- College of Economics and Management, Nanjing Forestry University, Nanjing, China
Increasing farmers’ grain profits is crucial for enhancing the sustainability of grain production and ensuring food security. As a widely applied agricultural management means at present, agricultural productive services are of great significance to study whether they can help increase farmers’ grain profits. This paper uses the micro-farmer data from the 2020 China Rural Revitalization Survey (CRRS). It employs an endogenous switching regression model and causal mediation analysis methods, which can address endogenous issues, to examine the impact of agricultural productive services on farmers’ grain profits and their underlying mechanisms. The results show: (1) Agricultural productive services significantly increase farmers’ grain profits and this conclusion is still robust after the robustness test. (2) The mechanism analysis indicates that agricultural productive services increase farmers’ grain profits through three pathways: yield enhancement, cost reduction, and efficiency improvement. (3) The heterogeneity analysis shows that agricultural productive services have a greater impact on increasing grain profits for farmers with lower grain profits, smaller operating scales, and lower degrees of farmland fragmentation. Our findings suggest that increasing support for agricultural productive services, fully leveraging the radiative driving role of service providers, and offering farmers various forms of productive services will be key measures to enhance farmers’ grain profits, promote sustainable grain production, and ensure food security.
1 Introduction
Food security is a prerequisite for a nation’s stable development and a key issue of high concern for the international community (Laborde et al., 2020). The key to ensuring food security lies in enhancing farmers’ enthusiasm and stability in grain cultivation to ensure the sustainability of grain production. To ensure the sustainability of grain production, farmers must be able to earn sufficient profits from grain cultivation (Zhang X. et al., 2023). However, in many developing countries like China, the economic benefits of grain cultivation for farmers remain low, significantly weakening their enthusiasm and hindering the sustainable production of grain. In 2021, the average income per mu of grain in China was 824 yuan, the highest since 2017. However, it remains significantly lower than the income per mu from cash crops and far below the 2 to 3 months’ wages that farmers can earn from migrant work (Rahman and Mishra, 2020). To ensure that farmers benefit from grain production, China has implemented a series of pro-agriculture policies, including the minimum grain purchase price policy and temporary storage policies (Qian et al., 2020). These measures have effectively increased farmer benefits of grain production. However, these measures have not fundamentally solved the issue of low profits from grain cultivation for farmers (Li and Jiang, 2023). In this context, how to improve farmers’ profits from grain cultivation while maintaining stable food prices has become a pressing issue for many developing countries.
Agricultural production services (APS), as a form of agricultural socialized services that spans the entire agricultural production operation chain, are carried out directly or in collaboration by entities such as family farms, large-scale professional farms, farmer cooperatives, leading enterprises, and specialized service companies (Lu et al., 2022; Shi et al., 2024). These services encompass pre-production, production, and post-production activities including procurement of agricultural inputs, land preparation, fertilization, pest control, irrigation, and harvesting. They promote specialization and deepen division of labor in agricultural production (Gong, 2020). Given the realistic backdrop where a large number of farmers have transferred to cities to engage in non-agricultural work with higher income, and the aging and weakening of agricultural production have become more and more serious, APS is regarded as an effective means to solve the era’s hidden concern of “who will farm and how to farm” (Zhang et al., 2018; Xie et al., 2023). Most developing countries characterized by small-scale household operations have long faced challenges such as constraints in resource endowment, difficulty in accessing markets, and compounded risks (Ren et al., 2023). Farmers can obtain APS through purchases to alleviate various constraints in agricultural production (Qian et al., 2022). International agricultural practices demonstrate that APS play a crucial role in providing technical support, agricultural machinery and equipment, risk diversification, market information, and optimizing input factors (Peng et al., 2022; Ai et al., 2023; Na et al., 2024). In recent years, APS have experienced rapid development driven by national policy support and farmer demand. By 2023, China had 1.041 million agricultural socialized service organizations, covering service areas totaling nearly 1.87 billion times, benefiting over 89 million small farmers and making significant contributions to agricultural production in China (Yang et al., 2023). In light of this, does APS contribute to increasing farmers’ grain profits and thereby ensuring the sustainability of grain production? Exploring this issue is of significant practical importance for improving the APS system and ensuring food security. Across existing research, discussions on agricultural productive services and grain production mainly focus on the following two aspects: Firstly, exploring the factors influencing farmers’ adoption of APS. Through empirical research, some scholars have discovered that factors such as the quantity of household labor, the proportion of grain cultivation, farmland tenure, government support, as well as non-farm employment opportunities and income, all play a role in influencing farmers’ decisions on the adoption of APS (Cai and Wang, 2021; Xu C. et al., 2022; Li and Guo, 2023). Qiu and Luo (2021) found that in the realistic situation where a large amount of labor force is flowing out, the cost of hired workers is constantly rising, and agricultural machinery assets are highly specialized and costly, adopting APS has become a rational choice for most farmers. However, in many developing countries, due to inadequate road infrastructure, severe fragmentation of farmland and incomplete service markets, opportunities for small farmers to access agricultural production services are limited (Xu M. et al., 2024). Some scholars found that land fragmentation not only reduces the possibility of using APS efficiently, but also increases the service cost and reduces the service effect (Niroula and Thapa, 2005; Lu et al., 2019). Furthermore, Wei and Tang (2022) have found that uncertainties in the quality of agricultural production services operations, coupled with risks from uncertain operational timing due to the urgency of agricultural tasks, have increased APS outsourcing costs and decreased APS outsourcing benefits, resulting in a contraction of the APS service market. Secondly, the analysis of the impact of APS on grain production behavior and benefits. In light of the reality of farmer differentiation and the aging of agricultural production, some scholars have explored the effect of APS on the efficiency of grain production (Min et al., 2021; Zhang and Guo, 2021). They contend that APS not only serves as an effective substitute for agricultural labor (Peng et al., 2019), but also acts as a feasible means for technology dissemination (Wang and Wang, 2023). It is beneficial for promoting agricultural specialization and optimizing the resource allocation in agricultural production (Xu B. et al., 2024), thereby effectively alleviating the adverse impacts brought about by the substantial non-agricultural transfer of labor on agricultural production. Some scholars have also carried out research on the relationship between APS and grain yield, and found that APS can significantly enhance the grain yield per unit area (Liu et al., 2022a; Li et al., 2024; Wu et al., 2024). Zhao X. et al. (2021) conducted an empirical analysis on the impact of APS on farmers’ income and found that adopting APS can significantly raise farmers’ income. And there are differences in its impact on farmers of different farm sizes, and certain differences also exist in the impact effects of different types and links of APS. Nevertheless, some scholars argue that labor-intensive productive services like agricultural machinery services merely substitute labor inputs without bringing about efficiency improvements. Hence, they do not have a significant impact on grain production efficiency (Zhang and Yi, 2015). In addition, other scholars have explored the impact of different type of APS, such as labor-intensive and technology-intensive services, on grain production (Sun et al., 2018).
Existing research has provided important references for this paper, but there are also some shortcomings, mainly manifested in three aspects. Firstly, existing studies mostly conduct research on the impact of agricultural productive services on grain production from the perspectives of grain output and production efficiency. There are relatively few studies on the profit of grain planting, and insufficient attention has been paid to the impact of APS on farmers’ grain profit and its mechanism. Considering the realistic background where the comparative returns of grain crops have been low for a long time and the cost of productive services is constantly rising, the increase in grain production and efficiency does not necessarily mean an increase in the profit of grain planting. The profit of grain planting is the basis for improving farmers’ enthusiasm for grain planting, promoting sustainable grain production, and ensuring food security. Ignoring the impact of APS on the profit of grain planting may not be conducive to a comprehensive understanding of the potential impact of APS on food security. Secondly, if APS have a positive effect on farmers’ grain profits, is the impact on different farmers the same and which type of farmers is more affected? Existing studies have limited attention to the heterogeneity of production conditions and resource endowments and lack further heterogeneity analysis and discussion. In addition, existing studies have overlooked the constraint analysis of the application effect of APS. Especially for China, where the problem of fragmented farmland is prominent, fragmented farmland not only increases the cost of land management but also increases the difficulty of agricultural machinery operation, which seriously affects the application effect of APS (Yang et al., 2022). In many cases, fragmented farmland cannot obtain the required APS. Therefore, it is necessary to further discuss the important constraint of farmland fragmentation on the application effect of APS. Thirdly, the existing literature on the quantitative analysis of the impact mechanisms of APS on grain production lacks a thorough examination of endogeneity issues. Insufficient attention has been paid to potential reverse causality and omitted variable problems in these studies. The presence of such endogeneity issues may result in biased estimation outcomes and inaccurate research conclusions, thereby affecting the scientific understanding of the relationship between APS and farmers’ grain profits, as well as the formulation of relevant policy recommendations.
The main contributions of this paper are threefold: Firstly, using representative micro-survey data and an Endogenous Switching Regression model, which effectively mitigates endogeneity issues, this study empirically investigates the relationship between APS and farmers’ grain profits from the perspective of ensuring the sustainability of grain production by increasing farmers’ grain planting profits. This research provides further empirical evidence for studies related to APS and farmers’ grain profits, thereby enriching the existing literature. Food security has long been a popular research topic among scholars, but little is known about the role of APS in increasing farmers’ grain profits, motivating them to plant more grain, and thereby promoting the sustainability of grain production. In the current context where APS is widely applied, this study delves into the intrinsic relationship and functional mechanisms between APS and farmers’ grain profits. It aims to reveal the pathways and effects of APS on farmers’ grain profits, filling the research gap in this area. Moreover, it provides scientific evidence and decision-making references for policymakers to optimize APS policies and enhance farmers’ grain profits. Furthermore, existing studies have not systematically analyzed and rigorously empirically tested the mechanisms through which APS impacts farmers’ grain profits. This paper systematically argues, through three pathways of increasing yield, cost savings, and efficiency improvement, the impact mechanisms of APS on farmers’ grain profits. It employs a causal mediation analysis model to empirically test the specific impact mechanisms of APS on farmers’ grain profits, thus providing a beneficial supplement to previous research in this field. Thirdly, this study explores the heterogeneous impact of APS on farmers with different scales of farmland and levels of grain profit. It finds that APS has a greater positive impact on the farmers’ grain profits with smaller operational scales and lower grain profit levels. This suggests that APS can address the profitability challenges faced by small-scale farmers in grain production. Additionally, the study examines the role of farmland fragmentation in influencing the impact of APS on farmers’ grain profit, revealing that farmland fragmentation hinders the effectiveness of APS. This provides empirical evidence for formulating targeted land consolidation policies to mitigate the adverse effects of farmland fragmentation, further unlocking the potential of APS and promoting the sustainability of grain production. The subsequent sections of the paper are organized as follows. Section 2 introduces the theoretical framework and proposes three theoretical hypotheses; Section 3 describes the data, methodology and variables selection; Section 4 discusses the baseline regression results and conducts the impact mechanism identification and heterogeneity analysis; Section 5 presents the main conclusions, discussion and policy implications.
2 Mechanism analysis and research hypotheses
Agricultural production includes multiple links, such as sowing, irrigation, fertilization, pesticide application, and harvesting. The separability of agricultural production links provides a practical basis for the vertical division of labor in agriculture and broad development space for APS. APS, through specialized division of labor, mechanized operations, and the introduction of new technologies, can transform farmers from traditional experiential production and small-scale decentralized management to modern production methods of scale, mechanization, and intensification (Yang et al., 2019), which is beneficial for improving yield, saving costs, enhancing efficiency, and consequently influencing farmers’ grain profits.
Increasing farmers’ grain profits need to focus on improving grain yield. APS with multiple advantages, such as technology and management, provide strong support for achieving this goal. On the one hand, APS can increase grain yield by providing high-quality agricultural materials and advanced technological equipment. Compared to small farmers, service providers have more advantages in the agricultural material market, providing them with higher quality seeds, fertilizers, pesticides and other production materials at a lower cost, supplemented by professional farming, fertilization, pesticide application, and irrigation production technologies, thus forming a comprehensive production model that combines good varieties and methods and agricultural machinery and technology support, effectively avoiding the problem of high input and low output in agriculture and significantly improving yield, thereby increasing farmers’ grain profits (Xu Y. et al., 2022). On the other hand, APS can also improve yield by providing scientific management methods. Compared to small farmers who lack scientific production management methods and rely on experience to produce, APS organizations have more professional technical personnel, which can provide scientific production guidance to farmers, help them optimize the quantity and structure of factor inputs, carry out reasonable water and fertilizer management, disease and pest prevention and control, alleviate the problem of insufficient technical management ability of individual farmers (Zang et al., 2022), reduce the loss of grain production and achieve an increase in yield.
To increase farmers’ grain profits, efforts should also be made to reduce the cost of grain cultivation. Adopting APS can reduce the cost of food production materials and labor, thereby increasing farmers’ grain profits. On the one hand, compared to individual farmers, APS entities have scale advantages in purchasing agricultural materials, providing farmers with centralized purchasing services such as “high-quality fertilizers and varieties” of agricultural materials, achieving “double savings” in transaction costs and production material costs. Moreover, the specialization, facility, standardization, and precision of service subjects are higher (Cao and Wu, 2023), which reduces the loss of production materials and improves utilization efficiency, which is conducive to reducing the cost of production material investment. On the other hand, in the context of a large transfer of the rural labor force, labor prices are increasingly rising, and agricultural machinery shows a price advantage for labor (Zheng and Xu, 2017). Adopting APS instead of hiring workers can save production costs and increase net profit from grain production. Tang et al. (2018) found through a study of 3,421 rice growers that agricultural services contribute to cost savings.
Improving agricultural production efficiency is another key factor in increasing farmers’ grain profits. APS can optimize the allocation of labor, land, capital, and other factors by leveraging their advantages in equipment, technology, and talent, achieving efficiency improvement, and thereby improving farmers’ grain profits. First, the division of labor effect of APS can effectively improve the efficiency of various production links. Farmers outsource single or all production processes, such as cultivation, pesticide application, fertilization, and irrigation, to professional service entities, forming a specialized division of labor without changing the land ownership relationship. According to Smith’s division of labor theory, the division of labor can achieve improvement in production efficiency (Smith, 2002). A service entity with professional service talent, mechanical equipment, production technology, etc., plays a comparative advantage in various aspects of grain production, making the input of various production factors more scientific and reasonable and ensuring the efficient utilization of factors. Second, APS have a labor substitution effect, which can alleviate the labor constraints of farmers (Tian et al., 2023). With the continuous transfer of rural labor to nonagricultural sectors, the opportunity cost of agricultural labor is increasing, and the degree of parttime and aging agricultural production is gradually deepening. There is a structural shortage of rural labor, which restricts the improvement of agricultural production efficiency. The essence of farmers’ adopting APS is to replace labor with capital (Zhao Q. et al., 2021) to achieve relatively low costs and greater output elasticity of agricultural machinery and equipment to replace labor with relatively high costs and limited production capacity and to improve the technical efficiency of grain production through standardized mechanical operations, thereby providing effective support for ensuring farmers’ grain profits.
The influence path of agricultural productive services on farmers’ grain profits is shown in Figure 1. Based on the above analysis, this paper proposes the following hypothesis.
Hypothesis 1: There is a significant difference in the average grain profit earned between householders who adopted APS and those who did not. APS is conducive to the increase of farmers’ grain profits.
Hypothesis 2: APS primarily increase farmers’ grain profits through three paths: increasing grain yield, reducing production costs, and improving the efficiency of grain production.
The analysis regarding the impact of APS on farmers’ grain profits above is conducted under the assumption that farmers are homogeneous. In fact, there may be differences in the impact of APS on farmers’ grain profits. First, APS can introduce modern production factors into small farmers, which has advantages in helping farmers with weaker grain production capacity and poor production conditions achieve increased production, cost reduction, and efficiency improvement (Deng et al., 2020). That is, APS can enable different farmers to have similar production management methods, thereby eliminating the income gap between farmers with lower and higher grain yields. Second, studies have found that APS have a universal function of increasing efficiency and income. Under the role of factor supplementation and substitution brought by APS, the increase in production efficiency and household income of small farmers is significantly higher than that of largescale households. Compared to small-scale farmers who mainly rely on agriculture and have better basic agricultural production conditions, APS have a more significant effect on improving grain yields for smallholder farmers. Finally, the implementation of the household contract responsibility system has led to a generally small scale and high degree of fragmentation of agricultural land in China (Li et al., 2023). The fine distribution of agricultural land will increase the service operation time and difficulty, which is not conducive to the efficient operation of agricultural machinery (Lu et al., 2018). Therefore, the fragmentation of farmland not only restricts the service supply of service subjects (Chen et al., 2022) but also limits the scope and quality of APS and restricts the improvement of grain production profits brought by APS. Therefore,
Hypothesis 3: There are differences in the effects of agricultural productive services on farmers’ grain profits among heterogeneous farmers with grain profits, scales, and the degree of farmland fragmentation.
3 Research design
3.1 Data sources
The data used in this paper can be found in the “China Rural Revitalization Survey “(CRRS) Database of the Institute of Rural Development, Chinese Academy of Social Sciences in 2020. The CRRS project comprehensively considers the level of economic development, regional location, and agricultural development. Sample provinces are randomly selected from the eastern, central, western, and northeastern regions of China. Sample counties are randomly selected using the equidistant random sampling method based on the per capita GDP at the county level for the entire province, ensuring as much spatial coverage as possible. Using the same sampling method, sample townships (towns) and villages are randomly selected based on the economic development level of the local townships and villages. Finally, sample households are randomly selected according to the roster provided by the village committee. The CRRS ultimately selected 10 provinces and autonomous regions, including Guangdong, Zhejiang, Shandong, Anhui, Henan, Heilongjiang, Guizhou, Sichuan, Shaanxi, and Ningxia. The survey encompassed 50 counties and 156 townships nationwide. A total of 300 village questionnaires and over 3,800 farmer questionnaires were collected, gathering family member information from more than 15,000 individuals. This indicates a highly representative sample. The survey covers multiple aspects, such as agricultural production, farmers’ lives, and rural development, providing detailed data support for this study. Because this paper focuses on the impact of APS on farmers’ grain profits, the main research object is agricultural operators engaged in grain production. Therefore, to better identify causality, after selecting variables, this paper deleted samples of farmers who did not engage in grain cultivation and excluded samples with missing and abnormal data values. In the end, this study obtained a total of 1,523 valid samples of farm households. Therefore, the data used in this paper is cross-sectional data containing 1,523 sample households.
3.2 Variable description
3.2.1 Dependent variable
The dependent variable in this study is the net profit from grain production (Profit1), represented by the net income from grain production. Considering that purchasing APS also increases agricultural production costs, this article uses the net profit of grain production, which can better reflect the cost-effectiveness of food production, as the dependent variable for the baseline regression. In addition, we use the net profit per mu of grain production (Profit2) as the dependent variable for robustness testing. In order to mitigate the impact of heteroscedasticity on model estimation and address the issue of extreme values in income variables, this study logarithmically transformed the two variables mentioned above.
3.2.2 Independent variable
Whether to adopted APS (APS1) is the core independent variable of this paper. This study designates households who adopted APS in at least one of the six links of cultivation, sowing, pesticide application, fertilization, irrigation, harvesting and transportation as the treatment group. The control group comprises households that did not adopt APS in any of the aforementioned six links. In addition, this study utilizes number of links in which farmers adopt APS (APS2) and the proportion of expenditure on purchasing APS to total expenses to measure the degree of APS utilization (APS3). These are considered as core independent variables in the robustness testing section.
3.2.3 Control variable
Considering that household head characteristics, family characteristics, agricultural production characteristics, village characteristics, and regional features may all have an impact on farmers’ grain profits, this study, referring to the research conducted by Chang et al. (2023) and Wang et al. (2023), selects relevant control variables from these various dimensions. The individual characteristics variables of household heads include gender, age, education, health, and political identity (Liu et al., 2022b). Family characteristics variables include family size, labor force, subsidies, internet, social capital, and financial capital. For agricultural production characteristics variables, farmland size and planting structure are included. Village characteristics variables include the distance and economic level. At the same time, considering the differences in policies, natural conditions, and other factors among different regions, this study also controls the region.
3.2.4 Instrumental variable
The econometric method employed in this study necessitates at least one instrumental variable for estimating the model. Referring to the research conducted by Liu and Wu (2022) and Zhang Y. et al. (2023), this study utilizes the proportion of other households in the village adopting APS, the average number of links in which other households in the village adopting APS, and the average proportion of service expenses adopted by other farmers in the village to the total cost as instrumental variables. According to social network theory, whether other farmers in the same village adopt APS and the degree of APS adoption can influence a farmer’s decision to adopt APS. However, it is evident that this does not directly affect the farmer’s own grain production profits. Therefore, the instrumental variables chosen in this study theoretically satisfy both relevance and exogeneity, making them suitable instrumental variables.
3.2.5 Mechanism variables and moderating variables
Based on the theoretical analysis mentioned above, this paper posits that APS primarily enhance farmers’ grain profits through three paths: increasing grain yield, reducing production costs, and improving the efficiency of grain production. To validate these mechanisms, the paper selects yield, cost, and technical efficiency of grain production as mechanism variables, reflecting the effects of increased yield, cost savings, and efficiency improvements, respectively. Additionally, this study selects the degree of farmland fragmentation as a moderating variable to examine the heterogeneous impact of APS on farmers with different farmland endowment. The definitions and descriptive statistics of the above variables are shown in Table 1.
3.3 Descriptive statistics
From the descriptive statistical data of each variable reported in Table 1, it can be seen that out of the 1,523 sample households, 1,172 households adopted APS, accounting for approximately 76.95% of the total sample households. The average age of the surveyed household head is 55.38 years old, and the average education level is 7.76 years. The education level of the sample household heads is generally low, and the average age is relatively old. The average scale of farmland operated by the sample households is 25.89 mu, and the proportion of the planting area of the three main grains to the total operating area is 92.71% on average. The average number of farming plots managed by households is 5.64, indicating a high degree of fragmentation of the farmland operated by the sample farmers.
3.4 Econometric models and methods
3.4.1 Baseline regression model
To estimate the impact of APS on farmers’ grain profits, this paper establishes the following econometric model:
In Equation 1, the dependent variable represents the grain production profits of farm-household i; the core independent variable is whether farm-household i adopted APS; represents other variables that affect the grain profits from farm-household i, including personal characteristics of the head of household, family characteristics, agricultural production characteristics, village characteristics, and regional characteristics; , and are the coefficients to be estimated; and is the random error term.
3.4.2 Estimation methods
Firstly, this paper uses the ordinary least square (OLS) to estimate the baseline regression model to preliminarily determine the impact of APS on farmers’ grain profits. Then, two stage least square (2SLS) is used to estimate the model to preliminarily determine whether there is an endogeneity problem in the model and to test whether the selected instrumental variables are valid.
As rational individuals, whether or not farmers adopt APS is often a “self-selection” that takes into account their own benefits. There are unobservable factors that affect both farmers’ decisions on adopt APS and farmers’ grain profits. The existence of such endogeneity issues can result in biased estimation results. In view of this, this study uses the endogenous switching regression model (ESR) proposed by Lee (1982) to estimate the profits effects of APS, so as to solve the problem of model endogenous. ESR has three main advantages. First, it simultaneously considers both observable and unobservable factors affecting the decision to adopt APS and farmers’ grain profits, thereby minimizing selection bias and endogeneity issues in the decision to adopt APS. Second, it enables the estimation of the factors influencing farmers’ grain profits for both households adopting and those not adopting APS. Third, it can establish a “counterfactual” estimate to further measure the impact of adopting APS on the changes in farmers’ grain profits, thus demonstrating more directly the relationship between APS and farmers’ grain profits (Ma and Abdulai, 2019).
This method mainly includes two stages. The first stage is the selection equation, which examines the influencing factors of whether farmers adopt APS. Whether farmers adopt APS is influenced by many factors, such as the personal characteristics of the head of household, family characteristics, and production characteristics. Farmers make production decisions by balancing internal and external conditions to maximize their own utility. The second stage is the result equation, which estimates the impact of various factors on grain production profits of two types of farmers who adopt and do not adopt APS. To further analyze the overall impact of APS, the ESR model can be used to estimate the average treatment effect on treatment (ATT) of the impact of APS on farmers’ grain profits. The calculation formula for ATT is:
Evaluating the impact of APS on farmers’ grain profits requires information about the outcome of farmers adopting APS (actual outcome) and the outcome of these farmers had they not adopted APS (counterfactual outcome). In Equation 2, represents the profits in grain production of farm-household i who adopted APS, represents the profits in grain production of farm-household i who did not adopt APS.
3.4.3 Mechanism analysis method
The above methods only examined the impact of APS on farmers’ grain profits but failed to examine how APS affect farmers’ grain profits. At present, most of the research on the impact mechanism in the academic community relies on the mediation effect model. However, the stepwise regression method used in general mediation effect analysis does not fully consider the “counterfactual” causal inference framework, which cannot effectively identify the causal relationship between independent variables, mechanism variables, and dependent variables. In view of this, this paper refers to the research of Cai et al. (2022) and used the causal mediation analysis (CMA) model to explore the mechanism of the impact of APS on farmers’ grain profits. Compared with traditional mediation analysis methods, the advantage of this model is that on the one hand, it estimates the “average mediation effect” and “direct effect” based on the “counterfactual” framework, achieving causal inference between processing variables, mediation variables, and outcome variables. On the other hand, the basic approach of CMA to identify causal mediation effects is the Quasi-Bayesian Monte Carlo approximation, which is a method based on computer-simulated random experiments. Therefore, CMA can nest various linear, nonlinear, and even non-parametric estimation models within its framework and effectively address challenges such as selection bias that are often encountered in traditional statistical analyses. The specific identification procedures can be referred in Imai et al. (2010) and will not be detailed in this paper.
4 Empirical results analysis and discussion
Before conducting econometric analysis, we first tested the correlation and collinearity between the independent and control variables. The correlation test results show that the correlation coefficients between variables are all below 0.4, indicating that there is not a strong correlation between variables. The multicollinearity test also showed that the VIF values of the variance expansion factor for each variable were all below 1.2, indicating the absence of multicollinearity among the variables. Therefore, it is appropriate to conduct econometric analysis on the above variables.
4.1 Baseline regression results
The OLS regression results in columns (1) and (2) of Table 2 indicate that, without controlling for relevant variables, the estimated coefficient for the impact of APS on the profits of grain production is 1.424. After controlling for relevant variables, the estimated coefficient changes to 0.782. In both cases, the coefficients are statistically significant at the 1% level, demonstrating that APS improve farmers’ grain profits. This conclusion is generally consistent with the findings of Luan et al. (2022), based on a survey of 620 wheat farmers in Hebei, Shandong, and Henan provinces, which also reported a significantly positive impact of APS on grain profits. The estimation results of the 2SLS model indicate that the test statistic for the endogeneity test is 16.133, and it is significant at the 1% level. This suggests that the independent variable is an endogenous variable, indicating a substantial endogeneity issue in the model. In column (3), the results show that IV_APS1 has a significantly positive impact on APS1, indicating that the selected instrumental variable is strongly correlated with the endogenous independent variable. The K-P rk LM test statistic is 310.580 and is statistically significant at the 1% level, indicating the absence of identification issues. In the weak instrument test, the K-P rk Wald F test value is 577.394, exceeding the critical value of 16.38 provided by Stock-Yogo at the 10% significance level. Consequently, we reject the null hypothesis of “weak instrument” affirming the absence of weak instrument problems. After correcting for endogeneity issues using the instrumental variable approach, the regression results still demonstrate that APS significantly increase farmers’ grain profits.
Column (5) in Table 2 presents the estimation results of the equation determining the decision to adopt APS. Columns (6) and (7) respectively display the estimation results of the grain profits determination equation for households that did not adopt APS and those that did adopt APS. The Wald test statistic for the independence of equations is 16.02, and it is statistically significant at the 1% level, indicating the presence of correlation between the selection equation and the outcome equation. ρ1 and ρ0 represent the correlation coefficients between the error terms of the decision model for adopting APS and the income effect models for both the adopting and non-adopting groups of households. The estimated value of ρ1 is statistically significant at the 1% level, suggesting the existence of unobservable factors simultaneously influencing farmers’ grain profits and the decision to adopt APS. This implies the presence of sample selection bias, and correcting for it is necessary to avoid biased estimation. The Wald chi2 value for the goodness of fit of the ESR model is significant at the 1% significance level, indicating a good model fit and overall stability of the estimation results.
According to the estimation results of the selection equation in the ESR model, when other conditions remain unchanged, IV_APS1 has a significant positive impact on APS1. That is, the higher the proportion of farmers adopting APS in the same village is, the greater the likelihood of farmers adopting APS. The younger the head of household is, the higher the education level, the lower the proportion of the household labor force, the more social capital the household have, the more the planting structure tends to be nongrain oriented, and the better the transportation conditions in the village are, the greater the probability of adopting APS. According to the estimated results of the effect equation of the adopting group and non-adopting group in Table 2, it can be seen that the education, family size, internet, social capital of farmers who have not adopted APS have a significant impact on their grain profits. For farmers who adopt APS, the health status of the household head, family size, government subsidies, planting structure, the economic level of the village, and regional factors have a significant impact on farmers’ grain profits. In addition, regardless of whether farmers adopt APS, the size of farmland has a significant impact on farmers’ grain profits. To obtain more intuitive results on the impact of APS on farmers’ grain profits, it is necessary to further estimate ATT.
The second row of Table 3 reports the actual and counterfactual estimates and the average treatment effect (ATT) of APS on farmers’ grain profits based on the ESR model. Compared to the mean differences presented in columns (6) and (7) of Table 2, the estimated results of the average treatment effects in Table 3, within the counterfactual framework, correct for observable and unobservable factors causing selection bias. The results indicate that adopting APS enhances the net income of grain production by 36.45% for treat group. Similarly, for households in control group, adopting APS leads to a 76.49% increase in the net income of grain production. This result is consistent with the findings of Sang et al. (2023), implying that farmers’ adopting APS significantly enhances their grain production profits. Based on this, the hypotheses H1 have been validated. This conclusion provides theoretical support for the phenomenon of promoting the widespread adoption of APS in agricultural development.
4.2 Robustness test
To test the robustness of the above regression results, this paper adopts four methods for robustness testing: replacing the dependent variable, changing the sample size, changing the measurement method, and replacing the core independent variable.
4.2.1 Replace dependent variable
Taking into account the impact of farmland scale, this paper replaces the dependent variable with the net income per mu of grain production of households to test the robustness of the baseline regression. The first row of Table 4 shows the estimated average treatment effect (ATT) of the impact of APS on farmers’ grain profits based on the ESR model. The estimated results show that the ATT is 1.111, which is significant at the 1% statistical level, indicating that excluding sample selection bias, adopting APS by households can effectively improve farmers’ grain profits. Specifically, adopting APS will increase the net income per mu of grain production by 20.04%, which is consistent with the average treatment effect of APS on grain profits in Table 3. Hypothesis H1 has been validated again, and the results are relatively robust.
4.2.2 Change in sample size
To test the robustness of the baseline regression results, this paper retained 1,282 households that only planted three main grains and used the ESR model for further estimation. The second row of Table 4 reports the estimated average treatment effect (ATT) based on subsample regression. The results show that the estimated ATT value is 2.180 and passes the 1% significance level test, indicating that for farmers who use APS, their grain production profits will increase by 41.12%, slightly higher than the treatment results shown in Table 3. However, overall, the results are basically consistent, and the research findings remain stable.
4.2.3 Change measurement method
This paper also utilizes the endogenous treatment effect model (ETEM) to re-estimate the impact of APS on farmers’ grain profits, testing the robustness of the conclusions. The estimated results are presented in the third row of Table 4. The ATT value for the impact of APS on farmers’ grain profits, estimated using ETEM, is 1.629, indicating that adopting APS can increase the net income of grain production by 26.99%. The ATT results are essentially consistent with those shown in Table 3, reaffirming the robustness of the research findings.
4.2.4 Replace independent variables
The core independent variable of the baseline regression mentioned above is the binary selection variable, which only measures whether to use but failed to quantify the extent of the use of APS. Therefore, this paper selects the number of links in which farmers adopt APS (APS2) and the proportion of expenditure on purchasing APS to total expenses (APS3) as independent variables to conduct robustness checks on the baseline regression. As both of the above variables are continuous, this study employs the 2SLS method to estimate the model, and the estimation results are shown in Table 5. The results show that the coefficient of APS2 is 0.618, which is significant at the 1% statistical level. This indicates that the greater the number of links in the adopt of APS, the more it contributes to enhancing farmers’ grain profits. The coefficient of APS3 is 4.395, indicating that enhancing the application proportion of APS is beneficial for enhancing farmers’ grain profits. Further validation has confirmed the positive impact of APS on farmers’ grain profits.
4.3 Mechanism analysis
In order to reveal the mechanism of the impact of APS affect farmers’ grain profits, combined with the aforementioned theoretical analysis, and based on the theoretical analysis mentioned earlier, this paper employs the CMA model for the test of the mediating effect. According to the assumption “if the confidence interval does not include zero,” that is, when the upper and lower limits of the confidence interval are both positive (or negative), it indicates a significant positive (or negative) mediating effect. The estimated results of the Average Causal Mediation Effect (ACME) from Table 6 indicate that the ACME values for yield increase, cost reduction, and efficiency improvement are 0.061, 0.071, and 0.048, respectively. The confidence intervals for all these effects are positive and do not include zero, suggesting that APS significantly promote the enhancement of farmers’ grain profits through the mediating effects of increased yield, cost reduction, and efficiency improvement. Based on this, the hypotheses H2 have been validated.
Simultaneously, the profits-increasing effect of APS is composed of 7.78% from the transmission mechanism of yield increase, 9.14% from the transmission mechanism of cost reduction, and 6.14% from the transmission mechanism of efficiency improvement. In comparison, the impact on farmers’ grain profits enhancement brought about by APS through the cost reduction effect is the largest, followed by the yield increase effect, with the mediation effect of the efficiency improvement being the smallest. This phenomenon could be attributed to the direct engagement of cost reduction in economic benefits related to resource utilization, resulting in a substantial direct influence on the net income of farmers. Although increasing production positively affects the overall yield, its mediating effect tends to be comparatively smaller when factoring in the consideration of costs.
In addition, the above mediation effect test results are based on the “sequence negligibility hypothesis,” which is the correlation coefficient (ρ) between the disturbance term of the mediation variable predictive model and the disturbance term of the outcome variable predictive model is zero. However, in practice, there may be unobservable confounding factors that violate this strong assumption, leading to biased estimates of ACME. Therefore, a sensitivity analysis is needed to verify the robustness of the causal mediation effect estimation results. This paper refers to the approach of Imai et al. (2010), by relaxing the assumption that ρ is zero and providing different values for ρ, we obtain the trajectory of ACME as ρ varies. If a small ρ results in a significantly different ACME compared to the case where ρ is zero, it indicates sensitivity to the violation of the sequence negligibility hypothesis, suggesting that the estimation results are not robust. Table 6 shows the values of ρ when the ACME for the three mediating variables is zero, which are 0.076, −0.062, and 0.076, respectively. Taking the technical effect as an example, ρ = 0.076 implies that if there are unobserved confounders, the variance of at least one confounder in the mediation test model accounts for no less than 0.076 of the variances of the error term. The adjusted R-squared values for the mediation test models are 0.031 and 0.126, and the corresponding R-squared values for the error terms are 0.969 and 0.874. Multiplying by 0.076 gives the proportion of the unobserved confounder variance to the total model variance, which is 0.074 and 0.066, respectively. With around 15 control variables, the explanatory power of our control variables exceeds the cumulative explanatory power of multiple covariates. Therefore, we have reason to believe that the possibility of such con-founders is extremely low, indicating the robustness of the estimation results.
4.4 Heterogeneity analysis
4.4.1 Heterogeneity analysis based on the level of farmers’ grain profits
This study not only focuses on whether and how APS affect farmers’ grain profits but also examines the impact of APS on the grain profits disparity among rural house-holds. This paper uses the instrumental variable quantile regression (IVQR) method to test the impact of APS on farmers’ grain profits at different levels. Thus, it can be determined whether APS is beneficial for improving the grain profits of low-profit households and reducing the disparity in grain production profits among households.
As shown in Table 7, as the grain profits of households varies from the 10th percentile to the 90th percentile, the estimated coefficients of APS sequentially decrease. Moreover, at the 90th percentile, the estimated coefficient is no longer significant. This indicates that the effect of adopting APS on increasing grain profits is more pronounced for households with lower grain profits. In other words, APS can significantly reduce the profits disparity in grain production among households. This may be attributed to the fact that low-profits farmers often encounter constraints in terms of technology and resources. The provision of APS can significantly mitigate these limitations, thereby exerting a more pronounced impact on their grain production profits.
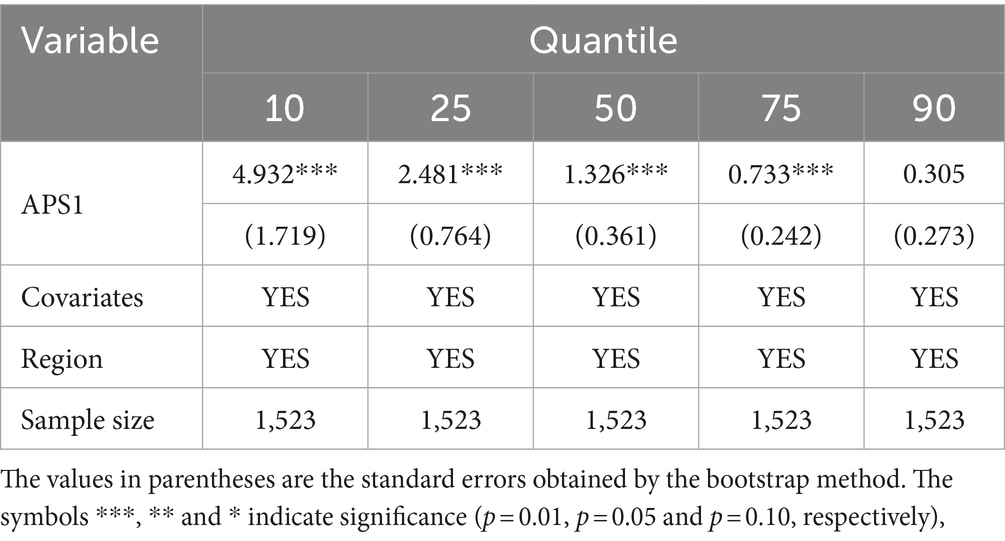
Table 7. The estimated impact of APS on farmers’ grain profits at different levels of grain profits.
4.4.2 Heterogeneity analysis based on the size of farmland
The above analysis treats the sample households as a homogeneous whole. However, in reality, due to differences in operating scale, households may vary significantly in production capacity, operational goals, resource endowments, service needs, etc. As a result, the impact of APS on grain profits may vary among different households. Therefore, following the classification criteria of the Food and Agriculture Organization of the United Nations, this paper categorizes households with an operating area of less than 30 mu (2 hectares) as small-scale households and households with 30 mu or more as large-scale households. Subsequently, it further analyses the impact of APS on the grain profits of households with different scales. As shown in Table 8, columns (1) and (2), adopting APS leads to a 1.46% increase in grain profits for small-scale households. However, for large-scale households, adopting APS does not have a significant impact on their grain profits. This conclusion aligns with the findings of Zhang Z. et al. (2023), indicating that the increase in production efficiency and income for small-scale households is significantly higher than for large-scale operating house-holds through adopting APS.
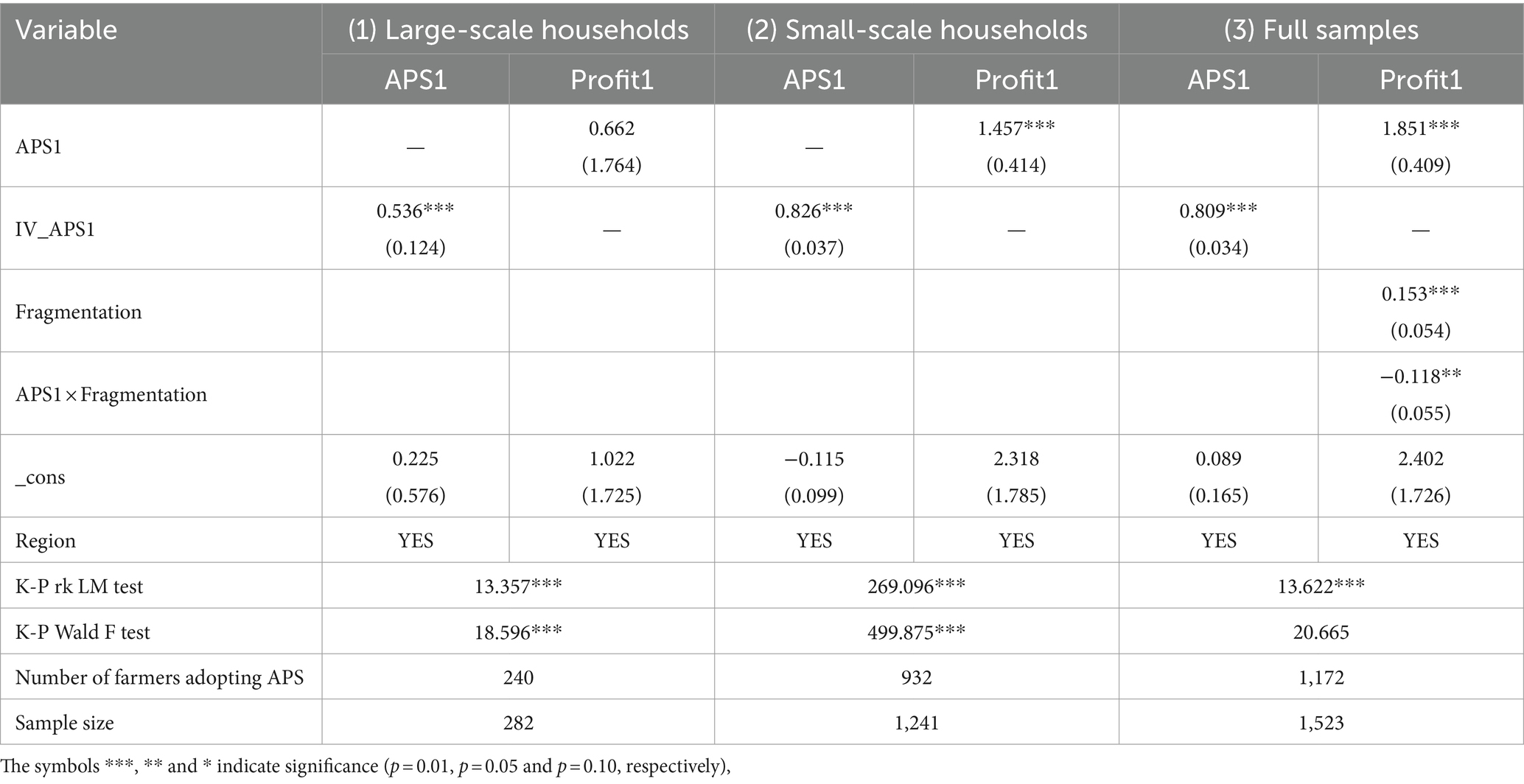
Table 8. The estimated heterogeneity effects of APS on grain profits for households with different levels of farmland operating scale and fragmentation degree.
4.4.3 Heterogeneity analysis based on farmland fragmentation
The impact of APS on farmers’ grain profits may be constrained by the fragmentation of farmland. To verify this inference, this paper adds the degree of farmland fragmentation and its interaction with APS in Equation 1 to examine the moderating effect of farmland fragmentation on the relationship between APS and farmers’ grain profits. The estimated results are shown in column (3) of Table 8. The estimated coefficient between the degree of farmland fragmentation and the interaction term of APS is-0.118, which is significant at the statistical level of 5%. It can be observed that the impact of APS on grain profits is significantly higher for households with low farm-land fragmentation compared to those with high farmland fragmentation. In other words, farmland fragmentation weakens the positive effect of APS on farmers’ grain profits. These findings further corroborated some of the research findings of Hao et al. (2023) and Wang et al. (2020).
5 Discussion
This study analyzes the role of agricultural productive services in increasing farmers’ grain profits. Empirical research shows that agricultural productive services play a positive role in responding to the impact of small-scale farmers’ scattered management on grain profits. In the context of the global food crisis and food trade protection, the results of this study provide important guidance for policymakers. It should be emphasized that there are many types of agricultural productive services, and the impact effects of different types of agricultural productive services on farmers’ grain profits are not the same. Some agricultural productive services may even have costs that exceed their benefits. Therefore, it is necessary to conduct in-depth research on the impact of different types of agricultural productive services on grain profits in the future. For example, further exploring the different impacts of adopting APS on grain profits in the links of plowing, sowing, management, and harvesting. Which one has a greater impact on grain profits, labor-intensive productive services or technology-intensive productive services. In addition, our research is an empirical analysis based on cross-sectional data, and it has not tested the long-term impact of agricultural productive services on grain profits. We hope to use large-sample panel data for further exploration in the future to verify and deepen our research results, and more accurately and comprehensively analyze the impact of agricultural productive services on grain profits and food security.
6 Conclusion and policy implications
6.1 Conclusion
Under the impetus of policy support and the demands of farmers, agricultural productive services have gradually emerged as an effective means to bridge the gap between small-scale farmers and modern agriculture. Leveraging data from the 2020 Chinese Rural Revitalization Survey encompassing 1,523 households engaged in grain cultivation, this study employs the ESR model and causal mediation analysis to examine the impact and underlying mechanisms of agricultural productive services on farmers’ grain profits. Furthermore, the research delves into the heterogeneous effects of agricultural productive services on grain profits across different types of farming households. The primary findings of this study can be summarized as follows.
Firstly, there is a significant difference on the average grain profit earned between householders who adopted agricultural productive services and those who did not. Agricultural productive services significantly enhance farmers’ grain profits, and this conclusion remains robust even after altering the dependent variable, sample size, core independent variables, and econometric methods. Secondly, Agricultural productive services contribute to increased grain profits through three pathways: increasing production, saving costs, and improving efficiency. Among these, the cost reduction pathway has the most substantial impact on enhancing farmers’ grain profits. Thirdly, there is heterogeneity in the impact of agricultural productive services on farmers’ grain profits. The positive effect of agricultural productive services is more pronounced for farmers with lower grain profits, while its influence is less significant for farmers with higher grain profits. This contributes to narrowing the income gap among farmers. Compared to larger-scale farms, agricultural productive services have a more noticeable effect on increasing grain profits for small-scale farms. The degree of farmland fragmentation significantly diminishes the positive impact of agricultural productive services on grain profits.
6.2 Policy implications
The concluding policy implications of this study are as follows. First, it is essential to fully recognize the importance of agricultural productive services in increasing farmers’ grain profits and promoting the sustainable production of grain, establish a sound agricultural productive service system, vigorously carry out agricultural productive services for the vast number of farmers, and fully unleash the role of agricultural productive services in reducing costs, improving quality, enhancing efficiency, and increasing income in grain production. Specifically, local governments in all regions should create a favorable institutional environment and financial support for the development of the agricultural productive service market, encourage all regions to support service organizations in providing public welfare production services for farmers through methods such as government adopt of services, rewards instead of subsidies, and subsidies after services, so as to promote the increase of farmers’ grain profits through service scale and ensure the sustainable production of grain. Second, it is necessary to give full play to the role of agricultural productive services in promoting the organic connection between small householders and modern agriculture, innovate service methods, optimize service capabilities, so that more small householders can obtain high-quality services and technical support at a lower cost, and thereby increase the grain production profits of small householders and enhance their enthusiasm for growing grain. Third, it is crucial to create favorable external conditions for the development of agricultural productive services to ensure that more farmers can benefit from them. Specifically, it is necessary to gradually strengthen the governance of fragmented cultivated land, promote the moderately scaled operation of contiguous land, and remove obstacles for the promotion and application of agricultural productive services, so that agricultural productive services can give full play to their advantages and better provide farmers with all-round and multi-level professional services.
Data availability statement
Publicly available datasets were analyzed in this study. This data can be found at: China Rural Revitalization Survey Database.
Author contributions
GH: Conceptualization, Formal analysis, Funding acquisition, Writing – original draft, Writing – review & editing. WC: Data curation, Methodology, Project administration, Writing – review & editing. XC: Visualization, Software, Writing – review & editing. QG: Supervision, Validation, Funding acquisition, Writing – review & editing.
Funding
The author(s) declare that financial support was received for the research, authorship, and/or publication of this article. This study was supported by the National Natural Science Foundation of China (No. 72273070); Postgraduate Research & Practice Innovation Program of Jiangsu Province (No. KYCX23_1100).
Conflict of interest
The authors declare that the research was conducted in the absence of any commercial or financial relationships that could be construed as a potential conflict of interest.
Publisher’s note
All claims expressed in this article are solely those of the authors and do not necessarily represent those of their affiliated organizations, or those of the publisher, the editors and the reviewers. Any product that may be evaluated in this article, or claim that may be made by its manufacturer, is not guaranteed or endorsed by the publisher.
References
Ai, J., Hu, L., Xia, S., Xiang, H., and Chen, Z. (2023). Analysis of factors influencing the adoption behavior of agricultural productive services based on logistic—ISM model: a case study of rice farmers in Jiangxi province, China. Agriculture 13:162. doi: 10.3390/agriculture13010162
Cai, L., and Wang, L. (2021). Analysis on outsourcing service behavior of rice pest and disease control based on Heckman selection model—a case study of ten counties in Fujian Province. PLoS One 16:e0254819. doi: 10.1371/journal.pone.0254819
Cai, L., Wang, L., and Ning, M. (2022). Farmers’ livelihood differentiation and pesticide application: empirical evidence from a causal mediation analysis. Sustain. For. 14:8502. doi: 10.3390/su14148502
Cao, G., and Wu, P. (2023). How to "serve well" and "drive well" small farmers: based on the perspective of agricultural machinery socialized services. Iss. Agric. Econ. 10, 22–31. doi: 10.13246/j.cnki.iae.2023.10.004
Chang, Q., Deng, Y., and Zhao, M. J. (2023). Can outsourcing in the production process support China's food security? Evidence from major grain-producing areas. J. Huazhong Agric. Uni. (Soc. Sci. Ed.) 4, 11–24. doi: 10.13300/j.cnki.hnwkxb.2023.04.003
Chen, T., Rizwan, M., and Abbas, A. (2022). Exploring the role of agricultural services in production efficiency in Chinese agriculture: a case of the socialized agricultural service system. Landscape 11:347. doi: 10.3390/land11030347
Deng, X., Xu, D., Zeng, M., and Qi, Y. (2020). Does outsourcing affect agricultural productivity of farmer households? Evidence from China. China Agric. Econ. Rev. 12, 673–688. doi: 10.1108/CAER-12-2018-0236
Gong, B. (2020). Agricultural productivity convergence in China. China Econ. Rev. 60:101423. doi: 10.1016/j.chieco.2020.101423
Hao, W., Hu, X., Wang, J., Zhang, Z., Shi, Z., and Zhou, H. (2023). The impact of farmland fragmentation in China on agricultural productivity. J. Clean. Prod. 425:138962. doi: 10.1016/j.jclepro.2023.138962
Imai, K., Keele, L., and Tingley, D. (2010). A general approach to causal mediation analysis. Psychol. Methods 15, 309–334. doi: 10.1037/a0020761
Laborde, D., Martin, W., Swinnen, J., and Vos, R. (2020). COVID-19 risks to global food security. Science 369, 500–502. doi: 10.1126/science.abc4765
Lee, L. (1982). Some approaches to the correction of selectivity bias. Rev. Econ. Stud. 49, 355–372. doi: 10.2307/2297361
Li, R., Chen, J., and Xu, D. (2024). The impact of agricultural socialized service on grain production: evidence from rural China. Agriculture 14:785. doi: 10.3390/agriculture14050785
Li, X., and Guo, X. (2023). Can policy promote agricultural service outsourcing? Quasi-natural experimental evidence from China. Sustain. For. 15:1009. doi: 10.3390/su15021009
Li, J., and Jiang, C. (2023). China's grain supply and demand situation: historical review, risk challenges, and policy implications. J. Nanjing Agric. Univ. (Soc. Sci. Ed.) 23, 168–179. doi: 10.19714/j.cnki.1671-7465.2023.0045
Li, B., Qian, Y., and Kong, F. (2023). Does outsourcing service reduce the excessive use of chemical fertilizers in rural China? The moderating effects of farm size and plot size. Agriculture 13:1869. doi: 10.3390/agriculture13101869
Liu, Y., Heerink, N., Li, F., and Shi, X. (2022a). Do agricultural machinery services promote village farmland rental markets? Theory and evidence from a case study in the North China plain. Land Use Policy 122:106388. doi: 10.1016/j.landusepol.2022.106388
Liu, Y., Shi, X., and Gao, F. (2022b). The impact of agricultural machinery services on cultivated land productivity and its mechanisms: a case study of Handan city in the North China plain. Front. Environ. Sci. 10:1008036. doi: 10.3389/fenvs.2022.1008036
Liu, T., and Wu, G. (2022). Does agricultural cooperative membership help reduce the overuse of chemical fertilizers and pesticides? Evidence from rural China. Environ. Sci. Pollut. R. 29, 7972–7983. doi: 10.1007/s11356-021-16277-0
Lu, Q., Du, X., and Qiu, H. (2022). Adoption patterns and productivity impacts of agricultural mechanization services. Agric. Econ. 53, 826–845. doi: 10.1111/agec.12737
Lu, H., Xie, H., He, Y., Wu, Z., and Zhang, X. (2018). Assessing the impacts of land fragmentation and plot size on yields and costs: a translog production model and cost function approach. Agric. Syst. 161, 81–88. doi: 10.1016/j.agsy.2018.01.001
Lu, H., Xie, H., and Yao, G. (2019). Impact of land fragmentation on marginal productivity of agricultural labor and non-agricultural labor supply: a case study of Jiangsu. China Habitat Int. 83, 65–72. doi: 10.1016/j.habitatint.2018.11.004
Luan, J., Han, Y., and Gao, Y. (2022). Can agricultural productive services ensure Farmers' grain income? Agric. Tech. Econ. 5, 35–48. doi: 10.13246/j.cnki.jae.20211214.008
Ma, W., and Abdulai, A. (2019). IPM adoption, cooperative membership and farm economic performance: insight from apple farmers in China. China Agric. Econ. Rev 11, 218–236. doi: 10.1108/CAER-12-2017-0251
Min, S. H. I., Paudel, K. P., and Chen, F. B. (2021). Mechanization and efficiency in rice production in China. J. Integr. Agric. 20, 1996–2008. doi: 10.1016/S2095-3119(20)63439-6
Na, H., Yan, X., Xing, R., and Jiang, A. (2024). The empirical effect of agricultural social services on pesticide inputs. Sci. Rep. 14:15907. doi: 10.1038/s41598-024-67016-7
Niroula, G. S., and Thapa, G. B. (2005). Impacts and causes of land fragmentation, and lessons learned from land consolidation in South Asia. Land Use Policy 22, 358–372. doi: 10.1016/j.landusepol.2004.10.001
Peng, L., Chi, Z., Fu, J., and Yu, Y. (2019). Research on the adjusting effects of agricultural machinery operation services and agricultural technology training on grain production under the background of aging labor force: microscopic survey data from Jiangxi Province. Agric. Tech. Econ. 9, 91–104. doi: 10.13246/j.cnki.jae.2019.09.009
Peng, J., Zhao, Z., and Liu, D. (2022). Impact of agricultural mechanization on agricultural production, income, and mechanism: evidence from Hubei province, China. Front. Environ. Sci. 10:838686. doi: 10.3389/fenvs.2022.838686
Qian, J., Ito, S., and Zhao, Z. (2020). The effect of price support policies on food security and farmers’ income in China. Aust. J. Agric. Resour. Econ. 64, 1328–1349. doi: 10.1111/1467-8489.12398
Qian, L., Lu, H., Gao, Q., and Lu, H. (2022). Household-owned farm machinery vs. outsourced machinery services: the impact of agricultural mechanization on the land leasing behavior of relatively large-scale farmers in China. Land Use Policy 115:106008. doi: 10.1016/j.landusepol.2022.106008
Qiu, T., and Luo, B. (2021). Do small farms prefer agricultural mechanization services? Evidence from wheat production in China. Appl. Econ. 53, 2962–2973. doi: 10.1080/00036846.2020.1870656
Rahman, A., and Mishra, S. (2020). Does non-farm income affect food security? Evidence from India. J. Dev. Stud. 56, 1190–1209. doi: 10.1080/00220388.2019.1640871
Ren, C., Zhou, X., Wang, C., Guo, Y., Diao, Y., Shen, S., et al. (2023). Ageing threatens sustainability of smallholder farming in China. Nature 616, 96–103. doi: 10.1038/s41586-023-05738-w
Sang, X., Luo, X., Razzaq, A., Huang, Y. Z., and Erfanian, S. (2023). Can agricultural mechanization services narrow the income gap in rural China? Heliyon 9:e13367. doi: 10.1016/j.heliyon.2023.e13367
Shi, R., Shen, Y., Du, R., Yao, L., and Zhao, M. (2024). The impact of agricultural productive service on agricultural carbon efficiency—from urbanization development heterogeneity. Sci. Total Environ. 906:167604. doi: 10.1016/j.scitotenv.2023.167604
Smith, A. (2002). An inquiry into the nature and causes of the wealth of nations. In Readings Econ. Sociol. 1, 6–17. doi: 10.1002/9780470755679
Sun, D., Rickaille, M., and Xu, Z. (2018). Determinants and impacts of outsourcing pest and disease management: evidence from China’s rice production. China Agric. Econ. Rev. 10, 443–461. doi: 10.1108/CAER-01-2017-0011
Tang, L., Liu, Q., Yang, W., and Wang, J. (2018). Do agricultural services contribute to cost saving? Evidence from Chinese rice farmers. China Agric. Econ. Rev. 10, 323–337. doi: 10.1108/CAER-06-2016-0082
Tian, Y., Gao, Y., and Pu, C. (2023). Do agricultural productive services alleviate farmland abandonment? Evidence from China rural household panel survey data. Front. Environ. Sci. 11:1072005. doi: 10.3389/fenvs.2023.1072005
Wang, Y., Li, X., Lu, D., and Yang, J. (2020). Evaluating the impact of land fragmentation on the cost of agricultural operation in the southwest mountainous areas of China. Land Use Policy 99:105099. doi: 10.1016/j.landusepol.2020.105099
Wang, Y., and Wang, Z. (2023). Socialized services, technical cognition, and Farmers' adoption behavior of water-saving irrigation technology: an empirical analysis based on survey data of 775 wheat and corn planters in Hebei Province. J. Arid Land Resour. Env. 37, 76–83. doi: 10.13448/j.cnki.jalre.2023.115
Wang, X., Xu, Z., Li, G., Zhou, Y., and Zou, W. (2023). Farmland transfer and income distribution effect of heterogeneous farmers with livelihood capital: evidence from CFPS. Landscape 12:1398. doi: 10.3390/land12071398
Wei, S., and Tang, Z. (2022). Why agricultural machinery outsourcing service turns from prosperity to decline: an explanation based on the influence of transaction risk on Farmers' agricultural machinery adoption behavior. Agric. Tech. Econ. 12, 4–22. doi: 10.13246/j.cnki.jae.2022.12.001
Wu, A., Elahi, E., Cao, F., Yusuf, M., and Abro, M. I. (2024). Sustainable grain production growth of farmland–a role of agricultural socialized services. Heliyon 10:e26755. doi: 10.1016/j.heliyon.2024.e26755
Xie, S., Zhang, J., Li, X., Chen, Z., Zhang, X., and Xia, X. (2023). Impact of farmer participation in production chain outsourcing services on agricultural output level and output risk: evidence from the Guanzhong plain, China. Agriculture 13:2263. doi: 10.3390/agriculture13122263
Xu, B., Baležentis, T., Štreimikienė, D., and Shen, Z. (2024). Enhancing agricultural environmental performance: exploring the interplay of agricultural productive services, resource allocation, and marketization factors. J. Clean. Prod. 439:140843. doi: 10.1016/j.jclepro.2024.140843
Xu, M., Chen, C., and Ahmed, M. A. (2024). Market-oriented farmland transfer and outsourced machinery services: evidence from China. Economic Analysis and Policy 81, 1214–1226. doi: 10.1016/j.eap.2024.02.014
Xu, Y., Lyu, J., Xue, Y., and Liu, H. (2022). Does the agricultural productive service embedded affect farmers’ family economic welfare enhancement? An empirical analysis in black soil region in China. Agriculture 12:1880. doi: 10.3390/agriculture12111880
Xu, C., Wang, Q., Fahad, S., Kagatsume, M., and Yu, J. (2022). Impact of off-farm employment on farmland transfer: insight on the mediating role of agricultural production service outsourcing. Agriculture 12:1617. doi: 10.3390/agriculture12101617
Yang, B., Duan, Y., and Zhao, Q. (2022). The effect of land fragmentation on farmers’ rotation behavior in rural China. Front. Environ. Sci. 10:1042755. doi: 10.3389/fenvs.2022.1042755
Yang, Q., Zhang, N., Lu, Q., and Han, X. (2023). Family net income, input factor prices and agriculture services selection behavior of maize farmers. Agriculture 14:62. doi: 10.3390/agriculture14010062
Yang, Z., Zhang, J., and Zhu, P. (2019). Can agricultural socialized services promote small Farmers' integration into agricultural modernization? A perspective from technological efficiency. Agric. Tech. Econ. 9, 16–26. doi: 10.13246/j.cnki.jae.2019.09.002
Zang, L., Wang, Y., Ke, J., and Su, Y. (2022). What drives smallholders to utilize socialized agricultural services for farmland scale management? Insights from the perspective of collective action. Landscape 11:930. doi: 10.3390/land11060930
Zhang, L., Dong, Y., Liu, C., and Bai, Y. (2018). Off-farm employment over the past four decades in rural China. China Agric Econ. Rev. 10, 190–214. doi: 10.1108/CAER-11-2017-0212
Zhang, H., and Guo, X. (2021). Development of agricultural productive services and improvement of agricultural Total factor productivity: regional differences and spatial effects. Agric. Tech. Econ. 5, 93–107. doi: 10.13246/j.cnki.jae.2021.05.007
Zhang, Z., Pan, B., and Gao, M. (2023). Agricultural socialized services: convergence and Empowermentor discrimination and crowding out? Agric. Tech. Econ. 5, 129–144. doi: 10.13246/j.cnki.jae.2023.05.007
Zhang, X., Wan, X., and Liu, P. (2023). The impact of participation in agricultural industry organizational models on crop yields: evidence from Chinese wheat growers. Sci. Rep. 13:17779. doi: 10.1038/s41598-023-43879-0
Zhang, Z., and Yi, Z. (2015). Research on the impact of agricultural productivity service outsourcing on Rice productivity: an empirical analysis based on 358 rural households. Issues Agricul. Econ. 36, 69–76. doi: 10.13246/j.cnki.iae.2015.10.009
Zhang, Y., Yin, Y., Li, F., Duan, W., Xu, K., and Yin, C. (2023). Can the outsourcing improve the technical efficiency of wheat production with fertilization and pesticide application? Evidence from China. J. Clean. Prod. 422:138587. doi: 10.1016/j.jclepro.2023.138587
Zhao, Q., Bao, H. X., and Zhang, Z. (2021). Off-farm employment and agricultural land use efficiency in China. Land Use Policy 101:105097. doi: 10.1016/j.landusepol.2020.105097
Zhao, X., Zhang, Z., and Ren, J. (2021). Does agricultural productive service affect Farmers' income? Empirical analysis based on 800 administrative villages using the propensity score matching model. Agric. Tech. Econ. 1, 32–45. doi: 10.13246/j.cnki.jae.2021.01.003
Keywords: agricultural productive services, farmers’ grain profits, the sustainability of grain production, endogenous switching regression, causal mediation analysis
Citation: Han G, Cui W, Chen X and Gao Q (2024) The sustainability of grain production: the impact of agricultural productive services on farmers’ grain profits. Front. Sustain. Food Syst. 8:1430643. doi: 10.3389/fsufs.2024.1430643
Edited by:
Tibebu Kassawmar, Addis Ababa University, EthiopiaReviewed by:
Zewdu Getachew, Madda Walabu University, EthiopiaBin Huang, Sichuan Agricultural University, China
Meseret Chanie Abate, Hunan University of Science and Engineering, China
Copyright © 2024 Han, Cui, Chen and Gao. This is an open-access article distributed under the terms of the Creative Commons Attribution License (CC BY). The use, distribution or reproduction in other forums is permitted, provided the original author(s) and the copyright owner(s) are credited and that the original publication in this journal is cited, in accordance with accepted academic practice. No use, distribution or reproduction is permitted which does not comply with these terms.
*Correspondence: Wenchao Cui, cuiwenchao@njfu.edu.cn