- 1International Center for Agricultural Research in the Dry Areas (ICARDA), Ariana, Tunisia
- 2National Institute of Agronomy of Tunisia (INAT), University of Carthage, Tunis, Tunisia
- 3Research Group Landscapes and Geo-Mediation, Higher Agronomic Institute of Chott-Mariem (ISA-CM), University of Sousse, Sousse, Tunisia
- 4Centre for International Cooperation in Agricultural Research for Development (CIRAD), Paris, France
Integration of productivity, resource management, and institutional innovations is crucial across different system levels. Traditional research and extension services face challenges in disseminating innovations effectively, leading to the emergence of the agricultural innovation system (AIS) approach. AIS involves collaboration among various stakeholders to improve the technological, managerial, and institutional aspects of agriculture. Intermediary actors play a pivotal role in facilitating innovation exchange and learning processes. In Tunisia, farmer organizations (FOs) such as Groupements de Développement Agricole (GDAs) and Sociétés Mutuelles de Services Agricoles (SMSAs) are key intermediaries. However, there is a lack of comprehensive assessments of FOs' innovation roles, which this study aims to address using the social network analysis (SNA) method. The findings aim to shed light on FOs' effectiveness as innovation intermediaries and identify potential challenges and benefits associated with their roles in innovation processes through the comparison of three farmers' groups divided by their membership status to detect the effect of FO presence or absence on innovation facilitation and actor's configuration. The studied sample involves 592 farmers from the Kef and Siliana governorates in northwestern Tunisia. The results show that FOs are playing a crucial role in facilitating collaboration and knowledge exchange among farmers and other agricultural actors. However, their effectiveness varies depending on membership status and is always contextually influenced. While FOs contribute to reshaping sales channels and optimizing access to services, they face limitations in promoting inclusivity and equitable knowledge sharing. The recommendations include strengthening FOs' social dimension, addressing trust barriers, and investing in reinforcement measures, especially in human capital, to enhance their functionality. Strengthening measures can take many forms, such as facilitating regulation and investing in capacity building. Long-term strategies should re-evaluate the effectiveness of collective action and focus on fostering a culture of shared learning and local empowerment.
1 Introduction
Commonly, sustainable intensification (SI) aims at enhancing the economic returns generated from an agricultural activity unaccompanied by negative impacts on natural resources and ecosystems (Pretty and Bharucha, 2014). Shifting to a sustainably intensified agricultural system entails moving beyond conventional practices that rely on external inputs to a more restorative form of agriculture that prioritizes internal ecological processes (Garnett et al., 2013). SI addresses universal worries about agricultural activity's detrimental impact on environmental quality and natural resource availability, as well as the necessity of achieving effective increases in yields on existing land without further depleting natural habitats (Godfray and Garnett, 2014). This kind of balance between achieving future food security and environmental safeguarding makes SI foreseen as a promoting and important pathway for agriculture (Thomson et al., 2019). Even if it addresses ensuring food security with a strong productivism approach, SI alone is not a sufficient condition for the sustainable development of food systems and food security insurance (Belton et al., 2020). SI requires integrating productivity, natural resource management, and institutional innovations in an agile way to ensure the pragmatic and rational use of the existing agroecological, anthropic, and financial resources across different system levels in a specific context (Robinson et al., 2015). Even if the importance of institutional innovation as a supporter of SI is acknowledged, more attention must be given to this type of innovation in productivity and natural resource management (Schut et al., 2016). So, we can understand that SI and agricultural innovation are closely linked, and achieving the purpose of sustainability still definitely depends on how we consider agricultural innovation (Dicks et al., 2019).
In general, innovation is one of the drivers of economic development, as any technological progress is an essential contributor to ensuring resource use efficiency (Jorgenson, 2011). This optimization has always been part of humans' evolutionary mindset (Sterelny, 2020). Simply, “innovation” can refer to anything newly introduced into an economic or social process in a specific context. Agricultural innovation always has hurdles to increasing productivity in some way or another in total households and/or in the agricultural sector. It also involves adapting to changing environmental conditions, addressing social and economic challenges, and promoting sustainable practices that benefit both farmers and the environment (Ogundari and Bolarinwa, 2018; Pigford et al., 2018).
Traditional research and extension services face several challenges in facilitating the dissemination of agricultural innovation, such as weakened interactions among institutions and stakeholders. Furthermore, it has been shown by research and development (R&D) experts that top-down strategies are often unsuccessful in meeting the context-specific needs of smallholders. These challenges have paved the way for the emergence of a new concept, which is the “agricultural innovation system” (Faure et al., 2012). This concept always insists that it should be considered from a systematic and integrated perspective, aiming for sustainability promotion and addressing multiple challenges at different system levels (Gutiérrez Cano et al., 2023).
To promote innovation in Tunisia, a tentative reform took place starting in the early 2000's (Canesse, 2011), based on the idea of irrigation management restructuration through the creation of a new organizational form called agricultural development groups (GDAs; Ben Mustapha et al., 2015). These farmer's associations were expected to be involved in local, rural, and agricultural development (Elloumi, 2006). These GDAs participate in other activities such as agricultural extension, common resource management, governance, and farmer networking (Mhadhbi, 2021). Besides the GDAs, another organizational form was reintroduced in the early 2000's (2005 law): the mutual companies for agricultural services (SMSAs), a renewed form of the cooperatives. In the post-revolution socio-political context, it was highly promoted and encouraged (Canesse, 2011). These choices have shown many limitations, and the positioning of these FOs in AIS has received a lot of criticism (Dolinska, 2017).
While the establishment and strengthening of these GDAs and SMSAs have consistently been central to national policies and numerous R&D initiatives, there remains a lack of efficacy assessments of FO roles in the Tunisian context within the existing literature. These deficits and limitations encompass various aspects, including the methodology for evaluating the roles and impacts of these associations as innovation intermediaries. The main aim of this research is to conduct a comprehensive assessment of the innovation roles played by FOs within the Tunisian context. It also seeks to provide a better understanding of FOs functioning as key intermediaries in the innovation process and critical issues that challenge the efficacy of these FOs. The added value of this study is that it addresses these existing knowledge gaps.
2 Conceptual framework
2.1 Theoretical background
The classic definition of “innovation system” insists on three elements: the actors involved in generating and disseminating the new knowledge or the innovation itself; the co-learning through the process; and the institutional formal and informal linkage behind it (North, 1995). Other definitions insist that it is a totality based on knowledge generation, exchange, and use (Spielman, 2006). AIS, as introduced by the World Bank in 2008, is based on the application of an approach derived from the industrial sector and is a rising concept advocated for system approaches (Gutiérrez Cano et al., 2023).
The core idea behind this AIS approach is concentrated on smoothing the complex interaction and the effective reconfiguration of the actors' tissue in service of technical, social, and institutional development in an inclusive way (Schut et al., 2016). This certainly requires the involvement of different stakeholder categories. The facilitation of interactive learning among these actors goes through intermediary actors and structures that play a crucial role in the innovation process (Meyer and Kearnes, 2013). Howells (2006) defines the intermediary actors in AIS as the organizations playing the role of brokers of access to knowledge and services from one actor to another. The two major functions of these organizations are the support of innovation through knowledge mediation through the reinforcement of information exchange and fostering the learning process, and the second function is innovation material intermediation, which encloses providing the required inputs, facilitating access to market opportunities, and providing related services (Kilelu et al., 2011). According to Yang et al. (2014), many actors from the food system in developing countries can play this role, for example: non-governmental organizations (NGOs), national extension systems (NESs), FOs, and producers' cooperatives. The FOs (the subject of this study) fulfill these functions as intermediaries in supporting agricultural innovations.
2.2 Innovation intermediaries: a definition
The concept of agricultural innovation intermediation or brokerage involves joining actors' roles, such as learning, co-generation of knowledge, and scaling efforts to disseminate innovation among the AIS (Hermans et al., 2013). Various studies, including those by Howells (2006), Kilelu et al. (2011), Yang et al. (2014), and Iyabano et al. (2022) explored the functions and roles of innovation intermediaries in the agricultural innovation process. These studies reveal that innovation intermediaries, also called by Dicecca et al. (2016) “brokers,” play a set of roles, including facilitating collaboration, brokering knowledge, transferring technology, building capacity, connecting farmers to various actors, and facilitating access to inputs (such as retailers), extension services, processors, and markets.
Agricultural innovation intermediaries exist in various forms. Public intermediaries, which are generally government-funded or linked to public institutions provide research, extension, and policy advocacy services, although these may be restricted by bureaucracy and financial barriers, leading to a lower response rate toward changing needs (Klerkx et al., 2010). Private intermediaries (private companies, NGOs, and cooperatives) are often more market-responsive but may have limited access for smallholder farmers due to their profit-driven nature (Turner et al., 2016).
Furthermore, intermediaries can be classified into two types: formal and informal intermediaries. Structured and reliable results are provided by formal intermediaries such as research institutions and universities, but these intermediaries may lack the necessary flexibility to effectively address local needs. Power dynamics and resource availability are the main factors influencing the effectiveness of these intermediaries. Making solid connections with policymakers can improve the influence of intermediaries on agricultural policies and facilitate finding funding opportunities (Klerkx et al., 2010). An intermediary's ability to foster innovation is often influenced by the availability of financial, human, and informational resources, although public intermediaries usually have budget constraints while private intermediaries are encountered by market forces during their operations (Howells, 2006). In addition, the effectiveness of an intermediary is always determined by various aspects, such as the production system, market conditions, and socioeconomic determinants (Spielman, 2007).
2.3 FOs as innovation brokers
In developing countries, FOs play a central role as innovation brokers through the mobilization of their network to disseminate knowledge among farmers and at the community level (Dutrénit et al., 2012). In a review study by Bizikova et al. (2020) in a set of countries of Sub-Saharan Africa and India, the impacts of FO membership on smallholders demonstrated positive impacts on farmers' income, yield and production, as well as on food security and the environment, through the dissemination of “good” agricultural practices and the facilitation of farmers' access to information, technical knowledge and support.
2.4 Conceptual framework
Figure 1 illustrates the innovation's intermediary functions of FOs, highlighting their roles in brokering networks, providing innovation support, and offering material and non-material assistance to promote agricultural innovation. From an institutional perspective, FOs help to build networks with other stakeholders within the agricultural sector through various ways, including collaboration and partnerships (Gouët and Van Paassen, 2012). They facilitate the establishment of formal or informal connections with government agencies, research institutions, input suppliers, and market actors. These linkages are the basis of the intermediation role and innovation support (Cofré-Bravo et al., 2019).
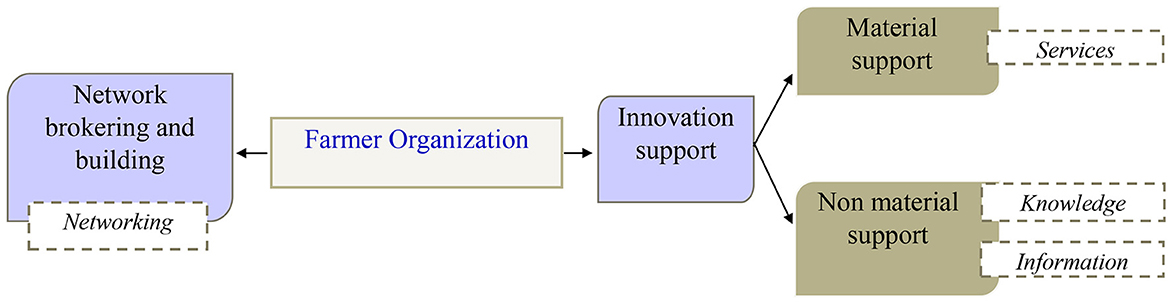
Figure 1. FOs' innovation intermediation functions (own elaboration; based on Howells, 2006; Kilelu et al., 2011; Yang et al., 2014; Iyabano et al., 2022).
From an innovation perspective, the type of support provided by the FOs to farmers and communities can be material or non-material (Chandrakar et al., 2023). Material support is related to facilitating access to machinery, financial support, inputs, and markets (Shrivastava et al., 2022; Kumari et al., 2022). Non-material support is related to knowledge and information exchange with farmers (Parthiban Sakhti et al., 2015). This non-material support includes the organization of training sessions to spread new agricultural practices, technologies, and innovations (Msuta and Urassa, 2015). In terms of information sharing, FOs facilitate the exchange of information among farmers and other actors related to market trends, opportunities, and new research findings (Krush et al., 2015). This can help farmers stay updated on cutting-edge innovations and best practices (He et al., 2018). Those functions are to be explored to better assess the role of FO in agricultural innovation processes.
3 Methodology
The research methodology was based on a quantitative analysis. The study was conducted in the northwest region of Tunisia, characterized by a mixed tree-crop-livestock farming system.
3.1 Case study
To evaluate the effectiveness of FO roles as innovation intermediaries in the Tunisian context, four FOs (SMSA Chouarnia, GDA Sers, SMSA El Rhahla, and SMSA Kesra) were selected according to their engagement and participation in a set of research for development programs for SI and promoting agroecology. The study area was located in the transect of Kef-Siliana governorates in northwestern Tunisia (Figure 2), in the context of crop-livestock rainfed systems. SMSA Chouarnia and GDA Sers promoted innovations and agricultural practices related to conservation agriculture, small machinery uses for byproduct valorization, and forage mixtures incorporated in rotation. The SMSA El Rhahla focused more on sustainable soil and water conservation techniques in the context of land degradation and water scarcity. The SMSA Kesra was working on developing the local products market and agroforestry activities, as it was based in a mountainous area (Alary et al., 2022).
3.2 Methods and data collection
To assess the effectiveness of FO innovation intermediation and facilitation roles as described in Figure 1 and to perform the needed analysis (Table 1), data were gathered through surveys carried out between November 2022 and January 2023, with each session lasting ~60 min. Interviews were conducted in-person and guided by a set of close-ended questions designed to investigate (i) farmers' profiles and farming activity characterization and (ii) farmers' relations with various actors in the agricultural system, knowledge, information, and service flow. The first section focused on household demography, labor, land use, income, livestock inventory, products' self-consumption rates, input use, and costs. The second section focused on addressing questions on membership status, tracing the existence or absence of links with a predefined list of actors, and the type, nature, and frequency of these links.
A disproportionate stratified sampling method was used. This method is particularly suitable when studying specific subgroups that may be smaller in number but are critical to the research objectives (Levy and Lemeshow, 2008). It enhances the generalizability and validity of the study while minimizing research biases (Kim and Wang, 2019). A representative sample touching a total of 592 farmers from the studied zone (Figure 2) included three strata: members of the correspondent FO (164 farmers), non-member beneficiary farmers (216), and non-member and non-beneficiary farmers (212). A simple random sampling was applied within each stratum (Hansen et al., 1953). This segregation unfolded in three types and was the basis of comparison in this study. “Members,” corresponded to farmers who had paid an annual or monthly subscription to the FO. For “non-member beneficiary farmers,” they were those who did not pay the subscription fees but participated in the events organized by the FOs and/or had access to paid or free services provided by the FO. “Non-member and non-beneficiary farmers” were those farmers who were out of the FO's network. They did not have any kind of collaboration with the FO in terms of services or any other form of support. Farmers' interviews helped to capture the overview of farming activity (the context) and the innovation intermediation roles (Figure 1) played by the FOs from the agricultural innovation final users' perspective.
The various steps of analysis and their respective aims are described in Table 1 to contextualize the studied sample. The farming profiles were reflected through an assessment of six domains: human capital, production potential, availability of financial resources, farm characteristics, production intensification, and production goals. The choice of the variables was based on a review of a set of studies related to farm typology and farm characterization, such as those by Alvarez et al. (2018) and El Ansari et al. (2020). This characterization was followed by a Kruskal–Wallis test to compare these farming profiling variables among three groups of farmers, depending on their membership or relationship with FO. The choice of this test is explained by the number of groups (3) and only one categorical variable, which is membership (McKight and Najab, 2010).
The second step was basically done using the SNA methodology to assess the intermediation roles among the three farmers' groups (members, non-members, and beneficiaries; non-member and non-beneficiary). This method offers a clear understanding of the relationship between the studied farmers and their position in the entire network or agricultural system (Devi and Tripathi, 2020). It also demonstrated its efficiency under an AIS approach (Schut et al., 2017). It is also considered as a flexible method (El Amrani and Beni Houd, 2022). Even though SNA is a useful and recommended tool, it faces significant limitations (Redhead et al., 2023). As it heavily relies on self-reported data, it can introduce biases such as social desirability and recall bias, which affect the results' reliability (Kolleck, 2013). So, applying this method required caution to prevent oversimplification and maintain the accuracy of the assumptions.
For, the collected and compiled data, as presented in Table 2, in the subsequent analysis, certain variables were utilized directly, while for others, specific calculations were conducted to derive more reflective variables. Furthermore, a list of actors, along with the acronyms or abbreviations associated with a description, is presented in Appendix 1 to facilitate understanding the role of each.
For contextualization, all data manipulation and analysis were executed using IBM SPSS 25. While the SNA was performed using Gephi software. The results of SNA have been reflected in four main indicators, which were calculated using the following formulas:
; A is the adjacency matrix, and Avu = 1 if there is an edge between node v and node u, and 0 otherwise
; wvu is the weight or the frequency of the edge between node v and node u
; d(v, u) is the shortest distance between node v and node u
; λ is a constant (the largest eigenvalue of the adjacency matrix A) and EC(u) is the eigenvector centrality of node u.
4 Results
4.1 Contextualization
Table 3 presents a comparative analysis pertaining to distinct domains that delineate farmers' profiles within three distinct farmers' groups. A significant difference (at 95% level) among the three groups existed in human capital, notably in terms of educational level, FO members exhibited the highest mean education level with a value of 6.35 years (corresponding to a primary school level) compared to 4.05 and 4.68, respectively. Additionally, a significant difference in family size was observed between groups.
Conversely, the production potential demonstrated no statistically significant differences in terms of land size and irrigation. Regarding farm characteristics, livestock (sheep, cattle, and goat), trees, annual crops, and grazing-related indicators revealed no discernible disparities among the three groups.
The same level of intensification, as reflected by metrics such as manure utilization, seed cost, and on-land pressure, was observed across all three groups. In terms of production goals, no statistically significant differences emerged among the groups. The results revealed that the main differences between groups were very limited, and the FO affiliation was not dependent on the compared characteristics except for two significant differences related to human capital.
4.2 Network brokering
Table 4 provides a comparison of collaboration dynamics within distinct farm groups (Figure 3). Notably, the frequency of collaborations with various actors exhibited a clear difference among members, beneficiaries, and non-beneficiaries. Members, on average, engaged with 4.7 actors, reflecting an extensive collaboration. In contrast, the beneficiary group demonstrated an average collaboration with 3.73 actors, while non-beneficiaries engaged with a more limited pool, averaging 2.33 actors. The same pattern was also relevant for collaboration intensity, reflected in weighted degrees, where members had the highest value of 45.65, followed by beneficiaries at a level of 37.4, and non-beneficiaries with the lowest value of 21.44.
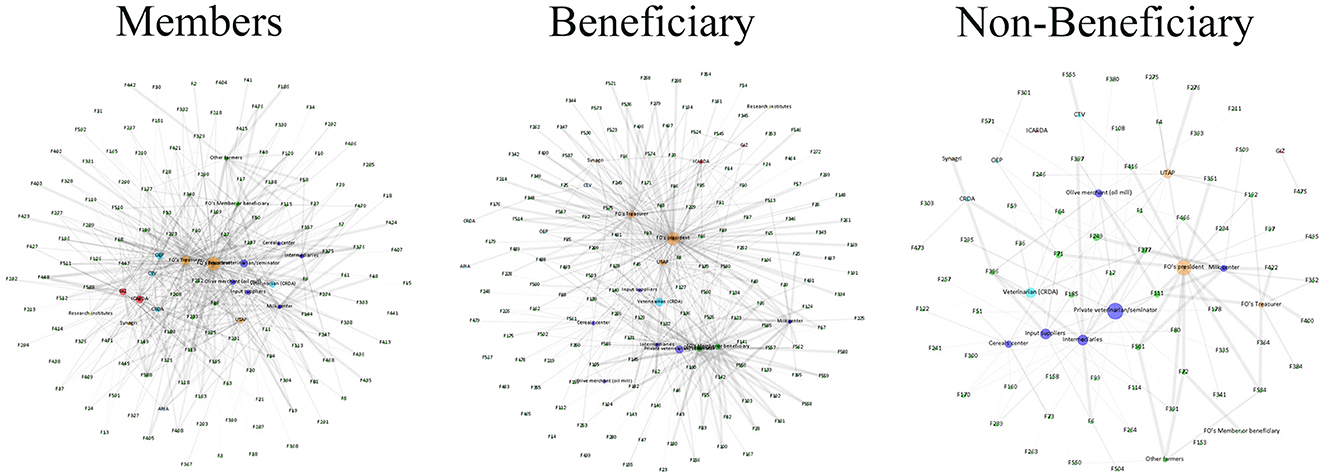
Figure 3. Social network graphic illustrations for network brokering function (legend: green = farmers; orange = farmer organization; red = NGOs; blue = business/private; turquoise = development, administration and extension system; yellow= research and university).
Exploring eigenvector centrality, members exhibited the highest values, particularly with regard to the FO's president (1), FO treasurer (0.75), and collaboration with international organizations such as ICARDA (0.6) and OEP (0.56), along with a private veterinarian (0.56) and GIZ (0.49). Beneficiary farmers similarly prioritized collaboration with the FO's president (1) but exhibited elevated values for collaboration with other farmers (0.51) and private and public veterinarians (0.64 and 0.69, respectively). In contrast, non-beneficiaries placed a premium on collaboration with private veterinarians (0.78), FO's president (0.62), and input suppliers (0.58).
Closeness and centrality showed other patterns. Members exhibited a peak value of 0.65 for the FO president and close values ranging between 0.4 and 0.45 for actors from international organizations and other farmers. Beneficiaries mirrored this same pattern, while non-beneficiaries deviated, with the highest closeness centrality attributed to GIZ (0.65), followed by private veterinarians. Results reflected the nuances in collaborative dynamics across diverse farmers' groups.
4.3 Knowledge exchange
In the context of the knowledge exchange network (Table 5 and Figure 4), among members, FO's president held the highest centrality score at 0.54. International organizations exhibited notable scores of 0.47. Other actors within this group had scores ranging from 0.31 to 0.4. For the beneficiary group, OEP emerged with the highest closeness centrality score of 1. FO's president followed closely with a score of 0.57, while the Milk Center maintained a score approaching 0.5. Conversely, null scores characterized CRDA, CTV, APIA, Synagri, and research institutes. Examining the non-beneficiary group, intermediaries attained the highest closeness centrality score of 1, with moderate scores ~0.45 for CTV, olive merchants, and input suppliers. In contrast, international organizations (CRDA, OEP, and APIA) registered null scores.
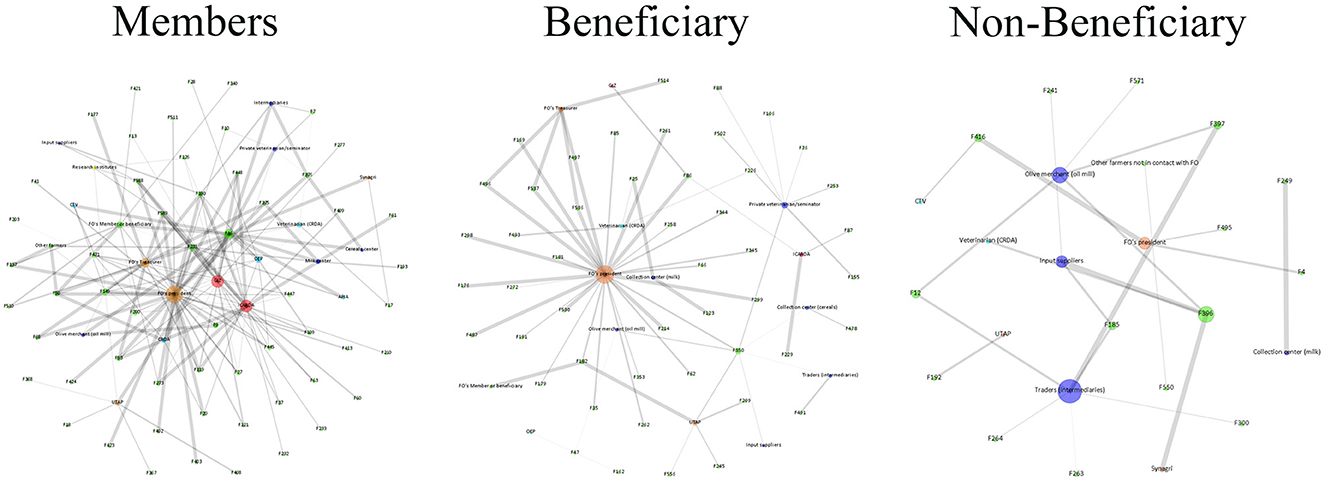
Figure 4. Social network graphic illustrations for knowledge exchange function (legend: green = farmers; orange = farmer organization; red = NGOs; blue = business/private; turquoise = development, administration and extension system; yellow= research and university).
Turning to eigenvector centrality, within the members' group, FO's president commanded the highest score of 1. High values of 0.81 and 0.80 characterized ICARDA and GIZ, respectively, while FO's treasurer held a moderate centrality score of 0.56, followed by OEP with a value of 0.35. For beneficiary farmers, FO's president again took the lead in eigenvector centrality, while CRDA, OEP, APIA, and research institutes maintained null values. Other actors in this group exhibited relatively low scores, ranging from 0.01 to 0.17. In the non-beneficiary group, intermediaries had the highest eigenvector centrality score of 1, followed by olive merchants and input suppliers with scores of 0.77 and 0.49, respectively. Null values were observed for CRDA, APIA, research institutes, ICARDA, and GIZ.
4.4 Information exchange
In terms of information exchange, the closeness and centrality values presented in Table 6 revealed differences among various groups (Figure 5). For the first group (members), FO's president emerged as the most central figure, attaining the highest value of 0.62, followed closely by FO's treasurer with a score of 0.48. ICARDA and OEP exhibited comparable high values of 0.43, while CTV and CRDA closely trailed with scores approaching 0.4. In contrast, research institutes demonstrated relatively lower centrality, registering at 0.35. Examining the beneficiary farmers' group, GIZ stood out with the highest centrality value of 1. FO's president also retained a significant score of 0.49. Furthermore, the null values (indicated as 0) for CRDA and research institutes reflected the limitations of these actors. CTV and OEP exhibited diminished centrality values, recorded at 0.23 and 0.32, respectively, compared to the members' group. For non-beneficiaries, research institutes displayed null centrality values. Extension-related actors such as CRDA, CTV, and OEP consistently maintained moderate centrality values ~0.3. FO's president again boasted a high centrality value of 0.46.
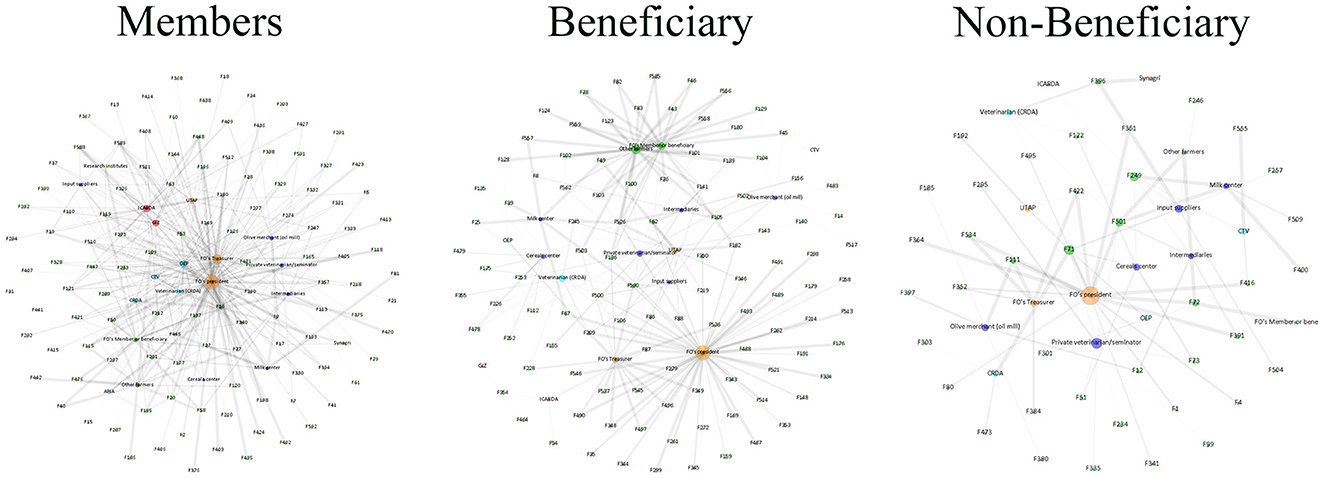
Figure 5. Social network graphic illustrations for information exchange function (legend: green = farmers; orange = farmer organization; red = NGOs; blue = business/private; turquoise = development, administration and extension system; yellow= research and university).
Concerning eigenvector centrality, within the members' network, FO's president commanded the highest centrality with a score of 1. OEP followed closely with a value of 0.58, while ICARDA also demonstrated a noteworthy score of 0.55. In contrast, research institutes exhibited the lowest eigenvector centrality value, reaching only 0.15. Among the beneficiary group, FO's president attained an eigenvector centrality score of 1. Other farmers and members/beneficiaries also demonstrated moderately important values of 0.56 and 0.48. UTAP exhibited a score of 0.4, followed closely by veterinarians and private veterinarians with 0.38 and 0.36, respectively. However, actors such as CRDA, research institutes, and Synagri registered a null eigenvector centrality score.
In contrast, for the non-beneficiary group, FO's president remained with a score of 1, while research institutes exhibited null eigenvector centrality values. CRDA, CTV, and OEP displayed relatively higher values of 0.15, 0.14, and 0.14, respectively, compared to their counterparts in the beneficiary group.
4.5 Services' provision
Looking at the closeness centrality in Table 7, there was not a big difference between the groups for most actors (Figure 6), except for the ones linked to the FO, such as members, the treasurer, the president, and the OEP. For members, the closeness score for private veterinarian was 0.51, followed by the FO president with 0.5. In the beneficiary group, the FO's president took the lead with a score of 0.56. For non-beneficiaries, the FO's M or B has the lowest score with a value of 0.00.
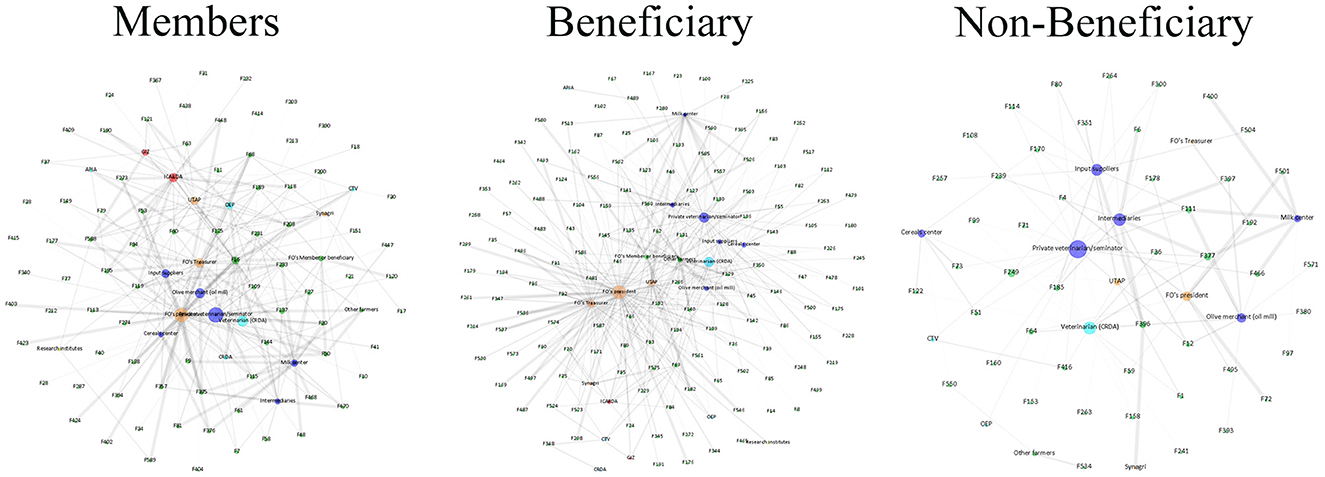
Figure 6. Social network graphic illustrations for services facilitation function (legend: green = farmers; orange = farmer organization; red = NGOs; blue = business/private; turquoise = development, administration and extension system; yellow= research and university).
In the case of eigenvector centrality, for members, the highest score was for the private veterinarian with a value of 1, followed by the FO president at 0.93 and the veterinarian at 0.78. The UTAP and Input suppliers followed with a score of 0.5. In the beneficiary group, the FO's president took the highest value with a score of 1, veterinarians followed closely with ~0.7, and UTAP scores 0.48. Non-beneficiaries had the private veterinarian leading with a value of 1, followed by intermediaries at 0.73, veterinarians (CRDA) at 0.65, and input suppliers at 0.61. Olive merchants had a score of 0.41.
5 Discussion
5.1 The transect Kef-Siliana, the diversity, and the disparity
The studied farmers in the Kef-Siliana transect represent a typical model of small-scale agriculture in north-west Tunisia, as defined by Khaldi and Saaidia (2017). This farming system in the semi-arid stage had especially low resilience to climate change, market volatility, input and resource degradation, and scarcity. It is characterized by limited plot sizes (Table 3), not exceeding 5 ha as described by Dhehibi et al. (2023), and a modest potential for intensification. Marzin et al. (2017) defined a similar system that combines livestock farming with annual crops, including arboriculture, mainly olive growing.
As a response to the challenges, many measures were taken by the agricultural system actors such as the reinforcement of system integration, income diversification, and the encouragement of collective action (Ben Nasr et al., 2021). In Tunisia, this collective action in agriculture took two forms of organizations: agricultural development groups (GDA) and mutual agricultural services company (SMSA; Dhraief et al., 2019). The determinants of farmer participation or adherence to these organizations, mentioned by Sitoe and Sitole (2019), are in line with the present study, as the only factor influencing membership or non-membership was human capital (Table 3). Specifically, educated individuals had a propensity to adhere to FOs (Etim et al., 2021). Obviously, the agricultural context in Kef-Siliana, whether in terms of production choice, production intensification, production objectives, or availability of financial resources, remains similar for members of FOs, beneficiaries only, or those not linked to FOs. This reflects that FO membership depends mostly on human capital, especially education level and household size. These results contradict the findings of Rokhani et al. (2021), who highlighted that the participation of FOs depends on farm characteristics, such as land tenure, farming choices, and other factors, rather than human capital. The comparison of social networks between the groups depends solely on whether they belong to the FOs. The farming systems are practically the same across the three farmer groups studied.
5.2 Network brokering and building
For the first intermediation function, networking and collaboration facilitation, FOs played a significant role in facilitating collaboration between farmers and other actors from the agricultural system, as presented in Table 4. These results are aligned with the findings of Beckie et al. (2012) and those of Singh et al. (2023). However, this role was often limited to members, less marked among beneficiaries, and virtually absent among non-beneficiaries. These results contradict the idea of the local influence of FOs mentioned by Nain et al. (2015), whereas they highlight the lack of equity and inclusiveness between members and beneficiaries, aligning with the findings of Bachke (2019).
More specifically, FOs facilitated collaboration between members and international organizations and between members and R&D agencies. This same idea of benefits sharing was discussed by Fischer and Qaim (2012), as they insisted that the advantages offered by the FO were appropriated by the active members and not all the FO members. In our case, as for beneficiaries, FOs facilitated farmer–farmer collaboration more than with other key actors. Unlike non-beneficiaries, who collaborated mainly with the private sector (specifically input suppliers), this could be explained from a human perspective as the farmers who were out from the FOs' network considered that their neighbors were their natural competitors, as described by Emery (2015). So, they oriented their collaboration to other different actors in the agricultural system. This individualist action issued from personal beliefs has shown that collaboration with some far-reaching actors, such as international organizations, was possible even without the intermediation of a FO, which is the case seen in the results (Table 3).
This limited networking is explained by some indigenous factors, such as the number of members or the network of the FO itself. For a highly membered FO with a large number of beneficiaries and a large local community, it will be naturally difficult to expand and facilitate collaboration for a large number of farmers. This same fact was mentioned by An et al. (2015), who explained that the effective functioning of FO relied on the aggregation size of farmers, which must not exceed a certain threshold. Furthermore, some other exogenous factors could threaten FO functioning, such as socioeconomic factors (Sparrow and Traoré, 2018). If the innovation system itself is weak, the FO will be automatically touched as it is a part of the system (Yoon et al., 2021). It is evident that FOs play a clear role in network brokering, linking farmers to other relevant actors from the agricultural system, but this intermediation function depends on several factors. This must be taken into consideration when judging the effectiveness of these structures, as its legitimacy was always challenged by local conflicts and limited impact, as cited by Yang et al. (2014).
5.3 Knowledge exchange facilitation
For innovation support with knowledge exchange facilitation, the FO was showing (Table 5) the same limitations seen for network brokering, as it facilitated access to knowledge for the members only. This fundamental function, as defined by Hellin and Camacho (2017), who considered knowledge as the main innovation support offered by a FO, was weakened by many barriers, as shown by the results when we compared different membership status and knowledge-relevant actors' configurations. Opportunistic and pragmatic thinking associated with weak trust limited the reach of the FO (Leite et al., 2021). More specifically, the results for members show that FOs were facilitating access to knowledge from research organizations (INRAT, INRGREF, IO, etc.) and from development and extension agencies (OEP, CTV, CRDA, etc.). These results are aligned with the findings of Dutrénit et al. (2012). For beneficiaries, the source of knowledge was essentially the president of the FO, who was considered a leader farmer in some extension theories, such as those described by Taylor and Bhasme (2018). For non-beneficiaries, the sources were more diversified over several actors, the majority of whom belonged to the private sector, such as intermediaries, input suppliers, product buyers, and milk collectors. Ma and Huang (2014) noted the diversity of private sector actors and their implications in knowledge exchange (Ma and Huang, 2014).
These results demonstrated another contradiction, namely that the idea behind the promotion of FOs and collective action, the decentralization of knowledge as described by Cottey (2014), created a new centralization of knowledge, in the president of the FO itself. So, the FO did not show an advantage for beneficiaries in particular, as this peer-to-peer knowledge sharing among farmers in similar developing countries did not show its efficiency (Chen et al., 2015). Controversially, to the recommendations of Kumar et al. (2015), who insisted on the role of peer-to-peer knowledge sharing as an alternative approach without giving importance to the existence of a formal organization such as FO.
5.4 Information exchange function
In terms of information exchange channels (Table 6), FOs facilitated access to information for all farmer groups, aligned with the results of Fischer and Qaim (2012). In this context, the FOs' president took the most important position reflected by centrality. This was explained by Wang et al. (2019), who insisted that information exchange is strongly linked to these organizations' leaders' motivation and wiliness. For the rest of the actors, there was no notable difference in the facilitation of information exchange between members, beneficiaries, non-beneficiaries, and those important actors such as extension and agricultural development organizations, which were described by Reed and Hickey (2016) as key actors in leadership positions, significantly controlling innovation potential, and spreading it through formal vertical common linkages. More specifically, information from most actors was exchanged in the same way for all three groups of farmers. The existence or absence of a FO did not show a clear effect. This can be explained by the rarity of the information itself, aligned with the findings of He et al. (2018), which show that information sharing is permanently reliant on the availability and accessibility of information. Otherwise, the exchange is limited when public information provision is either too low or high or private information provision is high.
5.5 Access to services
The FOs exhibited a distinctive capacity to reshape the sales channel, minimizing dependence on intermediaries. In contrast, non-beneficiary farmers were relying more heavily on the private sector.
Shortcutting the traditional sales channels by mitigating the role of intermediaries facilitated by the FO is aligned with the study of Hellin et al. (2009), which insists on the role played by collective action in fortifying farmers against the uncertainties inherent in market dynamics. The ability of FO to optimize the sales channel by minimizing intermediaries not only enhances economic viability but also reflects the resilience-reinforcement dimension within agricultural communities.
The limited reach in terms of access to services in this context can be explained by the weakness of the structures. In other words, the FOs were not able to attract the local community, as this required a set of reinforcement efforts as described by Lutz et al. (2017). This same point was evocated by Oktarina et al. (2020), who urged the need for reinforcement measures through training, advocacy, and group meetings to ensure efficient functioning. Furthermore, some other structural factors, such as the legislative text, accountability problems, and access to financing, explain this functioning jam even if the potential to have an impact exists (Bijman et al., 2016).
5.6 Assessment of FOs' innovation intermediation function: challenges and implications
It must be noted that collecting data related to social networks could possibly violate the right to privacy, as mentioned by Houghton and Joinson (2010). Furthermore, conducting focus group discussions or qualitative interviews was not possible due to logistical restrictions and socio-political realities. In addition, qualitative data were linked to the inability to generalize findings and issues related to data interpretation, as described by Tadesse et al. (2021), where the complexity of local languages and the lack of standardized procedures for capturing farmers' perspectives may complicate or impede the analysis.
The political environment in Tunisia favors FOs, but discussing it in a formal meeting, a focus group, or in a detailed interview is complex for the participants. This will potentially create the problem of distrust, possibly preventing participants from being fully open and disrupting the dynamics of focus groups or interviews, resulting in skewed and polarized feedback as described by Cavari and Freedman (2018).
6 Recommendations and conclusion
The involvement of FOs in innovation platforms and knowledge networks in Kef-Siliana has strengths but also many shortcomings. Therefore, it is crucial to complement FOs with additional actors to support agricultural innovation, especially in agroecology. In the short term, the social dimension of FOs, which is primarily human capital, needs reinforcement. Effective functioning depends on various contextual and socioeconomic factors. Effectiveness can be linked to a triangle with three sides: equity and inclusiveness, democratization and decentralization, and trust building. Addressing equity promotes inclusive collaboration, decentralization ensures equal access to knowledge, and building trust enhances FOs' reach. Investing in training, advocacy, and group meetings is essential, along with addressing legislative and financial issues. Government support should focus on guidelines over rigid regulations. Long-term, collective orientation must be reassessed, with an emphasis on entrepreneurship for small-family farming and connections to the private sector, ensuring support for non-member farmers.
Data availability statement
The raw data supporting the conclusions of this article will be made available by the authors, without undue reservation.
Ethics statement
The studies involving humans were approved by CGIAR RESEARCH ETHICS CODE Approved by the CGIAR System Board as a CGIAR Policy with effect from 3 November 2020 (Decision Reference SB/M17/EDP12). The studies were conducted in accordance with the local legislation and institutional requirements. Written informed consent for participation was not required from the participants or the participants' legal guardians/next of kin because, the consent was obtained verbally during the survey (collected on tablets).
Author contributions
HO: Conceptualization, Data curation, Formal analysis, Investigation, Methodology, Software, Visualization, Writing – original draft, Writing – review & editing. AF: Conceptualization, Funding acquisition, Project administration, Resources, Supervision, Writing – original draft, Validation, Writing – review & editing. AS: Validation, Writing – original draft. IC: Writing – original draft. ZS: Data curation, Formal analysis, Investigation, Software, Visualization, Writing – review & editing. BD: Validation, Writing – review & editing. HR: Supervision, Writing – review & editing.
Funding
The author(s) declare that financial support was received for the research, authorship, and/or publication of this article. This work was supported by the One CGIAR Initiative on Transformational Agroecology across Food, Land and Water Systems under a grant agreement (#200302) with the International Center for Agricultural Research in the Dry Areas (ICARDA—https://www.icarda.org/).
Conflict of interest
The authors declare that the research was conducted in the absence of any commercial or financial relationships that could be construed as a potential conflict of interest.
Publisher's note
All claims expressed in this article are solely those of the authors and do not necessarily represent those of their affiliated organizations, or those of the publisher, the editors and the reviewers. Any product that may be evaluated in this article, or claim that may be made by its manufacturer, is not guaranteed or endorsed by the publisher.
Author disclaimer
The opinions expressed here belong to the authors and do not necessarily reflect those of ICARDA or CGIAR.
Supplementary material
The Supplementary Material for this article can be found online at: https://www.frontiersin.org/articles/10.3389/fsufs.2024.1440086/full#supplementary-material
References
Alary, V., Frija, A., Ouerghemmi, H., Idoudi, Z., Rudiger, U., Rekik, M., et al. (2022). Context Assessment for Agroecology Transformation in the Tunisian Living Landscape. Tunis: International Center for Agricultural Research in the Dry Areas.
Alvarez, S., Timler, C. J., Michalscheck, M., Paas, W., Descheemaeker, K., Tittonell, P., et al. (2018). Capturing farm diversity with hypothesis-based typologies: an innovative methodological framework for farming system typology development. PLoS ONE 13:e0194757. doi: 10.1371/journal.pone.0194757
An, J., Cho, S., and Tang, C. S. (2015). Aggregating smallholder farmers in emerging economies. Prod. Oper. Manag. 24, 1414–1429. doi: 10.1111/poms.12372
Bachke, M. E. (2019). Do farmers' organizations enhance the welfare of smallholders? Findings from the Mozambican national agricultural survey. Food Pol. 89:101792. doi: 10.1016/j.foodpol.2019.101792
Beckie, M. A., Kennedy, E. H., and Wittman, H. (2012). Scaling up alternative food networks: farmers' markets and the role of clustering in western Canada. Agric. Hum. Val. 29, 333–345. doi: 10.1007/s10460-012-9359-9
Belton, B., Reardon, T., and Zilberman, D. (2020). Sustainable commoditization of seafood. Nat. Sustain. 3, 677–684. doi: 10.1038/s41893-020-0540-7
Ben Mustapha, A., Faysse, N., Marlet, S., and Jamin, J.-Y. (2015). Une action collective analysée par ses acteurs : une association d'irrigants en Tunisie. Nat. Sci. Soc. 23, 356–366. doi: 10.1051/nss/2015055
Ben Nasr, J., Chaar, H., Bouchiba, F., and Zaibet, L. (2021). Assessing and building climate change resilience of farming systems in Tunisian semi-arid areas. Environ. Sci. Pollut. Res. 28, 46797–46808. doi: 10.1007/s11356-021-13089-0
Bijman, J., Muradian, R., and Schuurman, J. (2016). Cooperatives, Economic Democratization and Rural Development. Cheltenham: Edward Elgar Publishing.
Bizikova, L., Nkonya, E., Minah, M., Hanisch, M., Turaga, R. M. R., Speranza, C. I., et al. (2020). A scoping review of the contributions of farmers' organizations to smallholder agriculture. Nat. Food 1, 620–630. doi: 10.1038/s43016-020-00164-x
Canesse, A.-A. (2011). Les groupements de développement agricole (GDA): entrepreneurs locaux ou relais administratifs. Entrepreneurs maghrébins, terrains en développement. Tunis: Karthala et Institut de recherche sur le Maghreb contemporain, 351–364.
Cavari, A., and Freedman, G. (2018). Polarized mass or polarized few? Assessing the parallel rise of survey nonresponse and measures of polarization. J. Polit. 80, 719–725. doi: 10.1086/695853
Chandrakar, K., Chandrakar, D. K., and Das, D. (2023). Farmers Producer Organization (FPO): how it is changing farming scenario. Int. J. Sci. Res. Archiv. 8, 761–765. doi: 10.30574/ijsra.2023.8.1.0082
Chen, Y., Shanthikumar, J. G., and Shen, Z. M. (2015). Incentive for peer-to-peer knowledge sharing among farmers in developing economies. Prod. Oper. Manag. 24, 1430–1440. doi: 10.1111/poms.12328
Cofré-Bravo, G., Klerkx, L., and Engler, A. (2019). Combinations of bonding, bridging, and linking social capital for farm innovation: how farmers configure different support networks. J. Rural Stud. 69, 53–64. doi: 10.1016/j.jrurstud.2019.04.004
Cottey, A. (2014). Knowledge production in a cooperative economy. Pol. Fut. Educ. 12, 469–481. doi: 10.2304/pfie.2014.12.4.4469
Devi, K., and Tripathi, R. (2020). “Social network analysis for efficient delivery of agricultural extension services,” in 2020 11th International Conference on Computing, Communication and Networking Technologies (ICCCNT) (Kharagpur), 1–6.
Dhehibi, B., Fouzai, A., Frija, A., Adhim, M. A., M'hamed, H. C., Ouerghemmi, H., et al. (2023). Assessing complementary synergies for integrated crop-livestock systems under conservation agriculture in Tunisian dryland farming systems. Front. Sustain. Food Syst. 6:1022213. doi: 10.3389/fsufs.2022.1022213
Dhraief, M. Z., Bedhiaf, S., Dhehibi, B., Oueslati-Zlaoui, M., Jebali, O., and Ben-Youssef, S. (2019). Factors affecting innovative technologies adoption by livestock holders in arid area of Tunisia. New Medit. 2019:nm1904a. doi: 10.30682/nm1904a
Dicecca, R., Pascucci, S., and Contò, F. (2016). Understanding reconfiguration pathways of agri-food value chains for smallholder farmers. Br. Food J. 118, 1857–1882. doi: 10.1108/BFJ-05-2016-0194
Dicks, L. V., Rose, D. C., Ang, F., Aston, S., Birch, A. N. E., Boatman, N., et al. (2019). What agricultural practices are most likely to deliver “sustainable intensification” in the UK? Food Energy Secur. 8:e00148. doi: 10.1002/fes3.148
Dolinska, A. (2017). Bringing farmers into the game. Strengthening farmers' role in the innovation process through a simulation game, a case from Tunisia. Agric. Syst. 157, 129–139. doi: 10.1016/j.agsy.2017.07.002
Dutrénit, G., Rocha-Lackiz, A., and Vera-Cruz, A. O. (2012). Functions of the intermediary organizations for agricultural innovation in Mexico: the Chiapas Produce Foundation. Rev. Pol. Res. 29, 693–712. doi: 10.1111/j.1541-1338.2012.00589.x
El Amrani, M., and Beni Houd, Y. (2022). Social network analysis : a useful tool for studying innovation diffusion processes. Economia agro-alimentare 2022:12059. doi: 10.3280/ecag2022oa12059
El Ansari, L., Chenoune, R. A, Yigezu, Y., Gary, C., and Belhouchette, H. (2020). Trade-offs between sustainability indicators in response to the production choices of different farm household types in drylands. Agronomy 10:70998. doi: 10.3390/agronomy10070998
Elloumi, M. (2006). “Les politiques de développement rural en Tunisie : acquis et perspectives,” in Politiques de développement rural durable en Méditerranée dans le cadre de la politique de voisinage de l\'Union Européenne, eds. C. J. P. and P. J. P. (Montpellier: CIHEAM), 55–65. Available at: http://om.ciheam.org/om/pdf/a71/06400057.pdf (accessed March 12, 2024).
Emery, S. B. (2015). Independence and individualism: conflated values in farmer cooperation? Agric. Hum. Val. 32, 47–61. doi: 10.1007/s10460-014-9520-8
Etim, N.-A. A., Udolnyang, S. P., Ebukiba, E. S., and Udoh, E. J. (2021). Households' decision to participate in cooperative organizations: evidence from farmers in Akwa Ibom State, Southern Nigeria. J. La Sociale 2, 28–36. doi: 10.37899/journal-la-sociale.v2i4.466
Faure, G., Desjeux, Y., and Gasselin, P. (2012). New challenges in agricultural advisory services from a research perspective: a literature review, synthesis and research agenda. J. Agric. Educ. Extens. 18, 461–492. doi: 10.1080/1389224X.2012.707063
Fischer, E., and Qaim, M. (2012). Linking smallholders to markets: determinants and impacts of farmer collective action in Kenya. World Dev. 40, 1255–1268. doi: 10.1016/j.worlddev.2011.11.018
Garnett, T., Appleby, M. C., Balmford, A., Bateman, I. J., Benton, T. G., Bloomer, P., et al. (2013). Sustainable intensification in agriculture: premises and policies. Science 341, 33–34. doi: 10.1126/science.1234485
Godfray, H. C. J., and Garnett, T. (2014). Food security and sustainable intensification. Philos. Trans. Royal Soc. B 369:20120273. doi: 10.1098/rstb.2012.0273
Gouët, C., and Van Paassen, A. (2012). Smallholder marketing cooperatives and smallholders' market access: lessons learned from the actors involved. J. Agric. Educ. Extens. 18, 369–385. doi: 10.1080/1389224X.2012.691784
Gutiérrez Cano, L. F., Zartha Sossa, J. W., Orozco Mendoza, G. L., Suárez Guzmán, L. M., Agudelo Tapasco, D. A., and Quintero Saavedra, J. I. (2023). Agricultural innovation system: analysis from the subsystems of R&D, training, extension, and sustainability. Front. Sustain. Food Syst. 7:1176366. doi: 10.3389/fsufs.2023.1176366
Hansen, M. H., Hurwitz, W. N., and Madow, W. G. (1953). Sample Survey Methods and Theory. New York, NY: John Wiley & Sons.
He, Q., Chen, Y., and Shen, Z. (2018). On the formation of producers' information-sharing coalitions. Prod. Oper. Manag. 27, 917–927. doi: 10.1111/poms.12852
Hellin, J., and Camacho, C. (2017). Agricultural research organisations' role in the emergence of agricultural innovation systems. Dev. Pract. 27, 111–115. doi: 10.1080/09614524.2017.1256373
Hellin, J., Lundy, M., and Meijer, M. (2009). Farmer organization, collective action and market access in Meso-America. Food Policy 34, 16–22. doi: 10.1016/j.foodpol.2008.10.003
Hermans, F., Stuiver, M., Beers, P. J., and Kok, K. (2013). The distribution of roles and functions for upscaling and outscaling innovations in agricultural innovation systems. Agric. Syst. 115, 117–128. doi: 10.1016/j.agsy.2012.09.006
Houghton, D. J., and Joinson, A. N. (2010). Privacy, social network sites, and social relations. J. Technol. Hum. Serv. 28, 74–94. doi: 10.1080/15228831003770775
Howells, J. (2006). Intermediation and the role of intermediaries in innovation. Res. Pol. 35, 715–728. doi: 10.1016/j.respol.2006.03.005
Iyabano, A., Klerkx, L., Faure, G., and Toillier, A. (2022). Farmers' Organizations as innovation intermediaries for agroecological innovations in Burkina Faso. Int. J. Agric. Sustain. 20, 857–873. doi: 10.1080/14735903.2021.2002089
Jorgenson, D. W. (2011). Innovation and productivity growth: T. W. Schultz Lecture. Am. J. Agric. Econ. 93, 276–296. doi: 10.1093/ajae/aaq191
Khaldi, R., and Saaidia, B. (2017). Analyse de la Filière Céréalière en Tunisie et Identification des Principaux Points de Dysfonctionnement à L'origine des Pertes. Tunis: ONAGRI.
Kilelu, C. W., Klerkx, L., Leeuwis, C., and Hall, A. (2011). Beyond knowledge brokering: an exploratory study on innovation intermediaries in an evolving smallholder agricultural system in Kenya. Knowl. Manag. Dev. J. 7, 84–108. doi: 10.1080/19474199.2011.593859
Kim, J. K., and Wang, Z. (2019). Sampling techniques for big data analysis. Int. Stat. Rev. 87, S177–S191. doi: 10.1111/insr.12290
Klerkx, L., Aarts, N., and Leeuwis, C. (2010). Adaptive management in agricultural innovation systems: the interactions between innovation networks and their environment. Agric. Syst. 103, 390–400. doi: 10.1016/j.agsy.2010.03.012
Kolleck, N. (2013). Social network analysis in innovation research: using a mixed methods approach to analyze social innovations. Eur. J. Fut. Res. 1:25. doi: 10.1007/s40309-013-0025-2
Krush, M. T., Pennington, J. R., Fowler, A. R., and Mittelstaedt, J. D. (2015). Positive marketing: a new theoretical prototype of sharing in an online community. J. Bus. Res. 68, 2503–2512. doi: 10.1016/j.jbusres.2015.06.037
Kumar, K. R., Nain, M. S., Singh, R., Chahal, V. P., and Bana, R. S. (2015). Analysis of farmers' communication network and factors of knowledge regarding agro meteorological parameters. Ind. J. Agric. Sci. 85, 1592–1596. doi: 10.56093/ijas.v85i12.54314
Kumari, N., Malik, J. S., Arun, D. P., and Nain, M. S. (2022). Farmer Producer Organizations (FPOs) for linking farmer to market. J. Extens. Syst. 37, 1–6.
Leite, A. R., Padilha, A. C. M., and Binotto, E. (2021). Cooperation challenges in agricultural cooperatives. Revista de Administração da UFSM 14, 809–826. doi: 10.5902/1983465964160
Levy, P. S., and Lemeshow, S. (2008). Stratification and stratified random sampling. Sampl. Popul. 5, 121–142. doi: 10.1002/9780470374597.ch5
Lutz, J., Smetschka, B., and Grima, N. (2017). Farmer cooperation as a means for creating local food systems-potentials and challenges. Sustainability 9:60925. doi: 10.3390/su9060925
Ma, Y.-N., and Huang, S. (2014). The collaboration pattern, trust relationship, and transferring and learning willingness: evidence of China's agribusiness and farmers. Int. J. Serv. Technol. Manag. 20, 31–46. doi: 10.1504/IJSTM.2014.063587
Marzin, J., Bonnet, P., Bessaoud, O., and Ton-Nu, C. (2017). Study on Small-Scale Family Farming in the Near-East and North Africa Region: Synthesis. Cairo: FAO.
McKight, P. E., and Najab, J. (2010). Kruskal-Wallis test. Corsini Encycl. Psychol. 1:491. doi: 10.1002/9780470479216.corpsy0491
Meyer, M., and Kearnes, M. (2013). Introduction to special section: intermediaries between science, policy and the market. Sci. Publ. Pol. 40, 423–429. doi: 10.1093/scipol/sct051
Mhadhbi, F. (2021). L'impact de l'intensification agricole sur le bien-être territorial dans un pays du sud: analyse empirique dans la situation tunisienne. Avignon Université, Avignon.
Msuta, P. B., and Urassa, J. K. (2015). The contribution of farmers organizations to smallholder farmers well-being: a case study of Kasulu district, Tanzania. Afr. J. Agric. Res. 10, 2343–2349. doi: 10.5897/AJAR2014.9261
Nain, M. S., Singh, R., Mishra, J. R., and Sharma, J. P. (2015). Utilization and linkage with agricultural information sources: a study of Palwal district of Haryana state. J. Commun. Mobil. Sustain. Dev. 10, 152–156.
North, D. C. (1995). “The new institutional economics and third world development,” in The New Institutional Economics and Third World Development, eds J. Harriss, J. Hunter, and C. Lewis (London: Routledge), 31–40.
Ogundari, K., and Bolarinwa, O. D. (2018). Impact of agricultural innovation adoption: a meta-analysis. Austr. J. Agric. Resour. Econ. 62, 217–236. doi: 10.1111/1467-8489.12247
Oktarina, S., Zulfiningrum, R., Zainal, A. G., and Wahyono, E. (2020). The role of communication and farmer institutional urgency to the agriculture development program. Multicult. Multireligious Understand. 7, 266–276.
Parthiban Sakhti., R, Nain, M. S., Singh, R., Kumar, S., and Chahal, V. P. (2015). Farmers' producer organisation in reducing transactional costs: a study of Tamil Nadu Mango Growers Federation (TAMAFED). Ind. J. Agric. Sci. 85, 1303–1307. doi: 10.56093/ijas.v85i10.52269
Pigford, A.-A. E., Hickey, G. M., and Klerkx, L. (2018). Beyond agricultural innovation systems? Exploring an agricultural innovation ecosystems approach for niche design and development in sustainability transitions. Agric. Syst. 164, 116–121. doi: 10.1016/j.agsy.2018.04.007
Pretty, J., and Bharucha, Z. P. (2014). Sustainable intensification in agricultural systems. Ann. Bot. 114, 1571–1596. doi: 10.1093/aob/mcu205
Redhead, D., McElreath, R., and Ross, C. T. (2023). Reliable network inference from unreliable data: a tutorial on latent network modeling using STRAND. Psychol. Methods 2023:519. doi: 10.1037/met0000519
Reed, G., and Hickey, G. M. (2016). Contrasting innovation networks in smallholder agricultural producer cooperatives: insights from the Niayes Region of Senegal. J. Co-operat. Org. Manag. 4, 97–107. doi: 10.1016/j.jcom.2016.09.001
Robinson, L. W., Ericksen, P. J., Chesterman, S., and Worden, J. S. (2015). Sustainable intensification in drylands: what resilience and vulnerability can tell us. Agric. Syst. 135, 133–140. doi: 10.1016/j.agsy.2015.01.005
Rokhani, R., Fauziyah, D., Supriono, A., Hariyati, Y., Raharto, S., Hapsari, T. D., et al. (2021). Factors affecting the participation of sugarcane and Tobacco Farmers in Farmer Groups, Associations and Cooperatives in Indonesia. J. Sustain. Agric. 36, 340–354. doi: 10.20961/carakatani.v36i2.46817
Schut, M., Andersson, J. A., Dror, I., Kamanda, J., Sartas, M., Mur, R., et al. (2017). Guidelines for Innovation Platforms in Agricultural Research for Development: Decision Support for Research, Development and Funding Agencies on How to Design, Budget and Implement Impactful Innovation Platforms, 88. Ibada: IITA and WUR.
Schut, M., van Asten, P., Okafor, C., Hicintuka, C., Mapatano, S., Nabahungu, N. L., et al. (2016). Sustainable intensification of agricultural systems in the Central African Highlands: the need for institutional innovation. Agric. Syst. 145, 165–176. doi: 10.1016/j.agsy.2016.03.005
Shrivastava, S., Jat, M., and Shrivastava, K. (2022). Present status, role and challenges of Farmer Producer Organization. Asian J. Agric. Extens. Econ. Sociol. 40, 39–47. doi: 10.9734/ajaees/2022/v40i830935
Singh, A., Singh, R., Nain, M. S., Mishra, J. R., Kumar, P., Sharma, D. K., et al. (2023). Linkage network structures of farmers: analysing FPOs of M.P. and Bihar in India. Ind. J. Extens. Educ. 59, 14–20. doi: 10.48165/IJEE.2023.59303
Sitoe, T. A., and Sitole, A. (2019). Determinants of farmer's participation in farmers' associations: empirical evidence from Maputo Green Belts, Mozambique. Asian J. Agric. Extens. Econ. Sociol. 37, 1–12. doi: 10.9734/ajaees/2019/v37i130259
Sosa, S. (2022). “Social network analysis,” in Encyclopedia of Animal Cognition and Behavior, eds. J. Vonk and T. K. Shackelford (Cham: Springer International Publishing), 6527–6544. doi: 10.1007/978-3-319-55065-7_1882
Sparrow, A. D., and Traoré, A. (2018). Limits to the applicability of the innovation platform approach for agricultural development in West Africa: socio-economic factors constrain stakeholder engagement and confidence. Agric. Syst. 165, 335–343. doi: 10.1016/j.agsy.2017.05.014
Spielman, D. J. (2006). A critique of innovation systems perspectives on agricultural research in developing countries. Innov. Strategy Tod. 2, 41–54.
Spielman, D. J. (2007). Pro-poor agricultural biotechnology: can the international research system deliver the goods? Food Pol. 32, 189–204. doi: 10.1016/j.foodpol.2006.05.002
Sterelny, K. (2020). Innovation, life history and social networks in human evolution. Philos. Trans. Royal Soc. B 375:20190497. doi: 10.1098/rstb.2019.0497
Tadesse, B., Tilahun, Y., Bekele, T., and Mekonen, G. (2021). Assessment of challenges of crop production and marketing in Bench-Sheko, Kaffa, Sheka, and West-Omo zones of southwest Ethiopia. Heliyon 7:e07319. doi: 10.1016/j.heliyon.2021.e07319
Taylor, M., and Bhasme, S. (2018). Model farmers, extension networks and the politics of agricultural knowledge transfer. J. Rural Stud. 64, 1–10. doi: 10.1016/j.jrurstud.2018.09.015
Thomson, A. M., Ellis, E. C., Grau Hé, R., Kuemmerle, T., Meyfroidt, P., Ramankutty, N., et al. (2019). Sustainable intensification in land systems : trade-offs, scales, and contexts. Curr. Opin. Environ. Sustain. 38, 37–43. doi: 10.1016/j.cosust.2019.04.011
Turner, J. A., Klerkx, L., Rijswijk, K., Williams, T., and Barnard, T. (2016). Systemic problems affecting co-innovation in the New Zealand Agricultural Innovation System: identification of blocking mechanisms and underlying institutional logics. Wageningen J. Life Sci. 76, 99–112. doi: 10.1016/j.njas.2015.12.001
Wang, Y., Jin, L., and Mao, H. (2019). Farmer cooperatives' intention to adopt agricultural information technology-mediating effects of attitude. Inform. Syst. Front. 21, 565–580. doi: 10.1007/s10796-019-09909-x
Yang, H., Klerkx, L., and Leeuwis, C. (2014). Functions and limitations of farmer cooperatives as innovation intermediaries: findings from China. Agric. Syst. 127, 115–125. doi: 10.1016/j.agsy.2014.02.005
Keywords: agricultural innovation, farmer organization, innovation intermediation, smallholders, social network analysis
Citation: Ouerghemmi H, Frija A, Souissi A, Carpentier I, Shiri Z, Dhehibi B and Rejeb H (2024) Are farmer organizations effective intermediaries and facilitators of agricultural innovations processes? Evidence from Tunisia. Front. Sustain. Food Syst. 8:1440086. doi: 10.3389/fsufs.2024.1440086
Received: 28 May 2024; Accepted: 12 August 2024;
Published: 13 September 2024.
Edited by:
Manjeet Singh Nain, Indian Agricultural Research Institute (ICAR), IndiaReviewed by:
Naomi Di Santo, University of Foggia, ItalyErika Valerio, University of New England, Australia
Copyright © 2024 Ouerghemmi, Frija, Souissi, Carpentier, Shiri, Dhehibi and Rejeb. This is an open-access article distributed under the terms of the Creative Commons Attribution License (CC BY). The use, distribution or reproduction in other forums is permitted, provided the original author(s) and the copyright owner(s) are credited and that the original publication in this journal is cited, in accordance with accepted academic practice. No use, distribution or reproduction is permitted which does not comply with these terms.
*Correspondence: Hassen Ouerghemmi, aC5vdWVyZ2hlbW1pQGNnaWFyLm9yZw==