- 1Farming Systems Ecology Group, Wageningen University & Research, Wageningen, Netherlands
- 2Field Crops, Wageningen University and Research, Lelystad, Netherlands
- 3Centre for Crop Systems Analysis, Wageningen University and Research, Wageningen, Netherlands
Intercropping is proposed as a promising strategy to meet future food demand while reducing agriculture’s environmental impact by re-diversifying agricultural fields. Strip cropping, a form of intercropping, has a potential to simultaneously deliver multiple ecosystem services including productivity, while facilitating management as strip width can be adjusted to the working width of available machines. While the yield performance of strip cropping systems is influenced by the interaction between neighboring crops, to date, empirical studies on the performance of various crop combinations in strip cropping systems are limited. Here we used three-year data (2020–2022) from a 64-ha organic strip cropping system in the Netherlands to (1) evaluate the effects of crop neighbors and strip cropping on yield and (2) explore if optimizing the allocation of crop neighbors in alternative strip cropping configurations can improve yield and revenue performances. We analyzed the edge effect and strip cropping effect on yield of six crops grown in strips, each neighboring a total of five crops. The yield data was then used to evaluate the performance of the current and alternative strip configurations in terms of LER and relative revenue. Results showed that except for the positive effect observed on potato when neighboring celeriac or broccoli, edge effects lacked statistical significance. Strip cropping effect varied per crop: positive for faba bean and parsnip, neutral for celeriac and potato, and negative for oat and onion. Analysis across crops showed an overall significant positive strip cropping effect on yield. These findings highlighted the value of analysis at the cropping system level in developing designs aimed at unlocking the potential of strip cropping. The positive but variable strip cropping effects observed in the current experimental design and the two alternative configurations suggests prioritizing an overall increased crop diversity over optimizing their spatial arrangement. While we demonstrated increased productivity with strip cropping, further research is needed to expand the database on optimal crop combinations, extending the evaluation beyond yield and revenue performances to facilitate broader adoption of strip cropping in the Netherlands and Western Europe.
1 Introduction
Agricultural diversification has been put forward as a way to lower agriculture’s environmental impact without penalizing its productivity (Tamburini et al., 2020), by reutilizing the agroecological functioning of agroecosystems that have been replaced by the intensive use of agrochemicals (IPES-Food, 2016). Among diversification practices, intercropping—the practice where multiple crops are grown simultaneously in the same field for at least part of their growing cycle—has been proposed as a promising strategy to simultaneously deliver multiple ecosystem services (Brooker et al., 2015; Beillouin et al., 2019a; Tamburini et al., 2020). In terms of yield, intercropping has been shown to increase yield quantity (see, e.g., Mu et al., 2013; Dong et al., 2018), quality (see, e.g., Bedoussac and Justes, 2010; Juventia et al., 2021), and stability (Stomph et al., 2019) compared to sole-crop monocultural systems. When implemented in organic systems, it has a potential to reduce the conventional-to-organic yield gap (Kremen and Miles, 2012; Ponisio et al., 2015).
In intercropping, crops can be arranged in different spatial arrangements, and the type and intensity of their interactions depend on the type of arrangement and species involved (Malézieux et al., 2009). In the Netherlands, dominated by intensive industrial agriculture, strip (inter-)cropping has received much attention (Schouten, 2018; Juventia et al., 2022). In strip cropping, long narrow strips consisting of multiple rows of one crop species are grown simultaneously next to strips of other crop species. Strips are wide enough to allow independent crop management by machinery but narrow enough for the crops to interact (Hauggaard-Nielsen, 2010; Juventia et al., 2021). The independent crop management strip cropping is considered easier to manage compared to other intercropping systems because the strip width can be adjusted to allow use of available farm equipment.
Several studies have shown the potential of strip cropping to deliver multiple ecosystem services. For instance, pest and disease control has been shown to be improved in strip cropping, compared to sole crop since the alternating strips of different crops can act as barriers in the field, thereby reducing the spread of pests and diseases (Bouws and Finckh, 2008; Ditzler et al., 2021b; Cuperus et al., 2023; Croijmans et al., 2024). Weed density was found to be significantly lower in strips compared to sole crops (Głowacka, 2013). Biodiversity is expected to increase as the spatial and temporal niche differentiation facilitates species to migrate to nearby strips during disturbance (Lopes et al., 2015; Hatt et al., 2017). Cultural ecosystem service in terms of aesthetic quality may be enhanced as the diversity within the field increases (Junge et al., 2015).
In addition to the above-mentioned ecosystem services, several studies have shown the potential of strip cropping to increase productivity compared to sole crop [see, e.g., Yu et al. (2015) and Zhu et al. (2023)], although the interactions between two crops that can benefit productivity in strip cropping are thought to mostly occur at the strip border, in the edge rows of the neighboring strips (Austin and Blackwell, 1980; Van Oort et al., 2020; Wang et al., 2020). This is in contrast to mixed intercropping where crops are sown together in no particular pattern or arrangement (Homulle et al., 2022). Facilitation and complementarity occurring through temporal, spatial, and chemical niche differentiation between the intercropped species are considered the main mechanisms to be responsible for increasing yield in intercropping systems (Justes et al., 2014; Duchene et al., 2017; Stomph et al., 2019). Temporal niche differentiation occurs when crops differ in crop growth and development patterns in time, leading to staggering in their concurrent nutrient and water requirements (Gebru, 2015; Dong et al., 2018). Spatial niche differentiation in leaf canopy or root system of the intercrops enables complementary use of available resources in terms of light interception as well as water and nutrient uptake from different canopy heights or different soil layers (Gebru, 2015). Intercrops may also have the ability to mobilize various chemical forms of nutrients, resulting in chemical differentiation (Homulle et al., 2022).
To minimize competition and promote complementarity or facilitation in strip cropping, the selection of crop combinations that would enable these mechanisms is essential. However, identifying crop combinations to optimize yield is a challenge given the currently limited empirical studies on the performance of different crop combinations in strip cropping systems (Isbell et al., 2017). Roughly 80% of meta-analyses on intercropping, species mixtures, and/or associated plant species focus on cereals and legumes (Beillouin et al., 2019b), with four legume species dominating 70% of the reviewed studies (Ditzler et al., 2021a). Given the growing need by current and aspiring farmers, advisors, and engaged researchers in the strip cropping network in the Netherlands, this paper aims to bridge the knowledge gap on what constitutes good crop combinations for optimizing yield. Furthermore, given that poor spatial configuration may lead to negative effects on the delivery of ecosystem services (Juventia et al., 2022), the potential of improving the production performance of the current crop rotation by optimizing the spatial allocation of crop neighbors will be explored.
To this end, we used three-year data (2020–2022) from a strip cropping system composed of eight arable crops at a commercial organic farm in the Netherlands to evaluate the effects of crop neighbors and strip cropping on yield and explore if optimizing the allocation of crop neighbors in alternative strip cropping configurations can improve yield and revenue performances. This question is divided in three research questions: (i) What is the effect of crop neighbors on the yield of the edge row compared to the center row in the strip (i.e., edge effect)?; (ii) What is the effect of strip cropping on yield per crop and across crops compared to sole crop reference (i.e., strip cropping effect)?; and (iii) What is the effect of the current and alternative strip cropping configurations compared to sole crop reference in terms of Land Equivalent Ratio (LER) and revenue? We hypothesized that the edge effect by the different crop neighbors would result in different yield performances and that the overall strip cropping effect would be neutral. We expected that an optimal configuration can be found by combining best performing combinations.
2 Materials and methods
2.1 Site description
The study was conducted from April 2020 to November 2022 on a 64-ha field of Exploitatie Reservegronden Flevoland (ERF B.V.) (52°23′33.1” N, 5°19′07.6″ E) representing an average farmsize in the Netherlands (BIN, 2023). ERF B.V. is the commercial part of a non-profit foundation Beheer en Exploitatie Reservegronden Flevoland and is the largest organic farm in the Netherlands with 15 employees and hired contract workers. The soil type is silty clay and the field is surrounded by a homogenous intensively farmed arable crop landscape with few natural elements (Kragten and De Snoo, 2008). The average temperature and annual mean rainfall between 1991 to 2020 were 10.5°C and 792 mm (KNMI, 2023). These were 11.7°C and 785 mm in 2020 (KNMI, 2020); 10.4°C and 806 mm in 2021 (KNMI, 2021); and 11.6°C and 729 mm in 2022 (KNMI, 2022).
Field management followed organic regulations, applying no pesticides and only organic manure. Land preparation was done by rotary tiller. Boom irrigation was applied in 2020 and was replaced by drip irrigation installed in 2021. Weeding was done by mechanical harrowing or by hand. Management practices for strip cropping and sole cropping were always the same to make valid comparisons between the two cropping systems. All crops were managed independently of the other crops. There were some variations in field management between the years concerning cultivar choice, irrigation, and sowing and harvest dates (Table 1).
2.2 Experimental design
Prior to the start of the strip cropping experiment in 2020, the field was under sole cropping of cabbages (2017), red beet (2018), and pea (2019). During the experiment, both strip and sole cropping systems followed a fixed crop rotation (Figure 1). The experimental design which includes choices on the strip width, crops and cultivars, and the spatio-temporal configuration of the strips (i.e., temporal crops sequence and spatial allocation of the crops in strips), was co-developed by the farmers and the researchers, with the focus on systematically testing the yield effect of crop neighbors in an applied practical setting. This means that the allocation of crop neighbor was not optimized ex-ante. The choice of strip width was considered based on a management and an agroecological perspectives. While a greater strip width allows for simultaneous and practical management, a narrower width allows for temporal niche differentiation and habitat continuity and contiguity (Landis et al., 2000; Lee et al., 2001; Juventia et al., 2022). A strip width of 6 meters was selected to align with the feasible operational width of the farm’s existing machinery. Eight crops were chosen to be grown in an eight-year rotation: grass—clover mixture (Lollium multiforum L., and Trifolium repens L.), followed by celeriac (Apium graveolens var. rapaceum), broccoli (Brassica oleracea var. italica), oat (Avena sativa L.), onion (Allium cepa L.), parsnip (Pastinaca sativa L.), faba bean (Vicia faba L.), and potato (Solanum tuberosum L.). In 2020, seed onion was cultivated for plant propagation material, which was changed to plant onion for consumption in 2021. Figure 1 shows the experimental layout which follows an incomplete block design with four blocks, each consisting of two sole crop references, 32 strips, and one perennial grass and flower strip. Across the field, each crop was cultivated in one sole crop reference and 16 strips that neighbor five different crops (Table 2). Per crop, each of the five neighboring crops were present in at least four blocks each year. The incomplete block design allows for comparison of strips with sole crops and of strips with the different crop neighbors across blocks and years.
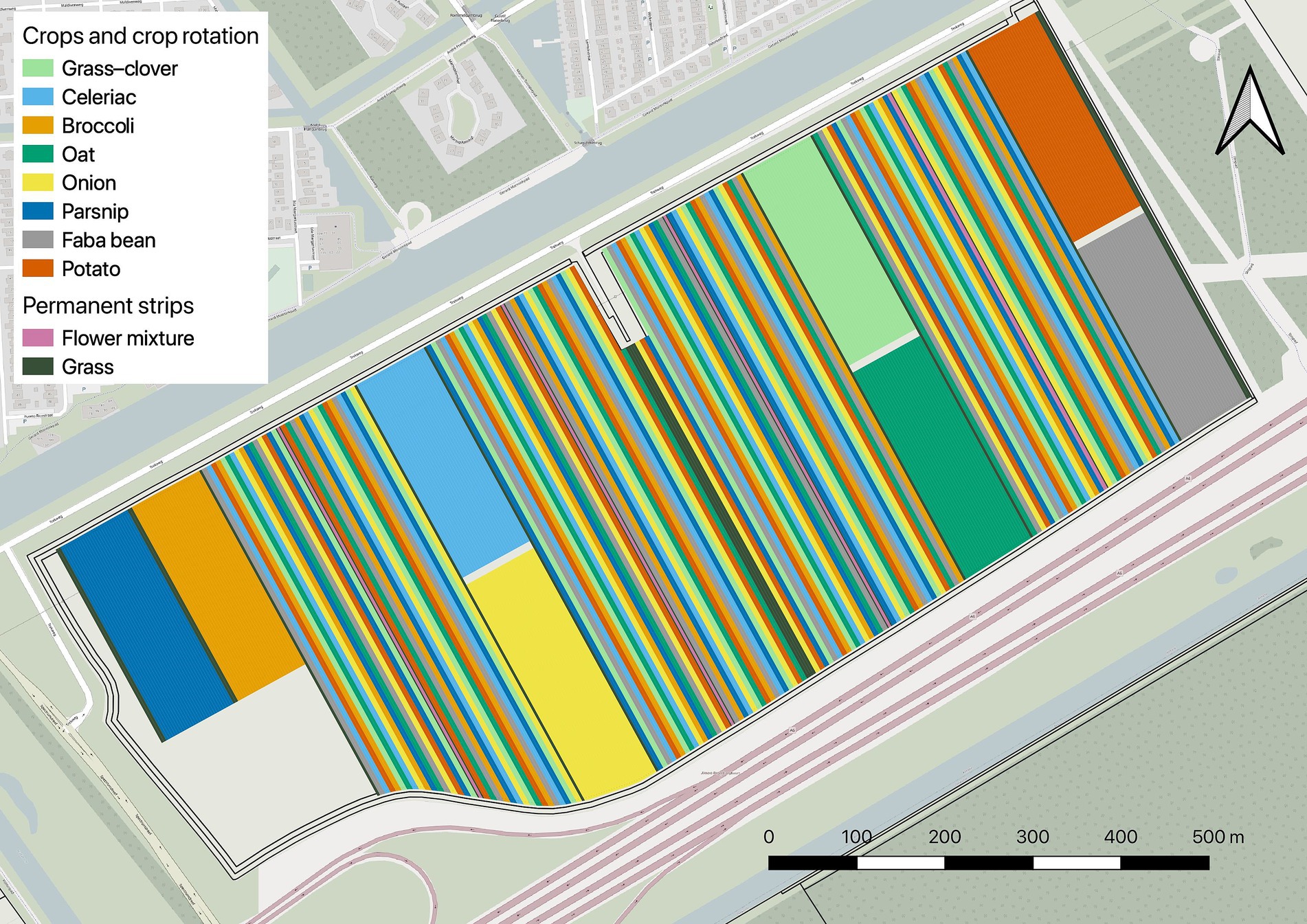
Figure 1. Overview of the strip cropping field in 2022. Colors represent the different crops. Each crop was cultivated in four strips per block, which equals a total of 16 strips over the field. Two sole-crop reference plots, each of a 2-ha area, were present per block. The black dashed lines separate the four blocks. The legend represents the sequence of the crop rotation when read from top to bottom.
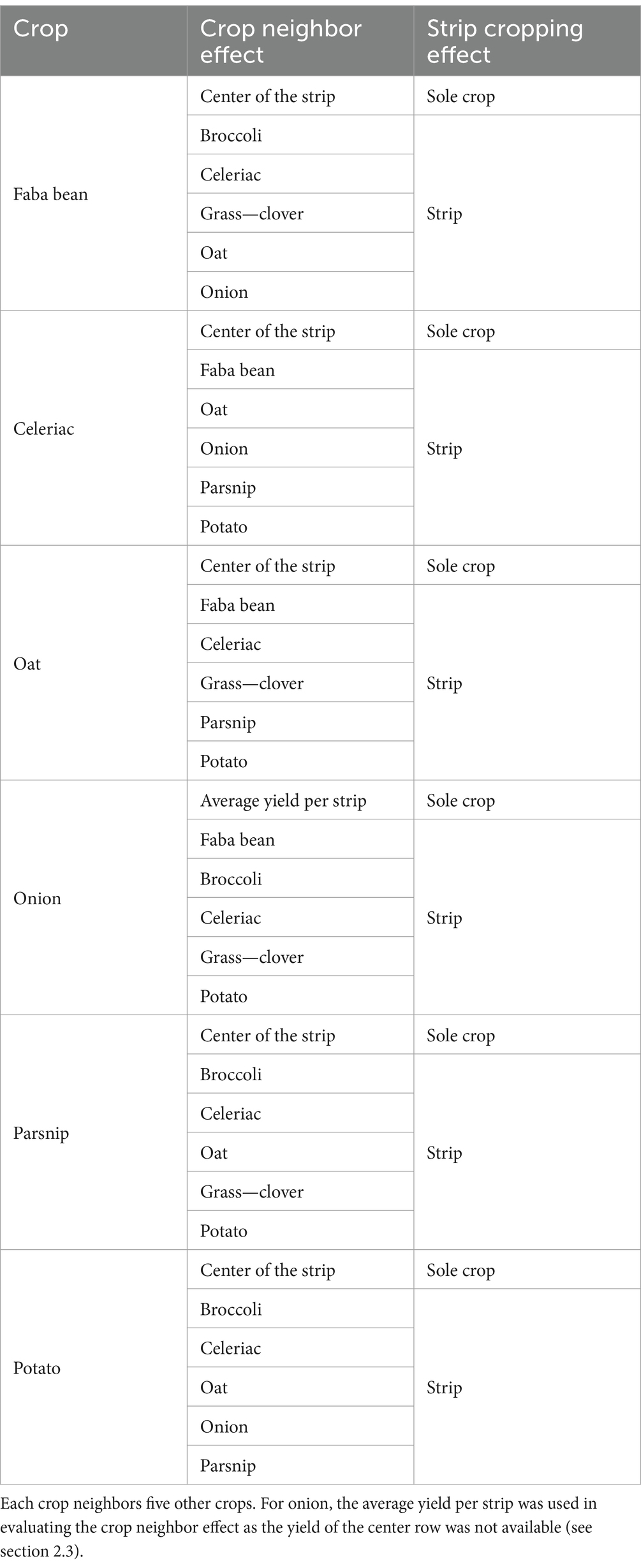
Table 2. Overview of the experimental setup in 2020–2022 to evaluate the effects of crop neighbors and strip cropping on the yield of the six crops.
The crops analyzed in this study were faba bean, celeriac, oat, onion, parsnip, and potato (Table 2). Grass—clover yield was not analyzed as its primary function for the farmers in the rotation is weed control and soil health, but not commercial revenue. Unfortunately, we were not able to obtain reliable yield data on broccoli and therefore this crop was removed from the analyses. Due to weather conditions and market demand broccoli cultivars, harvest moment, and criteria (for fresh market or industry) were changed within the field and years, making comparisons unreliable. All eight crops were included as crop neighbors. The perennial grass and flower strips were excluded as crop neighbors, due to a lack of repetition in the field.
The edge effect of a crop neighbor on yield was analyzed per crop, comparing the yield estimate of a crop on the edge row adjacent to a neighboring crop with its yield on the center row. The strip cropping effect of crops grown in strip versus sole crop was analyzed per crop and across crops, where the yield estimate of each crop grown in the strip was compared with its yield in the sole crop.
2.3 Data collection
Gross harvest yield (i.e., kg fresh harvestable matter per m2) of the six crops were collected from 2020 to 2022 during the harvest period from August to November. Yield was collected through manual sampling by hand, except for faba bean and oat where machine-harvest data per strip was available (Table 3). Hand-harvest samples were at least 100 meters away from the end of the strip. In 2020, for each crop, samples were collected from each strip at one randomly determined transect perpendicular to the direction of the strips. In 2021 and 2022 this was increased to two transects per strip. Per transect, three positions were sampled with exception for onion. In the strip, one center row and two edge rows were sampled, while in the sole crop, these were the three rows in the center of the sole crop reference. For onion, two swaths from the western half of the strip (i.e., the 1st-4th rows) and the eastern half (i.e., the 5th-8th rows) were sampled following mechanical lifting which lifted the onions from the ground and formed the two swaths. The sampling area per crop differed as this was based on the planting density of the crop and for reasons of feasibility (Table 3). The total fresh weight of the produce per sampling area was measured using a field scale, from which yield per unit area was calculated.
2.4 Data analyses
2.4.1 Relative yield to evaluate crop neighbor and strip cropping effect
Relative yield (RY), i.e., the ratio of intercrop to the reference yields (Willey, 1979), was calculated to evaluate the effect of crop neighbors and strip cropping. To analyze the crop neighbor effect, the center row within the strip was used as the reference as in Equation 1:
where IY is the intercrop fresh weight per unit area of the crop i. The e represents the edge row of crop i adjacent to a crop neighbor, while m represents the center row from the same strip of the crop i. Given that each crop neighbors five other crops (Table 2), five RY crop neighbor values per crop were calculated. An edge effect is present when RY crop neighbor is significantly different from one.
The effect of strip cropping was analyzed per crop and across the six crops using Equation 2:
where IY is the intercrop fresh weight per unit area and SY is the sole crop yield. For faba bean (2020–2021) and oat (2020–2022), machine-harvest yield per strip-and sole-cropped area was used. For all other crops where samples were collected by hand-harvest, only the yield from the center rows were included in analyzing the strip cropping effect as the center rows were considered to be representative of the yield per strip or sole crop (see Table 3). The absolute yields per year and per crop in both strip and sole crop systems are provided in Supplementary Table S4B. A strip cropping effect is present when RYstrip cropping is significantly different from one.
2.4.2 Alternative spatial configurations
To facilitate the focus of this experiment which was to systematically test the yield effect of as many crop neighbors as possible in an applied practical setting, two distinct sub-configurations (i.e., sub-configurations #1 and #2, Supplementary Figure S1) were combined into one configuration—the current experimental design (Figure 1). Both sub-configurations followed the same crop rotation and honored the ‘hop-skip-jump’ principle after Vereijken (1997), such that a crop is not planted in a strip adjacent to where it was last year, in order to create discontinuity for pest and disease in space (Juventia et al., 2022). While in sub-configuration #1 each crop had four neighbors, in sub-configuration #2 each crop neighbored three crops. Each of this sub-configuration was then used to build the alternative spatio-temporal configurations #1 and #2, respectively (Supplementary Figure S1).
2.4.3 Performance of the current and alternative configurations
Using the gross and relative yield data, we evaluated the overall performance of strip cropping on three indicators: (1) land saving proportion, (2) relative gross revenue, and (3) breakeven labor cost ratio. We compared the current experimental design (Figure 1) and the two alternative spatio-temporal configurations (Supplementary Figure S1).
2.4.3.1 Land saving proportion
The land equivalent ratio (LER) is one of the most frequently used indicators of productivity in intercropping studies because it captures in a single value the land area that might be saved by intercropping instead of sole cropping (Van der Werf et al., 2021). LER represents the ratio of land area needed under intercropping to the area of sole cropping to obtain an equal amount of yield at the same management level (Mead and Willey, 1980; Vandermeer, 1989). LER was calculated based on the fresh yield of the six crops across the 3 years of the experiment using Equation 3:
where IY is the intercrop fresh weight from the center row m per unit sole-crop area of crop i in strip configuration j and SY is the sole crop yield of crop i. LER > 1 means that the strip was more efficient in terms of land use compared to the sole crop. We calculated the LER for the current and alternative configurations. To evaluate the change in yield with respect to land required we calculated the associated land saving proportions for the current and alternative configurations using Equation 4 (Khanal et al., 2021; Van der Werf et al., 2021).
The proportion of land saved could be used for inclusion of non-productive elements of semi-natural habitats in or around the field like flower strips or hedgerows for increasing biodiversity (Bianchi et al., 2006; Sirami et al., 2019; Guo et al., 2022).
2.4.3.2 Relative revenue
Different crops usually have different monetary values, making it valuable to also compare total revenues (Khanal et al., 2021). Here we used the farm-gate selling price of each crop (Table 4) and calculated the relative total value (i.e., relative revenue) per unit area for the different strip cropping configurations and the sole crop using Equation 5:
where IY is the intercrop fresh weight from the center row m per unit sole-crop area of crop i in strip configuration j, SY is the sole crop yield of crop i, and P is the price of crop i. Here we assumed that the total gross harvest yield can be sold, not taking into account storage losses or difference in quality between treatments. Selling price was used to apply weight so as to complement the use of LER which has been criticized for failing to account for the different magnitude of changes in the yield of one crop component of an intercrop and in another (Khanal et al., 2021). An increase in relative revenue could be used for various purposes, such as allocating non-productive nature-inclusive areas, investing in new technologies, or addressing implementation challenges associated with strip cropping, thereby facilitating its broader adoption.
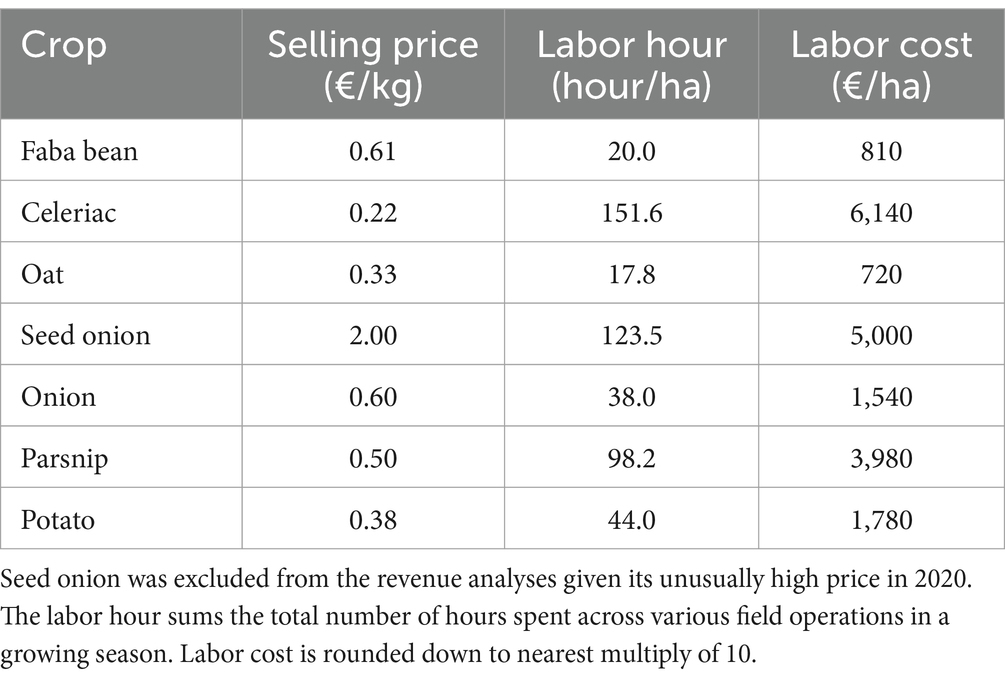
Table 4. Average farm-gate selling price, labor hour, and labor cost data per crop as reported by the farm.
2.4.3.3 Breakeven labor cost ratio
An increase in labor is often identified as one of the implementation challenges of a strip cropping system (Rosa-Schleich et al., 2019; Al-Amin et al., 2024). Hence, a breakeven labor cost ratio might be a good proxy to indicate the feasibility of implementing strip cropping. The breakeven point indicates where the difference in revenues between the strip and the sole cropping breaks even with the additional labor cost associated with strip cropping operations (Equation 6). From there, by dividing both sides by the sole crop labor cost (Equation 7), we calculated the breakeven labor cost ratio (Equation 8). This ratio shows how much of the increased labor cost from strip cropping can be offset by the extra revenue generated, making the practice economically viable.
where IH and SH are the total hours spent across field operations of crop i in strip configuration j and sole cropping respectively, w is the average labor cost per hour, IY is the intercrop fresh weight from the center row m per unit sole-crop area of crop i in strip configuration j, SY is the sole crop yield of crop i, and P is the price of crop i. For calculating labor requirements of sole cropping ( ), ERF B.V. uses standard reference values of Kwantitatieve Informatie Akkerbouw en Vollegrondsgroenteteelt (KWIN) (Van der Voort, 2022). KWIN reports for each crop, the total number of hours spent on average per field operation (e.g., soil preparation, sowing/planting, irrigation, fertilization, harvesting, etc.) and the average labor cost per hour (40.53€/hour) in the Netherlands. The total number of hours spent across various field operations is shown in Table 4.
2.5 Statistical analyses
For each crop, linear mixed-effect models (LMMs) were developed separately to assess the effect of crop neighbors and strip cropping on the response variable yield. To assess the effect of crop neighbors, the identity of the ‘crop neighbor’ was used as a fixed effect (Supplementary Table S2A). To assess the effect of strip cropping, strip or sole-crop ‘treatment’ was used as a fixed effect (Supplementary Tables S2B–C). The variable ‘year_block’ was included as random effect, to account for the combined effect of temporal and spatial variability across the 3 years and within the field. Model selection was based on the Akaike Information Criterion (AIC). Statistical analyses were conducted using one-way analysis of variance (ANOVA) and Sidak post-hoc test. We used the Shapiro–Wilk Normality test on the residuals (Shapiro and Wilk, 1965) and visual analysis of the QQ plots using the DHARMa package (Hartig, 2022) to confirm that the normality assumption was met. All analyses were performed using the R program, version 4.0.2 (R Core Team, 2020), and the lme4 package (Bates et al., 2015).
Model estimates were then used to calculate the RY crop neighbor which compared the yield of the edge row compared to the center row of the strip, and the RY strip cropping which compared the yield of the strip to the sole crop. This resulted in the RY of the referenced system (i.e., either the center row of a strip or the sole crop) always equals 1.
3 Results
3.1 Relative yield
3.1.1 Effect of crop neighbors on yield per crop
When analyzed across all crop neighbors (Supplementary Figure S3), a significant edge effect was observed for potato and celeriac, where higher yield was observed on the edge row of potato than the center row, and the other way around for celeriac (Supplementary Table S4A). Edge effect by specific crop neighbors was observed on faba bean and potato (Figure 2). The RY of the faba bean rows adjacent to broccoli and celeriac were significantly higher by 68 and 73% than the faba bean row next to onion (p < 0.001). Potato showed a higher RY by 15 to 38% when neighboring celeriac and broccoli compared to potato on the center row or when neighboring oat (p < 0.001).
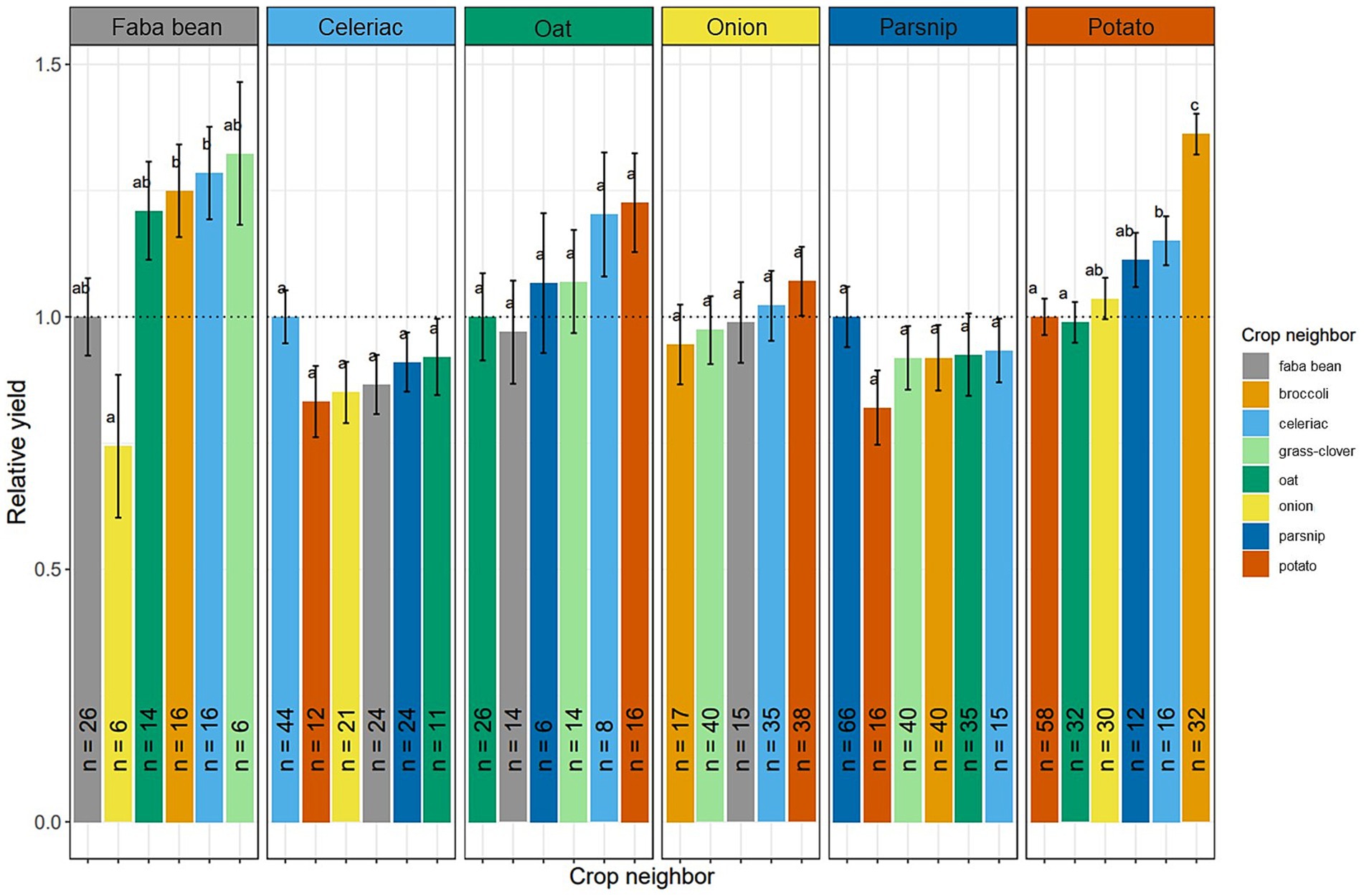
Figure 2. The relative yield of the six crops, comparing the edge rows that neighbor different crops to the center row. Bar color corresponds to the color of the crops presented in the legend and the field map (Figure 1). The RY of the center row which always equals 1 is represented by the first bar on the left side of each panel. For onion, the bar for the center row is absent since the average gross harvest yield of the two swaths per strip was used to calculate RY (see 2.3). Error bars indicate standard error. Letters indicate significant differences between the crop neighbor effects for each crop (p < 0.05). Sample sizes per crop neighbor are shown at the bottom of each bar.
3.1.2 Effect of strip cropping on yield per crop and across crops
Strip cropping effect varied with year in both systems for all the crops, except celeriac and onion (Supplementary Tables S2, S4B). Across the years, a significant effect of strip cropping was observed on the RY of faba bean, oat, onion, and parsnip (p < 0.05) (Figure 3). For faba bean and parsnip, RY was increased by 32 and 41%, respectively, in the strip compared to the sole crop. In contrast, the RY of oat and onion were lower by 6 and 10%, respectively, when cultivated in strips. When analyzed across all crops, an overall 8% higher relative yield was observed in the strip than in the sole crop (p < 0.001).
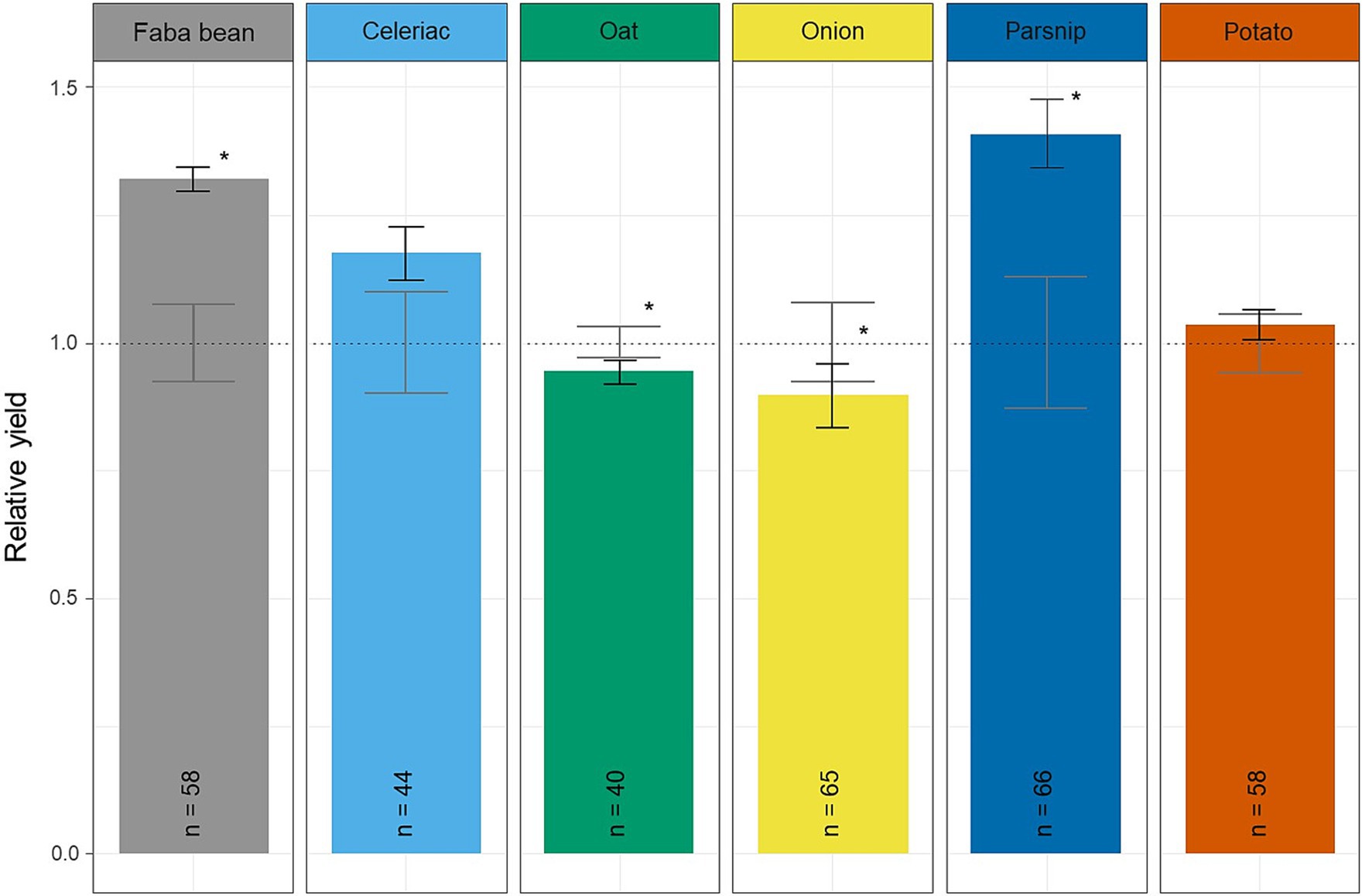
Figure 3. The relative yield of the strip compared to the sole crop for the six crops from 2020 to 2022. Bar color corresponds to the color of the crops presented in the field map (Figure 1). The RY of the sole crop which always equals 1 is represented by the dotted line. Error bars indicate the standard error of the strip (in black) and the sole crop (in grey). Asterisks indicate significant differences between the cropping systems (p < 0.05). Sample sizes per crop are shown at the bottom of each bar.
3.2 Performance of the current and alternative configurations
The current and alternative configurations resulted in LER and relative revenue higher than 1 (Table 5). LER values ranged from 1.06 to 1.11, translating to land saving proportions ranging between 5 to 10% by strip cropping to produce the same yield as in the sole crop. The current experimental design yielded a gross revenue of 12,150 €/ha while the sole crop yielded 11,560 €/ha. This means that an extra revenue of 590€/ha can be earned by strip cropping (i.e., relative revenue equals 1.05), which can accommodate a 24% increase in labor cost (i.e., breakeven labor cost ratio equals 1.24). Alternative configuration #2 showed a higher LER and an extra revenue of 759€/ha compared to the current design.
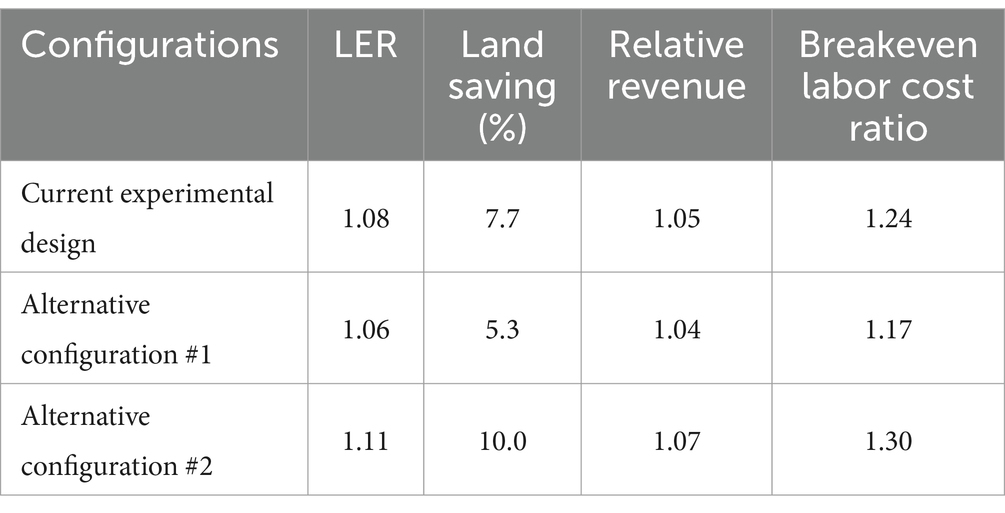
Table 5. Performance of the current experimental design and the two alternative configurations in terms of LER, land saving proportion, relative revenue, and breakeven labor cost ratio.
4 Discussion
4.1 Summary of findings
This study aimed to evaluate the effects of crop neighbors and strip cropping on yield and explore if optimizing the allocation of crop neighbors in alternative strip cropping configurations can improve yield and revenue performances. Only potato next to broccoli and celeriac showed an edge effect when comparing the edge row and the center row within a strip. We observed positive strip cropping effect in faba bean and parsnip and negative effect in oat and onion when comparing center row yield in strip with sole crop reference. Examining the overall performance of the strip cropping system across crops at the cropping system level revealed an overall positive strip cropping effect on yield. Alternative configuration #2 showed a higher LER and relative revenue compared to the current experimental design and the other alternative configuration.
4.2 Limited effects of crop neighbors
While the literature is sparse when it comes to the performance of specific crop combinations, we found that for oat, our result was consistent with Ghaffarzadeh et al. (1994) who observed non-significant but higher yield (p = 0.1) in the edge rows neighboring corn and soybean than in the center row. For potato, temporal niche differentiation due to the later sowing date of the parsnip, celeriac, and broccoli as neighboring crops may explain the trend of higher yield in the edge rows (Table 1). In 2022, the significantly higher potato yield on the edge row neighboring broccoli might be explained by the application of drip irrigation in the broccoli strip, while no irrigation was applied in the potato strip (Table 1).
One plausible reason for the limited edge effect is that positive effects are compensated by negative effect between the neighboring crops due to foliage damage at the strip border due to mechanization (Ghaffarzadeh et al., 1994; Seehusen et al., 2014). Only for potato a significant difference between yield in the edge row relative to the center row was observed despite reasonable sample size and effect size. We speculate that this might be due to the scale of observations (approximately 10 plants per sample) being insufficient to capture the variation caused by the different crop neighbors. The variation between the individual samples that neighbored the same crop was too large and could not be fully accounted for by including the variable ‘block_year’ and/or ‘transect’ as random effects in the linear mixed-effect models. The scale of large-scale systems as in this study might require sampling and/or analyzing techniques beyond plot scale. For instance, collecting yield samples from a larger area along the strip, or reducing variation between samples by accounting for spatial patterns from for example soil organic matter may allow capturing and detecting (potentially) significant effects of crop neighbors.
4.3 Significant effects of strip cropping for several crops
The effect of strip cropping compared to the sole crop was positive for faba bean and parsnip, and negative for oat and onion. The significantly higher faba bean yield in strips was in accordance with Luo et al. (2021) who observed around 30% overyielding of faba bean next to wheat. The increase in parsnip yield, however, was not aligned with the one-year experiment result in South Holland where no significant effect was observed (Hondebrink et al., 2019). The significantly lower oat yield in strips also contradicted Głowacka (2014) who found an increased grain number and weight per panicle due to positive edge effects next to corn and lupin in strips of 3.3 meters. For onion, the significantly lower yield in strips was consistent with several other studies (Broad et al., 2004; Motagally and Metwally, 2014; Luqman et al., 2020). This might be due to the low competing ability of onion against interspecific competition for light and below-ground resources, especially early in the season (Dunan et al., 1996; Ndjadi et al., 2022).
Surprisingly the inner rows in a 6-meter strip performed significantly differently than the sole crop reference. This might be because the crop neighbor effect on the edge rows of the strip seemed to be limited and the edge rows in our experiment only constitute up to one-quarter of the yield per strip. As postulated by Wang et al. (2020), the strip width, and thus the proportion of edge rows to the inner rows, determines the strength of the resulting relative yield: the higher the proportion of edge rows in a strip with a narrower width, the stronger the edge effect on the yield will be.
In the inner rows, a positive strip cropping effect might arise due to microclimate and/or reduced intraspecific competition from the poorly performing plants on the edge rows that suffer from higher interspecific competition. This might explain the higher yield observed in the center compared to the edge row for celeriac (Supplementary Table S4A). Perhaps similar to the mechanism in agroforestry or alley cropping systems, the modification of microclimate in terms of temperature, water distribution, and air movement, reduces heat and evaporative stress on the crops, thereby increasing the crop yield (Jose et al., 2004). This was often observed in the crop rows further away from the trees, where the potentially positive microclimate effect outweighs the crop-tree interspecific competition (Borin et al., 2010; Van Vooren et al., 2016).
A positive effect on yield might also arise due to the lower pest and disease pressure in strips. The potential for strip cropping to increase parasitism rate and reduce crop injury by herbivorous pests has been shown in strip-cropped cabbage, although the correlation between herbivore abundance and yield was not always consistent (Juventia et al., 2021; Croijmans, 2024; Croijmans et al., 2024). Similarly, lower Phytophthora infestans infection on potato was found in 3 m and 6 m wide strips. However, broccoli was excluded from the yield analysis and for potato, no difference in relative yield of strip compared to the sole crop was observed.
4.4 Significant effects of strip cropping across crops
A more pronounced strip cropping effect was observed when analysis was done at a higher aggregation level at the cropping system level across crops rather than per individual crop. There was an overall significantly positive effect, although only four out of six crops showed significant difference, two of which were positive and two were negative. The overyielding in parsnip, faba bean, and celeriac although not significant, apparently outweighed the under-yielding in onion and oat. This effect that was observable when the analysis was conducted across crops, indicates the value of observation at the cropping system level to evaluate the effect of strip cropping systems in practical settings and capture their potential.
4.5 Alternative strip cropping configurations
The current strip cropping design, which was co-developed by the farmers and the researchers, was aimed to systematically test the yield effect of crop neighbors, without optimizing their spatial allocation ex-ante. Alternative configuration #2 showed higher LER and relative revenue than those of the current experimental design and alternative configuration #1. Here the temporal effect of crop rotation was assumed to be the same as both the current and alternative configurations were based on the same crop rotation.
Exploring alternative crop rotations and subsequently alternative spatio-temporal strip cropping configurations using tools such as the ROTAT and RotaStrip as proposed in Dogliotti et al. (2003) and Juventia et al. (2022) could improve performance (LER equals 1.2, Supplementary Figures S5A,B). In this exploration each of the eight crops only neighbors two other crop neighbors (instead of five as in the current design, four in alternative configuration #1, or three in alternative configuration #2) such that these newly generated configurations comprise less of the lower-yielding combinations of crop neighbors. However a different crop rotation would be required for these newly generated configurations and its effects cannot be fully predicted since the temporal effect of the new crop rotation might outweigh the spatial configuration effect. As there might be trade-offs between temporal effects of rotation and spatial effects of the crop neighbor configurations, future studies should take both into account when designing strip cropping systems to optimize its performance.
4.6 Performance of the current and alternative strip cropping configurations
The advantage of the current design and alternative configurations in terms of LER that was higher than 1 (1.06–1.11) was consistent with a simulation study by Van Oort et al. (2020) and a meta-analysis on intercropping systems in Europe (Yu et al., 2015). However, our values were lower compared to previous studies that reported a strip cropping LER of 1.3 in Asia (Yu et al., 2015) and 1.49 globally (Zhu et al., 2023). LER advantage was shown to decrease under high nutrient input relative to low input (Zhu et al., 2023). This could explain our relatively lower LER values as the system studied here can be considered to be input-intensive, where nutrient application rates surpassed those in other regions globally (Lassaletta et al., 2014 in Silva et al., 2021). The 7.7% of land saved could be used to compensate for the area lost due to increased area needed for headlands or for semi-natural habitat such as flower strips within or around the field (currently taking up 3% of the strip cropping fields), so as to increase general biodiversity and pest control (Bianchi et al., 2006; Hatt et al., 2017).
The result of economic evaluation in terms of relative revenue, where the different magnitude of changes in the yield of the different crops were weighted using the farm gate price, was consistent with the LER evaluation result. The alternative configurations showed relative revenue higher than one, although lower than 1.33, the value from a global meta-analysis on intercropping systems (Martin-Guay et al., 2018). Even in the case of the lowest performing newly generated configurations, the increase in revenue of more than 500€/ha was higher than the maximum Common Agricultural Policy (CAP) additional eco-scheme subsidy in 2023 of 200€/ha that can be received through a combination of practices (e.g., strip cropping, flower strips, rest crops, alley cropping etc.) (European Commission, 2021a, 2021b; RVO, 2022).
The increase in gross revenue could be used to invest in technologies adapted to intercropping systems (Mamine and Farès, 2020; Ditzler and Driessen, 2022) and to cover potentially up to 25% increase in labor cost that may be incurred due to strip cropping. The scale of the field and experience of the farmer potentially led to no observed increase in labor costs. Other farmers associated strip cropping with more working hours due to, for example, more driving in/between the fields, more time needed for the strategic planning phase, and a higher labor demand for hand weeding when the choice of (narrow) strip width does not allow for mechanical/chemical weeding. More thorough cost–benefit and labor use efficiency analyses would be useful to assess the economic prospects of strip cropping implementation (Huang et al., 2015; Serebrennikov et al., 2020). Future studies should also take into account the potential benefit of strip cropping systems in terms of product quality and its associated price classes (e.g., higher price for fresh market quality than industry), which in turn will further improve the revenue (Juventia et al., 2021) to increase its economic feasibility.
4.7 Usefulness of the study and recommendation for future studies
The present study responds to the growing need by current and aspiring farmers, advisors, and engaged researchers in the strip cropping network in the Netherlands by bridging the knowledge gap on what constitutes good crop combinations (Isbell et al., 2017; Juventia et al., 2022), beyond plot-level scale and across seasons (Ditzler et al., 2021a). We expanded the focus beyond cereal-legume intercropping (Stomph et al., 2019) to widen the range of crop choices for strip cropping implementation. This is relevant as farmers in the Netherlands often opt for the spatially most complex ‘All crops’ configuration type which involves at least four crops, each neighboring two or more neighbors (Juventia et al., 2022). While further research and experience is needed to understand what constitute optimal crop combinations, the positive effect of strip cropping on yield and revenue, even without optimized spatial allocation suggests that the positive effect from the overall increase in diversity in the strip cropping system may compensate for the suboptimal neighbors. This may facilitate farmers to experiment with strip cropping systems. Lastly, similar to the finding by Ditzler et al. (2023), our study suggests that understanding the potential of crop diversification including strip cropping requires an evaluation at the cropping system level across crops, in addition to evaluating the effect per individual crop. This might be more evident in our study, which involved eight crops under strip cropping, than in other intercropping studies consisting of only two crops.
5 Conclusion
Currently empirical studies on what constitutes optimal crop combinations for yield in (strip) intercropping arrangements are limited. We were not able to detect edge effects on yield, with the exception for potato. When comparing the yield in strips to those in the sole crop, strip cropping yielded higher for faba bean and parsnip, and lower for oat and onion. However, when analyzed across crops, strip cropping increases both the overall yield and revenue. The proportion of land saved and the increased revenue gained by strip cropping could be used to offset the area lost to headlands or non-productive semi-natural habitats, invest in technologies adapted to strip cropping, and/or cover additional labor cost associated with it. This result especially showed the benefits of crop diversity beyond individual crop-by-crop comparison. The positive but variable strip cropping effects observed in the current experimental design and the two alternative configurations suggests prioritizing an overall increased crop diversity over optimizing their spatial arrangement. The lack of edge effect, along with the current inability of explain the mechanisms behind the observed strip cropping effects, suggests that the practical management considerations might be more important than focusing solely on yield optimization in determining crop neighbors. Further experience is needed to populate the database on what constitutes good crop combinations beyond yield performance and to test the effect of various designs in different farm contexts. This would foster the wider adoption of strip cropping in the Netherlands and Western Europe.
Data availability statement
The raw data supporting the conclusions of this article will be made available by the authors, without undue reservation.
Author contributions
SJ: Conceptualization, Data curation, Formal analysis, Investigation, Methodology, Visualization, Writing – original draft. DA: Conceptualization, Funding acquisition, Methodology, Project administration, Supervision, Writing – review & editing.
Funding
The author(s) declare that financial support was received for the research, authorship, and/or publication of this article. This project was financially supported by the TKI Agri & Food [grant number LWV19129] paid part of salary and experimental costs of SDJ and DvA, POP-3 [ZL-00442] paid for part of salary SDJ and CropMix [NWA.1389.20.160] paid for salary DvA.
Acknowledgments
We thank the two reviewers for their constructive feedback. We extend our gratitude to the ERF B. V. farm managers, Roy Michielsen and Dirk van de Weert, who made this work possible. We are grateful to the Unifarm staffs, colleagues, and the many students who contributed to the yield data collection. These include: Jelle Jolink, Joep Laan, Peter van der Zee, Bas Jonkers, Dirk Bloed, Frans Bakker, Wim Lieftink, Laura Riggi, Jiali Cheng, Andi Dirham Nasruddin, Titouan Le Noc, Marieke Datema, Cecillia Revol, Adham Muhammad, Anna de Rooij, Paolo Pezzolla, Pepijn van Hees, Julia Mechelse, Tom van Dijk, and Junjie Xu.
Conflict of interest
The authors declare that the research was conducted in the absence of any commercial or financial relationships that could be construed as a potential conflict of interest.
Publisher’s note
All claims expressed in this article are solely those of the authors and do not necessarily represent those of their affiliated organizations, or those of the publisher, the editors and the reviewers. Any product that may be evaluated in this article, or claim that may be made by its manufacturer, is not guaranteed or endorsed by the publisher.
Supplementary material
The Supplementary material for this article can be found online at: https://www.frontiersin.org/articles/10.3389/fsufs.2024.1452779/full#supplementary-material
References
Al-Amin, A. K. M. A., Lowenberg-DeBoer, J., Erickson, B. J., Evans, J. T., Langemeier, M. R., Franklin, K., et al. (2024). Economics of strip cropping with autonomous machines. Agron. J. 116, 572–589. doi: 10.1002/agj2.21536
Austin, R. B., and Blackwell, R. D. (1980). Edge and neighbour effects in cereal yield trials. J. Agric. Sci. 94, 731–734. doi: 10.1017/S0021859600028720
Bates, D., Mächler, M., Bolker, B., and Walker, S. (2015). Fitting linear mixed-effects models using lme4. J. Stat. Softw. 67, 1–48. doi: 10.18637/jss.v067.i01
Bedoussac, L., and Justes, E. (2010). The efficiency of a durum wheat-winter pea intercrop to improve yield and wheat grain protein concentration depends on N availability during early growth. Plant Soil 330, 19–35. doi: 10.1007/s11104-009-0082-2
Beillouin, D., Ben-Ari, T., and Makowski, D. (2019a). Evidence map of crop diversification strategies at the global scale. Environ. Res. Lett. 14:123001. doi: 10.1088/1748-9326/ab4449
Beillouin, D., Ben-Ari, T., and Makowski, D. (2019b). A global dataset of meta-analyses on crop diversification at the global scale. Data Brief. 24, 1–11. doi: 10.1016/j.dib.2019.103898
Bianchi, F. J. J. A., Booij, C. J. H., and Tscharntke, T. (2006). Sustainable pest regulation in agricultural landscapes: a review on landscape composition, biodiversity and natural pest control. Proc. R. Soc. Lond. B Biol. Sci. 273, 1715–1727. doi: 10.1098/rspb.2006.3530
Borin, M., Passoni, M., Thiene, M., and Tempesta, T. (2010). Multiple functions of buffer strips in farming areas. Eur. J. Agron. 32, 103–111. doi: 10.1016/j.eja.2009.05.003
Bouws, H., and Finckh, M. R. (2008). Effects of strip intercropping of potatoes with non-hosts on late blight severity and tuber yield in organic production. Plant Pathol. 57, 916–927. doi: 10.1111/j.1365-3059.2008.01860.x
Broad, S., Lisson, S., and Mendham, N. (2004). Strip cropping vegetables. 4th international crop science congress. Brisbane, Australia: Gosford, Regional Institute.
Brooker, R. W., Bennett, A. E., Cong, W. F., Daniell, T. J., George, T. S., Hallett, P. D., et al. (2015). Improving intercropping: a synthesis of research in agronomy, plant physiology and ecology. New Phytol. 206, 107–117. doi: 10.1111/nph.13132
Croijmans, L., Van Apeldoorn, D. F., Sanfilippo, F., Zangpo, T., and Poelman, E. H. (2024). Crop species diversity levels with attract and reward strategies to enhance Pieris brassicae parasitism rate by Cotesia glomerata in strip intercropping. Funct. Ecol. 38, 654–667. doi: 10.1111/1365-2435.14502
Cuperus, F., Ozinga, W. A., Bianchi, F. J. J. A., Croijmans, L., Rossing, W. A. H., and Van Apeldoorn, D. F. (2023). Effects of field-level strip and mixed cropping on aerial arthropod and arable flora communities. Agric. Ecosyst. Environ. 354:108568. doi: 10.1016/j.agee.2023.108568
Ditzler, L., and Driessen, C. (2022). Automating Agroecology: how to design a farming robot without a Monocultural mindset? J. Agric. Environ. Ethics 35:2. doi: 10.1007/s10806-021-09876-x
Ditzler, L., Rossing, W. A. H., Schulte, R. P. O., Hageman, J., and Van Apeldoorn, D. F. (2023). Prospects for increasing the resolution of crop diversity for agroecosystem service delivery in a Dutch arable system. Agric. Ecosyst. Environ. 351:108472. doi: 10.1016/j.agee.2023.108472
Ditzler, L., Van Apeldoorn, D. F., Pellegrini, F., Antichi, D., Bàrberi, P., and Rossing, W. A. H. (2021a). Current research on the ecosystem service potential of legume inclusive cropping systems in Europe. A review. Agron. Sustain. Dev. 41:26. doi: 10.1007/s13593-021-00678-z
Ditzler, L., Van Apeldoorn, D. F., Schulte, R. P. O., Tittonell, P., and Rossing, W. A. H. (2021b). Redefining the field to mobilize three-dimensional diversity and ecosystem services on the arable farm. Eur. J. Agron. 122:126197. doi: 10.1016/j.eja.2020.126197
Dogliotti, S., Rossing, W. A. H., and Van Ittersum, M. K. (2003). ROTAT, a tool for systematically generating crop rotations. Eur. J. Agron. 19, 239–250. doi: 10.1016/S1161-0301(02)00047-3
Dong, N., Tang, M.-M., Zhang, W.-P., Bao, X.-G., Wang, Y., Christie, P., et al. (2018). Temporal differentiation of crop growth as one of the drivers of intercropping yield advantage. Sci. Rep. 8:3110. doi: 10.1038/s41598-018-21414-w
Duchene, O., Vian, J.-F., and Celette, F. (2017). Intercropping with legume for agroecological cropping systems: complementarity and facilitation processes and the importance of soil microorganisms. A review. Agric. Ecosyst. Environ. 240, 148–161. doi: 10.1016/j.agee.2017.02.019
Dunan, C., Westra, P., Moore, F., and Chapman, P. (1996). Modelling the effect of duration of weed competition, weed density and weed competitiveness on seeded, irrigated onion. Weed Res. 36, 259–269. doi: 10.1111/j.1365-3180.1996.tb01655.x
European Commission (2021a). Key reforms in the new CAP. Brussels. Available at: https://agriculture.ec.europa.eu/common-agricultural-policy/cap-overview/
European Commission (2021b). List of potential agricultural practices that eco-schemes could support. Brussels. Available at: https://agriculture.ec.europa.eu/sustainability/environmental-sustainability/sustainable-agricultural-practices-and-methods_en
Gebru, H. (2015). A review on the comparative advantages of intercropping to mono-cropping system. J. Biol. Agricul. Healthcare 5, 1–13.
Ghaffarzadeh, M., Préchac, F. G., and Cruse, R. M. (1994). Grain yield response of corn, soybean, and oat grown in a strip intercropping system. Am. J. Altern. Agric. 9, 171–177. doi: 10.1017/S0889189300005932
Głowacka, A. (2013). The influence of strip cropping on the state and degree of weed infestation in dent maize (Zea mays L.), common bean (Phaseolus vulgaris L.), and spring barley (Hordeum vulgare L.). Acta Agrobotanica 66, 135–148. doi: 10.5586/aa.2013.015
Głowacka, A. (2014). The effects of strip cropping and weed control methods on yields of dent maize, narrow-leafed lupin and oats. Int. J. Plant Prod. 66, 185–194. doi: 10.5586/aa.2013.065
Guo, X., Bian, Z., Zhou, J., Wang, S., and Zhou, W. (2022). The effect of semi-natural habitat types on epigaeic arthropods: isolate habitats make critical contribution to biodiversity in agricultural landscape. Ecol. Indic. 145:109642. doi: 10.1016/j.ecolind.2022.109642
Hartig, F., (2022). DHARMa: Residual diagnostics for hierarchical (multi-level/mixed) regression models. R package version 0.4.6. Available at: https://cran.r-project.org/web/packages/DHARMa/DHARMa.pdf
Hatt, S., Lopes, T., Boeraeve, F., Chen, J., and Francis, F. (2017). Pest regulation and support of natural enemies in agriculture: experimental evidence of within field wildflower strips. Ecol. Eng. 98, 240–245. doi: 10.1016/j.ecoleng.2016.10.080
Hauggaard-Nielsen, H., (2010). Strip cropping system for sustainable food and energy production. ICROFS News-Newsletter from the International Centre for Research in Organic Food Systems, 2–3. Available at: https://orgprints.org/id/eprint/18961/4/18961.pdf
Homulle, Z., George, T. S., and Karley, A. J. (2022). Root traits with team benefits: understanding belowground interactions in intercropping systems. Plant Soil 471, 1–26. doi: 10.1007/s11104-021-05165-8
Hondebrink, M., Barbry, J., Himanen, S., Kristensen, H. L., Lepse, L., Trinchera, A., et al. (2019). Overview of farmers expected benefits of diversification: Report on national stakeholder involvement. Bunnik, the Netherlands: Louis Bolk Institute.
Huang, C., Liu, Q., Heerink, N., Stomph, T., Li, B., Liu, R., et al. (2015). Economic performance and sustainability of a novel intercropping system on the North China plain. PLoS One 10:e0135518. doi: 10.1371/journal.pone.0135518
IPES-Food (2016). “From uniformity to diversity: a paradigm shift from industrial agriculture to diversifed agroecological systems. International panel of experts on sustainable food systems” in. ed. J. Nick. Available at: R package version 0.4.6. Available at: https://ipes-food.org/wp-content/uploads/2024/03/UniformityToDiversity_FULL.pdf
Isbell, F., Adler, P. R., Eisenhauer, N., Fornara, D., Kimmel, K., Kremen, C., et al. (2017). Benefits of increasing plant diversity in sustainable agroecosystems. J. Ecol. 105, 871–879. doi: 10.1111/1365-2745.12789
Jose, S., Gillespie, A. R., and Pallardy, S. G. (2004). Interspecific interactions in temperate agroforestry. Agrofor. Syst. 61-62, 237–255. doi: 10.1023/B:AGFO.0000029002.85273.9b
Junge, X., Schüpbach, B., Walter, T., Schmid, B., and Lindemann-Matthies, P. (2015). Aesthetic quality of agricultural landscape elements in different seasonal stages in Switzerland. Landsc. Urban Plan. 133, 67–77. doi: 10.1016/j.landurbplan.2014.09.010
Justes, E., Bedoussac, L., Corre-Hellou, G., Fustec, J., Hinsinger, P., Jeuffroy, M.-H., et al. (2014). Les processus de complémentarité de niche et de facilitation déterminent le fonctionnement des associations végétales et leur efficacité pour l'acquisition des ressources abiotiques. Innov. Agronom. 40, 1–24. doi: 10.13140/2.1.1580.5125
Juventia, S. D., Norén, I. L. S., Van Apeldoorn, D. F., Ditzler, L., and Rossing, W. A. H. (2022). Spatio-temporal design of strip cropping systems. Agric. Syst. 201:103455. doi: 10.1016/j.agsy.2022.103455
Juventia, S. D., Rossing, W. A. H., Ditzler, L., and Van Apeldoorn, D. F. (2021). Spatial and genetic crop diversity support ecosystem service delivery: a case of yield and biocontrol in Dutch organic cabbage production. Field Crop Res. 261:108015. doi: 10.1016/j.fcr.2020.108015
Khanal, U., Stott, K. J., Armstrong, R., Nuttall, J. G., Henry, F., Christy, B. P., et al. (2021). Intercropping—evaluating the advantages to Broadacre systems. Agriculture 11:453. doi: 10.3390/agriculture11050453
KNMI, (2020). Maand en seizoensoverzichten jaar 2020 Koninklijk Nederlands Meteorlogisch Instituut. Available at: https://www.knmi.nl/nederland-nu/klimatologie/maand-en-seizoensoverzichten/2020/jaar
KNMI, (2021). Maand en seizoensoverzichten jaar 2021 Koninklijk Nederlands Meteorlogisch Instituut. Available at: https://www.knmi.nl/nederland-nu/klimatologie/maand-en-seizoensoverzichten/2021/jaar
KNMI, (2022). Maand en seizoensoverzichten jaar 2022 Koninklijk Nederlands Meteorlogisch Instituut. Available at: https://www.knmi.nl/nederland-nu/klimatologie/maand-en-seizoensoverzichten/2022/jaar
KNMI, (2023). Climate normals −1991-2020 climate normals by metric. https://dataplatform.knmi.nl/dataset/climate-normals-1991-2020-climate-normals-by-metric-1
Kragten, S., and De Snoo, G. R. (2008). Field-breeding birds on organic and conventional arable farms in the Netherlands. Agric. Ecosyst. Environ. 126, 270–274. doi: 10.1016/j.agee.2008.02.006
Kremen, C., and Miles, A. (2012). Ecosystem services in biologically diversified versus conventional farming systems: benefits, externalities, and trade-offs. Ecol. Soc. 17:40. doi: 10.5751/ES-05035-170440
Landis, D. A., Wratten, S. D., and Gurr, G. M. (2000). Habitat management to conserve natural enemies of arthropod pests in agriculture. Annu. Rev. Entomol. 45, 175–201. doi: 10.1146/annurev.ento.45.1.175
Lassaletta, L., Billen, G., Grizzetti, B., Anglade, J., and Garnier, J. (2014). 50 year trends in nitrogen use efficiency of world cropping systems: the relationship between yield and nitrogen input to cropland. Environ. Res. Lett. 9:105011. doi: 10.1088/1748-9326/9/10/105011
Lee, J. C., Menalled, F. D., and Landis, D. A. (2001). Refuge habitats modify impact of insecticide disturbance on carabid beetle communities. J. Appl. Ecol. 38, 472–483. doi: 10.1046/j.1365-2664.2001.00602.x
Lopes, T., Bodson, B., and Francis, F. (2015). Associations of wheat with pea can reduce aphid infestations. Neotrop. Entomol. 44, 286–293. doi: 10.1007/s13744-015-0282-9
Luo, C., Zhu, J., Ma, L., Guo, Z., Dong, K., and Dong, Y. (2021). Effects of nitrogen regulation and strip intercropping on faba bean biomass, nitrogen accumulation and distribution, and interspecific interactions. Crop Sci. 61, 4325–4343. doi: 10.1002/csc2.20556
Luqman, L., Hussain, Z., Ilyas, M., Khan, I., and Bakht, T. (2020). Influence of sowing orientation and intercropping of chilies on onion yield and its associated weeds in Peshawar, Pakistan. Pakistan J. Botany 52, 95–100. doi: 10.30848/PJB2020-1(6)
Malézieux, E., Crozat, Y., Dupraz, C., Laurans, M., Makowski, D., Ozier-Lafontaine, H., et al. (2009). “Mixing plant species in cropping systems: concepts, tools and models: a review” in Sustainable Agriculture. eds. E. Lichtfouse, M. Navarrete, P. Debaeke, S. Véronique, and C. Alberola (Netherlands, Dordrecht: Springer), 329–353.
Mamine, F., and Farès, M. H. (2020). Barriers and levers to developing wheat–pea intercropping in Europe: a review. Sustain. For. 12:6962. doi: 10.3390/su12176962
Martin-Guay, M.-O., Paquette, A., Dupras, J., and Rivest, D. (2018). The new green revolution: sustainable intensification of agriculture by intercropping. Sci. Total Environ. 615, 767–772. doi: 10.1016/j.scitotenv.2017.10.024
Mead, R., and Willey, R. W. (1980). Concept of a “Land Equivalent Ratio” and Advantages in Yields from Intercropping. Exp. Agric. 16, 217–228. doi: 10.1017/S0014479700010978
Motagally, F. A., and Metwally, A. (2014). Maximizing productivity by intercropping onion on sugar beet. Asian J. Crop Sci. 6, 226–235. doi: 10.3923/ajcs.2014.226.235
Mu, Y., Chai, Q., Yu, A., Yang, C., Qi, W., Feng, F., et al. (2013). Performance of wheat/maize intercropping is a function of belowground interspecies interactions. Crop Sci. 53, 2186–2194. doi: 10.2135/cropsci2012.11.0619
Ndjadi, S. S., Vissoh, P. V., Vumilia, R. K., Mondo, J. M., Mugumaarhahama, Y., Saidou, A., et al. (2022). Yield potential and land-use efficiency of onion (Allium cepa L.) intercropped with peanut (Arachis hypogaea L.) under organic soil fertility management in south-Kivu, eastern DR Congo. Bulgarian J. Agr. Sci. 28, 647–657.
Ponisio, L. C., M'Gonigle, L. K., Mace, K. C., Palomino, J., de Valpine, P., and Kremen, C. (2015). Diversification practices reduce organic to conventional yield gap. Proc. R. Soc. B 282:20141396. doi: 10.1098/rspb.2014.1396
R Core Team (2020). R: A language and environment for statistical computing. Vienna, Austria: R Foundation for Statistical Computing.
Rosa-Schleich, J., Loos, J., Mußhoff, O., and Tscharntke, T. (2019). Ecological-economic trade-offs of diversified farming systems–a review. Ecol. Econ. 160, 251–263. doi: 10.1016/j.ecolecon.2019.03.002
Schouten, C. (2018). Agriculture, nature and food: Valuable and connected. Vision Ministry of of Agriculture, Nature and Food Quality: The Netherlands as a leader in circular agriculture.
Seehusen, T., Børresen, T., Rostad, B. I., Fleige, H., Zink, A., and Riley, H. (2014). Verification of traffic-induced soil compaction after long-term ploughing and 10 years minimum tillage on clay loam soil in south-East Norway. Acta Agricul. Scand. Sec. B. 64, 312–328. doi: 10.1080/09064710.2014.907582
Serebrennikov, D., Thorne, F., Kallas, Z., and McCarthy, S. N. (2020). Factors influencing adoption of sustainable farming practices in Europe: a systemic review of empirical literature. Sustain. For. 12:9719. doi: 10.3390/su12229719
Shapiro, S. S., and Wilk, M. B. (1965). An analysis of variance test for normality (complete samples). Biometrika 52, 591–611. doi: 10.1093/biomet/52.3-4.591
Silva, J. V., Van Ittersum, M. K., Ten Berge, H. F. M., Spätjens, L., Tenreiro, T. R., Anten, N. P. R., et al. (2021). Agronomic analysis of nitrogen performance indicators in intensive arable cropping systems: an appraisal of big data from commercial farms. Field Crop Res. 269:108176. doi: 10.1016/j.fcr.2021.108176
Sirami, C., Gross, N., Baillod, A. B., Bertrand, C., Carrié, R., Hass, A., et al. (2019). Increasing crop heterogeneity enhances multitrophic diversity across agricultural regions. Proc. Natl. Acad. Sci. 116, 16442–16447. doi: 10.1073/pnas.1906419116
Stomph, T., Dordas, C., Baranger, A., De Rijk, J., Dong, B., Evers, J., et al. (2019). Designing intercrops for high yield, yield stability and efficient use of resources: are there principles? Adv. Agron. 160, 1–50. doi: 10.1016/bs.agron.2019.10.002
Tamburini, G., Bommarco, R., Wanger, T. C., Kremen, C., Van der Heijden, M. G. A., Liebman, M., et al. (2020). Agricultural diversification promotes multiple ecosystem services without compromising yield. Science. Advances 6:eaba1715. doi: 10.1126/sciadv.aba1715
Vandermeer, J. (1989). The Ecology of Intercropping. Cambridge: Cambridge University Press, 237. doi: 10.1017/CBO9780511623523
Van der Voort, M.P.J., (2022). KWIN-AGV 2022: Kwantitatieve Informatie Akkerbouw en Vollegrondsgroenteteelt. (Rapport / Wageningen Plant Research; No. WPR-OT 941). Wageningen Plant Research.
Van der Werf, W., Zhang, L., Li, C., Chen, P., Feng, C., Xu, Z., et al. (2021). Comparing performance of crop species mixtures and pure stands. Front. Agricul. Sci. Eng. 8, 481–489. doi: 10.15302/j-fase-2021413
Van Oort, P. A. J., Gou, F., Stomph, T. J., and Van der Werf, W. (2020). Effects of strip width on yields in relay-strip intercropping: a simulation study. Eur. J. Agron. 112:125936. doi: 10.1016/j.eja.2019.125936
Van Vooren, L., Reubens, B., Broekx, S., Pardon, P., Reheul, D., Van Winsen, F., et al. (2016). Greening and producing: an economic assessment framework for integrating trees in cropping systems. Agric. Syst. 148, 44–57. doi: 10.1016/j.agsy.2016.06.007
Vereijken, P. (1997). A methodical way of prototyping integrated and ecological arable farming systems (I/EAFS) in interaction with pilot farms. Eur. J. Agron. 7, 235–250. doi: 10.1016/S1161-0301(97)00039-7
Wang, R., Sun, Z., Zhang, L., Yang, N., Feng, L., Bai, W., et al. (2020). Border-row proportion determines strength of interspecific interactions and crop yields in maize/peanut strip intercropping. Field Crop Res. 253:107819. doi: 10.1016/j.fcr.2020.107819
Willey, R.W., (1979). Part 1. Competition and yield advantages. Intercropping its importance and research needs. MPKV, Maharastra: Field Crop Abstracts, 32, 1–10.
Yu, Y., Stomph, T.-J., Makowski, D., and Van der Werf, W. (2015). Temporal niche differentiation increases the land equivalent ratio of annual intercrops: a meta-analysis. Field Crop Res. 184, 133–144. doi: 10.1016/j.fcr.2015.09.010
Keywords: intercropping, diversified cropping systems, crops combinations, land equivalent ratio, relative revenue
Citation: Juventia SD and van Apeldoorn DF (2024) Strip cropping increases yield and revenue: multi-year analysis of an organic system in the Netherlands. Front. Sustain. Food Syst. 8:1452779. doi: 10.3389/fsufs.2024.1452779
Edited by:
Callum Eastwood, DairyNZ, New ZealandReviewed by:
Edward Dickin, Harper Adams University, United KingdomJames Lowenberg-DeBoer, Harper Adams University, United Kingdom
Copyright © 2024 Juventia and van Apeldoorn. This is an open-access article distributed under the terms of the Creative Commons Attribution License (CC BY). The use, distribution or reproduction in other forums is permitted, provided the original author(s) and the copyright owner(s) are credited and that the original publication in this journal is cited, in accordance with accepted academic practice. No use, distribution or reproduction is permitted which does not comply with these terms.
*Correspondence: Stella D. Juventia, anV2ZW50aWEuc3RlbGxhQHd1ci5ubA==