- 1State Key Laboratory of Aridland Crop Science, Lanzhou, China
- 2College of Agronomy, Gansu Agricultural University, Lanzhou, China
- 3Pratacultural College, Gansu Agricultural University, Lanzhou, China
Reducing carbon emissions from agricultural soils contributes to global greenhouse mitigation. Although the integration of no-tillage practices into maize/pea intercropping systems can achieve this reduction, the specific microbial mechanisms involved remain unclear. This study aimed to explore the effects of integrating maize/pea intercropping and no-tillage technologies on soil carbon emissions and microbial communities. The results indicated that intercropping no-till maize with peas reduced the average soil respiration rates by 19%. In 2021 and 2022, intercropping no-till maize with peas decreased soil carbon emissions by 25.1 and 30.4%, respectively. This practice resulted in a reduction of soil microbiota carbon and nitrogen by 26.9 and 19.7%, respectively, while simultaneously increasing the soil microbial gene beta diversity. Proteobacteria, Actinobacteria, Planctomycetes, Firmicutes, Bacteroidetes, and Acidobacteria collectively represented over 95% of the population and were predominant across all treatments. Intercropping no-till maize with peas decreased the abundance of carbohydrate-active enzymes in the soil. The structural equation modeling indicated that combined no-tillage and intercropping practices effectively decreased soil carbon emissions by modulating the community structure of soil microorganisms. This affected the abundance of carbohydrate-active enzymes and carbon-metabolizing genes in the soil. This study indicated that no-tillage and intercropping methods contributed to carbon reduction by influencing soil microbes. This study can provide microbial-level insights for refining agronomic practices to mitigate soil carbon emissions.
1 Introduction
In recent years, climate and environmental changes caused by greenhouse gas (GHG) emissions have received increasing attention. Agriculture is the second largest source of emissions after industry (Rahman et al., 2021; Pu et al., 2022; Yang et al., 2023). The annual agricultural GHG emissions in China are ~800 million tons, accounting for 59% of the agrarian soil GHG emissions (Liu et al., 2022; Xia et al., 2023). Soil respiration serves as the main pathway for soil carbon emissions, releasing soil carbon into the atmosphere as carbon dioxide through biological metabolism and other processes (Huang et al., 2020; Tian et al., 2022). Therefore, a thorough investigation of soil carbon reduction technologies and mechanisms presented an urgent challenge for realizing sustainable agricultural development.
The soil carbon emission reduction in farmland production can be achieved through optimizing management measures (Qiu et al., 2023; Zheng et al., 2023). Studies have indicated that techniques such as wet-dry alternation and drip irrigation (Bo et al., 2022; Wang C. et al., 2023), application of biochar (Xia et al., 2023), optimized cropping patterns (Chai et al., 2021; Wang W. et al., 2023; Yang et al., 2023), and conservation tillage (Rahman et al., 2021; Zhang et al., 2022; Yue et al., 2023) are effective methods for reducing emissions. Some of these methods can directly control soil carbon emissions, whereas others can indirectly mitigate emissions by enhancing soil carbon pools (Du et al., 2024; Sun et al., 2024). Combining one or more of these techniques in modern agriculture is conducive for low-carbon agriculture (Huang et al., 2019; Zhang et al., 2023). Among these, crop intercropping and conservation tillage have demonstrated significant potential for maintaining stable soil carbon pools and reducing soil carbon emissions (Shang et al., 2021).
Intercropping utilizes the ecological niches of different crops to reduce soil carbon emissions and enhance resource efficiency (Chai et al., 2021; Wang W. et al., 2023). Research has indicated that intercropping maize with peas, coupled with nutrient management, decreases greenhouse gas emissions compared to monocropping maize. Similarly, mulching and intercropping in maize/wheat systems can reduce soil CO2 emissions by 20% (Yang et al., 2023). Additionally, strategies such as rotating crops between bands, enhancing crop diversity, and integrating intercropping with conservation tillage also contribute to soil carbon emissions reduction (Chai et al., 2021; Gou et al., 2022). However, excessive human intervention in farmland ecosystems may destabilize them (Wang et al., 2020b; Zhao et al., 2023). Conservation tillage, recognized globally as a low-intervention agricultural practice, effectively reduces soil carbon emissions and promotes sustainable agriculture (Zhang et al., 2021, 2022; Ruis et al., 2022). Conservation tillage primarily reduces soil carbon emissions in two ways. First, it reduces soil greenhouse gas emissions by minimizing soil disturbance and altering substrate quantity or quality, thereby affecting the structure, function, and stability of soil microbial communities (Li et al., 2020; Kan et al., 2022; Zhao et al., 2022). Second, conservation tillage enhances soil carbon pools by improving soil structural stability, thereby reducing soil carbon emissions (Kopecký et al., 2021; Zheng et al., 2023). Studies have indicated that no-tillage mitigates soil respiration responses to climate change, promoting CO2 accumulation in soil and reducing CO2 emissions to the atmosphere (Zhang et al., 2022; Pearsons et al., 2023). Combining intercropping with no-tillage decreases soil disturbance and improves soil carbon pool stability to provide the potential for further soil carbon emission reduction in farmland.
Agricultural soils emit CO2 through respiration, which involves soil plants, animals, and microbial respiration (Jansson and Hofmockel, 2020; Zhang et al., 2021; Ren et al., 2022; Sánchez-Navarro et al., 2023). Previous studies have elucidated soil respiration mechanisms in agricultural soils by examining the effects of farming practices on soil conditions and crops (Yi et al., 2022; Liang et al., 2024). Soil microorganisms influence the fixation and release of soil carbon by participating in the decomposition and mineralization of organic matter (Bahram et al., 2018; Baldrian, 2019). For example, bacteria and fungi decompose organic matter, converting complex organic molecules into simpler compounds and ultimately releasing CO2 (Bahram et al., 2018; Baldrian, 2019; Domeignoz-Horta et al., 2020). Additionally, certain microbial communities have the potential to increase soil carbon storage, these microorganisms enhance the stability of soil carbon pools by producing stable organic substances such as humus (Lal et al., 2015; Zheng et al., 2022). Furthermore, microorganisms interact with plant roots, affecting carbon inputs from plants and root respiration (Jian et al., 2022). For instance, rhizosphere microorganisms can promote plant growth and root development, increasing the fixation of atmospheric CO2 by plants and thus reducing the release of CO2 from the soil (Jian et al., 2022; Ren et al., 2022). The advancements in high-throughput sequencing have facilitated deeper investigations of soil microorganisms within the carbon cycle (Tian et al., 2022; Zheng et al., 2022). Although research has linked soil microorganisms to the reduction of soil GHG emissions through farming practices, it has predominantly focused on relationships such as nitrifying microorganisms with N2O and methanogenic bacteria with methane emissions (Lin et al., 2023; Junwei et al., 2024). The microbiological mechanisms underlying carbon emission reduction in farmland soils from the combined practice of intercropping and no-tillage remain poorly understood.
Hence, this study was conducted in Northwest China using a maize-pea intercropping model integrating no-tillage practices. It monitored CO2 emissions resulting from the combination of farming techniques and analyzed soil enzyme activities and microbial composition. The objective of this study was to offer microbiological insights into how intercropping combined with no-tillage affected CO2 emissions. This study proposed the following hypotheses. (i) Integrating no-tillage with intercropping can further decrease soil CO2 emissions. (ii) These practices can reduce soil CO2 emissions by altering soil microorganisms.
2 Materials and methods
2.1 Site description
The experiment was conducted at the Oasis Agricultural Experiment Station of the Gansu Agricultural University in Wuwei City, Gansu Province, China (103°5′ E, 37°30′ N). This region experienced a temperate continental climate (Figure 1), with total solar radiation reaching 6,000 MJ·m−2 and an average annual sunshine duration exceeding 2,945 h. The annual average temperature was 7.2°C, with cumulative annual temperatures of ≥0°C totaling 3,513°C, and ≥10°C totaling 2,985.4°C. The annual rainfall remained below 160 mm, while the annual evaporation exceeded 2,400 mm. The soil was classified as Aridisol (Zhao et al., 2019), comprising 27.1% sand, 67.5% silt, and 5.4% clay, with a soil capacity of 1.57 g·cm−3 at a depth of 0–20 cm. Additionally, the total nitrogen, phosphorus, organic matter, and pH in the 0–20 cm soil layer were 0.68, 1.41, 8.31, and 8.3 g·kg−1, respectively. Strip intercropping, a high-yielding and efficient pattern, has been extensively practiced in this region over the long term.
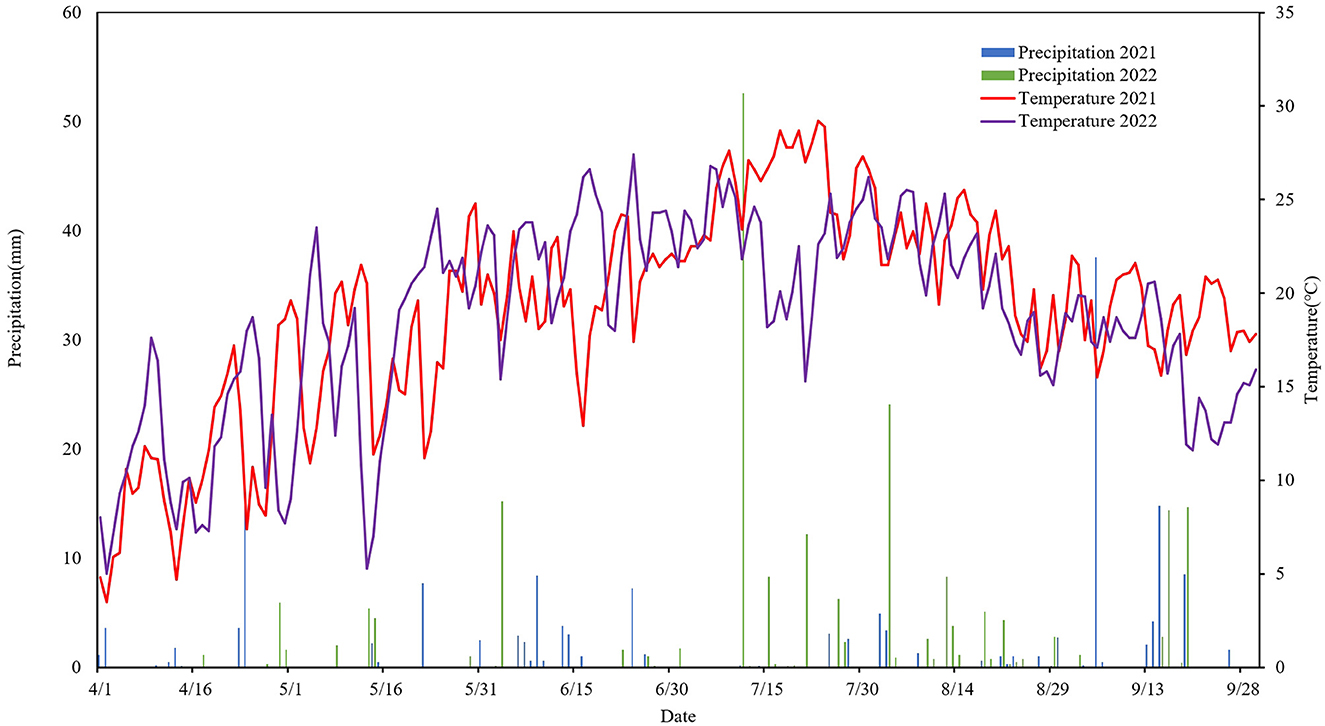
Figure 1. Changes in precipitation and temperature during the growing period of the crop in the test area between 2021 and 2022.
2.2 Experimental design and field management
The experiment commenced in 2016, this plot was part of a farm that had been under long-term maize cultivation with a high input of pure nitrogen (480 kg ha−1) prior to 2016. Adopting a split-zone trial design with three planting patterns: maize monoculture, pea monoculture, and maize/pea intercropping, along with two tillage modes: conventional tilling and no-tillage. There were five treatments, each replicated thrice, represented by the codes “CM, NM, CI, NI, SP” which are described as follows: CM (Conventional Tillage Maize) refers to maize monoculture under conventional tillage conditions. NM (No-tillage Maize) represents maize monoculture under no-tillage conditions. CI (Conventional Tillage Intercropping) indicates intercropping of maize and pea under conventional tillage. NI (No-tillage Intercropping) refers to intercropping of maize and pea under no-tillage. SP (Sole Pea) represents the monoculture of pea. Conventional tilling involved annual plowing to a depth of 30 cm after maize harvest, followed by leveling, fertilization, harrowing, and mulch application (0.01 mm thickness) the following spring. In contrast, the no-tillage treatment omitted tillage until maize was sown each year.
In the intercropping system, peas were planted in four rows spaced 80 cm apart, with 20 cm spacing between seeds, and a seeding rate of 11.7 kg·ha−1. Maize was planted in three rows, spaced 110 cm apart, with 40 cm spacing between seeds, and a seeding density of 37,000 plants·ha−1. Monocropped maize was planted at a density of 60,000 plants·ha−1, with 40 cm spacing, and monocropped peas were planted at a rate of 30 kg·ha−1. The data collection for this study was conducted in 2021 and 2022.
The maize (Zea mays L.) varieties, such as “Xian Yu 335,” are typically sown around April 20 annually and harvested around September 30 of the same year. Pea (Pisum sativum Linn.) varieties, such as “Long Wan 1,” are sown each year around April 1 and harvested around July 10 of that year.
The maize was provided with 360 kg·ha−1 of pure nitrogen, which was distributed as follows: 30% as a basal fertilizer, 50% at maize flowering, and the remaining 20% at maize filling. Additionally, 180 kg·ha−1 of pure phosphorus was applied as a basal fertilizer. Peas received 63 kg·ha−1 of pure nitrogen during their reproductive period and were all applied as basal fertilizers. Under the intercropping system, maize received 220 kg·ha−1 of pure nitrogen, following the same application regime as monoculture maize, and 110 kg·ha−1 of pure phosphorus was applied entirely as a basal fertilizer. Peas received 24.5 kg·ha−1 of pure nitrogen, applied entirely as a basal fertilizer.
2.3 Measurements
2.3.1 Soil sampling
The soil samples were collected in 2021 and 2022 during the intercropping system crop symbiosis, along with 2 weeks after the harvest of the early crop. Each plot was randomly collected three times, soil was sampled from depths of 5–20 cm, and subsequently mixed into one composite sample. The soil samples were collected separately from maize ribbon and pea ribbon strips within the intercropping setup. No soil samples were collected after the harvest of peas in the monocropping pea treatment. This process yielded a total of 78 soil samples, these samples were primarily collected from the gaps between crop growth and did not specifically target the rhizosphere soil. Subsequently, the samples were sieved through a two-millimeter sieve to eliminate plant root residue and impurities. Certain samples were stored in a 4°C incubator for soil enzyme activity and microbial carbon and nitrogen measurements, whereas others were stored in an ultra-low temperature refrigerator at −78°C for macro-genome sequencing.
2.3.2 Soil respiration
The soil respiration rate was assessed using a soil carbon flux meter (LI-8100A; LI-COR, USA). Before the measurement, a ring base matching the air chamber was buried 2 cm deep in the soil in each plot. Within the intercropping system, one base was buried in each crop strip. The measurements were conducted separately for the maize and pea belts of the intercropping system, with the weighted average of the measured data representing the soil respiration rate of one plot. Each plot was measured at 15-day intervals post-pea planting, followed by 3 consecutive days of measurements at 2-h intervals per day to determine the average respiration rate for that period. Additional measurements were adopted at the time of soil sample collection.
2.3.3 Carbon emission
Carbon emission (CE, kg ha−1) was calculated from soil respiration (Rs, μmol m−2 s−1) using the equation outlined by:
where CE is the total soil CO2-C emissions (kg C ha−1), Rs is the soil respiration rate (μmol CO2 m−2 s−1), i+1 and i are the dates of the two soil respiration measurements, respectively, t is the number of days after sowing, 0.1584 is the conversion factor of unit μmol CO2 m−2 s−1 to unit g CO2 m−2 h−2, 0.2727 is the conversion factor of unit g CO2 m−2 h−2 to unit g C m−2 h−2, and 24 and 10 are the conversion factors of unit g C m−2 h−2 to unit kg C ha−1.
2.3.4 DNA extraction and sequencing
DNA extraction from the microbial community was conducted using the NucleoSpin Soil Kit (Macherey-Nagel, Germany) following the manufacturer's instructions. The DNA quantification was performed using a Qubit Fluorometer with a Qubit dsDNA BR Assay kit (Invitrogen, USA), and the quality was checked by analyzing an aliquot on a 1% agarose gel.
Library construction: 1 μg of genomic DNA was randomly fragmented by Covaris, followed by the selection of the fragmented DNA to an average size of 200–400 bp using magnetic beads. The selected fragments then underwent end-repair, 3′-adenylation, adapter ligation, and PCR amplification, with subsequent purification of the products using magnetic beads. The double-stranded PCR products were heat-denatured and circularized using a splint oligo sequence. The resulting single-strand circle DNA (ssCir DNA) constituted the final library, which was qualified by QC before sequencing on the MGISEQ-2000 platform (BGI-Shenzhen, China).
2.3.5 Soil carbohydrate-active enzyme assays
The raw data were trimmed using SOAPnuke v.1.5.2. High-quality reads were then assembled de novo using Megahit software. Contigs with lengths < 300 bp were excluded from subsequent analyses (Chen et al., 2018). Gene prediction was performed over contigs using Meta-Gene Marker (2.10), followed by removal of redundant genes using CD-HIT with an identity cutoff of 95%. Taxonomic information was generated by aligning protein sequences of genes against the NR database using DIAMOND with an E-value cutoff of 1 e−5. Soil carbohydrate-active enzyme data were obtained by comparative annotation of the acquired DNA data from the Carbohydrate-Active Enzymes Database (Li et al., 2001; Zhu et al., 2010).
2.3.6 Statistical analyses
Before the data analysis, the normal distribution of the obtained data was assessed. The one-way analysis of variance (ANOVA, P < 0.05) was conducted using SPSS 26.0 to assess the variability of soil respiration, soil microorganisms, and CAZyme families among treatments. The two-way ANOVA was employed to examine the effects of no-tillage and intercropping on these indices and their interaction effects. The relationships between soil microorganisms and carbon metabolism, their functional genes, CAZyme abundance, and soil respiration were investigated using Pearson analysis and the Mantel test. Furthermore, structural equation modeling of soil respiration, soil microorganisms, functional genes of carbon metabolism, and CAZyme was performed using the lavaan software package in R.
3 Results
3.1 Soil respiration
3.1.1 Seasonal variations in soil respiration rates
In 2021 and 2022, intercropping and no-tillage treatments significantly decreased the average soil respiration rate during crop fertility (P < 0.001; Figure 2A). However, the interaction between intercropping and no-tillage differed between years. In 2021, this interaction significantly reduced the average soil respiration rate during crop fertility (P < 0.001), whereas by 2022, the reduction was not significant (P = 0.067). Specifically, in 2021, the intercropping system reduced mean soil respiration rates by 10.1–27.3% compared to monocropping, while no-till reduced rates by 4.2–22.6% compared to conventional tillage. Additionally, the NI treatment lowered the mean soil respiration rate by 30% compared to the CM treatment. In 2022, the intercropping system reduced mean soil respiration rates by 13.9–7.6% compared to monocropping. No-till decreased average soil respiration rates by 6.4–12.8% compared to conventional tillage, and the NI treatment reduced rates by 19% compared to the CM treatment.
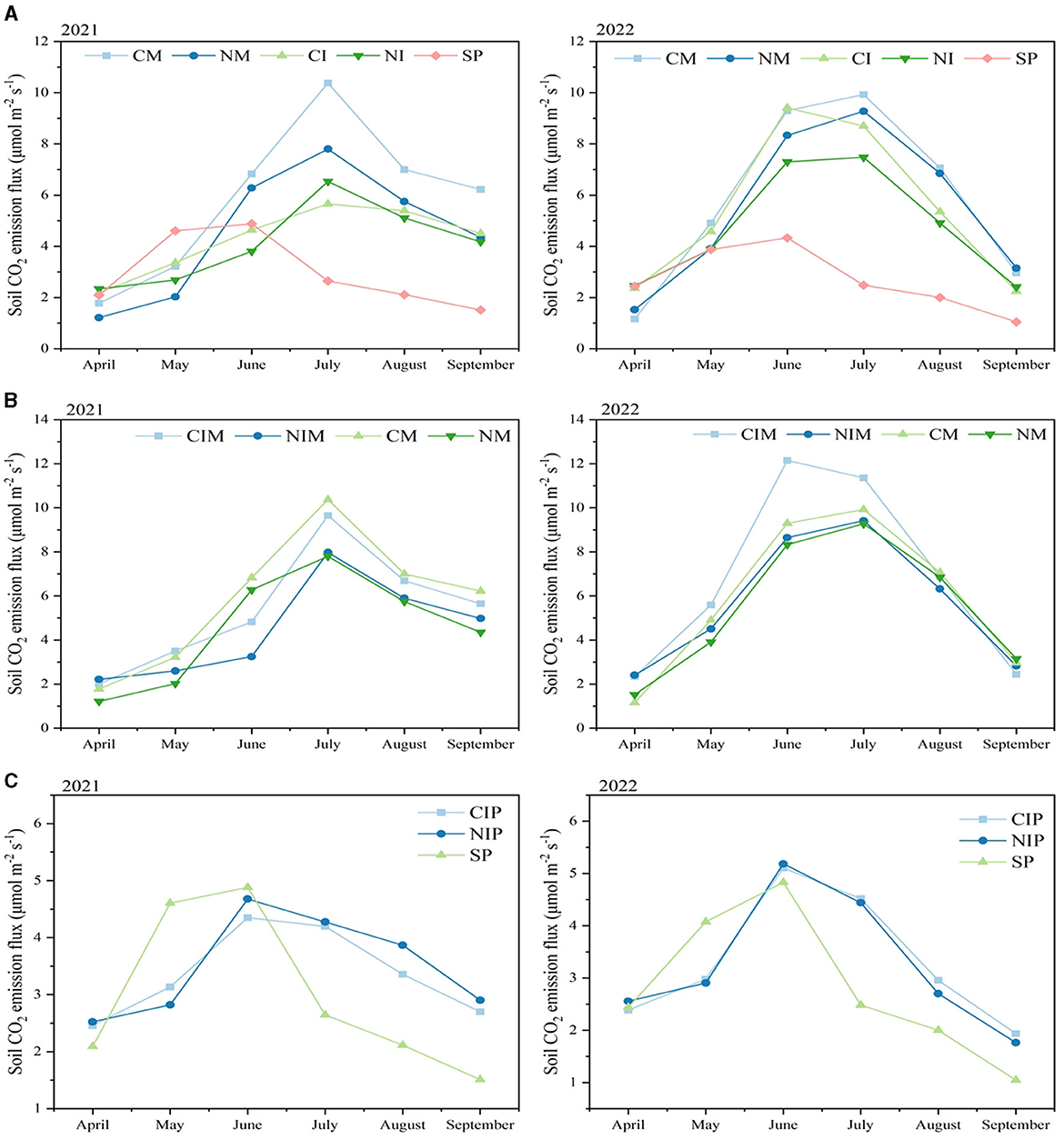
Figure 2. (A) Mean soil respiration rate of the system under different tillage practices and cropping patterns. (B) The soil respiration rate of maize in the maize strip and monoculture under different tillage practices and intercropping. (C) The soil respiration rate of peas in the pea strip and monoculture under different tillage practices. CM (Conventional Tillage Maize) refers to maize monoculture under conventional tillage conditions. NM (No-tillage Maize) represents maize monoculture under no-tillage conditions. CI (Conventional Tillage Intercropping) indicates intercropping of maize and pea under conventional tillage. NI (No-tillage Intercropping) refers to intercropping of maize and pea under no-tillage. SP (Sole Pea) represents the monoculture of pea. CIM (Conventional Tillage Intercropping with Maize) refers to intercropping under conventional tillage with an emphasis on maize. NIM (No-tillage Intercropping with Maize) denotes intercropping under no-tillage with an emphasis on maize. CIP (Conventional Tillage Intercropping with Pea) indicates intercropping under conventional tillage with an emphasis on pea. NIP (No-tillage Intercropping with Pea) represents intercropping under no-tillage with an emphasis on pea.
The monthly average soil respiration rates during the reproductive period were analyzed to evaluate seasonal variations (Figure 2A). The maximum soil respiration rates for both monocrop maize and maize-intercropped pea systems were observed in July. In June, July, and August 2021, the NI treatment significantly reduced the mean soil respiration rates by 55.7, 63.0, and 73.0%, respectively, compared with the CM treatment. Similarly, in June, July, and August 2022, the NI treatment significantly decreased the mean soil respiration rates by 21.5, 24.6, and 30.4%, respectively, compared to the CM treatment. Although the maximum soil respiration rate in the SP treatment occurred in June, the rate started to decline after the pea harvest.
3.1.2 Spatial differences in soil respiration rates
3.1.2.1 Maize strip
Intercropping and no-tillage significantly reduced the average soil respiration rate during crop fertility in the Maize Belt (P < 0.001), and the interaction effect of intercropping and no-tillage also significantly reduced the average soil respiration rate in 2021 (P < 0.001) and 2022 (P = 0.030; Figure 2B). Over the 2-year period, the mean soil respiration rate in the NI-treated Maize Belt (5.09 μmolm−2 s−1) was 13.7% lower than that in the CM-treated maize belt (5.90 μmolm−2 s−1).
Before pea harvest, the 2-year average soil respiration rate in the NIM was 13.1% lower (3.94 μmolm−2 s−1) compared to the CM Maize Belt (4.53 μmolm−2 s−1), while after pea harvest, it was 14.1% lower (6.24 μmolm−2 s−1) compared to the CM Maize Belt (7.26 μmolm−2 s−1; Figure 2B). The most significant difference in soil respiration rate between the NIM and CM Maize Belt occurred in June, with a 2-year average decrease of 29.7% in NIM compared to the CM Maize Belt. In 2022, intercropping increased CIM soil respiration rates, with the maximum increase compared with the CM Maize Belt, reaching 30.7% in June.
3.1.2.2 Pea strip
In the investigation of soil respiration rates within the pea belt, it was observed that intercropping decreased the soil respiration rates before the pea harvest, followed by an increase after the pea harvest (Figure 2C). Peak soil respiration rates in the pea zone were observed in June for both years, reaching 4.51 and 5.15 μmol m−2 s−1, respectively. No tillage had a significant effect on soil respiration in the pea zone within the intercropping system. The greatest disparity in soil respiration rates between the intercropped pea belt and sole pea belt before pea harvest occurred in May, with reductions of 35.3 and 27.8% observed in 2021 and 2022, respectively.
The intercropped pea belt exhibited higher soil respiration rates after pea harvest than solo peas (Figure 2C). In 2021, the intercropped pea belt increased soil respiration rates after the harvest by an average of 69.8% compared to the solo pea belt. In 2022, this increase was 65.5%. Over the entire crop reproductive period, intercropping increased the average soil respiration rates of solo peas by 15.6 and 16.8% in 2021 and 2022, respectively. Although intercropping reduced pre-harvest soil respiration rates in peas, it augmented soil respiration rates in the pea belt post-harvest and throughout the crop fertility period.
3.1.2.3 Differences between intercropped maize and pea strips
In the examination of soil respiration rates within the maize and pea belts of the intercropping system, soil respiration in the maize belt surpassed that of the pea belt significantly, emerging as the primary contributor to soil respiration in the intercropping system (Figure 2). In 2021 and 2022, soil respiration rates were 44.8 and 105.0% higher in the CIM treatment than in the CIP treatment, and 27.8 and 74.5% higher in the NIM treatment than in the NIP treatment, respectively. Before the pea harvest in 2021, soil respiration rates for maize and pea belts were not significantly different. However, after the pea harvest in 2021, CIM exhibited an 84.4% higher soil respiration rate than CIP and NIM showed a 70.7% higher soil respiration rate than NIP. However, post-harvest, CIM exhibited an 84.4% increase over CIP, and NIM showed a 70.7% increase over NIP. Conversely, in 2022, soil respiration rates in the maize belt were notably higher than those in the pea belt before pea harvest, with increases of 91.8 and 46.1% under conventional tillage and no-till conditions, respectively. After pea harvest, these differences increased to 119.7 and 108.5%, respectively.
3.2 Soil carbon emissions
3.2.1 Differences in total emissions
Both intercropping and no-tillage significantly decreased overall total soil carbon emissions (P < 0.001; Figure 3A). Over a 2-year period, intercropping reduced total soil carbon emissions by 20.0 and 14.0% under conventional tillage and no-tillage, respectively, compared to monocropping. Furthermore, no-tillage, whether under intercropping or monocropping conditions, led to reductions of 9.7 and 15.9%, respectively, compared with conventional tillage. Specifically, the NI treatments achieved reductions of 25.1 and 30.4% in total soil carbon emissions compared with the CM treatments in 2021 and 2022, respectively. Consequently, combining intercropping with no-tillage could further decrease total soil carbon emissions.
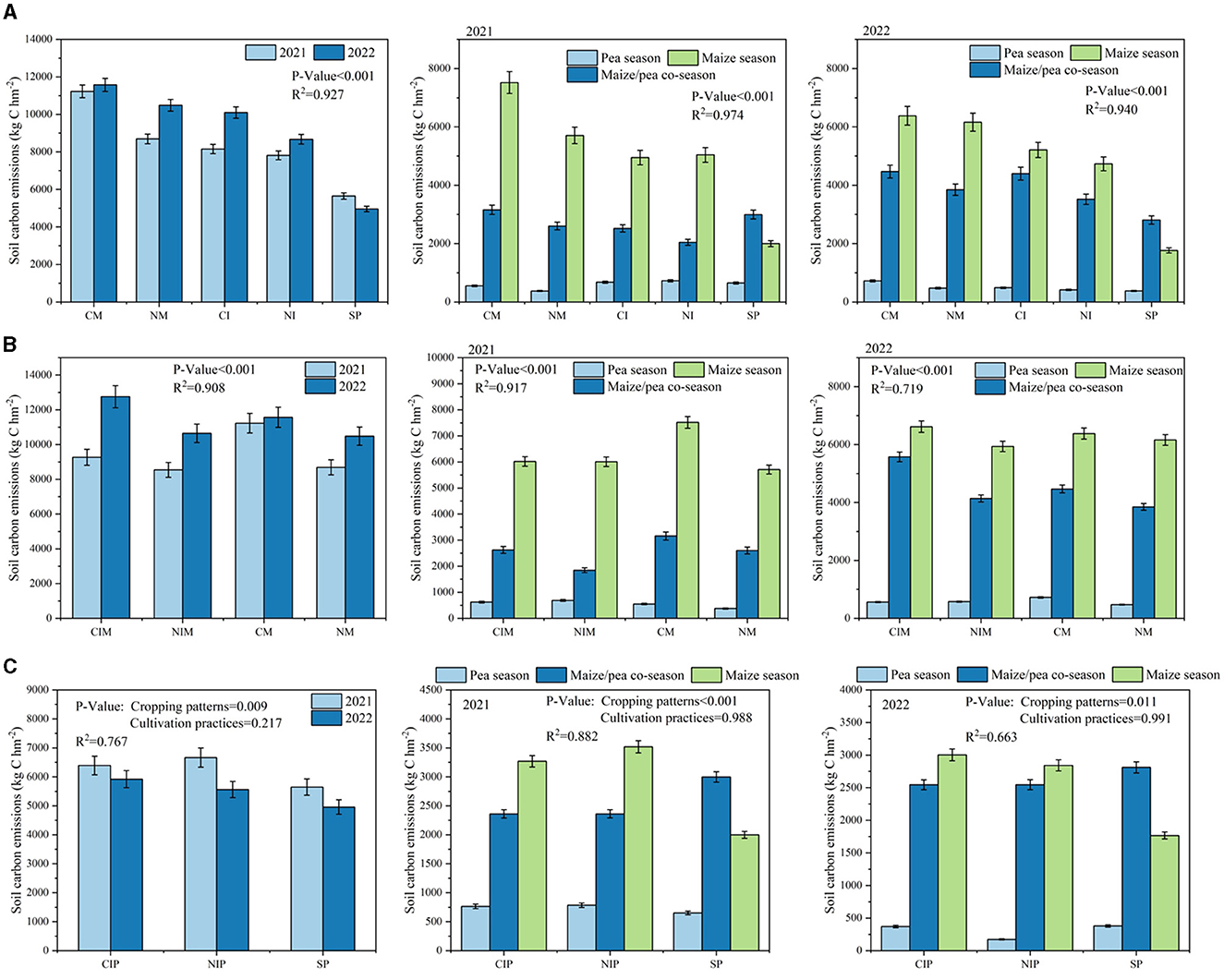
Figure 3. (A) Total soil carbon emissions from different tillage and cropping patterns. (B) Total soil carbon emissions from the corn belt under intercropping and no-tillage conditions and soil carbon emissions at different periods. (C) Total carbon emissions from the intercropped pea belt vs. the solo pea and soil carbon emissions at different periods. CM (Conventional Tillage Maize) refers to maize monoculture under conventional tillage conditions. NM (No-tillage Maize) represents maize monoculture under no-tillage conditions. CI (Conventional Tillage Intercropping) indicates intercropping of maize and pea under conventional tillage. NI (No-tillage Intercropping) refers to intercropping of maize and pea under no-tillage. SP (Sole Pea) represents the monoculture of pea. CIM (Conventional Tillage Intercropping with Maize) refers to intercropping under conventional tillage with an emphasis on maize. NIM (No-tillage Intercropping with Maize) denotes intercropping under no-tillage with an emphasis on maize. CIP (Conventional Tillage Intercropping with Pea) indicates intercropping under conventional tillage with an emphasis on pea. NIP (No-tillage Intercropping with Pea) represents intercropping under no-tillage with an emphasis on pea.
To comprehensively evaluate carbon emissions across different experimental stages, we divided the entire crop growth cycle into three periods: the pea season (before maize emergence), maize/pea co-season, and maize season (following pea harvest; Figure 3A). In 2021, intercropping and no-tillage significantly reduced soil carbon emissions in all three periods (P < 0.001). The interaction between the two had no significant effect on soil carbon emissions during the maize/pea co-season (P = 0.473). Similarly, in 2022, both intercropping and no-tillage reduced soil carbon emissions across all periods (P < 0.001). Over the 2-year average, the pea season contributed < 10% of the total soil carbon emissions, and the maize season contributed over 56.2%. In the monoculture pea treatment, the emissions during the maize/pea coexistence season accounted for 54.9% of the total emissions. Consequently, intercropping and no-tillage primarily reduced total carbon emissions by decreasing soil carbon emissions during the maize/pea symbiosis season and the subsequent maize season.
3.2.2 Maize strip
In the investigation of soil carbon emissions within maize strips, both intercropping and no-tillage significantly decreased total soil carbon emissions (P < 0.005), with the NIM treatments reducing emissions by 8.0 and 23.9% compared to CM maize strips (Figure 3B). In the 2021 pea season, intercropping increased soil carbon emissions in the maize strip (P < 0.001), and the no-tillage increased emissions in the intercropping system by 10.6% and decreased emissions in the monocropping system by 31.6%. Intercropping and no-tillage decreased emissions during the maize/pea and maize seasons (P < 0.001). In 2022, intercropping elevated emissions in the maize/pea co-season by 24.8%, and no-tillage reduced emissions in all three periods (P < 0.005). The soil carbon emissions during the maize season accounted for more than 64.9% (2021) and 51.9% (2022) of the total emissions. Therefore, despite the varying effects on emissions at different stages, overall, no-tillage and intercropping led to a reduction in total soil carbon emissions.
3.2.3 Pea strip
In contrast to maize strips, intercropping resulted in increased total soil carbon emissions in pea strips (P = 0.009), while tillage practices did not significantly affect soil carbon emissions in pea strips (P = 0.217; Figure 3C). Both CIP and NIP treatments led to an increase in soil carbon emissions by 16.1 and 15.3%, respectively, compared with SP. Intercropping reduced soil carbon emissions by 15.4% in the maize/pea co-season but increased emissions by 65.6% in the maize season. During the maize season, soil carbon emissions from the pea strip in the intercropping system accounted for over 50.7% of the total emissions, and the emissions from the solo pea strips were primarily concentrated in the maize/pea co-season, comprising 56.7% of the total emissions. Consequently, although intercropping can decrease soil carbon emissions in the maize/pea co-season, it may elevate emissions in the pea strip during the maize season.
3.3 Soil microbial communities
3.3.1 Soil microbiota and genetic diversity
To examine the potential impact of microorganisms on soil respiration, total soil microbial mass was initially evaluated (Figure 4A). The results revealed that intercropping decreased soil microbial carbon (P < 0.001), whereas no-tillage had the opposite effect, increasing soil microbial carbon (P = 0.002; Figure 4B). However, the interaction between intercropping and no-tillage did not significantly affect soil microbial carbon content (P = 0.720). Both intercropping and no-tillage led to reductions in soil microbial biomass nitrogen (P < 0.005), with no significant interaction between the two (P = 0.296). Specifically, microbial biomass carbon and nitrogen decreased by 26.9 and 17.9%, respectively, in the NI treatment compared with the CM treatment. Moreover, the analysis of microbial gene β-diversity in each treatment suggested that intercropping could enhance the gene β-diversity of soil microorganisms and increase the degree of gene variability within the group (Figure 4C).
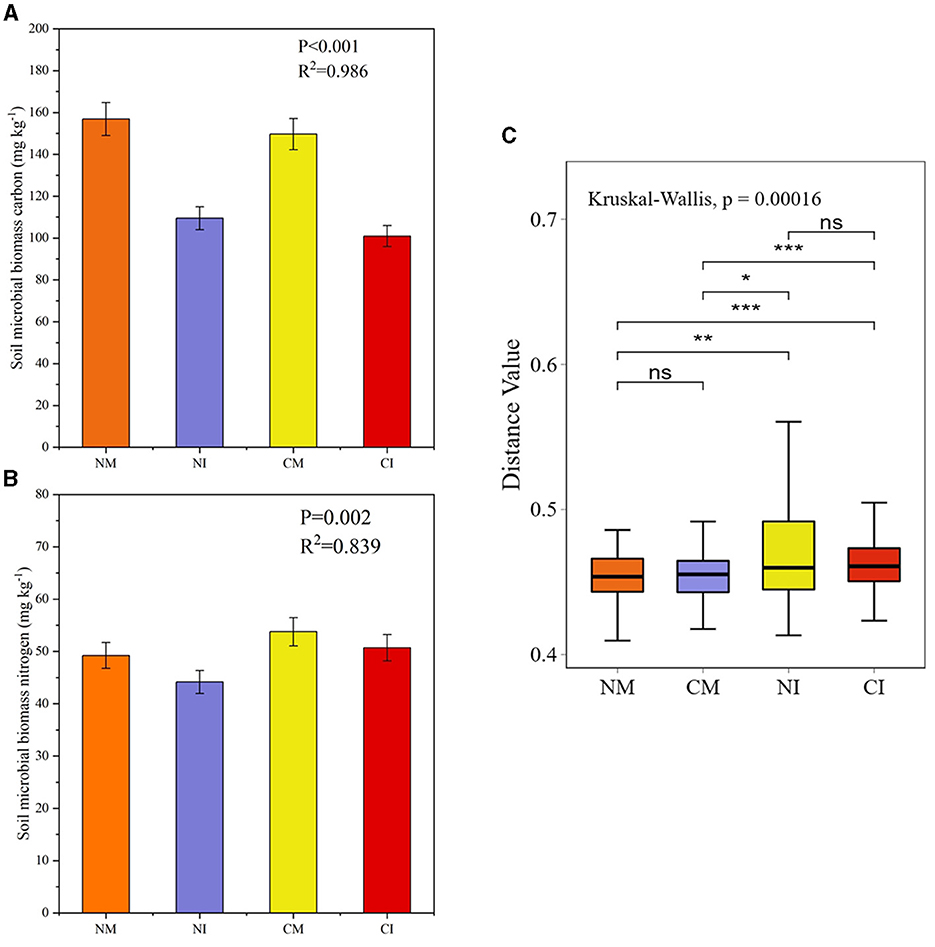
Figure 4. Soil microbiomass carbon (A), nitrogen (B), and differences in beta diversity of total gene volume (C). *Indicates a significant difference at the 0.05 level, **indicates a significant difference at the 0.01 level, and ***indicates a significant difference at the 0.001 level, and ns indicates no significant difference. CM (Conventional Tillage Maize) refers to maize monoculture under conventional tillage conditions. NM (No-tillage Maize) represents maize monoculture under no-tillage conditions. CI (Conventional Tillage Intercropping) indicates intercropping of maize and pea under conventional tillage. NI (No-tillage Intercropping) refers to intercropping of maize and pea under no-tillage.
3.3.2 Dominant communities of soil microorganisms
The structure of soil microbial communities was closely linked to soil respiration. The analysis of soil microbial α-diversity indicated that no-tillage and intercropping decreased the Simpson's and Shannon's indices within the group, but presented no significant impact on the Chao1 index (Figure 5). Consequently, no-tillage enhanced microbial community diversity in monoculture systems, whereas it decreased diversity in intercropping systems. Notably, no-tillage intercropping (NI) treatment reduced soil microbial community evenness. Both no-tillage and intercropping led to an increase in the soil microbial beta diversity index (Figures 6A, 7B). The annotation of microbial Kraken species in each treatment highlighted that microorganism predominantly belonging to Proteobacteria, Actinobacteria, Planctomycetes, Firmicutes, Bacteroidetes, and Acidobacteria comprised over 95% of the total, representing the dominant flora across all treatments (Figure 6B).
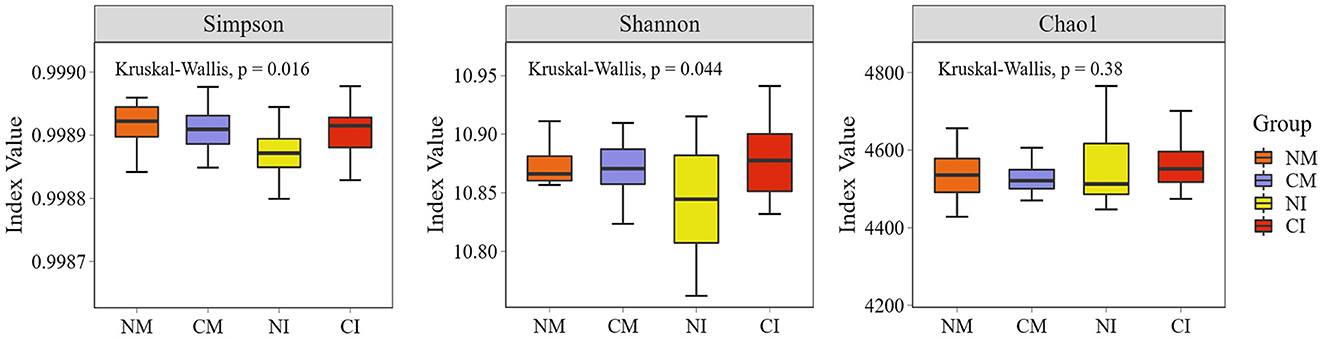
Figure 5. Box plots of species Alpha diversity. p < 0.05 indicates significant differences in the Alpha index between groups. CM (Conventional Tillage Maize) refers to maize monoculture under conventional tillage conditions. NM (No-tillage Maize) represents maize monoculture under no-tillage conditions. CI (Conventional Tillage Intercropping) indicates intercropping of maize and pea under conventional tillage. NI (No-tillage Intercropping) refers to intercropping of maize and pea under no-tillage.
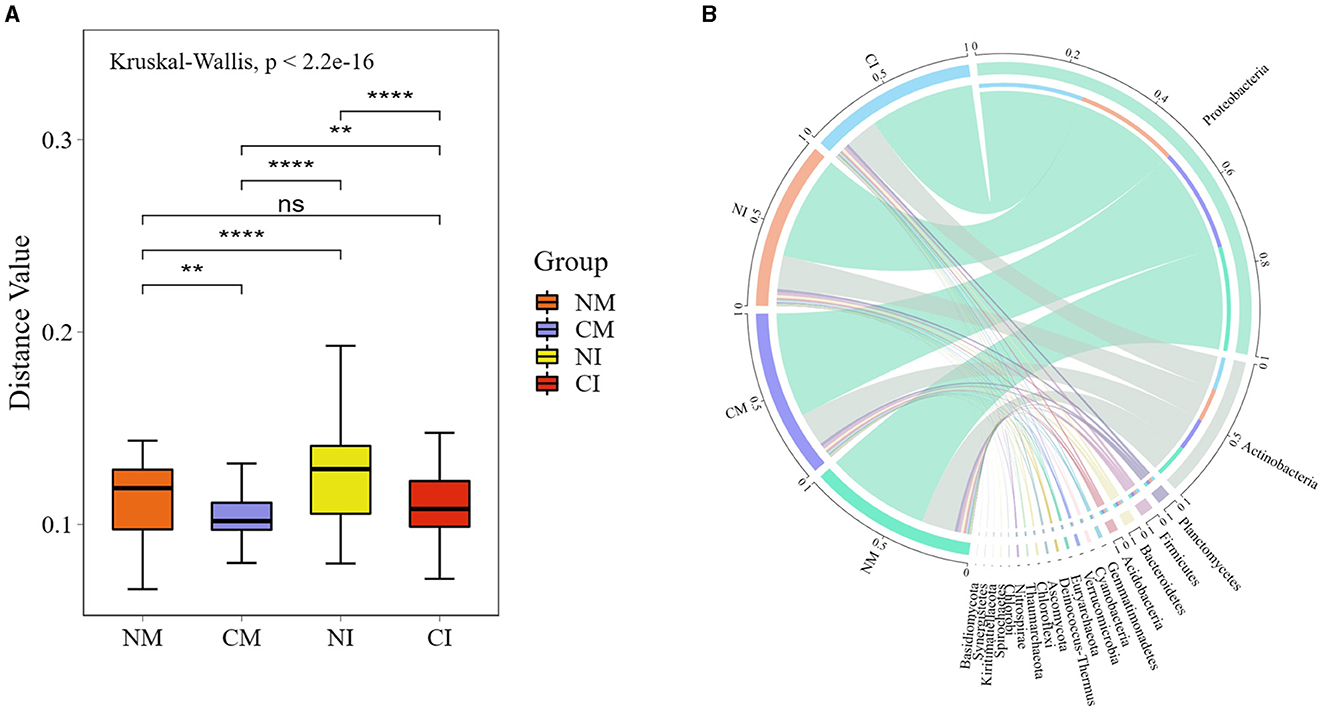
Figure 6. Species beta diversity boxplots and species distribution maps. (A) *Indicates a significant difference at the 0.05 level, **indicates a significant difference at the 0.01 level, ***indicates a significant difference at the 0.001 level, and **** indicates a significant difference at the 0.0001 level, and ns indicates no significant difference. (B) The circle is divided into two parts, left and right, with grouping information on the left and species classification information on the right. From the outside looking in, the outermost scale is the percentage of the species in the grouping or the proportion of different species in the grouping, the color of the inner arcs indicates the grouping/sample or the species, a connecting line between the arcs suggests the presence of the species in the sample, and the width of the line across the arcs indicates the percentage. CM (Conventional Tillage Maize) refers to maize monoculture under conventional tillage conditions. NM (No-tillage Maize) represents maize monoculture under no-tillage conditions. CI (Conventional Tillage Intercropping) indicates intercropping of maize and pea under conventional tillage. NI (No-tillage Intercropping) refers to intercropping of maize and pea under no-tillage.
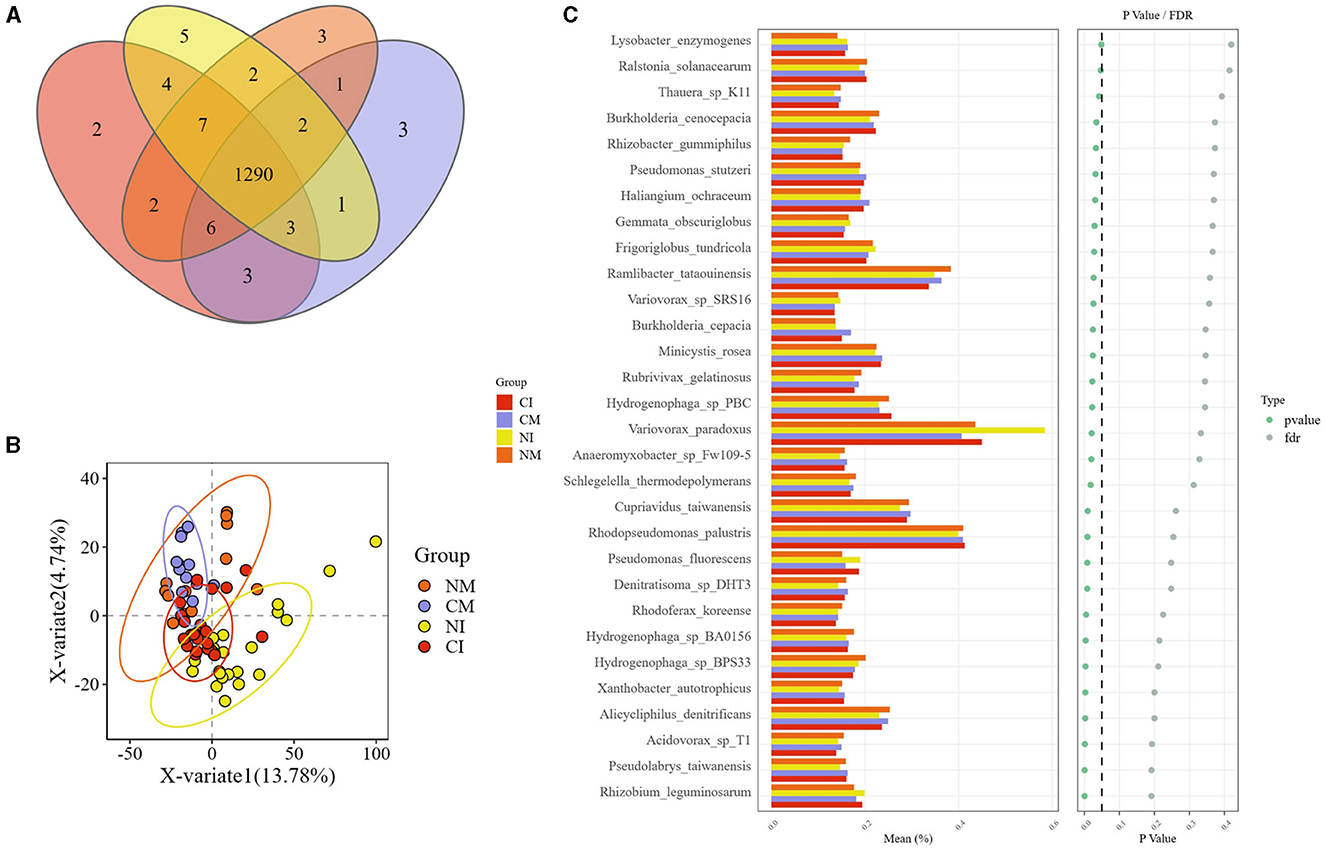
Figure 7. (A) Genus-level species difference Venn diagrams. Where each circle represents a subgroup, numbers in overlapping areas indicate the number of species common to two multiple subgroups, and numbers in non-overlapping areas indicate the number of species exclusive to that subgroup. (B) PLS-DA analysis scatterplot. Each point represents a sample, the color of the point indicates the grouping in which the sample is located, the horizontal and vertical coordinates indicate the PLS dimensions that separate the samples, the value in parentheses in the axis header is the rate of explanation of the samples by the downgraded dimensions, and the elliptical confidence intervals (which are only available for samples in a grouping of ≥4) are at a confidence level of 95%. (C) STAMP Extended histogram. In the left histogram, the vertical coordinate is the differing species, the horizontal coordinate is the grouped mean abundance (%), and the color of the bar represents the grouping; in the right scatterplot, the color of the dots indicates the results of different tests of significance, where the p-value is the result of the statistical test. FDR is the false discovery rate, a corrected p-value, and dots to the left of the dashed line in the plot (p < 0.05) indicate significant differences. CM (Conventional Tillage Maize) refers to maize monoculture under conventional tillage conditions. NM (No-tillage Maize) represents maize monoculture under no-tillage conditions. CI (Conventional Tillage Intercropping) indicates intercropping of maize and pea under conventional tillage. NI (No-tillage Intercropping) refers to intercropping of maize and pea under no-tillage.
The aforementioned microorganisms constituted the dominant flora across all treatments, with no discernible variation in their abundance. Therefore, identifying species that differ across treatments may offer greater insights into the microorganisms that mitigate carbon emissions. Notably, 1,290 species were identified at the genus level across all treatments. The CI treatment was characterized by the exclusive presence of Bibersteinia and Candidatus_Methanoplasma. Conversely, the CM treatment exhibited exclusivity to the genera Spartinivicinus, Anaerocolumna, and Actinobacillus genera. Unique to the NI treatment were genera Rickettsia, Arcobacter, Olleya, Shigella, and Wolbachia, absent in the NM treatment. Additionally, Oceanobacillus, Lachnospira, and Gillisia were exclusive to the NI treatment. Notably, the NI treatment featured significantly more exclusive genera than did the CM treatment (Figure 7A).
Through the STAMP analysis of microorganisms across treatments, 30 microorganisms exhibited significant differences, all belonging to the phyla Firmicutes, Thaumarchaeota, Armatimonadetes, and Candidatus_Omnitrophica. Firmicutes, and Thaumarchaeota had the highest numbers of distinct species (Figure 7C). Specifically, the NI treatment notably reduced Firmicutes levels, and the CI treatment increased the levels of Firmicutes and Thaumarchaeota. Despite identifying microorganisms with substantial differences between treatments, further analyses were required to determine their involvement in the soil carbon cycle, particularly regarding their functional genes.
3.3.3 Distribution and differences in functional genes of soil microorganisms
The β-diversity and PLS-DA analyses of soil microbial functions demonstrated that the NI treatment exhibited significantly greater soil microbial functional diversity than the other treatments (Figures 8A, B). The KEGG annotation of different gene functions indicated that the primary active genes of soil microbial functional genes were concentrated in the metabolism section, particularly within the global and overview maps. Genes related to carbohydrate metabolism, amino acid metabolism, and functional energy metabolism were the most prevalent (Figure 8C).
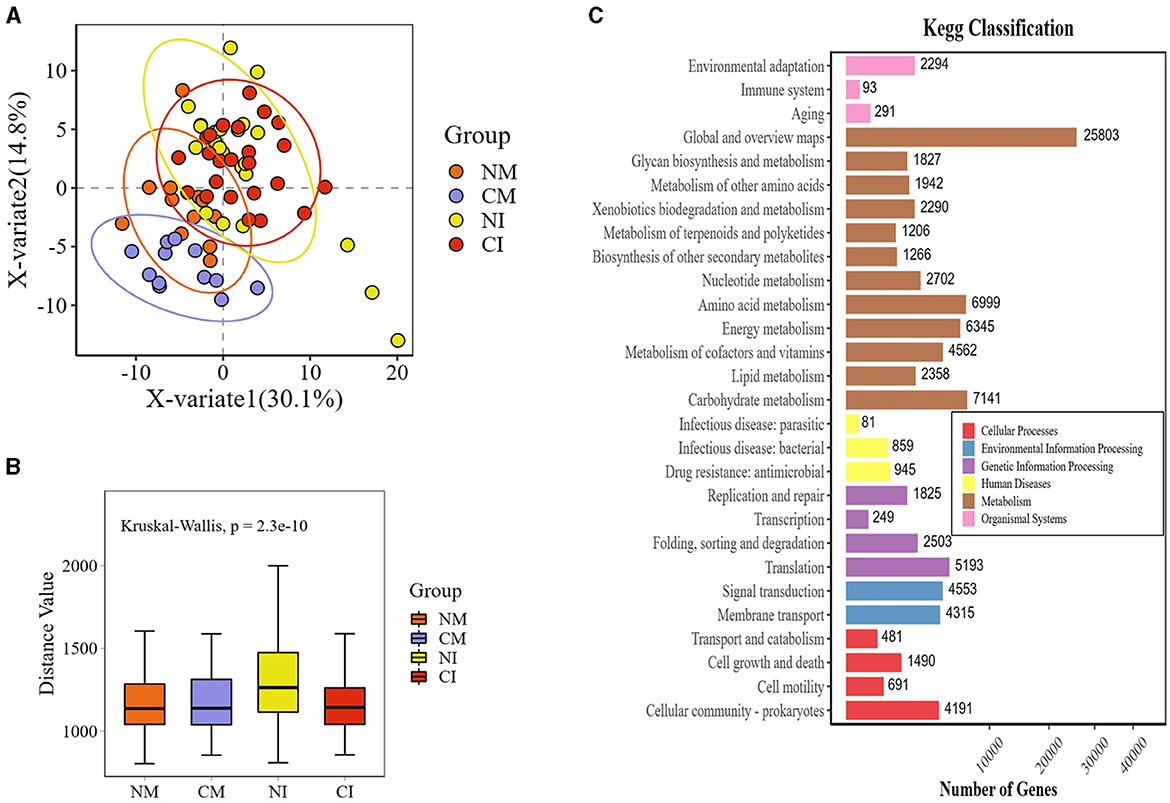
Figure 8. (A) PLS-DA analysis scatterplot. Each point represents a sample, the color of the point indicates the grouping in which the sample is located, the horizontal and vertical coordinates indicate the PLS dimensions that separate the samples, and the value in parentheses in the axis header is the rate of explanation of the samples by the downgraded dimensions, with an elliptical confidence interval at a 95% confidence level. (B) Function beta diversity box-and-line plot. Horizontal coordinates and box colors indicate different subgroups and vertical coordinates indicate the distance between samples. (C) Histogram of functional gene statistics. The horizontal coordinate is the number of genes; the vertical coordinate is the functional classification; the color of the bar indicates the grouping or functional classification; the length of the bar shows the number of genes; and the box on the right-side labels the first-level functional classification to which the second-level functional classification belongs. CM (Conventional Tillage Maize) refers to maize monoculture under conventional tillage conditions. NM (No-tillage Maize) represents maize monoculture under no-tillage conditions. CI (Conventional Tillage Intercropping) indicates intercropping of maize and pea under conventional tillage. NI (No-tillage Intercropping) refers to intercropping of maize and pea under no-tillage.
Functional genes were examined for intergroup variance using STAMP, and significant differences between treatments were identified (Figure 9A). A comparison with the KEGG database identified genes (K07795, K02078, K00249, K00549, K02277, and K00332) related to microbial carbon metabolism. Intercropping notably increased the abundance of the K02078 gene and decreased the abundance of other carbon metabolism genes. Furthermore, microorganisms harboring these functional genes, such as Candidatus_Omnitrophica and firmicutes, exhibited significant differences among the treatments (Figure 9B).
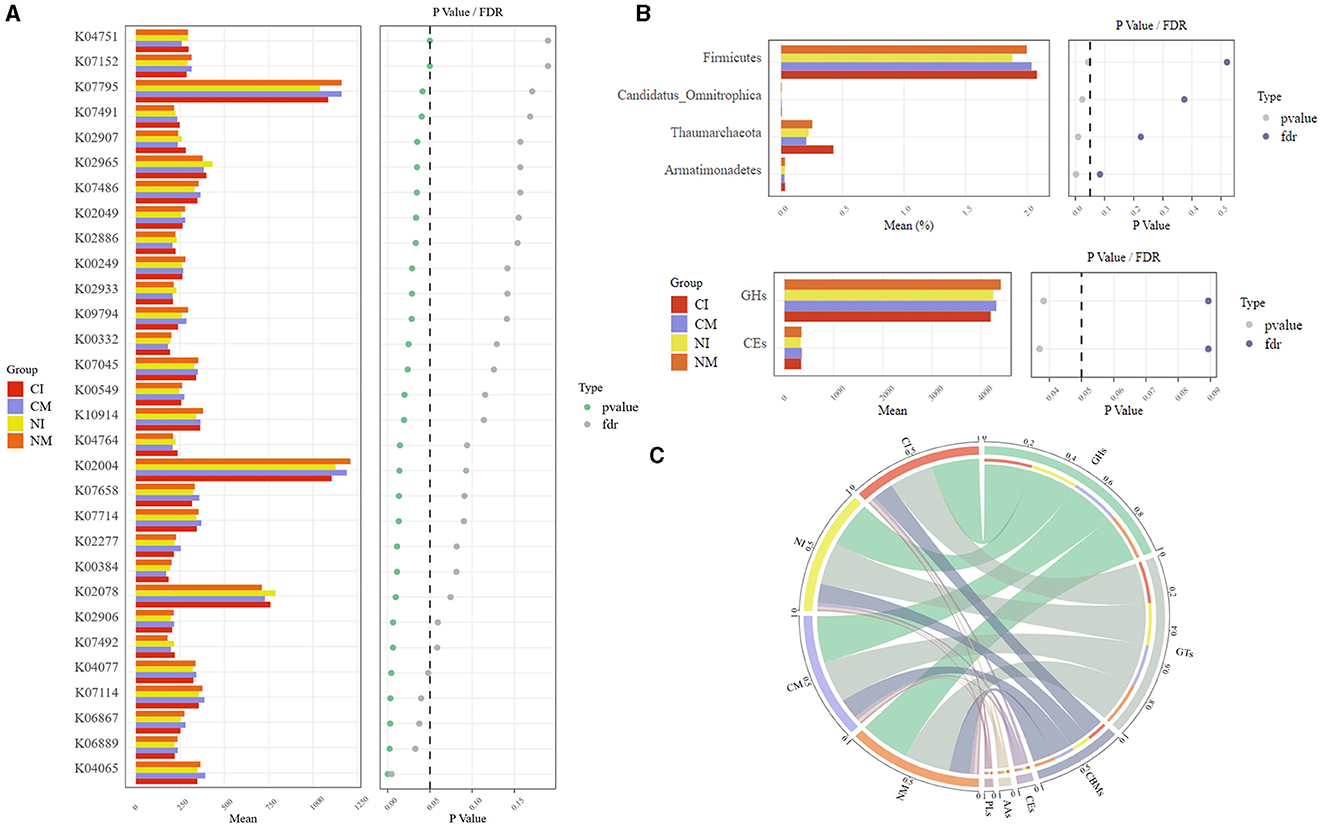
Figure 9. (A) Differential functional genes between treatments STAMP Extended histogram. (B) Differences between treatments Microbial and carbohydrate genes STAMP Extended histogram. In the left histogram, the vertical coordinate is the function of difference, the horizontal coordinate is the average abundance of the groups, and the color of the bar represents the groups; in the right scatter plot, the color of the dots indicates the results of different tests of significance, where the p-value is the result of the statistical test. The FDR is the false discovery rate, which is a corrected p-value, and the dots on the left side of the dotted line in the plot (p < 0.05) indicate significant differences; the middle area (the number of groups equals The central area shows the 95% confidence interval of the statistical test of the abundance difference between the two groups, where the dots are located is the mean value of the abundance difference, the color of the dots corresponds to the subgroups with higher abundance, and the boundaries of the line connecting the dots are the boundaries of the confidence interval. (C) Distribution of carbohydrate-active enzymes, the circle is divided into left and right parts, with grouping information on the left and species categorization information on the right. Looking from the outside in, the outermost scale is the percentage of the species in the grouping or the proportion of different species in the grouping, the color of the inner arc indicates the grouping/sample or the species, the line connecting the arcs suggests the presence of the species in the sample, and the width across the arcs indicates the percentage. CM (Conventional Tillage Maize) refers to maize monoculture under conventional tillage conditions. NM (No-tillage Maize) represents maize monoculture under no-tillage conditions. CI (Conventional Tillage Intercropping) indicates intercropping of maize and pea under conventional tillage. NI (No-tillage Intercropping) refers to intercropping of maize and pea under no-tillage.
Carbohydrate-active enzymes serve as key indicators of soil microbial response to carbon cycling. The analysis at the phylum level revealed that the Carbohydrate-Binding Modules (CBMs), Glycoside Hydrolases (GHs), and Glycosyl Transferases (GTs) families constituted over 90% of carbohydrate-active enzymes across all treatments, whereas the Polysaccharide Lyases (PLs), Carbohydrate Esterases (CEs), and Auxiliary Activities (AAs) families comprised < 10% (Figure 9C). Notably, the GHs and CEs exhibited significant differences among the treatments (Figure 9B). Overall, the monocrop systems exhibited higher levels of carbohydrate-active enzymes than the intercrop systems, with the NI treatment demonstrating the lowest content.
3.3.4 Relationships between soil respiration and essential microorganisms and functional genes for carbon metabolism
The correlation analysis among soil microorganisms demonstrated both positive and negative interactions (Figure 10A). Further investigation into the relationship between microorganisms and functional genes, using the Mantel test, indicated a negative correlation between Armatimonadetes and Candidatus_Omnitrophica with differential functional genes. Acidobacteria, Verrucomicrobia, and Chloroflexia showed a positive correlation with Cazy genes. Subsequently, these functional genes and associated microorganisms were analyzed using structural equation modeling to understand their relationship with soil respiration (Figure 10B). The results indicated that PLs and AAs in Cazy had a positive effect on soil carbon metabolism, with path coefficients of 0.247 and 0.271, respectively. Additionally, Verrucomicrobia and Acidobacteria promoted the up-regulation of PLs, and Verrucomicrobia and Thaumarchaeota suppressed AAs expression. Notably, metE significantly contributed to soil carbon metabolism, with Actinobacteria and Armatimonadets repressing its expression, and Candidatus_Omnitrophica also promoted it. The model explained soil respiration to the degree of 0.203. These microorganisms and functional genes were collectively affected by no-tillage and intercropping, thereby reducing soil carbon emissions through the modulation of soil microorganisms, regulation of carbon metabolism genes, and reduction of the soil respiration rate.
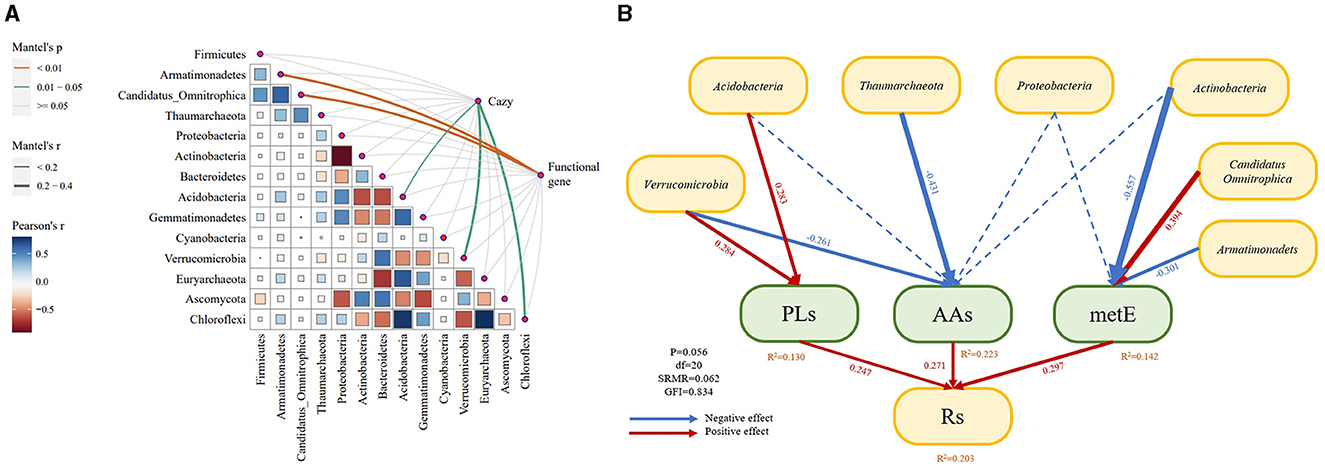
Figure 10. (A) Heat map of correlation between soil microbes, carbohydrate-active enzyme genes, and functional genes. Line thickness indicates a correlation, and line color indicates the mantel test P-value. (B) Structural equation modeling. Blue lines indicate negative effects, red lines indicate positive effects, and line thickness indicates effect size. Numbers indicate path coefficients.
4 Discussion
4.1 Tillage practices, intercropping, and soil carbon emissions
Agricultural soils contribute to the atmospheric CO2 levels (Huang et al., 2020). This study highlighted how no-tillage and intercropping strategies can mitigate agricultural soil respiration and the subsequent CO2 emissions. In conventional studies, the reduction in soil respiration through no-tillage has been attributed to its capacity to stabilize soil structure, enhance soil aggregates, regulate soil moisture and temperature, and minimize soil organic carbon mineralization, consequently limiting the exchange of soil carbon with the atmosphere (Zhao et al., 2019; Wang et al., 2020a; Rahman et al., 2021). Conversely, intercropping alters the field microclimate, optimizes light energy utilization via crop ecological niche differentiation, modulates topsoil temperature, and exhibits crop-specific variations in root respiration (Yin et al., 2018; Chai et al., 2021). Root respiration, constituting over 42% of soil respiration, underscores its pivotal role, as observed in the present study's significant variation in soil respiration across different crop bands within intercropping systems (Jian et al., 2022). The strategic intercropping of crops with lower root respiration rates demonstrates a potential reduction in the overall soil respiration rates within the system.
Additionally, conservation tillage, particularly no-till, can significantly reduce soil erosion, minimize soil surface disturbance, and consequently lower the decomposition rate of organic carbon (Lal et al., 2015; Zhang et al., 2022). This reduction not only stabilizes the soil carbon pool but also decreases carbon emissions to the atmosphere. On the other hand, intercropping systems, through crop species complementarity, enhance resource use efficiency by plants and reduce soil respiration and carbon emissions that may result from monocropping (Cong et al., 2015; Yang et al., 2023). Moreover, the diverse root structures and depths in intercropping systems create a more complex rhizosphere microenvironment, which helps to increase soil organic matter content and enhance soil carbon sequestration (Cong et al., 2015; Wang W. et al., 2023). Therefore, optimizing soil tillage and intercropping practices can achieve sustainable development of agroecosystems and effectively mitigate the negative impact of agriculture on the global carbon cycle.
4.2 Effect of no-till and intercropping on soil microbial communities
Soil microorganisms, integral to the soil-atmosphere elemental cycle, fluctuations in abundance and species diversity due to spatial and temporal variations (He et al., 2021). Moreover, human activities at the farm scale contribute to further variability in the soil microbial abundance and species composition by altering the soil environment (Baldrian, 2019; Garcia-Garcia et al., 2019). The decreases in microbial diversity, particularly bacterial diversity, have been linked to higher CO2 emissions owing to reduced soil carbon use efficiency (Domeignoz-Horta et al., 2020; Nottingham et al., 2022). Our study employed microbial β-diversity to assess the impact of no-till and intercropping on soil microbial diversity. Although no-till significantly increased soil microbial β-diversity, the effects of intercropping varied across different tillage systems. In addition, no-till systems exhibit higher microbial populations (Wang Z. T. et al., 2020; Zheng et al., 2022). Fierer (2017) have reported that soil microbial diversity globally is influenced by factors such as soil pH and moisture, with smaller-scale effects driven more by soil moisture and organic matter, whereas crop species impact specific microbial populations. Conservation tillage and crop diversification are known to enhance soil microbial populations, with both no-till and intercropping altering microbial diversity and populations by modifying soil physicochemical properties and microenvironmental conditions (Li et al., 2020; Kan et al., 2022; Zhao et al., 2022). The extent of these alterations is often contingent on the intensity of human activities.
Carbohydrate-active enzymes play a crucial role in decomposing complex carbohydrates such as cellulose, polysaccharides, starch, and glycogen, facilitating microbial metabolism and serving as indicators of soil microbial response to carbon turnover (Baldrian, 2019; Ren et al., 2021). Studies by Ren et al. have indicated that practices such as plant restoration and intercropping enhance soil microbial carbohydrate-active enzyme abundance, thereby improving soil fertility and organic matter content (Baldrian, 2019; Ren et al., 2021, 2022). Consequently, increased substrates for enzyme reactions result in higher enzyme abundance (Li et al., 2021; Ren et al., 2021). In this study, the no-till intercropping system significantly reduced the abundance of soil carbohydrate-active enzymes, particularly GHs and CEs. Despite offering ample substrates for enzyme activity, the drier soil conditions associated with no-tillage and intercropping may decrease soil bacterial diversity, thereby affecting carbohydrate-active enzyme levels (Canarini et al., 2021; Nottingham et al., 2022).
In our study, we investigated the effects of no-tillage and intercropping techniques on soil carbon emissions and microbial communities. However, we did not address the issue of hydrogen gas generation in nitrogen-fixing nodules. The production of hydrogen gas is an unavoidable byproduct of the nitrogen fixation process, which has a potentially significant impact on soil carbon sequestration, especially in the rhizosphere soil (Stein et al., 2005; Zulfiqar et al., 2021). According to the research by Stein et al., hydrogen gas produced during nitrogen fixation can significantly stimulate the process of carbon sequestration in soil, reducing carbon dioxide emissions, and may even inhibit carbon dioxide uptake (Stein et al., 2005). This finding is particularly important for the rhizosphere soil, as it is the area where plant roots and microbial interactions are most active, making carbon sequestration processes more pronounced.
Moreover, Rubisco (ribulose-1,5-bisphosphate carboxylase/oxygenase) is a key enzyme responsible for carbon dioxide fixation (Selesi et al., 2005; Burlacot et al., 2022). The study by Selesi et al. (2005) indicated that the expression levels of Rubisco genes in soil, particularly in the rhizosphere, can serve as a crucial indicator of carbon dioxide sequestration capacity. To further understand the impact of hydrogen gas on carbon sequestration, we recommend measuring the levels of Rubisco genes in soil, especially in the rhizosphere, in future research. This will help to comprehensively elucidate the microbial mechanisms by which no-tillage and intercropping techniques reduce soil carbon emissions, thereby providing microbial-level insights for optimizing agronomic practices to achieve more effective carbon reduction outcomes.
4.3 How no-till and intercropping regulate soil respiration through soil microorganisms
Agricultural soils represent a significant source of terrestrial carbon emissions. With the continuous development of sustainable agriculture, diverse carbon emission reduction techniques have been developed for agricultural soils (Chen et al., 2024; Jia et al., 2024). These techniques have diverse effects and mechanisms for emission reduction (Xia et al., 2023). This study focused on employing soil no-till and crop intercropping to regulate soil respiration and reduce carbon emissions. Agronomic measures do not directly affect soil respiration, because they do not induce respiration (Xia et al., 2023). Therefore, by focusing on the components of soil respiration (including soil animal respiration, soil microbial respiration, and plant root respiration; Chen et al., 2017; Huang et al., 2020), this study primarily investigated the responses of soil microorganisms to agonomic measures and their effects on soil respiration. The abundance of soil microbial carbohydrate-activated enzymes exhibited a trend similar to that of soil microbial load, with higher microbial loads correlating with increased enzyme abundance (Dove et al., 2021). This relationship presented the significance of reactive substrates in influencing enzyme abundance, as these enzymes played a pivotal role in carbohydrate decomposition within the soil (Bahram et al., 2018, 2020; Ren et al., 2021). Additionally, this study indicated that intercropping and no-tillage practices reduced soil carbon emissions by decreasing the total soil microbial population, increasing microbial species abundance, reducing microbial evenness, and suppressing the expression of key carbon cycle genes, ultimately reducing soil carbon emissions.
In addition to the previously discussed mechanisms, soil tillage and intercropping also play crucial roles in modulating soil microbial communities, which in turn influence soil respiration and carbon emissions (Zheng et al., 2022; Yang et al., 2023). These agronomic practices impact soil microorganisms in several ways. No-till systems create a more stable soil environment that promotes the proliferation of beneficial microorganisms and fungi, which are less active in decomposing organic matter compared to bacteria (Li et al., 2020; Wang Z. T. et al., 2020; Zheng et al., 2022). This leads to a reduction in the overall soil respiration rate (Ren et al., 2021, 2022). Similarly, intercropping introduces a variety of plant species with different root structures and exudates, fostering a diverse microbial community that can efficiently utilize available resources, further reducing soil microbial respiration (Bahram et al., 2018). Furthermore, the presence of different root systems in intercropping alters the soil microenvironment, enhancing microbial activity and diversity, while simultaneously reducing the dominance of any single microbial species. This leads to a decrease in microbial evenness and a shift in microbial community composition, favoring those microorganisms that are less effective at breaking down complex organic matter into CO2 (Bahram et al., 2020). The reduction in the expression of key carbon cycle genes, particularly those involved in the degradation of complex carbohydrates, also contributes to lower soil respiration rates and carbon emissions (Chen et al., 2017).
Thus, through the strategic implementation of no-till and intercropping practices, it is possible to influence soil microbial dynamics in a way that reduces soil respiration and carbon emissions. This approach highlights the importance of considering soil microbial ecology in the development of sustainable agricultural practices aimed at mitigating climate change.
5 Conclusion
This study examined the effects of intercropping and no-tillage on soil respiration rates and carbon emissions, as well as their effects on soil microbiota, microbial community structure, and functional genes. The NI treatment demonstrated a reduction in soil respiration rate and carbon emissions compared to the CM and CI treatments, demonstrating the potential of intercropping and no-tillage to mitigate soil respiration and carbon emissions. The soil microbial abundance, particularly that of bacteria such as Proteobacteria and Actinobacteria, decreased under intercropping and no-tillage conditions, reflecting their association with soil respiration. Furthermore, intercropping and no-tillage suppressed key carbon cycle functional genes, such as metE, as well as carbohydrate-active enzymes, such as PLs and AAs, contributing to reduced soil carbon cycling. These findings suggested that intercropping and no-tillage strategies effectively lowered soil carbon emissions by modulating soil carbon cycling microorganisms and their critical genes, thereby offering promising avenues for low-carbon agriculture practices.
Data availability statement
The datasets presented in this study can be found in online repositories. The names of the repository/repositories and accession number(s) can be found at: https://ngdc.cncb.ac.cn/gsa/, GSA: CRA017194.
Author contributions
Q-mW: Writing – original draft, Writing – review & editing, Data curation, Formal analysis, Funding acquisition, Methodology, Resources, Software. QC: Funding acquisition, Methodology, Project administration, Resources, Supervision, Writing – review & editing. X-cD: Funding acquisition, Methodology, Resources, Supervision, Writing – review & editing. WY: Methodology, Writing – review & editing. Y-lS: Data curation, Formal analysis, Methodology, Writing – original draft. F-lH: Investigation, Methodology, Supervision, Writing – review & editing. H-tL: Data curation, Formal analysis, Writing – original draft. Z-pL: Data curation, Formal analysis, Software, Writing – original draft. J-gW: Data curation, Formal analysis, Software, Writing – original draft. X-hX: Data curation, Formal analysis, Software, Writing – original draft.
Funding
The author(s) declare financial support was received for the research, authorship, and/or publication of this article. We are very grateful for financial support of the National Key Research and Development Project of China (2021YFD1700204), the Natural Science Foundation of China (U21A20218 and 32372238), the China Agriculture Research System (CARS-22-G-12), and the Science and Technology Program of Gansu (22JR5RA839).
Acknowledgments
We appreciate assistance in the field and laboratory by students of the Oasis Agricultural Experiment Station of Gansu Agricultural University. We are also grateful to the editor and reviewers for their constructive comments and suggestions on this manuscript.
Conflict of interest
The authors declare that the research was conducted in the absence of any commercial or financial relationships that could be construed as a potential conflict of interest.
Publisher's note
All claims expressed in this article are solely those of the authors and do not necessarily represent those of their affiliated organizations, or those of the publisher, the editors and the reviewers. Any product that may be evaluated in this article, or claim that may be made by its manufacturer, is not guaranteed or endorsed by the publisher.
References
Bahram, M., Hildebrand, F., Forslund, S. K., Anderson, J. L., Soudzilovskaia, N. A., Bodegom, P. M., et al. (2018). Structure and function of the global topsoil microbiome. Nature 560, 233–237. doi: 10.1038/s41586-018-0386-6
Bahram, M., Netherway, T., Hildebrand, F., Pritsch, K., Drenkhan, R., Loit, K., et al. (2020). Plant nutrient-acquisition strategies drive topsoil microbiome structure and function. New Phytol. 227, 1189–1199. doi: 10.1111/nph.16598
Baldrian, P. (2019). The known and the unknown in soil microbial ecology. Fems. Microbiol. Ecol. 95:fiz005. doi: 10.1093/femsec/fiz005
Bo, Y., Jägermeyr, J., Yin, Z., Jiang, Y., Xu, J., Liang, H., et al. (2022). Global benefits of non-continuous flooding to reduce greenhouse gases and irrigation water use without rice yield penalty. Glob. Change Biol. 28, 3636–3650. doi: 10.1111/gcb.16132
Burlacot, A., Dao, O., Auroy, P., Cuiné, S., Li-Beisson, Y., and Peltier, G. (2022). Alternative photosynthesis pathways drive the algal CO2-concentrating mechanism. Nature 605, 366–371. doi: 10.1038/s41586-022-04662-9
Canarini, A., Schmidt, H., Fuchslueger, L., Martin, V., Herbold, C., Zezula, D., et al. (2021). Ecological memory of recurrent drought modifies soil processes via changes in soil microbial community. Nat. Commun. 12:5308. doi: 10.1038/s41467-021-25675-4
Chai, Q., Nemecek, T., Liang, C., Zhao, C., Yu, A. Z., Coulter, J. A., et al. (2021). Integrated farming with intercropping increases food production while reducing environmental footprint. Proc. Natl. Acad. Sci. U. S. A. 118, 2106–2118. doi: 10.1073/pnas.2106382118
Chen, J., Luo, Y., Li, J., Zhou, X., Cao, J., Wang, R. W., et al. (2017). Costimulation of soil glycosidase activity and soil respiration by nitrogen addition. Glob. Change Biol. 23, 1328–1337. doi: 10.1111/gcb.13402
Chen, J., Zhao, Z., Yang, Y., Li, C., Yin, Y., Zhao, X., et al. (2024). Metallogenic prediction based on fractal theory and machine learning in Duobaoshan Area, Heilongjiang Province. Ore Geol. Rev. 168:106030. doi: 10.1016/j.oregeorev.2024.106030
Chen, Y., Chen, Y., Shi, C., Huang, Z., Zhang, Y., Li, S., et al. (2018). SOAPnuke: a MapReduce acceleration-supported software for integrated quality control and preprocessing of high-throughput sequencing data. GigaScience 7:gix120. doi: 10.1093/gigascience/gix120
Cong, W. F., Hoffland, E., Li, L., Six, J., Sun, J. H., Bao, X. G., et al. (2015). Intercropping enhances soil carbon and nitrogen. Glob. Change Biol. 21, 1715–1726. doi: 10.1111/gcb.12738
Domeignoz-Horta, L. A., Pold, G., Liu, X. A., Frey, S. D., Melillo, J. M., and DeAngelis, K. M. (2020). Microbial diversity drives carbon use efficiency in a model soil. Nat. Commun. 11:3684. doi: 10.1038/s41467-020-17502-z
Dove, N., Torn, M., Hart, S., and Taş, N. (2021). Metabolic capabilities mute positive response to direct and indirect impacts of warming throughout the soil profile. Nat. Commun. 12:2089. doi: 10.1038/s41467-021-22408-5
Du, C., Bai, X., Li, Y., Tan, Q., Zhao, C., Luo, G., et al. (2024). Storage, form, and influencing factors of karst inorganic carbon in a carbonate area in China. Sci. China Earth Sci. 67, 725–739. doi: 10.1007/s11430-023-1249-9
Fierer, N. (2017). Embracing the unknown: disentangling the complexities of the soil microbiome. Nat. Rev. Microbiol. 15, 579–590. doi: 10.1038/nrmicro.2017.87
Garcia-Garcia, N., Tamames, J., Linz, A. M., Pedros-Alio, C., and Puente-Sanchez, F. (2019). Microdiversity ensures the maintenance of functional microbial communities under changing environmental conditions. Isme J. 13, 2969–2983. doi: 10.1038/s41396-019-0487-8
Gou, Z., Yin, W., Asibi, A. E., Fan, Z., Chai, Q., and Cao, W. (2022). Improving the sustainability of cropping systems via diversified planting in arid irrigation areas. Agron. Sustain. Dev. 42:88. doi: 10.1007/s13593-022-00823-2
He, M. Y., Dong, J. B., Jin, Z., Liu, C. Y., Xiao, J., Zhang, F., et al. (2021). Pedogenic processes in loess-paleosol sediments: clues from Li isotopes of leachate in Luochuan loess. Geochimica et Cosmochimica Acta 299, 151–162. doi: 10.1016/j.gca.2021.02.021
Huang, J., Gómez-Dans, J. L., Huang, H., Ma, H., Wu, Q., Lewis, P. E., et al. (2019). Assimilation of remote sensing into crop growth models: current status and perspectives. Agr. Forest Meteorol. 276–277:107609. doi: 10.1016/j.agrformet.2019.06.008
Huang, N., Wang, L., Song, X. P., Black, T. A., Jassal, R. S., Myneni, R. B., et al. (2020). Spatial and temporal variations in global soil respiration and their relationships with climate and land cover. Sci. Adv. 6:8508. doi: 10.1126/sciadv.abb8508
Jansson, J. K., and Hofmockel, K. S. (2020). Soil microbiomes and climate change. Nat. Rev. Microbiol. 18, 35–46. doi: 10.1038/s41579-019-0265-7
Jia, Q., Sun, J., Gan, Q., Shi, N. N., and Fu, S. (2024). Zea mays cultivation, biochar, and arbuscular mycorrhizal fungal inoculation influenced lead immobilization. Microbiol. Spectr. 12, e03427–e03423. doi: 10.1128/spectrum.03427-23
Jian, J., Frissell, M., Hao, D., Tang, X., Berryman, E., and Bond-Lamberty, B. (2022). The global contribution of roots to total soil respiration. Glob. Ecol. Biogeogr. 31, 685–699. doi: 10.1111/geb.13454
Junwei, H., Kong, M., Francoys, A., Yarahmadi, F., Mendoza, O., Hassi, U., et al. (2024). Increased N2O emissions by the soil nematode community cannot be fully explained by enhanced mineral N availability. Soil Biol. Biochem. 191:109314. doi: 10.1016/j.soilbio.2024.109314
Kan, Z. R., Liu, W. X., Liu, W. S., Lal, R., Dang, Y. P., Zhao, X., et al. (2022). Mechanisms of soil organic carbon stability and its response to no-till: a global synthesis and perspective. Glob. Change Biol. 28, 693–710. doi: 10.1111/gcb.15968
Kopecký, M., Peterka, J., Kolár, L., Konvalina, P., Maroušek, J., Váchalová, R., et al. (2021). Influence of selected maize cultivation technologies on changes in the labile fraction of soil organic matter sandy-loam cambisol soil structure. Soil Till. Res. 207:104865. doi: 10.1016/j.still.2020.104865
Lal, R., Negassa, W., and Lorenz, K. (2015). Carbon sequestration in soil. Curr. Opin. Environ. Sustain. 15, 79–86. doi: 10.1016/j.cosust.2015.09.002
Li, W., Jaroszewski, L., and Godzik, A. (2001). Clustering of highly homologous sequences to reduce the size of large protein databases. Bioinformatics 17, 282–283. doi: 10.1093/bioinformatics/17.3.282
Li, X. F., Wang, Z. G., Bao, X. G., Sun, J. H., Yang, S. C., Wang, P., et al. (2021). Long-term increased grain yield and soil fertility from intercropping. Nat. Sustain. 4, 943–950. doi: 10.1038/s41893-021-00767-7
Li, Y., Song, D., Liang, S., Dang, P., Qin, X., Liao, Y., et al. (2020). Effect of no-tillage on soil bacterial and fungal community diversity: a meta-analysis. Soil Till. Res. 204:104721. doi: 10.1016/j.still.2020.104721
Liang, S., Zhao, Z., Li, C., Yin, Y., Li, H., and Zhou, J. (2024). Age and petrogenesis of ore-forming volcanic-subvolcanic rocks in the Yidonglinchang Au deposit, Lesser Xing'an Range: implications for late Mesozoic Au mineralization in NE China. Ore Geol. Rev. 165:105875. doi: 10.1016/j.oregeorev.2024.105875
Lin, J., Xu, Z., Xue, Y. M., Sun, R., Yang, R., Cao, X., et al. (2023). N2O emissions from soils under short-term straw return in a wheat-corn rotation system are associated with changes in the abundance of functional microbes. Agric. Ecosyst. Environ. 341:108217. doi: 10.1016/j.agee.2022.108217
Liu, Y., Gu, W., Liu, B., Zhang, C., Wang, C., Yang, Y., et al. (2022). Closing greenhouse gas emission gaps of staple crops in China. Environ. Sci. Technol. 56, 9302–9311. doi: 10.1021/acs.est.2c01978
Nottingham, A., Scott, J., Saltonstall, K., Broders, K., Montero-Sanchez, M., Püspök, J., et al. (2022). Microbial diversity declines in warmed tropical soil and respiration rise exceed predictions as communities adapt. Nat. Microbiol. 7, 1–11. doi: 10.1038/s41564-022-01200-1
Pearsons, K. A., Omondi, E. C., Zinati, G., Smith, A., and Rui, Y. (2023). A tale of two systems: does reducing tillage affect soil health differently in long-term, side-by-side conventional and organic agricultural systems? Soil Tillage Res. 226:105562. doi: 10.1016/j.still.2022.105562
Pu, C., Chen, J. S., Wang, H. D., Virk, A. L., Zhao, X., and Zhang, H. L. (2022). Greenhouse gas emissions from the wheat-maize cropping system under different tillage and crop residue management practices in the North China Plain. Sci. Total Environ. 819:153089. doi: 10.1016/j.scitotenv.2022.153089
Qiu, S., Yang, H., Zhang, S., Huang, S., Zhao, S., Xu, X., et al. (2023). Carbon storage in an arable soil combining field measurements, aggregate turnover modeling and climate scenarios. Catena 220:106708. doi: 10.1016/j.catena.2022.106708
Rahman, M. D. M., Aravindakshan, S., Hoque, M. A., Rahman, M. A., Gulandaz, M. A., Rahman, J., et al. (2021). Conservation tillage (CT) for climate-smart sustainable intensification: assessing the impact of CT on soil organic carbon accumulation, greenhouse gas emission and water footprint of wheat cultivation in Bangladesh. Envi. Sustainability Indic. 10:100106. doi: 10.1016/j.indic.2021.100106
Ren, C. J., Wang, J. Y., Bastida, F., Delgado-Baquerizo, M., Yang, Y. H., Wang, J., et al. (2022). Microbial traits determine soil C emission in response to fresh carbon inputs in forests across biomes. Glob. Change Biol. 28, 1516–1528. doi: 10.1111/gcb.16004
Ren, C. J., Zhang, X. Y., Zhang, S. H., Wang, J. Y., Xu, M. P., Guo, Y. X., et al. (2021). Altered microbial CAZyme families indicated dead biomass decomposition following afforestation. Soil Biol. Biochem. 160:108362. doi: 10.1016/j.soilbio.2021.108362
Ruis, S. J., Blanco-Canqui, H., Jasa, P. J., and Jin, V. L. (2022). No-till farming and greenhouse gas fluxes: insights from literature and experimental data. Soil Till. Res. 220:105359. doi: 10.1016/j.still.2022.105359
Sánchez-Navarro, V., Martínez-Martínez, S., Acosta, J. A., Almagro, M., Martínez-Mena, M., Boix-Fayos, C., et al. (2023). Soil greenhouse gas emissions and crop production with implementation of alley cropping in a Mediterranean citrus orchard. Eur. J. Agron. 142:126684. doi: 10.1016/j.eja.2022.126684
Selesi, D., Schmid, M., and Hartmann, A. (2005). Diversity of green-like and red-like ribulose-1,5-bisphosphate carboxylase/oxygenase large-subunit genes (cbbL) in differently managed agricultural soils. Appl. Environ. Microbiol. 71, 175–184. doi: 10.1128/AEM.71.1.175-184.2005
Shang, Z., Abdalla, M., Xia, L., Zhou, F., Sun, W., and Smith, P. (2021). Can cropland management practices lower net greenhouse emissions without compromising yield? Glob. Change Biol. 27, 4657–4670. doi: 10.1111/gcb.15796
Stein, S., Selesi, D., Schilling, R., Pattis, I., Schmid, M., and Hartmann, A. (2005). Microbial activity and bacterial composition of H2-treated soils with net CO2 fixation. Soil Biol. Biochem. 37, 1938–1945. doi: 10.1016/j.soilbio.2005.02.035
Sun, C., Chen, J., He, B., and Liu, J. (2024). Digitalization and carbon emission reduction technology R&D in a Stackelberg model. Appl. Econ. Lett. 2024, 1–6. doi: 10.1080/13504851.2024.2331659
Tian, P., Zhao, X., Liu, S., Sun, Z., Jing, Y., and Wang, Q. (2022). Soil microbial respiration in forest ecosystems along a north-south transect of eastern China: evidence from laboratory experiments. Catena 211:105980. doi: 10.1016/j.catena.2021.105980
Wang, C., Li, S., Wu, M., Wang, X., Wang, S., Guo, Z., et al. (2023). High efficiency and low greenhouse gas emissions intensity of maize in drip irrigation under mulch system. Agr. Ecosyst. Environ. 346:108344. doi: 10.1016/j.agee.2023.108344
Wang, W., Li, M. Y., Zhou, R., Zhu, S. G., Tao, H. Y., Khan, A., et al. (2023). Effects of interspecific interactions on soil carbon emission and efficiency in the semiarid intercropping systems. Soil Tillage Res. 234:105857. doi: 10.1016/j.still.2023.105857
Wang, X., Feng, Y., Yu, L., Shu, Y., Tan, F., Gou, Y., et al. (2020a). Sugarcane/soybean intercropping with reduced nitrogen input improves crop productivity and reduces carbon footprint in China. Sci. Total Environ. 719:137517. doi: 10.1016/j.scitotenv.2020.137517
Wang, X., Huang, J., Feng, Q., and Yin, D. (2020b). Winter wheat yield prediction at county level and uncertainty analysis in main wheat-producing regions of China with deep learning approaches. Remote. Sens. 12:1744. doi: 10.3390/rs12111744
Wang, Z. T., Li, T., Li, Y. Z., Zhao, D. Q., Han, J., Liu, Y., et al. (2020). Relationship between the microbial community and catabolic diversity in response to conservation tillage. Soil Till. Res. 196:104431. doi: 10.1016/j.still.2019.104431
Xia, L., Cao, L., Yang, Y., Ti, C., Liu, Y., Smith, P., et al. (2023). Integrated biochar solutions can achieve carbon-neutral staple crop production. Nat. Food 4, 236–246. doi: 10.1038/s43016-023-00694-0
Yang, L., Luo, Y., Lu, B., Zhou, G., Chang, D., Gao, S., et al. (2023). Long-term maize and pea intercropping improved subsoil carbon storage while reduced greenhouse gas emissions. Agric. Ecosyst. Environ. 349:108444. doi: 10.1016/j.agee.2023.108444
Yi, J., Li, H., Zhao, Y., Shao, M., Zhang, H., and Liu, M. (2022). Assessing soil water balance to optimize irrigation schedules of flood-irrigated maize fields with different cultivation histories in the arid region. Agric. Water Manag. 265:107543. doi: 10.1016/j.agwat.2022.107543
Yin, W., Guo, Y., Hu, F., Fan, Z., Feng, F., Zhao, C., et al. (2018). Wheat-maize intercropping with reduced tillage and straw retention: a step towards enhancing economic and environmental benefits in arid areas. Front. Plant. Sci. 9:1328. doi: 10.3389/fpls.2018.01328
Yue, K., Fornara, D. A., Heděnec, P., Wu, Q., Peng, Y., Peng, X., et al. (2023). No tillage decreases GHG emissions with no crop yield tradeoff at the global scale. Soil Till. Res. 228:105643. doi: 10.1016/j.still.2023.105643
Zhang, H., Hobbie, E. A., Feng, P., Niu, L. a, and Hu, K. (2022). Can conservation agriculture mitigate climate change and reduce environmental impacts for intensive cropping systems in North China Plain? Sci. Total Environ. 806:151194. doi: 10.1016/j.scitotenv.2021.151194
Zhang, T., Li, H., Yan, T., Shaheen, S. M., Niu, Y., Xie, S., et al. (2023). Organic matter stabilization and phosphorus activation during vegetable waste composting: multivariate and multiscale investigation. Sci. Total Environ. 891:164608. doi: 10.1016/j.scitotenv.2023.164608
Zhang, X., Xin, X., Yang, W., Ding, S., Ren, G., Li, M., et al. (2021). Soil respiration and net carbon flux response to long-term reduced/no-tillage with and without residues in a wheat-maize cropping system. Soil Till. Res. 214:105182. doi: 10.1016/j.still.2021.105182
Zhao, C., Chai, Q., Cao, W. D., Whalen, J. K., Zhao, L. X., and Cai, L. J. (2019). No-tillage reduces competition and enhances compensatory growth of maize (Zea mays L.) intercropped with pea (Pisum sativum L.). Field Crop. Res. 243:107611. doi: 10.1016/j.fcr.2019.107611
Zhao, X., He, C., Liu, W. S., Liu, W. X., Liu, Q. Y., Bai, W., et al. (2022). Responses of soil pH to no-till and the factors affecting it: a global meta-analysis. Glob. Change Biol. 28, 154–166. doi: 10.1111/gcb.15930
Zhao, Y., Wang, H., Song, B., Xue, P., Zhang, W., Peth, S., et al. (2023). Characterizing uncertainty in process-based hydraulic modeling, exemplified in a semiarid Inner Mongolia steppe. Geoderma 440:116713. doi: 10.1016/j.geoderma.2023.116713
Zheng, F., Liu, X., Zhang, M., Li, S., Song, X., Wang, B., et al. (2023). Strong links between aggregate stability, soil carbon stocks and microbial community composition across management practices in a Chinese dryland cropping system. Catena 233:107509. doi: 10.1016/j.catena.2023.107509
Zheng, F., Wu, X., Zhang, M., Liu, X., Song, X., Lu, J., et al. (2022). Linking soil microbial community traits and organic carbon accumulation rate under long-term conservation tillage practices. Soil Till. Res. 220:105360. doi: 10.1016/j.still.2022.105360
Zhu, W., Lomsadze, A., and Borodovsky, M. (2010). Ab initio gene identification in metagenomic sequences. Nucleic Acids Res. 38, e132–e132. doi: 10.1093/nar/gkq275
Keywords: soil carbon emissions, soil microorganism, intercropping, functional gene, Cazy
Citation: Wang Q-m, Chai Q, Dou X-c, Yin W, Sun Y-l, Hu F-l, Li H-t, Liu Z-p, Wei J-g and Xu X-h (2024) Microbial mechanism of soil carbon emission reduction in maize-pea intercropping system with no tillage in arid land areas of northwestern China. Front. Sustain. Food Syst. 8:1415264. doi: 10.3389/fsufs.2024.1415264
Received: 10 April 2024; Accepted: 26 July 2024;
Published: 15 August 2024.
Edited by:
Rishi Raj, Indian Agricultural Research Institute (ICAR), IndiaReviewed by:
Muhammad Saqlain Zaheer, Khwaja Fareed University of Engineering and Information Technology (KFUEIT), PakistanAnton Hartmann, Ludwig Maximilian University of Munich, Germany
Copyright © 2024 Wang, Chai, Dou, Yin, Sun, Hu, Li, Liu, Wei and Xu. This is an open-access article distributed under the terms of the Creative Commons Attribution License (CC BY). The use, distribution or reproduction in other forums is permitted, provided the original author(s) and the copyright owner(s) are credited and that the original publication in this journal is cited, in accordance with accepted academic practice. No use, distribution or reproduction is permitted which does not comply with these terms.
*Correspondence: Qiang Chai, Y2hhaXEmI3gwMDA0MDtnc2F1LmVkdS5jbg==; Xue-cheng Dou, ZG91eGMmI3gwMDA0MDtnc2F1LmVkdS5jbg==