- 1Department of Agronomy, Hasanuddin University, Makassar, Indonesia
- 2Department of Soil Science, Faculty of Agriculture, Hasanuddin University, Makassar, Indonesia
- 3Department of Agribusiness, Faculty of Agriculture, Hasanuddin University, Makassar, Indonesia
- 4Taiwan International Cooperation and Development Fund (TaiwanICDF), Taipei, Taiwan
- 5International Rice Research Institute, University of the Philippines Los Baños, Los Baños, Philippines
- 6Department of Agronomy and Horticulture, Faculty of Agriculture, IPB University, Bogor, Indonesia
- 7Agrotechnology Study Program, University of West Sulawesi, Majene, Indonesia
- 8Research Center for Food Crops, Research Organization for Agriculture and Food, National Research and Innovation Agency, Cibinong, Indonesia
- 9Department of Crop Sciences, University of Illinois Urbana-Champaign, Urbana, IL, United States
- 10Plant Production Department, College of Food and Agriculture Sciences, King Saud University, Riyadh, Saudi Arabia
Implementing a cropping intensity program with rice cultivation four times a year (CI 400) can be achieved using early maturing varieties of rice. However, this development needs to pay attention to the adaptability of the varieties planted to ensure successful implementation. The adaptability approach is a combination of assessing stability and productivity potential. This concept has been developed and applied in several studies, including research on rice. However, this approach is considered less comprehensive because it is non-parametric and only focuses on one stability analysis. Therefore, a systematic integration of various stability analyses, including index methods, is needed to comprehensively assess adaptability, particularly for early-maturing rice in South Sulawesi. This region is characterized by a dynamic climate zone and is one of the top four highest rice producers in Indonesia. Meanwhile, this study aims to develop a comprehensive adaptability index and select the best early-maturing rice varieties, especially in South Sulawesi. The investigation was conducted in Bone, Soppeng, and Gowa over two seasons using a nested randomized complete block design, with organized replications in each environment (location-season). Additionally, there was a significant focus on the application of five early-maturing and two check rice varieties, with each factor repeated three times at each location, totaling 126 experimental units. The results showed that the adaptability index, by combining stability rank accumulation with yield min max standardization, was effective at assessing the yield potential and stability of early-maturing rice varieties in supporting CI 400. Inpari 13 had the best index value at 0.55, followed by Cakrabuana at 0.31; hence both were recommended as adaptive early-maturing rice varieties, especially in South Sulawesi.
1 Introduction
The enhancement of rice production is essential to sustainability and food security programs in many parts of Asia, including Indonesia, the fourth most populous country globally (Rozaki, 2020a,b). Despite the significant production of rice (Yuan et al., 2022; Bin Rahman and Zhang, 2023), domestic demands have not been met, leading to a rise in importation alongside population growth (Rozaki, 2020b; Hutapea et al., 2022; Fitrawaty et al., 2023). Land conversion and climate change further worsen the challenges faced in reducing rice production (Rumanti et al., 2018; Vogel et al., 2019; Fan et al., 2021). Addressing these issues requires planned and systematic efforts to innovate rice production methods, which are crucial for maintaining food security.
Optimization of production locations and intensification systems offers a pathway to increase rice production in Indonesia. Among the five locations with high rice production potential, South Sulawesi needs to be optimized (Octania, 2021) because the population of inhabitants exceeds the production level compared to proportions observed in East, Central, and West Java, as well as South Sumatra (Paiman et al., 2022). South Sulawesi has unique agroclimatic conditions featuring three distinct rain zone patterns divided into west, east, and transitional locations (Herniwati and Kadir, 2009; Yassi et al., 2023). Such diverse rain patterns offer opportunities for production sustainability enhancement, thereby suggesting this province as an ideal location for evaluating and optimizing agricultural intensification programs.
The development of potential rice varieties, particularly early-maturing types, presents an efficient and effective genetic solution as an optimization effort to increase production amid climate change challenges (Acquaah, 2012; Syukur et al., 2015). Early-maturing varieties, often requiring less than 100 days to be fully grown (Yun, 2023), promote greater yield by escaping extreme global warming conditions through resilience both in the dry and rainy seasons (Rohaeni and Ishaq, 2015; Ren et al., 2023). These varieties are suitable for the government program of CI 300 and CI 400 implementation (Supriatna, 2012; Sudana, 2016; Subekti and Umar, 2022), which aims to maximize planting frequency to three times and four times per year (Musa et al., 2023; Widiastuti et al., 2023). The program facilitates higher land use for consistently increased production in the face of rice field erosion. Several early-maturing varieties released by the Ministry of Agriculture include Inpari 13, Inpari 19, Cakrabuana, and Padjajaran (Barokah et al., 2021; Subekti and Umar, 2022), along with M70D registered by some private companies (Palobo et al., 2019). However, the potential of varieties remains underexplored in South Sulawesi, a province promising substantial rice development. This highlights the need for comprehensive assessment through a variety of stability and adaptability tests.
The stability test is a fundamental aspect of developing rice genotypes or varieties in a location (Reckling et al., 2021). This enables the exploration of potential interactions among varieties, environments, and growing seasons through systematic statistical analysis (Oladosu et al., 2017; Reckling et al., 2021; Pour-Aboughadareh et al., 2022). Previously, Jaruchai et al. (2018), Al-kordy et al. (2019), Sitaresmi et al. (2019), Akbar et al. (2021), Rawte et al. (2021), Aswidinnoor et al. (2023), and Lee et al. (2023) conducted stability analysis on potential rice strains. These stability analyses are focused on the approach of comparing a genotype to the population average at a particular location. This concept is continually being developed, both parametrically and non-parametrically, and is adapted to field cases, thereby increasing the accuracy level of interpretation (Pour-Aboughadareh et al., 2022). However, the general concepts used in this analysis were independent with a lack of quantitative synchronization, showing the need for optimization through an index-based method. Cinelli et al. (2021) stated that index development and ranking offered effective means of decision-making based on various criteria. Greveniotis et al. (2023), Lee et al. (2023), and Utami et al. (2023) reported the use of the index in stability analysis for rice and fava beans, respectively. These indices use several approaches, such as the yield stability index (YSI), which focuses on a semi-objective assessment of the importance of the Shukla stability analysis (Kang, 1993). Another approach is the additive main effects and multiplicative interaction (AMMI) stability index (ASI), which assesses genotypes based on the interaction between genotype eigenvalues on PC1 and PC2 in the AMMI analysis (Jambhulkar et al., 2014). However, index development should not only focus on plant stability potential but also consider yield aspects, both of which could be achieved through the adaptability index method.
The adaptability index is an assessment approach that combines the yield potential of a genotype with its special trait’s potential (Anshori et al., 2021), including the potential for stability. According to Deng et al. (2023) and Rahimi and Debnath (2023), index-based variety assessment is more comprehensive than relying solely on independent stability analysis. This shows that assessing adaptability is a solution to see the potential performance of a genotype in several locations. The development of adaptability concept based on stability was pioneered by Kang (1993), who combined the potential stability of Sukhla with the productivity of the tested genotypes. In addition, Farshadfar (2008) and Utami et al. (2023) also used an assessment of adaptation potential by combining AMMI and productivity ranking. However, both index concepts are only based on a non-parametric approach and focus on one stability approach. This is considered less effective in describing the adaptation potential of a genotype, so optimizing the development of a more complex adaptation index through a combination of various approaches to stability and productivity performance needs to be carried out. Optimization of adaptability index development seems to be a viable strategy for assessing the potential of early-maturing rice varieties in South Sulawesi. Therefore, this study aims to develop a comprehensive adaptability index in assessing the potential of early-maturing rice varieties and identify the best varieties to support the CI 400 program in South Sulawesi, a location with significant opportunities for rice development.
2 Materials and methods
This study was conducted in three mapped districts based on climatological zones and production potential. Tangke Bajeng Village, Gowa Regency, represents the climatological zone for the west coast with coordinates 5°18′3.020″ S, 119°26′30.833″ E. Meanwhile, the east coast climatological zone comprised Pajekko, Bone Regency, with coordinates 4°36′30.971″ S, 120°17′41.633″ E and Lalabata, Soppeng Regency, with coordinates 4°20′44.693″ S, 119°54′54.029″ E. These three were selected based on the potential for rice production in South Sulawesi, and all exploration activities were performed in two growing seasons from January to April 2022 (the 1st season) and June–September 2022 (the 2nd season), with the rainfall pattern rhythm of the locations depicted in Figure 1.
2.1 Experimental design
This study used a nested randomized complete block design, with organized replications in six environments consisting of three locations × two seasons. Five early-maturing varieties, including Padjajaran, Cakrabuana, Inpari 13, Inpari 19, and M70D, as well as two check varieties comprising Ciherang and Inpari 32, were tested in each environment. Subsequently, each factor was repeated three times at each location, generating 126 experimental units. The selection of the five early maturing rice varieties was based on recommendations from the Ministry of Agriculture regarding their maturity and productivity potential in several regions, especially on Java Island (Barokah et al., 2021; Subekti and Umar, 2022). However, the potential comparison in South Sulawesi has not been studied, so the study of the five varieties was carried out as a preliminary study. Meanwhile, the selection of the Ciherang and Inpari 32 varieties as a comparison was based on the high demand for seeds of these two varieties in South Sulawesi (Sitaresmi et al., 2023; Qadir et al., 2024).
2.2 Research procedure
The procedure in this study started with land processing into mud form through plowing and ponding, followed by mapping adjustment to the number of experimental units in one environment. The plot size was determined using a meter of 3.5 m × 3.5 m, with a 1-m gap between replicates. Simultaneously, the seed-sowing process was conducted by inducing sprouts for 2 × 24 h before sowing in the seedbed. After 15 days, the seedlings were transferred to the field with a spacing of 20 cm × 20 cm, resulting in 416 plants per plot, and were maintained according to the method by Anshori et al. (2019).
Rice maintenance activities included replanting, weeding, irrigation, fertilization, and pest management. Replanting was performed 7 days after planting to replace dead seedlings and adjust varieties, with weeding conducted mechanically and chemically after 30 days. The mechanical process required removing weeds around the plant, and the chemical process was carried out by spraying herbicides using a sprayer. Irrigation was initiated 20 days after transplanting (6 days following initial fertilization) by pouring water into the experimental field until the height reached ±5 cm above the soil surface. This activity was stopped during the second fertilization, allowing the soil to reach wetness similar to clay. Irrigation was resumed 5 days after fertilization, and during the primordial phase, water levels were raised to ±10 cm from the soil surface to suppress the growth of new tillers. NPK fertilizer was applied at a ratio of 200 kg N:100 kg P2O5:100 kg K2O after 14 days of planting, and the second stage of fertilization used urea after 35 days.
Pest and disease management were performed in the morning by sprinkling and spraying molluscicide chemicals. This process was adjusted to pests or pathogens attacking rice plants according to the respective phenological phase. Finally, harvesting was conducted manually using a sickle to collect ripe grains into sacks when 2/3 of the panicle had entered the physiological maturity phase (yellowing straw) and rice grains at the panicle base were hardened. During harvesting, data were obtained by observing the plant parts before inclusion in the sample bag.
2.3 Observation parameters and data analysis
The examination of data in this study focused on yield characteristics and started with an ANOVA, followed by stability and heritability tests. The stability test used the Finlay and Wilkinson (1963); Eberhart and Russell (1966), Kang, and AMMI plot methods with PBSTAT software (Sitaresmi et al., 2019; Aswidinnoor et al., 2023). The selection of the stability approach was based on the intersection of several commonly used research reports and a combination of representative non-parametric and parametric approaches (Sitaresmi et al., 2019; Pour-Aboughadareh et al., 2022). Stability test results were continued with the stability rank index (SRI), derived from a combination of rankings from each stability analysis. SRI applied the min-max standardization (SRIMMS ) method to combine ranking data from all stability analyses or the sum of stability rank (SSR). Next, SRIMMS was combined with a yield that has been standardized to develop an it is the adaptability index. The formula of this SRI_MMS and Adaptability Index was as follows:
3 Results
Table 1 shows the results of variety testing at several locations in two seasons in 2022. Each of the seasons, locations, and varieties factors as well as the interactions between seasons-locations, varieties-seasons, varieties-locations, and varieties-seasons-locations significantly affected yield. This phenomenon suggested that diverse local varieties had various interaction dynamics in the same and different seasons. The dynamic variations necessitated the systematic analysis of the performance of early-maturing rice varieties in each environment based on interactions between environments and seasons.
The yield of early-maturing rice varieties against locations and seasons is presented in Table 2. Bone planting locations in the first season showed the highest average yield compared to other environments. However, the lowest average yield was observed in the Gowa district in the first season. Different seasons had various impacts on yield, as the first growing season was relatively better than the second in the Gowa and Soppeng districts. The Gowa district recorded the highest yield in the second growing season. Additionally, Inpari 32 was a variety with the best yield performance compared to other rice types. The M70D had the lowest yield, but in some locations, this variety and Inpari 19 produced a similar result. Moreover, all locations showed high heritability with a value greater than 50%, particularly, the Bone location recorded the highest heritability.
The results of the Finlay–Wilkinson, Eberhart–Russel, and Kang stability analysis are shown in Table 3. Through the evaluation performed using the Finlay–Wilkinson method, all varieties and the comparators had average stable yield, except for the Padjajaran early maturing rice variety (bi = 0.7). In contrast, based on Eberhart–Russell’s deviation from regression (s2di) parameter, all varieties were considered to have unstable yield. Kang’s stability method showed that Inpari 32 (YSi = 2) was deemed to have the best stability rating, followed by Ciherang (YSi = 1) and Inpari 13 (YSi = −1).
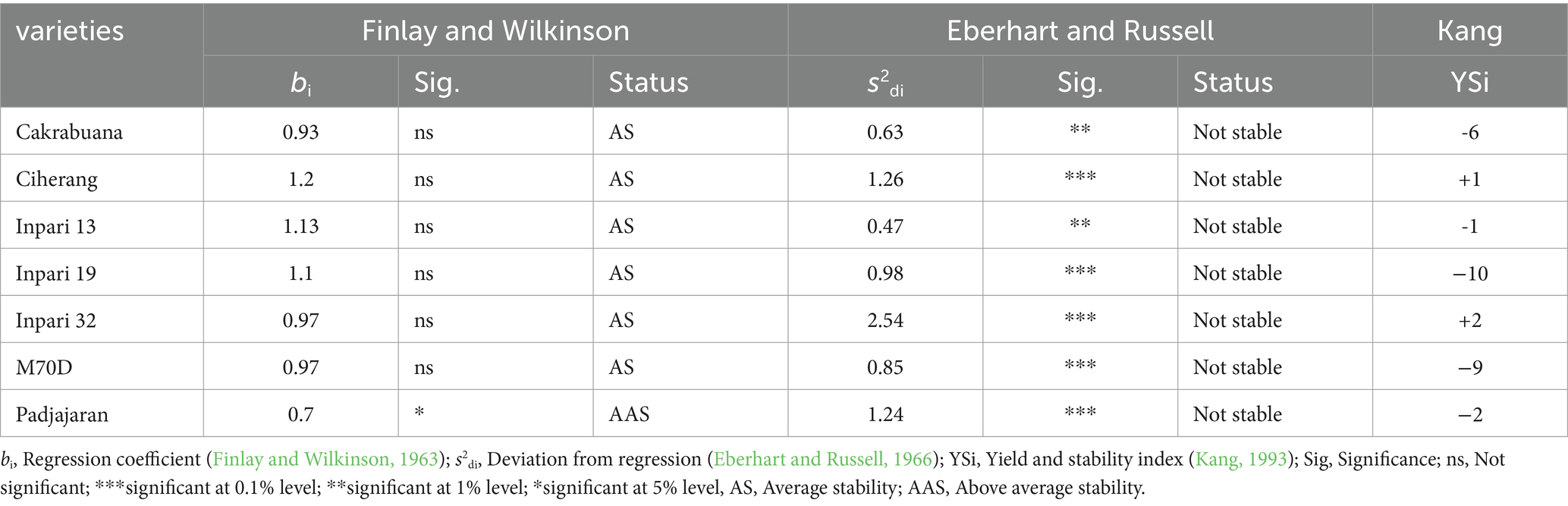
Table 3. The yield stability testing based on Finlay–Wilkinson, Eberhart–Russel, and Kang’s analysis.
According to Figure 3, the Additive Main Effects and Multiplicative Interaction (AMMI) analysis results are presented in two-dimensional forms. Gowa 1, Soppeng 2, and Soppeng 1 were found to be relatively stable compared to other environments. Based on varietal potential, Inpari 13 was the only genotype in the stability circle, followed by Ciherang and Inpari 19 varieties. Meanwhile, the specific variety with low AMMI stability was observed in the Padjajaran location.
The results of the entire stability analysis were converted into SRI and Adaptability Index, as shown in Table 4. The Padjajaaran variety (0.75) had the highest SRI, followed by Cakrabuana (0.5) and Inpari 32 (0.5), while Inpari 13 (0.29) had the lowest SRI. The highest adaptability index belonged to Inpari 32 (0.5), followed by Ciherang (0.44) and Inpari 13 (0.14), while the lowest was generated by the M70D variety (−0.41).
The potential harvesting age of each genotype was presented in Table 5, showing that Inpari 32 (116.0 days) had the longest harvesting age, followed by Ciherang (108.8 days). In contrast, the M70D variety (91.3 days) was the slowest, followed by Inpari 19 (93.1 days). These age results were converted into the harvest index, where M70D had an optimal CI 400 potential. Other varieties, such as Cakrabuana, Inpari 13, Inpari 19, and Padjajaran, had CI 400 potential under the growing condition of the seedling system. Meanwhile, the Padjajaran variety showed the most significant projected annual production potential, with a value of 28.24 tons per ha, and the Ciherang variety presented the lowest value at 23.37.
The results of the interaction between SRI_MMS and yield per year formed the annual adaptability index, as presented in Table 6. Varieties with a positive annual adaptability index included Cakrabuana (0.31), Inpari 13 (0.55), and Padjajaran (0.25). In contrast, M70 D (−1.27), Inpari 32 (−0.38), Inpari 19 (−0.43), and Ciherang (−0.46) had negative adaptability index values. Meanwhile, based on the adaptation relationship (Figure 4), Cakrabuana and Inpari 13 are in the same group with good potential on all three indices [SRI, Adaptability Index per Season (AIS), and Adaptability Index per Year (AIY)]. Inpari 32 and Ciherang are in the same group with good SRI and AIS potential. The Padjajaran variety is in a separate group with good SRI and AIY potential. The M70D variety also has good SRI potential. On the other hand, Inpari 19 is also grouped alone with low potential on these three indices.
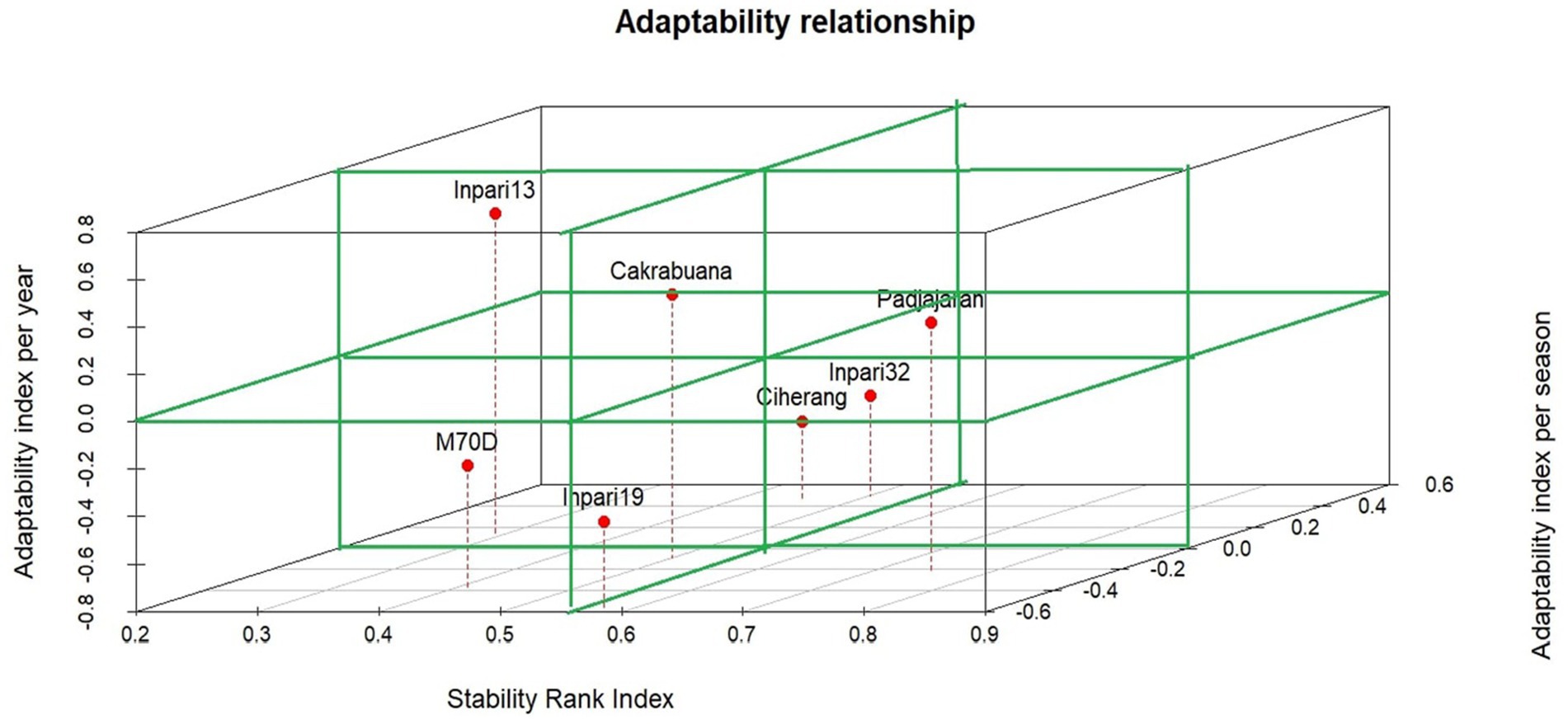
Figure 4. Adaptability relationship among stability rank index, adaptability index per season, and adaptability index per year.
4 Discussion
The results of the multi-site analysis showed significant differences between locations and seasons due to the disparities in rainfall patterns and agroecosystems, which varied between locations, particularly Gowa (Figure 1). The location of Gowa has a climatological profile contrasting with that of the Bone and Soppeng districts. According to Gérardeaux et al. (2021) and Rezvi et al. (2023), differences in rain patterns greatly determine the potential for crop production, especially rice as a C3 crop. Rice requires a high amount of water with sufficient light levels (Materu et al., 2018; Mallareddy et al., 2023). These optimal conditions can support rice production potential. On the other hand, if water conditions are lacking or sunlight is less intense, then the production potential will not be optimal or tends to be much different from the potential yield of the variety (Mallareddy et al., 2023; Loaiza et al., 2024). Especially for early-maturing rice varieties, the distribution pattern of water adequacy in these varieties is very important because they have a period of rapid change from phase to phase (Yun et al., 2023), so the influence of the seasons and their interactions on the variety will be very large in early-maturing rice varieties. Furthermore, differences in soil conditions, especially in the Gowa area compared to the Bone and Soppeng areas, cause significant differences between environments. The land used for planting in Bajeng, Gowa Regency, contains sedimentary or alluvial soil near the coast, while Soppeng and Gowa are situated close to the mountains. These differences made all interactions significantly affect the response of early-maturing rice yield. A real interaction would influence the specific potential of variety in the growing environment, including both the location and season (Satoto et al., 2016; Oladosu et al., 2017; Sharifi et al., 2017; Krishnamurthy et al., 2021; Senguttuvel et al., 2021). This served as evidence that the current study conducted a deeper analysis of the potential stability of optimal early-maturing rice yield.
The potential of Bone Regency for rice production was highly recommended during the evaluation process. This was observed from the average yield potential and yield heritability per location. Determining yield heritability per location is very important in assessing the potential of a location as part of the experimental environment. Generally, higher heritability promotes the evaluation process accuracy in assessing the potential of variety (Tal, 2012; Khan et al., 2022; Zaid et al., 2022), including the interaction made with the environment. Therefore, Bone Regency was optimally recommended as a suitable environment for testing rice yield potential in South Sulawesi, specifically on the east coast.
The results showed that the Inpari 32 variety had the highest yield potential in most locations, followed by Ciherang. These results were reported by both Agustian et al. (2022) and Qadir et al. (2024). Inpari 32 and Ciherang were found to be relatively long-lived with a more extensive phenotype than early-maturing rice varieties. This difference was observed by Musa et al. (2023) during fertilizer dose evaluation in early-maturing varieties and by Aswidinnoor et al. (2023) while testing new rice types in Indonesia. In general, rice age is primarily assessed with the vegetative phase (Paiman et al., 2022; Sheng et al., 2022; Afa et al., 2023). The vegetative phase determines the potential photosynthetic area of plants (Hidayati et al., 2016; Paiman et al., 2022), leading to low yield among plants with shortages. However, under certain conditions, the potential of these early-maturing varieties can be optimized with specific interactions manifested. This study identified that the potential yield of early-maturing varieties in some locations nearly exceeded the potential yield of Inpari 32 and Ciherang. Early-maturing rice varieties with high potential values were Padjajaran, followed by Inpari 13. Additionally, the potential yield of the Padjajaran variety was reported by Surdianto et al. (2021), Syafruddin et al. (2023), and Musa et al. (2023). Based on this, stability and specific interactions of the yield of early-maturing rice varieties were tested.
The variety stability test in this study used several dynamic methods to assess the response of all examined genotypes. This aims to strengthen the determination of variety stability levels because each method has unique advantages and specifications complementing one another (Oladosu et al., 2017; Jaruchai et al., 2018; Pour-Aboughadareh et al., 2022; Lee et al., 2023). The Finlay–Wilkinson and Eberhart–Russel methods are an essential combination in estimating genotype dynamic stability through the regression response of each object and error on the regression line (Satoto et al., 2016; Jaruchai et al., 2018; Sitaresmi et al., 2019; Aswidinnoor et al., 2023). This suggests that stable genotypes respond linearly to changes in the environment, and the changes have a strong precision level characterized by a slight error deviation of the regression line. Varieties M70D, Inpari 13, Cakrabuana, and Inpari 19 were genotypes considered relatively stable from the combination. However, in the Eberhart–Russell analysis, the values of the four varieties were declared unstable. These four had standard error values compared to the check varieties Inpari 32 and Ciherang; hence, M70D, Inpari 13, Cakrabuana, and Inpari 19 were described as early-maturing varieties with good stability based on the combination. The potential of the four varieties was affirmed by the Kang and AMMI methods, which identified Inpari 13 as a stable variety with good yield potential. This assessment is supported by the Kang stability value, which is close to positive compared to other early-maturing varieties. Kang’s method offers the advantage of providing a rough or subjective midpoint between stability and yield (Sitaresmi et al., 2019; Pour-Aboughadareh et al., 2022).
The AMMI method also reinforces the stability evaluation concept by using the principal component analysis (PCA) to partition the genetic–environment interaction (GEI) diversity into simpler dimensions while retaining most of the original diversity (Adjebeng-Danquah et al., 2017; Sharifi et al., 2017; Sitaresmi et al., 2019; Pour-Aboughadareh et al., 2022). This is enhanced by using the biplot method to visualize simplified interaction dimensions (Sharifi et al., 2017; Senguttuvel et al., 2021; Pour-Aboughadareh et al., 2022), facilitating the identification of genotype stability based on the central axis coordinates. Inpari 13 was found as the only variety with coordinates close to the central axis, suggesting that it had stability toward the compaction of diversity and decomposition of interactions in all environments. Meanwhile, the Padjajaran variety showed environment-specific potential in Gowa during the second season, making it recommended for cultivation in Gowa. This study identified Inpari 13 as the most stable early-maturing variety, and the conducted analysis was further refined objectively through the adaptability index value.
Adaptability index development in this study focused on ranking and min-max standardization to improve the classification of potential combinations of stability and yield (Cinelli et al., 2021; Shantal et al., 2023). The use of this index serves as a midpoint for combining data of different natures, as demonstrated in this study. The stability index is an accumulation of rankings from several stability analysis approaches, resulting in non-parametric data (Pour-Aboughadareh et al., 2022; Utami et al., 2023). Meanwhile, the resulting data are continuous and parametric (Pour-Aboughadareh et al., 2022). The combination of these two data needs to be bridged by looking at the potential max-min limits. This standardization will produce a data range of 0–1, allowing for the combination of the two (Cinelli et al., 2021; Shantal et al., 2023). The effectiveness of using min-max standardization for crop evaluation was reported by Blüthgen et al. (2006), Farid et al. (2020), and Noel et al. (2021), hence this systematic method was implemented in the adaptability index development.
The results also showed that Inpari 13 was an early-maturing rice variety with a good adaptability index per season. This index was less than the values obtained for Inpari 32 and Ciherang. The Cakrabuana variety could be categorized as good despite possessing a negative adaptability index. The index value of standardization close to 0 suggests that the response of a genotype is near the general average (Peternelli et al., 2017; Anshori et al., 2021; Rawte et al., 2021), making it worthy of consideration in the selection process. In contrast, the Padjajaran variety had a low adaptability index value. In this study, the adaptability index method provided a reasonably objective penalty for genotypes that were not consistent with stability and yield potential. The adaptability index is effective in dynamically assessing genotype stability and yield potential, but it needs to be developed based on the cropping index.
Based on plant age evaluation, all early-maturing rice can be optimized for planting four times per year through a program known as Cropping Intensity (CI) 400, while the check varieties are limited to 2–3 seasons. This showed that the annual yield of the check varieties Inpari 32 and Ciherang was less than optimal despite possessing a good adaptability index per season. Early-maturing varieties can increase yield potential to exceed the value observed among the two comparators through the CI 400 program. Most of these varieties require an abducted seedling system or seedling before harvest to reach CI 400, except for M70D, which often matures very early (Palobo et al., 2019). Due to the adaptability index manifested in one season, M70D is classified as highly non-adaptive and dependent on environmental conditions. These characteristics were reflected by the high yield observed during the first season in the Bone location, while the lowest was recorded in the second season, suggesting the unsuitability of M70D for the CI 400 system, specifically on a repetitive basis. The ineffectiveness of M70D was further reflected by the negative adaptability index per year found in this variety, along with Inpari 19 and the two comparators, namely Inpari 32 and Ciherang. Inpari 13, Cakrabuana, and Padjajaran were varieties with a positive annual adaptability index, supporting the recommendation of Inpari 13 and Cakrabuana for the CI 400 planting system in South Sulawesi. Additionally, Padjajaran had good potential and responsive traits, leading to the suitability of this variety for specific environmental requirements, such as in the Gowa location. This result is also supported by Musa et al. (2023), where the Cakrabuana and Inpari 13 varieties have good consistency in responding to NPK fertilization in the Bone and Gowa environmental interactions. This phenomenon indicates that planting early-maturing rice varieties such as Inpari 13 and Cakrabuana can be widely carried out under random nutritional conditions, especially in two climate zones in South Sulawesi. The use of these two varieties will correlate with the stability of farmer income. Areas with optimal water potential per year will be in accordance with the CI 400 program, where Inpari 13 and Cakrabuana are the main varieties. Meanwhile, rainfed land still has the potential to plant 1–2 times per year, which can be optimized to 2–3 times per year by utilizing the early maturing varieties Inpari 13 and Cakrabuana (Sulaiman et al., 2019; Oue and Laban, 2020). However, this research still needs to be studied extensively considering the limitations of covering the data and analysis process in this research.
The limitations of this research are closely related to three aspects. The first aspect is the wide diversity of genotypes tested. The genotypes used in this research are still limited to early-maturing rice varieties that have been released by the Indonesian government. The use of other genotypes, whether from the breeding process, results of exploration, or introduction, can be used as material for wider studies. However, the potential of these five varieties could be the first step in developing an adaptation index to support the CI 400 program. The second aspect of limitation is the development of other climate patterns in South Sulawesi, where the development of this concept has not yet reached the transition climate area. It is possible that the transition climate has a different response pattern from the two climate patterns in this study. However, this pattern will not have a drastic impact because water availability in the transition area is sufficient throughout the year (Yassi et al., 2023), so research focuses more on the two western and eastern climate zones. In addition, the two zones in this study can be a general representation of several regions outside Sulawesi where climate patterns are relatively similar. The third limitation is the development of stability analysis that does not yet include machine learning concepts. The development of machine learning analysis has become a development that is widely used in various fields (Kobler and Ademic, 2000; Chang and Lin, 2011; Pham et al., 2017; Guo et al., 2021, 2022, 2023). This potential analysis requires more precise data with wider dimensions so that the accuracy of the assessment can be better (Guo et al., 2021, 2022, 2023). This can be used as reference material in the future to analyze the concept of stability more precisely, including in assessing the adaptation potential of early-maturing rice varieties. However, the analytical concept in this research has become a sufficient initial basis for overcoming the problem of assessing the adaptability of early-maturing rice varieties. Therefore, the results of this research, both from the analysis concept and determining the adaptability of varieties, can be a reference and recommendation for sustainable early maturing rice cultivation.
5 Conclusion
In conclusion, this study identified the use of several climatological zone-based environments in South Sulawesi to be very effective in assessing the potential stability of early-maturing rice varieties. Moreover, the assessment of adaptability achieved through the index method, derived from a combination of various stability analyses, could serve as a reference in evaluating the potential yield and stability of early-maturing rice varieties, both per season and year. Inpari 13 and Cakrabuana are recommended as stable and adaptive early-maturing rice varieties suitable for the CI 400 program. The Padjajaran variety showed potential adaptability, but it exhibited specificity for certain locations, particularly in Gowa Regency. Therefore, these three varieties needed broader investigation, specifically in the transitional climatological zone of South Sulawesi.
Resource identification initiative: the project uses STAR 2.0.1 from IRRI, PBStat 3.1, and Excel Office 2016 version for varieties resources from the rice seed market in Indonesia.
Data availability statement
The raw data supporting the conclusions of this article will be made available by the authors, without undue reservation.
Author contributions
MA: Writing – review & editing, Writing – original draft, Validation, Software, Methodology, Investigation, Formal Analysis, Conceptualization. YM: Writing – review & editing, Validation, Supervision, Conceptualization. MF: Writing – review & editing, Methodology, Investigation. MJ: Writing – review & editing, Methodology, Investigation. AB: Writing – review & editing, Methodology, Investigation. AY: Writing – review & editing, Methodology, Investigation. AS: Writing – review & editing, Resources, Funding acquisition. YH: Writing – review & editing, Resources, Funding acquisition, Conceptualization. MC: Writing – review & editing, Supervision, Resources, Conceptualization. LL: Writing – review & editing, Supervision. WS: Writing – review & editing, Writing – original draft, Visualization, Software, Formal Analysis. MP: Writing – review & editing, Methodology, Investigation. HS: Writing – review & editing, Supervision, Resources. BP: Writing – review & editing, Data curation. DW: Writing – review & editing, Funding acquisition. MS: Writing – review & editing, Validation, Funding acquisition.
Funding
The author(s) declare that financial support was received for the research, authorship, and/or publication of this article. Funding for this study was provided by Researchers Supporting Project number (RSPD2024R751) from King Saud University, Riyadh, Saudi Arabia, and The International Collaboration Research (Icore) 2023–2024 scheme (grant number: 04966/UN4.22/PT.01/0.03/2023) involving Hasanuddin University, the International Cooperation and Development Fund (ICDF) in Taiwan, and the International Rice Research Institute, over a period of 3 years (2022–2024).
Acknowledgments
We thank the Researchers Supporting Project number (RSPD2024R751) from King Saud University, Riyadh, Saudi Arabia, for developing and supporting this manuscript. We are grateful to the Ministry of Education, Culture, Research, and Technology of the Republic of Indonesia, the International Cooperation and Development Fund (ICDF) in Taiwan, and the International Rice Research Institute for funding this study during two seasons through the scheme of International Collaboration Research (Icore) 2023 (grant number: 04966/UN4.22/PT.01.03/2023). Furthermore, we also thank Hasanuddin University’s publication management center for providing facilities, such as training workshops, and Enago for proofreading this article.
Conflict of interest
The authors declare that the research was conducted in the absence of any commercial or financial relationships that could be construed as a potential conflict of interest.
Publisher's note
All claims expressed in this article are solely those of the authors and do not necessarily represent those of their affiliated organizations, or those of the publisher, the editors and the reviewers. Any product that may be evaluated in this article, or claim that may be made by its manufacturer, is not guaranteed or endorsed by the publisher.
References
Acquaah, G. (2012). Principles of Plant Genetics and Breeding. Second Edition. West Sussex: John Wiley & Sons.
Adjebeng-Danquah, J., Manu-Aduening, J., Gracen, V. E., Asante, I. K., and Offei, S. K. (2017). AMMI stability analysis and estimation of genetic parameters for growth and yield components in cassava in the Forest and Guinea Savannah ecologies of Ghana. Int. J. Agron. 2017, 1–10. doi: 10.1155/2017/8075846
Afa, L. O., Ansi, A., Zulfikar, Z., Muhidin, M., and Al Qadri, A. (2023). Growth and yield of local upland Rice (Oryza sativa L.) Wakawondu cultivar in various plant populations and balanced fertilization. Bulet. Peneli. Sosial Ekon. Pertan. Fakultas Pertan. Univ. Haluoleo 24, 88–98. doi: 10.37149/bpsosek.v24i2.288
Agustian, A., Aldillah, R., Nurjati, E., Yaumidin, U. K., Muslim, C., Ariningsih, E., et al. (2022). Analysis of the utilization of rice seeds of improved variety (Inpari 32) in Indramayu District, West Java. IOP Conf. Ser. Earth Environ. Sci. 1114:012098. doi: 10.1088/1755-1315/1114/1/012098
Akbar, M. R., Purwoko, B. S., Dewi, I. S., and Suwarno, W. B., and Sugiyanta (2021). Genotype × environment interaction and stability analysis for high yielding doubled haploid lines of lowland Rice. Turk. J. Field Crops 26, 218–225. doi: 10.17557/tjfc.1033784
Al-kordy, M. A., Ibrahim, H. F., El-Mouhamady, A. B. A., and Abdel-Rahman, H. M. (2019). Genetic stability analysis and molecular depiction in elite entries of rice (Oryza sativa L.). Bull. Natl. Res. Cent. 43:85. doi: 10.1186/s42269-019-0100-3
Anshori, M. F., Purwoko, B. S., Dewi, I. S., Ardie, S. W., and Suwarno, W. B. (2019). Selection index based on multivariate analysis for selecting doubled-haploid rice lines in lowland saline prone area. SABRAO J. Breed. Genet. 51, 161–174.
Anshori, M. F., Purwoko, B. S., Dewi, I. S., Ardie, S. W., and Suwarno, W. B. (2021). A new approach to select doubled haploid Rice lines under salinity stress using indirect selection index. Rice Sci. 28, 368–378. doi: 10.1016/j.rsci.2021.05.007
Aswidinnoor, H., Listiyanto, R., Rahim, S., Holidin,, Setiyowati, H., Nindita, A., et al. (2023). Stability analysis, agronomic performance, and grain quality of elite new plant type rice lines (Oryza sativa L.) developed for tropical lowland ecosystem. Front. Sustain. Food Syst. 7:1147611. doi: 10.3389/fsufs.2023.1147611
Barokah, U., Nugroho, R., and Huda, M. (2021). Uji Adaptasi Varietas Unggul Baru Padi Sawah Berbasis Penerapan Teknologi Terpadu Di Kecamatan Karangsambung Kabupaten Kebumen. Cermin 5, 36–50. doi: 10.36841/cermin_unars.v5i1.764
Bin Rahman, A. N. M. R., and Zhang, J. (2023). Trends in rice research: 2030 and beyond. Food Energy Secur. 12, 1–17. doi: 10.1002/fes3.390
Blüthgen, N., Menzel, F., and Blüthgen, N. (2006). Measuring specialization in species interaction networks. BMC Ecol. 6:9. doi: 10.1186/1472-6785-6-9
Chang, C.-C., and Lin, C.-J. (2011). LIBSVM: a library for support vector machines. ACM T Intel. Syst. Techcol. 2, 1–27. doi: 10.1145/1961189.1961199
Cinelli, M., Spada, M., Kim, W., Zhang, Y., and Burgherr, P. (2021). MCDA index tool: an interactive software to develop indices and rankings. Environ. Syst. Decis. 41, 82–109. doi: 10.1007/s10669-020-09784-x
Deng, C. H., Naithani, S., Kumari, S., Cobo-Simón, I., Quezada-Rodríguez, E. H., Skrabisova, M., et al. (2023). Genotype and phenotype data standardization, utilization and integration in the big data era for agricultural sciences. Database 2023:baad088. doi: 10.1093/database/baad088
Eberhart, S. A. T., and Russell, W. A. (1966). Stability parameters for comparing varieties. Crop Sci. 6, 36–40. doi: doi: 10.2135/cropsci1966.0011183X000600010011x
Fan, X., Zhu, D., Sun, X., Wang, J., Wang, M., Wang, S., et al. (2021). Impacts of extreme temperature and precipitation on crops during the growing season in South Asia. Remote Sens. 14:6093. doi: 10.3390/rs14236093
Farid, M., Nasaruddin, N., Musa, Y., Anshori, M. F., Ridwan, I., Hendra, J., et al. (2020). Genetic parameters and multivariate analysis to determine secondary traits in selecting wheat mutant adaptive on tropical lowlands. Plant Breed. Biotechnol. 8, 368–377. doi: 10.9787/PBB.2020.8.4.368
Farshadfar, E. (2008). Incorporation of AMMI stability value and grain yield in a single non-parametric index (GSI) in bread wheat. Pak. J. Biol. Sci. 11, 1791–1796. doi: 10.3923/pjbs.2008.1791.1796
Finlay, K. W., and Wilkinson, G. N. (1963). Adaptation in a plant breeding programme. Aust. J. Agric. Res. 14, 742–754. doi: 10.1071/AR9630742
Fitrawaty,, Hermawan, W., Yusuf, M., and Maipita, I. (2023). A simulation of increasing rice price toward the disparity of income distribution: an evidence from Indonesia. Heliyon 9:e13785. doi: 10.1016/j.heliyon.2023.e13785
Gérardeaux, E., Falconnier, G., Gozé, E., Defrance, D., Kouakou, P.-M., Loison, R., et al. (2021). Adapting rainfed rice to climate change: a case study in Senegal. Agron. Sustain. Dev. 41:57. doi: 10.1007/s13593-021-00710-2
Greveniotis, V., Bouloumpasi, E., Zotis, S., Korkovelos, A., Kantas, D., and Ipsilandis, C. G. (2023). Genotype-by-environment interaction analysis for quantity and quality traits in Faba beans using AMMI, GGE models, and stability indices. Plan. Theory 12:3769. doi: 10.3390/plants12213769
Guo, Y., Chen, S., Li, X., Cunha, M., Jayavelu, S., Cammarano, D., et al. (2022). Machine learning-based approaches for predicting SPAD values of maize using multi-spectral images. Remote Sens. 14:1337. doi: 10.3390/rs14061337
Guo, Y., Fu, Y., Hao, F., Zhang, X., Wu, W., Jin, X., et al. (2021). Integrated phenology and climate in rice yields prediction using machine learning methods. Ecol. Indic. 120:106935. doi: 10.1016/j.ecolind.2020.106935
Guo, Y., Xiao, Y., Hao, F., Zhang, X., Chen, J., de Beurs, K., et al. (2023). Comparison of different machine learning algorithms for predicting maize grain yield using UAV-based hyperspectral images. Int. J. Appl. Earth Obs. Geoinf. 124:103528. doi: 10.1016/j.jag.2023.103528
Herniwati,, and Kadir, S. (2009). “Potensi Iklim, Sumber Daya Lahan Dan Pola Tanam Di Sulawesi Selatan” in Prosiding seminar nasional serealia.
Hidayati, N., Triadiati,, and Anas, I. (2016). Photosynthesis and transpiration rates of Rice cultivated under the system of Rice intensification and the effects on growth and yield. HAYAT 23, 67–72. doi: 10.1016/j.hjb.2016.06.002
Hutapea, R. T. P., Prayogo Ramadhan, R., Meity, A. T., and Ngongo, Y. (2022). Competitiveness of Indonesian Rice prices in the international market. E3S Web Conf. 361, 1–12. doi: 10.1051/e3sconf/202236101016
Jambhulkar, N.N., Bose, L.K., and Singh, O.N. (2014). AMMI stability index for stability analysis. ed. Mohapatra, T. Central Rice Research Institute, Cuttack-IN. 35, 15.
Jaruchai, W., Monkham, T., Chankaew, S., Suriharn, B., and Sanitchon, J. (2018). Evaluation of stability and yield potential of upland rice genotypes in north and Northeast Thailand. J. Integr. Agric. 17, 28–36. doi: 10.1016/S2095-3119(16)61609-X
Kang, M. S. (1993). Simultaneous selection for yield and stability in crop performance trials: consequences for growers. Agron. J. 85, 754–757. doi: 10.2134/agronj1993.00021962008500030042x
Khan, M. M. H., Rafii, M. Y., Ramlee, S. I., Jusoh, M., and Al Mamun, M. (2022). Hereditary analysis and genotype × environment interaction effects on growth and yield components of Bambara groundnut (Vigna subterranea (L.) Verdc.) over multi-environments. Sci. Rep. 12, 1–19. doi: 10.1038/s41598-022-19003-z
Kobler, A., and Ademic, M. (2000). Identifying brown bear habitat by a combined GIS and machine learning method. Ecol. Model. 135, 291–300. doi: 10.1016/S0304-3800(00)00384-7
Krishnamurthy, S. L., Sharma, P. C., Sharma, D. K., Singh, Y. P., Mishra, V. K., Burman, D., et al. (2021). Additive main effects and multiplicative interaction analyses of yield performance in rice genotypes for general and specific adaptation to salt stress in locations in India. Euphytica 217, 1–15. doi: 10.1007/s10681-020-02730-7
Lee, S. Y., Lee, H. S., Lee, C. M., Ha, S. K., Park, H. M., Lee, S. M., et al. (2023). Multi-environment trials and stability analysis for yield-related traits of commercial Rice cultivars. Agriculture 13. doi: 10.3390/agriculture13020256
Loaiza, S., Verchot, L., Valencia, D., Guzmán, P., Amezquita, N., Garcés, G., et al. (2024). Evaluating greenhouse gas mitigation through alternate wetting and drying irrigation in Colombian rice production. Agric. Ecosyst. Environ. 360:108787. doi: 10.1016/j.agee.2023.108787
Mallareddy, M., Thirumalaikumar, R., Balasubramanian, P., Naseeruddin, R., Nithya, N., Mariadoss, A., et al. (2023). Maximizing water use efficiency in Rice farming: a comprehensive review of innovative irrigation management technologies. WaterSA 15. doi: 10.3390/w15101802
Materu, S. T., Shukla, S., Sishodia, R. P., Tarimo, A., and Tumbo, S. D. (2018). Water use and rice productivity for irrigation management alternatives in Tanzania. WaterSA 10. doi: 10.3390/w10081018
Musa, Y., Farid, M., Nasaruddin, N., Anshori, M. F., Adzima, A. F., Maricar, M. F., et al. (2023). Sustainability approach in cropping intensity (CI) 400 through optimizing the dosage of compost and chemical fertilizers to early-maturing rice varieties based on multivariate analysis. J. Agric. Food Res. 14:100907. doi: 10.1016/j.jafr.2023.100907
Noel, D. D., Kablan, G. A. J., Alui, K. A., Lallié, H. D., Dagnogo, D., Diarrassouba, N., et al. (2021). Normality assessment of several quantitative data transformation procedures. Biostat. Biomed. Open Access J. 10. doi: 10.19080/bboaj.2021.10.555786
Octania, G. (2021). The Government’s role in the Indonesian Rice supply chain. Cips-Indonesia, 8–42.
Oladosu, Y., Rafii, M. Y., Abdullah, N., Magaji, U., Miah, G., Hussin, G., et al. (2017). Genotype × environment interaction and stability analyses of yield and yield components of established and mutant rice genotypes tested in multiple locations in Malaysia*. Acta Agric. Scand. Sect. B Soil Plant Sci. 67, 590–606. doi: 10.1080/09064710.2017.1321138
Oue, H., and Laban, S. (2020). Water use of rice and mung bean cultivations in a downstream area of an irrigation system in South Sulawesi in the 2nd dry season. Paddy Water Environ. 18, 87–98. doi: 10.1007/s10333-019-00766-7
Paiman, B. H. I., Aziez, A. F. S., and Salisu, M. A. (2022). The role of agronomic factors in Salibu Rice cultivation. Open Agric. J. 16, 1–7. doi: 10.2174/18743315-v16-e2112170
Palobo, F., Tirajoh, S., and Thamrin, M. (2019). Pengembangan Padi Sawah Melalui Pendekatan Pengelolaan Ramah Lingkungan di Kabupaten Merauke. J. Budidaya Pertan. 15, 44–50. doi: 10.30598/jbdp.2019.15.1.44
Peternelli, L. A., Moreira, É. F. A., Nascimento, M., and Cruz, C. D. (2017). Artificial neural networks and linear discriminant analysis in early selection among sugarcane families. Crop Breed. Appl. Biotechnol. 17, 299–305. doi: 10.1590/1984-70332017v17n4a46
Pham, B. T., Tien Bui, D., Prakash, I., and Dholakia, M. B. (2017). Hybrid integration of multilayer perceptron neural networks and machine learning ensembles for landslide susceptibility assessment at Himalayan area (India) using GIS. Catena 149, 52–63. doi: 10.1016/j.catena.2016.09.007
Pour-Aboughadareh, A., Khalili, M., Poczai, P., and Olivoto, T. (2022). Stability indices to deciphering the genotype-by-environment interaction (GEI) effect: an applicable review for use in plant breeding programs. Plan. Theory 11:414. doi: 10.3390/plants11030414
Qadir, A., Suhartanto, M. R., Widajati, E., Budiman, C., Zamzami, A., Rosyad, A., et al. (2024). Commercial rice seed production and distribution in Indonesia. Heliyon 10:e25110. doi: 10.1016/j.heliyon.2024.e25110
Rahimi, M., and Debnath, S. (2023). Estimating optimum and base selection indices in plant and animal breeding programs by development new and simple SAS and R codes. Sci. Rep. 13, 18977–18978. doi: 10.1038/s41598-023-46368-6
Rawte, S., Saxena, R. R., and Verulkar, S. B. (2021). Analysis of yield stability of rice (Oryza sativa L.) landraces under drought conditions with three different approaches. Ind. J. Gen. Plant Breed. 81, 236–244. doi: 10.31742/IJGPB.81.2.6
Reckling, M., Ahrends, H., Chen, T. W., Eugster, W., Hadasch, S., Knapp, S., et al. (2021). Methods of yield stability analysis in long-term field experiments. A review. Agron. Sustain. Dev. 41:27. doi: 10.1007/s13593-021-00681-4
Ren, H., Bao, J., Gao, Z., Sun, D., Zheng, S., and Bai, J. (2023). How rice adapts to high temperatures. Front. Plant Sci. 14:1137923. doi: 10.3389/fpls.2023.1137923
Rezvi, H. U. A., Tahjib-Ul-Arif, M., Azim, M. A., Tumpa, T. A., Tipu, M. M. H., Najnine, F., et al. (2023). Rice and food security: climate change implications and the future prospects for nutritional security. Food Energy Secur. 12:e430. doi: 10.1002/fes3.430
Rohaeni, W., and Ishaq, M. (2015). Evaluasi varietas padi sawah pada display varietas unggul baru (VUB) di kabupaten karawang, Jawa Barat. Agriculture 27, 1–7. doi: 10.24246/agric.2015.v27.i1.p1-7
Rozaki, Z. (2020a). COVID-19, agriculture, and food security in Indonesia. Rev. Agric. Sci. 8, 243–260. doi: 10.7831/ras.8.0_243
Rozaki, Z. (2020b). “Food security challenges and opportunities in Indonesia post COVID-19” in Advances in Food Security and Sustainability, ed. M. J. Cohen (Cambridge,US: Elsevier), 6, 119–168.
Rumanti, I. A. A., Hairmansis, Y., Nugraha, U., Susanto, P., Wardana, R. E., and Subandiono, (2018). Development of tolerant Rice varieties for stress-prone ecosystems in the coastal deltas of Indonesia. Field Crop Res. 223, 75–82. doi: 10.1016/j.fcr.2018.04.006
Satoto, S., Rumanti, I. A., and Widyastuti, Y. (2016). Yield stability of new hybrid rice across locations. Agriculture 38, 33–39. doi: 10.17503/agrivita.v38i1.675
Senguttuvel, P., Sravanraju, N., Jaldhani, V., Divya, B., Beulah, P., Nagaraju, P., et al. (2021). Evaluation of genotype by environment interaction and adaptability in lowland irrigated rice hybrids for grain yield under high temperature. Sci. Rep. 11, 15825–15813. doi: 10.1038/s41598-021-95264-4
Shantal, M., Othman, Z., and Bakar, A. A. (2023). A novel approach for data feature weighting using correlation coefficients and min–max normalization. Symmetry 15:2185. doi: 10.3390/sym15122185
Sharifi, P., Aminpanah, H., Erfani, R., Mohaddesi, A., and Abbasian, A. (2017). Evaluation of genotype × environment interaction in Rice based on AMMI model in Iran. Rice Sci. 24, 173–180. doi: 10.1016/j.rsci.2017.02.001
Sheng, R. T. C., Huang, Y. H., Chan, P. C., Bhat, S. A., Wu, Y. C., and Huang, N. F. (2022). Rice growth stage classification via RF-based machine learning and image processing. Agriculture 12, 1–23. doi: 10.3390/agriculture12122137
Sitaresmi, T., Hairmansis, A., Widyastuti, Y., Rachmawati,, Susanto, U., Wibowo, B. P., et al. (2023). Advances in the development of rice varieties with better nutritional quality in Indonesia. J. Agric. Food Res. 12:100602. doi: 10.1016/j.jafr.2023.100602
Sitaresmi, T., Willy, S., Gunarsih, C., Nafisah, N., Nugraha, Y., Sasmita, P., et al. (2019). Comprehensive stability analysis of Rice genotypes through multi-location yield trials using Pbstat-Ge. SABRAO J. Breed. Genet. 51, 355–372.
Subekti, A., and Umar, A. (2022). Keragaan Dua Belas Varietas Unggul Baru Padi Pada Agroekosistem Lahan Pasang Surut Di Kalimantan Barat. Agric. Ekstensia 16, 8–13.
Sudana, W. (2016). POLA PENELITIAN VS POLA TANAM PETANI response to IP Padi 400 policy: the improved vs the existing cropping patterns. Anal. Kebijak. Pertan. 8, 103–117. doi: 10.21082/akp.v8n2.2010.103-117
Sulaiman, A. A., Candradijaya, A., and Syakir, M. (2019). Technological advancement and the economic benefit of Indonesian rainfed farming development. Adv. Agric. 2019, 1–8. doi: 10.1155/2019/9689037
Supriatna, A. (2012). Meningkatkan Indeks Pertanaman Padi Sawah Menuju IP Padi 400. Agriculture 16, 1–18. doi: 10.20884/1.agrin.2012.16.1.123
Surdianto, Y., Sunandar, B., Kurnia,, and Sutrisna, N. (2021). Growth and productivity of new superior rice varieties and respondents’ preference in Majalengka regency. E3S Web Conf. 306, 1–11. doi: 10.1051/e3sconf/202130601033
Syafruddin,, Wahyuni, A. N., Risna,, Rahayu, H. S. P., and Saidah, (2023). Introduction of new superior varieties and Jajar Legowo super cultivation technology to increase rice productivity in Central Sulawesi. IOP Conf. Ser. Earth Environ. Sci. 1172:012009. doi: 10.1088/1755-1315/1172/1/012009
Syukur, M., Sujiprihati, S., and Yunianti, R. (2015). Plant Breeding Techniques Revised Edition. Depok-ID: Penebar Swadaya (Indonesia).
Tal, O. (2012). The impact of gene-environment interaction and correlation on the interpretation of heritability. Acta Biotheor. 60, 225–237. doi: 10.1007/s10441-011-9139-8
Utami, D. W., Maruapey, A., Maulana, H., Sinaga, P. H., Basith, S., and Karuniawan, A. (2023). The sustainability index and other stability analyses for evaluating superior Fe-tolerant Rice (Oryza sativa L.). Sustainability 15, 1–22. doi: 10.3390/su151612233
Vogel, E., Donat, M., Alexander, L., Meinshausen, M., Ray, D., Karoly, D., et al. (2019). The effects of climate extremes on global agricultural yields. Environ. Res. Lett. 14:054010. doi: 10.1088/1748-9326/ab154b
Widiastuti, N. N., Lestari, E., and Permatasari, P. (2023). Farmer participation in IP400 Rice cultivation in Kebakkramat District Karanganyar regency. Indones. J. Soc. Responsib. Rev. 2, 128–139. doi: 10.55381/ijsrr.v2i2.188
Yassi, A., Demmallino, E. B., and Sultani, H. R. (2023). Tropical climate change and its impact on horticultural plants in Enrekang District, South Sulawesi, Indonesia. Biodiversitas 24, 3073–3079. doi: 10.13057/biodiv/d240601
Yuan, S., Stuart, A. M., Laborte, A. G., Rattalino Edreira, J. I., Dobermann, A., Kien, L. V. N., et al. (2022). Southeast Asia must narrow down the yield gap to continue to be a major rice bowl. Nat. Food 3, 217–226. doi: 10.1038/s43016-022-00477-z
Yun, Y. (2023). Changes in the growth and yield of an extremely early-maturing Rice variety according to transplanting density. Agriculture 13:717. doi: 10.3390/agriculture13030717
Yun, Y., Kim, G., Cho, G., Lee, Y., Yun, T., and Kim, H. (2023). Effect of nitrogen application methods on yield and grain quality of an extremely early maturing Rice variety. Agriculture 13:832. doi: 10.3390/agriculture13040832
Keywords: adaptability index, Oryza sativa , rice yield, tropical region, stability analysis
Citation: Anshori MF, Musa Y, Farid M, Jayadi M, Bahrun AH, Yassi A, Sulaiman AA, Huang YC, Casimero M, Llorca L, Suwarno WB, Putra MM, Sembiring H, Purwoko BS, Wasonga DO and Seleiman MF (2024) A new concept in assessing adaptability index for superior potential cropping intensity in early-maturing rice. Front. Sustain. Food Syst. 8:1407880. doi: 10.3389/fsufs.2024.1407880
Edited by:
Matteo Balderacchi, Independent researcher, Piacenza, ItalyReviewed by:
Yheni Dwiningsih, University of Arkansas, United StatesYahui Guo, Central China Normal University, China
Copyright © 2024 Anshori, Musa, Farid, Jayadi, Bahrun, Yassi, Sulaiman, Huang, Casimero, Llorca, Suwarno, Putra, Sembiring, Purwoko, Wasonga and Seleiman. This is an open-access article distributed under the terms of the Creative Commons Attribution License (CC BY). The use, distribution or reproduction in other forums is permitted, provided the original author(s) and the copyright owner(s) are credited and that the original publication in this journal is cited, in accordance with accepted academic practice. No use, distribution or reproduction is permitted which does not comply with these terms.
*Correspondence: Muhammad Fuad Anshori, fuad.anshori@unhas.ac.id