working on your advantage […] I mean, realistically, it should be, you know, it should be my data, and it shouldn’t be anybody else’s unless I give permission for it to be used. Right? (G2)
5 Discussion
The barriers and challenges to adoption for our interviewees clearly align with standard findings of technology acceptance research, with the strongest indicators of early adoption lying in economics, expectations, and experience. Unfortunately, concerns in effort expectancy and facilitating conditions tend to outnumber the expected benefits of new technology which, when combined with low levels of experience with technology in our subject pool, largely explains low adoption rates even where a technology has proven performance benefits.
So, I mean, I mean, we've been on the cutting edge of a lot of things over the years past and that cost you a lot of money, to be honest. (G5)
Perceived ease of use and impacts on profitability are among the most cited concerns for acceptance of digital technology. Since ag tech adoption is generally low among their peers, there is no pressure to adopt; social influence is more likely to reduce adoption behavior in our sample.
Early adopters showed signs they were willing to experiment, and they were comfortable with less refined solutions. In our sample, late adopters tended to be older farmers and there is evidence of some regret for waiting as they realized the lost opportunities.
I never had a customer come back to me when they decided to move forward with, eh, [Product], and say I regret buying this. Every time is “why didn’t I buy this five or ten years ago?” And the, their biggest regret is why didn’t they do it sooner. (C10)
Unfortunately, in some cases it is the lack of supporting infrastructure that restricts the pace of adoption.
[T] he RTK I mentioned, right, you need to connect to a cell network to be able to do that, in order to get your corrections, you might be in some area where there just isn’t, you know, isn’t into a lot of cell infrastructure or there are [sic] not very solid cell infrastructure. (C6)
Digital agriculture adoption among small-to medium-sized family growers is distinctly shaped by economics, particularly the challenge of large up-front investments required for advanced technology. Unlike large-scale growers with thousands of acres to allocate the investment across, small farms face significantly higher per-acre costs.
…for me to get harvest equipment I would probably have to spend four hundred thousand dollars. For four hundred thousand dollars stretched across 55 acres is a very high dollar per acre amount right? Now if I had a thousand acres it makes sense to have your own harvest equipment because it’s four hundred thousand dollars spread over a thousand acres instead of fifty-five. So the scale is very very important here. You’re going to find that the smaller the farmer the more difficult it is to adopt the high tech kind of stuff. You know? (G1)
This challenge extends beyond operational functions to essential business support services such as accounting and billing, where it only becomes cost-effective to invest once the volume of transactions reaches a certain threshold.
I'm sure if you're a big [grower] they farm 50,000 acres. I'm sure they have lots of technology in regards to accounting, right? For, for sending bills. I'm sure, you know, every guy's got a tablet and they have a budget and they have to. I know they log their time on their digital device right where we haven't gone there yet. We haven't needed to. We're probably going to get to that point if we're getting bigger, right? But you know, so there are certain thresholds in any size for certain technologies that fit. Yeah. (G9)
One strategy to mitigate this barrier is outsourcing, which allows small farms to access sophisticated technology without bearing the full cost. However, this approach has its limitations, as it may not always be possible to find service providers equipped with the latest technology, leaving these growers at a competitive disadvantage. Consequently, while outsourcing offers a partial solution, it often does not fully address the technology adoption disparity between small and large growers.
I would love to be able to implement on my farm, like, lower dust technology for harvest. But again, I’m small right, and I’m hiring all this stuff out. And I don’t know of any local service providers who have that type of technology, and if I did, I would consider having them come do mine. (G1)
Another advantage that enables large-scale growers to implement new technologies more quickly is their ability to hire full-time information technology staff to support users and manage systems.
…it goes back to you asking about the IT guy on staff, something I feel like just maybe I live in a bubble. But from my experience, that type of deal only works on like a big scale operation. Because when you're working with like, say, two to like ten guys, all of those guys have tasks that need to do, and most of the time it's not [IT]. (G4)
It matters how big you are. Oh yeah, I got my 10000 acre square is probably usually have a tech guy. On the 200 acre guys, probably not. Five acre guys definitely not. Yeah, I think it's, it's like any business like your scale if you can afford to or not. (C9)
In contrast, smaller growers must often operate without making new hires, placing additional pressure on existing workers to not only upskill but also to find the time to take on these extra responsibilities.
…so yeah, me and my father-in-law are the IT team. (G6)
Considering these findings, it is important to reflect upon the transformations in social structure created by digital agriculture and how this potentially influences adoption behaviors. Some scholars have pointed out that reliance upon digitized systems has shifted traditional farming knowledge, which has in turn restructured farmer livelihoods and identities (Carolan, 2020). Early adopters may view using digital agriculture as being a part of a suite of good farming practices; they may accept digital agriculture as a standard operating procedure, whereas late adopters do not (Carolan, 2020). However, among all our participants there is an implied acknowledgement that data are routinely collected by agricultural tech companies. Our observation of the lack of immediate concern about data management suggests a privacy calculus (Dinev and Hart, 2006) where perceived privacy risk is low and is consistent with moralistic trust (Gardezi and Stock, 2021). We have evidence that growers do not consider their data to be of high value to an external attacker:
…because they know exactly what I know, which is nobody cares about your GPS maps. You know, there's no value in that to someone (G10)
And little evidence that our participants have thought about how agtech companies could exploit aggregate farm data for profit at the expense of their client growers (i.e., the data subjects), although one grower noted:
So, the only issue with that that we talked about was if they use that data against us and say that we’re using too much water (G7)
Gardezi and Stock note that farmers are increasingly dependent upon the technology companies that collect their data, “… agricultural technology companies have successfully positioned their knowledge products as superior to farmers’ experiential knowledge, thereby ensuring farmers’ sustained engagement with [precision agriculture] technologies for the purposes of data capture and capital accumulation” (Gardezi and Stock, 2021, p. 1). Though this leaves agricultural producers in a vulnerable position with how their data are used, and ushers in concern about the fate of late adopters, the use of data by agricultural tech companies appears to be accepted as “fait accompli.” Though some studies indicate that farmers may distrust agtech company use of their data (Fielke et al., 2022; Jakku et al., 2019; Wiseman et al., 2019), we interpret our finding of an absence of concern about data management as moralistic trust in the collection and use of data in digital agriculture. Producers internalize that data sharing is part of the digital agriculture process and a required aspect of the new way of farming, trusting their data will not be abused. The complex system of interdependent actors that makes up the modern agri-food value chain is poised for technology-enabled disruption, especially where data monetization opportunities exist. While growers may be comfortable for now and view the grower-agtech firm relationship as cooperative, we anticipate this position will change once they recognize the value of their data in the development of new agtech services and begin to manage data like any other business resource.
5.1 A way forward
Based on our analysis, we suggest a few approaches that target the economic, trust, and facilitating conditions concerns we heard in our interviews. First, addressing economic concerns, we suggest firms look closely at their business models. Leasing or service subscriptions seem more appealing (e.g., Precision ag as a Service, or PAaaS) for encouraging adoption, as they require less up-front investment. These PAaaS models can also address some of the support-related issues by including maintenance and troubleshooting as part of the subscription.
That's where subscription service model, where it's OK, you get the subscription. We'll send a technician out every so often or someone on call to come out or, hey, we got this new technology we’ll install it for the same price, whatever, and it is like, we can readjust the plan. Something like that might be more attractive to something like that, because then you're also getting the better technology. (G4)
[…] if somebody is paying for the subscription, I'm always there to pick up the phone for them. And that's where, you know, like I try to make that difference is, you know, understanding that these guys have been burned. I want to make sure that they feel that like they can feel secure. (C3)
However, this model requires that the technology demonstrates long-lasting benefits for the grower, or they will simply cancel the subscription once they have maximized the return.
[…] the farmer will use it for six months or seven months and love it. And then he'll pay the subscription for another two years and then finally just drop it and be like, I don't even use this anymore. You know? ‘Cause a lot of the things are fixed after your six months of using that, whatever it is you're using, […] after six months where your inefficiencies are at. Right. But then for the next two years, you end up paying for it because you think you need it. But then it’s like I already know. And it's, I've already cleaned it up and everything else you're showing me now is something I can't fix, you know? And so, then, they ended up getting dropped and it's not worth the subscription fee then. (C2)
Further, incentives do have a positive impact on adoption but can often be met with negativity. Mostly growers mentioned how some regulations are forcing them to adopt; a stick rather than a carrot that leaves a negative taste because typically the incentive is to meet reporting requirements to avoid fines.
If I'm getting a benefit from the technology and helps me save this money, get this grant money I think it’s a win-win. So that, so those incentives do help! You know? They really do. I do find those, sometimes they want to incentivize stuff but it’s not the most practical of things. You know? With this one it happened to be very practical for me, but some of the stuff they are incentivizing are really unpractical. (G1)
As soon as a new law or regulation comes out, there's a financial incentive to become more efficient to play within the boundaries and the rules of that regulation. You know, something like SGMA2 right, is really made this irrigation thing. (G10)
The relationship between experience and trust is dynamic and agtech firms can leverage this dynamic to build trust and demonstrate the effectiveness of new technology by engaging in beta testing or low-cost demonstration implementations with early adopters. These activities not only showcase tangible results but also establish a base of users who can serve as peer support for new customers, thereby enhancing the facilitating condition of technical support. Additionally, trust is reinforced through social influence within the community.
And so we partnered with a company [Company]. And so they've, they've kind of been working on different versions of doing that in different ways, and we've kind of given our ranch as a place to test that out. (G10)
Growers identify interoperability as a facilitating condition of adoption; there is a need to think in terms of systems and how new products interact with existing technology to provide information for decision-making. In essence, there’s a need for general infrastructure support, for technologies that interoperate; systems are needed, not more siloed components. Increased interoperability and complementary systems increase yields and improve economic profitability (DeLay et al., 2022).
… a yield harvest monitor is what's allowed the Midwest to become what they become with technology. You know, because none of this data makes any sense, really, until you can overlay it with a yield map … (G9)
Moreover, we concur with recommendations from other authors who have examined barriers to technology adoption in agricultural systems, and who have concluded that enhancing farmer trust is a lubricant for facilitating technology adoption. Eastwood et al. (2023) recommend using system approaches to design and develop technology, and to “… design and develop data governance, business models, and standards for data that are transparent, inspire trust, and share benefits of digital technologies among supply chain stakeholders” (p. 1). Fielke et al. (2022) note the benefits of redistributing trust between industry and farmers, and that “… providing institutional mechanisms to empower those actors that feel disempowered allows for progress in reducing antagonistic power relations and creating space for exploration of alternative arrangements” (p. 128). McGrath et al. (2023) point out the value of widening inclusion and farmer engagement in designing technology from the perspective of end-users. To summarize, we advocate for farmer engagement in product design, data governance, and innovation at all levels of the supply chain as a conduit to increasing trust, and thus technology adoption.
Our findings add to the literature on moralistic trust and expand upon the work of Gardezi and Stock (2021), although we contemplate whether there are regional influences from American subjects who may be more accepting of sharing farm data, compared to the Australian farmers in the Wiseman et al. (2019), Jakku et al. (2019), and Fielke et al. (2022) studies. Gardezi and Stock’s work was conducted in South Dakota and Vermont (USA). Moreover, our subjects in California’s Central Valley are within two hours of Silicon Valley, which is recognized as an international hub for technological innovation; hence, there is a possibility that familiarity with innovation creates a comfort level and acceptance of data sharing. In sum, we contribute to the literature that suggests that farmers aren’t overly concerned about data privacy, though the potential for regionalism merits additional exploration.
6 Conclusion
In summary, our study shows that adoption of digital agriculture technology follows similar patterns with other industries, according to the TAM-3 and UTAUT-2 models. Our findings are also consistent with those of Gardezi and Stock (2021) promulgating that producers adopt precision agriculture as a feature of moralistic trust. However, given the importance of food production and the prevalence of small farmers, without making the value of tech adoption clear, small farmers may lag their peers and have difficulty catching up, which may further marginalize this population. To “bridge the disconnect between farmers and the tech community” (Nolet, 2018, p. 1) we must identify the value proposition of proposed digital agriculture solutions.
The benefits of engaging small farmers may pay off, in a way that advances digital technology to improve food security and minimize environmental impacts for all, write Dorin et al. (2022, p. 1): “Agricultural technology can potentially reduce poverty, increase well-being and food security, and drive economic development, but it has not yet, and it will not, unless changes are made in how it is created, applied and socially integrated”.
Systems of agtech are needed to fully realize benefits of on-farm digitalization; siloed products cannot hope to address the complexities involved, and often do not improve economic profitability (DeLay et al., 2022). Development of whole systems requires collaboration and cooperation across the agtech industry, something that may need policy intervention to realize. Examples of needed cooperation include the development of standards so that smart devices such as sensors are interoperable or “platform agnostic”.
To increase adoption, it is not enough to demonstrate theoretical economic benefits. The intelligent route forward is to first engage a broad set of stakeholders in identifying the most pressing needs. While growers are aware of potential economic benefits, their willingness to adopt new technologies often hinges on how well those technologies address their most significant challenges. It is critical to maintain this stakeholder engagement during development and testing phases and use partner-growers to demonstrate effectiveness. Such an approach not only ensures the most valuable systems are developed, but also builds strategic trust dimensions in both social and technology domains.
This work demonstrates the importance of profitability and trust in growers’ adoption decisions and that data collection by agtech companies may not be the barrier for implementation of IoT technologies such as sensors. In future work, we will be looking more deeply at this interplay between profit, data, and trust through experiments to identify, for example, the willingness to pay for technology with select features. Our current work is limited in the small sample size of participants and the focus on organizational-level adoption decisions. Future work could explore the adoption by farm workers, including the impact of new technologies on the nature of their work.
Data availability statement
The raw data supporting the conclusions of this article will be made available by the authors, without undue reservation.
Ethics statement
The studies involving humans were approved by University of California Merced Institutional Review Board (Study # UCM 2021-115). The studies were conducted in accordance with the local legislation and institutional requirements. The participants provided their written informed consent to participate in this study.
Author contributions
MY: Conceptualization, Data curation, Formal analysis, Methodology, Project administration, Supervision, Visualization, Writing – original draft, Writing – review & editing, Software, Validation. CK: Conceptualization, Data curation, Funding acquisition, Investigation, Methodology, Project administration, Resources, Supervision, Visualization, Writing – original draft, Writing – review & editing.
Funding
The author(s) declare financial support was received for the research, authorship, and/or publication of this article. This study was funded by the National Science Foundation NSF 19-503 Gen-4 Engineering Research Centers, award number (FAIN): EEC-1941529.
Acknowledgments
The authors extend appreciation to UC-Merced graduate students Alisha Nesslage, Christopher (Mikey) Bernal, and Nicolas Goncalves who assisted with data collection and analysis. The authors acknowledge the use of Chat-GPT 40 (https://chat.openai.com/) in light copy editing, for example, to improve the caption for Table 1. A supplemental document with all prompts and responses is available on request.
Conflict of interest
The authors declare that the research was conducted in the absence of any commercial or financial relationships that could be construed as a potential conflict of interest.
The author(s) declared that they were an editorial board member of Frontiers, at the time of submission. This had no impact on the peer review process and the final decision.
Publisher’s note
All claims expressed in this article are solely those of the authors and do not necessarily represent those of their affiliated organizations, or those of the publisher, the editors and the reviewers. Any product that may be evaluated in this article, or claim that may be made by its manufacturer, is not guaranteed or endorsed by the publisher.
Footnotes
1. ^We use the Economic Research Service farm typology (Hoppe and MacDonald, 2013) to define small and mid-sized family farms. This typology considers the ownership structure (owned by family) and gross cash farming income (GCFI) to further classify small (low and moderate sales), medium, and large family farms. The farms represented in our sample span the small and medium GCFI categories ranges.
2. ^California’s Sustainable Groundwater Management Act (SGMA) https://water.ca.gov/Programs/Groundwater-Management/SGMA-Groundwater-Management
References
Ajzen, I. (1991). The theory of planned behavior. Organ. Behav. Hum. Decis. Process. 50, 179–211. doi: 10.1016/0749-5978(91)90020-T
Aubert, B. A., Schroeder, A., and Grimaudo, J. (2012). IT as enabler of sustainable farming: an empirical analysis of farmers’ adoption decision of precision agriculture technology. Decis. Support. Syst. 54, 510–520. doi: 10.1016/j.dss.2012.07.002
Barnes, A. P., Soto, I., Eory, V., Beck, B., Balafoutis, A. T., Sanchez, B., et al. (2019). Influencing incentives for precision agricultural technologies within European arable farming systems. Environ. Sci. Pol. 93, 66–74. doi: 10.1016/j.envsci.2018.12.014
Basso, B., and Antle, J. (2020). Digital agriculture to design sustainable agricultural systems. Nat. Sustain. 3, 254–256. doi: 10.1038/s41893-020-0510-0
Carolan, M. (2020). Acting like an algorithm: digital farming platforms and the trajectories they (need not) lock-in. Agric. Hum. Values 37, 1041–1053. doi: 10.1007/s10460-020-10032-w
Davis, F. D. (1989). Perceived usefulness, perceived ease of use, and user acceptance of information technology. MIS Q. 13, 319–340. JSTOR. doi: 10.2307/249008
de Vries, J. R., Turner, J. A., Finlay-Smits, S., Ryan, A., and Klerkx, L. (2023). Trust in Agri-food value chains: a systematic review. Int. Food Agribus. Manag. Rev. 26, 175–198. doi: 10.22434/IFAMR2022.0032
DeLay, N., Thompson, N., and Mintert, J. (2020). Farm data usage in commercial agriculture. Center For Commercial Agriculture. Available at: https://ag.purdue.edu/commercialag/home/wp-content/uploads/2020/01/202001_Delay_FarmDataUsageinCommercialAgriculture.pdf
DeLay, N., Thompson, N. M., and Mintert, J. R. (2022). Precision agriculture technology adoption and technical efficiency. J. Agric. Econ. 73, 195–219. doi: 10.1111/1477-9552.12440
Dinev, T., and Hart, P. (2006). An extended privacy Calculus model for E-commerce transactions. Inf. Syst. Res. 17, 61–80. doi: 10.1287/isre.1060.0080
Dorin, A., Penn, A. S., and Siqueiros, J. M. (2022). AgTech that doesn’t cost the earth: creating sustainable, ethical and effective agricultural technology that enhances its social and ecological contexts. ALIFE 2022: The 2022 Conference on Artificial Life.
Dubois, A., and Gadde, L.-E. (2002). Systematic combining: an abductive approach to case research. J. Bus. Res. 55, 553–560. doi: 10.1016/S0148-2963(00)00195-8
Dubois, A., and Gadde, L.-E. (2014). “Systematic combining”—a decade later. J. Bus. Res. 67, 1277–1284. doi: 10.1016/j.jbusres.2013.03.036
Eastwood, C., Turner, J. A., Romera, A., Selbie, D., Henwood, R., Espig, M., et al. (2023). A review of multi-scale barriers to transitioning from digital agriculture to a digital bioeconomy. CABI Rev. 2023:2. doi: 10.1079/cabireviews.2023.0002
Faik, I., Sengupta, A., and Deng, Y. (2024). Inclusion by design: requirements elicitation with digitally marginalized communities. MIS Q. 48, 219–244. doi: 10.25300/MISQ/2023/17225
Farooq, M. S., Riaz, S., Abid, A., Abid, K., and Naeem, M. A. (2019). A survey on the role of IoT in agriculture for the implementation of Smart farming. IEEE access 7, 156237–156271. doi: 10.1109/ACCESS.2019.2949703
Fielke, S., Taylor, B. M., Coggan, A., Jakku, E., Davis, A. M., Thorburn, P. J., et al. (2022). Understanding power, social capital and trust alongside near real-time water quality monitoring and technological development collaboration. J. Rural. Stud. 92, 120–131. doi: 10.1016/j.jrurstud.2022.03.022
Finlay-Smits, S., Ryan, A., de Vries, J. R., and Turner, J. (2023). Chasing the honey money: transparency, trust, and identity crafting in the Aotearoa New Zealand mānuka honey sector. J. Rural. Stud. 100:103004. doi: 10.1016/j.jrurstud.2023.03.012
Gallardo, R. K., and Brady, M. P. (2015). Adoption of labor-enhancing technologies by specialty crop producers: the case of the Washington apple industry. Agric. Finance Rev. 75, 514–532. doi: 10.1108/AFR-05-2015-0022
Gallardo, R. K., and Sauer, J. (2018). Adoption of labor-saving Technologies in Agriculture. Ann. Rev. Resour. Econ. 10, 185–206. doi: 10.1146/annurev-resource-100517-023018
Gardezi, M., and Stock, R. (2021). Growing algorithmic governmentality: interrogating the social construction of trust in precision agriculture. J. Rural. Stud. 84, 1–11. doi: 10.1016/j.jrurstud.2021.03.004
Gefen, D., Karahanna, E., and Straub, D. W. (2003). Trust and TAM in online shopping: an integrated model. MIS Q. 27, 51–90. doi: 10.2307/30036519
Glaser, B., and Strauss, A. (2017). Discovery of grounded theory: strategies for qualitative research. New York: Routledge.
Hoppe, R. A., and MacDonald, J. M. (2013). Updating the ERS farm typology (economic information bulletin 110; p. 40). USDA. Available at: https://www.ers.usda.gov/webdocs/publications/43742/36482_eib110.pdf?v=754.3
Jakku, E., Taylor, B., Fleming, A., Mason, C., Fielke, S., Sounness, C., et al. (2019). “If they don’t tell us what they do with it, why would we trust them?” trust, transparency and benefit-sharing in Smart farming. Wageningen J. Life Sci. 90-91, 1–13. doi: 10.1016/j.njas.2018.11.002
Kagan, C. R., Arnold, D. P., Cappelleri, D. J., Keske, C. M., and Turner, K. T. (2022). Special report: the internet of things for precision agriculture (IoT4Ag). Comput. Electron. Agric. 196:106742. doi: 10.1016/j.compag.2022.106742
Klerkx, L., Jakku, E., and Labarthe, P. (2019). A review of social science on digital agriculture, smart farming and agriculture 4.0: new contributions and a future research agenda. Wageningen J. Life Sci. 90-91, 1–16. doi: 10.1016/j.njas.2019.100315
Kumari, S., Jeble, S., and Patil, Y. B. (2018). Barriers to technology adoption in agriculture-based industry and its integration into technology acceptance model. Int. J. Agric. Resour. Gov. Ecol. 14:338. doi: 10.1504/IJARGE.2018.098022
Liu, Y., Ma, X., Shu, L., Hancke, G. P., and Abu-Mahfouz, A. M. (2021). From industry 4.0 to agriculture 4.0: current status, enabling technologies, and research challenges. IEEE Trans. Industr. Inform. 17, 4322–4334. doi: 10.1109/TII.2020.3003910
Lowder, S. K., Sánchez, M. V., and Bertini, R. (2021). Which farms feed the world and has farmland become more concentrated? World Dev. 142:105455. doi: 10.1016/j.worlddev.2021.105455
Lowenberg-DeBoer, J. M., and Erickson, B. (2019). Setting the record straight on precision agriculture adoption. Agron. J. 111, 1552–1569. doi: 10.2134/agronj2018.12.0779
Marra, M., Pannell, D. J., and Abadi Ghadim, A. (2003). The economics of risk, uncertainty and learning in the adoption of new agricultural technologies: where are we on the learning curve? Agric. Syst. 75, 215–234. doi: 10.1016/S0308-521X(02)00066-5
McCormack, M., Buckley, C., and Kelly, E. (2021). Using a technology acceptance model to investigate what factors influence farmer adoption of a nutrient management plan. Irish J. Agric. Food Res. 60, 142–151. doi: 10.15212/ijafr-2020-0134
McGrath, K., Brown, C., Regan, Á., and Russell, T. (2023). Investigating narratives and trends in digital agriculture: a scoping study of social and behavioural science studies. Agric. Syst. 207:103616. doi: 10.1016/j.agsy.2023.103616
McKnight, D. H., Carter, M., Thatcher, J. B., and Clay, P. F. (2011). Trust in a specific technology: an investigation of its components and measures. ACM Trans. Manag. Inf. Syst. 2, 1–25. doi: 10.1145/1985347.1985353
McKnight, D. H., and Chervany, N. L. (2006). “Reflections on an initial trust-building model” in Handbook of trust research. eds. R. Bachmann and A. Zaheer (Edward Elgar Publishing), 29–51. Available at: https://experts.umn.edu/en/publications/reflections-on-an-initial-trust-building-model
McKnight, D. H., Choudhury, V., and Kacmar, C. (2002). Developing and validating trust measures for e-commerce: an integrative typology. Inf. Syst. Res. 13, 334–359. doi: 10.1287/isre.13.3.334.81
Neubauer, S. (2021). The place of data in precision agricultural data asset management. Insti. Adm. J. Adm. Sci. 1, 52–61. doi: 10.54201/iajas.v1i2.23
Nolet, S. (2018). Seeds of success: Advancing digital agriculture from point solutions to platforms (p. 14). United States studies Centre at the University of Sydney. Available at: http://united-states-studies-centre.s3.amazonaws.com/attache/1a/d0/a5/4e/af/c1/0a/6a/ab/5c/13/fe/ed/4f/99/9b/Advancing-Digital-Agriculture-in-Australia.pdf
Osrof, H. Y., Tan, C. L., Angappa, G., Yeo, S. F., and Tan, K. H. (2023). Adoption of smart farming technologies in field operations: a systematic review and future research agenda. Technol. Soc. 75:102400. doi: 10.1016/j.techsoc.2023.102400
Parker, C., Scott, S., and Geddes, A. (2019) in Snowball sampling. eds. P. Atkinson, S. Delamont, A. Cernat, J. W. Sakshaug, and R. A. Williams (SAGE research methods foundations). doi: 10.4135/9781526421036831710
Parmaksiz, O., and Cinar, G. (2023). Technology acceptance among farmers: examples of agricultural unmanned aerial vehicles. Agronomy 13:77. doi: 10.3390/agronomy13082077
Parra-López, C., Reina-Usuga, L., Garcia-Garcia, G., and Carmona-Torres, C. (2024). Functional analysis of technological innovation systems enabling digital transformation: a semi-quantitative multicriteria framework applied in the olive sector. Agric. Syst. 214:103848. doi: 10.1016/j.agsy.2023.103848
Reddy, N. V., Reddy, A. V. V. V., Pranavadithya, S., and Kumar, J. J. (2016). A Critical Review on Agricultural Robots. International Journal of Mechanical Engineering and Technology, 7, 183–188.
Rezaei, R., Safa, L., and Ganjkhanloo, M. M. (2020). Understanding farmers’ ecological conservation behavior regarding the use of integrated pest management-an application of the technology acceptance model. Global Ecol. Conserv. 22:e00941. doi: 10.1016/j.gecco.2020.e00941
Schilke, O., and Wirtz, B. W. (2012). Consumer acceptance of service bundles: an empirical investigation in the context of broadband triple play. Inf. Manag. 49, 81–88. doi: 10.1016/j.im.2011.12.003
Shepherd, M., Turner, J. A., Small, B., and Wheeler, D. (2018). Priorities for science to overcome hurdles thwarting the full promise of the ‘digital agriculture’ revolution. J. Sci. Food Agric. 100, 5083–5092. doi: 10.1002/jsfa.9346
Shi, Y., Siddik, A. B., Masukujjaman, M., Zheng, G., Hamayun, M., and Ibrahim, A. M. (2022). The antecedents of willingness to adopt and pay for the IoT in the agricultural industry: an application of the UTAUT 2 theory. Sustain. For. 14:6640. doi: 10.3390/su14116640
Silva, A. G., Canavari, M., and Sidali, K. L. (2017). A technology acceptance model of common bean growers’ intention to adopt integrated production in the Brazilian central region. Die Bodenkultur: J. Land Manag. Food Environ. 68, 131–143. doi: 10.1515/boku-2017-0012
Soma, T., and Nuckchady, B. (2021). Communicating the benefits and risks of digital agriculture technologies: perspectives on the future of digital agricultural education and training. Frontiers. Communication 6:762201. doi: 10.3389/fcomm.2021.762201
Tubtiang, A., and Pipatpanuvittaya, S. (2015). A study of factors that affect attitude toward deploying smart-farm technologies in Tanud subdistrict, Damnoen Saduak district in Ratchaburi province. J. Food Sci. Agric. Technol. 1:144–148.
USDA . (2024). USDA census of agriculture highlights: Farm producers (p. 2). Available at: https://www.nass.usda.gov/Publications/AgCensus/2022/index.php. https://www.nass.usda.gov/Publications/Highlights/2024/Census22_HL_FarmProducers_FINAL.pdf
van der Burg, S., Wiseman, L., and Krkeljas, J. (2021). Trust in farm data sharing: reflections on the EU code of conduct for agricultural data sharing. Ethics Inf. Technol. 23, 185–198. doi: 10.1007/s10676-020-09543-1
Venkatesh, V., and Bala, H. (2008). Technology acceptance model 3 and a research agenda on interventions. Decis. Sci. 39, 273–315. doi: 10.1111/j.1540-5915.2008.00192.x
Venkatesh, V., Thong, J. Y. L., and Xu, X. (2012). Consumer acceptance and use of information technology: extending the unified theory of acceptance and use of technology. MIS Q. 36, 157–178. doi: 10.2307/41410412
Weersink, A., Fraser, E., Pannell, D., Duncan, E., and Rotz, S. (2018). Opportunities and challenges for big data in agricultural and environmental analysis. Ann. Rev. Resour. Econ. 10, 19–37. doi: 10.1146/annurev-resource-100516-053654
Wiseman, L., and Sanderson, J. (2019). Agricultural data rules: Enabling best practice. Coast, Queensland: School of Law and Society; University of the Sunshine, 21 Available at: https://research.usc.edu.au/esploro/outputs/report/Agricultural-data-rules-Enabling-Best-Practice/99451393602621.
Wiseman, L., Sanderson, J., Zhang, A., and Jakku, E. (2019). Farmers and their data: an examination of farmers’ reluctance to share their data through the lens of the laws impacting smart farming. Wageningen J. Life Sci. 90-91:100301, 1–10. doi: 10.1016/j.njas.2019.04.007
Woodcock, A. (2014). Late adopters and laggards: should we care? Int. J. Design Manag. Prof. Pract. 7, 53–61. doi: 10.18848/2325-162X/CGP/v07i03/38609
Wysel, M., Baker, D., and Billingsley, W. (2021). Data sharing platforms: how value is created from agricultural data. Agric. Syst. 193:103241. doi: 10.1016/j.agsy.2021.103241
Keywords: Internet of Things (IoT), technology adoption, UTAUT-2, TAM3, agricultural technology
Citation: Yeo ML and Keske CM (2024) From profitability to trust: factors shaping digital agriculture adoption. Front. Sustain. Food Syst. 8:1456991. doi: 10.3389/fsufs.2024.1456991
Edited by:
Paul Edwards, DairyNZ, New ZealandReviewed by:
James Alan Turner, AgResearch Ltd., New ZealandEmma Jakku, Commonwealth Scientific and Industrial Research Organisation (CSIRO), Australia
Copyright © 2024 Yeo and Keske. This is an open-access article distributed under the terms of the Creative Commons Attribution License (CC BY). The use, distribution or reproduction in other forums is permitted, provided the original author(s) and the copyright owner(s) are credited and that the original publication in this journal is cited, in accordance with accepted academic practice. No use, distribution or reproduction is permitted which does not comply with these terms.
*Correspondence: M. Lisa Yeo, bHllbzJAdWNtZXJjZWQuZWR1
†These authors have contributed equally to this work