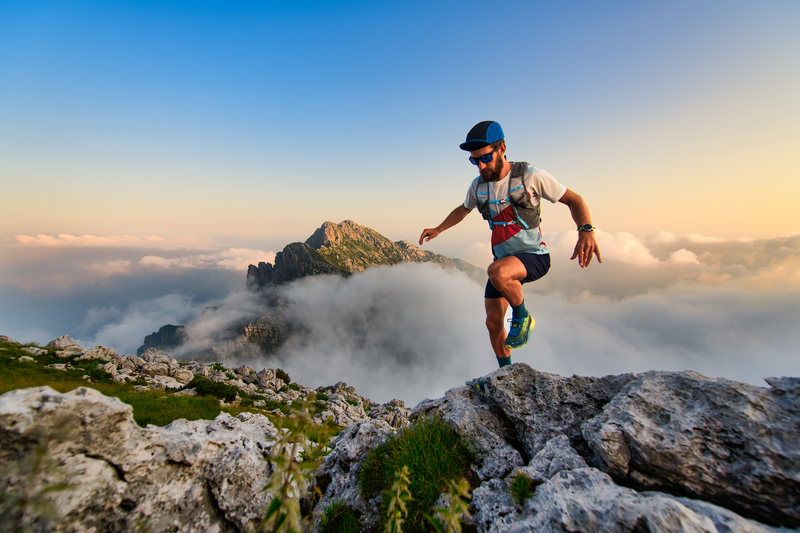
95% of researchers rate our articles as excellent or good
Learn more about the work of our research integrity team to safeguard the quality of each article we publish.
Find out more
ORIGINAL RESEARCH article
Front. Sustain. Food Syst. , 06 August 2024
Sec. Agricultural and Food Economics
Volume 8 - 2024 | https://doi.org/10.3389/fsufs.2024.1434604
Introduction: The rapid aging of the labor force has serious implications for socioeconomic development and poses challenges to food sustainability. Existing studies have focused on the impact of labor force aging on land productivity, but little attention has been paid to the comprehensive capacity of agricultural production, namely the total factor productivity of agriculture.
Methods: We estimate the impact of labor force aging on total factor productivity in agriculture using data from 170,506 sample farm households from the National Fixed Point Survey data from 2003 to 2020. We estimate the results using a panel fixed effects approach and mitigate the endogeneity problem using an instrumental variables approach to ensure the robustness of the estimates.
Results and discussion: The results show that labor force aging has an adverse effect on the agricultural total factor productivity of farm households and remains robust after addressing endogeneity and substituting explanatory variables. The aging effect shows significant heterogeneity across different food functional areas with different types of farm households. The negative impact of labor force aging on total factor productivity in agriculture is mainly concentrated in the main grain-production and grain-producing and marketing-balanced areas. We also find that the negative impact of labor force aging is more significant among large-scale farmers. The mechanism analysis finds that the aging of the labor force adversely affects the agricultural total factor productivity by inhibiting technological progress and reducing the efficiency of agricultural resource allocation. These insights, when considered in the light of global trends towards agricultural labor aging, suggest that policy interventions aimed at promoting technological adoption, enhancing resource allocation efficiency, and supporting the transitioning of older farmers may hold promise for maintaining food sustainability and addressing the challenges posed by an aging agricultural workforce in numerous developing nations.
Population aging has significant impacts on socioeconomic development, and population ageing in the agricultural sector also threatens sustainable agricultural development. The aging of the agricultural population is a phenomenon that occurs not only in developed countries but is also common in developing countries. This issue is particularly relevant for developing nations where agricultural labor forms a substantial part of the workforce. Many agricultural laborers have turned to non-agricultural employment amidst the accelerated urbanization and industrialization in China. The number of agricultural laborers has declined from its peak of 390.98 million in 1991 to 177.15 million in 2020. Similarly, the proportion of primary industry workers has decreased from a peak of 60.1 to 23.6%. Concurrently, the aging of agricultural producers and operators is progressing rapidly, attributed to the escalating rural life expectancy and declining birth rates. In 1997, only 9.86% of Chinese farm households comprised individuals over the working age of 60 for men and 55 for women (National Bureau of Statistics, 1997). However, by the end of 2016, the proportion of agricultural workers aged 55 and above had risen to a substantial 33.6% (National Bureau of Statistics, 2016).
Ensuring food security has always been a crucial strategic issue, not only for China but for many countries facing similar demographic and economic transitions with tight human-land resource constraints. To guarantee food security, it is not only necessary to ensure sufficient cultivated land area and increase food production but also to focus on enhancing the comprehensive agricultural production capacity, i.e., improving agricultural total factor productivity (TFP) (Wang et al., 2019; Sheng et al., 2020). Improving comprehensive agricultural production capacity implies a stable and efficient food supply. Therefore, enhancing agricultural TFP is significant for achieving a sustainable food supply. Sustainable food supply is a crucial issue related to human health, social stability, and global sustainable development, affecting the well-being of individuals and society. The aging of the agricultural labor force may affect the cultivated land area and influence the comprehensive agricultural production capacity, which is related to the sustainable development of agriculture (Zou et al., 2018; Zhang et al., 2023). Thus, exploring the impact of labor force aging on agricultural TFP in the context of China can provide valuable insights for other developing countries facing similar challenges, revealing whether labor force aging will threaten the sustainable development of food production globally.
Indeed, Existing literature has conducted a series of studies examining the impact of labor force aging on various aspects of agricultural production within the framework of its influence on agricultural sustainability. The existing research primarily focuses on the following aspects: Firstly, it analyzes the impact of labor force aging on agricultural production decisions, including its influence on agricultural production inputs. There are differences in agricultural production motivations between older and younger farmers. Compared to older farmers, younger farmers have stronger economic motivations in agricultural production, leading them to invest more funds (Van et al., 2007; Guo et al., 2022). Due to physical and mental limitations, older farmers may lack the incentive to expand production, thus reducing investments in fertilizers, pesticides, and machinery during the production process (Potter and Lobley, 1992). Secondly, it examines the impact of labor force aging on land use. As farmers age and lack successors, they tend to lease their land to other farmers (Zou et al., 2018). However, this may also lead to land being idled or abandoned, especially for plots in remote areas far from main roads or with steep slopes (Lee et al., 2021). Thirdly, scholars have studied the impact of an aging labor force on production efficiency, including land use and technical efficiency (Rigg et al., 2020). With an increasing proportion of older farmers, the technical efficiency level of household agricultural production may decline. There are various reasons for this, such as older farmers lacking financial support to expand their scale of operations and thus not purchasing more agricultural machinery equipment. Additionally, older farmers may be risk-averse and have a negative attitude toward adopting new technologies, making them unable to adapt to new technological developments (Li and Sicular, 2013). These studies provide valuable support for our understanding, but there is a scarcity of research focusing on the impact of agricultural labor force aging on agricultural TFP. This gap prevents us from revealing the influence of labor force aging on the comprehensive agricultural production capacity and its impact on sustainable food production.
Chinese agriculture is currently transitioning and upgrading, making it crucial to enhance the comprehensive agricultural production capacity for achieving sustainable food production. This issue is not unique to China; many developing countries face similar challenges in the context of agricultural transition and labor aging. Against the backdrop of increasing labor aging, exploring the household-level labor force’s impact on farmers’ agricultural TFP can provide a deeper understanding of how agricultural labor aging affects the comprehensive agricultural production capacity. Based on this, the overall objective of this study is to investigate the impact of labor aging on the agricultural TFP of Chinese farmers. This paper processed household-level input–output data to measure the agricultural TFP of Chinese farmers accurately, using the fixed observation points of China’s Ministry of Agriculture and Rural Development (MAFRD) from 2003 to 2020. The selection of crop farming for measurement is grounded in two aspects. Firstly, it aligns closely with the research objective, which aims to delve into the influence of labor aging on the comprehensive production capacity of grain production within the broader context of sustainable food production. Secondly, the focus on crop farming ensures comparability and relevance, given the similar structural disparities in input–output between livestock and crop farming in countries facing tight human-land resource constraints. On this premise, we investigate the impact of agricultural labor aging on agricultural TFP. When exploring how labor aging affects the agricultural TFP of farmers, this paper incorporates the efficiency of agricultural resource allocation into the analytical framework, expanding the mechanism explanation for this issue.
The marginal contributions of this study are as follows. Firstly, in terms of research perspective, existing literature has discussed the impact of labor aging on agricultural TFP from a macro level or focused on a single crop. This approach has two drawbacks: First, there are differences between macro-level agricultural TFP growth and micro-level agricultural TFP growth mechanisms among farmers. Second, the agricultural TFP of a single crop at the micro-level farmer may only partially reflect the comprehensive production capacity of farmers and is more susceptible to interference from other factors. Secondly, most existing literature considers rural population aging, encompassing overall or rural labor aging. However, there needs to be more focus on the aging of agricultural labor engaged in household farming. Thirdly, in exploring how labor aging affects the agricultural TFP of farmers, the mechanism of agricultural resource allocation efficiency has been ignored. Labor aging can lead to path dependency and increased information asymmetry among farmers, thus affecting resource allocation efficiency. Improving the efficiency of agricultural resource allocation is an essential pathway to enhancing agricultural TFP. This mechanism is relevant for policymakers and researchers in various developing countries, as efficient resource allocation remains a common challenge.
The structural arrangement of this chapter is as follows: Section 2 proposes the analytical framework of this paper based on the descriptive analysis of the characteristics of labor aging and agricultural TFP of farmers combined with the existing literature. Section 3 introduces the data sources, research methods, and variable selection. Section 4 presents the empirical analysis results of this paper. Section 5 is a mechanistic study of how the labor force aging affects total factor productivity in agriculture. Section 6 is the research conclusion and policy recommendations.
The aging of the agricultural labor force has deepened. The rural population has shown a rapid aging trend over the past two decades, which has led to a rapid increase in the age of agricultural operators. Several reasons aggravate the aging of the agricultural labor force. In recent years, the decline in rural fertility rates has led to a decreasing number of young laborers. However, the main reason is the acceleration of economic development and urbanization, the increase in the number of peasants working outside the home, and the transfer of many young laborers to the industrial sector. In 2000, China’s elderly population aged 60 reached 130 million, or 10.3%, but by 2021, it reached 270 million, or 18.9% of the total population.1 Figure 1 reflects the trend in the average age of the agricultural labor force in the sample from 2003 to 2020, with the average age of the agricultural labor force showing an upward trend from 47.25 in 2003 to 57.34 in 2020 and the trend in the age of the sampled farm households is consistent with the national aging trend. From the perspective of grain-growing regions, the average age of the agricultural labor force in the main grain-producing areas is the largest, higher than the average level in China, and the average age in non-main grain-producing areas is lower than the national level. The main grain-producing areas are primarily areas with traditional agriculture and low economic levels, where some young laborers flow to developed areas or industrial sectors, and grain production relies mainly on older laborers, leading to relatively more severe aging of the labor force.
Based on the analytical framework of resource allocation efficiency (Adamopoulos et al., 2022), this paper argues that labor force aging will affect agricultural TFP of farm households from two main channels: first, it affects agricultural TFP directly by influencing technological progress. The second is indirectly affecting agricultural TFP by affecting agricultural resource allocation efficiency.
First, the aging labor force affects agricultural TFP by influencing technological progress in two main ways: One, changes in human capital triggered by the aging of the labor force and a decline in the physical strength and fitness of workers can lead to changes in the supply of effective labor in agriculture (Van et al., 2007). Older farmers will invest more labor to compensate for the adverse effects of their declining physical capacity. Due to their agricultural experience, older people can offset the adverse effects of the decline in physical fitness in experience-dependent agricultural production segments (Skirbekk, 2004). However, in agricultural production, the demands on human capital gradually increase. Older farmers are at a disadvantage in terms of accepting new information and learning new knowledge, particularly when it comes to leveraging smartphones and computers for accessing advanced farming techniques and market insights, especially among farmers residing in mountainous or remote areas. They may not be able to adapt to the new period of agricultural production, thus inhibiting the adoption of new technologies and, in general, adversely affecting the efficiency of agricultural production (Li and Sicular, 2013). Second, an aging labor force can affect farmers’ productive investment in agriculture. Much of the increase in agricultural TFP comes from agricultural productive investment (Adamopoulos and Restuccia, 2014). In general, productive investment in agriculture consists of the capital invested by farmers to enhance their productive capacity, including the cost of fertilizers, seedlings, agricultural diesel, pesticides, and the use of mechanical services in agricultural production, and it can measure the adoption of general-purpose technologies for agricultural production (De Brauw and Rozelle, 2008). The development of agricultural machinery and agricultural machinery services can effectively replace the agricultural labor force and, therefore, alleviate the labor supply shortage problem (Tang and MacLeod, 2006). According to the theory of induced technological change, older farmers will increase the relative abundance of cheaper factor inputs to compensate for the adverse effects of declining physical capacity. It is inferred that older farmers will compensate for labor shortages by investing more in fertilizers and pesticides, resulting in a factor substitution effect. However, this substitution relationship is not inevitable; the aging of the labor force may instead reduce the capital input of older farmers in agricultural production, which arises from the rise of agricultural production materials. In recent years, with the rapid rise in agricultural capital and the cost of mechanical services, agricultural production materials are no longer advantageous compared to labor. Farmers consider alternative factor costs, considering the potential value of productive inputs. Older farmers are more likely to reduce productive investment in favor of “conservative” forms of production due to financial constraints, thus discouraging technological progress and having a negative effect on agricultural TFP.
Second, the aging labor force affects agricultural TFP through agricultural resource allocation efficiency. Agricultural resource allocation efficiency optimization is characterized by farmers with high agricultural TFP receiving a larger share of output and more access to factor inputs (Gai et al., 2020). The means to optimize resource allocation efficiency is to allow farmers who are able and willing to farm to hold more factor resources. Labor force aging affects agricultural resource allocation in two main ways. First, the aging of the labor force implies a shortage of young adults in household agricultural production, and the increasing age of agricultural operators can lead to path dependence on agricultural production. In other words, older farmers will rely more on their experience to engage in agricultural production and farming experience to a certain extent. Farming experience can compensate to some extent for reduced physical strength. However, it will also make them seldom adjust their factor allocation, resulting in a mismatch between the level of productivity and the share of production. These factor misallocations can manifest in two ways: First, as farmers get older, they are more influenced by traditional farming ideas. They are more likely to have a land-dependence complex. So, no matter what level of productivity, they are cautious about the loss of land, not willing to abandon the land or transfer it to others; even if they can not afford to cultivate, they will keep a part of the planting to meet their own ration needs, at this time the efficiency of agricultural production is not the primary goal; Secondly, even if part of the farmers’ productivity is at a high level, they are not willing to plant more land, access to more factors of production. As farmers age, they expect their operating capacity to decline, and for risk aversion purposes, they will not expand their scale of operation. Second, the adverse impact of labor aging on agricultural resource allocation is also reflected in asymmetric information and transaction costs. Due to the higher cost of market information access for elderly farmers, it is necessary to pay higher transaction costs to realize the free and fast flow of factors. For example, older people face more constraints in the credit market and must pay higher costs to obtain more credit to invest in agriculture. Such high transaction costs due to credit constraints can adversely affect agricultural resource allocation and productivity (Guirkinger and Boucher, 2008). Thus, an aging labor force can negatively affect agricultural TFP by inhibiting the efficient allocation of resources.
The data in this paper comes from the National Fixed Point Survey (NFP), a micro-farm household survey collected by the Research Center of Rural Economy (RCRE) of the Chinese Ministry of Agriculture beginning in 1986. The survey employed a stratified sampling method, selecting an equal number of rich, medium, and poor counties from each province based on economic levels. Subsequently, villages were chosen within these counties using the same criteria. Overall, the sampled survey data exhibit representativeness at the national level. The survey samples approximately 20,000 farm households annually, making it China’s largest farm-level tracking research data. The surveyed farmers use a book-keeping method to collect data, and the surveyed farmers are familiar with the data that need to be recorded, so the data are of high quality (Benjamin et al., 2005). The NFP data contain detailed information on household agricultural production, household employment, income, and consumption, including detailed records of agricultural inputs and outputs, thus aiding the research in this paper. NFP data includes more than 21,000 households in about 360 administrative villages in 31 provinces (municipalities) in China annually. In this paper, to study the impact of labor force aging on agricultural TFP of farm households, the variables need to use the age of family members, the information of family members only began to supplement the survey in 2003, and the structure of the survey changed substantially in 2003. Therefore, we use the data from 2003 to 2020 for data comparability to study the impact of labor force aging on agricultural TFP. This paper focuses on plantation production, including five food crops and five cash crops. Therefore, only farmers with numerical values of plantation inputs and outputs were retained in the sample processing. After processing outliers and missing values, the study ended up with 170,506 samples.
From the existing studies, accounting for agricultural TFP at the household level usually uses the classical C-D production function (Chari et al., 2021; Adamopoulos et al., 2022). In this paper, we focus on agricultural TFP at the household level, which is also applicable to the C-D production function form in the short-run average sense, as farmers are weak and relatively slow to adjust to input factors such as land and capital (Lin, 1992; Fan and Pardey, 1997; Cao and Birchenall, 2013). The input variables of the accounting equation contain labor input, capital input, and land input. Labor input is measured using the total amount of labor invested in agricultural production, which is more accurate than the number of laborers used or the number of people using them; capital input is measured using the number of intermediate goods invested, which encompasses the cost of purchasing seedlings, fertilizers, diesel fuel for agricultural use, plastic film, and pesticides. Land inputs are measured by the sown area, which is the sum of the sown area of the 10 crops; the sum of the sown area can take into account the situation of replanting and set-cropping, which can more accurately reflect the land inputs of the farmers. Due to the differences in prices between crops, applying a simple summation of yields is not appropriate. So, it is more reasonable to convert to a summation of the production value for each crop, calculated in the same way as in the previous section. In data processing, the variables involving prices and costs in this paper are deflated using deflators, and the final actual value is the price of the 2003-based period. Based on the previous discussion and the C-D production function, the agricultural production function for households in this paper is shown in Eq. (1).
In Eq. (1), i denotes the household, and t denotes the year. denotes the total output of household i in year t, and denotes the agricultural TFP. where denotes the capital input, denotes the land input, and denotes the labor input.
Next, Eq. (2) is used to estimate factor elasticities, and then Eq. (3) is used to calculate agricultural TFP at the household level.
To further explore the path of labor aging on agricultural TFP, agricultural resource allocation efficiency is introduced into the analytical framework. The inefficiency of resource allocation between sectors is also known as resource misallocation. In this paper, the OP covariance decomposition method is used to calculate agricultural resource allocation efficiency with reference to existing studies (Olley and Pakes, 1996). The method decomposes agricultural TFP into a mean term and a variance term. One is the unweighted average agricultural TFP across all households, and the other is the covariance of household output shares with agricultural TFP (i.e., the OP covariance term). In other words, the growth in agricultural TFP is an absolute increase in the mean agricultural TFP and an increase due to improved resource allocation efficiency. The OP covariance term, which is the concern of this paper, is used to measure resource allocation efficiency.
In Eq. (4), where denotes the farm household plus total agricultural TFP in year t, which is obtained by first deriving the household level TFP and then by weighted summing, using the method of Eq. (3), denotes the share of the household i’s output in total agricultural TFP in that year, denotes the absolute value of the household i’s agricultural TFP, the first term to the right of the equal sign is the unweighted mean value of agricultural TFP, and the second term is the OP covariance term.
In this study, we primarily employ a two-way fixed effects (FE) model to estimate the impact of aging on agricultural TFP. The two-way fixed effects model controls for both household-specific and time-specific effects, allowing us to account for unobserved heterogeneity that could bias our results. The two-way fixed effects model can be represented as follows:
In Eq. (5), where denotes agricultural TFP and is the core explanatory variable of this paper and is expressed in terms of the average age of household agricultural operators as a measure of the aging of the household labor force. denotes a set of control variables affecting agricultural TFP, including individual characteristics of agricultural business decision makers, household resource endowment, and village characteristics. and denote year effect and individual effect respectively, denotes a random disturbance term, and, and are parameters to be estimated.
There may be potential endogeneity in the aging of the labor force. On the one hand, endogeneity may arise from omitted variables, such as unobservable geotechnical factors. In agricultural production, the older the labor force may be, the more they tend to cultivate plots with good land fertility or accessibility to compensate for their deficiencies. On the other hand, there may be reverse causality, where agricultural TFP affects households’ expectations of income from cultivation, leading to a reconfiguration of the age of the labor force engaged in agriculture, resulting in the aging of household farm operators.
To solve the possible endogeneity problem between labor force aging and agricultural TFP of farm households, as well as to more accurately and comprehensively assess the impact of the aging level of agricultural production on productivity, this paper has two strategies to address the problem: firstly, using a fixed-effects model, which removes the unobserved effects, and thus can address part of the endogeneity problem; and, secondly, this paper goes further by using instrumental variables method for estimation. There may also be a reverse causality problem between agricultural TFP and labor force aging, i.e., a situation may arise where low productivity leads to a tendency for households to choose older labor in their labor allocation to agricultural operations. We use the share of construction workers in the labor force population at the village level as an instrumental variable for labor force aging. The two-stage instrumental variable model is set up as follows:
In Eq. (6), where denotes the level of labor force aging; and denote farm household characteristics and village-level control variables for other farm household agricultural TFP, respectively; In Eq. (7), denotes household-level farm household agricultural TFP; and denotes the instrumental variable, measured by the share of construction workers in the labor force population in the village.
The explained variable in this paper is agricultural TFP, which is accounted for using the Cobb–Douglas production function, which is logarithmized using the growth accounting method to derive the agricultural TFP for cultivation at the household level.
We focus on the aging of the agricultural labor force on the productivity of agricultural, using the average age of agricultural operators to measure the degree of aging (Aging). Where agricultural operators refer to the family members who are involved in agricultural labor during the year, there are two reasons for measuring the age profile of the agricultural labor force rather than the household labor force as a whole: First, if the older labor force is engaged in off-farm employment, the accuracy of this variable may be affected; Second, the use of the average age is a more accurate measure of the degree of aging of the agricultural labor force within the household compared to the use of the presence or absence of the agricultural production operator. To ensure the robustness of the results, we also utilize the share of agricultural producers older than 55/60/65 years old as an explanatory variable for the robustness test.
This paper controls for other factors affecting agricultural TFP. They mainly include famer characteristics, household characteristics, and village characteristics. Specifically, (1) Education level (Education), the number of years of education of agricultural business decision makers. (2) Health level (Health), the health degree of agricultural business decision makers, with a value range of 1–5, representing loss, poor, medium, good, and excellent of labor capacity, respectively. (3) The proportion of non-agricultural labor (Non-agricultural Employment), measured by the proportion of household non-agricultural labor to the total household labor force. (4) Cultivated land area (Farmland), measured by the area of cultivated land of the household at the end of the year. (5) The number of the cultivated plots (Land plots), the number of land plots at the end of the household year. (6) Amount of agricultural subsidies (Subsidy), the income from government subsidies received during the year. (7) The number of agricultural labor (labor), the number of household members who contribute more than 0 labor in the agricultural production process. (8) Development of village infrastructure (Infrastructure), the share of hardened roads in the village where the household is located. Infrastructure development in the village affects agricultural production and marketing channels, etc., and thus has an impact on productivity (see Table 1).
This paper adopts the share of construction workers in a farmer’s village as an instrumental variable for labor force aging. The variable number of construction workers at the village level has only been counted since 2009, so the instrumental variable uses data from 2009-2020 (IV). On the one hand, there is a correlation between the share of construction workers in the total labour force in villages and the degree of aging of the household labour force. The construction industry offers better incomes compared to agriculture and requires some skills and expertise. This is more attractive to young people, while older farming households are often unable to fulfill the physical requirements of the construction industry. Therefore older members of the household are engaged in agriculture in the villages, increasing the aging of the household agricultural labour force. On the other hand, the share of construction workers within villages is something that does not directly affect individual farm households and fulfills exogenous requirements. Table 2 reports the descriptive statistics of the variables in the model. The mean value of agricultural TFP of farm households is 4.697, which is consistent with the measured value of Wang et al. (2020). In terms of the degree of aging, the average age of the labor force engaged in agricultural production is 52.123 and shows an upward trend from year to year, with the maximum and minimum values of 18 and 89 years old, respectively. The description of other variables will not be repeated here.
Figure 2 reports the evolution of agricultural TFP over the period 2003–2020. Overall, agricultural TFP has not risen significantly, and resource allocation efficiency is decreasing. The figure reports agricultural TFP using the median, weights, and simple arithmetic mean, where weights refer to the share of each household’s total output of all farmers for the year. We find that the two calculations, the simple arithmetic mean and the median, show a consistent trend with an overall upward trend. However, there are decreases in individual years, and the growth rate shows a process from fast to slow. This measurement is consistent with the conclusions of most scholars (Wang et al., 2020). At the beginning of the 20th century, China’s agricultural TFP rose rapidly due to the rapid growth of inputs such as fertilizers and machinery. In recent years, agricultural productivity gains have gradually shifted from rapid growth to gradual optimization due to the diminishing marginal effects of input factors, and this also indicates a slowdown in agricultural TFP growth driven by factor inputs. Based on the trend of changes in the weighted average, agricultural TFP has exhibited a downward trajectory since 2017. Notably, agricultural TFP in 2010 shifted from exceeding the simple average to falling below it, indicating that farmers with higher agricultural output do not necessarily possess superior productivity. This observation suggests a decline in resource allocation efficiency.
Figure 2. Variation trend of agricultural TFP from 2003 to 2020. Data source: the national fixed point survey.
This section primarily examines the impact of an aging worker on agricultural TFP for rural households, and Table 2 presents the regression results. This section employs a stepwise regression approach, with Model 1 and Model 2 representing estimation results excluding and including all control variables, respectively. Model 3 represents the sample estimation excluding municipalities. The findings reveal that labor force aging has a significant negative impact on agricultural TFP for rural households, all significant at the 1% confidence level. Model 1 reports a regression coefficient of −0.002 for labor force aging on farmers’ agricultural TFP. Upon incorporating control variables, the coefficient value shifts to −0.001, indicating a reduction in the inhibitory effect of labor force aging on farmers’ agricultural TFP. These results align with the total sample when municipalities are excluded. These findings are consistent with existing literature. For example, Barnes (2023) also found that the aging agricultural workforce hinders technological progress and overall productivity. Similarly, Duesberg et al. (2017) reported that the increase in the average age of farmers leads to a decline in the adoption of innovative practices and technologies, thus reducing agricultural productivity. From a theoretical perspective, while older farmers may have experience in agricultural production, leading to higher output per unit area compared to the average level, the physical limitations of older farmers ultimately result in a decrease in output per unit area. This aging of the labor force ultimately suppresses agricultural TFP, as it faces opposing forces of enhancement and weakening. However, Rigg et al. (2020) discovered that the reduction in agricultural productivity in Thailand was not attributed to the aging workforce but to other factors. This discrepancy may arise from differences in regional agricultural practices, socio-economic conditions, and the varying roles of technology and infrastructure in agricultural productivity across different countries.
In the context of control variables, a positive correlation is observed between farmers’ health status and agricultural TFP. This finding underscores the importance of human capital in the agricultural production process. Farmers in better health can invest their labor more effectively in agricultural activities and possess energy to allocate resources (Davis et al., 2009). Furthermore, the proportion of non-farm labor negatively influences agricultural TFP for farm households. An increase in the share of non-farm labor corresponds to a decrease in the proportion of agricultural production in household income. As agricultural production plays a less significant role in income generation, farm households lack the incentive to invest in agricultural production. Consequently, agricultural production tends to remain at a basic level, leading to inefficient resource allocation and, ultimately, lower agricultural TFP (Li and Sicular, 2013). Additionally, our findings reveal a significant contribution of the number of arable land blocks and agricultural laborers to agricultural TFP. Notably, while more cultivated plots may exacerbate land fragmentation, they may also promote crop diversity and flexibility, allowing farmers to select more suitable crops, thereby enhancing output. Moreover, a more significant number of family farm laborers typically leads to a more granular division within the household, ultimately enhancing labor productivity and agricultural TFP.
This paper performs robustness tests by addressing possible endogeneity and substituting explanatory variables.
(1) Addressing endogenous issues
This paper addresses the endogeneity problem using 2SLS estimation; this paper performs the unidentifiable and weak instrumental variables tests on the instrumental variables. The p-value of the LM statistic is 0.001, and the F-statistic is 28.438, indicating no unidentifiable and weak instrumental variables problem. Table 3 reports the estimation results of the instrumental variable method; columns (1) and (2) show the 2SLS first and second-stage estimation results. It reports that the coefficient of labor force aging remains significantly negative; this indicates that labor force aging still plays a negative role in land productivity and TFP when endogeneity is addressed. It proves that the main findings of this paper are robust.
(2) Replacement of explanatory variables
The paper also performs robustness estimation by replacing the measures of the independent variables based on the baseline regression. Table 4 reports the estimation results, with the explanatory variables in Models 1, 2, and 3 being the share of farmers older than 55 years, 60 years, and 65 years, respectively. As can be seen from the table, the results of the effect of labor force aging on the TFP of household agriculture are still significantly negative, after replacing the independent variables. This indicates the robustness of the baseline regression results. Further analysis reveals that the coefficient decreases the higher the age threshold. It also tentatively suggests that the older the age of the farm household has a more negative effect on agricultural TFP.
To ensure food security, people should pay attention to the impact of agricultural quality improvement in the main grain-production area, and the external variability between the main grain-marketing areas and the grain-producing and marketing-balanced areas should also be considered (Rozelle and Swinnen, 2004; Ye et al., 2024). There may be differences in the impact of labor force aging on farm household agricultural TFP across food functional areas. This paper explores the differences in the impact of aging in different food functional areas, which is essential for coordinating the roles of each food production functional area. It is also crucial for ensuring food security in China. Columns (1)–(3) of Table 5 show the sample estimation results for the main grain-production area, the main grain-marketing areas, and the grain-producing and marketing-balanced areas.
The results show that labor force aging reduces farm household agricultural TFP for the sample farm households in the main grain-production area and the grain-producing and marketing-balanced areas. However, this effect is insignificant for farm households in the main grain-marketing areas; this suggests that labor force aging has a much smaller negative effect on farm household agricultural TFP in the main grain-marketing areas. The main food marketing areas tend to be more economically developed regions. These areas are mainly dominated by industrialization and urbanization (Hao et al., 2024). Local rural residents will be more absorbed into non-agricultural employment, and these farmers will be less dependent on land. Studies by Yin et al. (2006) and Zhang et al. (2021) show that the factor market for agricultural land is more active in the main grain marketing areas, which is conducive to the reallocation of agricultural land resources. Such factor reallocation is conducive to the improvement of resource allocation efficiency. Moreover, these regions have a better level of agricultural technology and agricultural equipment. Therefore, the aging labor force’s negative impact on farmers’ agricultural TFP in this region is minor.
The main objective of this section is to analyze whether changes in agricultural TFP productivity differ between large-scale and small-scale farmers when faced with the challenge of an aging labor force. There has yet to be a consensus on the definition of a sizeable household. However, the purpose of this paper is not to define the size of households but mainly to distinguish the differences exhibited by different sizes. Therefore, we employ the median to distinguish between large-scale and small-scale farmers. The regression results are shown in Table 6, with Model 1 and Model 2 representing the estimation results for large-scale and small-scale households. Models 3 and 4 represent the estimation results for scale households and small farmers in the primary production area sample.
There is a significant negative effect of labor force aging on agricultural TFP. For scale households, the coefficient is −0.001. It is significant at a 1% confidence level. However, there is no significant effect of labor force aging on agricultural TFP for small-scale households. Based on the aforementioned research findings, two primary conclusions can be drawn:
First, the negative impact of labor aging on agricultural TFP primarily originates from large-scale farmers. Large-scale farmers tend to invest more in fixed assets for agricultural production compared to small-scale farmers, purchasing agricultural machinery such as harvesters and rotary tillers. These machines not only substitute labor to create scale efficiency but also advance agricultural technology, as evidenced by the adoption of practices like deep plowing and conservation tillage. However, operating these machines requires higher proficiency and more advanced agricultural knowledge and management skills. As agricultural laborers age, their knowledge base and learning capacity decline, as do their abilities to operate machinery and manage farmland effectively (Li and Sicular, 2013). Consequently, large-scale farmers are more affected by labor aging, which negatively impacts agricultural TFP.
Second, for small-scale farmers, labor aging does not significantly affect agricultural TFP. This suggests that the rise in age among family agricultural labor does not lead to a noticeable decline in agricultural productivity. We attribute this to the characteristics of small-scale farming, particularly in terms of agricultural investment. Due to the smaller land scale, most small-scale farmers prefer to purchase smaller agricultural machines and outsource some production processes to service organizations. In recent years, agricultural production outsourcing has made significant progress in China. By the end of 2020, there were over 900,000 social service organizations providing agricultural production outsourcing. Many farmers outsource agricultural production processes to agricultural service organizations (Liu et al., 2024). This widespread adoption of agricultural production outsourcing among small-scale farmers helps mitigate the negative effects of physical limitations associated with aging farmers. This is similar to the observations made by Jansuwan and Zander (2021) in Thailand.
This paper uses two indicators to examine farmers’ adoption of new technologies to estimate the impact of labor force aging on technological progress; the first is the machinery input ratio, which refers to the ratio of the cost of machinery use per mu to the amount of labor input. It can reflect the substitution intensity of machinery for labor. Generally speaking, agricultural machinery and labor are vital in agricultural production. The greater the substitution intensity of machinery for labor, the more it reflects the progress of agricultural technology (Foster and Rosenzweig, 2022). Second, agricultural productive inputs. Empirical studies in developing countries have shown that increasing productive investment in agriculture is conducive to enhancing agricultural productivity (Adu-Baffour et al., 2019). Increased productive investment in agriculture can reflect the progress of agricultural production technology. Therefore, this paper will estimate the effect of labor force aging on the ratio of machinery inputs to agricultural productive investment to verify whether labor force aging reduces agricultural TFP by inhibiting technological progress. This is consistent with the conclusions of Asravor et al. (2024).
Table 7 shows the estimation results, with the explanatory variable for model 1 being the share of mechanized labor. Higher values of this ratio indicate more robust substitution. The explanatory variable of model 2 is the productive inputs to agriculture per unit area. The estimation results of model 1 show that the share of mechanical labor in agricultural production decreases significantly as the age of farm households increases; this indicates that the aging of the labor force reduces the substitution of machinery for labor in agricultural production. In other words, the older the farm household, the less inclined it is to mechanical labor substitution. The estimation results of Model 2 show that the higher the labor force aging, the lower the productive inputs to agriculture by farm households will be. This phenomenon also exists in other Asian countries, such as South Korea (Seok et al., 2018). This finding verifies that labor force aging inhibits agricultural technological progress. Fried and Tauer (2016) also reached the same conclusion, noting that an aging labor force slows down technological advancement. There are two main reasons for this; one is that older farmers tend to operate smaller farms and have the advantage of using their machinery compared to larger farms. They prefer to substitute mechanization with family labor in their production operations. Older farmers prefer investing more time in family labor to compensate for their declining physical capacity rather than substituting labor with advanced machinery and equipment (Chen et al., 2009). However, agricultural mechanization plays an essential role in promoting the upgrading of agricultural production methods and the modernization of agriculture (Liu et al., 2024). Secondly, due to the increase in the price of agricultural productive inputs, older farmers face more significant capital constraints (Qiu et al., 2021). They are more willing to invest less to save costs than to invest more capital in production; this is a choice that maximizes the interest of small farmers even if they expect a decrease in production. Thus, in this case, the aging labor force negatively affects agricultural TFP by inhibiting technological progress in agriculture.
We estimate the effect of the aging labor force on resource allocation efficiency, as shown in Table 8. Model 1 and Model 2 are for the whole and primary production area samples, respectively. The estimation results show that the aging labor reduces farm households’ resource allocation efficiency. In other words, the older the labor force is, the more farmers may be unable to maximize their resource advantages and improve the efficiency of agricultural output. As previously analyzed, older farm operators are more risk-averse (Li and Sicular, 2013) and are reluctant to expand the scale of their operations even if their productivity is high; this prevents their advantages from being maximized. On the other hand, they have a solid attachment to the land and are unwilling to transfer all their land to others for farming. Even if they do not have enough capacity, they still want to keep part of their land for operation, preventing productive farmers from acquiring more land. As age increases, the credit constraints farmers face also increase, preventing the efficiency of resource allocation from reaching the optimal level. The comparison of the coefficients in columns (1) (2) shows that the negative impact of labor force aging on resource allocation efficiency is smaller than the total sample in the main grain-production areas; this indicates a negative impact of the aging labor force on resource allocation efficiency for farmers in the main grain-production areas. However, the extent of this effect is smaller than the overall sample. The reason for this is related to the policies in the main grain-producing areas. China has a series of agricultural subsidies for the main grain-producing areas, and these policy subsidies alleviate factor mismatch.
One of the limitations of this study is the constraint related to data availability. Specifically, the study did not include input–output data from emerging agricultural entities such as agribusiness firms and cooperatives. As a result, the findings may not fully represent these new types of agricultural management entities. The exclusion of data from these modern agricultural operations could limit the generalizability of the conclusions, as the dynamics of agricultural productivity might differ significantly between traditional rural households and more organized agricultural enterprises.
To address these data limitations, future research should incorporate comprehensive datasets that encompass both traditional rural households and emerging agricultural entities such as agribusiness firms and cooperatives. Additionally, examining the impact of technological advancements and infrastructure development on agricultural productivity across various regions could provide a more nuanced understanding of the factors influencing agricultural TFP. Further studies might also explore the role of policy interventions in mitigating the adverse effects of an aging labor force on agricultural productivity.
After entering the 21st century, the development of aging has further accelerated, and many scholars have also done research on whether population aging affects agricultural production potential and food supply security (Jaquet et al., 2015; Rigg et al., 2020; Ren et al., 2023). However, few studies have analyzed the impact of labor force aging on agricultural TFP from a micro perspective.
In this study, we investigated the impact of the aging labor force on agricultural TFP using a large sample of farm household survey data in China from 2003 to 2020. This paper wants to investigate whether labor force aging impacts the comprehensive capacity of agricultural production and threatens the stability and efficiency of the food supply.
Our study has mainly made the following discoveries. First, labor aging significantly negatively impacts agricultural TFP among farmers. This conclusion remains robust even after addressing endogeneity issues and replacing explanatory variables. Expanding on our findings, it is noteworthy that similar conclusions regarding the negative impact of labor aging on agricultural TFP have been drawn in developing countries, notably Ghana (Asravor et al., 2024), thereby suggesting that this phenomenon is not exclusive to any particular level of economic development, but rather a universal challenge faced by developing nations. Due to their declining physical abilities and aversion to risk, older farmers are not well-suited to the development of new technologies and lack the motivation to expand their operations, resulting in lower levels of agricultural TFP. Second, through heterogeneity analysis, we found that labor aging has significant differences in its impact on agricultural TFP among farmers in different grain functional areas in China. It has a significant adverse effect on the main grain-production area and grain-production and marketing-balanced areas. However, its impact on farmers in the main grain-marketing areas is insignificant. Additionally, labor aging has a significant negative impact on large-scale farmers’ agricultural TFP but no significant impact on small farmers. Third, further analysis revealed that labor aging negatively affects agricultural TFP by inhibiting technological progress and reducing the efficiency of agricultural resource allocation. As farmers age, older individuals are disadvantaged in receiving new information and may have a negative attitude toward adopting new technologies. Labor aging also reduces the efficiency of resource allocation, as older farmers tend to rely more on their experience in agricultural production and rarely adjust their factor allocation, resulting in a mismatch between their productivity levels and shares; this leads to inefficient resource allocation and thus suppresses agricultural TFP.
Based on the above analysis, it can be recognized that the aging of the agricultural labor force, as an irreversible trend, is a real problem that must be faced for some time to come. This issue is not unique to China but is also prevalent in many developing countries. There are three policy implications based on the findings of this paper:
Firstly, more young people should be absorbed into the agricultural sector across developing countries to better utilize the advantages of modern agricultural production technology and to enhance the comprehensive capacity of agricultural production. Concurrently, it is essential to provide technical training for the existing elderly farmers to improve their acceptance and utilization of new technologies.
Secondly, attention should be paid to the coordinated development of the functional food production areas. Additionally, developing countries must focus on the aging agricultural labor force in food-exporting regions. Enhancing the compensation mechanisms for leading grain production areas and providing more supportive policies for these regions is crucial. This includes ensuring fair compensation and robust support for regions that are vital to national and global food security. Thirdly, optimizing resource allocation and strengthening infrastructure development is necessary to improve conditions for agricultural production, including transportation, communication, and other essential infrastructures. Improving the construction of factor markets is also important to reduce the barriers faced by older farmers in these markets. Developing countries must focus on improving infrastructure and resource allocation to support aging agricultural populations, which is essential for maintaining productivity and sustainability in the agricultural sector.
The original contributions presented in the study are included in the article/supplementary material, further inquiries can be directed to the corresponding authors.
TT: Conceptualization, Data curation, Formal analysis, Funding acquisition, Investigation, Methodology, Project administration, Software, Supervision, Validation, Visualization, Writing – original draft, Writing – review & editing. FY: Data curation, Formal analysis, Methodology, Visualization, Writing – original draft, Writing – review & editing. QZ: Validation, Writing – original draft. WL: Validation, Writing – review & editing. YD: Formal analysis, Writing – original draft. YL: Conceptualization, Writing – original draft. GL: Resources, Supervision, Writing – original draft, Writing – review & editing.
The author(s) declare financial support was received for the research, authorship, and/or publication of this article. The research is financially supported by the National Social Sciences Foundation of China (NSSFC no. 18ZDA072).
The authors declare that the research was conducted in the absence of any commercial or financial relationships that could be construed as a potential conflict of interest.
All claims expressed in this article are solely those of the authors and do not necessarily represent those of their affiliated organizations, or those of the publisher, the editors and the reviewers. Any product that may be evaluated in this article, or claim that may be made by its manufacturer, is not guaranteed or endorsed by the publisher.
Adamopoulos, T., Brandt, L., Leight, J., and Restuccia, D. (2022). Misallocation, selection, and productivity: a quantitative analysis with panel data from China. Econometrica 90, 1261–1282. doi: 10.3982/ECTA16598
Adamopoulos, T., and Restuccia, D. (2014). The size distribution of farms and international productivity differences. Am. Econ. Rev. 104, 1667–1697. doi: 10.1257/aer.104.6.1667
Adu-Baffour, F., Daum, T., and Birner, R. (2019). Can small farms benefit from big companies' initiatives to promote mechanization in Africa? A case study from Zambia. Food Policy 84, 133–145. doi: 10.1016/j.foodpol.2019.03.007
Asravor, J. , Tsiboe, F. , Asravor, R. K. , Wiredu, A. N., and Zeller, M. Technology and managerial performance of farm operators by age in Ghana. J. Prod. Anal., 61: 279–303. (2024). doi: 10.1007/s11123-023-00679-y
Barnes, A. P. (2023). The role of family life-cycle events on persistent and transient inefficiencies in less favoured areas. J. Agric. Econ. 74, 295–315. doi: 10.1111/1477-9552.12506
Benjamin, D., Brandt, L., and Giles, J. (2005). The evolution of income inequality in rural China. Econ. Dev. Cult. Chang. 53, 769–824. doi: 10.1086/428713
Cao, K. H., and Birchenall, J. A. (2013). Agricultural productivity, structural change, and economic growth in post-reform China. J. Dev. Econ. 104, 165–180. doi: 10.1016/j.jdeveco.2013.06.001
Chari, A., Liu, E. M., Wang, S. Y., and Wang, Y. (2021). Property rights, land misallocation, and agricultural efficiency in China. Rev. Econ. Stud. 88, 1831–1862. doi: 10.1093/restud/rdaa072
Chen, Z., Huffman, W. E., and Rozelle, S. (2009). Farm technology and technical efficiency: evidence from four regions in China. China Econ. Rev. 20, 153–161. doi: 10.1016/j.chieco.2009.03.002
Davis, J., Caskie, P., and Wallace, M. (2009). Economics of farmer early retirement policy. Appl. Econ. 41, 35–43. doi: 10.1080/00036840600994211
De Brauw, A., and Rozelle, S. (2008). Migration and household investment in rural China. China Econ. Rev. 19, 320–335. doi: 10.1016/j.chieco.2006.10.004
Duesberg, S., Bogue, P., and Renwick, A. (2017). Retirement farming or sustainable growth–land transfer choices for farmers without a successor. Land Use Policy 61, 526–535. doi: 10.1016/j.landusepol.2016.12.007
Fan, S., and Pardey, P. G. (1997). Research, productivity, and output growth in Chinese agriculture. J. Dev. Econ. 53, 115–137. doi: 10.1016/S0304-3878(97)00005-9
Foster, A. D., and Rosenzweig, M. R. (2022). Are there too many farms in the world? Labor market transaction costs, machine capacities, and optimal farm size. J. Polit. Econ. 130, 636–680. doi: 10.1086/717890
Fried, H. O., and Tauer, L. W. (2016). The aging US farmer: should we worry? In: Advances in Efficiency and Productivity, (eds) J. Aparicio, C. Lovell, and J. Pastor. (Cham. Switzerland: Springer).
Gai, Q., Cheng, M., Zhu, X., and Shi, Q. H. (2020). Can land rent improve land allocation's efficiency?-Evidence from National Fixed Point Survey. J. Econ. 20, 321–340. doi: 10.13821/j.cnki.ceq.2020.04.17
Guirkinger, C., and Boucher, S. R. (2008). Credit constraints and productivity in Peruvian agriculture. Agric. Econ. 39, 295–308. doi: 10.1111/j.1574-0862.2008.00334.x
Guo, L., Song, Y., Zhao, S., Tang, M., Guo, Y., Su, M., et al. (2022). Dynamic linkage between aging, mechanizations and carbon emissions from agricultural production. Int. J. Environ. Res. Public Health 19:6191. doi: 10.3390/ijerph19106191
Hao, S., Wang, G. G., Yang, Y. T., Zhao, S. C., Huang, S. N., Liu, L. P., et al. (2024). Promoting grain production through high-standard farmland construction: evidence in China. J. Integr. Agric. 23, 324–335. doi: 10.1016/j.jia.2023.11.021
Jansuwan, P., and Zander, K. K. (2021). What to do with the farmland? Coping with ageing in rural Thailand. J. Rural Stud. 81, 37–46. doi: 10.1016/j.jrurstud.2020.12.003
Jaquet, S., Schwilch, G., Hartung-Hofmann, F., Adhikari, A., Sudmeier-Rieux, K., Shrestha, G., et al. (2015). Does outmigration lead to land degradation? Labour shortage and land management in a western Nepal watershed. Appl. Geogr. 62, 157–170. doi: 10.1016/j.apgeog.2015.04.013
Lee, J., Oh, Y. G., Yoo, S. H., and Suh, K. (2021). Vulnerability assessment of rural aging community for abandoned farmlands in South Korea. Land Use Policy 108:105544. doi: 10.1016/j.landusepol.2021.105544
Li, M., and Sicular, T. (2013). Aging of the labor force and technical efficiency in crop production: evidence from Liaoning province, China. China Agric. Econ. Rev. 5, 342–359. doi: 10.1108/CAER-01-2012-0001
Lin, J. Y. (1992). Rural reforms and agricultural growth in China. Am. Econ. Rev. 82, 34–51. doi: 10.1093/oso/9780199240609.003.0010
Liu, J. C., Mao, S. Z., Zheng, Q. F., and Xu, Z. G. (2024). Can whole steps of grain production be outsourced? Empirical analysis based on the three provinces of Jiangsu, Jilin, and Sichuan in China. J. Integr. Agric. 23, 336–347. doi: 10.1016/j.jia.2023.09.034
National Bureau of Statistics , (1997). (NBS) of the People’s Republic of China. Accessed on December 1,2023. Available at: https://www.stats.gov.cn/sj/tjgb/nypcgb/qgnypcgb/202302/t20230206_1902094.html
National Bureau of Statistics , (2016). (NBS) of the People’s Republic of China. Accessed on December 1,2023. Available at: https://www.stats.gov.cn/sj/tjgb/nypcgb/qgnypcgb/202302/t20230206_1902105.html
Olley, G. S., and Pakes, A. (1996). The dynamics of productivity in the telecommunications equipment industry. Econometrica 64, 1263–1297. doi: 10.2307/2171831
Potter, C., and Lobley, M. (1992). Aging and succession on family farms: the impact on decision-making and land use. Sociol. Rural. 32, 317–334. doi: 10.1111/J.1467-9523.1992.tb00935.X
Qiu, T., Choy, S. T. B., Li, Y., and Li, J. (2021). Farmers' exit from land operation in rural China: does the price of agricultural mechanization services matter? Chin. World. Econ. 29, 99–122. doi: 10.1111/cwe.12372
Ren, C., Zhou, X., Wang, C., Guo, Y., Diao, Y., Shen, S., et al. (2023). Ageing threatens sustainability of smallholder farming in China. Nature 616, 96–103. doi: 10.1038/s41586-023-05738-w
Rigg, J., Phongsiri, M., Promphakping, B., Salamanca, A., and Sripun, M. (2020). Who will tend the farm? Interrogating the ageing Asian farmer. J. Peasant Stud. 47, 306–325. doi: 10.1080/03066150.2019.1572605
Rozelle, S., and Swinnen, J. F. M. (2004). Success and failure of reform: insights from the transition of agriculture. J. Econ. Lit. 42, 404–456. doi: 10.1257/0022051041409048
Seok, J. H., Moon, H., Kim, G., and Reed, M. R. (2018). Is aging the important factor for sustainable agricultural development in Korea? Evidence from the relationship between aging and farm technical efficiency: 7. Sustain. For. 10:2137. doi: 10.3390/su10072137
Sheng, Y., Tian, X., Qiao, W., and Peng, C. (2020). Measuring agricultural total factor productivity in China: pattern and drivers over the period of 1978-2016. Aust. J. Agric. Resour. Econ. 64, 82–103. doi: 10.1111/1467-8489.12327
Skirbekk, V. (2004). “Age and individual productivity: a literature survey” in Vienna Yearbook of Population Research, vol. 1, 133–154.
Tang, J., and MacLeod, C. (2006). Labor force ageing and productivity performance in Canada. Can. J. Econ. 39, 582–603. doi: 10.1111/j.0008-4085.2006.00361.x
Van, S., Nevens, F., Mathijs, E., and Van, G. (2007). Measuring farm sustainability and explaining differences in sustainable efficiency. Ecol. Econ. 62, 149–161. doi: 10.1016/j.ecolecon.2006.06.008
Wang, S. L., Huang, J., Wang, X., and Tuan, F. (2019). Are China's regional agricultural productivities converging: how and why? Food Policy 86:101727. doi: 10.1016/j.foodpol.2019.05.010
Wang, L., Yang, R. D., and Wu, B. (2020). A study on Total factor productivity of agricultural production of rural households in China. Manage. World 36, 77–93. doi: 10.19744/j.cnki.11-1235/f.2020.0185
Ye, F., Qin, S., Li, H., Li, Z., and Tong, T. (2024). Policy-driven food security: investigating the impact of China’s maize subsidy policy reform on farmer’productivity. Front. Sustain. Food Syst. 8:1349765. doi: 10.3389/fsufs.2024.1349765
Yin, P., Fang, X., Tian, Q., and Ma, Y. (2006). The changing regional distribution of grain production in China in the 21st century. J. Geogr. Sci. 16, 396–404. doi: 10.1007/s11442-006-0402-x
Zhang, Q. Q., Gao, X. X., Abdullahi, N. M., Yue, W., and Huo, X. X. (2023). Asset specificity and farmers' intergenerational succession willingness of apple management. J. Integr. Agric. 22, 2553–2566. doi: 10.1016/j.jia.2023.04.016
Zhang, D., Wang, H., and Lou, S. (2021). Research on grain production efficiency in China’s main grain-producing areas from the perspective of grain subsidy. Environ. Technol. Innov. 22:101530. doi: 10.1016/j.eti.2021.101530
Keywords: aging labor force, food security, agricultural TFP, food sustainability, China
Citation: Tong T, Ye F, Zhang Q, Liao W, Ding Y, Liu Y and Li G (2024) The impact of labor force aging on agricultural total factor productivity of farmers in China: implications for food sustainability. Front. Sustain. Food Syst. 8:1434604. doi: 10.3389/fsufs.2024.1434604
Received: 18 May 2024; Accepted: 19 July 2024;
Published: 06 August 2024.
Edited by:
Olutosin Ademola Otekunrin, University of Ibadan, NigeriaReviewed by:
Omarsherif Mohammed Jemal, Arsi University, EthiopiaCopyright © 2024 Tong, Ye, Zhang, Liao, Ding, Liu and Li. This is an open-access article distributed under the terms of the Creative Commons Attribution License (CC BY). The use, distribution or reproduction in other forums is permitted, provided the original author(s) and the copyright owner(s) are credited and that the original publication in this journal is cited, in accordance with accepted academic practice. No use, distribution or reproduction is permitted which does not comply with these terms.
*Correspondence: Feng Ye, eWV4aXdlbjE5OTVAanhhdS5lZHUuY24=; Gucheng Li, bGdjYWJjQG1haWwuaHphdS5lZHUuY24=
Disclaimer: All claims expressed in this article are solely those of the authors and do not necessarily represent those of their affiliated organizations, or those of the publisher, the editors and the reviewers. Any product that may be evaluated in this article or claim that may be made by its manufacturer is not guaranteed or endorsed by the publisher.
Research integrity at Frontiers
Learn more about the work of our research integrity team to safeguard the quality of each article we publish.