- 1School of Management, Dalian Polytechnic University, Dalian, Liaoning, China
- 2School of Business Administration, Zhongnan University of Economics and Law, Wuhan, Hubei, China
Considering agriculture's substantial carbon emissions, it is essential to examine how the digital economy can stimulate green innovation and low-carbon development by impacting these emissions. This article employs panel data from 31 provinces in China, covering the period from 2011 to 2020, to empirically analyze the effects of digital economy development on agricultural carbon emissions. The results indicate that: (1) Agricultural green total factor productivity has a partial mediating effect in the impact of the digital economy on agricultural carbon emissions, and green finance has a negative regulatory effect. (2) Green finance positively regulates the inhibitory effect of agricultural green total factor productivity on agricultural carbon emissions. (3) The development of the digital economy can inhibit agricultural carbon emissions, and this negative inhibitory effect follows a “U” shape. Our research offers a scientific foundation for the promotion of green and low-carbon development within Chinese agriculture, while also providing valuable references and insights for the global integration and advancement of agricultural emissions reduction alongside the digital economy.
1 Introduction
Agriculture is an significant contributor to carbon emissions, yet it also plays a crucial role in achieving peak carbon neutrality. China is a big agricultural country with a long history of agricultural production and planting. Under the traditional agricultural production model, problems such as the use of fertilizers and pesticides in agricultural production have led to more agricultural carbon emissions, resulting in a series of climate issues. Consequently, reducing carbon emissions and promoting low-carbon development have become primary objectives in contemporary agricultural policies. Document No. 1 of the CPC Central 2023 continues to focus on the development of green agriculture in the overall promotion of rural revitalization. In this context, the rapid development of the digital economy emerges as a vital driver for agricultural carbon emission reduction. Factors such as the enhancement of green total factor productivity and the expansion of green finance also play essential roles in facilitating this reduction. Therefore, as efforts to establish a robust agricultural nation intensify, the pursuit of low-carbon agricultural development must be supported by advancements in the digital economy, improvements in green total factor productivity, and the promotion of green finance. The selection of China as the subject of the study is of great value and significance globally. China's expansive and diverse agricultural sector, along with its innovative approaches to green and low-carbon policies, positions it as a crucial player in global climate change mitigation. The rapid development of its digital economy and its application in agriculture serves as a model for the integration of the digital economy and agricultural practices worldwide. Thus, analyzing China as a case study not only aids in advancing the green transformation of its agriculture but also offers valuable insights for global strategies that seek to align agricultural emission reductions with digital economy development.
The academics' research on the relationship between the digital economy, agricultural green total factor productivity, green finance and agricultural carbon emissions focuses on the following.
The first is the impact of the digital economy on agricultural carbon emissions. Liu and Xu (2024) found that the impact of the digital economy on carbon emissions varies in different regions and at different levels of carbon emissions. Wang and Mei (2024) used a spatial Durbin model to empirically demonstrate that with the evolution of the digital economy, agricultural carbon emissions have significantly decreased. The second is the impact of agricultural green total factor productivity on agricultural carbon emissions. Wang et al. (2023) found that the agricultural green total factor productivity in the Dongting Lake area is relatively high, and the total agricultural carbon emissions are in a declining state. Xu et al. (2019) constructed a global Malmquist Luenberger index to consider agricultural carbon emissions as an unexpected output, and measured agricultural development efficiency based on green total factor productivity. The third is the impact of green finance on agricultural carbon emissions. Li et al. (2024) empirically demonstrated that green finance and green innovation can suppress carbon emissions. Wang et al. (2024) used spatial effects and found that improving the level of green finance can significantly suppress carbon emissions in the Pearl River Delta region. The fourth is the impact of the digital economy on the green total factor productivity of agriculture. Chen et al. (2023) concluded using a fixed effects model that the digital economy can enhance agricultural total factor productivity and that green technology innovation positively regulates the relationship between the digital economy and agricultural green total factor productivity. Zhang et al. (2023) believe that the development of the digital economy has a significant positive effect on improving agricultural GTFP. The fifth is the regulatory effect of green finance. The research by Che and Shen (2022) shows that green finance promotes the integration of digital technology with traditional agriculture by providing financial support for agricultural green technology, thereby improving agricultural green total factor productivity. He (2024) green finance promotes the improvement of green total factor productivity of enterprises and suppresses carbon emissions by enhancing the efficiency of green technology innovation and optimizing resource allocation structure.
While domestic and international scholars have achieved significant advancements in the areas of digital economy, agricultural carbon emissions, agricultural green total factor productivity, and green finance, further research is needed. Few studies integrate these elements into a single framework, particularly regarding the mediating role of green total factor productivity and the regulatory role of green finance. This article argues for the necessity of expanding research in this area and develops a mediating effect model to analyze the direct impact of the digital economy on agricultural carbon emissions, as well as the mediating effect of agricultural green total factor productivity and the regulatory influence of green finance.
2 Literature review and hypothesis development
2.1 The direct utility mechanism of digital economy to agricultural carbon emissions
The digital economy affects agricultural carbon emissions through the following aspects: First, the digital economy can enhance agricultural production processes and optimize resource allocation efficiency. By improving production and transportation logistics, the digital economy facilitates more effective information transmission, reduces the costs associated with information collection and transactions, and fosters a more rational structure within the agricultural industry, ultimately enhancing agricultural carbon emission efficiency (Jin and Xu, 2022; Goldfarb and Tucker, 2019). Second, the digital economy can advance agricultural technological innovation. As a new economic paradigm, the development of the digital economy drives the continuous evolution of agricultural technologies and facilitates the transition from traditional to green agriculture, thereby improving resource utilization efficiency and enhancing productivity while maintaining the same input levels, which in turn increases carbon emission efficiency. Third, the deep integration of the digital economy across various industries and sectors fosters the creation of digital platforms. These platforms indirectly reduce agricultural carbon emissions by consolidating technology, information, and resources, enabling farmers to stay informed about agricultural practices and enhance resource efficiency, agricultural carbon emission efficiency. Therefore, hypothesis 1 is proposed.
Hypothesis 1: the development of digital economy has a significant negative impact on agricultural carbon emissions.
2.2 The intermediary mechanism of green total factor productivity in agriculture
The enhancement of agricultural green total factor productivity (GTFP) is crucial for promoting sustainable agricultural development and mitigating agricultural carbon emissions. Existing literature indicates that improvements in GTFP facilitate the flow of various states within regional green total factor productivity, optimizing resource allocation and increasing energy utilization efficiency, thereby leading to a reduction in agricultural carbon emissions. Furthermore, advancements in agricultural GTFP also serve as a catalyst for the influence of the digital economy on agricultural carbon emissions. The rapid evolution of digital technology enables the continuous integration of data as a production factor within agricultural production and management practices, fostering the synergy between data and traditional production inputs. By thoroughly considering the input of production factors alongside energy resource consumption, the widespread application of green production technologies has markedly improved GTFP and, in turn, contributed to the indirect reduction of agricultural carbon emissions. Moreover, enhancing agricultural GTFP raises awareness of green and sustainable development principles, thereby minimizing resource waste and further promoting agricultural carbon reduction initiatives. Therefore, hypotheses 2 and 3 are proposed.
Hypotheses 2: The improvement of agricultural green total factor productivity has a significant negative inhibitory effect on agricultural carbon emissions.
Hypotheses 3: Agricultural green total factor productivity plays an intermediary role in the impact of digital economy on agricultural carbon emissions.
2.3 The mechanism of green finance on agricultural carbon emissions
As a critical instrument for rural financing and credit relationship management, green finance serves as a significant financial leverage in the advancement of low-carbon agriculture. Increased financial support for low-carbon agricultural technology innovation not only fosters the progress of green technologies but also reduces energy consumption, which indirectly contributes to the reduction of agricultural carbon emissions. Furthermore, green finance facilitates the development of green agriculture by alleviating financing pressures. By providing essential financial support, green finance enhances the efficiency of agricultural financing, promotes the green transformation of agricultural practices, and aids in the transition from traditional to sustainable agriculture, thereby minimizing energy waste and further decreasing agricultural carbon emissions. Additionally, green finance plays a vital role in risk sharing for the development of green agriculture, thereby enhancing the engagement of principal agricultural entities. During the transition from conventional to green agricultural practices, stakeholders—including cooperatives and family farms—face various potential risks. The development of green finance encourages those with lower risk tolerance to adopt and integrate low-carbon agricultural technologies effectively. Therefore, hypothesis 4 is proposed.
Hypotheses 4: The development of green finance has a significant negative inhibitory effect on agricultural carbon emissions.
2.4 The regulatory effect of green finance
Green finance is a vital tool for rural financing and credit management, playing a significant role in advancing low-carbon agriculture. By providing increased financial support for low-carbon agricultural technology innovations, green finance fosters green technology development and reduces energy consumption, thereby indirectly lowering agricultural carbon emissions. Additionally, green finance alleviates financing pressures for the development of green agriculture, improving the efficiency of agricultural financing and aiding the transition from traditional to sustainable practices. This transition minimizes energy waste and further decreases carbon emissions. Moreover, green finance enhances risk-sharing for agricultural stakeholders, encouraging entities such as cooperatives and family farms to adopt low-carbon technologies during their shift to green agriculture, particularly those with lower risk tolerance Therefore, hypotheses 5 and 6 are proposed.
Hypotheses 5: Green finance plays a regulatory role in the impact of the digital economy on the total factor productivity of green agriculture.
Hypotheses 6: Green finance plays a moderating role in the impact of agricultural green total factor productivity on agricultural carbon emissions.
Based on the above assumptions, the research framework of this paper is shown in Figure 1.
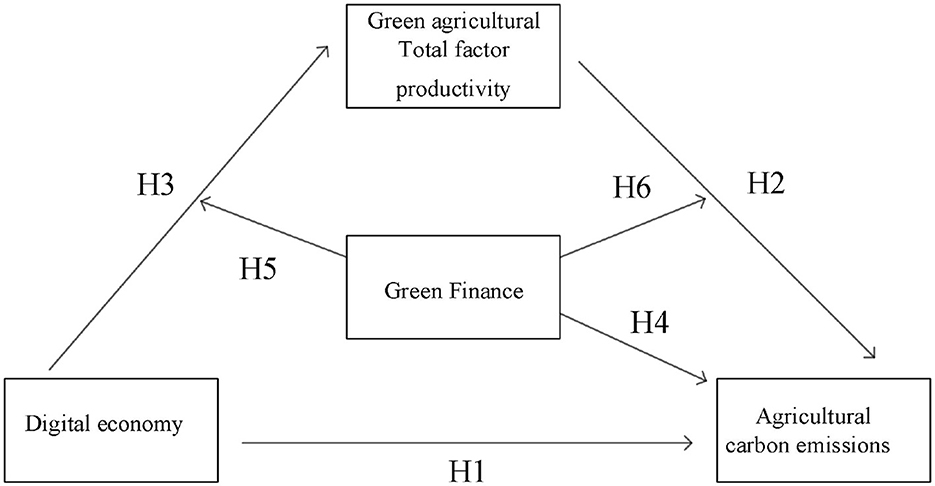
Figure 1. Assumes model (Zhao et al., 2021).
3 Research design
3.1 Model setting
3.1.1 Benchmark model and mediating effect model
In order to explore the direct effect of the digital economy on agricultural carbon emissions and the mediating effect of green total factor productivity in agriculture, this paper draws on the research of Xie et al. (2022) and He et al. (2021) by constructing a model of mediating effects, to test the mediating effect of green total factor productivity. The construction model is as follows:
In this context, i and n respectively represents the province and the year; CIin represents the agricultural carbon emissions of i province in n years, DEDIin represents the digital economic development level of i province in n years, GTFPin represents the agricultural green total factor productivity of i province in n years; Xin is the control variable group, including industrial structure (IS), economic development level (GDP), urbanization level (URBAN), government intervention level (GID), innovation level (INN); εin, θin, μin are random perturbation terms; α0, β0, γ0 are constant terms.
Equation 1 is the total effect model of DEDI on CI, and α1 is the total effect level. If α1 is significant, the total effect of DEDI on CI is significant, Equation 2 is the direct effect model of DEDI on GTFP, and β1 is the direct effect level of both. If β1 is significant, the direct effect of DEDI on GTFP is significant. Equation 3 is the direct effect model of DEDI on CI, γ1 is the direct effect level of DEDI on CI, and γ2 is the direct effect level of GTFP on CI. If the coefficient of γ1 is not significantly reduced or reduced, when the γ2 is significant, GTP acts as a complete or partial mediator.
3.1.2 Regulatory effect model
To test whether the digital economy has an impact on the green total factor productivity of agriculture through the role of green finance, and whether agricultural green finance has an impact on agricultural carbon emissions through the role of green finance, this paper draws on the research of Jiang (2022), and adds the interaction terms of digital economy and green finance (DEDI×GFI) and green total factor productivity and green finance (GTFP×GFI) on the basis of the benchmark model (1), the following models of moderating effects were constructed:
Among them, b3 reflects the regulatory role of green finance in adjusting the impact of digital economy on green total factor productivity; c3 reflects the regulatory role of green finance in adjusting the impact of green total factor productivity on agricultural carbon emissions.
3.2 Select variables
3.2.1 The interpreted variable
This article defines the explanatory variable as the intensity of Agricultural carbon emissions (CI). The calculation method used in this article is based on the coefficient indicators in IPCC and the method proposed by Li and Zhang (2012), and the following formula for calculating agricultural carbon emissions is established:
Among them, Ci is the total carbon emissions from agriculture, is the carbon emissions from various carbon sources, Ti is the carbon emissions from various carbon sources, δi carbon emission coefficients from various carbon sources are shown in Table 1. After calculating the total agricultural carbon emissions of each province, divided by the total agricultural output value of each province, the agricultural carbon emission intensity CI of each province (10,000 tons/100 million yuan) was obtained.
According to the Equation 4, we can calculate the total agricultural carbon emissions of each province (city and district) from 2011 to 2020, divide the total agricultural output value of each province, and get the agricultural carbon emission intensity of each province as shown in Figure 2. The top 10 provinces were Jilin, Inner Mongolia, Anhui, Gansu, Ningxia, Xinjiang, Xizang, Shanxi, Hebei, and Tianjin, there are five in Gharbia Governorate, three in Midlands Province and two in Eastern Province, mainly in the less developed areas of western China.
3.2.2 Core explanatory variables
The core explanatory variable is the level of igital economy development (DEDI). Based on the research of Zhao et al. (2020) and Sidorov and Sencehnko (2020), this paper constructs the index system of digital economy development, as shown in Table 2. The weight of each index is calculated by entropy method, and the data is standardized by principal component analysis.
3.2.3 Mediating variable
In this paper, the intermediate variable is green agricultural total factor productivity (GTFP). Based on the research results of Guo and Liu (2023) and Ye et al. (2023), this paper constructs an agricultural green total factor productivity measurement system with the integration of economy, resources and environment as shown in Table 3, and the SBM-GML index method is used to calculate.
3.2.4 Adjustable variable
The regulatory variable of this article is Green Finance (GFI). This article draws on the research results of Lin et al. (2024) and Uche et al. (2024), and constructs a measurement system for the Green Finance Index as shown in Table 4. The comprehensive evaluation index of Green Finance is calculated using the entropy method.
3.2.5 Control variables
To ensure the validity of the estimates, other factors affecting agricultural carbon emissions were introduced into the model as control variables (shown in Table 5), including (1) Industrial structure (IS), as measured in terms of the ratio of second or tertiary sector of the economy output to GDP; (2) The level of economic development (GDP) is expressed in the form of natural logarithm of GDP; (3) The level of urbanization (URBAN) is expressed by the ratio of URBAN population; (4) The degree of government intervention (GID) is measured by the ratio of fiscal expenditure to GDP. (5) The innovation level (INN) is measured by the logarithm of the acceptance of domestic invention patent applications.
3.3 Data sources
This paper selects the balance panel data of 31 provinces (Excluding Hong Kong, Macao and Taiwan) China from 2011 to 2020. The sample data were collected from Statistical Yearbook of China, China Rural Statistical Yearbook, Peking University Digital Inclusive Finance Index, and statistical yearbooks from provinces such as Jilin, Inner Mongolia, Anhui, etc. The descriptive statistical analysis of the main variables is shown in Table 6.
According to Table 6, it can be seen that there is a large gap in the development level of green finance among 31 provinces, cities, and districts in China from 2011 to 2020, with a maximum value of 10.758 and a minimum value of 0.489. Similarly, the maximum value of green total factor productivity is 2.363, and the minimum value is 0.755; the maximum value of innovation level is 12.285, and the minimum value is 4.394. This indicates that there is a certain gap in the development level of green finance, green total factor productivity, and innovation level among various provinces and cities in China. In addition, the mean value of agricultural carbon emission intensity is 0.198, and the mean value of digital economic development level is 0.594, indicating that China attaches great importance to agricultural carbon reduction and digital economy development.
4 Empirical analysis
4.1 Steady return
According to Table 7, it can be seen that the variable “digital economic development level,” “green total factor productivity of agriculture,” and “significance p-value of green finance” are 0.002***, 0.001***, and 0.000*** respectively, indicating that they all have a significant impact on agricultural carbon emissions. In addition, the overall significance p-value of the model is 0.000***, and all VIF values are < 10, indicating that the model does not have multicollinearity problems and is well-constructed.
4.2 Test of mediating effect
In order to explore the impact of digital economy on agricultural carbon emissions, this paper selects agricultural green total factor productivity as an intermediary variable to test. Table 8 shows the test results of agricultural green total factor productivity as an intermediary variable. Model 1 was the direct effect of digital economy (DEDI) on agricultural carbon emissions (CI), Model 2 was the direct effect of green total factor productivity (GTFP) on agricultural carbon emissions (CI), and Model 1 was the direct effect of digital economy (DEDI) on agricultural carbon emissions (CI) Model 3 is the indirect effect model of DEDI on CI.
The empirical results from Table 8 show that the P-value of DEDI in Model 1 is 0.009, and the P-value of DEDI in Model 2 is 0.002, the P-values of DEI and GTFP in Model 3 were 0.034 and 0.006, respectively, which indicated that they all passed the 1% level significance test. The standardized coefficient of DEDI in Model 1 was −0.307, and the regression coefficient was negative, which indicated that the development of DEDI had a negative significant inhibitory effect on CI. The standardized coefficient of DEDI in Model 2 was 0.447, and the positive regression coefficient indicated that DEDI could promote GTFP, and thus indirectly affect CI. The results of Model 3 indicate that the standardized coefficient of GTFP is −0.13. The negative regression coefficient suggests that GTFP has a significant inhibitory effect on CI, confirming Hypothesis 2.
4.3 Bootstrap mediation effect test
Green total factor productivity in agriculture can significantly curb carbon emissions from agriculture. Can green total factor productivity in agriculture be used as an intermediary variable for the impact of the digital economy on carbon emissions from agriculture? Based on this, this paper uses Bootstrap sampling to further test the significance of mediating effect of agricultural green total factor productivity. The empirical results from Table 9 show that, where C represents the regression coefficient (when there is no mediator variable GTFP in the model) for DEDI vs. CI in Model 1, that is, the total effect; A represents the regression coefficient for DEDI vs. GTFP in Model 2; B represents the regression coefficient for GTFP vs. CI in Model 3, and a*b is the product of a and b, that is, the mediating effect.
95% BootCI represents the 95% confidence interval obtained by Bootstrap sampling, which is −0.12 to −0.01. The interval does not include 0, indicating significance. “c” represents the regression coefficient of DEDI on CI in model 3 (when there is a mediator variable GTFP in the model), that is, the direct effect. Because a and b are significant, c' is significant, and a*b has the same sign as c', it indicates that the inhibitory effect of DEDI on CI is reduced after adding GTFP to the regression equation, which has a partial mediating effect. Through the intermediary effect of β1γ2/α1, that is, a*b/c, it can be known that the intermediary effect of GTFP is 18.84%, that is, in the process of DEDI inhibiting CI, GTFP played an intermediary effect of 18.84%, thus verifying hypothesis 3.
4.4 Test for regulatory effect
4.4.1 The role of green finance in regulating the comprehensive productivity of green agriculture in the digital economy
The results of Table 10 show that based on the interaction term of digital economy level (DEDI)* Green Finance (GFI), the P-value is 0.046**, the regression coefficient is −0.085, and the interaction term of Model 4 is significant This implies that the regulatory variable GFI has a negative moderating effect on the impact of DEDI on green total factor productivity in agriculture (GTFP), thereby validating the hypothesis 5. The digital economy has a positive effect on green total factor productivity in agriculture, and green finance has a positive effect on green total factor productivity in agriculture, the reason may be that when green finance is added to the green total factor productivity of agriculture, some of the investment in the digital economy may have gone to green finance, indirectly, the digital economy is investing less in green total factor productivity in agriculture.
4.4.2 Green finance plays a regulatory role in the relationship between agricultural green total factor productivity and agricultural carbon emissions
The results in Table 11 show that Model D is the indirect effect of green finance (GFI) on agricultural carbon emissions (CI) with a P-value of 0.003*** and a regression coefficient of −0.006, the results showed that GFI had a significant negative inhibitory effect on CI, which verified hypothesis 4. Based on the interaction of agricultural green total factor productivity (GTFP)* Green Finance (GFI), the P-value was 0.013**, the regression coefficient was 0.016, and the interaction of Model 5 was significant The implication is that the regulatory variable GFI positively regulates the effect of GTFP on CI, thus validating hypothesis 6.
4.5 Quantile regression
This article further explores the inhibitory effect of the digital economy on agricultural carbon emissions. It adopts quantile regression analysis to investigate its differential performance under different levels of agricultural carbon emissions. The SPSS 27.0 software is used for analysis, estimating the corresponding parameter values of agricultural carbon emissions at different quantiles. The regression results are shown in Table 12.
The results of Table 12 show that the impact of digital economy from low to high quantile on agricultural carbon emissions is significantly inhibited, and with the increasing of agricultural carbon emission intensity, the inhibition of digital economy on agricultural carbon emissions is U-shaped.
The digital economy appears to incentivize businesses and farmers to adopt new production methods and enhance green innovation aimed at reducing agricultural carbon emissions, particularly when the intensity of these emissions is low. At this stage, the existing digital economy sufficiently meets the needs for agricultural carbon emission reduction, leading enterprises and farmers to allocate resources to their production operations, thereby diminishing the inhibitory effect of the digital economy on agricultural carbon emissions. Conversely, as the intensity of agricultural carbon emissions rises, enterprises and farmers face increased pressure to reduce emissions, prompting greater reliance on the digital economy to stimulate green innovation. Consequently, the role of the digital economy becomes more pronounced under these conditions. In this study, the coefficient for green total factor productivity (GTFP) as an intermediary variable was not significant at lower quantiles; however, it exhibited significant effects at higher quantiles, with a negative regression coefficient that displayed an upward trend in absolute value. This suggests that the impact of GTFP on agricultural carbon emissions strengthens as carbon intensity increases, indicating that GTFP becomes increasingly effective at inhibiting emissions. Additionally, green finance, serving as an adjustment variable in this analysis, showed no significant effect on agricultural carbon emissions at lower quantiles, but demonstrated significant effects at higher quantiles. This trend indicates that the inhibitory effect of green finance on agricultural carbon emissions intensifies as emissions increase. Moreover, when control variables were included, both industrial structure and economic development levels were found to significantly contribute to agricultural carbon emissions, exhibiting clear negative inhibitory effects.
5 Conclusions and recommendations
5.1 Research conclusion
This article analyzes the impact of the digital economy on agricultural carbon emissions using provincial panel data from 31 provinces in China covering 2011 to 2020. It finds that the development of the digital economy significantly reduces agricultural carbon emissions directly and indirectly by enhancing the level of socialized services in agriculture. Additionally, agricultural green total factor productivity partially mediates this relationship, while green finance exerts a negative regulatory effect. Specifically, the digital economy improves agricultural green total factor productivity, promoting sustainable agricultural practices and reducing emissions. Furthermore, green finance facilitates the rationalization of industrial structure, enhancing the development of the digital economy and improving agricultural productivity. Heterogeneity analysis reveals that the negative impact of the digital economy on agricultural carbon emissions follows a “U” shape, indicating that as agricultural carbon emissions increase, the inhibitory effect of the digital economy first weakens and then strengthens.
5.2 Policy recommendations
5.2.1 Establish and improve the digital economy, perfect the mechanism for green development in agriculture
First, support for advanced technologies like big data and the internet should be strengthened to integrate digital technology with traditional agriculture, advancing green agriculture. Technologies such as sensors and drones can help farmers optimize fertilization, reduce reliance on chemicals, and lower carbon emissions. Continued research and development of green agricultural technologies are also crucial. Second, the government should improve talent acquisition policies and increase financial support for training, establishing educational institutions focused on agricultural technology while providing subsidies for high-tech skills. This approach will promote the development of green agricultural technologies and reduce emissions. Lastly, enhancing the promotion of green agricultural technologies will facilitate their adoption, improve production efficiency, and further decrease carbon emissions.
5.2.2 Developing a differentiated development strategy to promote the coordinated development of the digital economy
First, the government should enhance the promotion of digital technology in agriculture, focusing on increasing agricultural digitization in the western region by deploying technical personnel and popularizing agricultural technology to reduce carbon emissions. Second, economically developed regions, like the central and eastern areas, should be encouraged to support the development of the western regions, thereby narrowing economic disparities and fostering a coordinated economic environment for sustainable agricultural growth. Additionally, the government should strengthen digital learning platforms, allowing farmers to freely access knowledge and improve their skills. By disseminating information on planting techniques, farmers in areas with lower educational levels can enhance their production capabilities, facilitating the integration of agricultural technology with traditional practices and promoting low-carbon agricultural development.
5.3 Theoretical contribution
Our research empirically investigates the direct impact of the digital economy on agricultural carbon emissions by constructing a mediation effect model. It further explores the mediating role of agricultural green total factor productivity and the regulatory effect of green finance. Based on the research findings, the article proposes effective strategies for reducing agricultural carbon emissions, offering a fresh perspective and theoretical reference for the scientific formulation of agricultural carbon reduction policies. Additionally, this study addresses a significant gap in existing literature regarding the mediating role of agricultural green total factor productivity and the regulatory role of green finance.
5.4 Discussion
Liu and Liu (2022), Xin (2023), and Zhongwei and Can (2023) all studied the impact of various variables on agricultural carbon emissions from a single perspective. This article empirically examines the direct impact of the digital economy on agricultural carbon emissions through a mediation effect model, focusing on the roles of agricultural green total factor productivity and green finance. The findings suggest effective pathways for reducing agricultural carbon emissions, providing a new perspective for formulating carbon reduction policies. Additionally, this study addresses the gap in research concerning the mediating role of agricultural green total factor productivity and the regulatory function of green finance.
5.5 Limitations and prospects
The shortcomings of this study primarily relate to sample data selection and indicator calculation. Firstly, the research is constrained by the availability of raw data for each variable, resulting in a limited time span of China, which produces a small overall sample size. Secondly, the calculation of agricultural carbon emissions is based on only six main carbon sources, omitting other significant categories and leading to a lack of comprehensiveness. Future research should consider more detailed survey data or narrow the cross-sectional focus to other city or county levels to enhance reliability. Additionally, improvements in calculating agricultural carbon emissions should incorporate life cycle theory and account for a broader range of carbon sources to increase representativeness.
Data availability statement
The original contributions presented in the study are included in the article/supplementary material, further inquiries can be directed to the corresponding author.
Ethics statement
The studies were conducted in accordance with the local legislation and institutional requirements. Written informed consent for participation was not required from the participants or the participants’ legal guardians/next of kin in accordance with the national legislation and institutional requirements. Written informed consent was obtained from the individual(s) for the publication of any potentially identifiable images or data included in this article.
Author contributions
XX: Conceptualization, Data curation, Formal analysis, Funding acquisition, Investigation, Methodology, Project administration, Resources, Software, Supervision, Validation, Visualization, Writing – review & editing. SW: Data curation, Funding acquisition, Resources, Supervision, Validation, Visualization, Conceptualization, Formal analysis, Investigation, Methodology, Project administration, Software, Writing – original draft. XQ: Data curation, Funding acquisition, Resources, Supervision, Validation, Visualization, Writing – original draft. YH: Conceptualization, Resources, Validation, Writing – review & editing.
Funding
The author(s) declare financial support was received for the research, authorship, and/or publication of this article. This study was supported by Basic Research Program of Liaoning Provincial Department of Education: Research on Low-Carbon Technological Innovation Path and Power Mechanism of Liaoning Province Based on Green Transition and Dual-Carbon Target (LJKMR20220911), Liaoning Provincial Social Science Planning Project: Research on the Evaluation System of Green and Low-Carbon Technological Innovation and the Implementation Path of Scientific and Technological Carbon Reduction Policies in Liaoning Province (L22BJY008), and Key Project of Social Sciences Joint Project of Dalian Polytechnic University in 2024: Research on Theoretical and Practical Issues of Promoting Agricultural Power with New Quality Productivity in the New Era.
Conflict of interest
The authors declare that the research was conducted in the absence of any commercial or financial relationships that could be construed as a potential conflict of interest.
Publisher's note
All claims expressed in this article are solely those of the authors and do not necessarily represent those of their affiliated organizations, or those of the publisher, the editors and the reviewers. Any product that may be evaluated in this article, or claim that may be made by its manufacturer, is not guaranteed or endorsed by the publisher.
References
Che, H., and Shen, Y. (2022). Green finance promotes a new approach to the development of the digital economy. Gansu Soc. Sci. 2022, 218–225.
Chen, Y., Hu, S., and Wu, H. (2023). The digital economy, green technology innovation, and agricultural green total factor productivity. Agriculture 13:101961. doi: 10.3390/agriculture13101961
Duan, H., Zhang, Y., and Zhao, J. (2011). Carbon footprint analysis of farmland ecosystem in China. J. Soil Water Conserv. 25, 203–208. doi: 10.13870/j.cnki.stbcxb.2011.05.020
Goldfarb, A., and Tucker, C. (2019). Digital economics. J. Econ. Literat. 2019, 3–43. doi: 10.1257/jel.20171452
Guo, H., and Liu, X. (2023). Can digital rural development promote agricultural green total factor productivity by reducing resource element mismatch? Ningxia Soc. Sci. 2023, 107–117. Available at: https://kns.cnki.net/kcms2/article/abstract?v=At0rObma_qO0fZ143-Y58G2890kvxbaRW4hiBjoH-Ic1KQD8-cCHpuNQ4ikh9K3fkeFOH8Qz1xSM38A_ghH-Av7peYnQgD8PKCHJ9t8WpHaX5CV3vqZiZ2B_ZJrWezoPcbB2vnauXQJq99XQynofTEjyYFz1_dbvHL727v7Pd-SviXIbmMgX0g1kO5hrkkrZQPreydTIXiM=&uniplatform=NZKPT&language=CHS
He, Y., Wang, J., Gao, X., Wang, Y., and Choi, B. R. (2021). Rural tourism: does it matter for sustainable farmers' income? Sustainability 13, 10440–10440. doi: 10.3390/su131810440
He, Z. (2024). Green finance, carbon regulation and corporate green total factor productivity. Statist. Decision 40, 155–159. doi: 10.13546/j.cnki.tjyjc.2024.05.028
IPCC (2013). Climate Change: The Physicle Science Basis Technical Summary. Available at: https://www.ipcc.ch/report/ar5/wg1/
Jiang, T. (2022). Mediating effects and moderating effects in causal inference. China Industr. Econ. 2022, 100–120. doi: 10.19581/j.cnki.ciejournal.2022.05.005
Jin, F., and Xu, C. (2022). Research on the nonlinear impact of digital economy development on carbon emissions. Mod. Econ. Res. 2022, 14–23. doi: 10.13891/j.cnki.mer.2022.11.005
Li, B., and Zhang, J. (2012). Decoupling of China's agriculture carbon emissions and economic development based on the input perspective. Econ. Surv. 2012, 27–31. doi: 10.15931/j.cnki.1006-1096.2012.04.004
Li, K., Lin, W., Jiang, T., Mao, Y., and Shi, W. (2024). Driving carbon emission reduction in China through green finance and green innovation: an endogenous growth perspective. Environ. Sci. Pollut. Res. Int. 31, 14318–14332. doi: 10.1007/s11356-024-32067-w
Lin, J., Zhang, L., and Dong, Z. (2024). Exploring the effect of green finance on green development of China's energy-intensive industry-a spatial econometric analysis. Resour. Environ. Sustainabil. 2024:16100159. doi: 10.1016/j.resenv.2024.100159
Liu, R., and Liu, M. (2022). The impact of green finance development on agricultural carbon emissions—based on the perspective of agricultural technology innovation. Agricult. Technol. 42, 160–163. doi: 10.19754/j.nyyjs.20220815037
Liu, Y., and Xu, S. (2024). The impact of digital economy development on agricultural carbon emissions: an empirical study from major grain-producing areas. J. Econ. Bus. Manag. 12:780. doi: 10.18178/joebm.2024.12.1.780
Ma, T. (2011). Evaluation of the current status of carbon sources and sinks in Shanghai's agriculture and analysis of the potential for increasing carbon sinks. J. Agricult. Resour. Environ. 28, 38–41. Available at: https://kns.cnki.net/kcms2/article/abstract?v=At0rObma_qPux31Q861_KTkPDGav9tMr428ZTmAIsqxR1cAoHieYcX5JZfzYXT4vXLj-SBYZI3ommXyxzwWWvOtdcLx7hddKZbpQMgruVo6-8g9iH0HWy1chSkJnBiDvb0io5vNmElxl0i6t6sMyaoAcyLoTPc2KEJr2FjTDkDBt_DzT4yKJno8FOYUSGOO_&uniplatform=NZKPT&language=CHS
Sidorov, A., and Sencehnko, P. (2020). Regional digital economy: assessment of development levels. Mathematics 8, 2143–2143. doi: 10.3390/math8122143
Uche, E., Das, N., and Ngepah, N. (2024). Green environments reimagined through the lens of green finance, green innovations, green taxation, and green energies. Wavelet quantile correlation and rolling window-based quantile causality perspective. Renew. Energy 228:120650. doi: 10.1016/j.renene.2024.120650
Wang, B., and Zhang, W. (2016). A research of agricultural eco-efficiency measure in China and space-time differences. China Popul. Resour. Environ. 26, 11–19. Available at: https://link.cnki.net/urlid/37.1196.n.20160603.1124.042
Wang, C., Liu, P., Ibrahim, H., and Yuan, R. (2024). The temporal and spatial evolution of green finance and carbon emissions in the Pearl River Delta region: an analysis of impact pathways. J. Clean. Product. 446:141428. doi: 10.1016/j.jclepro.2024.141428
Wang, H., Liu, H., and Cheng, Z. (2023). Study on agricultural green total factor productivity around Dongting lake. Agricult. Forest. Econ. Manag. 6. doi: 10.23977/AGRFEM.2023.060202
Wang, W., and Mei, T. (2024). Research on the effect of digital economy development on the carbon emission intensity of agriculture. Sustainability 16:41457. doi: 10.3390/su16041457
Xie, W., Gao, K., and Yu, J. (2022). Digital economy, industrial upgrading and carbon emissions. Statist. Decision 38, 114–118. doi: 10.13546/j.cnki.tjyjc.2022.17.022
Xin, D. (2023). The mechanism and effect of digital economy to promote CO2 emission reduction: An empirical investigation based on the green technological progress. Sci. Tech. Prog. Policy 40, 22–32. Available at: https://link.cnki.net/urlid/42.1224.G3.20230928.1305.002
Xu, X., Huang, X., Huang, J., Gao, X., and Chen, L. (2019). Spatial-temporal characteristics of agriculture green total factor productivity in China, 1998–2016: based on more sophisticated calculations of carbon emissions. Int. J. Environ. Res. Publ. Health 16, 3932–3932. doi: 10.3390/ijerph16203932
Ye, F., Yang, Z., Yu, M., Watson, S., and Lovell, A. (2023). Can market-oriented reform of agricultural subsidies promote the growth of agricultural green total factor productivity? empirical evidence from maize in China. Agriculture 13, 251–251. doi: 10.3390/agriculture13020251
Zhang, F. Y., Ji, X. M., and Zheng, Z. X. (2023). Digital economy, agricultural technology innovation, and agricultural green total factor productivity. SAGE Open 13:21582440231194388. doi: 10.1177/21582440231194388
Zhao, T., Zhang, Z., and Liang, S. (2020). Digital economy, entrepreneurship, and high-quality economic development: empirical evidence from urban China. J. Manag. World 36, 65–76. doi: 10.19744/j.cnki.11-1235/f.2020.0154
Zhao, X., Cao, S., and Ding, L. (2021). The impact of internet dependence on household carbon emissions-the chain interm nediary role of income gap and consumption upgrade. J. Beijing Inst. Technol. 23, 49–59.
Zhongwei, C., and Can, T. (2023). Impact of digital economy development on agricultural carbon emissions and its temporal and spatial effect. Sci. Technol. Manag. Res. 43, 137–146. Available at: https://kns.cnki.net/kcms2/article/abstract?v=At0rObma_qM1FLl9n7QPi0JWB4becKFyYOJmtqOfYtF5LqgS_6tPR2UNrbMwoBWCgOnzWWQOfEpHCmr0ypbgAvsjCNTE5jcIiGJn2RLDwvU8B5fr1K_lThFqKiPRnLTuROM4Rh3qIXNkpXBcWH0BMKd5hdbGE_HchebitYibLKrmP_1frJWJz-9W9OnUUxqY7CimhJO1wCI=&uniplatform=NZKPT&language=CHS
Keywords: digital economy, agricultural green total factor productivity, green finance, agricultural carbon emissions, empirical test
Citation: Xu X, Wang S, Qin X and Han Y (2024) Can the digital economy help reduce agricultural carbon emissions? An empirical test based on the moderated mediating effect model. Front. Sustain. Food Syst. 8:1454636. doi: 10.3389/fsufs.2024.1454636
Received: 01 July 2024; Accepted: 11 November 2024;
Published: 05 December 2024.
Edited by:
Abbas Ali Chandio, Guizhou University, ChinaReviewed by:
Martinson Ankrah Twumasi, Chengdu University of Technology, ChinaNawab Khan, Shandong Agricultural University, China
Copyright © 2024 Xu, Wang, Qin and Han. This is an open-access article distributed under the terms of the Creative Commons Attribution License (CC BY). The use, distribution or reproduction in other forums is permitted, provided the original author(s) and the copyright owner(s) are credited and that the original publication in this journal is cited, in accordance with accepted academic practice. No use, distribution or reproduction is permitted which does not comply with these terms.
*Correspondence: Xutian Qin, NjE3NDAzMjkwJiN4MDAwNDA7cXEuY29t