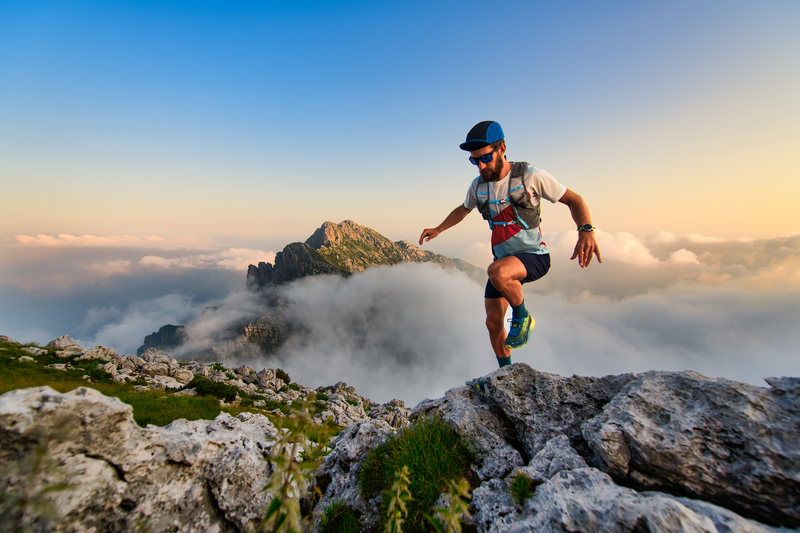
94% of researchers rate our articles as excellent or good
Learn more about the work of our research integrity team to safeguard the quality of each article we publish.
Find out more
ORIGINAL RESEARCH article
Front. Sustain. Food Syst. , 05 June 2024
Sec. Agroecology and Ecosystem Services
Volume 8 - 2024 | https://doi.org/10.3389/fsufs.2024.1392746
Australian agriculture and the rural communities that depend upon it are expected to experience significant impacts from climate change. The recognition of the human role in climate change is central in the design and implementation of effective strategies to mitigate and adapt to its impacts. Understanding the extent to which members of the public, such as private landholders, acknowledge human-caused climate change is critical, given their role as custodians of large tracts of natural resources. Rural social benchmarking studies are a useful tool for understanding landholder values and beliefs. Here, we use a rural social benchmarking survey to examine landholder agreement regarding the extent to which humans contribute to climate change across four Australian agricultural regions. We perform hierarchical clustering analysis to determine subgroups of landholders with similar patterns of survey responses. We then evaluate this effect of cluster membership and demographic characteristics using Bayesian ordinal regression on levels of agreement with the statement “that human activities are influencing climate change.” Our findings reveal three distinct clusters based on patterns of responses to survey questions eliciting participants values beliefs and norms. Cluster membership exhibits the strongest positive influence on agreement (0.52, 95% CI: 0.37 to 0.67). This was followed by higher education levels (0.32, 95% CI: 0.22 to 0.41). Gender showed a moderately uncertain but positive influence. Years residing on the property, participant age, and property size showed very little influence, while rainfall zones showed a negative influence of-0.29 (95% CI: −0.47 to-0.12). Our results underscore the need for extension programs to consider landholder typologies based on a combination of lived experience and demographics.
Terrestrial biodiversity across the globe is experiencing rapid decline due to the increasing, cumulative impacts of land-use change, habitat loss and climate change (IPCC Climate Change, 2013; Allen et al., 2018; Arias et al., 2021). Climate change negatively impacts native vegetation by changing the suitability requirements needed for the persistence of species (Archibald et al., 2024) resulting in changes to the distribution of certain species or species extinction where habitats become entirely unsuitable (Archibald et al., 2024). Climate change is also likely to have negative and profound impacts on agriculture and food systems (McCarl, 2010; Hatfield et al., 2011). Australia is vulnerable to climate change due to the compounding impacts of significant weather variability increasing the frequency and intensity of natural disasters (Garnaut, 2008; King et al., 2017; Beggs et al., 2021; Prosser et al., 2021). As a result, Australian agricultural productivity has been reported to be declining (Hochman et al., 2017). In the future, these declines may be between 2 and 50% (Hughes et al., 2022). The successful design and implementation of effective strategies to mitigate and adapt to climate change impacts is contingent upon the recognition of the human role in climate change.
While scientific evidence overwhelmingly supports the reality of climate change, perceptions of landholders regarding human-driven climate change can be influenced by contextual factors. Previous experiences with natural disasters and variability in climatic patterns may shape these perceptions. Various studies (e.g., Dunlap et al., 2001; Niles and Mueller, 2016; Weber, 2016; Zanocco et al., 2018; Hughes et al., 2022) have explored the connection between concerns about climate change and exposure to variable climatic patterns. These studies suggest that changes in climate change opinion can differ based on the type and severity of weather event experienced. For example, direct exposure to extreme flooding events can increase the salience of climate change (Demski et al., 2017). However, weather pattern variability relative to long term trends may have an uncertain impact on an individual’s opinion about climate change (Weber, 2016). For example, elevated levels of precipitation may not significantly increase climate change concern (Carmichael and Brulle, 2017), but lower-than-average rainfall may have an effect (Hughes et al., 2022). Perceptions of variability may be subjective in relation to key issues arising, or the availability of infrastructure for natural resource control. For example, increased perceived rainfall by farmers in certain areas, despite no evidence to suggest such trends, may be linked to perceived water availability resulting from establishing water retention infrastructure (Niles and Mueller, 2016).
Landholders may also hold unique perspectives that merge ecological, economic and social dimensions (Adams et al., 2014). Consequently, while beliefs, including those regarding climate change, are thought to be guided by knowledge (Ajzen, 1991), several studies mention that knowledge alone does not produce societal or behavioral change, particularly concerning environmental and sustainability behaviors (Pannell, 2010; Rogers et al., 2012). This phenomenon is commonly termed the knowledge-action gap (Frick et al., 2021) where the gap commonly plays out in the contested interfaces of science and policy. This gap is attributed to the causal interactions of socio-cultural knowledge production, use and governance (Cornell et al., 2013; Van Kerkhoff, 2017), and risks not only inaction, but apathy (Stern et al., 2016; Soubry et al., 2020). Understanding the extent to which members of the public, such as private landholders, acknowledge human-caused climate change is critical, given their role as custodians of large tracts of natural resources. This is particularly important because biodiversity values on private land are vulnerable to increases in agricultural intensification (Smith et al., 2013).
Local participation is key to achieving national and international sustainability goals, with local communities working together with government agencies, NGOs, and other stakeholders to reduce land management practices that are environmentally harmful (Minato et al., 2010; Curtis and Mendham, 2015). Understanding local beliefs is particularly important because landholders also consider other landholders to be one of the more trusted sources of information, including for information on climate change (Burgess et al., 2020). Indeed, restorative, sustainable and regenerative management of natural environments and rural landscapes remains a principal area of focus for natural resource management organizations (Lockwood et al., 2009), emphasizing the importance of bottom-up, community-based initiatives in addressing environmental challenges (Brosius et al., 2005).
Engagement with community-based sustainability initiatives is notably varied (Emtage and Herbohn, 2012), influenced by differences in values (Pannell et al., 2006); psychographic variables (such as property management goals); financial barriers; and external factors such as regional governance and the biophysical characteristics of the land (Bizikova et al., 2020). Local participation, and the trust landholders place in information from their peers, both play a pivotal role in shaping land management practices (Barletti et al., 2020). As such, the factors relating to individual’s values, attitudes, beliefs, norms and background factors could have profound implications for acceptance and beliefs surrounding climate change. Understanding this nuanced relationship can help tailor effective strategies toward a collective understanding and commitment toward addressing climate challenges at both local and broader scales (Cardoso and James, 2012).
Rural social benchmarking studies undertaken over many years are building an understanding of landholder characteristics and drivers of decision-making in relation to farm and rural landscape management (Curtis et al., 2008; Lockwood et al., 2009; Minato et al., 2010; Toman et al., 2019; Curtis et al., 2020; Groth-Joynt et al., 2020; Luke et al., 2020, 2022). The combination of landholder characteristics, particularly when derived through social benchmarking surveys, can be used to derive typologies of landholders using multivariate clustering approaches (Curtis et al., 2005; Morrison et al., 2008). Indeed, research using social benchmarking surveys has identified both stability and patterns of change in Australian farming regions, ultimately concluding that certain types of landholders are more likely to invest in more sustainable or regenerative land management practices, such as reducing erosion through the use of cover-cropping (Toman et al., 2019; Alexanderson et al., 2023). Still, there is much to be understood about the relationship between those who agree that human activities are driving climate change, and key social-psychological conditions, including a willingness to invest in regenerative or sustainable land management practices.
While many studies have explored public perceptions of climate change, limited research has specifically focused on private landholder views within the context of human-induced climate change. We examine whether landholders agree that humans cause climate change, using data from rural social benchmarking surveys from four Australian agricultural regions. We specifically examine drivers of agreement in relation to a multitude of factors, including socio-cultural context, property use and land management practices. Based on this premise, we explore the attributes of landholders who agree, disagree or are so far undecided about the relationship between human activity and climate change. Ultimately, our study provides a nuanced perspective of factors influencing agreement in anthropogenic climate change. An improved understanding of the factors influencing perceptions of the Australian farmer may better support future research, funding priorities, extension programs and advocacy.
This paper draws on a large data set derived from the Cooperative Research Centre for High Performing Soils (Soil CRC) Social Benchmarking of Rural Landholders. This study is part of a broader program of research exploring the role of private landholders in regional land use and natural resource management, within the Soil CRC (Curtis et al., 2020; Luke et al., 2020, 2021, 2022). This methodology has a long history in the Australian context, having been applied many times in the context of social benchmarking in rural Australia since 1994 (Curtis and Mendham, 2015).
The original surveys contained core questions, common across surveys, and also customized questions relating to local topics, that were co-designed with regional partners. Local topics were excluded from further analysis, hence we used only the questions that were consistent across all regions. This survey applies the elements of Stern’s causal framework to explain landholder characteristics (Stern, 2000). Within this structure, the core elements of values (biospheric, altruistic, egoistic) (Jorgensen and Stedman, 2001); beliefs (justification for actions, self-assessed capacity to take actions); and personal norms (personal norms such as attitude toward soil health; and subjective norms such as the views of other farmers), follow a modified Theory of Planned Behavior framework that builds on the work of Mazur et al. (2013), Figure 1. According to this framework, human behavior is generally guided by three main considerations: beliefs about consequences of behaviors (behavioral beliefs), beliefs about the expectations of others (normative beliefs) and beliefs about the factors that are barriers or enablers of the behavior (control beliefs) (Ajzen et al., 2018) Within these broader groupings, behavioral beliefs produce attitudes (negative or positive) toward the behavior. Normative beliefs result in perceived social or subjective norms (i.e., the individual’s assessment about whether or not most people will approve or disapprove of the behavior). Finally, control beliefs produce perceived behavioral control (i.e., the relative difficulty or ease in performing the behavior). All of the above variables are influenced by personal traits (such as individual values, attitudes and predisposition toward risk), demographic (including age, gender, income, personal experience, knowledge, or exposure to information (Ajzen et al., 2018).
Figure 1. Schematic representation of the survey structure. Survey questions were classified according to values behavioral beliefs, normative beliefs and control beliefs. These were used to form clusters of landholders. We then explored the relationships between these clusters along with demographic characteristics to form and understanding of the which factors characterize agreement that human activities are driving climate change.
A question on agreement concerning human induced climate change is the principle focus of this analysis. From the broader data set, we drew a subset of 42 questions which are thought to influence attitudes to climate change (Sulemana and James, 2014; Cook and Lewandowsky, 2016), having previously emerged as significantly related. These were: (i) experience with land management issues (lived experience), (ii) personal values (held values) (McIntyre et al., 2008); (iii) reasons for valuing their property (attached values) (Stedman, 2002; Meertens and Lion, 2008); (iv) self-assessed knowledge and understanding of different issues; (v) personal beliefs about farm and resource management; (vi) personal norms and normative views/expectations of others (Meertens and Lion, 2008); and (vii) perceived behavioral control (i.e., having sufficient time and money to implement changes).
The general survey approach is a physical and systematic mail-out of paper notices and booklets to private landholders of rural properties across a chosen geographical region. The survey method is founded on probability theory, which states that inferences can be made about a carefully defined population through the systematic selection of a sample that includes a few hundred or thousand individuals (Dillman, 1991). Following this method, it is possible to make inferences about the prevalence of opinions, views and behaviors of the larger population with assurance (Dillman et al., 2014).
Social benchmarking surveys were implemented within four agricultural regions in the Australian states of South Australia (SA), New South Wales (NSW), Western Australia (WA) and Tasmania (Tas). The agricultural regions included in this study were identified based on the participation of one or more local organizations within the Soil CRC, and defined based on logical boundaries (e.g., a cluster of local government areas within a region, or river catchment management areas) (Luke et al., 2021). To identify addresses for mail-box distribution, rate-payer data was sourced from local councils who partnered on the project in both WA and NSW. For SA and Tasmania, existing regional rate payer lists were used to draw a sample, excluding properties less than ten hectares. On the Eyre Peninsula of SA and in the Northern WA Wheatbelt, a census of all properties was conducted, while in Central West New South Wales and Tasmania, a random sample was drawn, receiving response rates between 24 and 35 percent (Table 1, Figure 2).
Figure 2. Boundaries of the four study regions included in this analysis. Predominant land-uses are shown as an indicative measure of enterprise variation within and between regions. Land-use data were obtained from ABARES (2022).
Our goal was to determine clusters of landholders based on patterns of responses to questions about their behavioral beliefs, normative beliefs and control beliefs; to align these clusters with their demographics and; understand the influence of cluster membership demographic characteristics on levels of agreement that humans are driving climate change. Our analysis was performed over three stages: Stage 1: filling missing values, Stage 2: hierarchical clustering, and Stage 3: implementing a Bayesian ordinal regression for participant demographics and cluster membership, against their agreement that humans are driving climate change. All analysis were performed in R v. 4.3.1 (R Core Development Team, 2013).
Survey responses included in this analysis were recorded on a 5-point Likert agreement scale ranging from strongly disagree to strongly agree (Joshi et al., 2015). Responses were re-coded to a numeric interval scale (1:5) for all analyses. To accommodate for missing data (which is expected in surveys, as respondents may skip or refuse to answer questions), we used multiple imputations (Little and Rubin, 2019). Multiple imputations account for the uncertainty caused by missing data by creating plausible values for the missing responses based on their relationship with other variables in the dataset. The imputed values were extracted using the mice package (Van Buuren, 2011) to create a complete dataset. After trailing several thresholds for acceptable missingness, we considered responses with an average missing value below 30% as acceptable for imputation (Supplementary Table S1). We considered variables that had a correlation coefficient > 0.7 highly correlated and removed these variables from further analysis. After subsetting the entire survey dataset for survey questions from the categories mentioned in Section 2.1, and implementing this cleaning procedure, the number of survey responses in the imputed dataset was 1,393.
We then performed hierarchical clustering analysis with Ward’s distance (Murtagh and Contreras, 2012; Rodriguez et al., 2019) to determine subgroups of individuals with similar patterns of responses to these survey questions (i.e., of views, lived experience (issues), values and future plans). Cluster analysis is appropriate in this context as responses to these questions are expected to determine typologies landholders, beyond demographics alone. The optimal number of clusters was determined based on visual inspection of a dendrogram at a height (or measure of cluster dissimilarity) corresponding to 70% of the maximum height (Supplementary Figure S1) and having a low but acceptable Cophenetic correlation coefficient (0.37). We further evaluated the overlap between clusters using ANOVA (Supplementary information Table S9).
After performing hierarchical clustering, each participant was assigned membership to the cluster with the nearest cluster centroid in the optimal solution. Subsequently, we explored cluster membership based on demographic characteristics of the participants. The characteristics we used were age, sex, education, income, rainfall zone, property size, length of ownership, landholder type, whether the respondent made a profit from their land; and whether the property was used for cropping or grazing purposes.
Finally, we implemented a Bayesian ordinal regression model using cluster membership and demographic characteristics (Table 2) as predictors for agreement that human activities are driving climate change. Our model used weakly informative priors and was implemented in the brms package (Bürkner, 2017). We iterated our model by adjusting chain and iteration parameters after evaluating each iteration model using trace plots, Gelmen-Rubin diagnostics, Geweke diagnostic and MCMC density plots (Supplementary information Figures S3, S24).
While our study allows for a detailed exploration factors shaping landholders’ perceptions, the survey distribution method was conducted within regions of a limited size. The method relies on physical mail-outs of paper booklets and notices linking to an online survey. Some sample selection bias is possible, as those individuals who are both motivated and willing to complete and return the mail-out survey may possibly lean toward older farmers, while online completions tended to be younger and female, as has been identified in previous studies (Patrick et al., 2021). We acknowledge that the while the survey may not capture the perspectives of all landholders, we are fairly confident in the generalizability of our findings across the regions surveyed.
We observed a low Cophenetic correlation coefficient (0.37). We consider that surveys are exploratory research tools, where the primary goal is to uncover insights and trends rather than to produce definitive clustering solutions. Considering this exploratory aim, a Cophenetic correlation coefficient of 0.37 may still provide valuable insights into the underlying structure of the data, helping to identify meaningful clusters or subgroups for further investigation. Furthermore, results of ANOVA analysis reveal significant differences between clusters. Thus, despite its relatively low value, the coefficient still offers valuable insights into underlying data structures, guiding meaningful interpretations and further investigations.
Of the 1,393 imputed responses, most participants resided in New South Wales (n = 495) and South Australia (n = 373), with 354 in Tasmania and 171 in Western Australia. Overall, fewer full-time farmers in all regions tended to agree with the statement that ‘Human activities are influencing changes in climate’ when compared with other types of landholders. Hobby farmers and non-farmers showed higher levels of agreement, with hobby farmers having the highest level of agreement. Respondents who made income from and resided on their property were more likely to agree with this statement, compared with those who did not. Gender made a large difference regarding climate beliefs, though most respondents were male, with a smaller sample of females (n = 111). Over half of male respondents (56.4%) and most female respondents (78.6%) agreed or strongly agreed that humans were driving climate change. Respondents with a University qualification comprised 4.3% of the total participants, with the largest number of participants indicating that they had a Year 12 education (33.4%). Of those who strongly agreed that humans were causing climate change, most individuals held a Year 12 education (54.2%) whereas the majority of those who disagreed had Year 10 as their highest level of education (34.7%) (see Table 2).
Table 2. Variables included in a Bayesian Ordinal Regression model determining the level of agreement that humans are driving climate change.
Respondents from Tasmania showed higher levels of agreement compared to other jurisdictions (74.8% of respondents agreeing or strongly agreeing). Of the respondents who disagreed or strongly disagreed (n = 231), a quarter of these were from New South Wales (n = 61, 26.6%). There were no discernible overall trends in participant’s agreement by rainfall zone, with low rainfall zone respondents being approximately half of those who both strongly agreed and strongly disagreed. Respondents who were not producing crops on their property comprised 66.2% of respondent’s strongly agreeing that humans are driving climate change. The same was true for graziers, with 70.8% of those strongly agreeing that humans are driving climate change managing a grazing enterprise (i.e., sheep or cattle farming) (Table 3). We provide the mean, standard deviation (SD), median, and range of responses for each survey question across the four regions, as well as the overall range of responses for survey questions across all regions (Supplementary Tables S2, S8).
Table 3. General survey trends for participant responses to the question: humans activities are driving climate change.
Three discernible clusters emerged, reflective of distinct patterns responses to subjective norms, lived experiences, perceived behavioral control and behavioral beliefs (Supplementary Table S10). These clusters, developed from responses to survey questions, reveal slightly different demographic assemblages to the overall survey data. Cluster 1 is predominantly composed of full-time farmers. Within this cluster, half of the participants indicated that they “agree” (50.9%) with the notion that climate change is human induced. Unlike the general survey patterns, Cluster 2, characterized by a higher representation of hobby farmers, expressed the highest proportion of disagreeing views (22%), when compared with the overall value of 17%. Still, Cluster 1 (Agree) and Cluster 2 (Disagree) have similar total percentages for the combined responses to “Strongly Agree” and “Agree” (73.0 and 66.8%, respectively), indicating that participants in Cluster 2 express varied levels of agreement. Cluster 2 also showed lower percentages of full-time farmers compared to the overall total (22.3% compared to 52.1%). Participants in this category equally reported making an income from their property and had diverse education levels, but only 2.8% of respondents in this cluster held University qualifications. Cluster 3, the most expansive group, adopts a neutral stance, comprising 60.3% of the overall sample and featuring a mix of occupations. In this cluster, there was a lower percentage of full-time farmers compared to how many full-time farmers completed the survey overall (22.3% vs. 52.1%). Cluster 2, the slightly more disagreeing group, had a higher percentage of respondents who did not receive an income from their property and had the highest percentage of smaller properties (between 0–50 ha, 42.5%). Notably, it stands out with a higher presence of individuals from regions experiencing lower rainfall. Meanwhile, Clusters 1 and 3 show differences in terms of occupation and income, with Cluster 1 having more full-time farmers (50.4%), residing in Tasmania (39.8%) and slightly higher percentages living on their property compared with overall survey trends (88.1% vs. 83.6%) (Figures 3, 4).
Figure 3. Respondent agreement that humans are driving climate change. Panels (A–F) represent participants’ demographics. (A) Does the participant reside on the property?; (B) Participant’s gender; (C) If the participant made an income from their farm in the survey year (2019); (D) Highest education obtained. Tafe stands for Technical and Further Education; (E) What is the participant’s job description; (F) The participant’s self-nominated rainfall zone. Each bar on corresponds to the total number of participants within the demographic category, color-coded based on their response and separated by their cluster allocation.
Figure 4. Respondent agreement that humans are driving climate change. Panels (A–D) represent participants’ demographics. (A): Location; (B) Age (years) as categorical bins; (C) Years property has been owned or owned by family members; (D) Property size (ha) in categorical bins. Each dot on corresponds to the total number of participants within the demographic category, color-coded based on their response and separated by their cluster allocation.
The Gelman-Rubin statistic (Rhat) evaluated the convergence of the Markov Chain Monte Carlo (MCMC) chains. For all model parameters, Rhat values were observed to be close to 1, indicating excellent convergence. Additionally, the Effective Sample Size (ESS) was calculated to assess the efficiency of our MCMC sampling. The ESS values for the bulk and tail of the distribution were consistently high, exceeding 2,000 for each parameter, signifying sufficient effective samples for drawing reliable inferences.
To identify potential issues with the MCMC sampling, we examined the trace plots and identified no irregular patterns or trends, affirming the stability of the chains. We performed posterior predictive checks, including graphical assessment and p-values, to evaluate the model’s ability to replicate the observed data. The results of these checks indicated a good fit, with p-values ranging from 3.75 to 10.8, confirming agreement between the model predictions and the observed data. Additionally, the R-hat values of 1.00 indicate that the model has converged well, and the high effective sample sizes (ESS) suggest that the estimates are reliable. These findings support the robustness of the regression analysis.
Cluster membership indicated the strongest positive influence 0.52 (95% CI: 0.37 to 0.67) on the agreement that humans are causing climate change, indicating that this was a salient influence linking with other demographic and geographic categories. This was followed by education of 0.32 (95% CI: 0.22 to 0.41). Gender was a moderately uncertain predictor but indicated a positive estimate of 0.36 (95% CI: 0.09 to 0.64). Years residing on the property, participant age and size of the property had estimates closer to zero. Rainfall zones also had negative estimate of-0.29 (95% CI: −0.47 to-0.12).
This means that (when all other predictors are held constant), the odds of a higher agreement was 1.68 times higher for individuals in the “Agree” cluster compared to the “Neutral” cluster. For education, the odds of higher agreement are 1.38 times for each one-order increase in education level. For gender, this means that the odds of higher agreement for respondents who identified as women was 1.43 times higher than men. Finally, the odds of agreement decrease by 0.75 times for each decrease in rainfall zone (i.e., high to moderate) (Figure 5).
Figure 5. Influence of demographic characteristics and cluster membership on agreement that humans are causing climate change. Parameter estimates are Bayesian posterior median values and 95% CI for the 8 covariates (y-axis) The multinomial model provided estimates for respondent’s level of agreement that humans are causing climate change. Positive response estimates are indicated in blue; negative is indicated in yellow.
The combustion of fossil fuels for energy production, industrial processes, and transportation releases significant carbon-dioxide and other gasses into the atmosphere, amplifying natural greenhouse effects. Additionally, habitat loss and certain agricultural practices contribute to these emissions, further exacerbating climate change, notably extreme weather patterns and increasing seasonal variability (IPCC Climate Change, 2013; Allen et al., 2018). Changes in climatic conditions over the last few decades have significantly impacted many Australian farming enterprises (Whish et al., 2014; Smith et al., 2018). In the drought of 2019, Australia recorded its driest year on record (ABARES, 2022); followed by the widespread “Black Summer” bushfires of the 2019–20 Australian bushfire season (Burgess et al., 2020); and then by nationwide flooding in 2022. Increasingly frequent weather extremes requires better preparation for future extreme events (McDonald, 2021). This includes how to adapt to changes in seasonal conditions that are likely impacts on farmer livelihoods. Indeed, the seasonal economic impacts are already showing a negative trend for farm profits. For example, Hughes et al. (2022) indicate that average farm profits in Australia from 2001 to 2020 have dropped an estimated 23% per farm, with impacts set to increase into the future.
Enterprises are faced with a need to adapt to climate challenges such as changes in seasonal patterns of water availability and prepare for increasing weather events that decrease or destroy crop yields. Yet there are long established principles within the literature suggesting that barriers for rural landholder adoption of pro-environmental behavior are because landholders are not sufficiently convinced that such management practices will advance their goals in a way that outweighs the costs (Pannell et al., 2006). Private land enterprises play a pivotal role in mitigating and adapting to climate change. However, the success of mitigation initiatives depends on fostering a shared understanding of the drivers of climate change mitigation activities, necessitating further research and concerted efforts toward building awareness and consensus within the agricultural community (Hogan et al., 2011). Nevertheless, despite the increase in extreme weather events (Coumou and Rahmstorf, 2012; Clarke et al., 2022), our results indicate that many private landholders remain surprisingly neutral in their belief that humans are driving climate change, despite being at the forefront of extreme weather impacts.
We found that cluster membership strongly influenced perspectives on climate change by private landholders. Cluster membership was determined based on patterns of responses to individual’s beliefs, experiences and normative views. Thus, while it may be considered appropriate to target communication activities, including those relating to climate change impacts, based on demographic characteristics, our results highlight the need to consider psycho-social factors as well. This finding is corroborated by other studies showing that concern for environmental issues are sometimes, but not always, shaped by proximity to negative environmental impacts (McDonald et al., 2015).
Private land enterprises are at the front line in terms of both mitigation potential and vulnerability to impacts from climate change (Arbuckle et al., 2015; Soubry et al., 2020). Indeed, recent evidence suggests that a shift in beef production areas, changing feed composition and strategic land regeneration could enable emissions reductions of up to 85% globally (Castonguay et al., 2023). Still, these efforts rely on a shared understanding about the drivers of climate change, with the success of these initiatives underpinned by a shared understanding of the drivers of climate change. This necessitates further research and concerted efforts toward building awareness and consensus within the agricultural community (Hogan et al., 2011). For example, recent advancements in the study of agricultural discourse have pointed to the extractivist underpinnings of traditional agriculture (Gordon et al., 2023). Some authors have criticized approaches that unsustainably draw on human and natural resources (Anderson and Rivera-Ferre, 2021), particularly where practices cause ecological damage and the displacement of First Nations peoples (Leventon et al., 2021). Addressing the extractivist underpinnings of industrial agriculture and critiquing unsustainable practices is vital for shifting agricultural systems to more sustainable practices, including those practices that reduce emissions.
For example, decreasing nutrient availability, coupled with increased soil compaction and erosion have been predicted to be sources of economic risk to farmers (Howden et al., 2007; McCarl, 2010; Arbuckle et al., 2015). Likewise, the results of our clustering analyses reveal that issues of soil compaction, declining nutrients and low biological activity were significantly different between clusters. However, issues of soil erosion were not significantly different. This is not to say private landholders did not experience soil erosion issues, rather, that the majority of landholders across all clusters were either neutral (the disagree cluster, mean response = 2.74) or agreed (the agree and neutral clusters, respective means = 4.02 and 3.74) that soil erosion was a problem on their property (See Supplementary Table S9). This highlights soil erosion as a common issue among private landholders meaning that extension or communication activities that target soil erosion will likely draw the participation of individuals with varying climate beliefs.
Nevertheless, engaging with private landholders in relation to practice shifts will require an understanding of people’s values, normative views and beliefs. While climate change impacts increase the volatility of seasonal conditions, decisions for farm management reflect conditions experienced over several years (Park et al., 2012). For example, Robertson and Murray-Prior (2016) argue that, among other factors, the time-frames for farmer decision making are incompatible with the time horizons needed to consider climate change impacts. Our cluster analyses reveals that more individuals in the ‘Agree’ cluster indicated they had experienced the negative impacts of changing weather patterns (Supplementary Table S9). Furthermore, results of the cluster analysis suggests significant differences in lived experience regarding sufficient water for their livestock, with the agree cluster indicating having higher responses of individuals from whom water security was an issue (See Section 2.4 of Supplementary information). Consequently, the relationship between water availability as it impacts the temporal scale of farm decision-making is likely to influence climate related beliefs.
While we identify some factors that influence climate disbelief, there is an emerging and urgent need to understand the drivers of neutrality and then to design proactive measures addressing challenges faced by farming enterprises.
The documented negative trends in farm profits and the substantial economic impacts of recent extreme events highlight the vulnerability of the agricultural sector. Overcoming barriers to the adoption of pro-environmental practices among landholders is crucial for the broadscale implementation of effective climate adaptation strategies. Landholders have a key role to play in mitigating climate change through their land and farm management practices and approaches.
Engaging with private landholders requires an understanding of the factors influencing people’s behavioral beliefs, normative beliefs and control beliefs, which we have examined here in relation to climate beliefs. While demographic trends in climate-related beliefs may suggest broad associations, our results suggest that relying solely on demographics may oversimplify the complexity of individual perspectives within specific communities, such as the farming sector. Despite overarching trends indicating that individuals with higher education levels or women may be more likely to support climate-related beliefs, the nuanced reality among farmers challenges these generalizations. For example, the majority of farmers surveyed have educational backgrounds ranging from year 10 to year 12, and responses predominantly come from male participants. Members from the ‘agree’ cluster tended to have completed year 12 as the highest education level attained. This contrasts with broader demographic trends which suggest that agreement was common for individuals who continued on to university. While this may be an unexpected finding, this reinforces that demographic patterns among private landholders may not reflect general population trends (Statistics, Australian Demographic, 2010) and in such contexts, demographic factors alone may obfuscate the underlying trends in climate related beliefs when they fail to account for individual behavioral beliefs, normative beliefs and control beliefs.
Farmers’ lived experiences, particularly the extent to which they have experienced land management issues, may play a crucial role in influencing their beliefs about climate change, and, possibly their decisions related to sustainable land management practices. As previous studies have mentioned, the value of nature and sustainability can sometimes elicit disparaging attitudes, even among the conservation sector (Vucetich et al., 2015). In agricultural contexts, individuals may hold neutral or negative climate related beliefs due to a perceived displacement of rural economies by increased land zoning for nature (Lambin and Meyfroidt, 2011) which may influence climate related attitudes.
Understanding how psycho-social continue to shape individual perspectives is crucial for designing effective extension activities that aim to promote the adoption of sustainable and regenerative land management practices, particularly those geared toward reducing carbon emissions and sequestering carbon on private farms. Recognizing the diversity of viewpoints within the farming community ensures that outreach efforts are more tailored and responsive to the specific needs, values, and experiences of farmers, fostering a more inclusive and impactful approach to climate change mitigation and adaptation. Future research should explore more closely the effect of neutral attitudes on the willingness to implement land management practices that reduce emissions, both within and outside the Australian context.
The raw data supporting the conclusions of this article will be made available by the authors, without undue reservation.
The studies involving humans were approved by Ethics Committee, Southern Cross University. The studies were conducted in accordance with the local legislation and institutional requirements. The participants provided their written informed consent to participate in this study.
SH: Conceptualization, Data curation, Formal analysis, Funding acquisition, Investigation, Methodology, Project administration, Resources, Software, Supervision, Validation, Visualization, Writing – original draft, Writing – review & editing. HL: Conceptualization, Funding acquisition, Methodology, Resources, Validation, Writing – original draft, Writing – review & editing. MA: Conceptualization, Data curation, Funding acquisition, Methodology, Resources, Validation, Writing – review & editing.
The author(s) declare that financial support was received for the research, authorship, and/or publication of this article. This work was supported by the Soil Cooperative Research Centre (CRC) projects 1.2.4 and 1.2.5.
This research has been funded and supported by the Cooperative Research Centre for High Performance Soils (Soil CRC) whose activities are funded by the Australian Government’s Cooperative Research Centre Program.
The authors declare that the research was conducted in the absence of any commercial or financial relationships that could be construed as a potential conflict of interest.
All claims expressed in this article are solely those of the authors and do not necessarily represent those of their affiliated organizations, or those of the publisher, the editors and the reviewers. Any product that may be evaluated in this article, or claim that may be made by its manufacturer, is not guaranteed or endorsed by the publisher.
The Supplementary material for this article can be found online at: https://www.frontiersin.org/articles/10.3389/fsufs.2024.1392746/full#supplementary-material
ABARES (2022). Land use of Australia 2010–11 to 2015–16, 250 m. Australian Bureau of Agricultural and Resource Economics and sciences. Canberra: ABARES.
Adams, V. M., Pressey, R. L., and Stoeckl, N. (2014). Estimating landholders’ probability of participating in a stewardship program, and the implications for spatial conservation priorities. PLoS One 9:e97941. doi: 10.1371/journal.pone.0097941
Ajzen, I. (1991). The theory of planned behavior. Organ. Behav. Hum. Decis. Process. 50, 179–211. doi: 10.1016/0749-5978(91)90020-T
Ajzen, I., Fishbein, M., Lohmann, S., and Albarracín, D. (2018). The influence of attitudes on behavior. Handb. Attit. 1, 197–255,
Alexanderson, M. S., Luke, H., and Lloyd, D. J. (2023). Regenerative farming as climate action. J. Environ. Manag. 347:119063. doi: 10.1016/j.jenvman.2023.119063
Allen, M, Dube, OP, Solecki, W, Aragón-Durand, F, Cramer, W, Humphreys, S, et al. (2018). Special report: global warming of 1.5 C. Intergovernmental Panel on Climate Change (IPCC).
Anderson, M. D., and Rivera-Ferre, M. (2021). Food system narratives to end hunger: extractive versus regenerative. Curr. Opin. Environ. Sustain. 49, 18–25. doi: 10.1016/j.cosust.2020.12.002
Arbuckle, J. Jr., Gordon, W., Morton, L., and Hobbs, J. (2015). Understanding farmer perspectives on climate change adaptation and mitigation: the roles of trust in sources of climate information, climate change beliefs, and perceived risk. Environ. Behav. 47, 205–234. doi: 10.1177/0013916513503832
Archibald, C. L., Summers, D. M., Graham, E. M., and Bryan, B. A. (2024). Habitat suitability maps for Australian flora and fauna under CMIP6 climate scenarios. GigaScience 13:giae002. doi: 10.1093/gigascience/giae002
Arias, Paola, Bellouin, Nicolas, Coppola, Erika, Jones, Richard, Krinner, Gerhard, Marotzke, Jochem, et al. (2021). Climate change 2021: The physical science basis. Contribution of working group I to the Sixth Assessment Report of the Intergovernmental Panel on Climate Change; Technical Summary.
Barletti, J. P., Larson, A. M., Hewlett, C., and Delgado, D. (2020). Designing for engagement: A realist synthesis review of how context affects the outcomes of multi-stakeholder forums on land use and/or land-use change. World Dev. 127:104753:104753. doi: 10.1016/j.worlddev.2019.104753
Beggs, P. J., Zhang, Y., McGushin, A., Trueck, S., Linnenluecke, M. K., Bambrick, H., et al. (2021). The 2021 report of the MJA–lancet countdown on health and climate change: Australia increasingly out on a limb. Med. J. Aust. 215, 390–392.e22. doi: 10.5694/mja2.51302
Bizikova, L., Nkonya, E., Minah, M., Hanisch, M., Turaga, R. M. R., Speranza, C. I., et al. (2020). A scoping review of the contributions of farmers’ organizations to smallholder agriculture. Nat. Food 1, 620–630. doi: 10.1038/s43016-020-00164-x
Brosius, P. J., Tsing, A. L., and Zerner, C. (2005). Communities and conservation: Histories and politics of community-based natural resource management. Rowman Altamira: AltaMira Press.
Burgess, Tahnee, Burgmann, James R, Hall, Stephanie, Holmes, David, and Turner, Elizabeth. (2020). "Black summer: Australian newspaper reporting on the nation’s worst bushfire season." Monash climate change communication research hub:30.
Bürkner, P.-C. (2017). Brms: AnRPackage for Bayesian multilevel models UsingStan. J. Stat. Softw. 80, 1–28. doi: 10.18637/jss.v080.i01
Cardoso, S. P., and James, H. S. (2012). Ethical frameworks and farmer participation in controversial farming practices. J. Agric. Environ. Ethics 25, 377–404. doi: 10.1007/s10806-011-9306-6
Carmichael, J. T., and Brulle, R. J. (2017). Elite cues, media coverage, and public concern: an integrated path analysis of public opinion on climate change, 2001–2013. Environmental Politics. 26, 232–252.
Castonguay, A. C., Polasky, S., Holden, M. H., Herrero, M., Mason-D’Croz, D., Godde, C., et al. (2023). Navigating sustainability trade-offs in global beef production. Nat. Sustain. 6, 284–294. doi: 10.1038/s41893-022-01017-0
Clarke, B., Otto, F., Stuart-Smith, R., and Harrington, L. (2022). Extreme weather impacts of climate change: an attribution perspective. Environ. Res. Clima. 1:012001. doi: 10.1088/2752-5295/ac6e7d
Cook, J., and Lewandowsky, S. (2016). Rational irrationality: modeling climate change belief polarization using Bayesian networks. Top. Cogn. Sci. 8, 160–179. doi: 10.1111/tops.12186
Cornell, S., Berkhout, F., Tuinstra, W., Tàbara, D., Jäger, J., Chabay, I., et al. (2013). Opening up knowledge systems for better responses to global environmental change. Environ. Sci. Pol. 28, 60–70. doi: 10.1016/j.envsci.2012.11.008
Coumou, D., and Rahmstorf, S. (2012). A decade of weather extremes. Nat. Clim. Chang. 2, 491–496. doi: 10.1038/nclimate1452
Curtis, A., Byron, I., and MacKay, J. (2005). Integrating socio-economic and biophysical data to underpin collaborative watershed management. J. Am. Wat. Resour. Assoc. 41, 549–563. doi: 10.1111/j.1752-1688.2005.tb03754.x
Curtis, A., Luke, H., McDonald, S., and Curtis, S. (2020). Social benchmarking for natural resource Management: 2019 north Central Victoria. Lismore NSW, Australia: Southern Cross University.
Curtis, A., McDonald, S., Mendham, E., and Sample, R. (2008). Understanding the social drivers for natural resource management in the Wimmera region. Albury: Institute for Land, Water and Society.
Curtis, A., and Mendham, E. (2015). The social drivers of natural resource management. North Central Victoria: Institute for Land, Water and Society, Charles Sturt University.
Demski, C., Stuart, C., Nick, P., Robert, G. S., and Alexa, S. (2017). Experience of extreme weather affects climate change mitigation and adaptation responses. Climatic change. 140, 149–164.
Dillman, D. A. (1991). The design and administration of mail surveys. Annu. Rev. Sociol. 17, 225–249. doi: 10.1146/annurev.so.17.080191.001301
Dillman, D. A., Smyth, J. D., and Christian, L. M. (2014). Internet, phone, mail, and mixed-mode surveys: The tailored design method Hoboken, New Jersey: John Wiley & Sons.
Dunlap, R. E., Chenyang, X., and Aaron, M. M. (2001). Politics and environment in America: Partisan and ideological cleavages in public support for environmentalism. Environmental politics. 10, 23–48.
Emtage, N., and Herbohn, J. (2012). Assessing rural landholders diversity in the wet tropics region of Queensland, Australia in relation to natural resource management programs: a market segmentation approach. Agric. Syst. 110, 107–118. doi: 10.1016/j.agsy.2012.03.013
Frick, M., Neu, L., Liebhaber, N., Sperner-Unterweger, B., Stötter, J., Keller, L., et al. (2021). Why do we harm the environment or our personal health despite better knowledge? The knowledge action gap in healthy and climate-friendly behavior. Sustain. For. 13:13361. doi: 10.3390/su132313361
Gordon, E., Davila, F., and Riedy, C. (2023). Regenerative agriculture: a potentially transformative storyline shared by nine discourses. Sustain. Sci. 18, 1833–1849. doi: 10.1007/s11625-022-01281-1
Groth-Joynt, T. M., Curtis, A., Mendham, E., and Toman, E. (2020). Does rural landowner identity shape the adoption of sustainable farming practices? Aust. J. Environ. Manag. 27, 309–328. doi: 10.1080/14486563.2020.1786862
Hatfield, J. L., Boote, K. J., Kimball, B. A., Ziska, L. H., Izaurralde, R. C., Ort, D., et al. (2011). Climate impacts on agriculture: implications for crop production. Agron. J. 103, 351–370. doi: 10.2134/agronj2010.0303
Hochman, Z., Gobbett, D. L., and Horan, H. (2017). Climate trends account for stalled wheat yields in Australia since 1990. Glob. Chang. Biol. 23, 2071–2081. doi: 10.1111/gcb.13604
Hogan, A., Berry, H. L., Ng, S. P., and Bode, A. (2011). Decisions made by farmers that relate to climate change. Agric. Sci. 23, 36–39,
Howden, S. M., Soussana, J.-F., Tubiello, F. N., Chhetri, N., Dunlop, M., and Meinke, H. (2007). Adapting agriculture to climate change. Proc. Natl. Acad. Sci. 104, 19691–19696. doi: 10.1073/pnas.0701890104
Hughes, N., Lu, M., Soh, W. Y., and Lawson, K. (2022). Modelling the effects of climate change on the profitability of Australian farms. Clim. Chang. 172:12. doi: 10.1007/s10584-022-03356-5
Jorgensen, B. S., and Stedman, R. C. (2001). Sense of place as an attitude: lakeshore owners attitudes toward their properties. J. Environ. Psychol. 21, 233–248. doi: 10.1006/jevp.2001.0226
Joshi, A., Saket, K., Satish, C., and Kumar, D. P. (2015). Likert scale: Explored and explained. British journal of applied science & technology. 7, 396–403.
King, A. D., Karoly, D. J., and Henley, B. J. (2017). Australian climate extremes at 1.5 C and 2 C of global warming. Nat. Clim. Chang. 7, 412–416. doi: 10.1038/nclimate3296
Lambin, E. F., and Meyfroidt, P. (2011). Global land use change, economic globalization, and the looming land scarcity. Proc. Natl. Acad. Sci. 108, 3465–3472. doi: 10.1073/pnas.1100480108
Leventon, J., Abson, D. J., and Lang, D. J. (2021). Leverage points for sustainability transformations: nine guiding questions for sustainability science and practice. Sustain. Sci. 16, 721–726. doi: 10.1007/s11625-021-00961-8
Little, R. J. A., and Rubin, D. B. (2019). Statistical analysis with missing data. 3rd Edn: Hoboken, New Jersey: Wiley.
Lockwood, M., Davidson, J., Curtis, A., Stratford, E., and Griffith, R. (2009). Multi-level environmental governance: lessons from Australian natural resource management. Aust. Geogr. 40, 169–186. doi: 10.1080/00049180902964926
Luke, Hanabeth, Allan, Catherine, Curtis, Allan, McDonald, Simon, Lake, Warren, Baker, Claire, et al. (2021). "Surveying on-farm practices: drivers of farmer decision-making."
Luke, Hanabeth, Baker, Claire, Allan, Catherine, and McDonald, Simon. (2020). "Agriculture on the Eyre peninsula: Rural landholder social benchmarking report 2020."
Luke, Hanabeth, Lake, Warren, Allan, C, McDonald, Simon, Hernandez, Stephanie, and Alexanderson, Mathew. (2022). Agriculture in Central West NSW: rural Landholder Social Benchmarking Report.
Mazur, N., Curtis, A., and Rogers, M. (2013). Do you see what I see? Rural landholders' belief in climate change. Soc. Nat. Resour. 26, 75–85. doi: 10.1080/08941920.2012.686650
McCarl, B. A. (2010). Analysis of climate change implications for agriculture and forestry: an interdisciplinary effort. Clim. Chang. 100, 119–124. doi: 10.1007/s10584-010-9833-6
McDonald, M. (2021). After the fires? Climate change and security in Australia. Aust. J. Polit. Sci. 56, 1–18. doi: 10.1080/10361146.2020.1776680
McDonald, R. I., Chai, H. Y., and Newell, B. R. (2015). Personal experience and the ‘psychological distance’ of climate change: an integrative review. J. Environ. Psychol. 44, 109–118. doi: 10.1016/j.jenvp.2015.10.003
McIntyre, N., Moore, J., and Yuan, M. (2008). A place-based, values-centered approach to managing recreation on Canadian crown lands. Soc. Nat. Resour. 21, 657–670. doi: 10.1080/08941920802022297
Meertens, R. M., and Lion, R. (2008). Measuring an individual's tendency to take risks: the risk propensity scale 1. J. Appl. Soc. Psychol. 38, 1506–1520. doi: 10.1111/j.1559-1816.2008.00357.x
Minato, W., Curtis, A., and Allan, C. (2010). Social norms and natural resource management in a changing rural community. J. Environ. Pol. Plan. 12, 381–403. doi: 10.1080/1523908X.2010.531084
Morrison, Mark, Durante, Jeanette, Greig, Jennifer, and Ward, John. (2008). "Encouraging participation in market based instruments and incentive programs."
Murtagh, F., and Contreras, P. (2012). Algorithms for hierarchical clustering: an overview. Wiley Interdisc. Rev. Data Min. Knowl. Disc. 2, 86–97. doi: 10.1002/widm.53
Niles, M. T., and Mueller, N. D. (2016). Farmer perceptions of climate change: Associations with observed temperature and precipitation trends, irrigation, and climate beliefs. Global Environmental Change. 39,133–142. doi: 10.1016/j.gloenvcha.2016.05.002
Pannell, D. J., Marshall, G. R., Barr, N., Curtis, A., Vanclay, F., and Wilkinson, R. (2006). Understanding and promoting adoption of conservation practices by rural landholders. Aust. J. Exp. Agric. 46, 1407–1424. doi: 10.1071/EA05037
Park, S. E., Marshall, N. A., Jakku, E., Dowd, A. M., Howden, S. M., Mendham, E., et al. (2012). Informing adaptation responses to climate change through theories of transformation. Glob. Environ. Chang. 22, 115–126. doi: 10.1016/j.gloenvcha.2011.10.003
Patrick, M. E., Mick, P. C., Michael, J. P., Virginia, L., and John, E. S. (2021). Comparison of a web‐push survey research protocol with a mailed paper and pencil protocol in the Monitoring the Future panel survey. Addiction. 116, 191–199.
Prosser, I. P., Chiew, F. H. S., and Smith, M. S. (2021). Adapting water management to climate change in the Murray–Darling basin, Australia. Water 13:2504. doi: 10.3390/w13182504
R Core Development Team (2013). R: a language and environment for statistical computing computer program. Vienna, Austria: R Core Development Team.
Robertson, M., and Murray-Prior, R. (2016). Five reasons why it is difficult to talk to Australian farmers about the impacts of, and their adaptation to, climate change. Reg. Environ. Chang. 16, 189–198. doi: 10.1007/s10113-014-0743-4
Rodriguez, M. Z., Comin, C. H., Casanova, D., Bruno, O. M., Amancio, D. R., Costa, L. d. F., et al. (2019). Clustering algorithms: a comparative approach. PLoS One 14:e0210236. doi: 10.1371/journal.pone.0210236
Rogers, M., Curtis, A., and Mazur, N. (2012). The influence of cognitive processes on rural landholder responses to climate change. J. Environ. Manag. 111, 258–266. doi: 10.1016/j.jenvman.2012.07.015
Smith, E. F., Lieske, S. N., Keys, N., and Smith, T. F. (2018). The socio-economic vulnerability of the Australian east coast grazing sector to the impacts of climate change. Reg. Environ. Chang. 18, 1185–1199. doi: 10.1007/s10113-017-1251-0
Smith, F. P., Prober, S. M., House, A. P. N., and McIntyre, S. (2013). Maximizing retention of native biodiversity in Australian agricultural landscapes—the 10:20:40:30 guidelines. Agric. Ecosyst. Environ. 166, 35–45. doi: 10.1016/j.agee.2012.01.014
Soubry, B., Sherren, K., and Thornton, T. F. (2020). Are we taking farmers seriously? A review of the literature on farmer perceptions and climate change, 2007–2018. J. Rural. Stud. 74, 210–222. doi: 10.1016/j.jrurstud.2019.09.005
Statistics, Australian Demographic . (2010). "Australian bureau of statistics." Available at: http://www.abs.gov. au (Accessed18 May 2012).
Stedman, R. C. (2002). Toward a social psychology of place. Environ. Behav. 34, 561–581. doi: 10.1177/0013916502034005001
Stern, P. C. (2000). New environmental theories: toward a coherent theory of environmentally significant behavior. J. Soc. Issues 56, 407–424. doi: 10.1111/0022-4537.00175
Stern, P. C., Perkins, J. H., Sparks, R. E., and Knox, R. A. (2016). The challenge of climate-change neoskepticism. Science 353, 653–654. doi: 10.1126/science.aaf6675
Sulemana, I., and James, H. S. (2014). Farmer identity, ethical attitudes and environmental practices. Ecol. Econ. 98, 49–61. doi: 10.1016/j.ecolecon.2013.12.011
Toman, E., Curtis, A. L., and Mendham, E. (2019). Same as it ever was? Stability and change over 15 years in a rural district in southeastern Australia. Soc. Nat. Resour. 32, 113–132. doi: 10.1080/08941920.2018.1505014
Van Buuren, S., and Groothuis-Oudshoorn, K. (2011). Mice: multivariate imputation by chained equations inR. J. Stat. Softw. 45, 1–67. doi: 10.18637/jss.v045.i03
Van Kerkhoff, L., and Pilbeam, V. (2017). Understanding socio-cultural dimensions of environmental decision-making: A knowledge governance approach. Environ. Sci. Pol. 73, 29–37. doi: 10.1016/j.envsci.2017.03.011
Vucetich, J. A., Bruskotter, J. T., and Nelson, M. P. (2015). Evaluating whether nature's intrinsic value is an axiom of or anathema to conservation. Conserv. Biol. 29, 321–332. doi: 10.1111/cobi.12464
Weber, E. U. (2016). What shapes perceptions of climate change? New research since 2010. WIREs Clim Change 7, 125–134. doi: 10.1002/wcc.377
Whish, G., and Department of Agriculture, Fisheries and Forestry (DAFF), Toowoomba, Qld, AustraliaCowley, R. A.Department of Primary Industries and Fisheries (DPIF), Katherine, NT, AustraliaDepartment of Agriculture, Fisheries and Forestry (DAFF), Toowoomba, Qld, AustraliaDepartment of Agriculture, Fisheries and Forestry (DAFF), Toowoomba, Qld, Australia , et al. . (2014). Impacts of projected climate change on pasture growth and safe carrying capacities for 3 extensive grazing land regions in northern Australia. Trop. Grassl. Forrajes Trop. 2, 151–153. doi: 10.17138/TGFT(2)151-153
Keywords: landholder attitudes, climate change, Bayesian ordinal regression, social science research, lived experience
Citation: Hernandez S, Luke H and Alexanderson MS (2024) Is human activity driving climate change? Perspectives from Australian landholders. Front. Sustain. Food Syst. 8:1392746. doi: 10.3389/fsufs.2024.1392746
Received: 28 February 2024; Accepted: 08 May 2024;
Published: 05 June 2024.
Edited by:
Gabrielle Roesch-McNally, American Farmland Trust, United StatesReviewed by:
Xiangjin Shen, Chinese Academy of Sciences (CAS), ChinaCopyright © 2024 Hernandez, Luke and Alexanderson. This is an open-access article distributed under the terms of the Creative Commons Attribution License (CC BY). The use, distribution or reproduction in other forums is permitted, provided the original author(s) and the copyright owner(s) are credited and that the original publication in this journal is cited, in accordance with accepted academic practice. No use, distribution or reproduction is permitted which does not comply with these terms.
*Correspondence: Stephanie Hernandez, c3RlcGhhbmllLmhlcm5hbmRlekBlbnZpcm9ubWVudC5uc3cuZ292LmF1
Disclaimer: All claims expressed in this article are solely those of the authors and do not necessarily represent those of their affiliated organizations, or those of the publisher, the editors and the reviewers. Any product that may be evaluated in this article or claim that may be made by its manufacturer is not guaranteed or endorsed by the publisher.
Research integrity at Frontiers
Learn more about the work of our research integrity team to safeguard the quality of each article we publish.