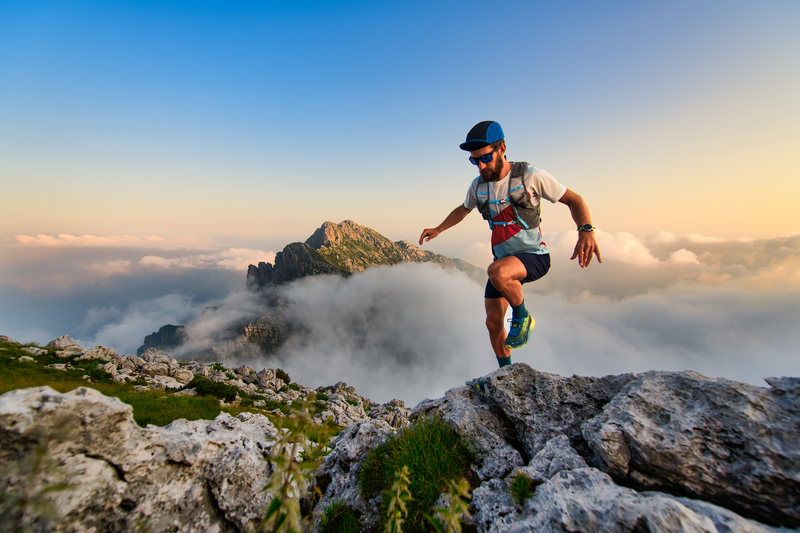
94% of researchers rate our articles as excellent or good
Learn more about the work of our research integrity team to safeguard the quality of each article we publish.
Find out more
ORIGINAL RESEARCH article
Front. Sustain. Food Syst. , 04 December 2024
Sec. Agricultural and Food Economics
Volume 8 - 2024 | https://doi.org/10.3389/fsufs.2024.1455732
This article is part of the Research Topic Sustainable Innovations in Agriculture: Economic Analysis of Climate Smart Practices View all 3 articles
Introduction: The rapid advancement of digital technology has catalyzed the emergence of “digital villages,” presenting both new opportunities and challenges for the digital transformation of agriculture. This transformation has become a core driving force behind sustainable agricultural development. This paper utilizes panel data from 30 provincial-level administrative regions of mainland China (excluding Tibet) for the period 2011 to 2020 to explore how digital rural development (DRD) impacts agricultural green total factor productivity (AGTFP) and examines its underlying mechanisms.
Methods: Regarding variable measurement, the study employs the entropy weighting method to quantify the DRD and uses an extended Data Envelopment Analysis-Malmquist model to assess AGTFP. For the research approach, the study constructs a two-way fixed effects model to evaluate the impact of DRD on AGTFP and explores the underlying mechanisms. Specifically, the study conducts benchmark regression analysis, endogeneity tests, robustness tests, mechanism examinations, and heterogeneity tests.
Results: (1) DRD significantly enhances AGTFP, a conclusion that remains robust following the province-year clustering robust standard error estimates, winsorization tests, and excluding COVID-19-affected years. (2) Mechanistic analysis indicates that DRD primarily boosts AGTFP by promoting the development of digital inclusive finance (DIF) and facilitating the integration of primary, secondary, and tertiary industries (IPST). (3) Heterogeneity tests show that the positive impact of DRD on AGTFP is more pronounced in regions with higher levels of informatization and central regions of China.
Discussion: To harness DRD’s potential more effectively in fostering sustainable agricultural development, policymakers should concentrate on DIF and the IPST. Additionally, the government should pay close attention to disparities in informatization levels and resource allocation across regions and develop targeted strategies accordingly. Fundamentally, this study aims to elucidate China’s efficacy in rural digitalization and the green transformation of agriculture to an international audience. It seeks to provide insights and guidance on formulating more scientific, efficient, and sustainable strategies for agricultural development worldwide, thereby promoting green agricultural growth and supporting rural revitalization.
The global climate crisis, characterized mainly by climate warming and the frequent occurrence of extreme weather, is one of the significant contributors to food shortages. The food crisis has forced some countries to adopt a low-quality, energy-intensive, and crude model of agricultural development. In this cycle of cumulative causality, environmental degradation, and food shortages have become two of the century’s most significant challenges to global sustainable development (Sakariyahu et al., 2024). Concurrently, advanced information technologies catalyze the emergence of innovative models, technologies, and products worldwide, facilitating the digital transformation of rural locales (Marousek, 2014; Kliestik et al., 2023). Digital rural development (DRD) exemplifies the pivotal role contemporary technologies play in bolstering global food security and enhancing environmental stewardship, thereby serving as a dynamic impetus for the high-quality economic advancement of rural regions (Jiang et al., 2024).
Considering the projected increase in the global population and the food crisis, advancements in agricultural technology to enhance production efficiency are evolving rapidly (Maroušek et al., 2023a,b,c). Consequently, the digital transformation in agriculture has become a focal point of concern for nations worldwide (Zscheischler et al., 2022). In 2015, the Indian government initiated the “Digital India” strategy to enhance rural network connectivity and enable access to e-governance and telemedicine services in rural areas. Following this, in 2016, Japan proposed the “Next Generation Agriculture, Forestry, and Fisheries Technology” program, which integrates intelligent machinery with sophisticated information technology to enhance the effectiveness of agricultural resource management and develop smart agriculture. A year later, in 2017, the European Union’s “Smart Villages” initiative focused on enhancing network infrastructure in rural areas and promoting innovative agricultural technologies to improve the agricultural production process. Concurrently, responding to emerging trends in rural transformation, China has prioritized accelerating the DRD to boost informatization in agriculture and rural regions. This initiative aims to facilitate the transition of agriculture towards greener production methods, thereby promoting sustainable and high-quality advancement of Chinese agriculture (Liu et al., 2024).
According to the National Bureau of Statistics of China (n.d.), the gross output value of agriculture, forestry, animal husbandry, and fisheries in China increased from 437.2 billion dollars in 2004 to 2.32 trillion dollars in 2022. This demonstrates a robust development trend in the agricultural economy. Nevertheless, the rapid expansion of agriculture has placed continuous pressure on resources and the environment, revealing the vulnerability of China’s agrarian development (Luo et al., 2022). Environmental pollution and the irrational use of resources, including carbon dioxide emissions, soil degradation, and excessive application of chemical fertilizers and pesticides, have exerted significant pressure on the ecological environment. This has led many rural economic regions into a quandary of “growth without development” (Wu, 2024) and has even triggered the “resource curse” phenomenon (Yang C. et al., 2024). Faced with these challenges, constructing digital villages to achieve a green transformation of the agricultural economy becomes particularly important. The DRD in China encompasses the application of a range of modern technologies, from intelligent agricultural equipment to big data analytics, cloud computing, the Internet of Things, and artificial intelligence, ensuring optimal resource allocation and environmental protection in agrarian production (Sharma et al., 2020; Papadopoulos et al., 2024). By implementing smart agriculture and digital financial services, the DRD enables the recombination of agricultural production factors, enhances resource utilization efficiency in rural areas, and optimizes agricultural management (Hong et al., 2024).
In this process, it is essential to effectively assess the impact of the DRD on the development of agriculture and rural areas. The agricultural green total factor productivity (AGTFP) provides an integrated approach to evaluating the efficiency of agriculture and its environmental impacts. By assessing the input and output efficiency of various resources such as land, labor, capital, and technology in agricultural production and emphasizing the production process’s impact on the environment, the AGTFP has become a crucial tool for evaluating the influence of digitalization on agricultural sustainability (Ge et al., 2018; Tang et al., 2023). Nevertheless, research is scarce on the correlation between digital villages and the overall efficiency of agricultural production in terms of environmental sustainability. Therefore, this study constructs a comprehensive evaluation system based on the concept of digital villages and employs a two-way fixed effects model to analyze the relationship between the DRD and AGTFP across 30 provincial-level administrative regions of mainland China (excluding Tibet) from 2011 to 2020. The study is guided by three specific objectives: (1) Assess the influence of DRD on AGTFP and enhance the scholarly discourse in this field; (2) Examine the underlying mechanisms linking them, thereby guiding the strategic development of digital rural infrastructure; (3) Investigate the regional disparities in the impacts of DRD on AGTFP and identify the factors contributing to these variations to promote balanced development across different regions. This study aims to provide fresh perspectives and empirical evidence for evaluating the impact of the DRD on achieving green transformations in agriculture. Furthermore, it seeks to offer decision-making support to policymakers striving to balance efficiency in agricultural production with environmental protection, thereby better facilitating global sustainable agricultural development.
This study presents the following marginal contributions: firstly, compared to traditional research on AGTFP, which focuses on established influencing factors, this study highlights the significance of digital villages as a critical enabler in the new era. Specifically, regarding the research object, this study has created a unique indicator system encompassing five dimensions of agricultural and rural digitalization: infrastructure, production, operation, circulation, and life services. This system enables an all-round and comprehensive assessment and analysis of the DRD. Secondly, this study enhanced the measurement of AGTFP by incorporating the afforestation area as an input indicator, building on existing metrics. This expansion improves the comprehensiveness of the AGTFP assessment and integrates the environmental contributions of forestry activities, which have frequently been overlooked in previous research. Thirdly, to address the limitations of the traditional Data Envelopment Analysis (DEA)-Malmquist model, the study employed the Super-Efficiency Epsilon-Based Measure-Malmquist (SE-EBM-Malmquist) model and the Super-Efficiency Slacks-Based Measure-Malmquist (SE-SBM-Malmquist) model to assess AGTFP accurately. These approaches were chosen to improve the credibility and scientific rigor of the study. Lastly, countries worldwide are exploring effective strategies to promote digital rural communities’ development and enhance agricultural ecological environmental construction. This study extensively investigates the mechanisms behind the DRD’s role in AGTFP, particularly from digital inclusive finance (DIF) perspectives and the integration of primary, secondary, and tertiary industries (IPST). This approach significantly enriches the research framework concerning their interrelationship. In addition, this study examines the impact of the DRD on AGTFP by analyzing the differences in informatization levels and geographic locations among provinces. This study provides empirical evidence for the targeted implementation of digital rural strategies and the comprehensive promotion of green transformation in agriculture worldwide. It also offers insights into DRD for countries experiencing regional development imbalances.
The structure of this paper is presented as follows. Section 2 focuses on literature review. Section 3 introduces research hypotheses. Section 4 presents the models and data used in the analysis. Section 5 discusses benchmark regression, endogeneity tests, robustness tests, and studies of mechanisms and heterogeneity. Section 6 concludes with a summary and discussion.
Digital transformation in rural areas is increasingly recognized as a critical driver of environmental sustainability and enhanced production efficiency, attracting significant global interest. Developed nations have been at the forefront of incorporating digital economy strategies into their agricultural sectors. Examples include large-scale smart agriculture in America, intelligent livestock farming in Germany, innovative greenhouse production in the Netherlands, and compact intelligent equipment in Japan, significantly boosting agricultural productivity and facilitating rural modernization (Dvorsky et al., 2023). The United Nations Food and Agriculture Organization promotes the “Digital Villages” initiative worldwide to foster broader participation in digital agriculture governance. This strategic effort is designed to drive the transformation of agricultural and food systems and to enhance the sustainability of agricultural practices globally. Globalization presents many opportunities for China’s digital villages, aligning them with international standards in technology application and facilitating collaboration and exchange. Moreover, it has enabled China to share its experiences and case studies of DRD globally, thereby contributing to the sustainable development of rural economies worldwide (Balsa-Barreiro et al., 2019).
Weak infrastructure and inadequate public services have long impeded the development of China’s rural areas (Liu D. et al., 2023). In response, China has initiated and is actively advancing digital village initiatives aimed at digitally transforming and upgrading traditional infrastructure in rural regions. These initiatives also foster deep integration between the Internet and specialized agricultural practices, gradually enhancing the socio-economic development of agricultural and rural communities. Moreover, the fundamental conditions in China differ significantly from those in developed nations, with notable disparities in development levels, economic structures, resource allocation, and urbanization between China’s eastern and inland regions (Liu W. W. et al., 2022). Through its strategy of promoting DRD, China is actively working to diminish the economic and social disparities between urban and rural areas and between the eastern, central, and western regions, aiming to achieve balanced regional development and harmonious national progress. Consequently, these disparities provide an ideal platform for examining the effectiveness of DRD in areas with uneven development. Thus, this study offers valuable insights for other nations grappling with similar challenges of regional development imbalance.
Given the urgent need for sustainable development, scientifically and effectively evaluating the performance of AGTFP has become a vital issue. Currently, the primary methods for measuring AGTFP include Stochastic Frontier Analysis and DEA. Compared to Stochastic Frontier Analysis, DEA can handle decision units with multiple input and output indicators simultaneously without requiring a specific production function to be set (Liu D. et al., 2021). These advantages make DEA more widely used. The traditional DEA model does not account for the slack in input–output variables, making it unable to calculate efficiency values that include undesirable outputs accurately. In recent years, incorporating undesirable outputs, such as environmental pollution, into the AGTFP measurement framework has become an essential aspect of productivity research. Furthermore, although a range of models within the DEA framework are prevalently utilized for assessing static efficiency, they do not capture the temporal dynamics of efficiency trends among decision-making units (DMUs).
The Malmquist productivity index method allows dynamic panel data analysis from a time series perspective (Diewert et al., 1982). Integrating it with DEA can be utilized to calculate changes in productivity (Fare et al., 1994). Hence, Zhou and Zhang have attempted to use extended DEA-Malmquist models that consider undesirable outputs to measure AGTFP, aiming for more accurate results (Zhou and Zhang, 2024). To further ensure more accurate efficiency evaluations, researchers have applied extended DEA models to assess AGTFP, building on the studies by Tone (2002). For instance, Jiang et al. (2024) employed the SE-SBM-Global Malmquist-Luenberger index model to evaluate AGTFP across various Chinese agricultural provinces. Similarly, Lu F. et al. (2024) used the SE-SBM-Global Malmquist index to assess AGTFP in agricultural regions of China. Notably, the EBM, which combines radial and non-radial features, effectively addresses the challenges of calculating efficiency values inherent in traditional DEA and SBM models. This model merits further investigation for its potential to improve productivity assessments in AGTFP (Jin and Ren, 2022).
Input variables, desirable and undesirable output are integral to evaluating AGTFP within the DEA model. Yet, the academic field has not agreed on the specific inputs and outputs crucial for accurately measuring AGTFP. Existing research predominantly identifies key inputs in agricultural production as capital, labor, land resources, machinery, chemical fertilizers, diesel, agricultural water, and agricultural plastic film (Ge et al., 2018; Yang and Tong, 2023; Jiang et al., 2024). Future research needs to incorporate additional input factors into the analysis framework to more comprehensively assess the impact of resource utilization efficiency on AGTFP. Additionally, as resources become scarcer and the prices of resource factors rise, it is increasingly crucial to achieve greater output with minimal investment (Maroušek et al., 2020; Vochozka et al., 2020a; Vochozka et al., 2020b). Existing studies generally use metrics such as total agricultural output (Liu Y. et al., 2021) or agricultural value-added (Ge et al., 2018) when assessing desired agricultural outputs. The former quantifies the overall production scale, whereas the latter evaluates the net economic contribution of agricultural activities. The selection of traditional indicators in terms of undesirable output indicators was based on research by Lai et al. (2004) and Chen et al. (2006). These studies conclude that agricultural pollution measurements primarily stem from chemical oxygen demand, total nitrogen, and total phosphorus generated collectively by chemical fertilizers used on farmland, livestock and poultry farming, organic solid wastes from farmland, and rural living. However, the choice of these indicators has been contentious. For instance, manure from livestock has long been used as organic fertilizer in crop production, and its role as a pollutant is debatable (Maroušek and Maroušková, 2021). Given these issues, the academic community has revisited undesirable agricultural outputs in light of the Intergovernmental Panel on Climate Change reports and the realities of climate change. An increasing number of studies consider carbon emissions during agricultural production as a metric for assessing the AGTFP, such as those by Liu D. et al. (2021), Huang et al. (2022), Song et al. (2022), Luo et al. (2023) and Zhou and Zhang (2024), and this perspective is gaining increasing recognition. Simultaneously, achieving maximum efficiency in carbon emissions reduction and fostering the development of a circular economy has become a topic of intense discussion (Stávková and Maroušek, 2021; Maroušek et al., 2023a,b,c).
Using the DEA model as a foundation for evaluating AGTFP, researchers also examine the effects of environmental financing, fiscal expenditures, environmental legislation, technical advancements, and other variables on AGTFP. Regarding environmental finance, Li et al. (2023) employed moderating and mediation effects on panel data from 30 Chinese provinces (2015–2019) to verify that the growth of green finance can considerably raise the AGTFP, with an apparent regional heterogeneity and an inverted U effect. Liu S. Y. et al. (2023) verified that green trade restrictions favorably increased the nation’s AGTFP, using panel data for China and the Organization for Economic Co-operation and Development nations from 2005 to 2019. Regarding fiscal expenditures, Deng et al. (2023) confirmed that agricultural fiscal expenditures significantly increased the AGTFP of maize, and this effect was mainly realized through the enhancement of rural infrastructure. Concerning environmental regulation, the research by Sun and Zhang (2021) indicates that stricter environmental regulations are positively correlated with higher levels of AGTFP. Furthermore, by validating the “Porter Hypothesis,” they highlight the pivotal role of green innovation technologies in linking environmental regulation with AGTFP. In addition, Xiong et al. (2023) conducted a relevant investigation. In the field of technological advancement, Wang et al. (2024) utilized panel data from the China Family Panel Studies for the years 2016, 2018, and 2020 as their sample. Their research confirmed that farmers’ use of the internet significantly enhances AGTFP.
In the digital wave, the rapid emergence of modern digital information technologies, epitomized by the internet, is increasingly becoming a crucial driver in implementing digital rural strategies worldwide (Kliestik et al., 2024; Valaskova et al., 2024). Although digital technologies have significantly transformed rural areas, there is a scarcity of studies on the digitalization of rural settings (Cowie et al., 2020; Wang H. et al., 2022). Rural areas are characterized by their unique and diverse nature, and their challenges and technological contexts vary between countries. Consequently, there are different interpretations of the understanding of digital villages. Concerning the DRD’s conceptual clarity, the majority of studies have theorized the digital phenomenon considering regional experiences with rural living; hence, for instance, various researchers have given it different names, such as “Platform Ruralism” (Wang W. et al., 2022), “Smart Villages” (Bokun and Nazarko, 2023), “Rural Broadband” (Ali and Duemmel, 2019; Schmit and Severson, 2021), and “Digital Villages” (Irwansyah, 2020), etc. Simultaneously, the existing assessments of DRD encompass both multidimensional comprehensive indicators (Zhu and Chen, 2022) and single-dimension evaluation metrics (Wang et al., 2021). Nevertheless, research on accurately gauging the level of DRD remains scarce, and a mature indicator evaluation system still needs to be developed.
Previous studies on DRD have mainly concentrated on the urban–rural digital divide (Salemink et al., 2017). As digital technologies increasingly permeate rural areas, it is essential to shift our research focus from solely examining disparities in digital access to exploring the specific impacts of DRD on rural socio-economic development, quality of life enhancements, and the promotion of sustainable agricultural practices. Xia et al. (2019) believe that the DRD, supported by digital technology, may stimulate the high-quality growth of agriculture, contributing to economic development. Galperin et al. (2022) verified the beneficial effects of broadband deployment on labor income and employment in rural Ecuador to enhance quality of life. Additionally, DRD demonstrates significant potential for environmental protection and sustainable agricultural development. Demonstrated in their study that green growth in agriculture is driven by DRD, employing methods such as fixed effects models and mediated effects models. In their critical analysis of the research on intelligent agriculture’s efficacy, Zul Azlan et al. (2024) clarified how innovative agricultural technology can curb environmental hazards, spur green economic development, and advance social inclusion. Moreover, comparable research on the DRD and their ecological effects include those by Shen et al. (2022) and Fu et al. (2023).
As DRD progressively evolves, the academic community has comprehensively evaluated its environmental impacts. Current research primarily focuses on specific aspects of the DRD, such as the effects of digital applications on AGTFP, including areas like DIF (Gao et al., 2022; Shen et al., 2024), internet use (Wang et al., 2024), digital technology (Sun et al., 2023; Du et al., 2024), rural broadband (Li, 2024) and agricultural mechanization (Lu S. et al., 2024). However, comprehensive measurements of DRD to delve deeper into their relationship with AGTFP are scarce. Among the few studies, Du et al. (2023) have shown that DRD boosts AGTFP by promoting scaled agricultural operations and agricultural informatization. Additionally, the DRD indirectly enhances AGTFP by promoting green technological advancements and improving resource allocation efficiency (Guo, 2024). Similarly, Lu F. et al. (2024) found that DRD enhances AGTFP by stimulating entrepreneurial activities in rural areas, with the mediating effect of these activities accounting for 3% of the total effect. Nevertheless, more potential mechanisms remain to explore further to affirm the relationship between these factors.
In summary, the studies provide strong literature support for this paper’s research direction, indicator selection, and measurement methods. However, it should be noted that there are still some deficiencies in the current research. Regarding the indicators, on the one hand, a mature evaluation system for measuring DRD has not yet been established, necessitating a more multidimensional assessment. On the other hand, the indicators for AGTFP need further refinement. Additionally, more input factors should be included in the calculations to comprehensively reflect the resource use in the production process and its impact on AGTFP. Regarding research methodologies, while the conventional DEA-Malmquist model is widely used for productivity measurement, it has some limitations. More innovative methods are available for measuring AGTFP, ensuring greater accuracy in research findings. Regarding research content, most existing studies begin by examining the application of digital technologies in specific rural aspects to assess their impact on AGTFP. Literature that comprehensively measures DRD across multiple dimensions and explores its effects on AGTFP is rare. Furthermore, more potential mechanisms await discovery to further confirm the relationship between these two areas.
Given this, the paper expands and improves the existing research in four ways: initially, the study develops a comprehensive assessment framework for DRD, covering five critical dimensions: infrastructure, production, operation, circulation, and life services, which enriches the research on the evaluation system of DRD indicators. Secondly, in addition to traditional measures of AGTFP, the study incorporates afforestation area as an input indicator. This inclusion reflects the recognition that afforestation activities enhance the ecological value of land and are integral to sustainable agricultural strategies (Hou et al., 2020). Factoring in afforestation areas enables a more holistic assessment of agricultural activities’ environmental impacts and contributions. Thirdly, to mitigate the inherent shortcomings of conventional DEA models, this study initially applies the SE-SBM-Malmquist model for assessing AGTFP. This model moderates the strict proportional assumption between inputs and outputs by incorporating slack variables, enabling more accurate efficiency evaluations for DMUs. Following this, the EBM model, which includes both radial and non-radial characteristics, is utilized to resolve the issues encountered in the efficiency calculations of the radial DEA and SBM models. Consequently, the SE-EBM-Malmquist model is applied to re-assess AGTFP, enhancing the credibility and scientific rigor of the study. This approach yields two sets of AGTFP values, facilitating further analysis. Finally, this study comprehensively explores the impact of DRD on AGTFP from the perspectives of DIF and the IPST, thereby expanding the research framework between them. Previous literature typically considered DIF and IPST as independent variables, examining their relationship with AGTFP. This study treats these factors as mechanism variables, further deepening our understanding of how DRD influences AGTFP. Additionally, the paper conducts a heterogeneity analysis across provinces with different levels of informatization and geographic locations, revealing the asymmetric effects of DRD on AGTFP, thus providing more nuanced insights for policymakers.
The neoclassical theory of economic growth posits that continual increases in input factors and sustained enhancements in productivity are the fundamental drivers of long-term growth (Solow, 1999). Concurrently, endogenous growth theory points out that as inputs of material resources such as labor, capital, and land reach saturation, their marginal returns begin to decline, whereas the marginal returns on knowledge and technology tend to increase (Helpman, 1992; Kopf, 2007). Similarly, the significance of technological advancements in enhancing the quality and efficiency of production factors is underscored both in economic development theory (Becker, 1962) and innovation systems theory (Markard et al., 2015). Therefore, knowledge and technology are essential endogenous drivers of total factor productivity increase due to their positive externalities (Vu, 2013; Chou et al., 2014). Combined with the above theoretical analysis, we believe that in the current digital information era, integrating digital technology with the traditional agricultural economy and society significantly contributes to the growth of the AGTFP.
Specifically, the DRD encompasses five dimensions: digital infrastructure, digital production, digital operation, digital circulation, and life service digitalization. Each plays a significant role in driving AGTFP, so our hypothesis is structured around these five dimensions.
(1) Digital infrastructure: The DRD is epitomized by digital infrastructure. This foundational development in digital infrastructure has catalyzed the digitization of agricultural production, operations, circulation, and living services for rural residents. It has provided essential technical and informational support to enhance AGTFP. Furthermore, it has fostered the efficient utilization of resources and the sustainable development of rural environments.
(2) Digital production: The DRD is reflected in the digitization of agricultural production. Adopting digital technologies has facilitated a shift from labor-intensive agricultural practices to technology-driven methods (Klerkx et al., 2019). Various digital tools, including the Internet of Things, big data, and artificial intelligence, provide precise guidance for optimizing crop structures and irrigation systems. These technologies offer comprehensive technical support across all stages of agriculture—from seeding and nursery to planting, harvesting, and storage—effectively reducing production costs and enhancing agricultural efficiency (Papadopoulos et al., 2024).
(3) Digital operation: The DRD enables market entities to adapt to complex external environments through continuous production and operational strategy improvements. On the one hand, digital management via e-commerce platforms directly connects agricultural products with consumers, reducing intermediary steps and lowering transaction costs. This allows agricultural producers to better adjust their production strategies based on market demand, thereby avoiding overproduction and resource waste. On the other hand, more precise market positioning and product distribution contribute to reducing carbon footprints and environmental pressure (Ji et al., 2024).
(4) Digital circulation: Utilizing network media and the platform economy, DRD has expanded the functionality of integrated agricultural production and sales systems, establishing a deeply interconnected agricultural economic ecosystem. This cohesive technological strategy not only bolsters the efficiency of the entire agricultural supply chain but also mitigates carbon footprints through streamlined transportation logistics (Falter et al., 2023). Consequently, agricultural circulation becomes more eco-friendly, fostering the evolution of a sustainable agricultural model attuned to ecological preservation (Bosworth et al., 2023).
(5) Life service digitalization: DRD can leverage digital technologies to revitalize rural resource elements, enhance the application and conversion of knowledge and technology within the agricultural economy, and foster innovative services for rural living. These initiatives stimulate the growth of rural digital markets, effectively addressing disparities in resource allocation and spatial constraints typical in rural development. This not only ensures fairness in development but also significantly improves the quality of life of rural residents, thereby providing strong support for the sustainable development of the rural economy.
From the above analysis, the DRD enhances agricultural production’s technical efficiency and improves agriculture’s environmental sustainability and economic benefits (see Figure 1). Based on this, the following hypothesis 1 is proposed:
H1. The DRD can significantly promote AGTFP.
Figure 1. Diagram of research hypotheses. This figure illustrates the research hypotheses of the study, namely the direct impact of digital rural development on agricultural green total factor productivity and two indirect paths of influence. Icons made by Freepik (https://www.flaticon.com/authors/freepik) from www.flaticon.com.
The DRD offers the opportunity to achieve financial inclusion and sharing for all by enhancing the accessibility and convenience of financial services. This can effectively facilitate the redistribution of social resources and contribute to the advancement of high-quality rural development (Pang and Wang, 2023). In traditional setups, rural areas often face difficulties accessing financial services due to their remote locations and a lack of adequate banking infrastructure. Within the framework of the DRD, digital finance empowers farmers in these remote areas by providing access to financial services and credit resources through mobile payment platforms and online banking. Furthermore, DRD can effectively improve rural financial services’ coverage breadth and depth.
The DIF significantly contributes to the enhancement of AGTFP. By integrating digital financial services, agricultural practices can more effectively utilize financial resources and alleviate financing constraints enterprises face. On the one hand, regarding risk prevention and control, DIF provides farmers with a more flexible and broad coverage of agricultural insurance products. Banking financial institutions can automatically approve crop conditions based on technologies such as the Internet, satellite remote sensing, and remote video, conduct online insurance coverage for agriculture insurance, and improve the efficiency of credit services, thereby helping farmers cope with income instability caused by natural disasters or market volatility. On the other hand, in promoting the green transition to agricultural investment by providing startup funds, entrepreneurial loans, and subsidies specifically for sustainable farm projects, the DIF can encourage farmers to invest in water-saving irrigation systems, bio-fertilizers, and other environmentally friendly technologies. Adopting these technologies directly increases ecological sustainability, thereby boosting the AGTFP.
In summary, DIF boosts financial inclusion in rural areas, directly supporting agriculture’s modernization and green transformation. This transformation not only enhances the efficiency and environmental sustainability of agricultural production but also strengthens the overall competitiveness of rural economies. Hence, the study proposes the following hypothesis 2 (see Figure 1):
H2. The DRD can promote AGTFP through the effect of the DIF.
Rural industrial integration refers to agriculture-based, through industrial penetration, industrial linkage, etc., to complementarity and synergy between industries and the integration of the entire agricultural industry chain development model. Industry agglomeration theory posits that the IPST strengthens inter-industry connections and facilitates the sharing of technology, resources, and information (Fan and Scott, 2003). The DRD leverages digital technologies to integrate agriculture, manufacturing, and services seamlessly. This integration fosters the development of an industrial ecosystem that enhances the efficiency and sustainability of the agricultural economy (Yang et al., 2023). Significantly, it boosts AGTFP.
Specifically, the IPST influences the green development of agriculture primarily through three aspects: (1) Improving agricultural productivity: The DRD can enhance the efficiency and quality of agricultural production by integrating intelligent agricultural technologies. These smart systems improve resource efficiency and enable continuous environmental monitoring and management, advancing agriculture’s green development. (2) Promoting deep processing and value-added agricultural products: The DRD can merge agriculture and manufacturing through digital technologies, enhancing agricultural products’ deep processing and branding. This approach increases the market value of products and boosts farmers’ incomes. (3) Integrating agriculture and services through digital construction: Digital platforms, mainly e-commerce, enable agricultural products to move directly from producers to consumers. This shortens the supply chain, reduces resource consumption, and cuts carbon emissions in intermediate stages.
As the integration of the three industries in rural areas progresses, the increasing specialization and intensification of agricultural production not only enhance the efficiency of resource use and reduce waste but also lower the environmental costs associated with agriculture. This shift drives the greening of the agrarian output, establishing it as a trend for long-term and effective rural development. Therefore, hypothesis 3 is as follows (see Figure 1):
H3. The DRD can enhance AGTFP by promoting the IPST.
The core issue of this study is to identify the relationship between the DRD and the growth of AGTFP. Based on panel data, the empirical analysis employs a two-way fixed effects model for the benchmark regression model. The model is set as follows:
In Equation 1, , respectively indicate the province and the year; The study defines the value of green total factor productivity in agriculture measured by the SE-SBM-Malmquist model as AGTFP1 and by the SE-EBM-Malmquist model as AGTFP2; represents the digital rural development status in province during year ; indicates the set of control variables; denotes year fixed effects, for which the study employed interaction terms between control variables and year fixed effects; The term denotes province fixed effects, which were addressed by using interaction terms between control variables and provincial fixed effects; represents the random error term; is the constant.
The explained variable in this study is the AGTFP. To address the comparability issues of AGTFP across different periods, this study employs the MAXDEA9 software, utilizing both the E-SBM-Malmquist and SE-EBM-Malmquist models. These models were applied to evaluate the AGTFP of 30 provincial-level administrative regions of mainland China (excluding Tibet) from 2011 to 2020, designated as AGTFP1 and AGTFP2, respectively (SE-SBM-Malmquist: AGTFP1; SE-EBM-Malmquist: AGTFP2). Additionally, the raw scale productivity data may exhibit heteroscedasticity. To mitigate this issue, data were logarithmically transformed to conform more closely to statistical models’ assumptions and enhance the analysis’s interpretive power. The specific computational methods and indicators selected are detailed below.
The DEA model is a widely used method for measuring the efficiency of inputs and outputs. The efficiency value calculated by the traditional DEA model can only be kept in the interval (0,1], with efficient DMUs all assigned a value of 1, which makes it impossible to compare the effective DMUs further. Additionally, issues related to slack and undesirable outputs may introduce biases into the calculated efficiency scores. Tone (2001) formulated the SBM model to address the limitations, which adopts a non-radial and non-angular methodology. This model significantly enhances accuracy by overcoming weaknesses inherent in conventional DEA methodologies. Further advancing this approach, Tone (2002) integrated the advantages of super-efficiency models with the SBM model to create the SE-SBM model. This innovative model effectively handles undesirable output issues and comprehensively evaluates DMUs at the efficiency frontier. Drawing on the approach of Song et al. (2022), this study initially implemented the SE-SBM model to assess the AGTFP statically. Assuming a set of DMUs, denoted as , each DMUs is characterized by m inputs, represented by . Desired output is recorded as , and undesirable output as . The model is configured as stated in Equation 2:
where is the efficiency evaluation index; , , and are the input slack variable, desirable output slack variable, and undesirable output slack variable, respectively; and the is the weight of the DMU. If the efficiency value exceeds 1, the efficiency for that year is in an optimal state.
To ensure the robustness of the results, the SE-EBM model is employed for static measurement of AGTFP once again. The model is an improvement on the SE-SBM model. It facilitates the effective integration of both radial and non-radial approaches by relaxing the assumption of proportional reduction in radial function inputs. Additionally, it accommodates the radial ratios between the input frontier and actual values and the non-radial slack based on input differentiation. Consequently, this model effectively addresses the limitations of both radial and non-radial models and overcomes the deficiencies of the SE-SBM model (Tone and Tsutsui, 2010). The specific model configuration is as follows:
in the above Equation 3, is the slack variable for the desired output of class , is the slack variable for the class non-desired output; are the weighting indicators of each of the above, respectively; is the class non-desired output of decision cell ; is the class non-desired output of decision cell .
Various models under the DEA framework are extensively utilized for static efficiency assessments. However, these models fail to capture the dynamic trends in efficiency changes over time among DMUs. To address the limitations of DEA’s discontinuous description, this paper introduces the Malmquist index to evaluate the dynamic changes in AGTFP across provinces over time. This index measures changes in productivity by assessing the distance between DMUs and the production frontier (Malmquist, 1953). Consequently, this study integrates DEA with the Malmquist index, employing the SE-SBM-Malmquist and SE-EBM-Malmquist methods to analyze the dynamic changes in AGTFP across provinces over multiple periods. The change rate of AGTFP from period to can be measured by the Malmquist index between periods and . The model is set up as follows in Equation 4:
Input variables, desirable and undesirable outputs are essential for assessing AGTFP using the DEA model. In selecting input indicators, this study draws on the research of Jiang et al. (2024), Ge et al. (2018), and Yang and Tong (2023), selecting capital, labor, land resources, irrigation, pesticides, chemical fertilizers, agricultural plastic film, agricultural machinery, and diesel as input factors. Additionally, this study introduces afforestation area as a novel input indicator. An increase in afforestation area reflects investments in sustainable land management and environmental protection, which enhance the ecological value of the land, boost carbon sequestration, and increase vegetation productivity (Xiao et al., 2015). By including this input indicator, a more comprehensive assessment of AGTFP can be achieved. Moreover, agricultural carbon emissions exacerbate global climate change, leading to rising temperatures, altered precipitation patterns, and increased extreme weather events, accurately reflecting the negative environmental impacts of agricultural activities (IPCC, 2007). Hence, this paper follows Ding et al. (2022) in using calculated values of agricultural carbon emissions as a definition for undesired outputs, with sources including chemical fertilizers, pesticides, plastic film, diesel, plowing, and irrigation. Finally, inspired by the research of Guo and Liu (2020), this study selects the total agricultural output value as the desired output. The specific inputs and outputs selected are shown in Figure 2.
Figure 2. Input and output indicators (AGTFP). This figure displays the specific components of input factors (across 10 categories), desired output and undesired output employed in assessing agricultural green total factor productivity.
The explanatory variable in this study is DRD. Previous literature primarily focused on building indicators for DRD that concerned the digital economy, industry applications, and service innovation (OECD, 2014; Pant and Hambly Odame, 2017). Nevertheless, this paper aligns with Chinese policy documents and incorporates the research findings of Zhang et al. (2020), Yin et al. (2020), and Zhou and Deng (2023) to establish a comprehensive DRD evaluation indicator system. This system, detailed in Table 1, comprises five key dimensions: digital infrastructure, digital production, digital operation, digital circulation, and life service digitalization. Regarding the measurement approach, the study utilizes the entropy weight method to assess the extent of DRD, drawing on the analysis of Zhu and Chen (2022). The specific procedures involved in this measurement process are outlined as follows:
In the initial step, the extreme value method is employed for the non-quantitative normalization of primary data. The formulas used to handle the positive and negative indicators are distinct, as outlined in Equation 5 and Equation 6 below:
For positive variables:
For negative variables:
represents the normalized value of the th indicator for the th province in year , where i=1, 2…, m (m being the total number of provinces), and j=1, 2…, n (n being the total number of indicators). denotes the maximum value of the th indicator across all years, while represents the minimum value. These normalization techniques ensure that all indicators are scaled uniformly between 0 and 1, facilitating a more effective comparative analysis across different variables and periods.
For each indicator j of province i in year t, the proportional value, is computed as outlined in Equation 7:
The entropy threshold for indicator j in year t is calculated using the Equation 8:
The weight of each indicator j in year t is derived as shown in Equation 9:
Finally, the DRD index for each province i in year t is calculated specified in Equation 10:
This index combines the weighted contributions of all indicators to provide a comprehensive measure of the dynamic changes in each province.
To mitigate the impact of the missing variable, the study selected five control variables, each characterized by distinct attributes.
(1) Human capital level (HCL): The HCL captures the variation in educational attainment across provinces in China, significantly influencing labor productivity. This variation in human capital is particularly relevant during the digitization process; agricultural workers with a solid educational background and applicable skills are more adept at mastering and utilizing advanced digital technologies, thus enhancing agricultural productivity. The HCL is quantified by the ratio of students enrolled in higher education to the total population. A logarithmic transformation is applied to this ratio to normalize the data.
(2) Agricultural financial support (AFS): Adopting agricultural technology and advanced production management models heavily relies on government financial contributions. Increasing the AFS is crucial to promoting the development of high-quality agricultural productivity and fostering the establishment of low-energy agricultural production systems (Hu et al., 2021). Following the research by Tang and Sun (2022), the study selected the ratio of government financial support for agricultural development to total local fiscal expenditure as an indicator and applied a logarithmic transformation to it.
(3) Impact of natural disasters on agriculture (INDA): The frequent occurrence of severe natural disasters, such as floods, droughts, and hail, significantly reduces the effectiveness and availability of resources, thereby threatening agricultural productivity (Platania et al., 2022). Consequently, the study selected the area of farmland affected by these disasters as the control variable and applied a logarithmic transformation to the data (Data sources: National Bureau of Statistics of China, n.d.).
(4) Rural electricity level (REL): Modern electrical facilities support the use of environmentally friendly agricultural technologies, such as precision irrigation and low-carbon energy technologies. These advancements reduce dependence on fossil fuels and decrease the negative environmental impacts of agricultural activities. Consequently, the ratio of rural electricity consumption (in ten thousand kilowatt-hours) to the rural population (in ten thousand) is utilized as the control variable. This data is then subjected to logarithmic transformation.
(5) Innovation level (INL): Innovation plays a crucial role in transforming agricultural production methods, enhancing productive efficiency, and achieving sustainable agricultural development (Ren et al., 2023). Therefore, the study utilized the urban innovation index calculated by Kou and Liu (2017). The study aggregated city panel data according to the provinces in which they were located to obtain the innovation index for each province. This data was then subjected to logarithmic transformation.
This paper is based on sample data from 30 provincial-level administrative regions of mainland China (excluding Tibet) between 2011 and 2020. The relevant data are mainly from the China Statistical Yearbook, the China Rural Statistical Yearbook, the China Environmental Statistical Yearbook, the China Trade and Foreign Economic Statistics Yearbook, the Peking University Digital Comprehensive Financial Index, the Ali Research Institute (n.d.) report, and the monthly statistical report on imports and exports of Chinese agricultural products. Other sources include China’s Urban and Industrial Innovation Power Report 2017 and the official website of the National Bureau of Statistics of China (n.d.). The descriptive statistics of the above data are shown in Table 2.
Before executing the benchmark regression analysis, a collinearity assessment was conducted. The findings showed that the Variance Inflation Factors for all variables remained under 10, confirming the absence of significant collinearity within the model. Subsequently, the study utilized Equation 1 for the benchmark regression to explore the interactions among the primary variables of interest. In Table 3, the dependent variables are AGTFP1 and AGTFP2, and the primary explanatory variable is the DRD. Columns (1) and (3) show the estimation results when the control variables are included, with regression coefficients of 0.320 and 0.191, respectively, both significant at the 5% level. Additionally, this study incorporates year fixed effects into the model to mitigate the impact of time trends. The results, presented in columns (2) and (5), remain statistically significant. Moreover, recognizing that each province may have distinct cultural, economic, and policy environments that could affect the dependent variables, the study further integrated province fixed effects into our regression analysis to control for potential omitted variable bias due to unobserved provincial attributes. As indicated in columns (3) and (6), the results are significant at the 10% and 5% levels, with the coefficients being 0.292 and 0.177, respectively. These demonstrate that the DRD has significantly enhanced the AGTFP, confirming H1.
Existing research predominantly discusses the impact of DRD on green agricultural growth from singular perspectives, such as digital technological innovations (Yang R. et al., 2024), rural e-commerce (Ji et al., 2023), or digital inclusive finance (Gao et al., 2022; Liu D. et al., 2023). In contrast, our study constructs a multi-dimensional set of DRD indicators, further confirming a positive relationship between DRD and the enhancement of AGTFP. This conclusion aligns with the findings of a few studies, such as those by Jiang et al. (2022), Shen et al. (2022), Du et al. (2023), and Sun et al. (2023). Distinctly, unlike previous studies that often rely on a single dependent variable, our study employs the SE-SBM-Malmquist and SE-EBM-Malmquist models to calculate AGTFP and integrates these measurements into regression analyses, enhancing the reliability and robustness of the research findings.
The empirical analysis highlights that DRD holds significant practical implications for advancing green development in rural areas. On the one hand, by harnessing information technology and upgrading infrastructure, DRD facilitates precise agricultural management. This not only enhances crop yields but also mitigates agricultural carbon emissions and surface pollution (Maroušek, 2023), thus making a substantial contribution to the greening of agricultural production (Maroušek et al., 2023a,b,c). On the other hand, the development of e-commerce platforms and the enhancement of rural logistics networks through DRD have significantly broadened the distribution channels for agricultural products. This enables agricultural producers to align directly with market demands, streamline supply chains, and reduce the consumption of resources and production inputs, thereby playing a pivotal role in fostering the green transition and diversified development of the agricultural economy (Wang H. et al., 2022).
To mitigate potential selection bias, this study implements propensity score matching (PSM) to align observable variables between the treatment and control groups, thereby reducing the influence of selection bias on the regression results (Rosenbaum and Rubin, 1983; Shipman et al., 2017). Provinces with DRD exceeding the yearly average are designated as the treatment group (coded as 1), with the remainder forming the control group (coded as 0). This study employs the widely used nearest-neighbor matching method, which involves pairing units from the treatment and control groups based on the smallest absolute difference in propensity scores. After matching, only control group provinces that closely match the characteristics of those in the treatment group are retained. Subsequently, the study conducted a balance test, with results showing that the standardized biases for most variables were below 10%. This indicates an enhanced balance between the treatment and control groups following the matching, as depicted in Figure 3.
Following variable matching, balance was established between the treatment and control groups, aligning with the balance assumption of PSM. The matched samples obtained through PSM effectively reduced the systematic differences among control variables, providing a solid data foundation for more accurate regression analysis. The regression results are displayed in Table 4. Estimates incorporating control variables are shown in columns (1) and (4), while columns (2) and (5) include additional year fixed effects, and columns (3) and (6) integrate province fixed effects. The analysis revealed that the estimated coefficients consistently achieved significance at the 1% level. Compared to baseline regression analyses, the results after PSM appear more significant. This indicates that the presence of endogeneity may lead to an underestimation of ordinary least squares results, failing to isolate the effects of other factors on AGTFP. The use of PSM effectively circumvents this issue.
Simultaneity bias might arise between independent and dependent variables, wherein they mutually influence each other, complicating the determination of causality. This paper employs a lagged variable approach to mitigate this immediate interaction and address potential issues of reverse causality and endogeneity. By regressing the independent variables at a one-period lag, the study examines the delayed impact of DRD on AGTFP. This methodology effectively reduces the immediate feedback loops among variables, as the lagged values are not immediately affected by the current values of the dependent variables. As demonstrated in Table 5, the regression coefficients for the dependent variables lnAGTFP1 and lnAGTFP2 remain significantly positive, confirming that the baseline regression results are robust.
Considering the potential correlations among data points across different years within the same province and provinces within the same year in economic, social, and cultural aspects, this study adopts the methodology from Shen et al. (2023). It incorporates province-year interaction clustered robust standard errors to address potential biases in standard error calculations that may arise from these correlations. The province-year interaction clustering not only enhances the inferential statistics of the model but also increases the reliability of the research findings. As shown in Table 6 columns (1) and (4) present the estimates with control variables, columns (2) and (5) include estimates with year fixed effects, and columns (3) and (6) add province fixed effects to the existing model, with all results proving significant. The findings in Table 6 reinforce those of Table 3, demonstrating that even after accounting for intra-group correlations (within provinces) and autocorrelations (within years), the main variables of the model still exhibit significant statistical effects.
This study employed a winsorization analysis to assess the robustness of the model results, especially in the presence of potential outliers. Compared to truncation analysis, winsorization is better suited for small sample datasets where preserving all data points is crucial (Jose and Winkler, 2008). This approach involves replacing extreme observations: values below the 1st percentile are replaced with the 1st percentile value, and values above the 99th percentile are replaced with the 99th percentile value. After this exclusion, the model parameters were re-estimated, and the results remained robust (see Table 7), enhancing the research findings’ reliability.
During the COVID-19 pandemic, agriculture faced unconventional operational challenges, such as labor shortages, logistics disruptions, and market demand changes. These challenges may temporarily distort agricultural production efficiency and patterns (Liu Y. et al., 2022), making it difficult to assess the impact of normal-level DRD on AGTFP. Therefore, the study excludes the years during the pandemic and retained the data from 2011 to 2019 to better reflect the true and sustained impact of DRD on AGTFP. Table 8 shows columns (1) and (4), which present the estimation results with control variables included. Columns (2) and (5) show results by adding year fixed effects. Columns (3) and (6) further incorporate provincial fixed effects. The results are significant and consistent with the benchmark regression findings.
The findings discussed earlier demonstrate that DRD can foster the AGTFP. But what mechanisms drive this impact? To address this question, the study develops a mechanism effect model grounded in our research hypotheses:
In the Equation 11, serve as mechanical variables, for which the study has selected IPST and DIF as the mechanical variables; denotes year fixed effects, for which the study employed interaction terms between control variables and year fixed effects; The term denotes province fixed effects, which were addressed by using interaction terms between control variables and provincial fixed effects. Our study on DIF draws upon the research by Guo et al. (2020), employing the Digital Inclusive Finance Index compiled by the Institute of Digital Finance Peking University (n.d.). This index comprises three dimensions: the breadth of digital finance coverage, depth of usage, and the degree of digitalization in inclusive finance, providing a comprehensive depiction of the development of DIF in China. Financial data frequently exhibit significant heteroscedasticity due to variations in institutional sizes or transaction volumes. Logarithmic transformation is utilized to stabilize the variance of variables, which is why the study applies logarithmic transformations to DIF. To measure the IPST, our approach builds on the studies by Xiao and Du (2019), developing an evaluation system for integrating rural industries. This system comprises five dimensions: extension of the agricultural industry chain, expansion of agricultural multifunctionality, development of new agricultural business, economic benefits, and social benefits, with 11 criteria layers, detailed in Table 9. Data for all indicators can be downloaded from the website of the National Bureau of Statistics of China (n.d.). Subsequently, the entropy weighting method is employed to calculate the IPST Index, using the methodology described earlier. The results of the mechanical tests are presented in Table 10.
The underlying premise of the DIF mechanism posits that the DRD enhances the accessibility and convenience of financial services in rural areas. Such improvements foster a green transformation in agricultural risk management and investment through digital innovations, thus supporting the growth of AGTFP. Following this rationale, the study further examines the role of DIF within this context. The results, as presented in columns (1) and (2) of Table 10, indicate that after including control variables and year fixed effects in column (1) and further adding province fixed effects in column (2), the estimations are both significantly positive at the 1% level. This demonstrates that DRD significantly enhances DIF, substantially increasing the AGTFP, thereby confirming H2. These findings illustrate the vital role of digital finance in facilitating a transition towards greener and more sustainable agricultural practices. Previous studies (Liu S. Y. et al., 2023; Xie et al., 2023) have examined the direct effects of DIF on AGTFP. In contrast, our research conceptualizes DIF as a mechanistic variable, thus clarifying the relationship between DRD and DIF, and more clearly delineating the pathway through which DRD enhances AGTFP. This approach reveals more intricate mechanisms of influence and helps fill the research gap in the literature on enhancing AGTFP through agricultural digitalization and suggesting strategic directions for promoting DIF within DRD.
The fundamental premise of the industrial integration and development mechanism is that the DRD enhances agricultural production efficiency, facilitates the deep processing and value addition of agricultural products, and fosters the growth of rural e-commerce and services. This digital advancement drives the IPST, leading to a more cohesive and efficient economic structure in rural areas. As rural integration of the three industries progresses, the specialization and intensification of agricultural production can enhance the efficiency of agricultural resource use, reduce resource waste, and lower environmental costs in agriculture (Samusevych et al., 2021). Consequently, this contributes to an improvement in the AGTFP. Building on this rationale, the study conducted a mechanical analysis. In column (3) of Table 10, control variables along with year fixed effects were included, and the coefficient is significantly positive at the 1% level, estimated at 0.328. Column (4) extends the model by integrating provincial fixed effects to account for potential biases due to provincial characteristics. The resulting coefficient is positive at the 1% significance level, recorded at 0.406. These confirm that the DRD notably enhances AGTFP by promoting IPST, thereby validating H3.
The impact of DRD on AGTFP is likely to be heterogeneous, owing to significant variations in resource endowments, informatization development status, and the baseline levels of rural green development across different regions in China. The study further explores this heterogeneous impact by examining the varying levels of informatization within DRD and by analyzing the specific geographical locations of the provinces. To gauge the informatization level, the study utilizes the total post and telecommunications business ratio to the gross domestic product (Data sources: National Bureau of Statistics of China, n.d.). A province is classified as having a high level of informatization if its value exceeds the median of the sample for that particular year; otherwise, it is considered to have a low level of informatization. The sample includes 146 provinces categorized as high informatization and 147 as low informatization. For regional classification, the study adheres to the standards set by the National Bureau of Statistics of China (n.d.), dividing the sample into eastern, central, and western provinces to conduct heterogeneity analyses.
The regression results are shown in Figure 4. It is observed that DRD has a significant positive impact on AGTFP in provinces with high informatization levels. However, the coefficients of DRD on AGTFP are not statistically significant in provinces with low informatization levels. The possible reasons for this are: In rural areas with advanced information technology, local farmers are often more capable of adopting new technologies and implementing modern management techniques. This enhances their ability to use digital technologies efficiently, boost agricultural productivity, optimize resource allocation, and reduce waste. Furthermore, areas with high levels of information technology infrastructure—including high-speed internet, data processing centers, and advanced communication facilities—benefit from strong technical support for agricultural production. This infrastructure facilitates the rapid, accurate collection and dissemination of agricultural data. Conversely, regions with limited access to information technology often face economic and technological disadvantages, exhibit low farm digitization and technology adoption levels, and lack the capacity for large-scale agricultural operations. These limitations impede the improvement of AGTFP.
The regression results in Figure 4 demonstrate that the estimated coefficients of DRD on AGTFP are consistently positive in the central region. This suggests that DRD significantly contributes to enhancing AGTFP in the central region. Nevertheless, the calculated coefficients for DRD on AGTFP in both the eastern and western regions are not statistically significant. The geographical disparities in the success of DRD can be attributed to variations in economic structure and agricultural dependence across different regions (He et al., 2019). Regions vary significantly in terms of economic development. For instance, eastern regions tend to be more economically advanced, boasting well-established agricultural sectors and superior infrastructure. In such settings, the potential enhancements achievable through DRD intervention are relatively minimal, making it challenging to establish statistical significance. Conversely, in the less developed western regions, where infrastructure is deficient, and the economy is relatively weak, DRD holds the potential for significant improvements. However, these improvements may not significantly impact productivity due to the region’s weak foundational conditions or other constraints, such as low educational levels and challenging geographic conditions. In contrast, with its greater reliance on agriculture and a higher proportion of its economy attributed to agricultural activities, the central region is likely to experience more noticeable impacts on its economic indicators from enhancements in agricultural productivity. In the heterogeneity analysis, Jin and Ren (2022) argue that the influence of DRD on AGTFP is more marked in the eastern provinces. However, our study observes a more pronounced effect in the central provinces. This discrepancy may be attributed to differences in measurement methods and the selection of indicators. Further research will be necessary to verify these findings.
Amid the backdrop of rural revitalization and agricultural digital transformation, our study investigates how DRD can leverage modern information technology to enhance agricultural productivity and ecological benefits, aligning with the demands of contemporary development and offering practical guidance. This research initially seeks to evaluate the effects of DRD on AGTFP empirically. We test our hypotheses using a fixed effects model with data from 30 provincial-level administrative regions of mainland China (excluding Tibet) from 2011 to 2020. The findings demonstrate that DRD enhances AGTFP, thereby validating H1. Furthermore, the research confirms H2 and H3, unveiling the facilitative mechanisms between DIF and IPST in the relationship between DRD and AGTFP. This provides a clear direction for global digital rural initiatives. Additionally, results from heterogeneity tests reveal that DRD significantly enhances AGTFP in regions with higher informatization levels and in the central province of China. The central provinces serve as potential hotspots for the DRD due to their strategic geographic advantages—acting as a bridge between the economically vibrant eastern regions and the developing western areas—rich industrial diversity spanning agriculture, manufacturing, and services, and strong government support. This conclusion can guide policymakers to tailor strategies according to local conditions, implementing region-specific development approaches to promote balanced growth across different areas better. In essence, this study leverages empirical analysis to showcase China’s experience and practices in DRD to the global audience. It aims to offer insights and guidance for devising more scientific, efficient, and sustainable agricultural development strategies worldwide, thereby promoting green agricultural growth and facilitating rural revitalization.
Based on the research findings, the study proposes the following policy recommendations.
Firstly, accelerating the development of digital villages is crucial for optimizing green agricultural practices. This study demonstrates that constructing digital villages can significantly enhance the AGTFP. Therefore, the government can advance the DRD across five strategic dimensions: laying a solid foundation for digital infrastructure in agriculture and rural areas by promoting the widespread adoption of broadband internet and the expansion of 5G networks into rural regions; integrating smart agricultural technologies (Marousek et al., 2012); supporting farmers in leveraging e-commerce platforms to sell their products; enhancing the management of digital supply chains for agricultural products; and improving access to and quality of digital services in education, healthcare, and entertainment. These initiatives form a comprehensive strategy that boosts agricultural efficiency and sustainability and ensures the modernization of rural communities.
Secondly, the government should promote the development of DIF to advance DRD, thereby aiding agriculture’s green and high-quality transformation. This initiative starts by supporting and promoting DIF products and services, which provide farmers with more accessible and cost-effective financial resources. For instance, farmers can adopt advanced agricultural equipment and eco-friendly technologies by offering low-interest loans and subsidies tailored to eco-agricultural projects (An et al., 2023). Moreover, the government should promote the integration of DIF with sustainable agricultural practices and encourage financial institutions to develop innovative financial products and tools. These initiatives will guide agricultural enterprises towards adopting green and low-carbon production methods, ensuring the provision of high-quality financial services for advancing green agriculture (Metzker et al., 2021).
Thirdly, the government should drive the deep IPST under the DRD initiative through policy incentives and financial support. This approach begins by promoting the adoption of intelligent agricultural technologies such as precision farming techniques, utilizing big data and artificial intelligence to optimize planting structures and enhance crop yields (Marousek et al., 2013). Additionally, digital technology is used to improve the efficiency and quality of agricultural product processing, such as employing automated food processing and packaging equipment. Finally, developing e-commerce platforms facilitates the direct sale of agricultural products and provides agricultural consultancy and technical services using digital tools. By implementing these specific strategies and actions, the government can effectively promote the integration of the three industries, achieve modernization of agriculture, and enhance both the economic and environmental benefits in rural areas.
Finally, policymakers should focus on the disparities in informatization levels and resource allocation between regions to formulate targeted development strategies accordingly. On the one hand, continuing to enhance digital village infrastructure with a focus on supporting the informatization of agriculture is essential for advancing agricultural technology and optimizing the agricultural industry structure. For areas with lower levels of informatization, governments should increase investment and improve local digital infrastructure, such as enhancing internet coverage and providing more technical support services. On the other hand, digital village construction should be advanced in a manner tailored to the economic development levels of different regions. Implementing an unbalanced development strategy will continually promote the enhancement of the AGTFP, thereby driving the high-quality, green development of global agriculture.
It should be noted that this study, constrained by data limitations, primarily utilizes data from Chinese provinces to examine the impact of DRD on AGTFP. Future research could gather multi-dimensional data and consider using data from prefecture-level cities and counties, employing various methods to delve deeper into the effects of DRD on AGTFP. Additionally, this research adopts the entropy weighting method to derive the comprehensive development index, paralleling the approach of numerous studies. However, this quantitative method overlooks diverse regions’ development needs and objectives. It is recommended that subsequent studies refine evaluative metrics and frameworks to align with the unique developmental contexts and requisites of different geographical areas. Finally, the scope of future studies could expand from focusing solely on China’s DRD to encompassing global digital rural initiatives. This broader perspective would enable a more precise analysis of the mechanisms at play.
The raw data supporting the conclusions of this article will be made available by the authors, without undue reservation.
FZ: Conceptualization, Data curation, Formal analysis, Funding acquisition, Project administration, Writing – original draft. YZ: Conceptualization, Methodology, Investigation, Resources, Software, Visualization, Writing – original draft. BW: Funding acquisition, Investigation, Resources, Supervision, Writing – review & editing.
The author(s) declare that financial support was received for the research, authorship, and/or publication of this article. This research was supported by the Key Research Base of Humanities and Social Sciences of Universities in Guangxi Zhuang Autonomous: China-ASEAN Collaborative Innovation Center for Regional Development.
The authors declare that the research was conducted in the absence of any commercial or financial relationships that could be construed as a potential conflict of interest.
All claims expressed in this article are solely those of the authors and do not necessarily represent those of their affiliated organizations, or those of the publisher, the editors and the reviewers. Any product that may be evaluated in this article, or claim that may be made by its manufacturer, is not guaranteed or endorsed by the publisher.
Ali, C., and Duemmel, M. (2019). The reluctant regulator: the rural utilities service and american broadband policy. Telecommun. Policy 43, 380–392. doi: 10.1016/j.telpol.2018.08.003
Ali Research Institute (n.d.). Ali Research Institute. http://www.aliresearch.com/EN/index (Accessed October 15, 2024).
An, C., He, X., and Zhang, L. (2023). The coordinated impacts of agricultural insurance and digital financial inclusion on agricultural output: evidence from China. Heliyon 9:e13546. doi: 10.1016/j.heliyon.2023.e13546
Balsa-Barreiro, J., Li, Y., Morales, A., and Pentland, A. S. (2019). Globalization and the shifting centers of gravity of world’s human dynamics: implications for sustainability. J. Clean. Prod. 239:117923. doi: 10.1016/j.jclepro.2019.117923
Becker, G. S. (1962). Investment in human capital: a theoretical analysis. J. Polit. Econ. 70, 9–49. doi: 10.1086/258724
Bokun, K., and Nazarko, J. (2023). Smart villages concept — a bibliometric analysis and state-of-the-art literature review. Prog. Plan. 175:100765. doi: 10.1016/j.progress.2023.100765
Bosworth, G., Whalley, J., Fuzi, A., Merrell, I., Chapman, P., and Russell, E. (2023). Rural co-working: new network spaces and new opportunities for a smart countryside. J. Rural. Stud. 97, 550–559. doi: 10.1016/j.jrurstud.2023.01.003
Chen, M., Chen, J., and Lai, S. (2006). Inventory analysis and spatial distribution of chinese agricultural and rural pollution. China Environ. Sci. 26, 751–755.
Chou, Y., Hao-Chun Chuang, H., and Shao, B. B. M. (2014). The impacts of information technology on total factor productivity: a look at externalities and innovations. Int. J. Prod. Econ. 158, 290–299. doi: 10.1016/j.ijpe.2014.08.003
Cowie, P., Townsend, L., and Salemink, K. (2020). Smart rural futures: will rural areas be left behind in the 4th industrial revolution? J. Rural. Stud. 79, 169–176. doi: 10.1016/j.jrurstud.2020.08.042
Deng, H., Zheng, W., Shen, Z., and Štreimikienė, D. (2023). Does fiscal expenditure promote green agricultural productivity gains: an investigation on corn production. Appl. Energy 334:120666. doi: 10.1016/j.apenergy.2023.120666
Diewert, W., Caves, D., and Christensen, L. (1982). The economic theory of index numbers and the measurement of input, output, and productivity. Econometrica 50, 1393–1414. doi: 10.2307/1913388
Ding, B. G., Zhao, Y., and Deng, J. H. (2022). Calculation, decoupling effects and driving factors of carbon emission from planting industry in China. Chin. J. Agric. Resour. Reg. Plan. 43, 1–11. doi: 10.7621/cjarrp.1005-9121.20220501
Du, X. Y., Zhang, J. N., Gao, X. L., and Ali, M. (2024). The impact of informatization on Agri-income of china’s rural farmers: ways for digital farming. Front. Sustain. Food Syst. 8:1329674. doi: 10.3389/fsufs.2024.1329674
Du, J. J., Zhang, Y. D., Liu, B. M., and Dong, R. H. (2023). Impact of digital village construction on agricultural green total factor productivity and its mechanisms. China Popul. Resour. Environ. 33, 165–175. doi: 10.12062/cpre.20221013
Dvorsky, J., Bednarz, J., and Blajer-Golebiewska, A. (2023). The impact of corporate reputation and social media engagement on the sustainability of smes: perceptions of top managers and the owners. Equilibrium 18, 779–811. doi: 10.24136/eq.2023.025
Falter, M., Jóhannesson, G. T., and Ren, C. (2023). Hacking hekla: exploring the dynamics of digital innovation in rural areas. Sociol. Rural. 63, 328–347. doi: 10.1111/soru.12412
Fan, C. C., and Scott, A. J. (2003). Industrial agglomeration and development: a survey of spatial economic issues in east asia and a statistical analysis of chinese regions. Econ. Geogr. 79, 295–319. doi: 10.1111/j.1944-8287.2003.tb00213.x
Fare, R., Grosskopf, S., Norris, M., and Zhang, Z. (1994). Productivity growth, technical progress, and efficiency change in industrialized countries. Am. Econ. Rev. 84, 66–83.
Fu, Z., Yao, S., Saen, R. F., Zhong, K., and Liu, Y. (2023). Impact of digital transformation on agricultural ecological efficiency: empirical findings from China. Environ. Dev. Sustain. doi: 10.1007/s10668-023-04245-2
Galperin, H., Katz, R., and Valencia, R. (2022). The impact of broadband on poverty reduction in rural Ecuador. Telematics Inform. 75:101905. doi: 10.1016/j.tele.2022.101905
Gao, Q., Cheng, C. M., Sun, G. L., and Li, J. F. (2022). The impact of digital inclusive finance on agricultural green total factor productivity: evidence from China. Front. Ecol. Evol. 10:905644. doi: 10.3389/fevo.2022.905644
Ge, P. F., Wang, S. J., and Huang, X. L. (2018). Measurement for china’s agricultural green tfp. China Popul. Resour. Environ. 28, 66–74. doi: 10.12062/cpre.20171010
Guo, H. (2024). Digital rural construction, misallocation of resource elements, and agricultural green total factor productivity. J. China Univ. Geosci. (Soc. Sci. Ed.) 24, 102–116. doi: 10.16493/j.cnki.42-1627/c.20231213.001
Guo, H., and Liu, X. (2020). Time-space evolution of china’s agricultural green total factor productivity. Chin. J. Manag. Sci 28, 66–75. doi: 10.16381/j.cnki.issn1003-207x.2020.09.007
Guo, F., Wang, J., Wang, F., Kong, T., Zhang, X., and Cheng, Z. (2020). Measuring china’s digital financial inclusion: index compilation and spatial characteristics. China Econ. Quart. 19, 1401–1418. doi: 10.13821/j.cnki.ceq.2020.03.12
He, S., Haifeng Liao, F., and Li, G. (2019). A spatiotemporal analysis of county economy and the multi-mechanism process of regional inequality in rural China. Appl. Geogr. 111:102073. doi: 10.1016/j.apgeog.2019.102073
Helpman, E. (1992). Endogenous macroeconomic growth theory. Eur. Econ. Rev. 36, 237–267. doi: 10.1016/0014-2921(92)90083-9
Hong, X., Chen, Q., and Wang, N. (2024). The impact of digital inclusive finance on the agricultural factor mismatch of agriculture-related enterprises. Financ. Res. Lett. 59:104774. doi: 10.1016/j.frl.2023.104774
Hou, G., Delang, C. O., and Lu, X. (2020). Afforestation changes soil organic carbon stocks on sloping land: the role of previous land cover and tree type. Ecol. Eng. 152:105860. doi: 10.1016/j.ecoleng.2020.105860
Hu, Y., Liu, C., and Peng, J. (2021). Financial inclusion and agricultural total factor productivity growth in China. Econ. Model. 96, 68–82. doi: 10.1016/j.econmod.2020.12.021
Huang, X., Feng, C., Qin, J., Wang, X., and Zhang, T. (2022). Measuring china’s agricultural green total factor productivity and its drivers during 1998–2019. Sci. Total Environ. 829:154477. doi: 10.1016/j.scitotenv.2022.154477
Institute of Digital Finance Peking University (n.d.). Series of digital finance indexes. Available at: https://en.idf.pku.edu.cn/achievements/seriesofdigitafinanceindexes/index.htm (Accessed October 15, 2024).
IPCC (2007). Climate change 2007: Mitigation: Contribution of working group iii to the fourth assessment report of the intergovernmental panel on climate change: Summary for policymakers and technical summary. Cambridge: Cambridge University Press.
Irwansyah, (2020). The social contractual utilitarianism of a digital village in rural Indonesia. Technol. Soc. 63:101354. doi: 10.1016/j.techsoc.2020.101354
Ji, K. W., Hou, Q. Y., Yu, Y., and Pan, D. (2024). Rural e-commerce and agricultural carbon emission reduction: a quasi-natural experiment from china’s rural e-commerce demonstration county program based on 355 cities in ten years. Agriculture-Basel 14:75. doi: 10.3390/agriculture14010075
Ji, X., Xu, J., and Zhang, H. (2023). Environmental effects of rural e-commerce: a case study of chemical fertilizer reduction in China. J. Environ. Manag. 326:116713. doi: 10.1016/j.jenvman.2022.116713
Jiang, Y., Han, G., and Yu, D. (2024). Digital finance and agricultural green total factor productivity: the mediating role of digital village development. Financ. Res. Lett. 67:105948. doi: 10.1016/j.frl.2024.105948
Jiang, Q., Li, J. Z., Si, H. Y., and Su, Y. Y. (2022). The impact of the digital economy on agricultural green development: evidence from China. Agriculture-Basel 12:1107. doi: 10.3390/agriculture12081107
Jin, S. R., and Ren, Z. J. (2022). The impact of rural digitalization on agricultural green total factor productivity. Reformation 346, 102–118.
Jose, V. R. R., and Winkler, R. L. (2008). Simple robust averages of forecasts: some empirical results. Int. J. Forecast. 24, 163–169. doi: 10.1016/j.ijforecast.2007.06.001
Klerkx, L., Jakku, E., and Labarthe, P. (2019). A review of social science on digital agriculture, smart farming and agriculture 4.0: new contributions and a future research agenda. NJAS-Wagen J. Life Sci., 90–91. doi: 10.1016/j.njas.2019.100315
Kliestik, T., Kral, P., Bugaj, M., and Ďurana, P. (2024). Generative artificial intelligence of things systems, multisensory immersive extended reality technologies, and algorithmic big data simulation and modelling tools in digital twin industrial metaverse. Equilib. Quart. J. Econ. Econ. Policy 19, 429–461. doi: 10.24136/eq.3108
Kliestik, T., Nica, E., Durana, P., and Popescu, G. H. (2023). Artificial intelligence-based predictive maintenance, time-sensitive networking, and big data-driven algorithmic decision-making in the economics of industrial internet of things. Oecon. Copernic. 14, 1097–1138. doi: 10.24136/oc.2023.033
Kopf, D. A. (2007). Endogenous growth theory applied: strategies for University R&D. J. Bus. Res. 60, 975–978. doi: 10.1016/j.jbusres.2007.01.022
Kou, Z., and Liu, X. (2017). Find report on city and industrial innovation in China (2017). Fudan Institute of Industrial Development, School of Economics, Fudan University.
Lai, S., Du, P. F., and Chen, J. N. (2004). Evaluation of non-point source pollution based on unit analysis. J. Tsinghua Univ. 44, 1184–1187. doi: 10.16511/j.cnki.qhdxxb.2004.09.009
Li, Y. (2024). How does the development of rural broadband in China affect agricultural total factor productivity? Evidence from agriculture-related loans. Front. Sustain. Food Syst 8:1332494. doi: 10.3389/fsufs.2024.1332494
Li, G., Jia, X., Khan, A. A., Khan, S. U., Ali, M. A. S., and Luo, J. (2023). Does green finance promote agricultural green total factor productivity? Considering green credit, green investment, green securities, and carbon finance in China. Environ. Sci. Pollut. Res. 30, 36663–36679. doi: 10.1007/s11356-022-24857-x
Liu, Y., Dai, Z., and Zhao, X. (2024). Unveiling the blueprint for rural digital prosperity: a comparative examination of top 100 digital counties in China. Technol. Forecast. Soc. Chang. 208:123625. doi: 10.1016/j.techfore.2024.123625
Liu, D., Li, Y., You, J., Balezentis, T., and Shen, Z. (2023). Digital inclusive finance and green total factor productivity growth in rural areas. J. Clean. Prod. 418:138159. doi: 10.1016/j.jclepro.2023.138159
Liu, W. W., Liu, Z. W., Wang, L., Liu, H. M., and Wang, Y. (2022). Regional social development gap and regional coordinated development based on mixed-methods research: evidence from China. Front. Psychol. 13:927011. doi: 10.3389/fpsyg.2022.927011
Liu, Y., Liu, S., Ye, D., Tang, H., and Wang, F. (2022). Dynamic impact of negative public sentiment on agricultural product prices during covid-19. J. Retail. Consum. Serv. 64:102790. doi: 10.1016/j.jretconser.2021.102790
Liu, Y., Ouyang, Y., and Cai, H. (2021). Evaluation of china’s agricultural green tfp and its spatiotemporal evolution characteristics. J. Quant. Tech. Econ. 38:39. doi: 10.13653/j.cnki.jqte.2021.05.003
Liu, Z., Zhang, M., Li, Q., and Zhao, X. (2023). The impact of green trade barriers on agricultural green total factor productivity: evidence from China and oecd countries. Econ. Anal. Policy 78, 319–331. doi: 10.1016/j.eap.2023.03.011
Liu, S. Y., Zhu, S. P., Hou, Z. P., and Li, C. G. (2023). Digital village construction, human capital and the development of the rural older adult care service industry. Front. Public Health 11:1190757. doi: 10.3389/fpubh.2023.1190757
Liu, D., Zhu, X., and Wang, Y. (2021). China’s agricultural green total factor productivity based on carbon emission: an analysis of evolution trend and influencing factors. J. Clean. Prod. 278:123692. doi: 10.1016/j.jclepro.2020.123692
Lu, F., Meng, J., and Cheng, B. (2024). How does improving agricultural mechanization affect the green development of agriculture? Evidence from China. J. Clean. Prod. 472:143298. doi: 10.1016/j.jclepro.2024.143298
Lu, S., Zhuang, J., Sun, Z., and Huang, M. (2024). How can rural digitalization improve agricultural green total factor productivity: empirical evidence from counties in China. Heliyon 10:e35296. doi: 10.1016/j.heliyon.2024.e35296
Luo, J., Huang, M., Hu, M., and Bai, Y. (2023). How does agricultural production agglomeration affect green total factor productivity?: empirical evidence from China. Environ. Sci. Pollut. Res. 30, 67865–67879. doi: 10.1007/s11356-023-27106-x
Luo, Y. P., Wu, J. X., and Xu, Y. (2022). Can self-governance tackle the water commons?-causal evidence of the effect of rural water pollution treatment on farmers? Health in China. Ecol. Econ. 198:107471. doi: 10.1016/j.ecolecon.2022.107471
Malmquist, S. (1953). Index numbers and indifference surfaces. Trab. Estad. 4, 209–242. doi: 10.1007/BF03006863
Markard, J., Hekkert, M., and Jacobsson, S. (2015). The technological innovation systems framework: response to six criticisms. Environ. Innov. Soc. Trans. 16, 76–86. doi: 10.1016/j.eist.2015.07.006
Marousek, J. (2014). Novel technique to enhance the disintegration effect of the pressure waves on oilseeds. Ind. Crop. Prod. 53, 1–5. doi: 10.1016/j.indcrop.2013.11.048
Maroušek, J. (2023). Aluminum nanoparticles from liquid packaging board improve the competitiveness of (bio)diesel. Clean Techn. Environ. Policy 25, 1059–1067. doi: 10.1007/s10098-022-02413-y
Maroušek, J., Gavurová, B., Strunecký, O., Maroušková, A., Sekar, M., and Marek, V. (2023a). Techno-economic identification of production factors threatening the competitiveness of algae biodiesel. Fuel 344:128056. doi: 10.1016/j.fuel.2023.128056
Marousek, J., Itoh, S., Higa, O., Kondo, Y., Ueno, M., Suwa, R., et al. (2012). The use of underwater high-voltage discharges to improve the efficiency of Jatropha curcas l. Biodiesel production. Biotechnol. Appl. Biochem. 59, 451–456. doi: 10.1002/bab.1045
Marousek, J., Itoh, S., Higa, O., Kondo, Y., Ueno, M., Suwa, R., et al. (2013). Pressure shockwaves to enhance oil extraction from Jatropha curcas l. Biotechnol. Biotechnol. Equip. 27, 3654–3658. doi: 10.5504/BBEQ.2012.0143
Maroušek, J., Kolář, L., Strunecký, O., Kopecký, M., Bartoš, P., Maroušková, A., et al. (2020). Modified biochars present an economic challenge to phosphate management in wastewater treatment plants. J. Clean. Prod. 272:123015. doi: 10.1016/j.jclepro.2020.123015
Maroušek, J., and Maroušková, A. (2021). Economic considerations on nutrient utilization in wastewater management. Energies 14:3468. doi: 10.3390/en14123468
Maroušek, J., Maroušková, A., Gavurová, B., and Minofar, B. (2023b). Techno-economic considerations on cement substitute obtained from waste refining. J. Clean. Prod. 412:137326. doi: 10.1016/j.jclepro.2023.137326
Marousek, J., Strunecky, O., and Marouskova, A. (2023c). Insect rearing on biowaste represents a competitive advantage for fish farming. Rev. Aquac. 15, 965–975. doi: 10.1111/raq.12772
Metzker, Z., Maroušek, J., Hlawiczka, R., Belás, J., and Khan, K. (2021). The perception of the market and operational area of business by service sector and tourism companies in terms of CSR implementation. J. Tour. Serv. 12, 217–236. doi: 10.29036/jots.v12i23.328
National Bureau of Statistics of China (n.d.). National bureau of statistics of China. Available at: https://data.stats.gov.cn/english/ (Accessed June 22, 2024).
Pang, Y. N., and Wang, Q. (2023). Inclusiveness and competitiveness performance of digital financial inclusion: from the perspective of regional inequity. J. Compet. 15, 53–68. doi: 10.7441/joc.2023.04.04
Pant, L. P., and Hambly Odame, H. (2017). Broadband for a sustainable digital future of rural communities: a reflexive interactive assessment. J. Rural. Stud. 54, 435–450. doi: 10.1016/j.jrurstud.2016.09.003
Papadopoulos, G., Arduini, S., Uyar, H., Psiroukis, V., Kasimati, A., and Fountas, S. (2024). Economic and environmental benefits of digital agricultural technologies in crop production: a review. Smart Agric. Tech. 8:100441. doi: 10.1016/j.atech.2024.100441
Platania, F., Hernandez, C. T., and Arreola, F. (2022). Social media communication during natural disasters and the impact on the agricultural market. Technol. Forecast. Soc. Chang. 179:121594. doi: 10.1016/j.techfore.2022.121594
Ren, X., He, J., and Huang, Z. (2023). Innovation, natural resources abundance, climate change and green growth in agriculture. Res. Policy 85:103970. doi: 10.1016/j.resourpol.2023.103970
Rosenbaum, P. R., and Rubin, D. B. (1983). The central role of the propensity score in observational studies for causal effects. Biometrika 70, 41–55. doi: 10.1093/biomet/70.1.41
Sakariyahu, R., Fagbemi, T., Adigun, R., Lawal, R., Seyingbo, O., and Oyekola, O. (2024). Severity of environmental degradation and the impact on quality of life in africa. J. Environ. Manag. 356:120537. doi: 10.1016/j.jenvman.2024.120537
Salemink, K., Strijker, D., and Bosworth, G. (2017). Rural development in the digital age: a systematic literature review on unequal ict availability, adoption, and use in rural areas. J. Rural. Stud. 54, 360–371. doi: 10.1016/j.jrurstud.2015.09.001
Samusevych, Y., Maroušek, J., Kuzmenko, O. V., Streimikis, J., and Vysochyna, A. (2021). Environmental taxes in ensuring national security: a structural optimization model. J. Int. Stud. 14, 292–312. doi: 10.14254/2071-8330.2021/14-2/19
Schmit, T. M., and Severson, R. M. (2021). Exploring the feasibility of rural broadband cooperatives in the United States: the new new deal? Telecommun. Policy 45:102114. doi: 10.1016/j.telpol.2021.102114
Sharma, R., Kamble, S. S., Gunasekaran, A., Kumar, V., and Kumar, A. (2020). A systematic literature review on machine learning applications for sustainable agriculture supply chain performance. Comput. Oper. Res. 119:104926. doi: 10.1016/j.cor.2020.104926
Shen, Z. Y., Hong, T. Y., Blancard, S., and Bai, K. X. (2024). Digital financial inclusion and green growth: analysis of chinese agriculture. Appl. Econ. 56, 5555–5573. doi: 10.1080/00036846.2023.2257038
Shen, K., Lin, J., and Fu, Y. (2023). Network infrastructure construction, information accessibility and the innovation boundaries of enterprises. China Indust. Econ. 418, 57–75. doi: 10.19581/j.cnki.ciejournal.2023.01.014
Shen, Z., Wang, S., Boussemart, J., and Hao, Y. (2022). Digital transition and green growth in chinese agriculture. Technol. Forecast. Soc. Chang. 181:121742. doi: 10.1016/j.techfore.2022.121742
Shipman, J. E., Swanquist, Q. T., and Whited, R. L. (2017). Propensity score matching in accounting research. Accoun. Rev. Account. Rev. 92, 213–244. doi: 10.2308/accr-51449
Solow, R. M. (1999). “Chapter 9 neoclassical growth theory” in Handbook of macroeconomics (Elsevier), 637–667.
Song, Y., Zhang, B., Wang, J., and Kwek, K. (2022). The impact of climate change on china’s agricultural green total factor productivity. Technol. Forecast. Soc. Chang. 185:122054. doi: 10.1016/j.techfore.2022.122054
Stávková, J., and Maroušek, J. (2021). Novel sorbent shows promising financial results on p recovery from sludge water. Chemosphere 276:130097. doi: 10.1016/j.chemosphere.2021.130097
Sun, S. H., Liu, C. M., and Chen, X. N. (2023). Digital village, network spillover and agricultural green total factor productivity. Chin. J. Agric. Resour. Reg. Plan. 44, 45–59. doi: 10.7621/cjarrp.1005-9121.20230906
Sun, T., and Zhang, Y. (2021). The impact of environmental regulation on agricultural green total factor productivity: evidence from China. Int. J. Environ. Pollut. 70, 203–222. doi: 10.1504/IJEP.2021.132025
Tang, M., Cao, A., Guo, L., and Li, H. (2023). Improving agricultural green total factor productivity in China: do environmental governance and green low-carbon policies matter? Environ. Sci. Pollut. Res. 30, 52906–52922. doi: 10.1007/s11356-023-26090-6
Tang, L., and Sun, S. (2022). Fiscal incentives, financial support for agriculture, and urban-rural inequality. Int. Rev. Financ. Anal. 80:102057. doi: 10.1016/j.irfa.2022.102057
Tone, K. (2001). A slacks-based measure of efficiency in data envelopment analysis. Eur. J. Oper. Res. 130, 498–509. doi: 10.1016/S0377-2217(99)00407-5
Tone, K. (2002). A slacks-based measure of super-efficiency in data envelopment analysis. Eur. J. Oper. Res. 143, 32–41. doi: 10.1016/S0377-2217(01)00324-1
Tone, K., and Tsutsui, M. (2010). An epsilon-based measure of efficiency in dea – a third pole of technical efficiency. Eur. J. Oper. Res. 207, 1554–1563. doi: 10.1016/j.ejor.2010.07.014
Valaskova, K., Nagy, M., and Grecu, G. (2024). Digital twin simulation modeling, artificial intelligence-based internet of manufacturing things systems, and virtual machine and cognitive computing algorithms in the industry 4.0-based slovak labor market. Oecon. Copernic. doi: 10.24136/oc.2814
Vochozka, M., Horák, J., Krulicky, T., and Pardal, P. (2020a). Predicting future Brent oil price on global markets. Acta Montan. Slovaca 25, 375–392. doi: 10.46544/AMS.v25i3.10
Vochozka, M., Rowland, Z., Suler, P., and Marousek, J. (2020b). The influence of the international price of oil on the value of the eur/usd exchange rate. J. Compet. 12, 167–190. doi: 10.7441/joc.2020.02.10
Vu, K. M. (2013). Information and communication technology (ict) and singapore’s economic growth. Inf. Econ. Policy 25, 284–300. doi: 10.1016/j.infoecopol.2013.08.002
Wang, H., Fang, L., Mao, H., and Chen, S. J. (2022). Can e-commerce alleviate agricultural non-point source pollution? - a quasi-natural experiment based on a china’s e-commerce demonstration city. Sci. Total Environ. 846:157423. doi: 10.1016/j.scitotenv.2022.157423
Wang, W., Xu, H., and Liu, Y. (2022). Platform ruralism: digital platforms and the techno-spatial fix. Geoforum 131, 12–19. doi: 10.1016/j.geoforum.2022.02.009
Wang, Y., Xu, F., and Ye, X. (2021). Can digital village construction boost rural consumption upgrading? Manag. Rev. 33:135. doi: 10.14120/j.cnki.cn11-5057/f.2021.11.017
Wang, J., Yang, G., and Zhou, C. (2024). Does internet use promote agricultural green development? Evidence from China. Int. Rev. Econ. Financ. 93, 98–111. doi: 10.1016/j.iref.2024.03.009
Wu, J. (2024). The sword of damocles: understanding the carbon abatement effects of top-down environmental management practices -- insights from china’s campaign-style governance. J. Environ. Manag. 354:120306. doi: 10.1016/j.jenvman.2024.120306
Xia, X., Chen, Z., Zhao, H., and Zhang, M. (2019). Agricultural high-quality development: digital empowerment and implementation path. Chin. Rural Econ. 12, 2–15.
Xiao, W., and Du, Z. (2019). Integration of rural primary, secondary and tertiary industries: kernel interpretation of connotation, development status and future ideas. J. Northwest AF Univ. 19, 120–129. doi: 10.13968/j.cnki.1009-9107.2019.06.14
Xiao, J. F., Zhou, Y., and Zhang, L. (2015). Contributions of natural and human factors to increases in vegetation productivity in China. Ecosphere 6, 1–20. doi: 10.1890/ES14-00394.1
Xie, H., Wu, X., and Yang, L. (2023). Impact of rural inclusive finance on green total factor productivity in agriculture: from the perspective of spatial spillover effect. J. Chin. Agric. Mech. 44:239. doi: 10.13733/j.jcam.issn.2095-5553.2023.04.033
Xiong, H., Zhan, J., Xu, Y., Zuo, A., and Lv, X. (2023). Challenges or drivers? Threshold effects of environmental regulation on china’s agricultural green productivity. J. Clean. Prod. 429:139503. doi: 10.1016/j.jclepro.2023.139503
Yang, J., Chen, L. M., and Zhang, Y. D. (2023). Rural industrial convergence, urbanization development, and farmers’ income growth - evidence from the Chinese experience. Front. Sustain. Food Syst. 7:1237844. doi: 10.3389/fsufs.2023.1237844
Yang, C., Ji, X., Cheng, C., Liao, S., Obuobi, B., and Zhang, Y. (2024). Digital economy empowers sustainable agriculture: implications for farmers’ adoption of ecological agricultural technologies. Ecol. Indic. 159:111723. doi: 10.1016/j.ecolind.2024.111723
Yang, X., and Tong, J. (2023). Spatial spillover effects of agricultural product trade on agricultural green total factor productivitybased on the regulatory role of agricultural industrial agglomeration. J. China Agric. Resour. Reg. Plan. 44, 15–27. doi: 10.7621/cjarrp.1005-9121.20231102
Yang, R., Zhong, C., Yang, Z., Yang, S., Ji, G., Yu, J., et al. (2024). Study on china’s farmland–grain resource curse: empirical testing based on 31 provinces and 2843 counties. Land Use Policy 144:107241. doi: 10.1016/j.landusepol.2024.107241
Yin, H., Huo, P., and Wang, S. (2020). Agricultural and rural digital transformation: realistic representation. Impact Mechan. Promot. Strateg. Ref. 12, 48–56.
Zhang, H., Du, K. W., and Jin, B. Y. (2020). Research on evaluation of digital rural development readiness under rural revitalization strategy. J. Xi’an Univ. Financ. Econ 33, 51–60. doi: 10.19331/j.cnki.jxufe.2020.01.007
Zhou, F., and Deng, H. (2023). Creation or disruption? Doubts from the internet applications in china’s rural sector. J. Innov. Knowl. 8:100450. doi: 10.1016/j.jik.2023.100450
Zhou, X., and Zhang, Y. (2024). Administration or marketization: environmental regulation, marketization and agricultural green total factor productivity. J. Environ. Manag. 370:122433. doi: 10.1016/j.jenvman.2024.122433
Zhu, H., and Chen, H. (2022). Level measurement, spatial-temporal evolution and promotion path of digital village development in China. Agric. Econ. 2022:7. doi: 10.13246/j.cnki.iae.20220728.001
Zhu, H., and Chen, H. (2023). Measurement, spatial-temporal evolution and promotion path of digital development in China. Issues Agric. Econ. 3, 21–33.
Zscheischler, J., Brunsch, R., Rogga, S., and Scholz, R. W. (2022). Perceived risks and vulnerabilities of employing digitalization and digital data in agriculture – socially robust orientations from a transdisciplinary process. J. Clean. Prod. 358:132034. doi: 10.1016/j.jclepro.2022.132034
Keywords: digital rural development, agricultural green total factor productivity, sustainable development, fixed effects analysis, green transformation of agriculture, high-quality development
Citation: Zeng F, Zhou Y and Wei B (2024) Empowering sustainable development: revolutionizing agricultural green total factor productivity through rural digitalization. Front. Sustain. Food Syst. 8:1455732. doi: 10.3389/fsufs.2024.1455732
Received: 01 July 2024; Accepted: 21 October 2024;
Published: 04 December 2024.
Edited by:
Jose Balsa Barreiro, Massachusetts Institute of Technology, United StatesReviewed by:
Abbas Ali Chandio, Guizhou University, ChinaCopyright © 2024 Zeng, Zhou and Wei. This is an open-access article distributed under the terms of the Creative Commons Attribution License (CC BY). The use, distribution or reproduction in other forums is permitted, provided the original author(s) and the copyright owner(s) are credited and that the original publication in this journal is cited, in accordance with accepted academic practice. No use, distribution or reproduction is permitted which does not comply with these terms.
*Correspondence: Bin Wei, d2VpYmluQGd4dS5lZHUuY24=
Disclaimer: All claims expressed in this article are solely those of the authors and do not necessarily represent those of their affiliated organizations, or those of the publisher, the editors and the reviewers. Any product that may be evaluated in this article or claim that may be made by its manufacturer is not guaranteed or endorsed by the publisher.
Research integrity at Frontiers
Learn more about the work of our research integrity team to safeguard the quality of each article we publish.