- 1Department of Rural Economy, Environment and Society, SRUC, Edinburgh, United Kingdom
- 2International Livestock Research Institute (ILRI), Nairobi, Kenya
The heterogeneity in East African small-holder agricultural systems influences the farm households’ welfare outcomes. We examine the heterogeneity of rural farm households in Eastern Africa and the influence of such diversity on household food security and how these relate to intra-household decision-making dynamics. Using data from the Rural Household Multi-Indicator Survey (RHoMIS) 1,199 households in Ethiopia, Kenya, Malawi and Uganda were typologized using partition-based cluster analysis. These were grouped into relatively homogenous classes reflecting their socio-demographic characteristics, resource endowment and farm orientation based at a country level to reflect the unique institutional conditions within these countries. Additional analysis was then used to characterise the differences in food insecurity and intra-household decision-making of these clusters. Five distinct farm household clusters were identified in Ethiopia and Kenya, four in Malawi and three in Uganda. The main factors differentiating the farm clusters were educational attainment of the household head, levels of resource endowment, market orientation and off-farm income. Market orientated clusters and clusters comprising more educated household heads were generally more food secure across all countries. In Ethiopia, Kenya and Uganda, women had greater decision-making control in clusters comprising least educated household heads. Female decision-making control was negatively associated with the resource endowment of clusters in Kenya, Malawi and Uganda. This emphasises the importance of understanding rural farm household heterogeneity when tailoring interventions to target household food insecurity and gender inequity in decision-making in Eastern Africa.
1 Introduction
Eastern African farming systems are dominated by smallholders, who account for approximately 75% of all agricultural production (Salami et al., 2010). These smallholders traditionally practise mixed farming, cultivating small areas of food crops and are highly heterogenous in socio-economic conditions, ecological environments, livelihood strategies, and production objectives. These factors have been found to have strong conditioning effects on farm households’ welfare and food security (Babulo et al., 2008; Vanlauwe et al., 2014; Gebre and Rahut, 2021). Within the last decade, typology approaches have been used extensively to capture the heterogeneity of farming systems and understand their effect on: the environment and farm household sustainability (Tittonell et al., 2010; Mutoko et al., 2014; Sakane et al., 2014; Musafiri et al., 2020; Ulukan et al., 2022); agricultural adaptation strategies (García De Jalón et al., 2017; Douxchamps et al., 2016; Kansiime et al., 2021); livestock production and management (Houessou et al., 2019); farmer perceptions and motivations (Barnes et al., 2013; Barnes et al., 2022); and livelihood choices and outcomes (Babulo et al., 2008; Dunjana et al., 2018), amongst others. Farm households are commonly characterised according to their structural or functional characteristics (Tittonell et al., 2020). Less is known, however, about the associations between smallholder farmers’ heterogeneity and intra-household decision-making dynamics. However, an understanding of the patterns and outcomes of farmers’ heterogeneity on household food security and intra-household decision-making is central for developing systems, tailoring policies and promoting gender equality which reduces food insecurity in Eastern Africa (Coates et al., 2007; FAO, 2011).
Despite discriminatory laws and socio-cultural norms embedding gender disparities in Eastern African farming systems, gender relations are dynamic (Fischer and Qaim, 2012; Gallina, 2016). Studies are increasingly acknowledging a positive association between household food security and women’s decision-making control over income, resources and production (Okonya et al., 2021; Lufuke et al., 2023). While these studies are increasingly incorporating indicators of household food security into their typologies (Kuivanen et al., 2016; Nabuuma et al., 2021; Ulukan et al., 2022), few have included intra-household gender dynamics and indicators of female decision-making. Only Kamau et al. (2018), construct a farm typology according to structural and functional characteristics, dietary diversity and gender equity of decision-making control. Nevertheless, they only considered Kenya’s Kajiado and Murang’a counties and to date, it appears no typology study has combined a structural and functional approach, with the incorporation of both food security and female empowerment indicators, at the multi-country level in Eastern Africa.
Accordingly, this paper adds to the nascent literature on small-holder typologies and aims to assess the heterogeneity of households across Ethiopia, Kenya, Malawi and Uganda. We employ a rich dataset, the RhoMIS database, which covers a range of countries and agro-ecosystems in the Global South (Hammond et al., 2017). In so doing, our objective is to add to previous analysis of these data by comparing the heterogeneity of these typologies found against intra-household decision making dynamics and associated food security strategies.
2 Materials and methods
Using a data driven approach we derive our typologies at country level, recognising the diversity of institutional structures that define accessibility and gender dynamics across the countries. A range of methodological approaches can be applied to derive typologies, including statistical, participatory and expert-based approaches (Tittonell et al., 2020; Barnes et al., 2022). Participatory methods use local farmers’ knowledge to categorise farm systems, incorporating indigenous experiences and socio-cultural values into the typology that are often difficult to capture statistically. Kuivanen et al. (2016) construct a participatory typology of households in Northern Ghana based on meetings with local stakeholders and informal group sessions with farmers. Expert-based methods use subject matter experts to define or validate categorisations of farms in a locality, improving the comprehensibility of the typology for stakeholders (van de Steeg et al., 2010). Statistical-based typologies often employ multivariate techniques to characterise farming systems, with cluster analysis the most commonly used method among studies in SSA (Kansiime et al., 2018; Houessou et al., 2019; Musafiri et al., 2020). Additionally, a handful of studies use multi-dimensional scaling (Pacini et al., 2014) and Naïve Bayesian inference (Paas and Groot, 2017). Statistical typologies are advantageous in terms of their objectivity and reproducibility, enabling comparison across contexts (Kuivanen et al., 2016). Moreover, statistical typologies are often able to incorporate a greater number of variables than participatory or expert-based typologies, emphasising their multidimensional potential (Berre et al., 2019).
2.1 Data source and survey tool
Data for the analysis were sourced from recent cross-sectional farm household characterisation surveys collected using the Rural Household Multi-Indicator Survey (RHoMIS) tool, available at the Harvard Dataverse RHoMIS data repository (RHoMIS, 2019). The general design and principles of the RHoMIS tool are described in detail by Hammond et al. (2017) and van Wijk et al. (2020). In brief, RHoMIS is a standardised farm household survey approach that provides rapid characterisation of farming systems in a rural development context. RHoMIS collects information on a broad array of variables that are used to quantify a series of widely accepted indicators concerning farm and household characteristics, agricultural productivity, market integration, food security and poverty, among others (van Wijk et al., 2020). RHoMIS was designed in response to the need for improvements in household-level characterisation of agricultural systems, which has traditionally been hampered by poor-quality data arising from a lack of standardisation, poor survey design and biases (Hammond et al., 2017). Accordingly, the RHoMIS tool is flexible in its design to permit modifications that suit local contexts, while remaining adequately standardised to enable rapid implementation, analysis and comparison across multiple sites (Ritzema et al., 2019). As such the RHoMiS provides a randomised sample of small-holder households operating within a range of countries.
The data collection efforts conformed with the principles of the 1964 WMA declaration of Helsinki. Ethical approvals for the survey applications were obtained from the internal ethical review committees of the different institutes contributing datasets [e.g., the Internal Review Ethics Committee (IREC) of the International Livestock Research Institute] or for those partners without an internal ethical committee, from ethical evaluation by the senior management at each organization after careful evaluation of the content and methodology. Survey participants were not particularly vulnerable, had the possibility to skip questions, and explicit oral informed consent was obtained from all survey participants prior to survey enumeration (which is documented as the opening question in the questionnaire and dataset.1) In many cases written consent was also obtained, but this was not universal in cases of low literacy amongst study populations. If consent was denied, survey enumeration was terminated. Permission for obtaining oral rather than written consent from survey respondents was granted by the Internal Review Ethics Committee (IREC) of the International Livestock Research Institute and implementing research organizations. Respondent anonymity has been preserved at all times.
Moreover these data undergo a validation process to minimise measurement errors and ensure quality of the data collected (van Wijk et al., 2020). Data used in this study has been collated from several individual RHoMIS-based research projects that were administered between 2019 and 2020 and led by different organisations, illustrating the advantage of a standardised data collection tool.
2.2 Data cleaning
Data for all countries in the region of interest were cleaned and records with missing values were excluded from the dataset. To minimise bias and misclustering, selected variables were screened for outliers and values exceeding the 99th percentile threshold were trimmed from the dataset. RhoMIS has around 19 countries in Africa and the choice was made to focus on selected countries in East and Southern Agriculture for brevity of reporting. A total of 1,199 households from Ethiopia (270 households), Kenya (430 households), Malawi (177 households) and Uganda (322 households) were retained for analysis.
2.3 Data analysis
All statistical analyses were performed within the RStudio environment (version 2023.6.1.524) (Posit Team, 2023) for R, using the packages cluster (Maechler et al., 2022), Rtsne (Krijthe, 2015), ggplot2 (Wickham, 2016), factoextra (Kassambara and Mundt, 2020) and summarytools (Comtois, 2022). Statistical differences were considered significant at 0.05. Numeric variables were checked for normality through visual inspection, revealing all numeric variables followed a non-normal distribution. Comparisons between sites, in terms of farm household characteristics, food security status and female decision-making, were conducted using the chi-squared test (χ2) for categorical variables and the Kruskal–Wallis H test for numeric variables. When the tests indicated significant relationships, a post hoc test was performed using the standardised residuals method for χ2 and sequential, Bonferroni-corrected, Mann–Whitney U tests for Kruskal–Wallis. Medians were presented with their interquartile ranges.
Prior to clustering, numeric variables were logarithmically transformed to minimise bias in the clustering, due to the non-normal distributions of the data. Additionally, a correlation matrix was constructed to identify potential collinearities and redundant variables. The matrix revealed weak linear relationships, with absolute values of r < 0.36; hence all variables were retained for clustering. The partitioning around medoids (PAM) method, based on the Gower distance, was selected for clustering, due to its greater robustness to outliers than other partition-based algorithms (Jin and Han, 2010) and ability to cluster mixed data types. The Gower distance measures the dissimilarity between two observations with mixed numeric and non-numeric elements, generating a distance matrix. The PAM algorithm was then applied to the Gower distance matrix to map observations into a predetermined number of clusters (k) (Botyarov and Miller, 2022). The silhouette width method was used to determine the optimal number of clusters for each country (Sai Krishna et al., 2018). Silhouette plots are shown in Figure 1. The method computed an average silhouette coefficient of observations for different values of k. The coefficient takes a value between −1 and 1 and is an aggregated measure of how close observations in one cluster are to observations in neighbouring clusters, representing the separation distance between clusters (Kassambara, 2017). Additionally, the average silhouette coefficient was used as an internal cluster validation index, to determine the quality of clustering; where an average silhouette coefficient close to 1 indicates the observations are very well clustered and a negative silhouette coefficient indicates there are observations that are poorly clustered (Kassambara, 2017).
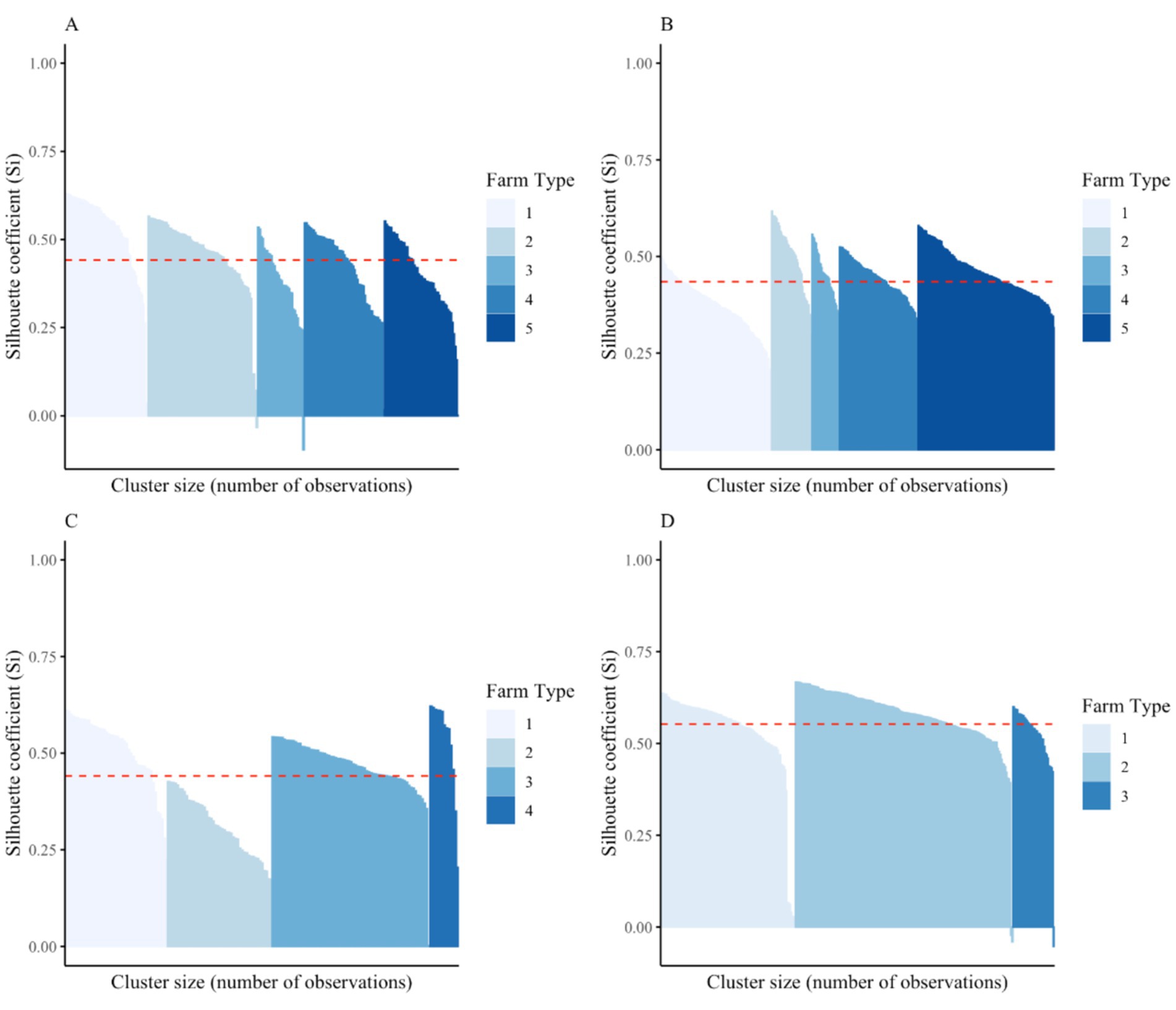
Figure 1. Silhouette plot of silhouette coefficients of farm clusters in (A) Ethiopia, where the average silhouette width is 0.44 for k = 5; (B) Kenya, where the average silhouette width is 0.43 for k = 5; (C) Malawi, where the average silhouette width is 0.44 for k = 4; and (D) Uganda, where the average silhouette width is 0.55 for k = 3. Five households had negative silhouette coefficients: in Ethiopia, the silhouette coefficients of one household in cluster 2 = −0.03, and one household in cluster 3 = −0.10; while in Uganda, the silhouette coefficients of two households in cluster 2 = −0.02 and − 0.04, and one household in cluster 3 = −0.05.
Interpretation of the clusters was conducted by exploring mean values and standard deviations for numeric variables and counts per cluster for categorical variables, with the exception of the FIES scores where means were used. Within countries, cluster averages for resource endowment and farm orientation variables were compared, using the country average as a reference point. Each cluster was labelled according to their key structural and functional characteristics and organised against a resource endowment gradient. Within each country, significance testing between farm clusters was performed using the test for categorical variables and the Kruskal–Wallis H test for numeric variable. A Monte Carlo simulation was applied to the test in instances where the test assumptions were not met. For significant relationships, a post hoc test was performed using the standardised residuals method for and sequential, Bonferroni-corrected, Mann–Whitney U tests for Kruskal–Wallis.
From the cluster analysis, it emerged that rural farming households within the study sites could be grouped into farm clusters that reflected their structural and functional diversity, as well as their heterogeneity in household food security status and gendered decision-making dynamics. For each country, the Silhouette Width was computed for two to 10 clusters and revealed the optimal number of farm clusters were five for Ethiopia, five for Kenya, four for Malawi and three for Uganda. The average silhouette coefficients for the typologies were 0.44 for Ethiopia, 0.43 for Kenya, 0.44 for Malawi, and 0.55 for Uganda, indicating the households are well clustered in each country.
3 Results and discussion
3.1 Description of selected variables and indicators
From the pool of information collected by RHoMIS, nine variables describing household-level sociodemographic characteristics, resource endowment and farm orientation were selected for constructing the farm household typologies. To analyse the relationship between the typologies, household food security status and gendered decision-making dynamics, one household-level food security indicator and one female decision-making indicator were included in the typology construction. The information underpinning these indicators is described in Table 1.
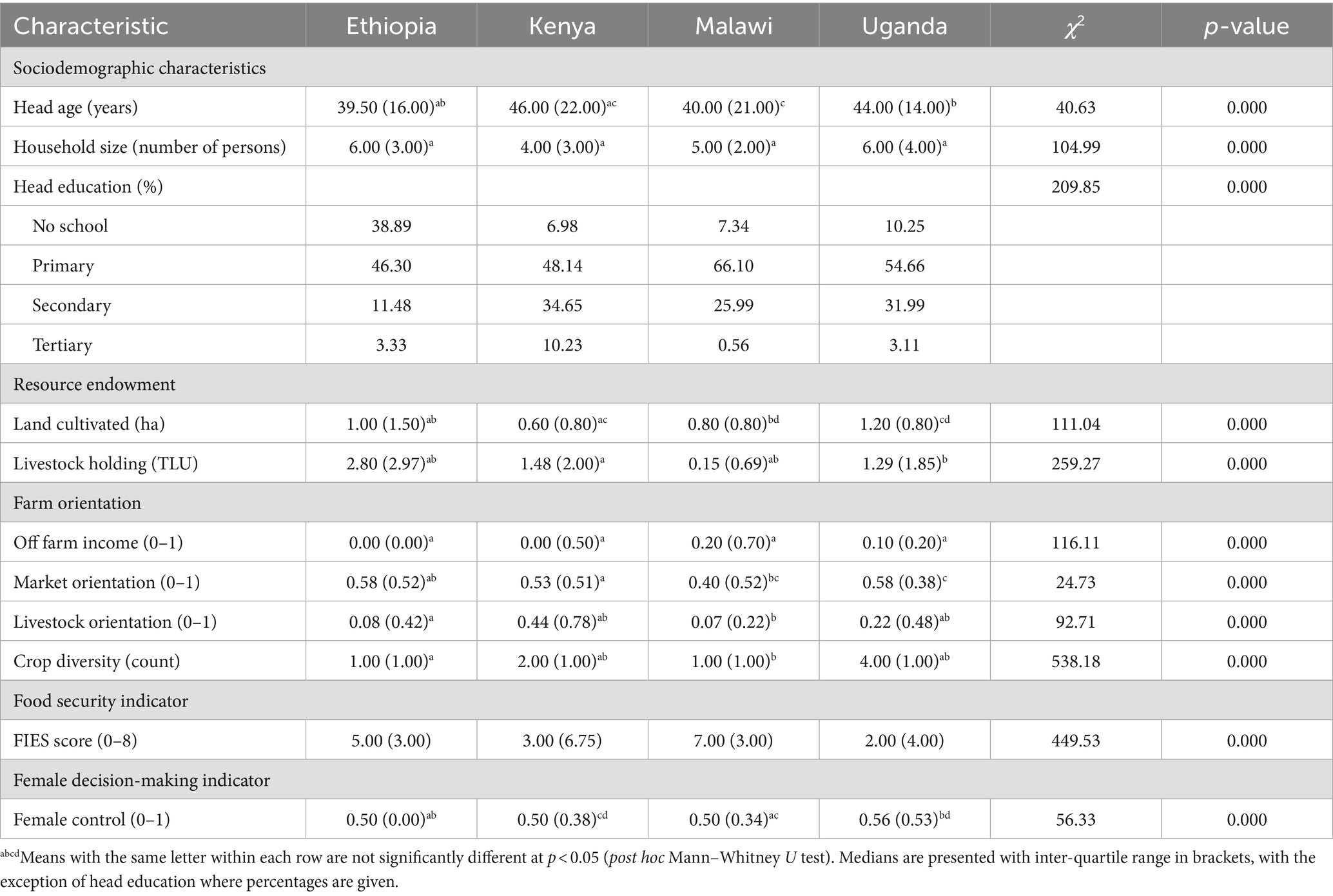
Table 1. Characteristics of rural farming households in Ethiopis (n = 270), Kenya (n = 430), Malawi (n = 177) and Uganda (n = 322).
Across the countries studied, households were reasonably small (median size 4 to 6 members) with heads who were predominantly middle-aged and moderately educated, generally completing either primary or secondary education. Nonetheless, significant differences existed between the countries (household size, age of household head, and education level of household head). Households in Ethiopia and Uganda were significantly larger than those in Kenya and Malawi. Household heads were significantly younger in Ethiopia and Malawi than they were in Kenya. Additionally, in Ethiopia, household heads were generally the least educated, with 38.9% having received no formal education. This is followed by Malawi, where 66.1% of household heads attained primary education. In contrast, household heads in Kenya and Uganda were generally the most educated, with 44.9 and 35.1%, respectively, attaining either secondary or tertiary education. Post hoc analysis confirmed Ethiopian household heads are more likely to receive no education and less likely to receive secondary education, while the reverse was true for household heads in Kenya. In male dominated households, farmer age and education levels has been found to interact with gender dynamics, however effects are nuanced and context dependant (Mutenje et al., 2016; Jost et al., 2016).
Farm sizes were typically small, with households cultivating an average of 0.6 to 1.2 ha; households in Ethiopia and Uganda cultivated significantly larger areas of land than households in Kenya and Malawi. Additionally, livestock numbers varied significantly between countries. Regarding the farm orientation of households, significant differences existed between countries. Households in Ethiopia and Kenya rarely depended on off-farm income, while in Uganda and Malawi off-farm income represented a relatively important source of income, contributing 10 and 20% of total household income, respectively. Households in Ethiopia and Uganda were significantly more market orientated, selling on average 58% of farm produce. Conversely, households in Malawi were least market orientated, selling on average 40% of farm produce. Ugandan and Kenyan households were significantly more livestock orientated, obtaining 22 and 44% of farm produce value from livestock, respectively. In contrast, households in Malawi and Ethiopia obtained only 7 and 8%, respectively, indicating a greater reliance on crop production. Additionally, Ugandan households exhibited significantly greater crop diversity, cultivating on average four crop varieties; while households in Ethiopia and Malawi were least diverse, cultivating one crop variety.
Given the complex and multidimensional nature of food security, the Food Insecurity Experience Scale (FIES) was selected for analysis of the food security status of the farm typologies (Wambogo et al., 2018; Manikas et al., 2023). The FIES is an experience-based indicator that captures the access dimension of food security and is constructed from eight questions related to an individual’s or household’s perceptions and behavioural responses to insecure food access (Manikas et al., 2023). FIES scores were significantly different at country level [ (24, N = 1,199) = 449.53, p < 0.001], resulting in substantial variation between the food security status of households. Households in Uganda and Kenya generally experienced food security, with average FIES scores of two and three, respectively. Households in Malawi generally experienced severe food insecurity, with an average FIES score of seven and significantly more scores of eight, than expected.
Intra-household decision-making dynamics were quantified using a female control indicator, which represents the proportion of total benefits generated from on and off-farm activities that are controlled by a female in the household. For each farm product and income source, households are asked whether a man or woman usually decides how much of a product to eat, when to eat it and who sells it (van Wijk et al., 2016). To construct the female control indicator, this information is aggregated into an overall score ranging from 0 to 1, where 0 implies women in the household have no decision-making control over the food and income benefits generated by on and off farm activities; 1 implies women have complete control; and 0.5 implies joint decision-making between men and women in the household (van Wijk et al., 2016). The indicator therefore reflects women’s decision-making agency over the benefits that result from these resources, rather than the ownership of resources (van Wijk et al., 2016). Female control scores differed significantly between countries [ (3) = 56.33, p < 0.001]. Ugandan households exhibited the greatest female decision-making power, with women on average having 56% of control over decisions related to productive resources and income. On the other hand, households in Ethiopia, Kenya and Malawi typically exhibited joint decision-making, with average female control scores of 0.50.
3.2 Structural and functional characteristics of farm clusters
Detailed analysis of the clusters by country are provided in the Supplementary material. Tables 2–5 provides a descriptive summary of each countries’ farm clusters, based upon their key structural and functional characteristics.
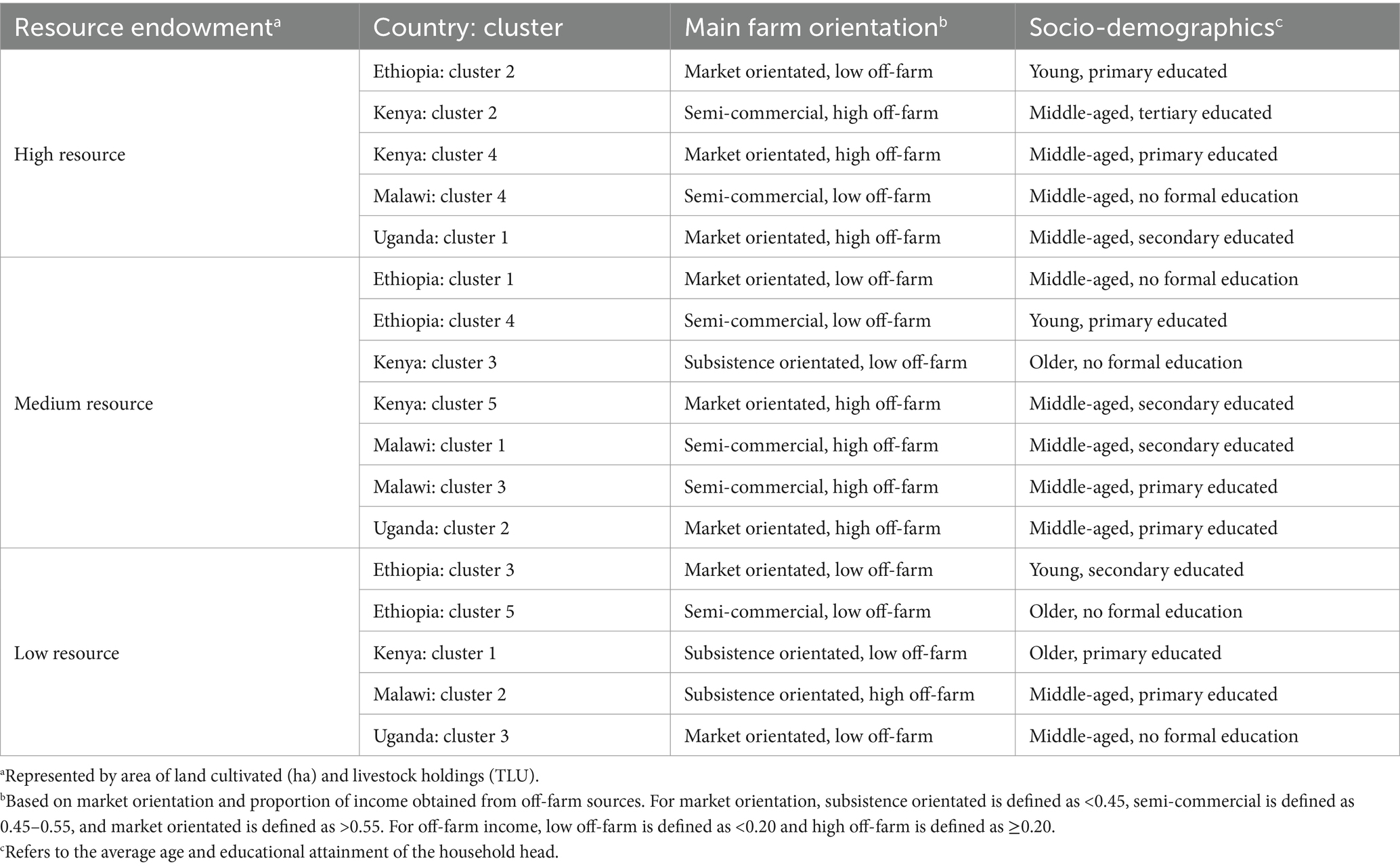
Table 2. Description of the key structural and functional characteristics of the farm clusters identified in Ethiopia, Kenya, Malawi and Uganda, organised by resource endowment.
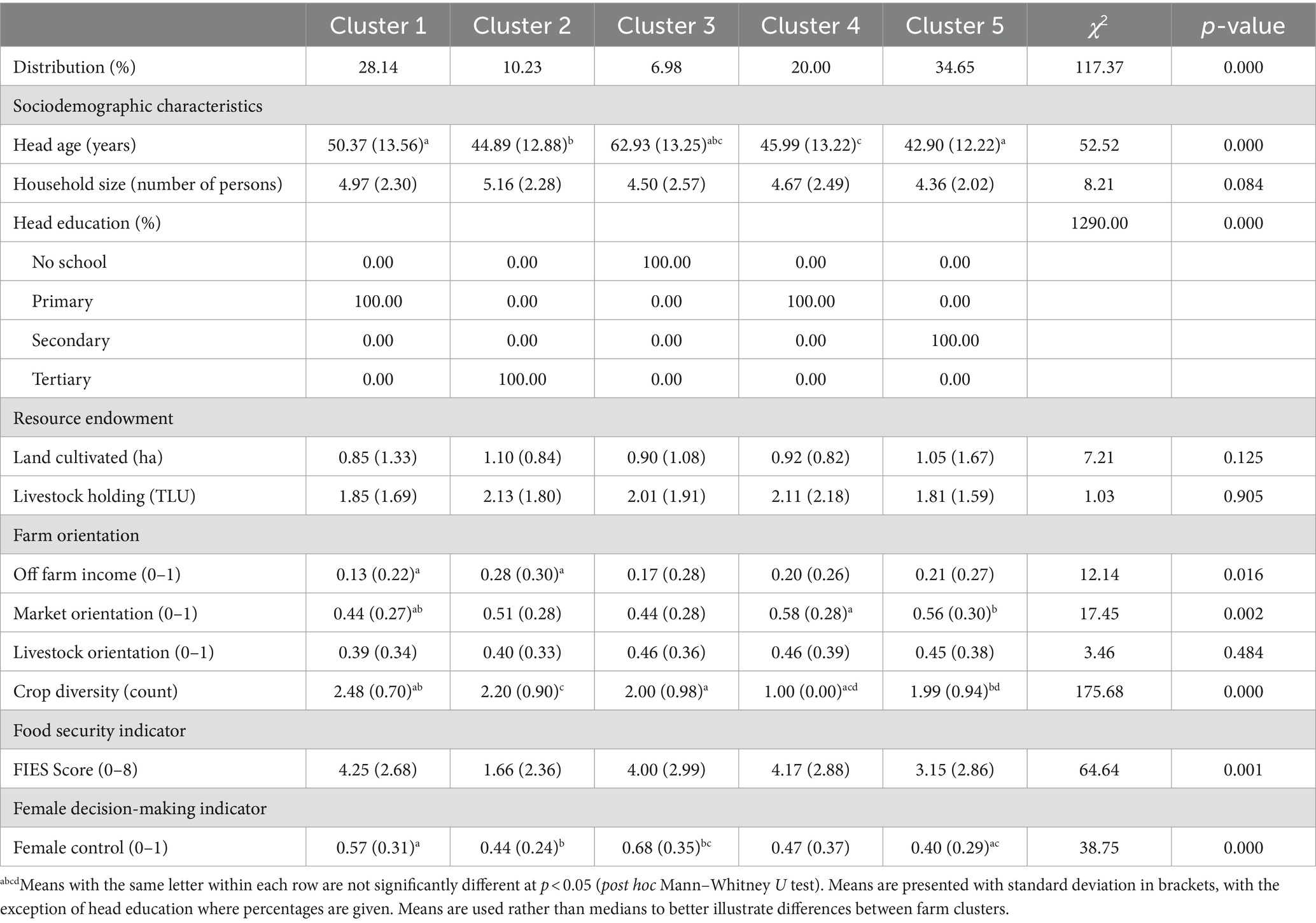
Table 3. Profile of the farm clusters identified in Kenya (n = 430): cluster 1 (n = 121), cluster 2 (n = 44), cluster 3 (n = 30), cluster 4 (n = 86) and cluster 5 (n = 149).
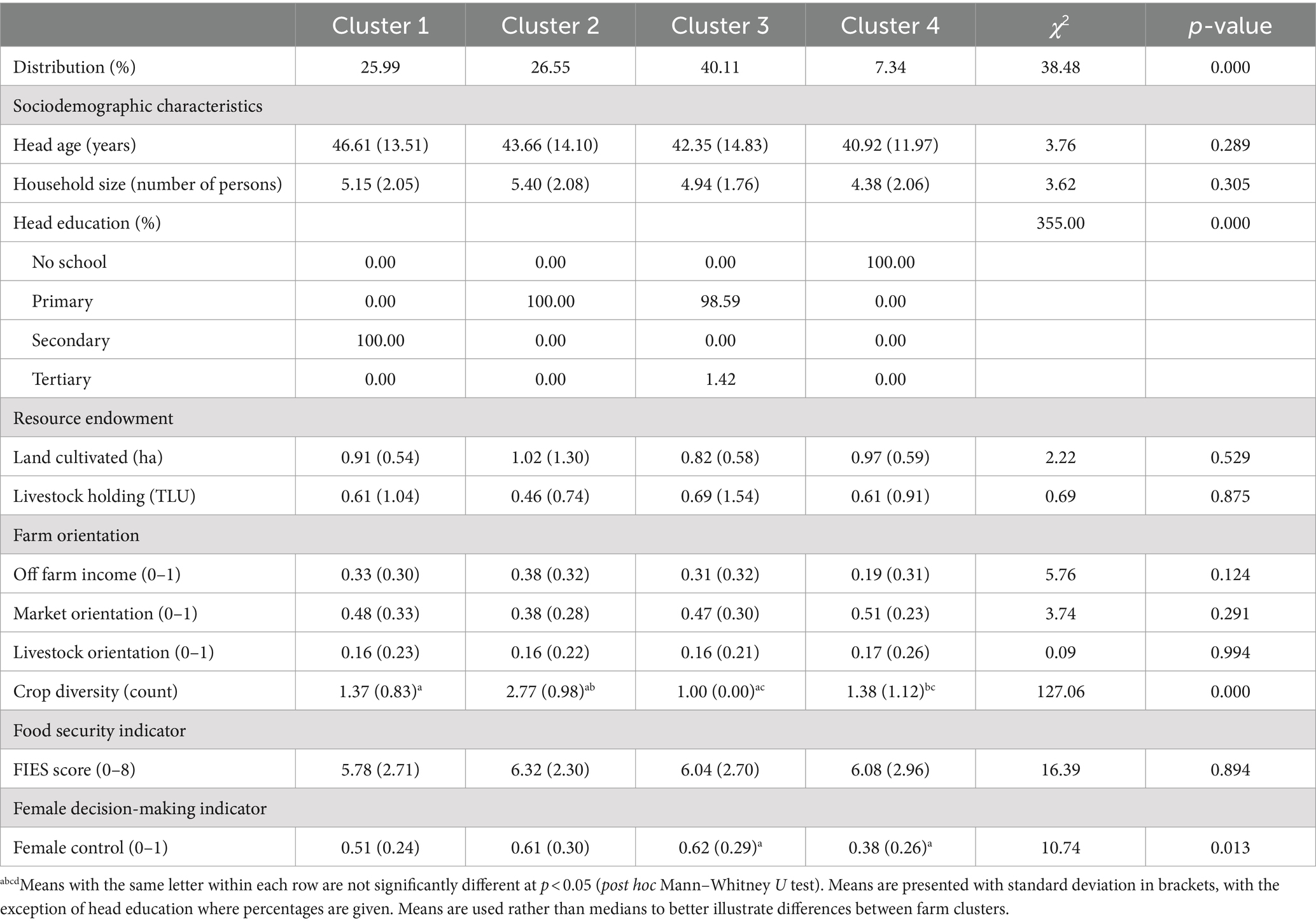
Table 4. Profile of the farm clusters identified in Malawi (n = 177): cluster 1 (n = 46), cluster 2 (n = 47), cluster 3 (n = 71) and cluster 4 (n = 13).
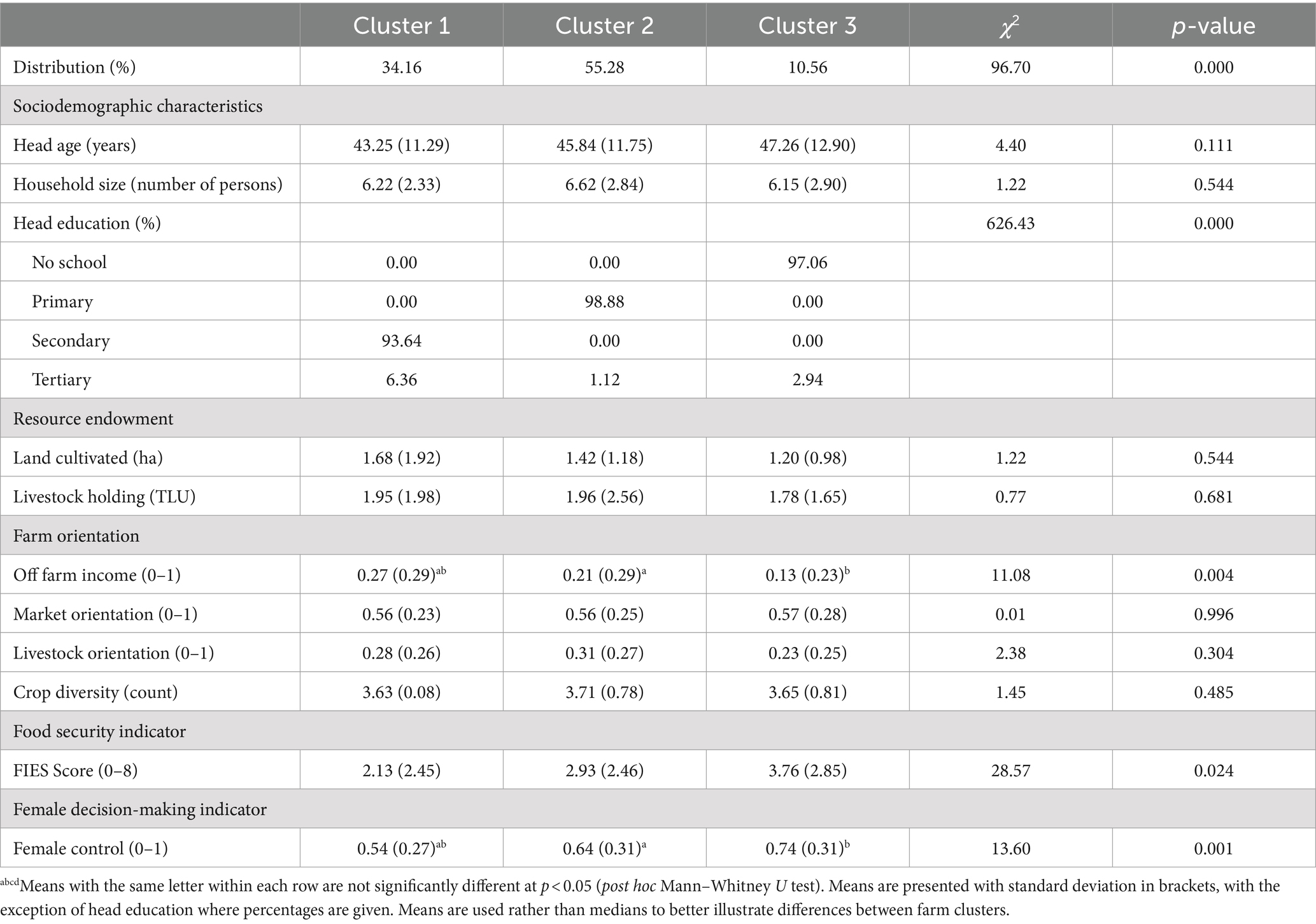
Table 5. Profile of the farm clusters identified in Uganda (n = 322): cluster 1 (n = 110), cluster 2 (n = 178) and cluster 3 (n = 34).
The five farm clusters were relatively homogenous regarding household size, which on average ranged between five and six members. The greatest heterogeneity is observed in the educational attainment of the household head, resource endowment and farm orientation of the different clusters.
The five farm clusters shared similar characteristics in terms of household size and crop diversity. Households on average comprised four to five members and cultivated between one and two crop varieties. The farm clusters were most distinguishable in terms of resource endowment, off-farm income, market orientation, livestock orientation and educational attainment of the household head.
The four farm clusters shared similarities in terms of household size, livestock orientation and crop diversity. Factors providing the greatest distinction between the farm clusters were educational attainment of the household head, resource endowment, off-farm income and market orientation.
The three farm clusters shared similarities regarding household size, crop diversity and market orientation, with the clusters cultivating, on average, four crop varieties and selling approximately 57% of farm produce. The farm clusters were most distinguishable in terms of the educational attainment of the household head, resource endowment, off-farm income, and livestock orientation.
3.3 Household food security status of farm clusters
Pearson’s chi-squared tests revealed statistically significant associations between farm clusters and FIES scores in Kenya [χ2 (NA2, N = 430) = 64.64, p < 0.01] and Uganda [χ2 (NA, N = 322) = 28.57, p < 0.05]. Although the association between farm clusters and FIES scores in Ethiopia and Malawi were not considered statistically significant, visual comparison of mean FIES scores revealed nuances in the food security status of the farm clusters. For Kenya, farm clusters 2 and 5 were considered food secure, with cluster 2 reporting the lowest mean FIES score. For Ethiopia, farm clusters 2 and 3 were conceived as food secure and reported similar mean FIES scores of 3.77 and 3.75, respectively. For Uganda, all farm clusters were conceived as food secure, with mean FIES scores ranging between 2.13 and 3.76 months. Specifically, cluster 1 reported the lowest mean FIES score, while cluster 3 reported the highest mean FIES score.
For Kenya, farm clusters 1, 3, and 4 were considered moderately food insecure, with mean FIES scores ranging between 4 and 4.25. Of these clusters, cluster 3 reported the lowest mean FIES score, while cluster 4 reported the highest. For Ethiopia, farm clusters 1, 4 and 5 were conceived as moderately food insecure, with mean FIES scores ranging between 4.13 and 4.92. Among these clusters, cluster 4 exhibited the lowest mean FIES score, while cluster 5 exhibited the highest. For Malawi, all farm clusters were considered moderately food insecure and exhibited the greatest FIES scores across all countries’ farm clusters. The FIES scores of Malawi’s farm clusters ranged between 5.78 and 6.32, with cluster 1 conceived as least food insecure and cluster 2 conceived as most food insecure. Clusters 3 and 4 displayed similar intermediate levels of moderate food insecurity.
The results in this study suggest that educational attainment of the household head has a strong influence on household food security across the countries, with the more educated generally reporting lower FIES scores. Wubetie et al. (2023) and Mutea et al. (2022) report that education of the household head is positively associated with food security in Eastern African countries. Rono et al. (2023) found that household heads with secondary or tertiary education are associated with a 27% increase in the probability of household food security in Northern Kenya. In this study Malawi and Uganda follow a similar trend, albeit less pronounced. The more modest variations in FIES scores between these countries reflect the education and political systems of the country as a whole. In Malawi, for instance, weak financial discipline has compromised human and financial resources in the education sector, with implications on the quality of education received (Kafumbu, 2020). More educated farmers are shown to have greater access to agricultural information and improved employment opportunities, increasing agricultural productivity, household income and economic access to food security (Bashir and Schilizzi, 2013). Furthermore, education improves nutritional knowledge and provision of higher-quality diets, increasing households’ ability to meet their nutritional requirements (Rono et al., 2023). This emphasises the need for long-term policies targeted at enhancing school funding and rural households’ access to quality education. This is particularly crucial in Ethiopia and Malawi, where more than 75% of farm household heads in the sample had not received education past primary level.
The typologies reveal a general association between commercialisation and household food security. All food secure farm clusters, with the exception of cluster 2 in Kenya, are market orientated. In contrast, the majority of food insecure clusters are subsistence orientated or semi-commercial. This aligns with the observations of Kirimi et al. (2013) and Kilimani et al. (2022) in Kenya and Uganda, respectively, who report commercialisation reduces food and nutrition insecurity by increasing household income. This additional income not only increases households’ economic access to dietary diversity but can enable purchase of agricultural inputs that promote food crop productivity on farm (Kirimi et al., 2013). Hence, interventions facilitating market participation among semi-commercial, and subsistence orientated farm groups are crucial for reducing household food insecurity in Eastern Africa. Such interventions include improving access to all-weather roads and market information systems, which promote commercialisation by reducing time and transport costs (Justus et al., 2015).
The influence of off-farm income on household food security of the farm clusters is less evident. In Kenya and Uganda, off-farm income is higher among the more food secure clusters. These findings corroborate with those of Owusu et al. (2011) and Mutea et al. (2019) in SSA, and more widely those of Duong et al. (2021) in Vietnam, who report off-farm employment enhances household income and is positively associated with household food security. In Ethiopia and Malawi, however, the trends between household food security and off-farm income appear more country-specific. Off-farm income is low across all farm clusters in Ethiopia, constituting 4 to 14% of total household income. This is consistent with Abera et al. (2021), who find off-farm income accounts for 7.5% of total household income of rural farmers in Chewaka district, Ethiopia. It is possible these households in Ethiopia are not engaging in off-farm employment regularly. Alternatively, they may face entry barriers to more remunerative off-farm opportunities (Alobo Loison, 2015). Future typology studies and data collection exercises would profit from incorporating more detail on the type of off-farm activities undertaken by households, to help discern the reasons behind low off-farm income shares among farm clusters. Conversely, off-farm income is considerably higher among Malawi’s farm clusters. Bhatti et al. (2021) report Malawian farmers with a higher share of off-farm income implement less efficient agricultural practices, resulting in lower farm profitability. This may explain the high off-farm income and low food security observed among Malawi’s farm clusters. It is also important to note that Malawi’s farm typology was constructed from data collected in 2019. In early March of that year, the country experienced a severe tropical cyclone and acute flooding (Government of Malawi, 2019). Given that in Malawi off-farm employment represents a crucial source of income for food purchase in times of deficit (Sitienei et al., 2014), it is possible that these farm clusters reflect households adopting off-farm income as a coping strategy under this event. Indeed, off-farm income constitutes more than one-third of total income in low to medium resource endowed clusters and only 19% of total income in high resource endowed clusters, suggesting off-farm employment is pursued as a survival strategy by poorer groups in Malawi. Collecting time series data through repeat RHoMIS surveys of the same households would therefore be beneficial for establishing a robust understanding of off-farm activity over time.
3.4 Intra-household decision-making dynamics of farm clusters
Kruskal–Wallis tests revealed significant differences in intra-household decision-making dynamics, measured as female control, of farm clusters in Ethiopia [χ2 (4) = 9.50, p < 0.05], Kenya [χ2 (4) = 38.75, p < 0.001], Malawi [χ2 (3) = 10.74, p < 0.05] and Uganda [χ2 (2) = 13.60, p < 0.01].
For Uganda, women had high decision-making power in all three farm clusters. Notably, cluster 3 reported the highest female control scores across all countries’ farm clusters, with women having on average 74% of control over decisions related to productive resources and income. While, in clusters 1 and 2 women had on average 54 and 64% of decision-making control, respectively. For Malawi, decision-making power was orientated towards women in three farm clusters. Women had similarly high decision-making authority in clusters 2 and 3, controlling 61 and 62% of decision-making, respectively. Cluster 1 households, however, exhibited less distinct gender differences, with women having 51% of decision-making control. For Kenya, clusters 1 and 3 displayed high female decision-making power, with women having 57 and 68% of control, respectively. Ethiopia’s typology comprised of two farm clusters where decision-making dynamics were orientated towards women in the household. Specifically, in clusters 1 and 5 women had on average 52 and 55%, respectively, of control over decisions related to productive resources and income.
Both Ethiopia’s and Kenya’s typology comprised three farm clusters with similarly low female decision-making authority. For Ethiopia, women in clusters 2, 3 and 4 controlled on average 46, 42 and 45%, respectively, of decision-making (Table 2). For Kenya, women in clusters 2, 4 and 5 controlled on average 44, 47 and 40%, respectively, of decision-making (Table 3). For Malawi, cluster 4 reported low female decision-making authority (Table 4). Notably, cluster 4’s female control score was the lowest across all countries’ farm clusters, with women controlling 38% of decisions related to productive resources and income.
In Ethiopia, Kenya and Uganda, female decision-making power is highest in farm clusters where household heads receive no formal education and is generally lowest in clusters where heads attain secondary or tertiary education. This is in contrast to the findings of an OECD (2015) study in Uganda that suggests commitment to gender equality is lower among people with lower education. However, Sell and Minot (2018) report educational equality between spouses has a greater influence on female decision-making power, than the level of education attained. It is therefore possible, that in this study, the farm clusters with household heads attaining secondary or tertiary education have a larger male educational advantage than clusters comprising less educated heads. Indeed, the literature indicates school absenteeism is significantly higher among women in Eastern Africa, especially during secondary education, due to factors such as gender-based violence, women’s household labour burden and limited access to decision-making (Tamiru et al., 2016). Hence, for a more conclusive understanding of the dynamics between education and female decision-making power, future typology studies should incorporate measures of educational attainment of spouses and distinguish between male- and female-headed households. Additionally, for educational policies to successfully empower women in Eastern Africa, it is crucial gender mainstreaming is at the forefront of these policies and focus is directed towards reducing the gender gap in education.
In Kenya, Malawi and Uganda, female control is typically lowest in high resource endowed farm clusters and tends to increase against a declining gradient of resource endowment. This aligns with the findings of Gebre et al. (2019) that female decision-making households have significantly smaller land and livestock holdings than male decision-making households. It is widely acknowledged that ownership and agency of assets is strongly gendered in Africa, reflecting restrictive social structures and gender norms, such as the patrilineal inheritance system, which limits women’s access to land (Quisumbing et al., 2015; Kinati et al., 2022). Hence, women in rural Eastern Africa should be targeted with interventions that tackle these structural disadvantages and build their capacity to claim ownership over assets. Kinati et al. (2022), for instance, highlight the importance of promoting women’s self-confidence, their social networks and membership to associations, for enhancing their control over resources. Not only will this likely prove valuable in lifting low resource endowed, female decision-making farm groups out of poverty but will also promote gender balance in decision-making among high resource endowed farm groups.
The typologies allude to an association between off-farm livelihood diversification and female decision-making power. In Ethiopia, Kenya and Uganda, female control scores are typically lower in farm clusters that have higher shares of off-farm income. Fischer and Qaim (2012) report a similarly negative relationship between female decision-making control and household engagement in off-farm employment. Moreover, Gebru and Beyene (2012) and Abera et al. (2021) find female-headed households are less likely to engage in off-farm income diversification than their male counterparts, due to cultural-based gender roles forcing women to engage more in domestic activities. Given the poverty-alleviating potential of livelihood diversification (Hammond et al., 2023), investments that generate off-farm employment opportunities should be directed towards households with low off-farm income. However, to minimise the negative effects that off-farm income can have on female decision-making control, it is crucial these investments are coupled with interventions that increase women’s capacity to take advantage of these off-farm opportunities. Such interventions include promoting women’s access to education and financial capital (Gebru and Beyene, 2012).
Conversely, in Malawi this trend is reversed, with high off-farm income associating with prominent female decision-making control in the farm clusters. Based on the 2008 Malawi Welfare Monitoring Survey, it is estimated that 88% of Malawian women engage in informal off-farm employment, compared to 65% of men (Slavchevska et al., 2016). This high level of engagement among women may explain the positive association between off-farm income and female control observed in the Malawi typology. Indeed, studies find that when women themselves engage in off-farm employment, they are more empowered in household decision-making (Maligalig et al., 2019). Additionally, it is possible that Malawi has experienced “feminization of agriculture,” where women’s involvement in agricultural production and decision-making control over productive assets and incomes increase as men participate in rural out-migration (Caulfield et al., 2021; Slavchevska et al., 2021). In the last 50 years, Malawi has experienced large-scale male out-migrations to neighbouring countries (Niboye, 2018). However, future typology studies should investigate the gender of those participating in off-farm employment to determine whether this is influencing gender decision-making dynamics in Malawi’s farm clusters. Nevertheless, this study highlights the influence of a country’s socio-economic and political context on off-farm income and intra-household decision-making dynamics.
4 Conclusion
Employing a data-driven approach identifies distinct clusters of farm households and the non-linear relationships between food security, gender dynamics and resource endowments. The most salient factors distinguishing the farm clusters are educational attainment of the household head, levels of resource endowment, market orientation and livelihood diversification through off-farm income.
Using the RHoMIS data we found female decision-making control is high across Uganda’s food secure farm clusters; corroborating the consensus in the literature that women’s empowerment in decision-making and control over income supports increases in household nutrition and food security. This is attributed to women typically allocating greater resources to food crop production and spending a higher proportion of income on education, health and food for family members, than their male counterparts. Conversely, in Ethiopia, Kenya and Malawi, female decision-making control is generally higher in the food insecure farm clusters. This disparity between the countries’ typologies highlight how context-dependent food security and intra-household decision-making dynamics are and warrants the need for further investigation into institutional factors that may mediate an association between the two variables. Nevertheless, it aligns with the observations of Gebre et al. (2021) in Ethiopia, who report female decision-making households have a higher probability of experiencing food insecurity than their male counterparts. The authors ascribe this to women’s increased vulnerability to climate variability and structural disadvantages, in terms of endowment of labour, productive assets, education, training and market information (Gebre et al., 2021). Moreover, these authors report that if female decision-making households had the same amount of resources and returns from resources as male decision-making households, their probability of food security would be expected to increase by 4.6 and 11.6%, respectively. Hence, policies that challenge the barriers women face in agriculture are critical for improving food security outcomes, particularly in Ethiopia, Kenya and Malawi. Additionally, outreach programmes should be initiated across farm household groups to raise awareness of the importance of female decision-making empowerment in rural development.
Rural farm households in Eastern Africa are highly heterogenous. Understanding this diversity is critical for the design and implementation of agricultural development policies and interventions that are tailored to local contexts. The typologies reveal considerable diversity in the structural and functional characteristics of rural smallholder farmers, as well as their food security status and intra-household decision-making dynamics. Additionally, variations in the way in which farm clusters form between countries reflect local institutional factors and the socio-political contexts of the countries and this should be recognised in future studies going forward. For instance, interventions that challenge the structural barriers women face in agriculture are crucial for improving food security outcomes in Ethiopia, Kenya and Malawi, and among low-resource endowed clusters where women’s decision-making power is prominent. Additionally, it is critical that gender mainstreaming is at the forefront of these tailored approaches, to ensure interventions that improve food security, through for example promoting off-farm income earning opportunities or access to education, do not foster gender disparities in the household and disenfranchise women’s decision-making power.
We find that promoting women’s self-confidence is likely to prove instrumental in lifting female decision-making out of poverty. Hence, “hard” social networks, through the establishment of women-only cooperatives, would need to be complemented by “softer” social networks which could be enabled through the targeting of knowledge exchange, peer-to-peer learning and advisory services. Each country has various levels of institutional engagement, but a clear policy recommendation is to ensure promotion of both types of interaction to formalise the decision-making power of females. Aligned to this, we find education equality between spouses to be positive towards female empowerment. Tackling school absenteeism through either directed incentives or intervention to support females, e.g., through targeting child support programmes, may enable policy makers to raise female decision-makers within the farming community.
Aligning with the widely held view that livelihood diversification is fundamental in alleviating rural poverty and food insecurity in developing countries (Douxchamps et al., 2016; Hammond et al., 2023), policies should focus on promoting off-farm opportunities among low off-farm income groups. The factors motivating households to engage in off-farm employment must be further explored, as they appear to differ, and both low and high off-farm income groups should be targeted with tailored interventions that increase their capacity to engage in high-return off-farm opportunities. Such interventions include education and entrepreneurial skills training, expanding rural infrastructure and developing rural town economies (Alobo Loison, 2015).
We have also offered recommendations to RHoMIS on the variables they choose which could expand this dimension of data collection. To fully understand the diversity in farming systems, a broader range of factors than currently in the RHoMIS need to be included, ideally capturing endowment of the different types of capital available to rural farm households. In addition, the dynamic nature of farming systems points to the need for regular resampling of households to update the typology and ensure it remains valid. Nevertheless, this study may represent a first step in improving understanding of farm heterogeneity and its implications for food security and intra-household decision-making dynamics.
Data availability statement
The original contributions presented in the study are included in the article/Supplementary material, further inquiries can be directed to the corresponding author.
Author contributions
AH: Writing – review & editing, Writing – original draft, Visualization, Methodology, Investigation, Formal analysis, Conceptualization. AB: Writing – review & editing, Writing – original draft, Validation, Supervision, Resources, Methodology, Funding acquisition, Data curation, Conceptualization. JH: Writing – review & editing, Writing – original draft, Validation, Supervision, Software, Data curation.
Funding
The author(s) declare that financial support was received for the research, authorship, and/or publication of this article. These authors wish to thank the BBSRC funded LegumeSELECT: Science-Driven Evaluation of LEgume Choice for Transformed Livelihoods (BB/R020590/1).
Conflict of interest
The authors declare that the research was conducted in the absence of any commercial or financial relationships that could be construed as a potential conflict of interest.
The author(s) declared that they were an editorial board member of Frontiers, at the time of submission. This had no impact on the peer review process and the final decision.
Publisher’s note
All claims expressed in this article are solely those of the authors and do not necessarily represent those of their affiliated organizations, or those of the publisher, the editors and the reviewers. Any product that may be evaluated in this article, or claim that may be made by its manufacturer, is not guaranteed or endorsed by the publisher.
Supplementary material
The Supplementary material for this article can be found online at: https://www.frontiersin.org/articles/10.3389/fsufs.2024.1397833/full#supplementary-material
Footnotes
1. ^An example of a RHoMIS survey template is available here: https://www.rhomis.org/uploads/1/1/9/9/119962631/rhomis_1.6_for_demonstration.pdf.
2. ^Our p-value was computed by Monte Carlo simulation hence degrees of freedom is not applicable.
References
Abera, A., Yirgu, T., and Uncha, A. (2021). Determinants of rural livelihood diversification strategies among Chewaka resettlers’ communities of southwestern Ethiopia. Agric. Food Secur. 10, 1–19. doi: 10.1186/s40066-021-00305-w
Alobo Loison, S. (2015). Rural livelihood diversification in Sub-Saharan Africa: a literature review. J. Dev. Stud. 51, 1125–1138. doi: 10.1080/00220388.2015.1046445
Babulo, B., Muys, B., Nega, F., Tollens, E., Nyssen, J., Deckers, J., et al. (2008). Household livelihood strategies and forest dependence in the highlands of Tigray, Northern Ethiopia. Agric. Syst. 98, 147–155. doi: 10.1016/J.AGSY.2008.06.001
Barnes, A. P., Islam, M. M., and Toma, L. (2013). Heterogeneity in climate change risk perception amongst dairy farmers: a latent class clustering analysis. Appl. Geogr. 41, 105–115. doi: 10.1016/J.APGEOG.2013.03.011
Barnes, A. P., Thompson, B., and Toma, L. (2022). Finding the ecological farmer: a farmer typology to understand ecological practice adoption within Europe. Curr. Res. Environ. Sustain. 4:100125. doi: 10.1016/J.CRSUST.2022.100125
Bashir, M. K., and Schilizzi, S. (2013). Determinants of rural household food security: a comparative analysis of African and Asian studies. J. Sci. Food Agric. 93, 1251–1258. doi: 10.1002/JSFA.6038
Berre, D., Baudron, F., Kassie, M., Craufurd, P., and Lopez-Ridaura, S. (2019). Different ways to cut a cake: comparing expert-based and statistical typologies to target sustainable intensification technologies, a case-study in Southern Ethiopia. Exp. Agric. 55, 191–207. doi: 10.1017/S0014479716000727
Bhatti, M. A., Godfrey, S. S., Ip, R. H. L., Kachiwala, C., Hovdhaugen, H., Banda, L. J., et al. (2021). Diversity of sources of income for smallholder farming communities in Malawi: importance for improved livelihood. Sustainability 13:9599. doi: 10.3390/SU13179599
Botyarov, M., and Miller, E. E. (2022). Partitioning around medoids as a systematic approach to generative design solution space reduction. Results Eng. 15:100544. doi: 10.1016/J.RINENG.2022.100544
Caulfield, M. E., Hammond, J., Fonte, S. J., Florido, M. A., Fuentes, W., Meza, K., et al. (2021). Unpicking the inter-relationships between off-farm livelihood diversification, household characteristics, and farm management in the rural andes. Front. Sustain. Food Syst. 5:724492. doi: 10.3389/fsufs.2021.724492
Coates, J., Swindale, A., and Bilinsky, P. (2007). Household Food Insecurity Access Scale (HFIAS) for measurement of household food access: indicator guide. Washington, DC: Food and Nutrition Technical Assistance Project, Academy for Educational Development.
Comtois, D. (2022) summarytools: tools to quickly and neatly summarize data. Available at: https://cran.r-project.org/web/packages/summarytools/index.html (Accessed March 23, 2023).
Douxchamps, S., van Wijk, M. T., Silvestri, S., Moussa, A. S., Quiros, C., Ndour, N. Y. B., et al. (2016). Linking agricultural adaptation strategies, food security and vulnerability: evidence from West Africa. Reg. Environ. Change 16, 1305–1317. doi: 10.1007/s10113-015-0838-6
Dunjana, N., Zengeni, R., Muchaonyerwa, P., and Wuta, M. (2018). Typological characterisation of farms in a smallholder food-cash crop production system in Zimbabwe—opportunities for livelihood sustainability. J. Agric. Rural. Dev. Trop. Subtrop. 119, 11–22.
Duong, P. B., Thanh, P. T., and Ancev, T. (2021). Impacts of off-farm employment on welfare, food security and poverty: evidence from rural Vietnam. Int. J. Soc. Welf. 30, 84–96. doi: 10.1111/IJSW.12424
FAO (2011). The state of food and agriculture 2010–2011: women in agriculture: closing the gender gap for development. Rome, Italy: Food and Agriculture Organization of the United Nations.
Fischer, E., and Qaim, M. (2012). Gender, agricultural commercialization, and collective action in Kenya. Food Secur. 4, 441–453. doi: 10.1007/s12571-012-0199-7
Gallina, A. (2016). Gender dynamics in dairy production in Kenya: a literature review. Copenhagen, Denmark: CGIAR Research Program on Climate Change, Agriculture and Food Security (CCAFS), 182.
García De Jalón, S., Silvestri, S., and Barnes, A. P. (2017). The potential for adoption of climate smart agricultural practices in Sub-Saharan livestock systems. Reg. Environ. Change 17, 399–410. doi: 10.1007/S10113-016-1026
Gebre, G. G., Isoda, H., Amekawa, Y., Rahut, D. B., Nomura, H., and Watanabe, T. (2021). What explains gender gaps in household food security? Evidence from maize farm households in southern Ethiopia. Soc. Indic. Res. 155, 281–314. doi: 10.1007/s11205-020-02600-8
Gebre, G. G., Isoda, H., Rahut, D. B., Amekawa, Y., and Nomura, H. (2019). Gender differences in the adoption of agricultural technology: the case of improved maize varieties in southern Ethiopia. Women's Stud. Int. Forum 76:102264. doi: 10.1016/J.WSIF.2019.102264
Gebre, G. G., and Rahut, D. B. (2021). Prevalence of household food insecurity in East Africa: linking food access with climate vulnerability. Clim. Risk Manag. 33:100333. doi: 10.1016/J.CRM.2021.100333
Gebru, G. W., and Beyene, F. (2012). Rural household livelihood strategies in drought-prone areas: a case of Gulomekeda District, eastern zone of Tigray National Regional State, Ethiopia. J. Dev. Agric. Econ. 4, 158–168. doi: 10.5897/JDAE12.026
Government of Malawi (2019) Malawi 2019 floods post disaster needs assessment (PDNA). Llongwe, Malawi: Government of Malawi.
Hammond, J., Fraval, S., van Etten, J., Suchini, J. G., Mercado, L., Pagella, T., et al. (2017). The Rural Household Multi-Indicator Survey (RHoMIS) for rapid characterisation of households to inform climate smart agriculture interventions: description and applications in East Africa and Central America. Agric. Syst. 151, 225–233. doi: 10.1016/J.Agsy.2016.05.003
Hammond, J., Pagella, T., Caulfield, M. E., Fraval, S., Teufel, N., Wichern, J., et al. (2023). Poverty dynamics and the determining factors among East African smallholder farmers. Agric. Syst. 206:103611. doi: 10.1016/J.AGSY.2023.103611
Houessou, S. O., Dossa, L. H., Diogo, R. V. C., Houinato, M., Buerkert, A., and Schlecht, E. (2019). Change and continuity in traditional cattle farming systems of West African coast countries: a case study from Benin. Agric. Syst. 168, 112–122. doi: 10.1016/J.AGSY.2018.11.003
Jin, X., and Han, J. (2010). “K-medoids clustering” in Encyclopedia of machine learning. eds. C. Sammut and G. I. Webb (Boston, MA: Springer), 564–565.
Jost, C., Kyazze, F., Naab, J., Neelormi, S., Kinyangi, J., Zougmore, R., et al. (2016). Understanding gender dimensions of agriculture and climate change in smallholder farming communities. Clim. Dev. 8, 133–144. doi: 10.1080/17565529.2015.1050978
Justus, O., Knerr, B., Owuor, G., and Ouma, E. (2015). Agricultural commercialization and household food security: The case of smallholders in Great Lakes Region of Central Africa. Milan, Italy: International Association of Agricultural Economists.
Kafumbu, F. T. (2020). An analytical report on the status of financing of secondary education in Malawi. Int. J. Educ. Dev. 72:102127. doi: 10.1016/J.IJEDUDEV.2019.102127
Kamau, J. W., Stellmacher, T., Biber-Freudenberger, L., and Borgemeister, C. (2018). Organic and conventional agriculture in Kenya: a typology of smallholder farms in Kajiado and Murang’a counties. J. Rural. Stud. 57, 171–185. doi: 10.1016/J.JRURSTUD.2017.12.014
Kansiime, M. K., Girling, R. D., Mugambi, I., Mulema, J., Oduor, G., Chacha, D., et al. (2021). Rural livelihood diversity and its influence on the ecological intensification potential of smallholder farms in Kenya. Food Energy Secur. 10:E254. doi: 10.1002/FES3.254
Kansiime, M. K., Van Asten, P., and Sneyers, K. (2018). Farm diversity and resource use efficiency: targeting agricultural policy interventions in East Africa farming systems. NJAS Wageningen J. Life Sci. 85, 32–41. doi: 10.1016/J.NJAS.2017.12.001
Kassambara, A. (2017). Practical guide to cluster analysis in R: unsupervised machine learning. Scotts Valley, CA: CreateSpace Independent Publishing Platform.
Kassambara, A., and Mundt, F. (2020) Factoextra: extract and visualize the results of multivariate data analyses. R Package Version 1.0.7. Available at: https://CRAN.R-project.org/package=factoextra (Accessed March 25, 2023).
Kilimani, N., Buyinza, F., and Guloba, M. (2022). Crop commercialization and nutrient intake among farming households in Uganda. Food Policy 113:102328. doi: 10.1016/J.FOODPOL.2022.102328
Kinati, W., Baker, D., Temple, E. C., Najjar, D., and Mulema, A. A. (2022). Empowerment resources, decision-making and gender attitudes: which matter most to livestock keepers in the mixed and livestock-based systems in Ethiopia? CABI Agric. Biosci. 3, 1–16. doi: 10.1186/s43170-022-00114-6
Kirimi, L., Gitau, R., and Olunga, M. (2013) Household food security and commercialization among smallholder farmers in Kenya. Fourth International Conference. Hammamet, Tunisia: African Association Of Agricultural Economists (AAAE).
Krijthe, J. H. (2015) Rtsne: T-distributed stochastic neighbor embedding using a Barnes-Hut implementation. Available at: https://cran.r-project.org/web/packages/Rtsne/Rtsne.pdf (Accessed March 25, 2023).
Kuivanen, K. S., Michalscheck, M., Descheemaeker, K., Adjei-Nsiah, S., Mellon-Bedi, S., Groot, J. C. J., et al. (2016). A comparison of statistical and participatory clustering of smallholder farming systems—a case study in Northern Ghana. J. Rural. Stud. 45, 184–198. doi: 10.1016/J.JRURSTUD.2016.03.015
Lufuke, M., Bai, Y., Fan, S., and Tian, X. (2023). Women’s empowerment, food security, and nutrition transition in Africa. Int. J. Environ. Res. Public Health 20:254. doi: 10.3390/IJERPH20010254
Maechler, M., Rousseeuw, P., Struyf, A., Hubert, M., and Hornik, K. (2022) Cluster: cluster analysis basics and extensions. Available at: https://cran.r-project.org/web/packages/cluster/citation.html (Accessed April 11, 2024).
Maligalig, R., Demont, M., Umberger, W. J., and Peralta, A. (2019). Off-farm employment increases Women’s empowerment: evidence from Rice farms in the Philippines. J. Rural. Stud. 71, 62–72. doi: 10.1016/J.JRURSTUD.2019.09.002
Manikas, I., Ali, B. M., and Sundarakani, B. (2023). A systematic literature review of indicators measuring food security. Agric. Food Secur. 12:10. doi: 10.1186/s40066-023-00415-7
Musafiri, C. M., Macharia, J. M., Ng'etich, O. K., Kiboi, M. N., Okeyo, J., Shisanya, C. A., et al. (2020). Farming systems’ typologies analysis to inform agricultural greenhouse gas emissions potential from smallholder rain-fed farms in Kenya. Sci. Afr. 8:e00458. doi: 10.1016/J.SCIAF.2020.E00458
Mutea, E., Bottazzi, P., Jacobi, J., Kiteme, B., Speranza, C. I., and Rist, S. (2019). Livelihoods and food security among rural households in the North-Western Mount Kenya region. Front. Sustain. Food Syst. 3:98. doi: 10.3389/Fsufs.2019.00098
Mutea, E., Hossain, M. S., Ahmed, A., and Ifejika Speranza, C. (2022). Shocks, socio-economic status, and food security across Kenya: policy implications for achieving the zero hunger goal. Environ. Res. Lett. 17:094028. doi: 10.1088/1748-9326/AC8BE8
Mutenje, M., Kankwamba, H., Mangisonib, J., and Kassie, M. (2016). Agricultural innovations and food security in Malawi: gender dynamics, institutions and market implications. Technol. Forecast. Soc. Change 103, 240–248. doi: 10.1016/J.Techfore.2015.10.004
Mutoko, M. C., Hein, L., and Shisanya, C. A. (2014). Farm diversity, resource use efficiency and sustainable land management in the western highlands of Kenya. J. Rural. Stud. 36, 108–120. doi: 10.1016/J.JRURSTUD.2014.07.006
Nabuuma, D., Ekesa, B., Faber, M., and Mbhenyane, X. (2021). Food security and food sources linked to dietary diversity in rural smallholder farming households in Central Uganda. AIMS Agric. Food 6, 644–662. doi: 10.3934/AGRFOOD.2021038
Niboye, E. P. (2018). International labour out-migration in Mzimba District, Malawi: why persistent? Int. J. Res. Geogr. 4, 9–21. doi: 10.20431/2454-8685.0402002
Okonya, J. S., Mudege, N. N., Nyaga, J. N., and Jogo, W. (2021). Determinants of women’s decision-making power in pest and disease management: evidence from Uganda. Front. Sustain. Food Syst. 5:693127. doi: 10.3389/fsufs.2021.693127
Owusu, V., Abdulai, A., and Abdul-Rahman, S. (2011). Non-farm work and food security among farm households in northern Ghana. Food Policy 36, 108–118. doi: 10.1016/J.FOODPOL.2010.09.002
Paas, W., and Groot, J. C. J. (2017). Creating adaptive farm typologies using naive Bayesian classification. Inf. Process. Agric. 4, 220–227. doi: 10.1016/J.Inpa.2017.05.005
Pacini, G. C., Colucci, D., Baudron, F., Righi, E., Corbeels, M., Tittonell, P., et al. (2014). Combining multi-dimensional scaling and cluster analysis to describe the diversity of rural households. Exp. Agric. 50, 376–397. doi: 10.1017/S0014479713000495
Posit Team (2023). Rstudio: integrated development environment for R, Posit Software. Boston, MA: PBC.
Quisumbing, A. R., Rubin, D., Manfre, C., Waithanji, E., van den Bold, M., Olney, D., et al. (2015). Gender, assets, and market-oriented agriculture: learning from high-value crop and livestock projects in Africa and Asia. Agric. Hum. Values 32, 705–725. doi: 10.1007/s10460-015-9587-x
RHoMIS (2019). The Rural Household Multiple Indicator Survey (RHoMIS) data of 13,310 farm households in 21 countries. 3rd Edn: Harvard Dataverse Available at: https://dataverse.harvard.edu/dataset.xhtml?persistentId=doi:10.7910/DVN/9M6EHS.
Ritzema, R. S., Douxchamps, S., Fraval, S., Bolliger, A., Hok, L., Phengsavanh, P., et al. (2019). Household-level drivers of dietary diversity in transitioning agricultural systems: evidence from the greater Mekong subregion. Agric. Syst. 176:102657. doi: 10.1016/J.AGSY.2019.102657
Rono, P. K., Rahman, S. M., Amin, M. D., and Badruddoza, S. (2023). Unraveling the channels of food security of the households in northern Kenya: evidence from an exclusive dataset. Curr. Dev. Nutr. 7:100005. doi: 10.1016/J.CDNUT.2022.100005
Sai Krishna, T. V., Yesu Babu, A., and Kiran Kumar, R. (2018). “Determination of optimal clusters for a non-hierarchical clustering paradigm K-means algorithm” in Proceedings of International Conference on Computational Intelligence and Data Engineering. eds. N. Chaki, N. Cortesi, and A. Devarakonda (Singapore: Springer).
Sakane, N., van Wijk, M. T., Langensiepen, M., and Becker, M. (2014). A quantitative model for understanding and exploring land use decisions by smallholder Agrowetland households in rural areas of East Africa. Agric. Ecosyst. Environ. 197, 159–173. doi: 10.1016/J.AGEE.2014.07.011
Salami, A., Kamara, A. B., and Brixiova, Z. (2010). Smallholder agriculture in East Africa: trends, constraints and opportunities, vol. 105. Tunis, Tunisia: Africain Development Bank.
Sell, M., and Minot, N. (2018). What factors explain women’s empowerment? Decision-making among small-scale farmers in Uganda. Women’s Stud. Int. Forum 71, 46–55. doi: 10.1016/J.Wsif.2018.09.005
Sitienei, I., Mishra, A. K., Gillespie, J., and Khanal, A. R. (2014). “Participation in informal off-farm labor market and its impact on household income and food security in Malawi” in Southern Agricultural Economics Association (SAEA) Annual Meeting (Dallas, Texas: Southern Agricultural Economics Association).
Slavchevska, V., Doss, C. R., Hillesland, M., and Mane, E. (2021). The impacts of rural outmigration on women’s empowerment: evidence from Nepal, Senegal, and Tajikistan. Washington, DC: International Food Policy Research Institute (IFPRI).
Slavchevska, V., Kaaria, S., and Taivalmaa, S.-L. (2016) Feminization of agriculture in the context of rural transformations: What is the evidence?
Tamiru, D., Argaw, A., Gerbaba, M., Ayana, G., Nigussie, A., and Belachew, T. (2016). Household food insecurity and its association with school absenteeism among primary school adolescents in Jimma zone, Ethiopia. BMC Public Health 16:802. doi: 10.1186/s12889-016-3479-x
Tittonell, P., Bruzzone, O., Solano-Hernández, A., López-Ridaura, S., and Easdale, M. H. (2020). Functional farm household typologies through archetypal responses to disturbances. Agric. Syst. 178:102714. doi: 10.1016/J.AGSY.2019.102714
Tittonell, P., Muriuki, A., Shepherd, K. D., Mugendi, D., Kaizzi, K. C., Okeyo, J., et al. (2010). The diversity of rural livelihoods and their influence on soil fertility in agricultural systems of East Africa—a typology of smallholder farms. Agric. Syst. 103, 83–97. doi: 10.1016/J.AGSY.2009.10.001
Ulukan, D., Bergkvist, G., Lana, M., Fasse, A., Mager, G., Öborn, I., et al. (2022). Combining sustainable livelihood and farm sustainability approaches to identify relevant intensification options: implications for households with crop-based and gathering-based livelihoods in Tanzania. Ecol. Indic. 144:109518. doi: 10.1016/J.ECOLIND.2022.109518
van de Steeg, J. A., Verburg, P. H., Baltenweck, I., and Staal, S. J. (2010). Characterization of the spatial distribution of farming systems in the Kenyan highlands. Appl. Geogr. 30, 239–253. doi: 10.1016/J.APGEOG.2009.05.005
van Wijk, M. T., Hammond, J., Frava, S., Ritzema, R. S., Bolliger, A. M., and Long, C. T. M. (2016). “Including gender equity in a survey tool for rural households” in A different kettle of fish? Gender integration in livestock and fish research (Amsterdam: LM Publishers).
van Wijk, M. T., Hammond, J., Gorman, L., Adams, S., Ayantunde, A., Baines, D., et al. (2020). The rural household multiple indicator survey, data from 13,310 farm households in 21 countries. Sci. Data 7, 1–9. doi: 10.1038/S41597-020-0388-8
Vanlauwe, B., Coyne, D., Gockowski, J., Hauser, S., Huising, J., Masso, C., et al. (2014). Sustainable intensification and the African smallholder farmer. Curr. Opin. Environ. Sustain. 8, 15–22. doi: 10.1016/J.COSUST.2014.06.001
Wambogo, E. A., Ghattas, H., Leonard, K. L., and Sahyoun, N. R. (2018). Validity of the food insecurity experience scale for use in sub-Saharan Africa and characteristics of food-insecure individuals. Curr. Dev. Nutr. 2:Nzy062. doi: 10.1093/CDN/NZY062
Keywords: small holder farmers, food security, cluster analysis, household heterogeneity, gender dynamics
Citation: Henderson A, Barnes AP and Hammond J (2024) Characterising diversity of rural farm households in Eastern Africa: implications for food security and intra-household decision-making. Front. Sustain. Food Syst. 8:1397833. doi: 10.3389/fsufs.2024.1397833
Edited by:
Godfrey Hampwaye, Southern African Institute for Policy and Research (SAIPAR), ZambiaCopyright © 2024 Henderson, Barnes and Hammond. This is an open-access article distributed under the terms of the Creative Commons Attribution License (CC BY). The use, distribution or reproduction in other forums is permitted, provided the original author(s) and the copyright owner(s) are credited and that the original publication in this journal is cited, in accordance with accepted academic practice. No use, distribution or reproduction is permitted which does not comply with these terms.
*Correspondence: Andrew P. Barnes, Andrew.Barnes@sruc.ac.uk