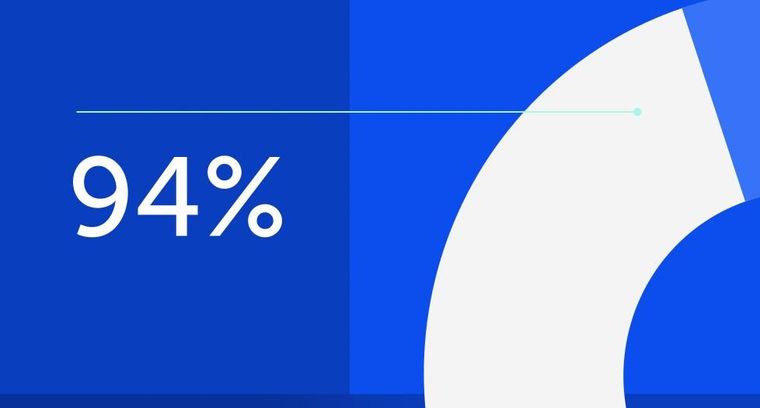
94% of researchers rate our articles as excellent or good
Learn more about the work of our research integrity team to safeguard the quality of each article we publish.
Find out more
ORIGINAL RESEARCH article
Front. Sustain. Food Syst., 27 June 2024
Sec. Climate-Smart Food Systems
Volume 8 - 2024 | https://doi.org/10.3389/fsufs.2024.1414858
To meet the objectives of the Paris Agreement, which aims to limit the increase in global temperature to 1.5°C, significant greenhouse gas (GHG) emission reductions will be needed across all sectors. This includes agriculture which accounts for a significant proportion of global GHG emissions. There is therefore a pressing need for the uptake of new technologies on farms to reduce GHG emissions and move towards current policy targets. Recently, precision livestock farming (PLF) technologies have been highlighted as a promising GHG mitigation strategy to indirectly reduce GHG emissions through increasing production efficiencies. Using Scotland as a case study, average data from the Scottish Cattle Tracing System (CTS) was used to create two baseline beef production scenarios (one grazing and one housed system) and emission estimates were calculated using the Agrecalc carbon footprinting tool. The effects of adopting various PLF technologies on whole farm and product emissions were then modelled. Scenarios included adoption of automatic weigh platforms, accelerometer-based sensors for oestrus detection (fertility sensors) and accelerometer-based sensors for early disease detection (health sensors). Model assumptions were based on validated technologies, direct experience from farms and expert opinion. Adoption of all three PLF technologies reduced total emissions (kg CO2e) and product emissions (kg CO2e/kg deadweight) in both the grazing and housed systems. In general, adoption of PLF technologies had a larger impact in the housed system than in the grazing system. For example, while health sensors reduced total emissions by 6.1% in the housed system, their impact was slightly lower in the grazing system at 4.4%. The largest reduction in total emissions was seen following the adoption of an automatic weight platform which reduced the age at slaughter by 3 months in the grazing system (6.8%) and sensors for health monitoring in the housed system (6.1%). Health sensors also resulted in the largest reduction in product emissions for both the housed (12.0%) and grazing systems (10.5%). These findings suggest PLF could be an effective GHG mitigation strategy for beef systems in Scotland. Although this study utilised data from beef farms in Scotland, comparable emission reductions are likely attainable in other European countries with similar farming systems.
Discussions surrounding land use policies, aimed at achieving net zero, are intensifying globally. The net zero target is defined as the point at which “anthropogenic emissions are equal to anthropogenic removals” over a specified timeframe (IPCC, 2018). Therefore, reaching the target will require significant greenhouse gas (GHG) mitigation efforts. Many countries have already committed to achieving net zero, including some of the world’s biggest polluters – China (Government of China, 2021), the United States (U.S. Department of State, 2021) and the European Union (European Commission, 2019). China have aimed to reach net zero by 2060, however, for the majority of countries, the target date is 2050. In some countries the target date for achieving net zero is even earlier. In 2019, Scotland introduced the Climate Change (Emissions Reduction Targets) Act, which brought forward the net zero target from 2050 to 2045.
Agriculture accounted for 18% of Scotland’s total greenhouse gas (GHG) emissions in 2020, with most of these emissions coming from methane (CH4) from the enteric fermentation of ruminant animals and nitrous oxide (N2O) from soils following application of fertiliser, manure or animal excreta on pasture (National Atmospheric Emissions Inventory, 2023). Achieving net zero will therefore require significant emission reductions from agriculture and in particular livestock. According to the Scottish Government’s Climate Change Update Plan, agriculture must reduce its emissions by 31% from 2018 levels by 2032.
There are several mitigation options available for farms to reduce their emissions, with many offering “win-win” solutions, whereby they not only reduce GHG emissions but also increase production efficiencies. Recently, precision livestock farming (PLF) technologies have been highlighted as a promising strategy to indirectly reduce GHG emissions by increasing production efficiencies (Balafoutis et al., 2017). Precision Livestock Farming allows continuous automated monitoring to gather data which can aid farmers’ decision-making (Wathes et al., 2008). Precision livestock farming technologies have the potential to support reproductive management (e.g., early detection for oestrus) or disease management through prediction models which can highlight deviations from normal health, welfare of behaviour (Tullo et al., 2019). Precision Livestock Farming can therefore aid management, improve animal health (Neethirajan, 2017), improve welfare and production (Berckmans, 2014) and monitor or reduce GHG emissions (Hammond et al., 2016).
There are several types of PLF technologies, for example, accelerometer-based sensors can provide real-time data on feeding, rumination and activity in both housed and grazing cattle (Iqbal et al., 2021; Nogoy et al., 2022). Animal-mounted (neck, leg, or ear) accelerometer-based sensors can improve fertility through oestrus detection from changes in temperature or activity (Roelofs et al., 2018). Efficient oestrus detection has the potential to decrease calving intervals, reduce heifer replacement rate and enhance genetic improvement (Nebel et al., 2011). In turn, this could improve overall efficiency and decrease GHG emissions per unit of product (i.e., meat from beef systems and milk from dairy systems). Accelerometer-based sensors have also been shown to allow early detection of disease and poor animal welfare through subtle changes in animal behaviour (Neethirajan, 2017; Chapa et al., 2020). Health sensors enable the detection of disease that may not be detected through traditional methods (Chapa et al., 2020; Bowen et al., 2021; Fan et al., 2022). The resulting reduction in morbidity and mortality could reduce GHG emissions per kg meat output. Another PLF technology which has the potential to reduce GHG emissions is automated monitoring of animal performance (i.e., liveweight). Automatic weigh platforms are fitted with electronic identification (EID) tag readers which identify individual animals and record animal weights every time they visit the feeder or water trough. These systems can be used both when housed and at grazing (where they can be powered by solar panels) (Brown et al., 2013; González et al., 2018). Automatic weigh platforms have the potential to reduce the age at slaughter by allowing animals to be selected for slaughter earlier than traditional methods, enabling more precise management of cattle nutrition and providing early indication of performance issues. Despite their various benefits, uptake of PLF technology remains low, particularly in beef systems (Makinde et al., 2022).
To date there have been no studies which have assessed the effects of PLF technologies on GHG emissions in beef systems. The aims of this work were to model the effects of adopting PLF technologies on whole farm and product emissions for both grazing and housed beef cattle. Using average data from the UK’s Cattle Tracing System (CTS), we demonstrate the realistic opportunities available for beef farmers to implement PLF technologies and reduce their GHG emissions.
To create two baseline farm scenarios for beef systems in Scotland, data were utilised from the UK Governments’ Cattle Tracing System (CTS). The CTS database contains details of all registered cattle births, deaths and movements in the UK (UK Government, 2023). Data were collated and average values were used to create one grazing and one housed baseline scenario, typical of those found in Scotland. Both scenarios were based on spring calving lowland suckler herds which finished animals on farm at 19–21 months. In the first baseline scenario (grazing), cattle were grazing for 6.7 months (56%) of the year and housed for 5.28 months (44%). In the second baseline scenario (housed), cattle were housed for 7.1 months (59%) of the year and grazed cattle for 4.9 months (41%). Although these scenarios are referred to as “grazing” and “housed,” it is important to note that neither system was exclusively grazing or exclusively housed. Diets were formulated by nutritionists from SAC Consulting to represent diets typical of those found in Scottish beef systems. Diets were formulated to provide 13% crude protein (CP) and 65% digestibility, consisting primarily of grass and grass silage, supplemented with barley and minerals. All calves received creep feed in both the housed and grazing scenarios so concentrate usage remained consistent. Full performance data for the grazing and housed baseline scenario can be found in Tables 1, 2. All manure was treated as farmyard manure (FYM) and all calves were finished on farm, for both baseline scenarios.
Table 1. Input data for all scenarios in the grazing system including baseline, adoption of automatic weigh platform which reduced age at slaughter by 1 (RAS1), 2 (RAS2) and 3 months (RAS3), fertility sensors, and health sensors.
Table 2. Input data for all scenarios in the housed system including baseline, adoption of automatic weigh platform which reduced age at slaughter by 1 (RAS1) and 2 months (RAS2), fertility sensors, and health sensors.
Baseline emission estimates were calculated using Agrecalc – a farm carbon calculator developed by Scotland’s Rural College (Agrecalc Ltd., Edinburgh). Agrecalc is aligned with ISO 14044 LCA guidelines and conforms to PAS2050 (2011) carbon footprinting standards. The system boundary of the tool is “cradle-to-gate,” and emissions are allocated on an economic basis. Agrecalc uses IPCC (2019) Tier 2 country-specific emissions calculations for all livestock enteric CH4 and N2O emissions from excreta deposited on grazing land. All CH4 and N2O emissions from manure management and direct soil N2O emissions utilise IPCC (2019) Tier 2 emission factors (EFs). Indirect N2O emissions and N2O emissions from crop residues employ IPCC (2019) Tier 1 calculations. For inputs, energy use values were taken from figures published in the Greenhouse Gas Protocol (DEFRA, 2012). Embedded emissions for fertilisers were sourced from Fertilisers Europe (2018) which reported fertiliser emissions by region. Finally, for purchased feed, Agrecalc used the Dutch Feedprint database (Vellinga et al., 2013). Emissions were expressed as Carbon Dioxide equivalents (CO2e) using Global Warming Potential over 100 years (GWP) from the fourth assessment report (AR4; IPCC, 2007). This meant the GWP Value was 25 for CH4 and 298 for N2O (IPCC, 2007). Emissions were expressed as both whole farm emissions (kg CO2e) and emissions per unit of output (kg CO2e/kg dwt).
Scenarios were then created to assess the impact of various PLF technologies on GHG emissions of both baseline beef farms. Adoption of an automatic weigh platform, accelerometer-based sensors for oestrus detection (fertility sensors) and accelerometer-based sensors for early disease detection (health sensors) were modelled on both the grazing and housed systems. Model assumptions were based on data from validated technologies, direct observations and practical experience from farmers utilising the technology and expert knowledge and insights provided by consultants from SAC Consulting, Edinburgh.
Scenarios for both the grazing and housed system included the adoption of an automatic weight platform, which reduced age at slaughter by 1 month (RAS1) and 2 months (RAS2), the adoption of fertility sensors and the adoption of health sensors. An additional scenario, involving the adoption of an automatic weigh platform which reduced the age at slaughter by 3 months (RAS3), was modelled for the grazing system due to its initially higher baseline slaughter age. The assumptions of each scenario are listed below.
Automatic weigh platform
1. Reduced age at slaughter by 1 month (RAS1)
Reduced killing out percentage (KO%) by 0.5% for both steers and heifers Live Weight Gain (LWG) increased by 0.05 kg/day to account for reduced age at slaughter.
2. Reduced age at slaughter by 2 months (RAS2)
Reduced KO% by 1% for both steers and heifers.
LWG increased by 0.09 kg/day to account for reduced age at slaughter.
3. Reduced age at slaughter by 3 months (RAS3) – grazing only
Reduced KO% by 1.5% for both steers and heifers.
LWG increased by 0.15 kg/day to account for reduced age at slaughter.
Final month of grazing prior to slaughter replaced with 1 month of housing.
Fertility sensors
Increase in calves born (4%) and reared (3%).
Decrease in replacement rate of 1%.
Age at first calving reduced to 24 months.
Cow weight decreased by 20 kg.
Health sensors
Increase in calves born (4%) and reared (4%).
Decrease in replacement rate of 1%.
Two fewer deaths (steer and heifer youngstock).
Reduced KO% by 1% for both steers and heifers.
LWG increase by 0.16 kg/day for both steers and heifers.
A full list of input data for all scenarios for both systems can be found in Tables 1, 2.
In both the grazing and housed baseline scenarios, the primary sources of emissions were enteric CH4, followed by N2O from soils and embedded emissions from fertiliser production (Figures 1, 2). The grazing system had slightly higher total baseline emissions (1,068,199 kg CO2e) than the housed system (1,050,648 kg CO2e). Carbon dioxide (CO2) emissions from inputs were higher in the housed system (with the exception of bedding) than in the grazing system, however, enteric CH4 and N2O emissions were higher in the grazing system. Product emissions between the grazing and housed system were very similar at 38.20 kg CO2e/kg dwt and 38.14 kg CO2e/kg dwt, respectively (Table 3).
Figure 1. Comparison of total farm emissions (kg CO2e) in the baseline grazing beef system and the subsequent impact of adopting an automatic weigh platform which reduced age at slaughter by 1 (RAS1), 2 (RAS2) and 3 months (RAS3), fertility sensors, and health sensors. Percentages represent emission reductions relative to the baseline.
Figure 2. Comparison of total farm emissions (kg CO2e) in the baseline housed beef system and the subsequent impact of adopting an automatic weigh platform which reduced age at slaughter by 1 (RAS1) and 2 months (RAS2), fertility sensors, and health sensors. Percentages represent emission reductions relative to the baseline.
Table 3. Comparison of product emissions in the baseline grazing and housed system and the subsequent impact of adopting an automatic weigh platform which reduced age at slaughter by 1 (RAS1), 2 (RAS2) and 3 months (RAS3), fertility sensors, and health sensors.
Adoption of all three PLF technologies resulted in a decrease in both total emissions (Figures 1, 2) and product emissions (Table 3) in both grazing and housed systems. Utilising an automatic weigh platform, which reduced the age at slaughter, reduced both total emissions and product emissions more in the housed system (Figure 2) than in the grazing system (Figure 1). As the age at slaughter was decreased following the adoption of an automatic weigh platform, total emissions and product emissions decreased linearly in both grazing and housed systems. The scenario in which the automatic weigh platform reduced age at slaughter by 3 months had the biggest reduction in total emissions (6.8%) in the grazing system (Figure 1).
Fertility sensors also reduced total emissions more in the housed system (Figure 2) than in the grazing system (Figure 1). However, fertility sensors reduced product emissions slightly more in the grazed system than in the housed system (Table 3). Health sensors had the largest reduction in total emissions in the housed system (6.1%, Figure 2) but a smaller reduction in total emissions in the grazing system. Heath sensors also resulted in the largest reduction in product emissions for both the housed (12.0%) and grazing systems (10.5%, Table 3).
To evaluate the impacts of PLF technologies on GHG emissions, it is crucial to firstly establish an accurate baseline level of emissions. In this study, we utilised the government’s CTS database to create baseline scenarios which were representative of average beef farms found in Scotland. As expected, average CTS data for both grazing and housed systems produced product emissions which were comparable with the normal ranges cited in literature (Edwards-Jones et al., 2009; Jones et al., 2014; Beattie, 2022). Total emissions were slightly higher in the grazing baseline scenario than in the housed. This difference was likely due to cattle in the grazing system taking (2 months) longer to finish and were therefore on farm longer, emitting more CH4 and N2O. However, the housed system had higher CO2 emissions associated with inputs such as fertiliser and feed. Product emissions were comparable in the grazing and housed systems. Although cattle in the grazing system took longer to finish, leading to higher total emissions, the sale weights of their steers and heifers were greater, resulting in a larger output for their emissions to be spread across.
Adoption of PLF technologies which reduced age at slaughter, improved fertility and improved health, reduced total emissions and product emissions in all scenarios in both the grazing and housed systems. These results are unsurprising, given that it is widely acknowledged that emissions from livestock are strongly influenced by factors such as animal efficiency, fertility, and health (Kumari et al., 2020). In general, adoption of PLF technologies had a larger impact in the housed system, both in terms of total emissions and product emissions. This could be attributed to the apparent higher baseline efficiency of the housed system, which had higher daily LWG and therefore finished cattle quicker. Although few studies have focused specifically on beef cattle, research in other species has indicated that PLF technologies have the potential to reduce emissions. For example, a recent study by Ferguson et al. (2024) found that fertility sensors reduced both total emissions (1.03–1.42%) and product emissions (1.94–2.78%) in three different Scottish dairy systems. Their modelling also found that health sensors reduced product emissions in all scenarios (6.36–6.62%) but slightly increased total emissions in two scenarios (0.16–0.43%) due to the increase in stock numbers from reduced morbidity and mortality. Another study by Pardo et al. (2022) estimated an 11% reduction in GHG emissions in dairy goat systems following the implementation of a PLF-platform which provided on-farm monitoring of individual animals through a smart phone.
Implementation of an automatic weigh platform which reduced age at slaughter by 3 months and increased daily LWG had the largest reduction in total emissions in the grazing system (6.8%, Figure 1). Automatic weigh platforms highlight performance issues and enable earlier selection for slaughter. With cattle spending less time on farm, there was a decrease in livestock CH4 and N2O emissions as well as a reduced demand for feed and fertiliser. There is limited research specifically evaluating the impact of PLF on GHG emissions, however, many studies have highlighted the emissions reductions possible through improvements in productivity. Flachowsky (2011), for example, reported that emissions from beef cattle could be reduced by 70% if cattle gained 1.5 kg/day compared to 1.0 kg/day. Although not directly measuring or modelling GHG emissions, there is extensive research on the impact of these PLF technologies on production efficiencies. Segerkvist et al. (2020) found frequent weighing from an automatic weighing station in dairy steers could provide early detection for weight gain abnormalities and therefore opportunities for disease detection. However, Segerkvist et al. (2020) found that there were only 0.71 usable weights per animal per day, likely due to more than one animal standing on the platform at once or if an animal has only two legs on the platform (Brown et al., 2013). This is likely a limitation of the platform assessed in this particular study, and unlikely a problem associated with commercially available solutions which have undergone robust assessment.
When comparing the product emissions of each scenario, health sensors had the largest reduction in both the grazing (10.5%) and housed systems (12%, Table 3). While health sensors led to an increase the number of calves reared, they also increased daily LWG and decreased replacement rates, resulting in a reduction in slaughter age and an increase in output. Although no studies have specifically evaluated the effects of health related PLF technology, the emission reductions found in this study were higher than others which modelled the effects of improvements in health on GHG emissions. For example, ADAS (2014) estimated a 6% reduction in GHG emission intensities in beef cattle following a 50% shift from the baseline towards a healthy cattle population. This reduction was primarily attributed to lower replacement rates (19%) and a reduction in mortality and morbidity, resulting in 28% fewer cows needed. However, the effects of these improvements in health will be highly dependent on the initial health status of the herd. Accelerometer-based sensors like those evaluated in this study have the potential to provide early detection of disease and lameness which can reduce the associated production losses. For example, de Mol et al. (2013) developed a model which automatically detected lameness in dairy cattle from accelerometer data with 88.5% sensitivity and 88.8% specificity based on seven activity variables including lying time, lying bouts, maximum and average length of lying bout, maximum and average length of standing bout and number of steps. Again, while GHG emissions were not directly measured or modelled in these studies, these increases in production efficiencies would result in a reduction in emissions per kg output (Hristov et al., 2013; Grossi et al., 2019).
The variation seen in the reduction of total emissions and product emissions in the grazing scenarios highlights an important issue whereby the most efficient farms or scenarios in terms of product emissions are not always those with the lowest total emissions. For example, while reducing the age at slaughter resulted in the greatest reduction in total emissions in the grazing system, it also led to a decrease in total deadweight and therefore did not affect product emissions to the same extent. Conversely, adoption of health sensors had the largest reduction in product emissions. However, due to the increased number of calves reared and higher sale weights, total emissions did not have the same level of reduction. This holds particular significance because although food production must be increased to feed a growing population, efficiency gains must be achieved sustainably to avoid an increase in total emissions (Costa et al., 2022). If production efficiencies were increased, it is likely farmers would increase their stock numbers, resulting in an increase in total emissions. This poses a challenge to current policy targets such as the net zero by 2045 target, which is defined as the point in which “anthropogenic emissions are equal to anthropogenic removals.”
Fertility sensors which increased the number of calves reared, decreased replacement rates and reduced age at first calving, were the second most effective PLF technology at reducing product emissions in both grazing (8.4%) and housed (8.2%) scenarios (Table 3). As mentioned above, the corresponding reduction in total emissions may not have been as high due to the increase in calves reared. Extensive research has been conducted on fertility sensors, particularly on their ability to enhance production efficiencies (Tzanidakis et al., 2023). Effective oestrus detection can shorten calving intervals and reduce the period in which cows are unproductive, reduce the number of cows culled due to failed conception and increase milk yields (Pfeiffer et al., 2020). For example, modelling by Rutten et al. (2014) found oestrus detection through an activity meter led to a shorter calving interval of 403 days and higher average annual milk production of 1,043,398 kg, when compared to visual oestrus detection which had a calving interval of 419 days and average annual milk production of 1,032,278 kg. However, the effects of implementing fertility sensors will be highly dependent on the pre-existing level of fertility on farm (Adenuga et al., 2020; Baruselli et al., 2023). As with the other technologies, limited research exists specifically on the effect of fertility sensors on GHG emissions, however, numerous studies have highlighted the emissions reductions possible through improvements in fertility (Smith et al., 2016). For example, Cullen et al. (2016) found beef herds with high reproductive performance had GHG emissions intensities that were 28 and 22% lower than typical herds in two different areas of Australia.
Despite many PLF technologies offering “win–win” solutions whereby they increase production efficiencies as well as reduce GHG emissions, uptake remains low in the beef and sheep sectors (Groher et al., 2020). One possible reason for this low uptake is the lack of perceived benefit of PLF technologies amongst some farmers (Lima et al., 2018; Makinde et al., 2022). Therefore, improved knowledge exchange and communication of the benefits of PLF technologies, both in terms of production efficiency and GHG mitigation could be key to increasing their adoption (Lima et al., 2018). Research like the current study, which clearly demonstrates the additional benefits of PLF technology adoption, could play a crucial role in accelerating their adoption, particularly if GHG mitigation was incentivised. However, even if technology is adopted, it does not always guarantee that technology will be utilised effectively (Schillings et al., 2021). Consequently, emission reductions from PLF may fail to reach their maximum technical potential.
As with most modelling work, some appropriate assumptions had to be made on the effects of the PLF technologies evaluated. Although these assumptions were based on the best available knowledge and expert opinion, there could still be an over- or under-estimation in the emission reductions possible following adoption. In future, a full sensitivity analysis should be performed to assess the effect of altering assumptions on the resulting GHG emission estimates. The validation of commercially available PLF tools is rarely subject to peer review, and there are limited studies which evaluate their effectiveness in increasing production efficiencies or reducing emissions. Therefore, more peer-reviewed studies are required to establish the efficacy of PLF technologies to enable more accurate modelling assumptions to be made. Moreover, this study was based on average data, which does not necessarily reflect the heterogeneity in the beef sector. Therefore, the emission reductions reported in this study could be significantly higher in some farms and lower in others.
The current study did not consider the range of socio-economic drivers and barriers to the uptake of PLF measures. While the significant initial costs of many PLF technologies are evident, there is a lack of data regarding the return on investment and net economic cost of these technologies (Banhazi et al., 2012). PLF has the potential to reduce costs through various means, including improvements in production efficiencies and therefore yields, reduced costs associated with disease and reduced labour costs (Hogeveen and Steeneveld, 2013). The multi-faceted nature of these factors makes it challenging to accurately calculate the net cost of PLF technologies. Similarly, the social implications of PLF technologies are hard to measure and can significantly influence how successful a technology will be. For example, Kamphuis et al. (2015) highlighted that automated milking systems (AMS) are the most widely adopted PLF technology globally, despite several studies indicating that AMS may result in unfavourable economic outcomes compared to conventional systems. Nevertheless, adoption is still high due to the reduction in labour and greater flexibility in working hours. Future work should account for these socio-economic implications, as they could substantially influence the maximum technical abatement potential of such measures.
This preliminary assessment has uniquely demonstrated the potential emission reductions which could be achieved by implementing various PLF technologies on beef farms in Scotland. All PLF technologies evaluated in this study including automatic weigh platforms, fertility sensors and health sensors resulted in a reduction in both total emissions and product emissions in grazing and housed systems. These finding suggest that PLF could be a promising GHG mitigation strategy for beef systems in Scotland and could therefore help the sector meet current environmental targets. Although this study utilised data from beef farms in Scotland, comparable emission reductions are likely attainable in other European countries with similar farming systems.
While the assumptions made in this modelling were based on best available knowledge, more peer-reviewed studies are needed to evaluate the effects of implementing PLF technologies on production efficiencies and GHG emissions. As a result, it is possible the estimated abatement potentials in this study could be over- or under-estimated. Despite most PLF technologies representing “win–win” solutions, the uptake of PLF remains low in beef systems. While it is well documented that PLF can increase production efficiencies, demonstrating their additional benefits (i.e., reducing GHG emissions) could accelerate the adoption of these measures.
The raw data supporting the conclusions of this article will be made available by the authors, without undue reservation.
LM: Investigation, Visualization, Writing – original draft. JBo: Conceptualization, Data curation, Formal analysis, Investigation, Methodology, Writing – review & editing. HF: Conceptualization, Data curation, Investigation, Methodology, Writing – review & editing. JBe: Data curation, Investigation, Writing – review & editing. RD: Conceptualization, Funding acquisition, Writing – review & editing. C-AD: Conceptualization, Funding acquisition, Writing – review & editing.
The author(s) declare that financial support was received for the research, authorship, and/or publication of this article. This work was funded by the Scottish Government Rural Affairs and the Environment Portfolio Strategic Research Program RD2.3.9 and DEFRA funding through FACCE ERA-GAS, ERANET SUSan and ICT-AGRI ERANET in the GrASTech project.
JBe was employed by Agrecalc Ltd.
The remaining authors declare that the research was conducted in the absence of any commercial or financial relationships that could be construed as a potential conflict of interest.
All claims expressed in this article are solely those of the authors and do not necessarily represent those of their affiliated organizations, or those of the publisher, the editors and the reviewers. Any product that may be evaluated in this article, or claim that may be made by its manufacturer, is not guaranteed or endorsed by the publisher.
ADAS UK Ltd. (2014). Study to model the impact of controlling endemic cattle diseases and conditions on national cattle productivity, agricultural performance and greenhouse gas emissions.
Adenuga, A. H., Jack, C., Olagunju, K. O., and Ashfield, A. (2020). Economic viability of adoption of automated oestrus detection technologies on dairy farms: a review. Animals 10:1241. doi: 10.3390/ani10071241
Balafoutis, A., Beck, B., Fountas, S., Vangeyte, J., Wal, T. V., Der Soto, I., et al. (2017). Precision agriculture technologies positively contributing to GHG emissions mitigation, farm productivity and economics. Sustain. For. 9:1339. doi: 10.3390/su9081339
Banhazi, T. M., Lehr, H., Black, J. L., Crabtree, H., Schofield, P., Tscharke, M., et al. (2012). Precision livestock farming: an international review of scientific and commercial aspects. Int. J. Agric. Biol. Eng. 5, 1–9. doi: 10.25165/ijabe.v5i3.599
Baruselli, P. S., de Abreu, L. Â., de Paula, V. R., Carvalho, B., Gricio, E. A., Mori, F. K., et al. (2023). Applying assisted reproductive technology and reproductive management to reduce CO2-equivalent emission in dairy and beef cattle: a review. Anim. Reprod. 20:e20230060. doi: 10.1590/1984-3143-AR2023-0060
Beattie, A. (2022). Farm management handbook 2022/23. Melrose: Farm Advisory Service (FAS), SAC Consulting. Available at: https://www.fas.scot/publication/farm-management-handbook-2022-23/
Berckmans, D. (2014). Precision livestock farming technologies for welfare management in intensive livestock systems. Rev. Sci. Tech. Int. Off. Epizoot. 33, 189–196. doi: 10.20506/rst.33.1.2273
Bowen, J. M., Haskell, M. J., Miller, G. A., Mason, C. S., Bell, D. J., and Duthie, C.-A. (2021). Early prediction of respiratory disease in preweaning dairy calves using feeding and activity behaviors. J. Dairy Sci. 104, 12009–12018. doi: 10.3168/jds.2021-20373
Brown, D. J., Savage, D. B., and Hinch, G. N. (2013). Repeatability and frequency of in-paddock sheep walk-over weights: implications for individual animal management. Anim. Prod. Sci. 54, 207–213. doi: 10.1071/AN12311
Chapa, J. M., Maschat, K., Iwersen, M., Baumgartner, J., and Drillich, M. (2020). Accelerometer systems as tools for health and welfare assessment in cattle and pigs – a review. Behav. Process. 181:104262. doi: 10.1016/j.beproc.2020.104262
Costa, C., Wollenberg, E., Benitez, M., Newman, R., Gardner, N., and Bellone, F. (2022). Roadmap for achieving net-zero emissions in global food systems by 2050. Sci. Rep. 12:15064. doi: 10.1038/s41598-022-18601-1
Cullen, B. R., Eckard, R. J., Timms, M., and Phelps, D. G. (2016). The effect of earlier mating and improving fertility on greenhouse gas emissions intensity of beef production in northern Australian herds. Rangel. J. 38, 283–290. doi: 10.1071/RJ15063
DEFRA (2012). Greenhouse gas reporting - Conversion factors. GOV.UK. Available at: https://www.gov.uk/government/publications/greenhouse-gas-reporting-conversion-factors-2011
de Mol, R. M., André, G., Bleumer, E. J. B., Van Der Werf, J. T. N., De Haas, Y., and Van Reenen, C. G. (2013). Applicability of day-to-day variation in behavior for the automated detection of lameness in dairy cows. J. Dairy Sci. 96, 3703–3712. doi: 10.3168/jds.2012-6305
Edwards-Jones, G., Plassmann, K., and Harris, I. M. (2009). Carbon footprinting of lamb and beef production systems: insights from an empirical analysis of farms in Wales, UK. J. Agric. Sci. 147, 707–719. doi: 10.1017/S0021859609990165
European Commission (2019). Going climate-neutral by 2050: a strategic long term vision for a prosperous, modern, competitive and climate neutral EU economy. Luxembourg: Publications Office of the European Union.
Fertilisers Europe (2018). The carbon footprint of fertiliser production: regional reference values. Available at: https://www.fertilizerseurope.com/wp-content/uploads/2020/01/The-carbon-footprint-of-fertilizer-production_Regional-reference-values.pdf
Fan, B., Bryant, R., and Greer, A. (2022). Behavioral fingerprinting: acceleration sensors for identifying changes in livestock health. J 5, 435–454. doi: 10.3390/j5040030
Ferguson, H. J., Bowen, J. M., McNicol, L. C., Bell, J., Duthie, C.-A., and Dewhurst, R. J. (2024). The impacts of precision livestock farming tools on the greenhouse gas emissions of an average Scottish dairy farm. Front. Sustain. Food Syst. 8:1385672. doi: 10.3389/fsufs.2024.1385672
Flachowsky, G. (2011). Carbon-footprints for food of animal origin, reduction potentials and research need. J. Appl. Anim. Res. 39, 2–14. doi: 10.1080/09712119.2011.570047
González, L. A., Kyriazakis, I., and Tedeschi, L. O. (2018). Review: precision nutrition of ruminants: approaches, challenges and potential gains. Animal 12, s246–s261. doi: 10.1017/S1751731118002288
Government of China (2021). China’s mid-century long-term low greenhouse gas emission development strategy. Beijing, China: Government of China.
Groher, T., Heitkämper, K., and Umstätter, C. (2020). Digital technology adoption in livestock production with a special focus on ruminant farming. Animal 14, 2404–2413. doi: 10.1017/S1751731120001391
Grossi, G., Goglio, P., Vitali, A., and Williams, A. G. (2019). Livestock and climate change: impact of livestock on climate and mitigation strategies. Anim. Front. 9, 69–76. doi: 10.1093/af/vfy034
Hammond, K. J., Waghorn, G. C., and Hegarty, R. S. (2016). The GreenFeed system for measurement of enteric methane emission from cattle. Anim. Prod. Sci. 56, 181–189. doi: 10.1071/AN15631
Hogeveen, H., and Steeneveld, W. (2013). Essential steps in the development of PLF systems for the dairy sector, In Proceedings of the 6th European conference of precision livestock farming. Presented at the 6th European conference of precision livestock farming, Leuven, Belgium, pp. 47–55.
Hristov, A. N., Oh, J., Firkins, J. L., Dijkstra, J., Kebreab, E., Waghorn, G., et al. (2013). SPECIAL TOPICS — mitigation of methane and nitrous oxide emissions from animal operations: I. A review of enteric methane mitigation options. J. Anim. Sci. 91, 5045–5069. doi: 10.2527/jas.2013-6583
IPCC (2007). “Climate Change 2007: Synthesis Report,” in Contribution of Working Groups I, II and III to the Fourth Assessment Report of the Intergovernmental Panel on Climate Change. eds. Core Writing Team, R. K. Pachauri, and A. Reisinger (Geneva, Switzerland: IPCC), 104 pp.
IPCC (2018). “Annex I: Glossary,” in: Global Warming of 1.5°C: IPCC Special Report on Impacts of Global Warming of 1.5°C above Pre-Industrial Levels in Context of Strengthening Response to Climate Change, Sustainable Development, and Efforts to Eradicate Poverty. Cambridge: Cambridge University Press, 541–562.
IPCC (2019). 2019 Refinement to the 2006 IPCC Guidelines for National Greenhouse Gas Inventories. Available at: https://www.ipcc.ch/report/2019-refinement-to-the-2006-ipcc-guidelines-for-national-greenhouse-gas-inventories/
Iqbal, M. W., Draganova, I., Morel, P. C. H., and Morris, S. T. (2021). Validation of an accelerometer sensor-based collar for monitoring grazing and rumination behaviours in grazing dairy cows. Animals 11:2724. doi: 10.3390/ani11092724
Jones, A. K., Jones, D. L., and Cross, P. (2014). The carbon footprint of lamb: sources of variation and opportunities for mitigation. Agric. Syst. 123, 97–107. doi: 10.1016/j.agsy.2013.09.006
Kamphuis, C., Steeneveld, W., and Hogeveen, H. (2015). “3.2. Economic modelling to evaluate the benefits of precision livestock farming technologies” in Precision livestock farming applications. ed. I. Halachmi (Wageningen, The Netherlands: Wageningen Academic Publishers), 87–94.
Kumari, S., Fagodiya, R. K., Hiloidhari, M., Dahiya, R. P., and Kumar, A. (2020). Methane production and estimation from livestock husbandry: a mechanistic understanding and emerging mitigation options. Sci. Total Environ. 709:136135. doi: 10.1016/j.scitotenv.2019.136135
Lima, E., Hopkins, T., Gurney, E., Shortall, O., Lovatt, F., Davies, P., et al. (2018). Drivers for precision livestock technology adoption: a study of factors associated with adoption of electronic identification technology by commercial sheep farmers in England and Wales. PLoS One 13:e0190489. doi: 10.1371/journal.pone.0190489
Makinde, A., Islam, M. M., Wood, K. M., Conlin, E., Williams, M., and Scott, S. D. (2022). Investigating perceptions, adoption, and use of digital technologies in the Canadian beef industry. Comput. Electron. Agric. 198:107095. doi: 10.1016/j.compag.2022.107095
National Atmospheric Emissions Inventory (2023). Greenhouse gas inventories for England, Scotland, Wales & Northern Ireland: 1990–2021 – DEFRA, UK: Department for Business, Energy & Industrial Strategy, National Atmospheric Emissions Inventory : London: Department for Business, Energy & Industrial Strategy. Available at: https://naei.beis.gov.uk/reports/reports?report_id=1110.
Nebel, R. L., Jones, C. M., and Roth, Z. (2011). “Reproduction, events and management | mating management: Detection of estrus,” in Encyclopedia of Dairy Sciences (Second Edition). ed. J. W. Fuquay (San Diego: Academic Press), 461–466.
Neethirajan, S. (2017). Recent advances in wearable sensors for animal health management. Sens. Bio-Sens. Res. 12, 15–29. doi: 10.1016/j.sbsr.2016.11.004
Nogoy, K. M. C., Chon, S., Park, J., Sivamani, S., Lee, D.-H., and Choi, S. H. (2022). High precision classification of resting and eating behaviors of cattle by using a collar-fitted triaxial accelerometer sensor. Sensors 22:5961. doi: 10.3390/s22165961
Pardo, G., del Prado, A., Fernández-Álvarez, J., Yáñez-Ruiz, D. R., and Belanche, A. (2022). Influence of precision livestock farming on the environmental performance of intensive dairy goat farms. J. Clean. Prod. 351:131518. doi: 10.1016/j.jclepro.2022.131518
Pfeiffer, J., Gandorfer, M., and Ettema, J. F. (2020). Evaluation of activity meters for estrus detection: a stochastic bioeconomic modeling approach. J. Dairy Sci. 103, 492–506. doi: 10.3168/jds.2019-17063
Roelofs, J. B., Erp-van, Van, and der Kooij, E. (2018). Estrus detection tools and their applicability in cattle: recent and perspectival situation. Estrus detection tools and their applicability in cattle: recent and perspectival situation 12, 498–504.
Rutten, C. J., Steeneveld, W., Inchaisri, C., and Hogeveen, H. (2014). An ex ante analysis on the use of activity meters for automated estrus detection: to invest or not to invest? J. Dairy Sci. 97, 6869–6887. doi: 10.3168/jds.2014-7948
Schillings, J., Bennett, R., and Rose, D. C. (2021). Exploring the potential of precision livestock farming technologies to help address farm animal welfare. Front. Anim. Sci 2:639678. doi: 10.3389/fanim.2021.639678
Segerkvist, K. A., Höglund, J., Österlund, H., Wik, C., Högberg, N., and Hessle, A. (2020). Automatic weighing as an animal health monitoring tool on pasture. Livest. Sci. 240:104157. doi: 10.1016/j.livsci.2020.104157
Smith, S. A., Popp, M. P., Keeton, D. R., West, C. P., Coffey, K. P., Nalley, L. L., et al. (2016). Economic and greenhouse gas emission response to pasture species composition, stocking rate, and weaning age by calving season, farm size, and pasture fertility. Agric. Resour. Econ. Rev. 45, 98–123. doi: 10.1017/age.2016.11
Tullo, E., Finzi, A., and Guarino, M. (2019). Review: environmental impact of livestock farming and precision livestock farming as a mitigation strategy. Sci. Total Environ. 650, 2751–2760. doi: 10.1016/j.scitotenv.2018.10.018
Tzanidakis, C., Tzamaloukas, O., Simitzis, P., and Panagakis, P. (2023). Precision livestock farming applications (PLF) for grazing animals. Agriculture 13:288. doi: 10.3390/agriculture13020288
UK Government (2023). Cattle Tracing System (CTS) online [WWW document]. Available at: https://www.gov.uk/cattle-tracing-online (Accessed February 2, 2024).
U.S. Department of State (2021). The long-term strategy of the United States: pathways to net-zero greenhouse gas emissions by 2050. Washington DC: United States Department of State and the United States Executive Office of the President.
Vellinga, T. V., Blonk, H., Marinussen, M., van Zeist, W. J., and Starmans, D. A. J. (2013). Methodology used in FeedPrint: a tool quantifying greenhouse gas emissions of feed production and utilization (No. 674). Lelystad: Wageningen UR Livestock Research.
Keywords: accelerometers, carbon footprint, fertility, health, modelling, production efficiency, sensors, sustainable agriculture
Citation: McNicol LC, Bowen JM, Ferguson HJ, Bell J, Dewhurst RJ and Duthie C-A (2024) Adoption of precision livestock farming technologies has the potential to mitigate greenhouse gas emissions from beef production. Front. Sustain. Food Syst. 8:1414858. doi: 10.3389/fsufs.2024.1414858
Received: 09 April 2024; Accepted: 14 June 2024;
Published: 27 June 2024.
Edited by:
Jagadeesh Yeluripati, The James Hutton Institute, United KingdomReviewed by:
Patricia D. Millner, Agricultural Research Service (USDA), United StatesCopyright © 2024 McNicol, Bowen, Ferguson, Bell, Dewhurst and Duthie. This is an open-access article distributed under the terms of the Creative Commons Attribution License (CC BY). The use, distribution or reproduction in other forums is permitted, provided the original author(s) and the copyright owner(s) are credited and that the original publication in this journal is cited, in accordance with accepted academic practice. No use, distribution or reproduction is permitted which does not comply with these terms.
*Correspondence: Louise C. McNicol, bG91aXNlLm1jbmljb2xAc3J1Yy5hYy51aw==
†These authors have contributed equally to this work and share first authorship
Disclaimer: All claims expressed in this article are solely those of the authors and do not necessarily represent those of their affiliated organizations, or those of the publisher, the editors and the reviewers. Any product that may be evaluated in this article or claim that may be made by its manufacturer is not guaranteed or endorsed by the publisher.
Research integrity at Frontiers
Learn more about the work of our research integrity team to safeguard the quality of each article we publish.