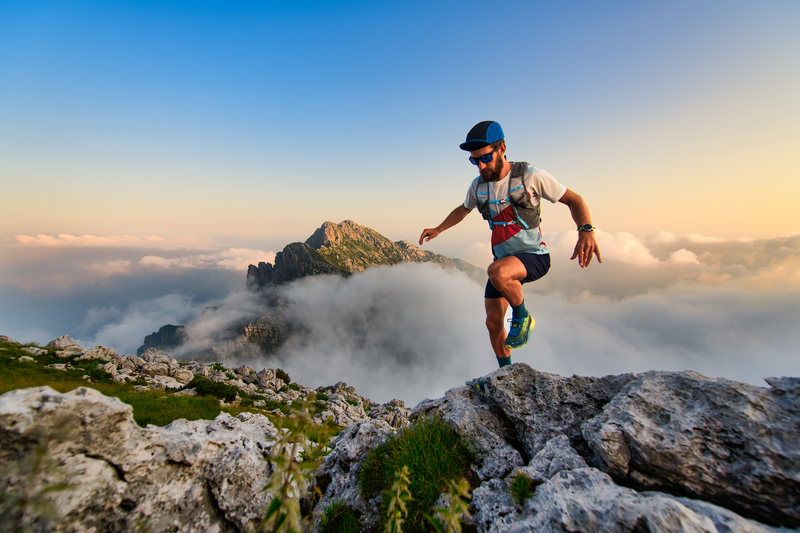
94% of researchers rate our articles as excellent or good
Learn more about the work of our research integrity team to safeguard the quality of each article we publish.
Find out more
ORIGINAL RESEARCH article
Front. Sustain. Food Syst. , 15 July 2024
Sec. Agricultural and Food Economics
Volume 8 - 2024 | https://doi.org/10.3389/fsufs.2024.1432184
This article is part of the Research Topic Strategies Of Digitalization And Sustainability In Agrifood Value Chains View all 23 articles
Introduction: The rapid development of agriculture has brought about significant negative impacts on the environment, such as land pollution and ecological degradation. The root cause of environmental issues lies in human behavior, with improper farming practices by farmers being a major contributor to agricultural pollution. This paper explores the relationship between farmers' digital literacy and their pro-environmental behaviors, examining the mediating roles of subjective norms, behavioral attitudes, and perceived behavioral control. Additionally, it investigates the varying impacts of digital literacy on PEB among farmers with different levels of education, social capital, and household income.
Methods: Based on data from the China Land Economic Survey (CLES), this study utilizes an ordered probit regression analysis method to analyze data from 923 sample respondents in 24 villages in Jiangsu Province. All analyses were conducted using Stata 15.0.
Results: The research findings indicate that digital literacy enhances the likelihood of farmers engaging in PEB. This practice is achieved by reinforcing farmers' subjective norms, strengthening their behavioral attitudes, and enhancing their perceived behavioral control (as the core elements in the Theory of Planned Behavior theory), thereby promoting the implementation of PEB among farmers. Further analysis reveals that digital literacy plays a crucial role in enhancing PEB among farmers with higher levels of education, social capital, and household income.
Discussion: The results of this study suggest that policymakers should enhance farmers' digital literacy and implement specific measures to improve farmers' subjective norms, behavioral attitudes, and perceived behavioral control. When the digital literacy and willingness for PEB are improved, farmers may engage in environmentally friendly practices.
With the development of the global economy, environmental issues in various countries are gradually becoming apparent, and the increasingly severe environmental risks are driving the world toward disaster (Yu et al., 2022). Agricultural systems have an extremely important impact on the environment. According to statistics, the global food system accounts for more than one-third of global anthropogenic greenhouse gas emissions, with the largest contribution coming from agricultural and land-use change activities (Crippa et al., 2021; Tubiello et al., 2021). Globally, especially for developing countries, the negative impacts of agricultural development on the environment are worsening (Cao et al., 2020). Over the past few decades, China's agricultural development has achieved remarkable accomplishments. China, with only 9% of the world's arable land, feeds nearly 20% of the global population, while also increasing farmers' income and quality of life (Mi et al., 2020). However, the rapid development of agriculture has also brought about significant negative impacts on the environment, such as land pollution and ecological degradation. According to data from the Food and Agriculture Organization (FAO) of the United Nations, in 2020, the average pesticide use per hectare of arable land in China was 1.95 kg, higher than the world average of 1.81 kg, with the annual insecticide usage being 70,804.73 tons, higher than that of the United States at 65,770.8 tons1. Therefore, changing China's traditional extensive agricultural production methods and promoting the green and sustainable development of agriculture are urgent issues that need to be addressed in China's future agricultural development process.
Fortunately, China has recognized the imbalance between economic development and environmental protection and has successively enacted a series of policies to promote the green and sustainable development of agriculture. However, the dispersed, concealed, and lagging characteristics of agricultural pollution determine that current measures such as post-pollution control through laws and regulations and point source control policies are difficult to achieve the desired effects (Guo and Zhao, 2014). The root cause of environmental issues lies in human behavior (Price and Leviston, 2014), and farmers, as the micro subjects of agricultural production, have engaged in inappropriate production practices, which have become the main cause of agricultural pollution in China (Liu et al., 2021). Farmers' PEB refers to their conscious adoption of low-pollution, reuse, and reduction agricultural management practices during the production process. Relying on farmers' PEB can effectively address agricultural pollution at its source (Cheng and Monroe, 2012; Shi et al., 2018). Therefore, a thorough analysis of the underlying mechanisms of farmers' PEB and guiding farmers to consciously practice PEB in agricultural models are crucial for overcoming agricultural ecological environmental challenges and promoting the green and sustainable development of agriculture.
Research on farmers' PEB primarily focuses on two aspects: on one hand, analyzing the influence of external factors such as policy support, farm size, farming conditions, and household characteristics on farmers' PEB (Khataza et al., 2018; Marr and Howley, 2019); on the other hand, examining the effect of farmers' personal endowments, such as gender, age, livelihood capital, social capital, and education level, on farmers' PEB (Botetzagias et al., 2015; Bakker et al., 2021; Yu et al., 2021). However, as farmers are individuals with independent thoughts, their PEB is not only influenced by external factors but also driven by internal factors. The farmers' inner acceptance and support for PEB are necessary prerequisites for engaging in PEB. Motivation, as the precursor of individual action, can propel farmers toward PEB (Hattie et al., 2020). Scholars have started to pay attention to the relationship between subjective factors such as values, cognition, and emotions, and farmers' PEB (Byrne and O'Regan, 2014; Zhang et al., 2016; Fu et al., 2017). For example, Ali et al. (2020) found that farmers' optimistic attitudes affect behavioral changes and the adoption of green technologies in rice production among Ghanaian farmers.
Farmers' attitudes toward PEB depend on both the information they possess and the information they obtain externally. The digital revolution has created opportunities for farmers to remotely and cost-effectively access various information. Digital technologies play a crucial role in changing farmers' cognition and attitudes by helping them acquire information. However, digital literacy, which determines the extent to which they can access and utilize information, is often overlooked in research on PEB. Firstly, existing research has not treated digital literacy as an independent variable, thus neglecting its significant role in farmers' PEB. Particularly in the digital economy era, farmers with higher digital literacy can better apply digital technologies to transform traditional agricultural practices, and the impact of digital literacy on farmers' PEB cannot be ignored (Huang et al., 2022). Secondly, the measurement of farmers' PEB is relatively singular, mostly focusing on a specific behavior, such as avoiding straw burning (Bell et al., 2016). This approach lacks a systematic measurement of farmers' PEB and overlooks the differences in PEB among different farmers. Thirdly, the willingness of farmers to engage in PEB has not been incorporated into the study to explore the relationships and pathways between relevant variables. As rational individuals, farmers are often guided by self-interest, and their PEB are mainly determined by their behavioral intentions (Cao et al., 2022). Therefore, the research questions of this paper are focused on farmers, aiming to investigate whether digital literacy, as a critical factor, can influence farmers' PEB, how this influence occurs, and the differences in its impact on various PEBs among different farmers. The goal is to better promote the adoption of PEBs by farmers and to reduce the environmental damage caused by agricultural production.
Building upon the existing literature and addressing its limitations, this study is grounded in the context of agricultural green transformation under the conditions of the digital economy. It focuses on the impact of digital literacy on farmers' PEB and integrates the Theory of Planned Behavior into the analytical framework to further explore the role of farmers' PEB intentions in the influencing mechanism. Subsequently, using data from the 2022 China Land Economic Survey (CLES) as a sample, this study empirically analyzes the influence of digital literacy on farmers' PEB from a micro perspective, the mediating role of farmers' behavioral intentions, and the differential impact of farmers' digital literacy on different types of PEB. These analyses provide valuable insights for standardizing farmers' PEB and comprehensively enhancing the level of agricultural sustainable development.
This study contributes to the literature in several aspects. Firstly, in the context of the digital economy era, it analyzes farmers' PEB from the perspective of digital literacy, offering a novel viewpoint to promote farmers' PEB. Secondly, it enriches the measurement of farmers' PEB by assessing it in three phases: pre-production, production, and post-production, providing a comprehensive reflection of farmers' PEB status. Additionally, it designs an index system for farmers' digital literacy from the perspectives of digital technology accessibility and depth of usage, and utilizes the entropy method to measure it, reflecting farmers' level of digital literacy. Thirdly, by incorporating the Theory of Planned Behavior into the analysis of the impact mechanism of digital literacy on farmers' PEB, it clarifies the relationships among digital literacy, farmers' behavioral intentions, and farmers' PEB through mediating effect tests, revealing new pathways to promote farmers' engagement in PEB. Furthermore, it explores the differential impact of farmers' digital literacy on different types of PEB, providing insights for formulating targeted green production guidance policies.
The remaining sections of this study are organized as follows. Section 2 reviews previous literature and formulates research hypotheses through theoretical analysis. Section 3 introduces the materials and research methods of the paper, including data collection, variable measurement, and the construction of econometric models. Section 4 presents the empirical analysis results. Section 5 discusses the research findings. Section 6 concludes the study and identifies future research directions.
According to farmer behavior theory, rational economic agents, farmers, aim to maximize operational profits in their production decisions. Therefore, the economic value brought by PEB becomes the driving force for farmers to adopt such behavior to a greater extent (Huang et al., 2018). Whether PEB can bring economic value is driven by external information such as production costs, expected returns, and government policies. Accessing extensive information helps farmers gain a deeper understanding of PEB and reduces their resistance to PEB due to insufficient information (Yu et al., 2020). In the digital economy era, digital information plays an increasingly important role. Timely, accurate, and abundant digital information provides basic data and reference for farmers' decision-making (Molinillo and Japutra, 2017). The digital divide resulting from the low level of digital literacy among farmers creates barriers to their acquisition and utilization of digital information. Existing research defines digital literacy as the ability of individuals to effectively obtain and appropriately use relevant information through internet platforms (Hargittai, 2005). For the farmers, who are the focus of this study, they first need to have access to digital devices for acquiring digital information. Furthermore, they must be able to comprehend, apply, and critically evaluate the digital information obtained in their production and daily life. This allows farmers to expand their sources of information, break free from information silos, and be more willing to engage in PEB. Therefore, this paper defines farmers' digital literacy as the ability of farmers to use digital devices to acquire digital information and apply the obtained digital information in their production and management processes. The impact of digital literacy on farmers' PEB can be specifically analyzed from the perspectives of inputs and outputs. In terms of input, farmers with high digital literacy have richer information reserves related to PEB, enabling them to accurately grasp the input of agricultural production factors, avoid excessive input of factors, and thus reduce the costs brought by PEB. In terms of output, the influence of digital literacy on PEB mainly manifests in expected output and unexpected output (Wang et al., 2023). For expected output, farmers obtain information about agricultural production through WeChat public accounts and agricultural service apps, choose suitable crop varieties according to market demand, and promote productivity improvement by adopting green technologies, thereby increasing the income brought by PEB (Wang et al., 2024). For unexpected output, the government usually formulates corresponding policies and systems to reward farmers engaged in green production to encourage PEB, and punish farmers who harm the environment to reduce environmentally harmful behavior. Farmers with high digital literacy have strong cognitive abilities regarding information and can timely access policy information about green production. In order to obtain PEB subsidies and avoid penalties, they correctly allocate production factors and reduce agricultural pollution (Song et al., 2020; Yang et al., 2024). Based on the above, this paper puts forward the first research hypothesis.
Hypothesis 1. Digital literacy has a significant positive impact on farmers' PEB.
Proposed by Ajzen (1991), the Theory of Planned Behavior (TPB) has been widely applied to study the formation of various human behaviors. According to TPB, farmers' PEB is determined by their behavioral intentions, which are in turn influenced by three core psychosocial structure variables, including subjective norms (SN), behavioral attitudes (BA), and perceived behavioral control (PBC) (Cao et al., 2022). In the process of agricultural production and management, PEB by farmers encompasses practices such as soil testing-based fertilization and environmentally-friendly pest control, which aim to balance economic, ecological, and social benefits (Yu et al., 2017). These behaviors exhibit characteristics of positive externalities, such as long-term investment returns, high risks, and requirements for large-scale operations, yet the positive externalities of PEB are challenging to internalize completely. As rational economic agents, farmers seek to maximize producer utility, with the narrow objective of profit maximization in economic markets (Dowlatshahi, 2010). The uncertainty of benefits derived from PEB contributes to a lower willingness among farmers to engage in such behavior. However, the improvement in farmers' digital literacy levels can lead to a clearer understanding of their SN, a more positive BA toward PEB, and a stronger PBC. This alteration from previously low profit expectations regarding PEB further enhances the willingness to engage in PEB and ultimately implement it. In summary, this study will analyze the indirect impact of digital literacy on farmers' PEB from the dimensions of SN, BA, and PBC.
Firstly, digital literacy strengthens farmers' SN and promotes their engagement in PEB. SN refers to the perception of social pressure individuals feel when adopting a specific behavior. SN emphasizes farmers' awareness of social pressure, as they often consider the pressure exerted by relevant stakeholders when deciding whether to adopt PEB in agricultural production and management. For farmers with higher levels of digital literacy, on one hand, they intuitively understand the harm to the environment caused by non-green practices in agricultural production through browsing images, watching videos, listening to news, etc., which stimulates a sense of moral responsibility and increases pressure for environmental protection, thereby strengthening their SN (Zeng, 2023). On the other hand, they can use modern communication technologies to enhance communication and exchange with other farmers, making it easier for them to perceive the environmental pressure exerted by other farmers implementing PEB under the influence of peer effects. Additionally, farmers with higher digital literacy are more likely to have their online interactions with other stakeholders open to public information, which is understood by other farmers, thereby forming an implicit supervision of farmers' PEB. Once environmental pollution issues in agricultural production are exposed, negative publicity will quickly ferment within the social network of the farmers, leading to irreversible and serious consequences (Halvorsen, 2012; Lakhan, 2015). Through the above analysis, farmers with high digital literacy can enhance their SN based on the pressure exerted by external stakeholders on PEB, forming the willingness to engage in PEB and promoting the implementation of PEB by farmers. Based on the above, this paper proposes the following research hypotheses.
Hypothesis 2a. Digital literacy promotes farmers' engagement in PEB by strengthening their SN.
Secondly, digital literacy enhances farmers' BA, thus promoting their engagement in PEB. BA refers to an individual's degree of self-acceptance of certain behaviors, reflecting their positive or negative evaluation of those behaviors. Farmers assess each specific PEB based on their own judgment, considering the advantages and disadvantages of different PEB, and formulating their own attitudes toward whether to engage in a particular behavior (Klöckner, 2013). Farmers with high digital literacy can leverage digital technologies to expand their access to agricultural information, gaining a better understanding of the importance of PEB for the environment (Wang et al., 2024). Additionally, farmers with high digital literacy often sell agricultural products through e-commerce platforms such as shopping websites and live streaming sales, enabling direct interaction between the production and consumption ends of agricultural products. Through this approach, farmers can gain insights into consumers' demand for environmentally friendly agricultural products and experience the benefits of green agricultural products, thereby increasing their acceptance and recognition of PEB (Kansiime et al., 2019). Through the aforementioned analysis, farmers with high digital literacy can expand their channels for accessing agricultural information, enhance their connection with the market, and form positive evaluations of PEB, thereby strengthening their willingness to implement PEB and adopting various PEB in agricultural production. Based on the above, this paper proposes the following research hypotheses.
Hypothesis 2b. Digital literacy promotes farmers' engagement in PEB by enhancing their BA.
Thirdly, digital literacy enhances farmers' PBC, thereby promoting their engagement in PEB. PBC reflects individuals' evaluation of challenges when taking specific actions, indicating the degree to which they perceive control and feasibility in the process of taking action. Digital literacy can enhance farmers' PBC in several ways. Firstly, farmers with high digital literacy are more likely to access loans, alleviating the financial constraints of PEB. Compared to traditional agricultural practices, PEB requires increased production costs. Farmers with higher digital literacy can overcome geographical limitations in obtaining loans by using digital financial tools. They can also apply for some unsecured and fast-loan credit services, thereby breaking financial constraints and enhancing PBC (Li and Zhou, 2023). Secondly, PEB by farmers inevitably involves new mechanical equipment. Farmers with high digital literacy are more likely to master efficient agricultural machinery to meet the equipment requirements for PEB, thereby reducing their perception of difficulties (Gong et al., 2024). Finally, PEB by farmers mainly involves the adoption of pro-environmental technologies, and their mastery of these technologies influences their PBC. Farmers with high digital literacy are often more capable of identifying, digesting, and applying relevant technological information. Possessing appropriate green production technologies makes it easier to address the challenges of implementing PEB, thereby enhancing their PBC (Bai et al., 2023). Through the above analysis, farmers with high digital literacy can access financial support, agricultural machinery, and technological assistance to address the challenges of implementing PEB, thereby strengthening their PBC and increasing their willingness to adopt PEB, making it more likely to engage in PEB. Based on the above, this paper proposes the following research hypotheses.
Hypothesis 2c. Digital literacy promotes farmers' engagement in PEB by enhancing their PBC.
For the subject farmers of this study, they belong to a highly heterogeneous group, so the impact of digital literacy on PEB may vary among farmers with different characteristics. Firstly, according to the theory of innovation diffusion, technological complexity is one of the main obstacles faced by adopters (Acikgoz et al., 2023). Farmers engaging in PEB require the acceptance of new knowledge and technology. Farmers with higher levels of education have higher cognitive and acceptance capabilities, possess better digital literacy, and are more likely to learn and master knowledge and skills related to agricultural green production. Therefore, the influence of digital literacy on PEB is more significant among farmers with higher education levels. Secondly, social capital, as the ability of individuals to allocate various resources in society, determines the extent to which digital literacy affects PEB among farmers. Farmers with high social capital have abundant social resources, making it easier for them to access knowledge and skills related to digitalization. Through frequent interactions with diverse entities, they enhance their digital literacy (Neumeyer et al., 2020). Finally, most farmers have a weak resistance to risk and tend to have a higher risk-averse attitude. Farmers with lower family income are less able to bear the potential losses associated with engaging in PEB (Wang et al., 2020). Therefore, compared to high-income farmers, the impact of digital literacy on PEB is smaller among low-income farmers. Based on the above, this paper proposes the following research hypotheses.
Hypothesis 3. Digital literacy is more likely to facilitate farmers with higher levels of education, higher social capital, and higher family income to engage in PEB.
The analysis framework illustrating how digital literacy promotes PEB among farmers is presented in Figure 1.
This study utilized the China Land Economic Survey (CLES) conducted by Nanjing Agricultural University in 2022 in Jiangsu Province. The CLES aims to provide reliable empirical evidence to illustrate the rural production and operation situation in Jiangsu Province, and to offer reference for government departments in formulating rational policy interventions. The survey questionnaire covers various aspects including rural infrastructure, factor markets, rural governance, inclusive finance, digital agriculture, living environment, and rural elderly care development. It reflects the micro-level individual farmers' situation of green production. The data's good representativeness and reliability provide comprehensive support for this study. The survey utilized the Probability Proportional to Size (PPS) sampling method, randomly selecting sample counties/districts and administrative villages to ensure sufficient diversity and balanced representation across different groups, thereby reducing sample selection bias. To further mitigate data bias, during the data cleaning and preprocessing stages, outliers were removed and missing values were imputed to ensure data consistency and accuracy. In the data analysis stage, various statistical methods were employed to verify the results, ensuring their robustness and consistency. The survey data encompassed six prefecture-level cities and 24 villages in Jiangsu Province. Based on the actual needs of the study, information on household characteristics, individual characteristics, and the implementation of pro-environmental behaviors was extracted from the raw data. After processing, a total of 923 valid questionnaires were obtained.
The dependent variable selected in this study is farmers' PEB. Farmers' PEB can be understood as the activities carried out by farmers in agricultural production to protect the agricultural environment, such as reducing expenses and the use of plastic film (Bell et al., 2016). As the agricultural production process consists of multiple stages, including pre-production, production, and post-production stages, and considering the diversity of agricultural production stages and the limitations of data, we referred to the studies by Zhou et al. (2019, 2020) and selected five specific behaviors for analysis. These behaviors include the use of soil testing and fertilization technology (pre-production), the use of high-efficiency, low-toxicity, and low-residue pesticides (production), the application of commercial organic fertilizers (production), the recycling of agricultural film (post-production), and the recycling of pesticide packaging (post-production). Each behavior is set as a binary variable, with a value of 1 if the farmer adopts the behavior and 0 if not. Finally, the values of these five variables are summed to obtain a composite value measuring the extent of farmers' PEB.
This study selects farmers' digital literacy as an explanatory variable. Based on the previous definition of digital literacy, the ability of farmers to utilize digital devices is a necessary prerequisite. Applying the acquired digital information to the production and management process can be specified as the use of digital finance and digital lifestyle. Following the content of the CLES questionnaire and the studies by Alant and Bakare (2021) and Du et al. (2023), farmers' digital literacy is measured from three dimensions: access to digital technology, use of digital finance, and digital lifestyle. Access to digital technology is represented by the number of smartphones and computers owned. The use of digital finance is represented by the level of understanding of digital credit services and the frequency of digital payments. Digital lifestyle is represented by the extent to which information is obtained online. However, it is important to note that the personal economic ability of farmers may lead to higher values for these indicators, potentially causing bias in the results. To address this issue, the study employs two approaches: first, it filters the data by excluding samples with high economic income and high numbers of smartphones, computers, or digital payments to avoid potential bias. Second, to further control the impact of farmers' economic ability on the measurement of digital literacy, the study includes household income as a control variable in the statistical model to account for potential bias. After non-dimensionalization of these five indicators, the weights of each indicator are obtained using the entropy weighting method. The specific indicators and weights are shown in Table 1.
Based on the previous analysis of the indirect relationship between digital literacy, behavioral intention, and farmer PEB, this study selects the mediating variables of farmer SN, farmer BA, and farmer PBC (Cao et al., 2022). By referring to existing research (Billari et al., 2009; Ajzen and Klobas, 2013), and the definitions of each concept, combined with the content of the questionnaire, farmer subjective norms are measured by “How do you rate the living environment in your village?”; farmer behavioral attitudes are measured by “How do you perceive your own environmental behaviors?”; and farmer perceived behavioral control is measured by “Your attitude toward income growth in the next 1–2 years.” These three items were chosen because villages with better living environments increase the social pressure felt by farmers. As other farmers pay attention to their environmental behaviors and the environment improves, farmers face environmental pressure exerted by their peers. Farmers' perceptions of their own environmental behaviors can fully reflect their self-acceptance of environmentally friendly behaviors. Estimations of future income increases by farmers can reflect their assessment of environmental challenges. If farmers believe that engaging in green behaviors is within their control, they will have an optimistic outlook on the future.
Based on previous studies (Diendéré et al., 2018; Melo et al., 2018), factors such as gender, age, education level, household size, and farm size are known to have an impact on rural PEB. Therefore, this study introduces three levels of control variables to reduce estimation bias. Regarding individual characteristics of farmers, this includes gender, age, educational level, health status, and training received by the household head. Regarding household characteristics, it includes the number of household members, household income, level of household diversification, and whether the household is a member of the Communist Party. Regarding land characteristics, it includes land size, land tenure status, and land fertility. The specific content is shown in Table 2.
The dependent variable in this study is discrete, and standard regression models cannot be used for empirical analysis. Therefore, according to econometric textbooks (Wooldridge et al., 2016), this study employs an Ordered Probit model to examine the impact of digital literacy on farmers' PEB. The model expression is as follows:
In Equation (1), PEB is the dependent variable, DL is the core explanatory variable, Xi represents a series of control variables that may affect the dependent variable, including individual characteristics of entrepreneurs, family characteristics, and land characteristics. εi is the random error term, α0 is the constant term, and α1 and α2 are the parameters to be estimated. Assuming ε ~ N (0, 1) distribution, the Ordered Probit model can be expressed as:
In Equation (2), r0 < r1 < r2 < r3 < r4 are the parameters to be estimated; PEB takes values ranging from 0 to 5, representing “not implemented” to “implemented 5 types of PEB” by farmers, where φ is the cumulative density function of the standard normal distribution. By constructing the likelihood function for each farmer's PEB, maximum likelihood estimation is then used to estimate the model parameters.
This study proposes that digital literacy may promote farmers' PEB through their SN, BA, and PBC. To verify the potential mediating mechanisms, drawing from the mediation effect testing method proposed by Jiang (2022), linear regression equations are constructed for the relationships between digital literacy and the intermediate variables SN, BA, and PBC. Where β0, γ0, δ0 are constants, β1, γ1, δ1, β2, γ2, δ2 represent their estimated coefficients. The specific testing models are as follows:
Before conducting the baseline regression, it is necessary to consider the issue of multicollinearity among variables. This study performed a collinearity diagnosis, and the results indicate that the maximum variance inflation factor (VIF) is 2.22 (< 10), suggesting that there is no severe multicollinearity issue among variables. The selection of explanatory variables is therefore deemed reasonable.
Table 3 presents the estimation results of the baseline Model (1) for the impact of digital literacy on farmers' PEB. Model (1-1) examines the direct effect of digital literacy on farmers' PEB. The results indicate a significant positive impact of digital literacy on farmers' PEB at the 1% level of significance. Controlling for individual, household, and land characteristics, the results of Model (1-2) show that digital literacy continues to have a positive impact on farmers' PEB, with a coefficient of 1.342, significant at the 1% level. This indicates that farmers with higher digital literacy are more likely to implement PEB. One possible explanation is that compared to farmers with lower digital literacy, those with higher digital literacy can access, communicate, and apply information through digital means, thereby enhancing the benefits of implementing PEB and reducing associated costs. Under the assumption of rationality, farmers adjust their agricultural production behaviors and decisions, thus promoting the implementation of PEB. Additionally, factors such as gender, education, health, and household income also influence farmers' PEB. Specifically, female farmers, those with higher levels of education, better health, and higher incomes are more likely to engage in PEB. Women may have higher levels of empathy and social responsibility, which can translate into a greater concern for environmental issues and a stronger commitment to PEB. Higher education levels typically lead to a better understanding of environmental issues and sustainable practices, enabling educated farmers to access and comprehend information about PEB. Good health allows farmers to participate more actively in labor-intensive PEB activities, as healthier farmers have the physical capacity to implement and maintain sustainable practices. Additionally, higher household income provides farmers with the financial resources to purchase the necessary tools and technologies for PEB and offers greater economic security, enabling them to bear potential short-term costs for long-term environmental benefits. Based on this, research hypothesis H1 is confirmed.
Given that farmers' PEB involves multiple processes in pre-production, production, and post-production, each stage of production behavior varies, and the costs and benefits of adopting PEB also differ. Therefore, after clarifying the ability of digital literacy to enhance farmers' PEB, this study further analyzes the impact of digital literacy on each type of PEB. The results in Model (1-4), (1-6), and (1-7) of Table 3 indicate that the improvement in digital literacy can promote farmers to use low-toxic and low-residue pesticides, recycle agricultural film, and recycle pesticide packaging. These three types of PEB are, respectively, in the production and post-production stages. On the one hand, using low-toxic and low-residue pesticides has low costs and high returns, which can directly bring economic income to farmers. On the other hand, post-production non-green behaviors may not only reduce agricultural output for the next year but also easily attract scrutiny from regulatory authorities, leading to penalties. Therefore, the positive impact of digital literacy on the above three types of PEB is significant. The results in Model (1-3) and (1-5) indicate that although the impact of digital literacy on soil testing and the use of commercial organic fertilizers is not significant, the relationship remains positive.
In fact, this study may also face potential endogeneity issues such as reverse causality or omitted variables, which could affect the reliability of the research findings. Specifically, on one hand, in the process where digital literacy enhancement influences PEB, the implementation of PEB may indirectly influence farmers, thereby enhancing their digital literacy. To address the endogeneity issues arising from the mutual causality, it is essential to employ appropriate econometric techniques. On the other hand, despite controlling for some variables based on data availability, controlling for all variables remains challenging, leading to potential omitted variable bias in the baseline model.
To overcome the potential endogeneity issues mentioned above, this study adopts the Conditional Mixed Process (CMP) estimation method. This method requires simultaneous estimation of two equations: the first equation estimates the impact of instrumental variables on digital literacy, while the second equation estimates the impact of digital literacy on farmers' PEB. By referring to the endogeneity test parameter Atanhrho-12 to discern the exogeneity of variables, if the parameter significantly differs from zero, it indicates the presence of endogeneity issues, and in this case, CMP estimation results are more accurate (Roodman, 2011). Regarding the selection of instrumental variables, this study follows the approach of Su and Peng (2022), choosing “the average digital literacy of other sampled households in the same village as the interviewed farmers” as the instrumental variable for digital literacy. The selection of this instrumental variable is primarily based on two points: firstly, in terms of correlation, when other households in the same village have higher digital literacy, the interviewed respondents, driven by the herd mentality, tend to imitate and learn from others, thereby improving their own digital literacy. Secondly, in terms of exogeneity, the digital literacy of other households in the village has no direct relationship with the PEB of the interviewed households. Therefore, the selection of instrumental variables meets the requirements of correlation and exogeneity, and then constructs a model to test them.
From Table 4, Model (2-1) indicates that the average digital literacy of other sampled households in the same village as the interviewed farmers exhibits statistical significance at the 1% level, with a positive effect on digital literacy. Moreover, the F-value exceeds 10, indicating the absence of a weak instrumental variable problem. The results of Model (2-2) regression show that, after controlling for potential endogeneity bias, digital literacy significantly and positively influences farmers' PEB at the 1% significance level. This conclusion aligns with the baseline regression findings. Additionally, the endogeneity test parameter atanhrho-12 based on the CMP method is statistically significant at the 1% level, indicating the presence of endogeneity issues in the baseline regression. Therefore, the conclusions drawn from the CMP method are more robust.
Based on the theoretical analysis presented earlier, digital literacy can enhance farmers' SN, strengthen their BA, and improve their PBC, thereby increasing their willingness to engage in PEB. To verify the indirect impact of digital literacy on PEB, this study uses the constructed models (3), (4), and (5) to test the mediation effect. The specific results are shown in Table 5. Model (3-1) indicates that the coefficient of digital literacy on farmers' SN is significantly positive at the 1% statistical level, verifying hypothesis H2a. This may be explained by the fact that farmers with higher levels of digital literacy can expand their sources of information, perceive the PEB of other farmers, and, under the influence of group effects and public opinion pressure, feel a heightened sense of moral responsibility to implement PEB themselves. The results of Model (3-2) demonstrate that digital literacy significantly positively influences farmers' BA at the 1% statistical level, with a coefficient of 0.448, validating hypothesis H2b. This promotion effect can be understood from two perspectives: On one hand, with the improvement of farmers' digital literacy, they can more easily access relevant information about green production, thus enhancing their environmental awareness. On the other hand, farmers with high digital literacy are more likely to learn about the premium prices of green agricultural products in the market through the internet, and they are more willing to engage in PEB in the context of increased profit expectations. Model (3-3) shows that digital literacy significantly positively influences farmers' PBC at the 1% statistical level, confirming hypothesis H2c. The possible explanation is that farmers with high levels of digital literacy can acquire the resources needed for PEB, such as funds, equipment, and technology, through digital means. The support of resources facilitates farmers' transition from the willingness to engage in PEB to actual implementation.
This study employs a grouped regression approach, Model, to investigate the influence of digital literacy on PEB among rural households with different levels of education, social capital, and household income. The specific estimation results are presented in Table 6.
Firstly, Digital literacy and PEB among rural households: Heterogeneity based on the level of education. Typically, the educational level of rural households can be categorized as high if it is at or above junior high school, and low if it is below junior high school. The average education level of the surveyed households in this study is 7.2 years, which corresponds to a junior high school education level. Based on this, the study divides households into two categories: high education level and low education level. Model (4-1) and (4-2), respectively, represent the impact of digital literacy on PEB among households with high and low education levels. The coefficients of digital literacy on PEB for high education level households and low education level households are 0.856 and 0.539, respectively. The results indicate that the marginal effect of improving digital literacy on PEB is more significant for households with higher education levels. This suggests that households with higher education levels have greater cognitive and learning advantages, enabling them to transform digital literacy into productive capacity more quickly and thereby promoting the implementation of PEB.
Secondly, Digital literacy and PEB among rural households: Heterogeneity based on social capital. In this study, “How much money do you typically give as a gift when attending weddings or other events for relatives or friends?” was used to measure the social capital of households. The average amount of money given as gifts by surveyed households was used as the standard to divide households into high and low social capital groups. Model (4-3) and (4-4) report the regression results for these two groups of households. The impact of digital literacy on PEB is significantly higher for households with high social capital compared to those with low social capital. This result may suggest that social capital provides households with abundant social resources, making it easier for them to access and learn digital knowledge, improve digital literacy, and thus become more willing to implement PEB.
Thirdly, Digital literacy and PEB among rural households: Heterogeneity based on household income. Using the average household income as the benchmark, households were divided into high-income and low-income groups. Model (4-5) and (4-6) demonstrate that digital literacy has a significant positive impact on PEB for both groups of households, but the magnitude of the impact differs. For the high-income group, the coefficient of digital literacy on PEB is 1.161, higher than the coefficient of 1.148 for the low-income group. This outcome may suggest that while implementing PEB requires financial support and involves certain risks, households with higher income levels face weaker financial constraints and have relatively higher risk-taking capabilities. Conversely, households with lower incomes require more financial support and tend to exhibit higher risk-averse tendencies. Therefore, the promoting effect of digital literacy on PEB is more pronounced among high-income households. Based on the aforementioned analysis, hypothesis H3 is confirmed.
To further ensure the reliability of the estimation results, this study conducted robustness tests on the main effect model from three aspects: changing the method of constructing explanatory variables, reducing the sample size, and altering the model. The results are shown in Table 7.
First, the robustness test of changing the index construction method. This study, based on the established indicator system, used principal component factor analysis to calculate the index of digital literacy for farmers. The Kaiser-Meyer-Olkin value of the relevant items was 0.641, exceeding 0.6, indicating the suitability of using principal component factor analysis. Based on the principle of retaining two main component factors with eigenvalues ≥1 to represent digital literacy, the index of digital literacy for farmers was derived from the factor loadings of each item, followed by regression analysis. Model (5-1) presents the regression results of the index of digital literacy derived from principal component analysis on farmers' PEB. The results show that digital literacy still positively influences farmers' PEB, consistent with the previous findings.
Second, the robustness test of excluding some samples. Compared to younger individuals, older farmers have weaker abilities in using digital technology and are less proactive in subjectively accepting external information, resulting in a weaker correlation between their PEB and digital literacy. Therefore, this study excluded some elderly individuals to verify the reliability of the estimation results. The World Health Organization defines individuals aged 60 and above as elderly people, among whom those aged 60–74 belong to the younger elderly category and still possess some learning capabilities. Therefore, this study excluded samples of farmers aged 74 and above, as shown in Model (5-2). The results still indicate that digital literacy influences farmers' PEB, suggesting that higher digital literacy scores are associated with a greater likelihood of implementing PEB, consistent with the previous findings.
Third, the robustness test using Probit models. The Probit method is generally suitable for cases where the dependent variable is a binary variable. Accordingly, this study divided farmers into two categories based on the extent of their PEB: the “low PEB” sample group consisted of farmers adopting 0–2 types of PEB, while the “high PEB” sample group consisted of farmers adopting 3–5 types of PEB. The regression results, as shown in Model (5-3), indicate that digital literacy significantly promotes farmers' PEB. The regression results are generally consistent with the previous findings, demonstrating good robustness.
Compared with existing studies, this paper focuses on Chinese farmers and incorporates digital literacy into the analytical framework of farmers' PEB. It establishes an evaluation index system for digital literacy and measures related variables to analyze the impact of digital literacy on farmers' PEB, thereby expanding the research on the factors influencing farmers' PEB. The study confirms the positive impact of digital literacy on farmers' PEB, indicating that digital literacy plays a significant facilitating role in farmers' implementation of PEB. Enhancing farmers' digital literacy can effectively improve the PEB in their production and management activities. This conclusion is consistent with existing literature (Huang et al., 2022; Du et al., 2023). Additionally, based on the TPB, this study incorporates behavioral intention into the analysis of farmers' PEB and quantifies it through subjective norms, behavioral attitudes, and perceived behavioral control. It examines the indirect effects of digital literacy on farmers' PEB, expanding the application of TPB in green agricultural production. The results confirm the mediating roles of subjective norms, behavioral attitudes, and perceived behavioral control in the relationship between digital literacy and farmers' PEB. This indicates that farmers' digital literacy triggers their intention to implement PEB, which eventually leads to the actual behavior, confirming that behavioral intention is a crucial driver of farmers' PEB. Specifically, this intention is influenced by farmers' subjective norms, behavioral attitudes, and perceived behavioral control, which serve as mediators in the positive impact of digital literacy on farmers' PEB. Furthermore, this study examines the roles of farmers' education levels, social capital, and household income in the process of digital literacy influencing PEB, considering the differences in personal and household characteristics among farmers. The results show that farmers with higher education levels, greater social capital, and higher household income are more likely to engage in PEB under the influence of digital literacy. These findings highlight the roles of behavioral intention and certain individual and household characteristics in the process of digital literacy impacting farmers' PEB, addressing gaps in existing research.
This study examines the relationship between farmers' digital literacy and PEB, confirming the positive impact of digital literacy on farmers' PEB. It emphasizes the mediating roles of subjective norms, behavioral attitudes, and perceived behavioral control in the relationship between digital literacy and farmers' PEB. Additionally, the study reveals the significant roles of farmers' education levels, social capital, and household income. The higher the education level, the stronger the social capital, and the higher the household income, the greater the impact of digital literacy on farmers' PEB.
The above research conclusions provide important insights for policymakers to promote farmers' environmental protection practices. On the one hand, the role of digital literacy in enhancing farmers' PEB should be recognized. The government should strengthen the construction of digital infrastructure and digital skills training in rural areas, ensuring that farmers can access and proficiently use digital devices and internet resources. By organizing training courses and providing online learning platforms, the digital literacy of farmers can be improved, thereby promoting their PEB. On the other hand, the influence of digital literacy on farmers' subjective norms, behavioral attitudes, and perceived behavioral control should be fully utilized to enhance farmers' willingness to engage in PEB. This will promote the implementation of PEB among farmers and reduce the environmental damage caused by agricultural production and management activities. Additionally, since digital literacy is more beneficial for farmers with higher education levels, social capital, and household income in implementing PEB, the government should invest in improving farmers' educational levels to enhance their knowledge. It should also encourage cooperation and communication among farmers to strengthen their social capital, and formulate policies that support rural economic development to increase farmers' income levels. These combined measures will enhance the effect of digital literacy on farmers' PEB.
However, due to various limitations, this study inevitably has certain constraints that warrant further investigation. First, the data in this study were only collected from Jiangsu Province. Farmers' personal, family, and agricultural production conditions may vary across different countries and regions. Future research should consider this issue more comprehensively by conducting surveys in broader areas of other countries to provide a more holistic view of farmers' PEB. Second, the questionnaire survey is based on cross-sectional data, which cannot reveal the temporal effects of digital literacy on farmers' PEB. Future studies could enhance the reliability of the findings by extending the data collection period and employing panel data. Additionally, this study is limited by the questionnaire content; the measurement of farmers' PEB is not sufficiently diverse, as it only includes five representative behaviors. Future research could consider incorporating behaviors such as soil management and pest, disease, and weed control to more fully capture the implementation of farmers' PEB.
The raw data supporting the conclusions of this article will be made available by the authors, without undue reservation.
SL: Conceptualization, Project administration, Software, Supervision, Visualization, Writing – original draft. ZS: Data curation, Investigation, Supervision, Writing – review & editing. MH: Funding acquisition, Project administration, Resources, Validation, Writing – review & editing.
The author(s) declare that no financial support was received for the research, authorship, and/or publication of this article.
The authors declare that the research was conducted in the absence of any commercial or financial relationships that could be construed as a potential conflict of interest.
All claims expressed in this article are solely those of the authors and do not necessarily represent those of their affiliated organizations, or those of the publisher, the editors and the reviewers. Any product that may be evaluated in this article, or claim that may be made by its manufacturer, is not guaranteed or endorsed by the publisher.
The Supplementary Material for this article can be found online at: https://www.frontiersin.org/articles/10.3389/fsufs.2024.1432184/full#supplementary-material
1. ^Data Source: FAO Database (https://www.fao.org/faostat/en/#home).
Acikgoz, F., Elwalda, A., and De Oliveira, M. J. (2023). Curiosity on cutting-edge technology via theory of planned behavior and diffusion of innovation theory. Int. J. Inf. Manag. Data Insights. 3:100152. doi: 10.1016/j.jjimei.2022.100152
Ajzen, I. (1991). The theory of planned behavior. Organ. Behav. Hum. Decis. Process. 50, 179–211. doi: 10.1016/0749-5978(91)90020-T
Ajzen, I., and Klobas, J. (2013). Fertility intentions: an approach based on the theory of planned behavior. Demogr. Res. 29, 203–232. doi: 10.4054/DemRes.2013.29.8
Alant, B. P., and Bakare, O. O. (2021). A case study of the relationship between smallholder farmers' ICT literacy levels and demographic data wrt their use and adoption of ICT for weather forecasting. Heliyon 7:e06403. doi: 10.1016/j.heliyon.2021.e06403
Ali, D., Bowen, D., and Deininger, K. (2020). Personality traits, technology adoption, and technical efficiency: evidence from smallholder rice farms in Ghana. J. Dev. Stud. 56, 1330–1348. doi: 10.1080/00220388.2019.1666978
Bai, Q., Chen, H., Zhou, J., Li, G., Zang, D., Sow, Y., et al. (2023). Digital literacy and farmers' entrepreneurial behavior—Empirical analysis based on CHFS2019 micro data. PLoS ONE 18:e0288245. doi: 10.1371/journal.pone.0288245
Bakker, L., Sok, J., van der Werf, W., and Bianchi, F. J. J. A. (2021). Kicking the habit: what makes and breaks farmers' intentions to reduce pesticide use? Ecol. Econ. 180:106868. doi: 10.1016/j.ecolecon.2020.106868
Bell, A., Parkhurst, G., Droppelmann, K., and Benton, T. G. (2016). Scaling up pro-environmental agricultural practice using agglomeration payments: proof of concept from an agent-based model. Ecol. Econ. 126, 32–41. doi: 10.1016/j.ecolecon.2016.03.002
Billari, F. C., Philipov, D., and Testa, M. R. (2009). Attitudes, norms and perceived behavioural control: explaining fertility intentions in Bulgaria. Eur. J. Popul. 25, 439–465. doi: 10.1007/s10680-009-9187-9
Botetzagias, I., Dima, A., and Malesios, C. (2015). Extending the Theory of Planned Behavior in the context of recycling: the role of moral norms and of demographic predictors. Resour. Conserv. Recycl. 95, 58–67. doi: 10.1016/j.resconrec.2014.12.004
Byrne, S., and O'Regan, B. (2014). Attitudes and actions towards recycling behaviours in the Limerick, Ireland region. Resour. Conserv. Recycl. 87, 89–96. doi: 10.1016/j.resconrec.2014.03.001
Cao, H., Li, F., Zhao, K., Qian, C., and Xiang, T. (2022). From value perception to behavioural intention: study of Chinese smallholders' pro-environmental agricultural practices. J. Environ. Manage. 315:115179. doi: 10.1016/j.jenvman.2022.115179
Cao, H., Zhu, X. Q., Heijman, W., and Zhao, K. (2020). The impact of land transfer and Farmers'Knowledge of farmland protection policy on pro-environmental agricultural practices: the case of straw return to fields in ningxia, China. J. Clean. Prod. 277:123701. doi: 10.1016/j.jclepro.2020.123701
Cheng, J. C. H., and Monroe, M. C. (2012). Connection to nature: children's affective attitude toward nature. Environ. Behav. 44, 31–49. doi: 10.1177/0013916510385082
Crippa, M., Solazzo, E., Guizzardi, D., Monforti-Ferrario, F., Tubiello, F. N., and Leip, A. J. N. F. (2021). Food systems are responsible for a third of global anthropogenic GHG emissions. Nat. Food 2, 198–209. doi: 10.1038/s43016-021-00225-9
Diendéré, A., Nguyen, G., Del Corso, J. P., and Kephaliacos, C. (2018). Modeling the relationship between pesticide use and farmers' beliefs about water pollution in Burkina Faso. Ecol. Econ. 151, 114–121. doi: 10.1016/j.ecolecon.2018.05.002
Dowlatshahi, S. (2010). A cost-benefit analysis for the design and implementation of reverse logistics systems: case studies approach. Int. J. Prod. Resour. 48, 1361–1380. doi: 10.1080/00207540802552642
Du, F., Zhao, X., Zheng, J., and Zhang, M. (2023). Can digital literacy promote green production for farmers?—Based on CLES data. World Agric. 10, 97–109. doi: 10.13856/j.cn11-1097/s.2023.10.009
Fu, X., Ueland, S. M., and Olivetti, E. (2017). Econometric modeling of recycled copper supply. Resour. Conserv. Recycl. 122, 219–226. doi: 10.1016/j.resconrec.2017.02.012
Gong, S., Sun, Z., Wang, B., and Yu, Z. (2024). Could digital literacy contribute to the improvement of green production efficiency in agriculture? SAGE Open 14:21582440241232789. doi: 10.1177/21582440241232789
Guo, L., and Zhao, J. (2014). Farmers pro-environmental behavior modeling and interventions policy in the case of the straw processing behavior. Agric. Econ. Iss. 12, 78–84+112. doi: 10.13246/j.cnki.iae.2014.12.010
Halvorsen, B. (2012). Effects of norms and policy incentives on household recycling: an international comparison. Resour. Conserv. Recycl. 67, 18–26. doi: 10.1016/j.resconrec.2012.06.008
Hargittai, E. (2005). Survey measures of web-oriented digital literacy. Soc. Sci. Comput. Rev. 23, 371–379. doi: 10.1177/0894439305275911
Hattie, J., Hodis, F. A., and Kang, S. H. (2020). Theories of motivation: integration and ways forward. Contemp. Educ. Psychol. 61:101865. doi: 10.1016/j.cedpsych.2020.101865
Huang, X., Yang, F., and Fahad, S. (2022). The impact of digital technology use on farmers' low-carbon production behavior under the background of carbon emission peak and carbon neutrality goals. Front. Environ. Sci. 10:1002181. doi: 10.3389/fenvs.2022.1002181
Huang, Y., Luo, X., Li, R., and Zhang, J. (2018). Farmer cognition, external environment and willingness of green agriculture production—Based on the survey data of 632 farmers in Hubei Province. Resour. Environ. Yangtze Basin 3, 680–687. doi: 10.11870/cjlyzyyhj201803024
Jiang, T. (2022). Mediating effects and moderating effects in causal inference. China Ind. Econ. 5, 100–120. doi: 10.19581/j.cnki.ciejournal.2022.05.005
Kansiime, M. K., Alawy, A., Allen, C., Subharwal, M., and Jadhav, A. P. M. (2019). Effectiveness of mobile agri-advisory service extension model: evidence from Direct2Farm program in India. World Dev. Perspect. 13, 25–33. doi: 10.1016/j.wdp.2019.02.007
Khataza, R. R. B., Doole, G. J., Kragt, M. E., and Hailu, A. (2018). Information acquisition, learning and the adoption of conservation agriculture in Malawi: a discrete-time duration analysis. Technol. Forecast. Soc. Change. 132, 299–307. doi: 10.1016/j.techfore.2018.02.015
Klöckner, C. A. (2013). A comprehensive model of the psychology of environmental behaviour—a meta-analysis. Global Environ. Change 23, 1028–1038. doi: 10.1016/j.gloenvcha.2013.05.014
Lakhan, C. (2015). Differences in self reported recycling behavior of first and second generation South Asians in Ontario, Canada. Resour. Conserv. Recycl. 97, 31–43. doi: 10.1016/j.resconrec.2015.02.006
Li, W., and Zhou, S. (2023). Reform of agricultural production mode in China under the background of digital economy: mechanism, contradiction and solution. J. Xi'an Jiaotong Univ. 1, 65–73. doi: 10.15896/j.xjtuskxb.202301007
Liu, Z., Qi, Z., Yang, C., and Chen, X. (2021). Effects of network embeddedness and reputation motivation on farmers'pro-environment behavior. Resour. Environ. Yangtze Basin 8, 1982–1991. doi: 10.11870/cjlyzyyhj202108019
Marr, E. J., and Howley, P. (2019). The accidental environmentalists: factors affecting farmers' adoption of pro-environmental activities in England and Ontario. J. Rural Study. 68, 100–111. doi: 10.1016/j.jrurstud.2019.01.013
Melo, P. C., Ge, J., Craig, T., Brewer, M. J., and Thronicker, I. (2018). Does work-life balance affect pro-environmental behaviour? Evidence for the UK using longitudinal microdata. Ecol. Econ. 145, 170–181. doi: 10.1016/j.ecolecon.2017.09.006
Mi, Q., Li, X. D., and Gao, J. Z. (2020). How to improve the welfare of smallholders through agricultural production outsourcing: evidence from cotton farmers in xinjiang, northwest China. J. Clean. Prod. 256:120636. doi: 10.1016/j.jclepro.2020.120636
Molinillo, S., and Japutra, A. (2017). Organizational adoption of digital information and technology: a theoretical review. Bottom Line 30, 33–46. doi: 10.1108/BL-01-2017-0002
Neumeyer, X., Santos, S. C., and Morris, M. H. (2020). Overcoming barriers to technology adoption when fostering entrepreneurship among the poor: The role of technology and digital literacy. IEEE Transactions on Engineering Management. 68, 1605–1618. doi: 10.1109/TEM.2020.2989740
Price, J. C., and Leviston, Z. (2014). Predicting pro-environmental agricultural practices: the social, psychological and contextual influences on land management. J. Rural Stud. 34, 65–78. doi: 10.1016/j.jrurstud.2013.10.001
Roodman, D. (2011). Fitting fully observed recursive mixed-process models with cmp. Stata J. 11, 159–206. doi: 10.1177/1536867X1101100202
Shi, Z., Jin, R., and Mu, H. (2018). Research on farmers'pro-environment behavior and influencing factors from the perspective of information cultivation theory—survey and analysis of 542 farmer households in 19 counties of Gansu Province. West. For. 2, 17–25+49. doi: 10.3969/j.issn.1674.8131.2018.02.003
Song, M., Wang, S., and Zhang, H. (2020). Could environmental regulation and RD tax incentives affect green product innovation? J. Clean. Prod. 258:120849. doi: 10.1016/j.jclepro.2020.120849
Su, L., and Peng, Y. (2022). Farmers' digital literacy, elite identity, and participation in rural digital governance. Agric. Technol. Econ. 1, 34–50. doi: 10.13246/j.cnki.jae.2022.01.004
Tubiello, F. N., Rosenzweig, C., Conchedda, G., Karl, K., Gütschow, J., Xueyao, P., et al. (2021). Greenhouse gas emissions from food systems: building the evidence base. Environ. Res. Lett. 16:065007. doi: 10.1088/1748-9326/ac018e
Wang, C., Tong, Q., Xia, C., Shi, M., and Cai, Y. (2024). Does participation in e-commerce affect fruit farmers' awareness of green production: Evidence from China. J. Environ. Plann. Manag. 67, 809–829. doi: 10.1080/09640568.2022.2133686
Wang, L., Wang, Y., Zhang, H., and Bai, X G. (2023). A study on the influence of farmers' information literacy on green production efficiency: threshold effect and its mechanism. Res. Agric. Modern. 44, 1047–1058. doi: 10.13872/j.1000-0275.2023.0092
Wang, Y., He, K., Zhang, J., and Chang, H. (2020). Environmental knowledge, risk attitude, and households' willingness to accept compensation for the application of degradable agricultural mulch film: evidence from rural China. Sci. Total Environ. 744:140616. doi: 10.1016/j.scitotenv.2020.140616
Wooldridge, J. M., Wadud, M., and Lye, J. (2016). Introductory econometrics: Asia pacific edition with online study tools 12 months. Cengage Australia.
Yang, C., Liang, X., Xue, Y., Zhang, Y., and Xue, Y. (2024). Can government regulation weak the gap between green production intention and behavior? Based on the perspective of farmers' perceptions. J. Cleaner Prod. 434:139743. doi: 10.1016/j.jclepro.2023.139743
Yu, L., Chen, C., Niu, Z., Gao, Y., Yang, H., and Xue, Z. (2021). Risk aversion, cooperative membership and the adoption of green control techniques: evidence from China. J. Clean. Prod. 279:123288. doi: 10.1016/j.jclepro.2020.123288
Yu, L., Liu, W., Yang, S., Kong, R., and He, X. (2022). Impact of environmental literacy on farmers' agricultural green production behavior: Evidence from rural China. Front. Environ. Sci. 10:990981. doi: 10.3389/fenvs.2022.990981
Yu, L., Zhao, D., Xue, Z., and Gao, Y. (2020). Research on the use of digital finance and the adoption of green control techniques by family farms in China. Technol. Soc. 62:101323. doi: 10.1016/j.techsoc.2020.101323
Yu, W., Luo, X., Li, R., Xue, L., and Huang, L. (2017). The paradox between farmer willingness and their adoption of green technology from the perspective of green cognition. Resour. Sci. 39, 1573–1583. doi: 10.18402/resci.2017.08.13
Zeng, J. (2023). The impact of different uses of the internet on professional farmers' adoption of green technology for preventing and controlling diseases and pests: based on 2544 farmers nationwide. J. Hunan Agric. Univ. 3, 35–44. doi: 10.13331/j.cnki.jhau(ss).2023.03.005
Zhang, S., Zhang, M., Yu, X., and Ren, H. (2016). What keeps Chinese from recycling: accessibility of recycling facilities and the behavior. Resour. Conserv. Recycl. 109, 176–186. doi: 10.1016/j.resconrec.2016.02.008
Zhou, J. H., Yang, Z. Y., Li, K., and Yu, X. H. (2019). Direct intervention or indirect support? The effects of cooperative control measures on farmers'implementation of quality and safety standards. Food Policy 86:101728. doi: 10.1016/j.foodpol.2019.05.011
Keywords: digital literacy, pro-environmental behavior, Theory of Planned Behavior, Ordered Probit modeling approach, agricultural sustainability
Citation: Lu S, Sun Z and Huang M (2024) The impact of digital literacy on farmers' pro-environmental behavior: an analysis with the Theory of Planned Behavior. Front. Sustain. Food Syst. 8:1432184. doi: 10.3389/fsufs.2024.1432184
Received: 16 May 2024; Accepted: 24 June 2024;
Published: 15 July 2024.
Edited by:
Isabelle Piot-Lepetit, INRAE Occitanie Montpellier, FranceReviewed by:
Yiping Zhong, Hunan Normal University, ChinaCopyright © 2024 Lu, Sun and Huang. This is an open-access article distributed under the terms of the Creative Commons Attribution License (CC BY). The use, distribution or reproduction in other forums is permitted, provided the original author(s) and the copyright owner(s) are credited and that the original publication in this journal is cited, in accordance with accepted academic practice. No use, distribution or reproduction is permitted which does not comply with these terms.
*Correspondence: Man Huang, aG1zZ2RkaHI2NjZAMTYzLmNvbQ==
Disclaimer: All claims expressed in this article are solely those of the authors and do not necessarily represent those of their affiliated organizations, or those of the publisher, the editors and the reviewers. Any product that may be evaluated in this article or claim that may be made by its manufacturer is not guaranteed or endorsed by the publisher.
Research integrity at Frontiers
Learn more about the work of our research integrity team to safeguard the quality of each article we publish.