- 1ICAR-National Institute of Abiotic Stress Management, Pune, India
- 2Department of Forestry, CCS Haryana Agricultural University, Hisar, India
- 3Faculty of Science, Zarqa University, Zarqa, Jordan
- 4ICAR-Central Coastal Agricultural Research Institute, Old Goa, India
- 5ICAR-Central Arid Zone Research Institute, Jodhpur, Rajasthan, India
- 6ICAR-Indian Institute of Soil and Water Conservation, Vasad, Gujarat, India
- 7Botany and Microbiology Department, College of Science, King Saud University, Riyadh, Saudi Arabia
- 8College of Science, King Saud University, Riyadh, Saudi Arabia
- 9Integrated Molecular Plant Physiology Research, Department of Biology, University of Antwerp, Antwerp, Belgium
- 10Department of Agronomy, Abdul Wali Khan University, Mardan, Khyber Pakhtunkhwa, Pakistan
- 11Department of SWCE, MPKV, Rahuri, Maharashtra, India
- 12Forest Research Institute, Dehradun, India
The integration of trees into diverse land-use systems holds potential for India to meet nationally determined contribution (NDC) targets under the Paris Climate Agreement. With a target of sequestering 2.5–3 billion tons of CO2 equivalent by 2030, the study focused on the widespread and economically viable eucalyptus-based agroforestry, practiced widely in various planting geometries tailored to meet industrial end-use requirements. In this context, a detailed study was conducted to quantify the influence of five planting geometries [3 m × 3 m, 6 × 1.5 m, 17 × 1 × 1 m (paired row) and two boundary plantations (east–west and north–south directions) at 2 m away from tree to tree] of eucalyptus on intercrops [dhaincha (Sesbania aculeata)—barley (Hordeum vulgare L.) rotation] biomass, soil properties, and carbon stock of the system during 2009–2016. Results revealed that biomass accumulation of different tree components was 62.50%–74.09% in stem; 6.59%–9.14% in branch; 3.18%–5.73% in leaves; 12.20%–20.44% in stump roots; and 1.71%–3.48% in fine roots across the planting geometries. The mean carbon content of the stem, branch, leaves, and roots was 49.00, 47.00, 43.00, and 49.00%, respectively. Over the 8-year period, geometry of 3 × 3 m performed better in terms of total biomass production (344.60 Mg ha− 1 by tree biomass and 62.53 Mg ha−1 by intercrops). The independent parameter, DBH2H (DBH: diameter at breast height and H: tree height), was found to be a very good predictor of dry weight, followed by DBH alone. Among various functions (linear, allometric, logistic, Gompertz, Chapman, and exponential), the best-fit equation was allometric, i.e., B = 300.96 × DBH2H0.93 (adjusted R2 = 0.96) for eucalyptus based on universal model adequacy and validation criteria. The carbon sequestration rate was maximum (20.79 Mg C ha−1 year−1) in 3 × 3 m followed by 17 × 1 × 1 m. The total carbon stock of eucalyptus-based system (tree + crop + soil) varied significantly under different planting geometries and sole crop rotation (dhaincha–barley). The higher carbon stock (237.27 Mg ha−1) was obtained from 3 × 3 m spacing and further partitioning carbon stock in trees—166.29 Mg ha−1, crops—25.01 Mg ha−1 and soil—45.97 Mg ha−1. The paired row spacing (17 × 1 × 1 m) yielded higher crop yield and net returns (Rs. 600,475 ha−1), underscoring wide spacing’s role in system productivity and sustainability. Tree-based systems were valuable components of agriculture, advocating for their widespread adoption to reduce CO2 emissions and generate income through carbon credits. These findings will provide crucial insights into sustainable land-use practices and advance India’s commitment toward adaptation of climate change mitigation strategies.
1 Introduction
In the agriculture, forestry, and land-use sectors (covering an area of 22 million km2), emissions contribute around 23% of the annual greenhouse gas output (Lynch, 2019; Masson-Delmotte et al., 2021). As an imperative to intensify agriculture for a projected global population of 10 billion by 2050 looms, emissions from this sector are set to rise (Masson-Delmotte et al., 2021; Pathak et al., 2021). The recent IPCC (2021) emphasizes the urgent need to curb emissions, highlighting the alarming increase in atmospheric carbon dioxide (CO2) concentration from 278 ppm (pre-industrial) to 415 ppm (Masson-Delmotte et al., 2021). From 1850 to 2015, land-use change released an estimated 145 ± 16 Pg of CO2 into the atmosphere, which is a substantial factor in the modification of global biogeochemical cycles (Houghton and Nassikas, 2017; Hong et al., 2018). Land-use change, particularly the conversion of forests to agriculture, has not only resulted in significant carbon emissions but has also led to the depletion of up to 60% of soil organic carbon (Aryal et al., 2022). Terrestrial ecosystems play a crucial role in offsetting 29% of anthropogenic carbon emissions by removing 3.2 ± 0.6 Pg of carbon annually through biomass and soil storage (Yue et al., 2020). In this context, agroforestry systems (AFSs), which combine trees with seasonal crops, emerge as strategic solutions, enhancing biomass and soil carbon storage while addressing climate change and economic uncertainties (Lorenz and Lal, 2014; Babu et al., 2020; Jhariya et al., 2021; Yadav et al., 2021; Jhariya et al., 2024).
Agroforestry increases carbon stocks in biomass or enhances soil through higher root production, rhizodeposition, and litter fall (Lorenz and Lal, 2014; Kumar et al., 2023). Watson et al. (2000) reported a 3.1 Mg C ha−1 year−1 increase in carbon sequestration when converting agricultural land to agroforestry. Ajit et al. (2016) reported carbon sequestration rates for different tree species ranging from 0.39 to 15.91 Mg C ha−1 year−1. Notably, Zomer et al. (2016) estimated a substantial 11.1 Pg C stored in tree biomass in agroforestry systems, highlighting the significant role of agroforestry in carbon sequestration and underscoring its importance in mitigating climate change. Agroforestry is projected to contribute around 27 Mg CO2 eq ha−1 year−1 based on 56 peer-reviewed publications (Kim et al., 2016). Despite these benefits, quantifying carbon stocks faces challenges, leading to uncertainties in mapping (Chave et al., 2014; Kuyah et al., 2016). In India, several fast-growing tree species, such as eucalyptus, poplar, acacia, leucaena, Gmelina arborea, Anthocephalus cadamba, Melia dubia, and others, are extensively utilized for various purposes owing to their short rotation, rapid growth, and industrial demand. In addition to their industrial applications, these trees can also contribute to carbon sequestration, given their high annual rates ranging from 0.25 to 19.14 Mg C ha−1 year−1 (Dhyani et al., 2016). The necessity for precise biomass equations is crucial for informing climate change policies, wood demands, and achieving the carbon sequestration goals (Ajit et al., 2011; Chave et al., 2019). Eucalyptus, a globally prominent tree with 700+ varieties, spans 90 countries, covering nearly 22 million hectares. Constituting 8% of the world’s plantation area, it ranks third after Pinus and Cunninghamia species (Food, 2010). Brazil leads with 5.7 million hectares, followed by China (4.5 million hectares) and India (3.9 million hectares). Introduced to India 250 years ago, eucalyptus gained popularity for its fast growth, adaptability, and diverse uses, covering 4 million hectares. In India, around 10% of global eucalyptus plantations exist, with approximately 0.15 million hectares added annually (Juhari, 2017). Eucalyptus is preferred for wood industries and farmers due to its adaptability, economic viability, and ecological soundness. In agroforestry plantations, the success of integrating crops with tree-based systems hinges on planting geometry and tree density, which strategically influence light, moisture, and nutrient availability. Linear or block planting is a common approach, initially incorporating intercropping for the first 2–3 years to reap benefits such as reduced tree establishment costs, income generation during the unproductive phase, and efficient resource use (Garrity, 1994; Deshmukh et al., 2023). Promoting agroforestry, especially with fast-growing species like eucalyptus, can mitigate climate change impact and build resilient farming communities (Chavan et al., 2023), with eucalyptus being planted in various geometries and densities ranging from 1 m × 1 m to 6 m × 1.5 m, accommodating 999–10,000 trees per hectare. Several studies, Ajit Rai et al. (2006), Prasad et al. (2010), Luna et al. (2014), and Ajit et al. (2016), highlighted the significant influence of tree density and planting geometries on biomass production.
Despite these advantages, diminishing crop yields often lead to the discontinuation of intercropping, causing farmers to perceive their land as unproductive and divert attention from the tree crop (Chavan and Dhillon, 2019; Chavan et al., 2022a). This underscores the critical need for well-defined planting geometry in agroforestry systems, ensuring optimal spacing to mitigate adverse effects on intercrop growth and yield (Khan and Chaudhry, 2007; Sirohi et al., 2022). Although eucalyptus-based agroforestry systems hold promise, there remains a knowledge gap regarding the influence of different eucalyptus planting geometries on intercrop biomass, soil properties, and carbon stock dynamics. Understanding the impact of planting geometry on crop yield, soil quality, biomass partitioning, and carbon stocks is essential for maximizing the benefits of agroforestry systems (Chavan et al., 2022b; Jinger et al., 2022; Rathore et al., 2023). Based on the existing literature and the preliminary findings outlined in the study, it is hypothesized that different planting geometries of eucalyptus will have varying effects on intercrop biomass production, soil properties, and carbon stock dynamics within the agroforestry system. We expect these optimized agroforestry systems to boost economic stability and income, especially in saline soil conditions. Dhaincha was chosen as a green manure crop to mitigate soil salinity, followed by barley, a widely cultivated salinity-tolerant crop in northwestern India. Eucalyptus was selected for its versatility in growing in problematic soils, such as saline ones. Consequently, a dhaincha–barley cropping system was established under eucalyptus-based agroforestry. Therefore, keeping in view, a long-term study was planned in eucalyptus to understand the effect of various planting geometry on intercrops, soil quality, biomass, carbon sequestration, and biomass equations. The findings on optimization of agroforestry practices will help practitioners, policymakers, and researchers toward sustainable and climate-resilient agriculture.
2 Materials and methods
2.1 Study site
The field experiment was set up during 2008–2009 in five spacing geometries [three spacing geometries of 3 m × 3 m, 6 × 1.5 m, and 17 × 1 × 1 m (paired row) at a uniform density of 1,111 trees ha−1 and two boundary plantations (east–west and north–south directions) at 2 m away from tree to tree with a density of 200 trees ha−1] at CCS Haryana Agricultural University, Hisar, India (29° 10′ N latitude and 75° 43′ E longitude at an elevation of 215 m above mean sea level; Figures 1, 2). The experimental area comes under a sub-tropical semiarid climate with four distinct seasons, namely, autumn (February to March), hot and dry summer (April to June), hot and humid monsoon (July to September), and winter (October to January). The annual average rainfall of the area ranges from 350 to 400 mm, with >70% of it received between July and September. The maximum temperature ranges from 40°C to 45°C in May and June, whereas in December and January, temperature ranges from 0°C to 20°C and falls to 0°C.
The experimental site was sandy loam in texture, having a pH of 8.30 and high soil electrical conductivity of 7.90 ds m−1. The soil contained 0.23% organic carbon, 109.00 kg ha−1 of available nitrogen, 9.80 kg ha−1 of available phosphorus, and 327.20 kg ha−1 of available potassium at the time of planting.
Annual crops, Sesbania aculeata (Dhaincha) in Kharif (June to October) and Hordeum vulgare (Barley) in Rabi (November to February), were intercropped under three planting geometries and two boundary plantations of eucalyptus. Dhaincha cultivation in the kharif season was discontinued due to a significant reduction in biomass production, rainfall variability, and increased shade, so it was kept fallow during kharif for the rest of the years. At 8 years of age, the mature eucalyptus trees under various planting geometries were harvested to quantify biomass and carbon stock and develop allometric equations.
2.2 Destructive sampling and biomass models
2.2.1 Field sampling of trees
A total of 70 eucalyptus trees from five planting geometries (16 from three planting geometries and 11 from two boundary plantations) were destructively harvested at the age of 8 years of planting, separated, sorted, sub-sampled, dried to constant weight at 63°C (Beets and Garrett, 2018), and weighted for biomass components [leaf, branch, bole, stump root (stump, coarse root, and fine root)] to extrapolate total component-wise dry biomass and fitting of models. The heights of selected trees were measured with the help of a measuring tape after being measured in meters (m) with an error of ±0.1 mm. The diameter at breast height (DBH) was also recorded with the help of an aluminum caliper in centimeters (m). The biomass of harvested trees was divided into two categories, i.e., aboveground biomass (stem, branch, and leaf) and belowground biomass (stump and fine–coarse roots) by using standard methodologies given by Snowdon et al. (2002) and Newaj et al. (2014). In case of fine and coarse root biomass, monoliths (50 cm × 50 cm × 50 cm) were randomly laid out at 1 m and 2 m away from the tree base in five planting geometries with five replications. The monolith sampling was done in the month of March 2017. After complete washing, the roots were filtered with 0.5-mm sieve and sorted into fine and coarse roots. All live and dead roots were sorted out and placed on soaking paper for a few minutes to minimize the water content. The root samples were dried at 63°C to a constant weight and then weighed. Furthermore, based on the monolith volume (50 cm3), total fine coarse roots (FCR) biomass was determined and extrapolated to hectare (Mg ha−1) and single trees (kg tree−1).
2.2.2 Construction and validation of biomass modeling
To construct accurate tree growth models, a combination of theoretical principles and practical observations relies on two distinct datasets—one for model estimation and another for validation. In the absence of an independent dataset for validation, the original datasets (derived from destructively harvested trees) were randomly split into two exclusive sets, comprising 80% for model estimation and 20% for validation, ensuring a pseudo-independent approach (Ajit et al., 2011).
a. Model estimation: Regression analysis was employed to explore relationships between dependent variables (tree biomass) and independent variables [DBH, height, DBH2H, DBH2, DBH2HWD, log (DBH)]. Total biomass data from harvested eucalyptus in five planting geometries were collectively analyzed to identify statistically and practically efficient independent variables. Once the superior independent variable was identified, various model forms, including linear, power (allometric), logistic, Gompertz, Chapman, and logarithmic, were applied to assess prediction efficiency.
b. Model validation: Validating a model consists of comparing its predictions with actual observations independent of those used for its fitting. Model validation consists of two aspects that are discussed in the following subheads:
i. Model optimization: The estimates of the parameter along with Wald confidence interval (95%), asymptotic standard errors (ASE), Akaike information criterion corrected (AICc), mean square error (MSE), and adjusted R2 (Gujarati, 1995) were used for judging the adequacy of the fitted model estimated by deploying the standard methodology (Ajit et al., 2011).
ii. Model diagnostics: Residual diagnostics includes plotting of probability plot of residuals, autocorrelation plots of residuals, plot of residuals against their expected values, and plot of residuals against independent variables (Ajit, 2008).
2.3 Carbon sequestration of crops, trees, and soil
2.3.1 Crop biomass
The crop biomass was determined by the quadrat method in replicated quadrates of 1 m2 each from the center of the tree rows of various spacing geometries. In case of boundary plantation (east–west and north–south directions), quadrate basis (1 m2) crops were harvested at the distances of 0–3, 3–6, 6–9, 9–12, 12–15, and 15–18 m from the tree lines on four aspects (northern and southern in east–west and eastern and western in north–south boundary plantation) in three replicates and averaged to get crop biomass per hectare basis.
The harvested green fodder yield was weighed in kg plot−1 and converted to Mg ha−1. The random multiple samples of 500 g were taken from fresh biomass, air-dried, and further kept in an oven at a temperature of 60°C for 72 h. Based on the dry matter content of these samples, the green fodder biomass was converted into dry fodder biomass (Mg ha−1).
2.3.2 Carbon content (tree and crop)
The study calculated carbon stock by multiplying dry biomass and the number of trees per hectare with their corresponding carbon values. Carbon content in all tree components was determined from oven-dried and ground samples, which were analyzed using a CHNS-O analyzer after passing through a 1.0 mm sieve. For each treatment, five trees (stem, root, leaves, and branch) were considered for carbon content estimation. Crop carbon was calculated using a reference value of 40% based on Stewart (1993).
2.3.3 Soil chemical properties
Soil samples were collected at depths ranging up to 90 cm (0–15, 15–30, 30–45, 45–60, 60–75, and 75–90 cm) to determine soil chemical properties. However, the mean value of six soil depths was considered. The soil pH and EC were measured using a pH meter and a conductivity meter in 1:2 soil-to-water ratio (Jackson, 2005). The soil available nitrogen (N), phosphorus (P), and potassium (K) were determined by the alkaline potassium permanganate distillation (Subbiah and Asija, 1956), Bray’s method (Bray and Kurtz, 1945), and ammonium acetate extractant method (Jackson, 2005), respectively.
2.3.4 Soil organic carbon (mg ha−1)
Random soil samples were collected in three replicates from the surface soil (0–15 cm) before planting trees and after 8 years of planting (0–90 cm) from five different geometries to determine bulk density and soil organic carbon (SOC). The bulk density of the soil was determined using metal core samplers of known volume. The soil samples were oven-dried at 105 ± 10°C for 48 h, and the bulk density of soil in g cm−3 was calculated by dividing the oven dry weight of the sample by the volume of the core sampler (Cresswell and Hamilton, 2002; Halli et al., 2022). The Walkley–Black method (Walkley and Black, 1934) was used to determine the SOC content. Samples were also collected from a control field for comparison.
Soil organic carbon stock (Mg ha−1) = soil organic carbon (%) × depth (cm) × bulk density (g/cm3).
2.3.5 System carbon sequestration potential over rotation
The total system carbon sequestration potential (Mg C ha−1) was computed as per the crop rotation in eucalyptus-based agroforestry systems. The formula is followed as:
2.3.6 Economics of system
The economic analysis was carried out by comparing various agroforestry systems with sole annual crops that cover one cycle of eucalyptus harvest. The costs associated with establishing the plantation were categorized into establishment cost (A), covering expenses incurred during the initial year of planting (including planting material, transportation, land preparation, transplanting, and plant protection). Operational cost (B) encompassed subsequent years’ expenses for crop and tree maintenance, irrigation, fertilizer application, crop seeds, hoeing, weeding, and inter-row cultivation to prevent unwanted vegetation during non-crop periods. The interest rate on working capital (A + B) was set at 9%. Other factors, such as management cost (10%) and risk cost (10%), along with year-wise existing rental value of land, were included for the estimation of financial analysis. The cost–benefit parameters used for comparing the systems were net returns, net present value (NPV) at a 12% discounting rate, internal rate of return (IRR), and benefit–cost ratio (BCR). The calculation of LEV has been done using the Faustmann model that combines annual revenue flow from intercropping production and final timber harvest of eucalyptus trees (Klemperer, 2003; Guo et al., 2006). The value (price) of trees was determined by referencing the Haryana Forest Development Corporation’s purchase list for eucalyptus, which is fixed on girth basis. Concurrently, the price of crops was computed based on the market rates prevailing in the respective years. The costs and income from intercropping and trees were also calculated. The economic viability of different planting geometries for eucalyptus-based food systems was assessed using standard methodology, aligning with previous research methodologies outlined by Chavan and Dhillon, 2019 and Chavan et al. (2022b).
Net present value: NPV was estimated as under:
Benefit–cost ratio (BCR): Discounted benefits were divided by the discounted costs to obtain the BC ratio. BCR can be expressed as follows:
Internal rate of return (IRR): Internal rate of return is defined as that rate of discount that equates the present value of a stream of net benefits with the initial investment outlay, or IRR, which is that rate at which the PNV of cash flow is zero.
2.4 Statistical analysis
The experiment was laid out in a randomized block design with five treatments (planting geometry) and five replications. Crop yield, tree biomass, tree carbon, soil carbon, and growth parameters in eucalyptus-based agroforestry were assessed using one-way ANOVA at the 5% significance level, followed by a post-hoc test of the least significant difference using the OPSTAT statistical software (Panse and Sukhatme, 1954; Sheoran et al., 1998). Five planting geometries were considered as fixed effects and replications as random effects. For carbon content in various components of eucalyptus trees and the rate of carbon sequestration in trees, one-way ANOVA followed by Tukey’s post-hoc test was conducted. Randomly harvested 70 trees of eucalyptus from five planting geometries were used to develop component-wise biomass models by using Sigma Plot version 14, involving computations of descriptive statistics, equation fitting, graph plotting for residual diagnostics, and model validation.
3 Results and discussion
3.1 Growth of eucalyptus
Tree growth is a function of age, spacing, and site quality. The growth of trees at various spacing differed considerably over 8 years of rotation (Figure 3). In the present study, maximum tree height (22.84 m) was found in 3 × 3 m, followed by east–west boundary system (21.06 m), while the east–west boundary plantation exhibited 16% more DBH than the other planting geometries of eucalyptus under study. The mean annual increment for height and DBH ranged from 2.28 to 2.86 m year−1 and 2.22 to 3.13 cm year−1 in different spacing, respectively. The decrease in tree diameter over low density may be attributed to a higher number of trees per unit area. Physiologically, tree grows more laterally in the absence of competition. Earlier studies reported that wider spacing facilitated higher DBH (Bernardo et al., 1998; De Oliveira et al., 2018). However, tree spacing affected considerably the MAI of height (2.28–2.86 m year−1) and DBH (2.28–2.86 m year−1) in our study, and it is in agreement with previous studies (Prasad et al., 2010, 2012), while in another study (Ajit et al., 2016), they reported that trees with closer spacing gain more height growth than DBH. However, Ajit et al. (2016) reported a higher MAI of 3.14–3.62 m for height and 3.22–3.88 cm for DBH at 8 years of rotation of eucalyptus in Central India. Hence, higher tree density (tree ha−1) leads to more competition for resources (light, water, and nutrients), which have several manifold phenotypic effects on an individual tree as well as the stand of tree. Moreover, empirical data indicate that increased competition resulting from higher tree density significantly impedes the development of the trees within the plantation (Binkley et al., 2004). This suggests that high stocking rates may lengthen the time necessary to produce trees of appropriate size for sawing and/or plywood production.
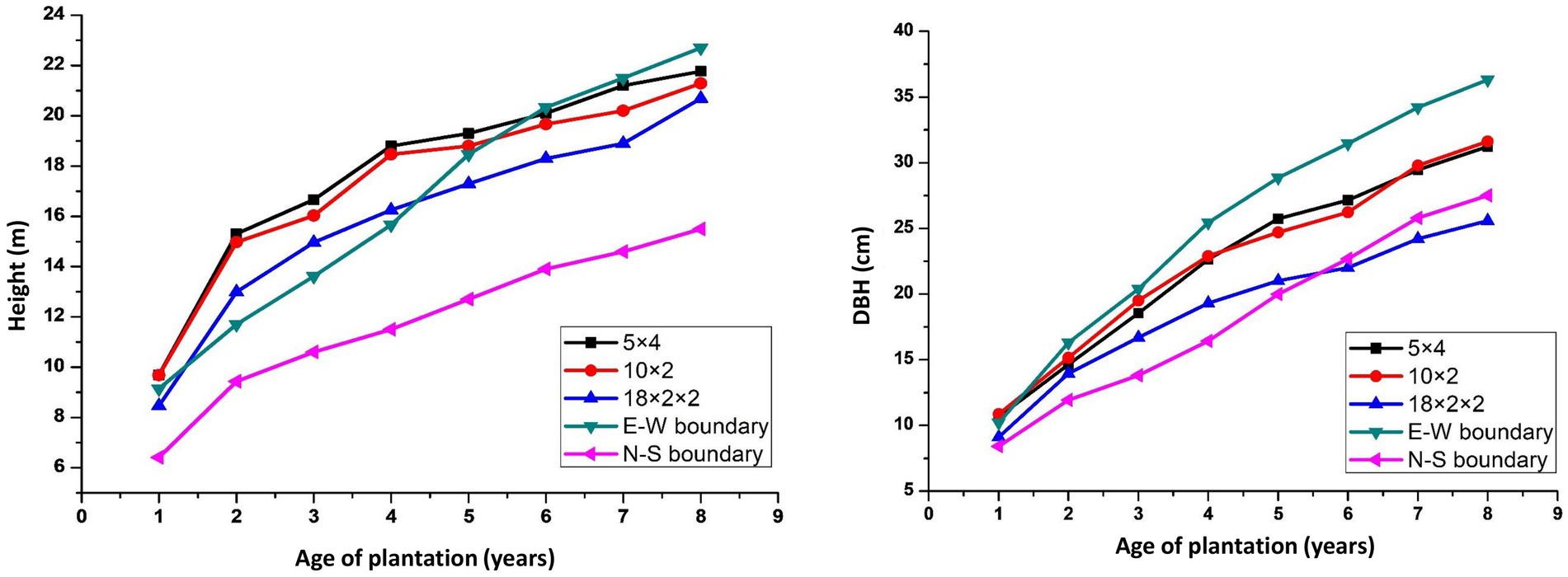
Figure 3. Tree height (A) and DBH (B) under different planting geometries of eucalyptus-based agroforestry.
3.2 Soil properties as influenced by eucalyptus
By integrating woody perennials into agricultural landscapes, soil health, an essential natural resource for sustainable agroecosystems, can be significantly improved. Trees contribute to soil nutrient improvement through mechanisms like biological nitrogen fixation, nutrient retrieval, reduced nutrient losses, and enhanced release from organic matter (Uthappa et al., 2015; Fahad et al., 2022; Jinger et al., 2023). The extent and nature of these changes depend on factors such as tree species, planting geometry, litter production, crop rotations, and soil type.
The impact of different eucalyptus planting geometries on soil chemical properties after an 8-year rotation is given in Table 1. Soil pH reduction was more pronounced in tree-based cropping systems compared to sole cropping, with an average 5% decrease at 8 years. Soil EC reduction was highest in 3 m × 3 m spacing (21.16%), followed by 6 m × 1.5 m (17.44%) compared to control. Block and boundary plantations showed a 54 and 48% reduction, respectively, while control had a 45% reduction. This pH and EC decline may be attributed to organic acids from decomposed litter and twigs, aligning with previous studies (Gupta and Sharma, 2008; Uthappa et al., 2015; Bhardwaj et al., 2017; Sultan et al., 2023). When compared to initial values, the EC has decreased from 7.90 ds m−1 to 3.39 ds m−1 in 3 × 3 m and 3.55 ds m−1 in 6 × 1.5 m in eucalyptus-based agroforestry systems. This research suggests that implementing agroforestry practices on saline soil can lead to a decrease in electrical conductivity (EC) over an 8-year period. Consequently, this approach may contribute to the reclamation of saline soils, making them suitable for the cultivation of agricultural crops.
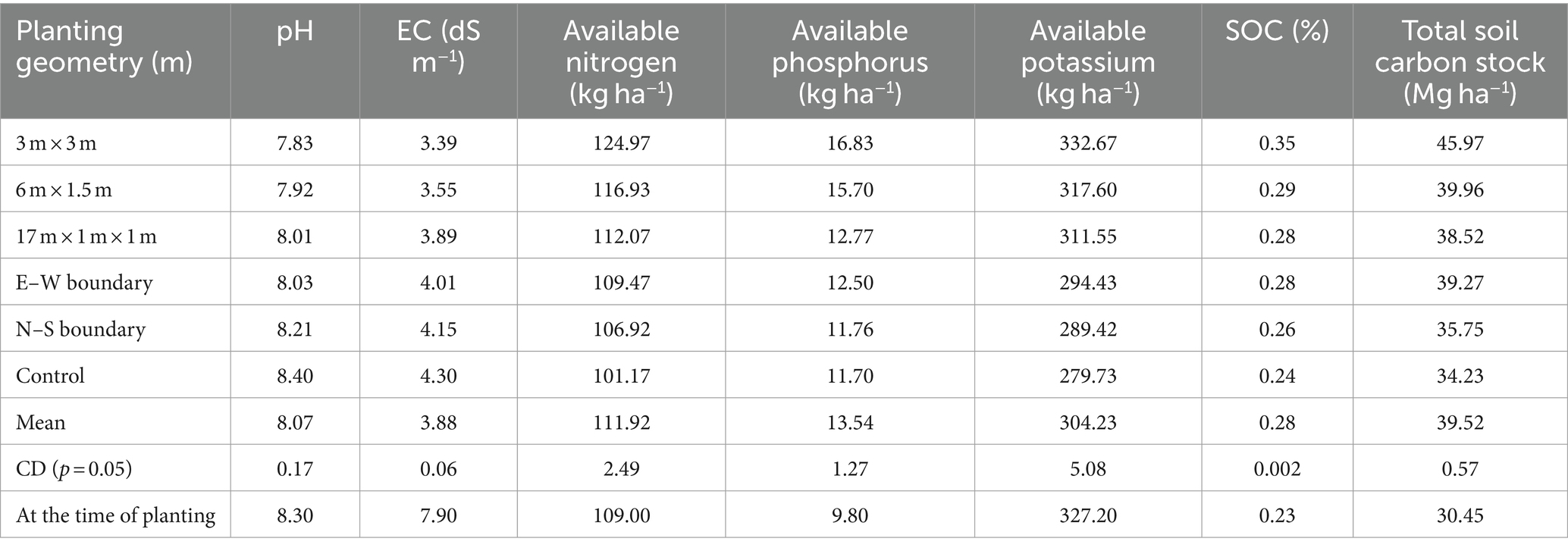
Table 1. Soil chemical properties and soil carbon stock under different planting geometries of eucalyptus (soil depth: 0–90 cm).
The study emphasized the significant influence of planting geometries on soil nutrients, viz., available nitrogen (N), phosphorus (P), and potassium (K). The 3 m × 3 m spacing exhibited the highest values for available N (124.97 kg ha−1), P (16.83 kg ha−1), and K (332.67 kg ha−1), surpassing other geometries (Table 1). The increase in available P under eucalyptus was linked to pH reduction, promoting organic acids’ release and subsequently enhancing phosphorus solubility. These findings are consistent with Bhardwaj et al. (2017), highlighting the positive impact of tree-based systems on soil enrichment through litter addition, root biomass recycling, and reduced organic matter oxidation. The findings are also in line with Singh (1975) and George (1982), who reported that eucalyptus plantations (1,111 tree ha−1) contributed 3.30 Mg ha−1 to 6.21 Mg ha−1 of leaf litter at 3- to 9-year-olds, which undergoes decomposition and mineralization, thus releasing a substantial amount of nutrients into the soil. Overall, this study reinforces the understanding of increased nutrient levels in tree-based agricultural systems.
This study also investigated the impact of tree spacing on soil organic carbon (SOC%), and soil carbon stock (Mg C ha−1). Results revealed significant variations among different tree spacings, with the highest SOC (0.35%) observed in the 3 × 3 m spacing, followed by 6 m × 1.5 m (0.29%) and 17 × 1 × 1 m (0.28%) spacing, while dhaincha–barley sole cropping exhibited the lowest SOC (0.24%). Comparing tree-based systems to control (sole cropping), SOC increased by 45.83% in 3 × 3 m, 20.83% in 6 × 1.5 m, 16.63% in 17 × 1 × 1 m, 15.83% in east–west, and 8.33% in north–south boundary plantations of eucalyptus. The maximum total SOC stock (45.97 Mg ha−1) was reported in 3 × 3 m, followed by 6 m × 1.5 m (39.96 Mg ha−1), whereas the lowest was recorded in dhaincha–barley crop rotation, i.e., control (34.23 Mg ha−1). In the present study, eucalyptus planting geometry with dhaincha–barley crop rotation stored 34.30% more carbon stock than control (sole cropping). The elevated percentage of soil organic carbon in agroforestry systems is linked to the input of litter fall, pruned materials, and the extensive rhizospheric root network of trees (Chaudhari et al., 2015; Kumar et al., 2018; Singh et al., 2023a,b; Anbessa and Utaile, 2024; Keerthika et al., 2024; Uthappa et al., 2024). Microclimate improvements, including changes in temperature, humidity, soil moisture, and light facilitated by trees, may contribute to higher SOC content. However, the complex interactions among soils, climate, trees, and their management, along with the chemical composition of leaf litter, determine soil carbon content (Lal, 2005). The findings underscore the potential of agroforestry systems in improving soil health by increasing organic matter content and optimizing soil parameters for carbon sequestration (Salunkhe et al., 2023). The substantial SOC stock recorded in tree-based systems highlights the positive impact of agroforestry on long-term soil sustainability and carbon sequestration.
3.3 Biomass modeling in eucalyptus
3.3.1 Descriptive statistics and selection of independent parameters
Tree height (20.03 m), DBH (20.87 cm), and total biomass (274.46 kg tree−1) under five planting geometries differed significantly. Variation was found to be high for the biomass components and ranged from 33.17 (aboveground biomass) to 46.06% (roots; Table 2). Ajit Rai et al. (2006), Rojo Alboreca et al. (2015), and Ajit et al. (2016) also reported variation in biomass components in eucalyptus. There was a moderate correlation between height (0.68) and total biomass; however, a strong positive correlation was observed between DBH (0.93) and total biomass. Kuyah et al. (2013) also reported that DBH was strongly and significantly correlated with above- and belowground biomass. The other parameters of statistics, such as skewness, kurtosis, and S-W normality test, proved that the datasets were derived from a normally distributed population. The negative skewed (−0.35) and kurtosis values (−0.74) were found for height and DBH in eucalyptus. This negative value indicated that distribution slightly flatter than a normal curve with the same mean and standard deviation. Kuyah et al. (2013) also reported negative skewness for height and DBH in biomass studies in eucalyptus.
The accuracy of a biomass equation depends on the selection of appropriate independent variables to develop best-fit equations. In this study, various combinations of independent (effect) variables [viz., DBH, Height, DBH2H, DBH2 × WD × H, DBH2, and ln (DBH)] were tried and plotted against the total biomass of eucalyptus to testify the accuracy and efficiencies in the prediction of biomass. On the basis of model adequacy criteria, DBH2H was found to be a better-fit independent variate than DBH alone. It was further confirmed that D2H, i.e., inclusion of height as an additional interpreter variable to DBH, increases the R2 for eucalyptus (Zewdie et al., 2009; Luna et al., 2014; Tewari, 2016) and other mixed tree species equations (Chave et al., 2001; Bastien-Henri et al., 2010).
3.3.2 Model fitting and validation of biomass equation
The destructive biomass sampling method is a prerequisite for precise and accurate modeling of tree biomass. Therefore, the sufficient number of field measurements is the precondition for developing biomass estimation models and for evaluating the biomass estimation results (Deo, 2008). In our study, 70 trees were randomly selected and harvested with a DBH range of 15.45–27.50 cm and measured component-wise to develop biomass equations. To obtain a good regression equation, at least 25 trees should be selected randomly from a wide range of DBH (de Gier, 2003).
Total biomass and DBH2H relationship for eucalyptus trees were investigated by fitting six regression functions such as allometric, logistic, Chapman, Gompertz, linear, and exponential (Table 3). Among the six fitted models, allometric model, i.e., biomass = 300.96 × DBH2H0.93 (adjusted R2 of 0.96) was the best-fit over the others. Global model characteristics like adjusted R2, F ratio, and AIC cannot guarantee model adequacy. Graphical examination of residuals (primarily studentized residuals) was used to evaluate the best-fit regression models in Figure 4 for independence, homogeneity, constant variance, and normality. There was also a detailed residual diagnosis for allometric function. Scatter plot of studentized residuals vs. predicted total biomass was clearly portrayed the homogeneity of variance over the entire range of predicted total biomass. Autocorrelation plots showed no relationships among allometric function residuals. Autocorrelation plot residuals were randomly distributed at zero slope and scattered within the confidence limit. The residuals’ independence was further shown. The quintile–quintile and probability plots showed that residuals were regularly distributed. Error term variance was constant too. Based on these statistical criteria, allometric model was best of six regression functions fitted. The results of biomass models in eucalyptus are consistent with the prior findings (Tandon et al., 1988; Dhanda and Singh, 1990; Tewari, 2016). However, these researchers did not do residual diagnosis and validation, which raises the question of reliability. The research conducted by earlier researchers (Ajit Rai et al., 2006; Kuyah et al., 2013; Ajit et al., 2016; Ounban et al., 2016) has demonstrated the superiority of allometric models in estimating biomass using residual diagnostic and validation criteria.
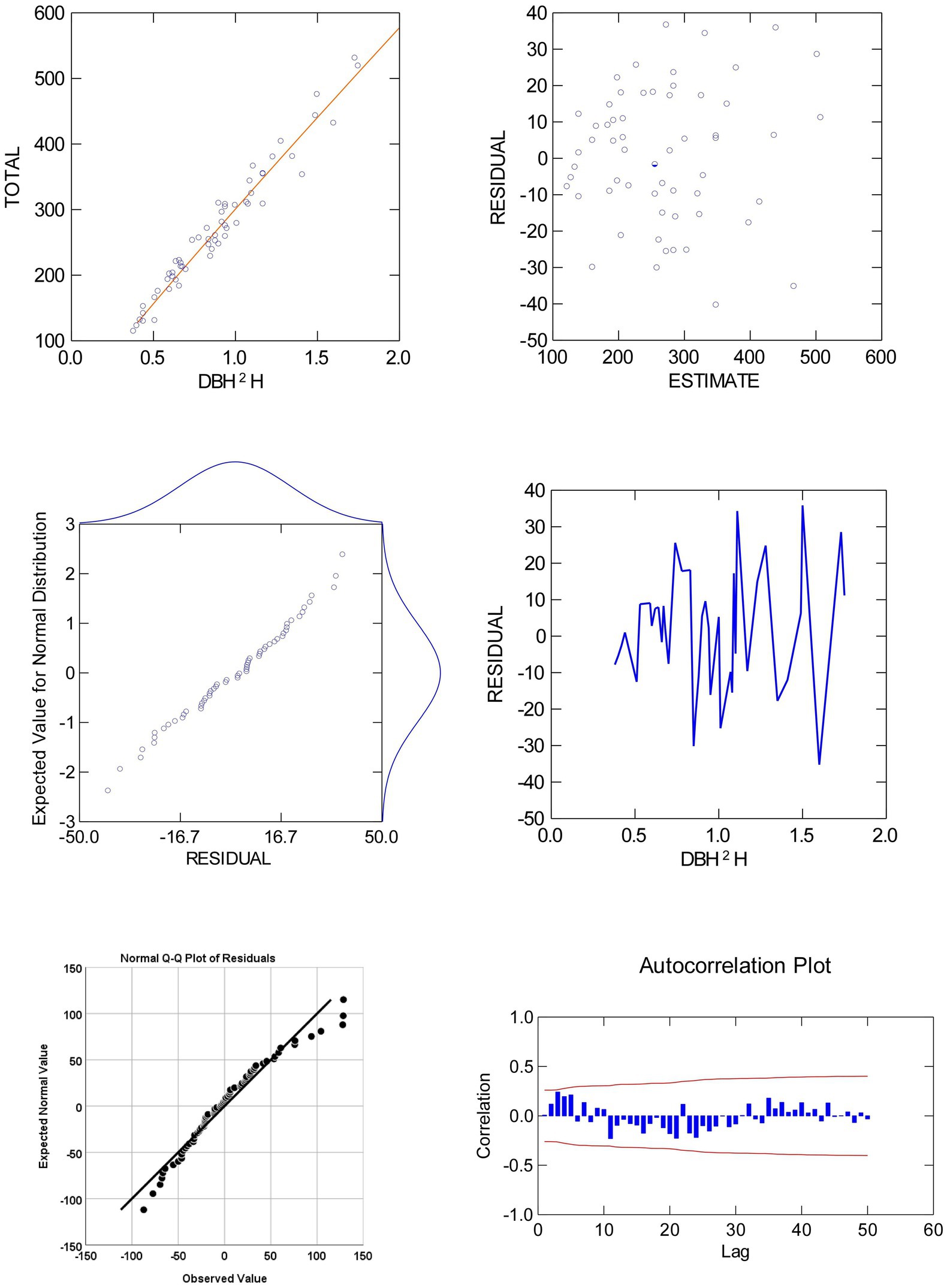
Figure 4. Plots of residual against the values of predicted and explanatory variates of total biomass of eucalyptus (clubbed data).
3.4 Carbon sequestration potential of eucalyptus-based agroforestry
3.4.1 Crop yield and biomass of dhaincha–barley crop rotation
Data pertaining to dhaincha–barley crop rotation under different planting geometries are presented in Tables 4, 5. During the Kharif season, dhaincha was planted in the initial 2 years of the study. The presence of moderate to dense shade, arising from various plant geometries of eucalyptus, led to a significant decrease in the green biomass of dhaincha. A notable 24.75% reduction in yield was observed in the 3 × 3 m spacing, whereas the least reduction was noted in the boundary plantation (2%–6%). A similar result was obtained by Prasad et al. (2010) in eucalyptus in that they reported 48%–53% loss in the post-rainy season.
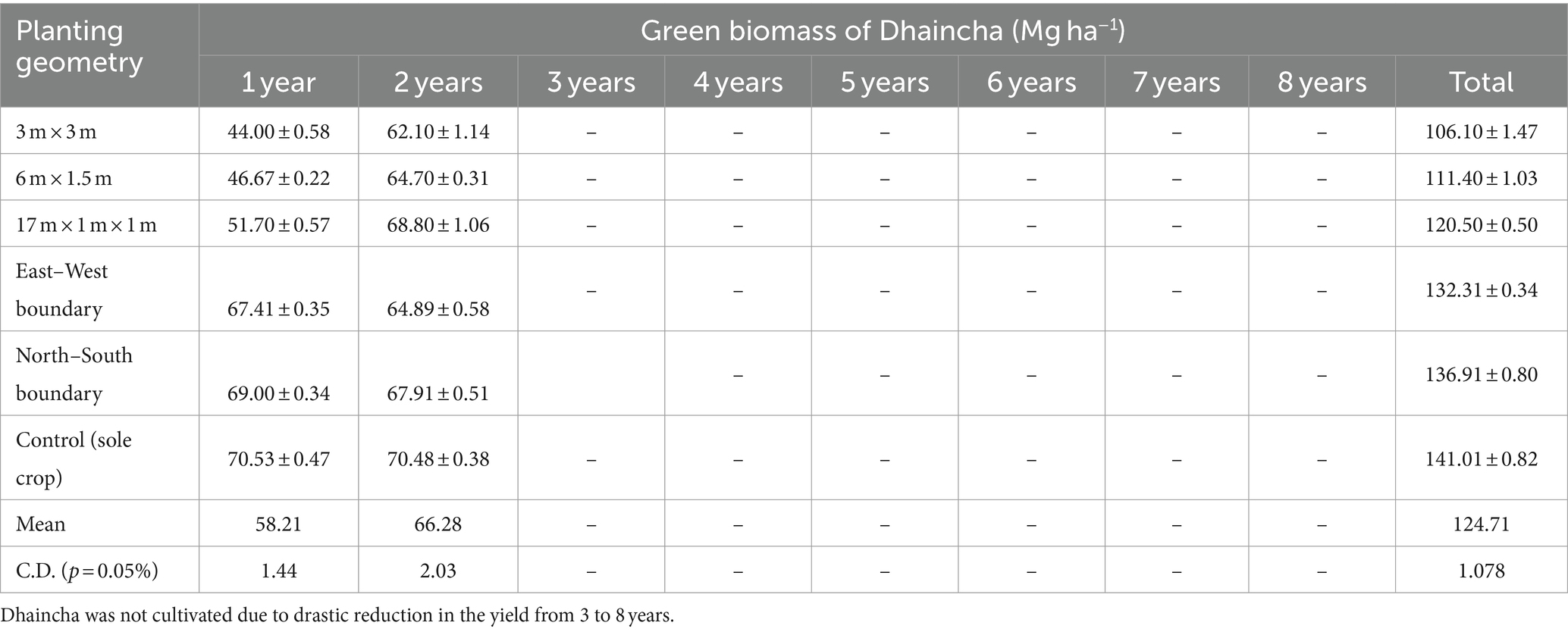
Table 4. Green biomass production of dhaincha (Mg ha−1) under different planting geometries of eucalyptus.
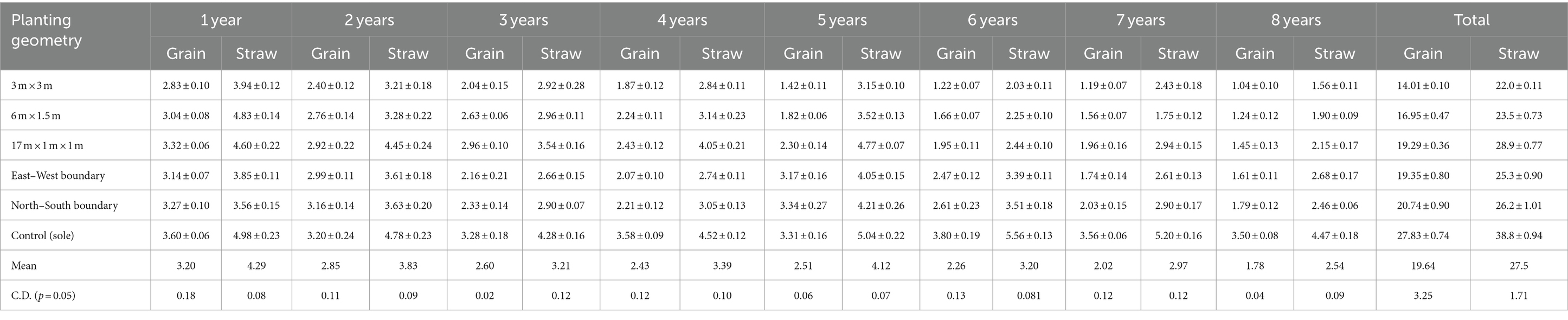
Table 5. Grain and straw yield of barley (Mg ha−1) under different planting geometries of eucalyptus at 8 years of rotation.
In the rabi season, barley was sown (every year) up to the harvesting of trees. In block spacing, the average higher grain yield of barley was obtained in paired row spacing (2.41 Mg ha−1), followed by 6 m × 1.5 m (2.12 Mg ha−1) and 3 × 3 m (1.75 Mg ha−1) spacing over a period of 8 years. Barley experienced an average yield loss of 8.92–39.0% under 1–4 years of plantation, and it further increased from 44.21 to 64.48% (fifth–eighth year of plantation) under block plantation of eucalyptus over control (sole cropping). The highest yield loss of 70.29% was recorded in 3 × 3 m at 8 years of planting. However, 20.21% higher yield in barley yield was observed when row spacing increased from 3 × 3 m to 6 m × 1.5 m spacing, and it further increased up to 36.71% in 17 × 1 × 1 m spacing at 8 years of rotation. This may be due to the fact that low light intensity intercepts decrease the rate of photosynthesis, affect directly the relative growth rate, reproductive, and ripening phases of crops, and ultimately lead to a loss of yield (Luna et al., 2009).
In boundary plantation, the maximum grain yield (2.59 Mg ha−1) was observed in the north–south in comparison to the east–west boundary plantation (2.42 Mg ha−1) but was less than control (3.24 Mg ha−1). The observation on barley crops under 8 years of plantation revealed that crop growth was severely affected near tree line (up to 6 m away), but growth increased and further improved with proceeding away from tree line in all four aspects of boundary plantation (data are not presented). Studies by Dhillon et al. (1979, 1982) revealed a maximum reduction in yield of wheat (64%), rice (58.4%), and potato (42.6%) near the tree baseline of the boundary plantation of eucalypt near the base line. In the present study, crops performed better in north–south planting of eucalyptus over other planting geometries. Similar trend was also observed in the straw yield of barley under various spacings of eucalyptus. Distance from tree line also played a noteworthy role in the yield of annual crops. Furthermore, the yield of crops increased with the increase in distance from the boundary tree lines. The results demonstrated in boundary plantation match with other studies by Singh and Kohli (1992), Kidanu et al. (2005), and Yadava (2010). Biomass and yield of dhaincha and barley crops were significantly affected due to various spacing geometries of trees. The magnitude of crop yield loss in the eucalyptus-based cropping system increased with the age of the trees due to increased competition for resources (light, moisture, and space) and subsequently hampered the growth and yield of agricultural crops. Increased competition with age is due to the increased size of the trees and their ability to mop up greater resources at the expense of crops (Dhyani and Tripathi, 1998; Prasad et al., 2010). The yield reduction was found to be higher in Kharif season as compared to the Rabi season. Tree density or spacing geometry of eucalyptus affects annual crop yield severely (Ahmed, 2004; Prasad et al., 2010, 2012).
Manipulation of planting geometry under agroforestry provides opportunity to choose appropriate crop combinations for higher yield and profitability. Therefore, modified planting geometry may help to reduce the yield reduction losses under tree-based agroforestry systems. Crop performance under eucalyptus was testified with various crop combinations by the number of authors throughout the country as well as the globe (Prasad et al., 2010; Kumar et al., 2013; Gill et al., 2016; Dhillon et al., 2018; Nyaga et al., 2019).
3.4.2 Biomass production and carbon stock of eucalyptus
Biomass plays a crucial role in the global carbon cycle and has emerged as an imperative solution for reducing climate change. Such region-specific periodic measurement studies on biomass accumulation can be used further to establish the value of agroforestry practice (Eamus et al., 2000). In this study, planting geometry had strong influence on total biomass production and partitioning among components under eucalyptus (Table 6).
The biomass production was recorded higher under 3 × 3 m (344.60 Mg ha−1) and 17 × 1 × 1 m (323.12 Mg ha−1) planting geometry, whereas the lowest (56.17 Mg ha−1) was found in north–south boundary plantation. However, the tree biomass was statistically at par between 3 × 3 m and 17 × 1 × 1 m spacing at 8 years of rotation. The annual biomass accumulation was maximum (43.04 Mg ha−1 year−1) in 3 × 3 m, followed by 17 × 1 × 1 m (40.39 Mg ha−1 year−1) and 6 m × 1.5 m (28.11 Mg ha−1 year−1) in block plantation. The aboveground biomass ranged from 42.73 to 279.17 Mg ha−1, whereas belowground biomass ranged from 13.43 to 65.43 Mg ha−1. George (1982) and Tandon et al. (1988) reported 301.50 Mg ha−1 and 302.1 Mg ha−1 at 8- to 9-year eucalyptus plantation, respectively. In addition to this, the growth of eucalyptus under block plantation was not as like in widely spaced trees, but biomass production was huge due to the high number of trees. This is a general rule that the number of trees per unit area gives a higher yield, and it is in accordance with earlier studies (Prasad et al., 2010; Forrester et al., 2013; Ajit et al., 2016). In agroforestry, tree biomass is enhanced due to management practices as compared to forest and sole plantation. However, the contribution of different tree components in total tree biomass was: 62.50%–74.09% of stem; 6.59%–9.14% of branch; 3.18%–5.73% of leaves; 12.20%–20.44% of roots; and 1.71%–3.48% of fine–coarse roots (Figure 5). The biomass portioning in the study is in similar line with earlier literature (Pande et al., 1987; Tandon et al., 1988; Prasad et al., 2010), where they reported approximately 70% biomass accumulated in eucalyptus stem/bole. Kuyah et al. (2012) reported that the contribution of different components to the total AGB was 73.7%, 22.3%, and 4.0% in stem, branch, and leaves, respectively. In case of eucalyptus, higher tree density helps to initiate self-pruning; ultimately, the percentage contribution of branches and leaves will be lower in compact spacing than in boundary plantation. Eucalyptus grows better under competition in stands (blocks), whereas tree tends to become heavily branched if planted wide apart, especially on boundaries.
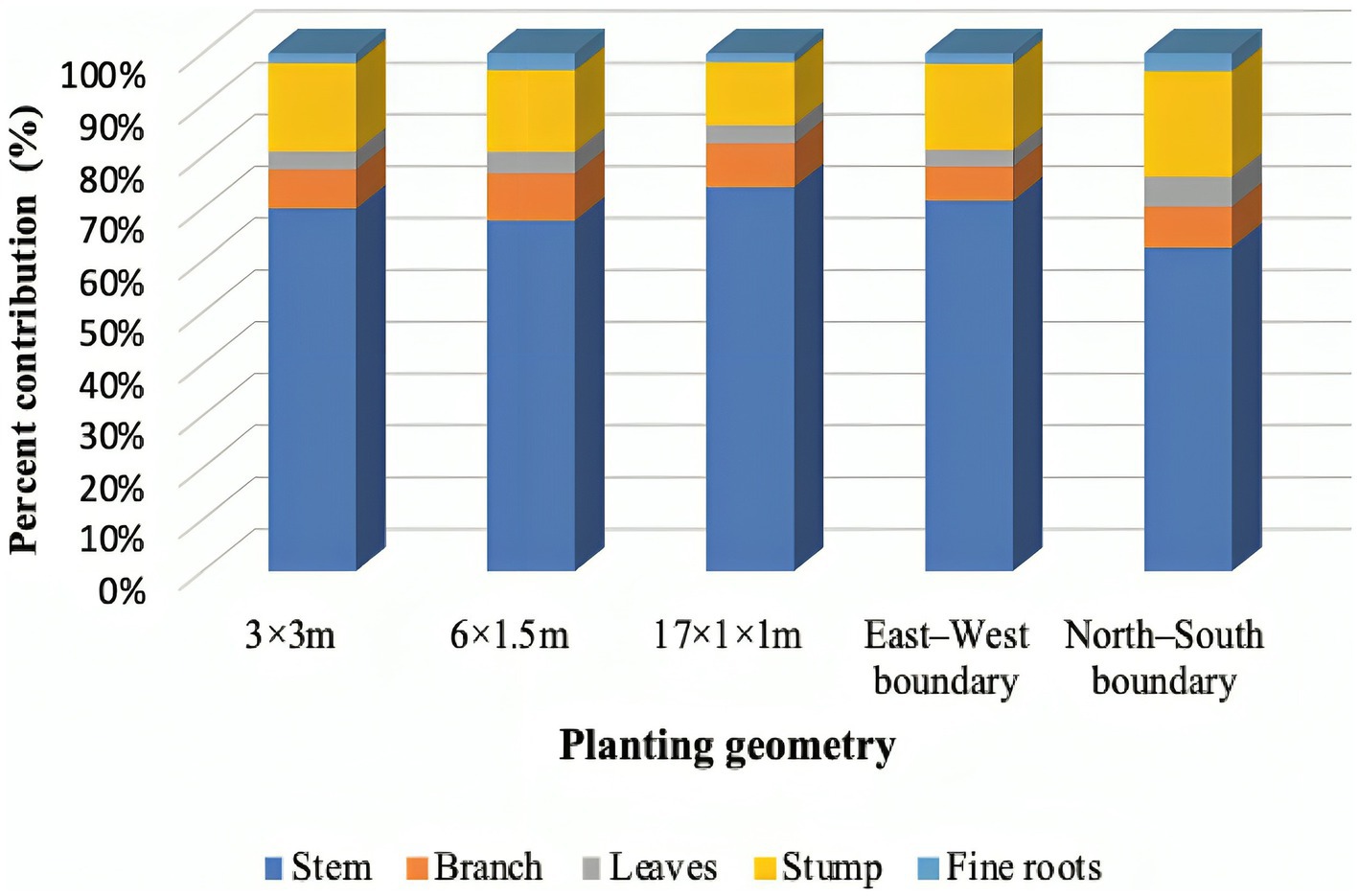
Figure 5. Tree biomass accumulations in various components of eucalyptus tree under various planting geometries.
Carbon accumulation in trees is in prime focus due to climate change mitigation measures. The percentage carbon concentration in different components of eucalyptus trees differed from 43% to 49% (Figure 6). The mean carbon content of the stem, branch, leaves, and roots in the present study was 49.00%, 47.00%, 43.00%, and 49.00%, respectively. Matthews (1993) compiled the carbon content of eucalyptus tree, which was 47.2%–49.5% in various parts. Ribeiro et al. (2015) also reported that the average carbon content in the stem, branch, leaf, and root compartments was 44.6%, 43.0%, 46.1%, and 37.8%, respectively. The highest amount of aboveground, belowground, and total carbon was estimated to be 134.29, 32.00, and 166.29 Mg ha−1 in 3 × 3 m, followed by 133.82, 21.98, and 155.80 Mg ha−1 in 17 × 1 × 1 m (paired row), respectively, while the lowest were 20.47, 6.57, and 27.03 Mg ha−1 in north–south boundary planting at 8 years of rotation (Table 7). An average carbon sequestration rate was 12.79 Mg C ha−1 year−1 in five spacing geometries (Figure 7). In boundary plantation, carbon sequestration rate was 6.78 and 3.38 Mg C ha−1 year−1 in east–west and north–south plantation, respectively, at 8 years of rotation, which was lower than block plantation. Therefore, block plantation of eucalyptus sequestrated four times more carbon than boundary plantation of eucalyptus after 8 years of rotation.
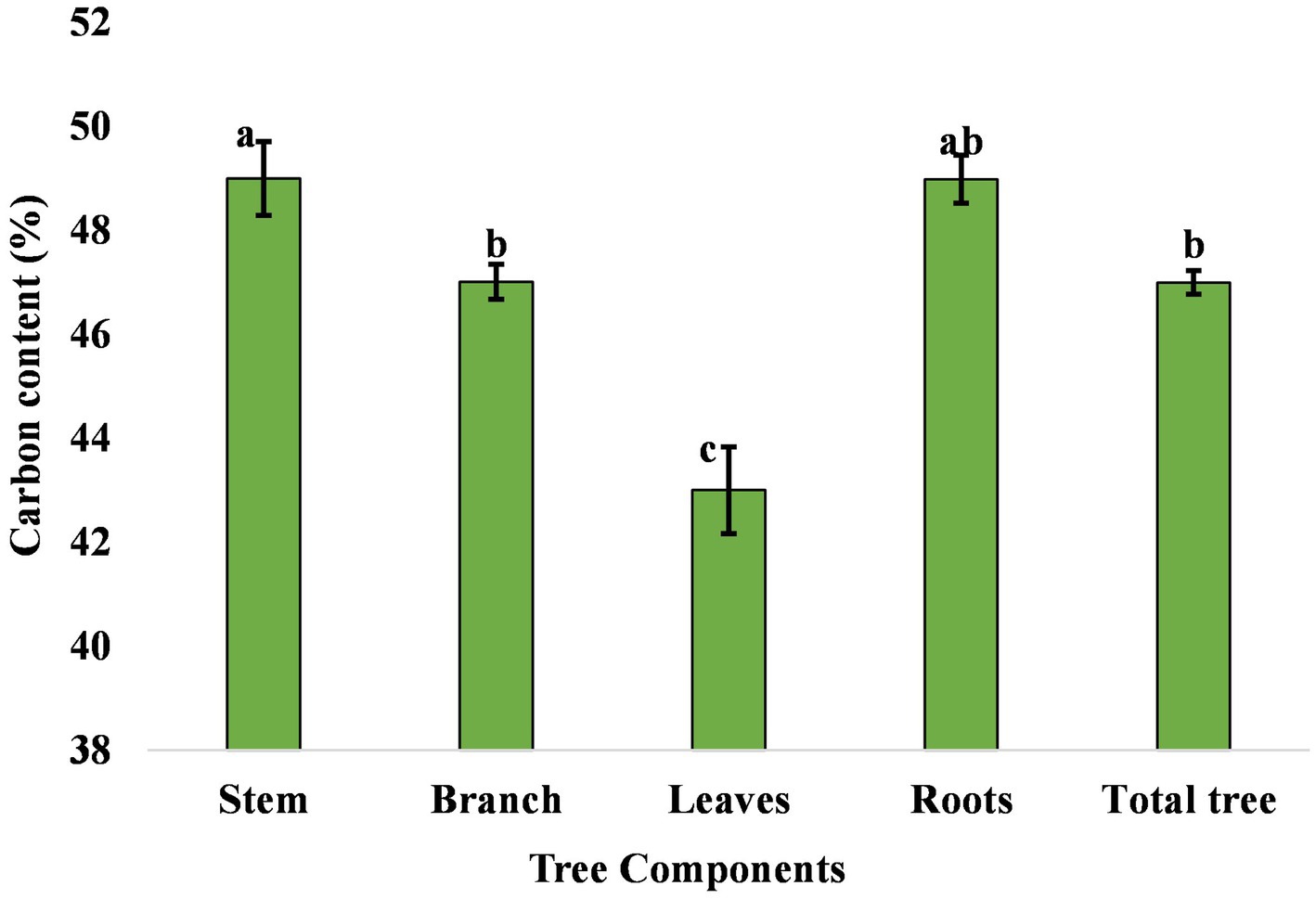
Figure 6. Carbon content in various components of eucalyptus tree. The error bar denotes the standard error. There is no statistically significant difference among the mean values that are with the same alphabets based on Tukey’s test (p < 0.05).
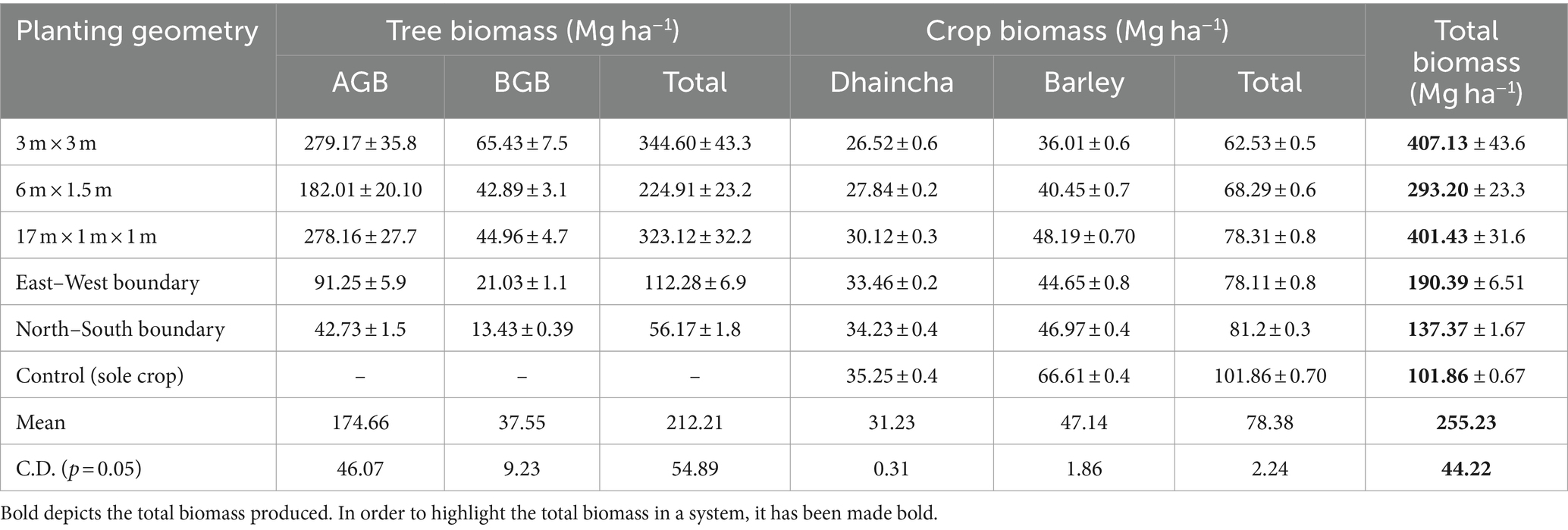
Table 7. Dry biomass production of (Mg ha−1) dhaincha–barley crop rotation under eucalyptus-based agroforestry system.
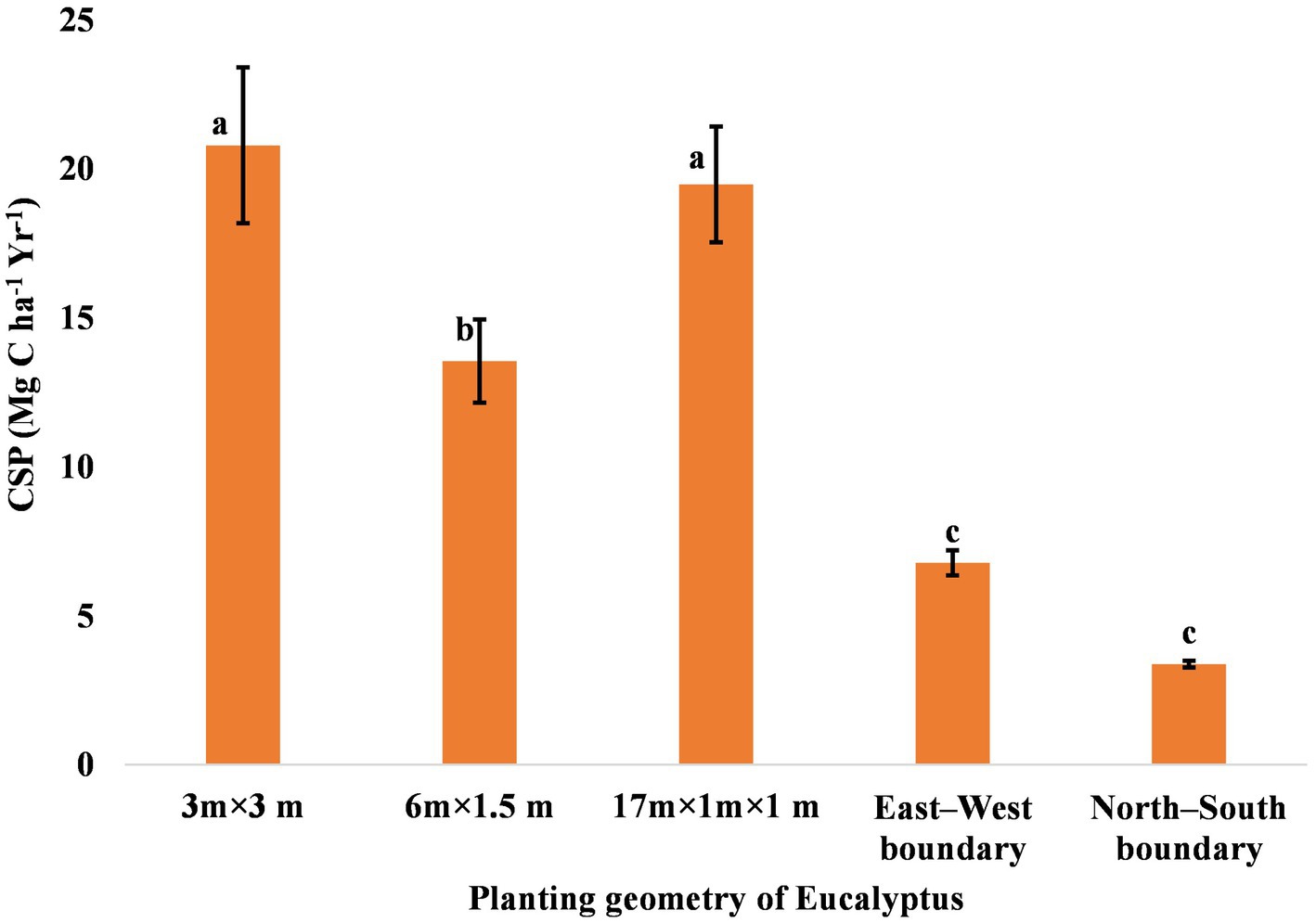
Figure 7. Rate of carbon sequestration (Mg C ha−1 year−1) in trees under various planting geometries of eucalyptus. The error bar denotes the standard error. There is no statistically significant difference among the mean values that are with the same alphabets based on Tukey’s test (p < 0.05).
Recent studies suggest that agroforestry systems (AFSs) have a higher carbon sequestration potential (Pandey, 2002; Nair et al., 2010; Ajit et al., 2016; Dhyani et al., 2016; Zhou et al., 2017; Chavan et al., 2022a,b). Zhou et al. (2017) observed an average sequestration rate of eucalyptus was 19.53 Mg C ha−1 year−1 in China. Ajit et al. (2016) also reported carbon sequestration rates for various tree species under agroforestry ranging from 0.39 to 15.91 Mg C ha−1 year−1. Specifically, carbon stock in AFSs can vary widely, with estimates ranging from 0.29 to 15.21 Mg ha−1 year−1 aboveground and 30–300 Mg C ha−1 up to a depth of 1 meter in the soil (Nair et al., 2010). In India, agroforestry systems have been estimated to sequester between 0.25 and 19.14 Mg C ha−1 year−1 for tree components and between 0.01 and 0.60 Mg C ha−1 year−1 for crop components (Pandey, 2002; Dhyani et al., 2016). There are several studies reporting either the lower or higher side of carbon sequestration rate. Hence, several factors, such as type, structure, and function of agroforestry, climate, planting geometry, soil type, socio-economic factors, management techniques, end-use, etc., significantly affect the carbon sequestration potential (Albrecht and Kandji, 2003; Tian et al., 2005) at different plantation. Among several factors, choice of planting density is a primary silvicultural decision in agroforestry, which reflects the trade-off between individual tree size and total biomass production, affecting the type, quantity, and quality of products throughout the rotation (Forrester et al., 2013).
3.4.3 Biomass and carbon stock of eucalyptus-based agroforestry (tree + crop + soil)
Under various planting geometries of eucalyptus, the total biomass varied from 137.37 to 407.13 Mg ha− 1 in dhaincha–barley crop rotation (Table 7). Over the 8-year period, spacing geometry of 3 × 3 m performed better in terms of total biomass production (407.13 Mg ha− 1), contributed by tree biomass (344.60 Mg ha− 1) and crop biomass (62.53 Mg ha− 1). Irrespective of spacing geometry, mean total biomass of eucalyptus was 285.79 Mg ha−1, whereas in control (treeless system), biomass production was only 101.87 Mg ha−1. It is interesting to point out that 80.61 and 49.93% biomass was contributed by tree components under block and boundary plantations, respectively over 8 years of rotation. Furthermore, eucalyptus-based cropping system exhibited 27.70% reduction in crop biomass over control (sole cropping). Toky et al. (1989) reported that 68% biomass was contributed by the trees in agri-hortisilvicultural system; these findings support the present investigation.
In the present study, carbon stock (Mg C ha−1) among various carbon pools indicates that vegetation stock (aboveground biomass followed by belowground biomass) and soil carbon contributed toward aggregate carbon pools (i.e., system total carbon) under various planting geometries at 8 years of study (Table 8).
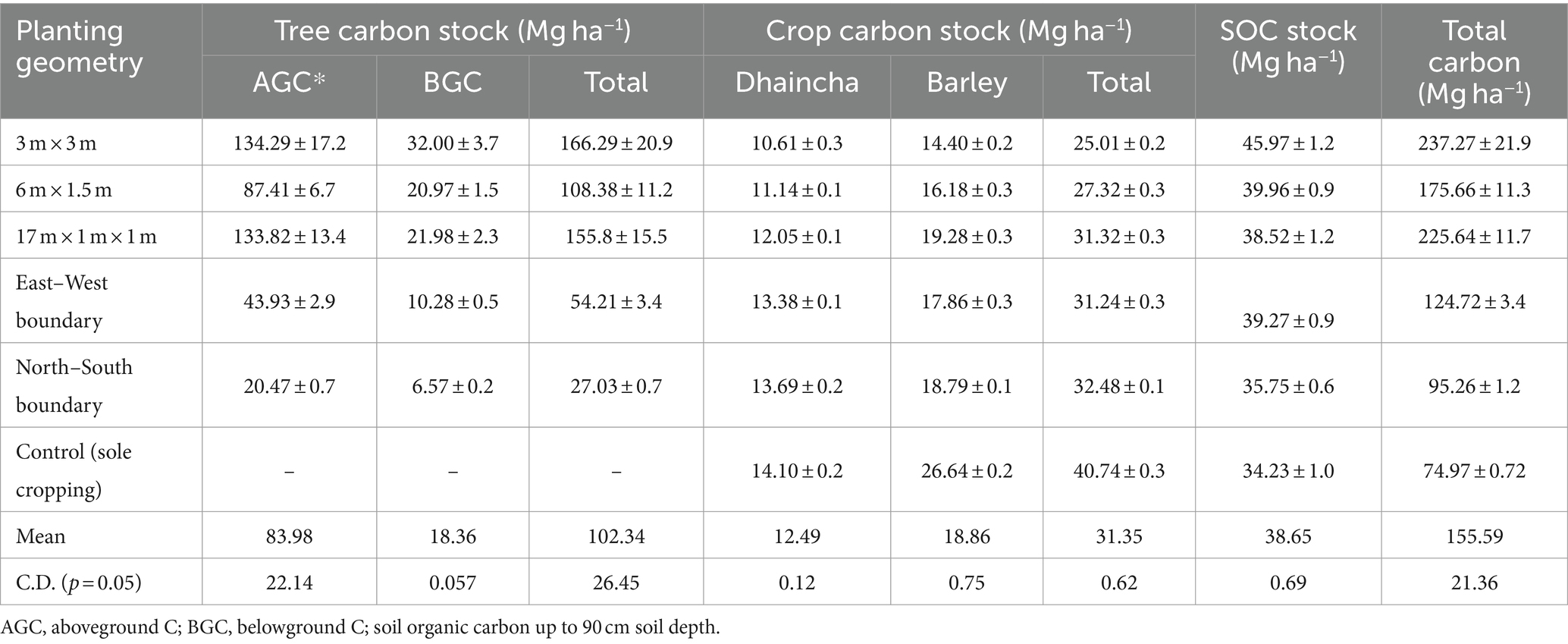
Table 8. Carbon stock (Mg ha−1) of dhaincha–barley crop rotation under eucalyptus-based agroforestry system.
The total carbon stocks of eucalyptus-based cropping systems (including trees, crops, and soil) exhibited significant variation across different planting geometries and sole crop rotations (dhaincha–barley). The findings clearly indicate that integrating tree components into sole cropping can enhance carbon stock by two to three times. Notably, the highest carbon stock (237.27 Mg ha−1) was observed in the 3 × 3 m spacing, with the breakdown showing 166.29 Mg ha−1 in trees, 25.01 Mg ha−1 in crops, and 45.97 Mg ha−1 in soil (Figure 8). Moreover, the total carbon stock in the system was notably influenced by tree density and planting geometry. In close-planted systems (block) and boundary plantation systems, trees contributed an average of 66.94 and 35.92%, crops contributed 13.32 and 29.57%, and soil contributed 19.73 and 34.51% of the total carbon stock, respectively. In contrast, in the control (sole crop), crops accounted for 54.34% and soil for 45.66%, respectively.
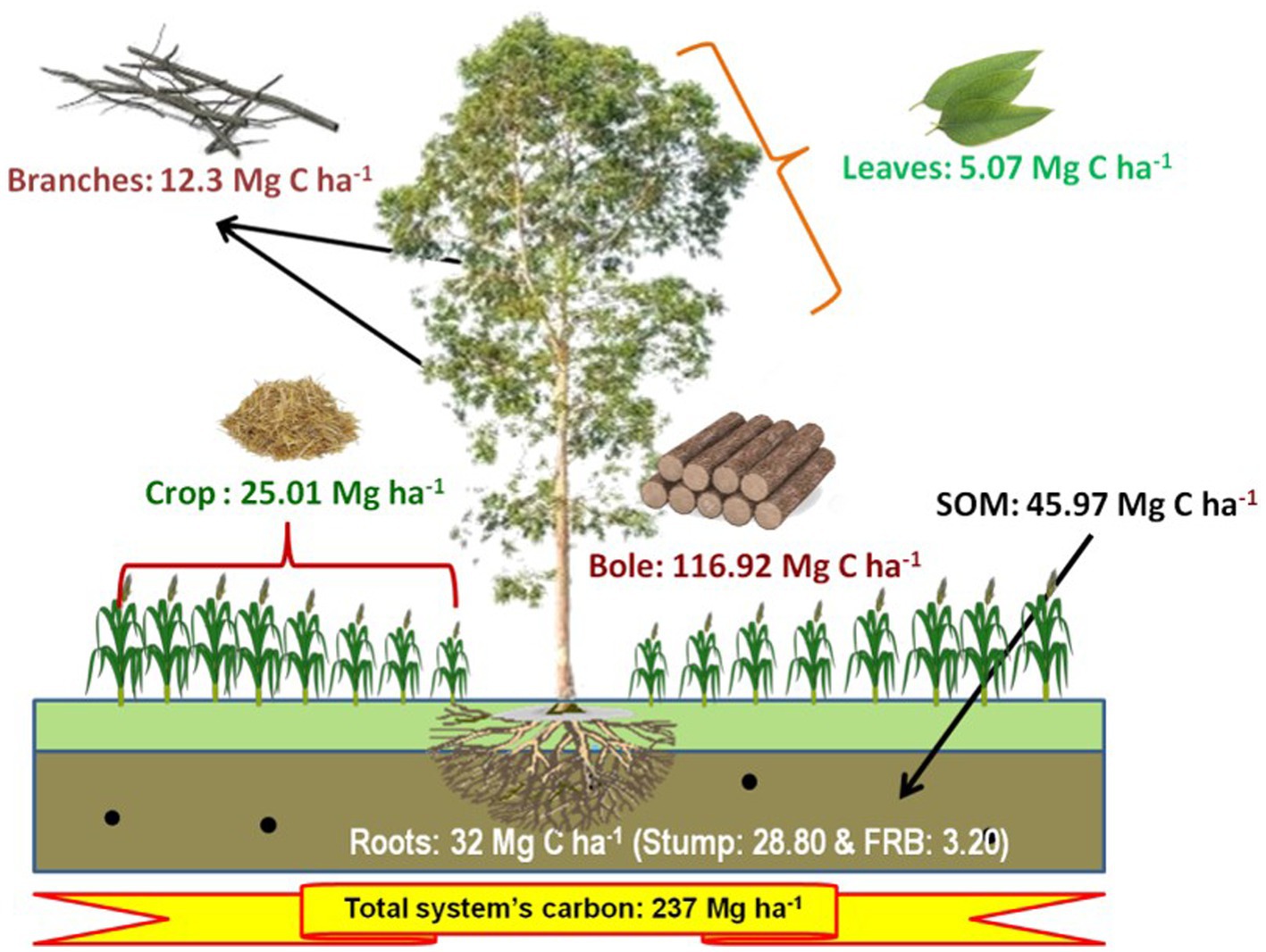
Figure 8. Total carbon stock (Mg ha−1) of dhaincha–barley crop rotation in eucalyptus-based agroforestry system (3 × 3 m) over a rotation of 8 years.
Du et al. (2015) reported similar findings in a eucalyptus-based cropping system in China. They found a total carbon content of 156 Mg C ha−1, with aboveground and belowground components (understory, root, and soil carbon) contributing 43.1 and 59.9%, respectively, in trees aged 6–7 years. The understory (shrub and herbaceous material), litter, and fine roots individually comprised <2% of the total carbon, while the soil contained between 53 and 94% of the total carbon. Zhou et al. (2017) highlighted the significant carbon sequestration potential of eucalyptus, noting that the total ecosystem carbon pool varied between 167.66 and 234.04 Mg C ha−1 in eucalyptus stands aged 7–10 years. Additionally, Luna et al. (2016) reported aboveground carbon stocks ranging from 14.38 to 408.97 Mg C ha−1 in eucalyptus plantations with a 3 × 3 m spacing in Hoshiarpur, Punjab. Consequently, the reported carbon stock values, encompassing contributions from trees, crops, and soil, ranging from 95.20 to 237.27 Mg C ha−1, align closely with findings from other studies conducted in India and abroad.
Therefore, the integration of eucalyptus in annual crops increased the carbon stock of sole cropping by 2.8 times in block planting (3 × 3 m, 6 m × 1.5 m, and 17 × 1 × 1 m) and 1.5 times in boundary plantation after 8 years of age. These results further indicate that, owing to the fast growth and adaptability of eucalyptus, it can produce adequate quantity of biomass carbon rapidly. Moreover, eucalyptus is a fast-growing tree species with high potential of biomass carbon sequestration than poplar.
3.5 Economics of eucalyptus-based agroforestry system
Eucalyptus is the most preferred species under agroforestry plantations in India due to the assured market, highly lucrative returns from trees, and supportive government policies, attracting farmers in a big way (Ramesh et al., 2023; Thumbar et al., 2023). The cost of cultivation under different planting geometries varied from Rs. 425,001 ha−1 (boundary plantation) to Rs. 548,231 ha−1 (17 × 1 × 1 m). The returns from agroforestry systems during the first year were negative due to higher initial investment costs (Table 9). A similar finding was also reported by Prasad et al. (2010) for eucalyptus and Singh and Mavi (2016) for poplar. A discount rate of 12% was used to calculate the economic analysis of different criteria. A higher gross return (Rs. 11,487,06 ha−1) was obtained for 17 × 1 × 1 m (paired row spacing), followed by 3 × 3 m (Rs. 936,295 ha−1). Out of total returns, eucalyptus trees contributed higher percentage, which was 81, 77, and 73% in 3 m × 3 m, 17 m × 1 m × 1 m, and 6 m × 1.5 m, respectively, whereas trees in boundary plantation contributed <50% of total gross returns (Figure 9). The net income from tree-based cropping system ranged from Rs. 303,158 ha−1 to Rs. 600,475 ha−1 in block plantations, Rs. 100,838 ha−1 to Rs. 189,132 ha−1 in boundary plantations, and Rs. 37,830 ha−1 in the control (sole cropping).
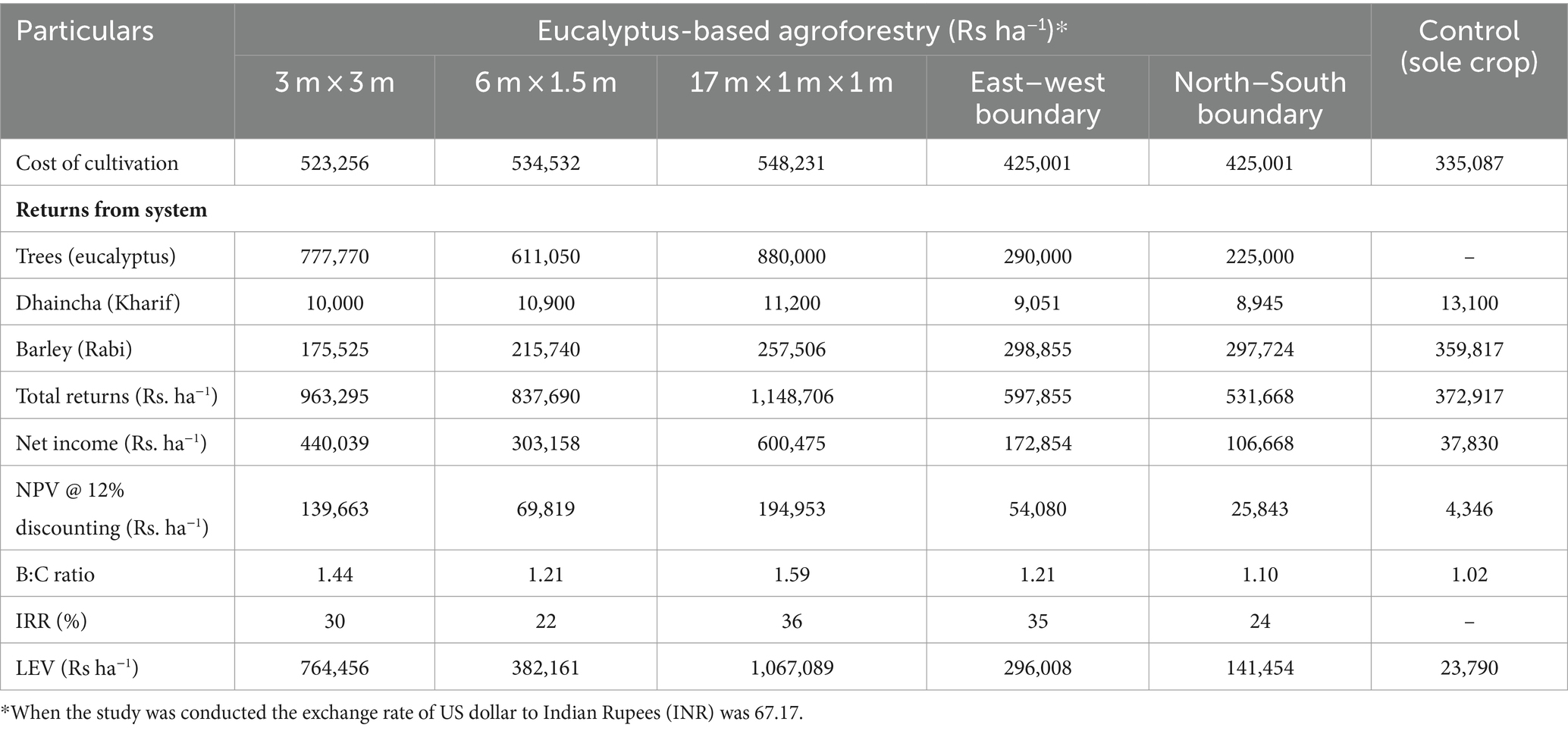
Table 9. Details of the economic evaluation of various planting geometries of eucalyptus-based cropping systems.
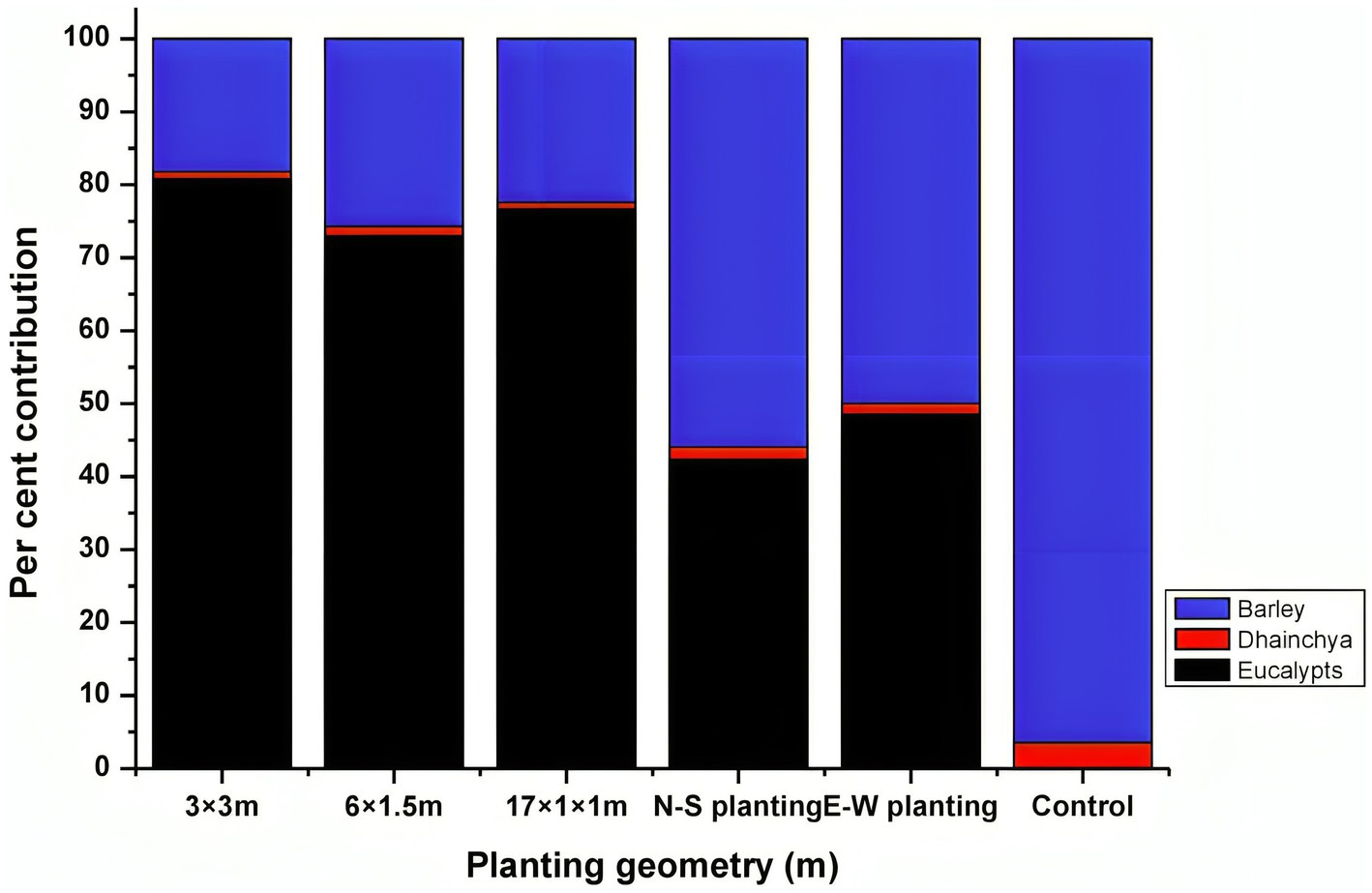
Figure 9. Percentage contribution (%) of various components of eucalyptus-based agroforestry to total gross income.
In the present study, the highest net present value (NPV) was obtained from paired row spacing (Rs. 194,953 ha−1), as more space permitted higher crop yields than other planting geometries, whereas very less (Rs. 4,346 ha−1) in the control. The mean NPV of the north–south and east–west boundary plantations were Rs. 54,080 ha−1 and Rs. 25,843 ha−1, respectively. Higher internal rate of return, IRR (36%), and B:C ratio (1.59) were recorded in paired row system with dhaincha–barley crop rotation over 8 years of rotation (Table 9). In the boundary plantation, east–west boundary plantation under dhaincha–barley crop rotation exhibited high IRR and B:C ratios of 35% and 1.21, respectively. The reduction in crop yield under different geometries was highly compensated for by trees under various cropping systems. This compensation mechanism underscores the resilience and adaptive capacity of agroforestry practices, where trees play a pivotal role in augmenting overall system productivity and sustainability.
In paired row spacing, the gross return from crops was the highest compared to other planting geometries and controls. This is because of less competition for light, nutrients, and moisture, resulting in high returns from the system. The findings of the study are in agreement with Johar’s (2017) multilayer agroforestry model of eucalyptus for 6-year rotation in north–western India. Dwivedi et al. (2016) reported similar findings in eucalyptus boundary plantations at 8 years of rotation in Saharanpur, Uttar Pradesh. In general, Singh et al. (2017) reported that farmers were unable to sacrifice meager crop yields in lieu of much higher returns from trees in block plantations at the end of rotation. The increased spacing between tree rows helps to enhance crop yield and permits cropping until harvesting. In the study, 17 × 1 × 1 m paired row spacing exhibited superior performance over other planting geometry. It further emphasizes the role of wide spacing in agroforestry systems, which is considered a crucial strategy for integrating different annual crops, minimizing the risk of crop yield loss, and addressing farmers’ concerns.
Agroforestry is recognized as a crucial tool for climate change adaptation and mitigation, aiding countries in meeting their net-zero targets. However, accurately estimating biomass and carbon sequestration in agroforestry presents practical challenges. Destructive sampling, necessary for precise field-based estimation, demands significant time, labor, and financial resources. This approach’s labor-intensive nature often limits spatial coverage to small sample plots, potentially overlooking biomass variability across the landscape. Moreover, biomass estimates can be influenced by seasonal fluctuations, challenging the accuracy of single-time sampling. Measurement techniques must be precise to avoid errors that could skew results. Additionally, variability in allometric equations used for biomass estimation introduces uncertainties. These practical difficulties underscore the complexity of agroforestry research and emphasize the need for careful planning and execution in biomass and carbon sequestration studies.
4 Conclusion
The study demonstrated the significant impact of planting geometry of eucalyptus trees on biomass and yield of annual fodder crops, thereby helping to reduce yield losses in tree-based agroforestry system. Additionally, the study reported the impact of planting geometry on carbon stock in trees, crops, and soil, with the 3 × 3 m spacing resulting in the highest carbon stock (237.27 Mg ha−1). The block and boundary plantation systems contributed differently to carbon stock, with the block system having a higher contribution from trees (66.94%) and the boundary system having a higher contribution from soil (34.51%). Integrating eucalyptus in annual crops increased carbon stock, with block planting showing a 2.8 times increase and boundary plantation showing a 1.5 times increase after 8 years of age. Paired row spacing (17 × 1 × 1 m) in eucalyptus agroforestry systems had higher crop yields and returns (Rs 600,475 ha−1), highlighting the importance of wide spacing for system productivity and sustainability. However, further research is needed to evaluate the long-term sustainability of these systems and their potential to mitigate climate change. Overall, the study provided valuable insights into the promising role of eucalyptus-based agroforestry systems, offering a pathway toward enhanced carbon sequestration and sustainable land-use practices in agricultural landscapes.
Data availability statement
The original contributions presented in the study are included in the article/supplementary material, further inquiries can be directed to the corresponding authors.
Author contributions
SC: Formal analysis, Investigation, Methodology, Resources, Software, Supervision, Validation, Visualization, Writing – original draft, Writing – review & editing, Conceptualization. RD: Conceptualization, Investigation, Methodology, Resources, Supervision, Visualization, Writing – original draft, Writing – review & editing. CS: Conceptualization, Investigation, Methodology, Resources, Supervision, Visualization, Writing – review & editing. IS: Data curation, Funding acquisition, Writing – review & editing. AU: Conceptualization, Methodology, Software, Visualization, Writing – original draft. AK: Formal analysis, Software, Validation, Writing – review & editing. DJ: Conceptualization, Methodology, Visualization, Writing – review & editing. HH: Data curation, Writing – review & editing. AP: Visualization, Writing – review & editing. VK: Conceptualization, Methodology, Resources, Writing – review & editing. AM: Formal analysis, Software, Validation, Writing – review & editing. AC: Formal analysis, Software, Validation, Writing – review & editing. GR: Visualization, Writing – review & editing. MO: Data curation, Funding acquisition, Writing – review & editing. IA: Data curation, Funding acquisition, Writing – review & editing. HA: Data curation, Funding acquisition, Writing – review & editing. SF: Funding acquisition, Visualization, Writing – review & editing. SN: Visualization, Writing – review & editing. RS: Writing – review & editing.
Funding
The author(s) declare that financial support was received for the research, authorship, and/or publication of this article. The authors extend their appreciation to the researchers supporting project number (RSP2024R176) at King Saud University, Riyadh, Saudi Arabia.
Acknowledgments
The authors extend their appreciation for the financial support provided by the Vice Deanship of Research Chairs at King Saud University. The authors extend their appreciation to the researchers supporting project number (RSP2024R176) at King Saud University, Riyadh, Saudi Arabia. The authors are highly grateful to the Department of Forestry, CCS Haryana Agricultural University, and Director, ICAR-Central Agroforestry Research Institute, Jhansi, for the necessary arrangements to carry out the research. The authors are also grateful to Indian council of Agricultural Research, New Delhi for supporting this research work.
Conflict of interest
The authors declare that the research was conducted in the absence of any commercial or financial relationships that could be construed as a potential conflict of interest.
The reviewer SR declared a shared affiliation with the author(s) SC, AU, AK, DJ, HH, AP, VK, AM, AC, and GR to the handling editor at the time of review.
Publisher’s note
All claims expressed in this article are solely those of the authors and do not necessarily represent those of their affiliated organizations, or those of the publisher, the editors and the reviewers. Any product that may be evaluated in this article, or claim that may be made by its manufacturer, is not guaranteed or endorsed by the publisher.
References
Ahmed, W. (2004). Effect of Eucalyptus camaldulensis on the yield of wheat and maize cropsafter reducing tree density. Pak J. Agric. Sci. 41, 62–64,
Ajit, D., Das, D. K., Chaturvedi, O. P., Jabeen, N., and Dhyani, S. K. (2011). Predictive models for dry weight estimation of above and below ground biomass components of Populus deltoides in India: development and comparative diagnosis. Biomass Bioenergy 35, 1145–1152. doi: 10.1016/j.biombioe.2010.12.001
Ajit, D. S. K., Handa, A. K., Newaj, R., Chavan, S. B., Alam, B., Prasad, R., et al. (2016). Estimating carbon sequestration potential of existing agroforestry systems in India. Agr. Syst. 90, 1101–1118. doi: 10.1007/s10457-016-9986-z
Ajit Rai, P., Handa, A. K., Choudhari, S., and Uma, R. (2006). Allometry for estimating above ground biomass of Eucalyptus tereticornis under energy and boundary plantations in Central India. Ann. Arid Zone 45, 175–182,
Ajit, N. J. (2008). Tree Growth Modelling: Indian Experiences. Available at: http://sscnars.icar.gov.in/Agro/1-Tree%20Growth%20Modelling-Statistical-Concepts.pdf (Accessed 30 June 2023).
Albrecht, A., and Kandji, S. T. (2003). Carbon sequestration in tropical agroforestry systems. Agric. Ecosyst. Environ. 99, 15–27. doi: 10.1016/S0167-8809(03)00138-5
Anbessa, G. N., and Utaile, Y. U. (2024). Scattered trees in smallholder farms improve soil properties and litter decomposition in humid-agroecosystems in Ethiopia. Agr. Syst. 1–13. doi: 10.1007/s10457-024-00982-z
Aryal, D. R., Morales-Ruiz, D. E., López-Cruz, S., Tondopó-Marroquín, C. N., Lara-Nucamendi, A., Jiménez-Trujillo, J. A., et al. (2022). Silvopastoral systems and remnant forests enhance carbon storage in livestock-dominated landscapes in Mexico. Sci. Rep. 12:16769. doi: 10.1038/s41598-022-21089-4
Babu, S., Mohapatra, K. P., Yadav, G. S., Lal, R., Singh, R., Avasthe, R. K., et al. (2020). Soil carbon dynamics in diverse organic land use systems in north eastern Himalayan ecosystem of India. Catena 194:104785. doi: 10.1016/j.catena.2020.104785
Bastien-Henri, S., Park, A., Ashton, M., and Messier, C. (2010). Biomass distribution among tropical tree species grown under differing regional climates. For. Ecol. Manage. 260, 403–410. doi: 10.1016/j.foreco.2010.04.035
Beets, P. N., and Garrett, L. G. (2018). Carbon fraction of Pinus radiata biomass components within New Zealand. N. Z. J. For. Sci. 48:5. doi: 10.1186/s40490-018-0119-5
Bernardo, A. L., Reis, M. G. F., Reis, G. G., Harrison, R. B., and Firme, D. J. (1998). Effect of spacing on growth and biomass distribution in Eucalyptus camaldulensis, E. Pellita and E. Urophylla plantations in southeastern Brazil. For. Ecol. Manage. 104, 1–13. doi: 10.1016/S0378-1127(97)00199-0
Bhardwaj, K. K., Dhillon, R. S., Kumari, S., Johar, V., Dalal, V., and Chavan, S. B. (2017). Effect of eucalyptus bund plantation on yield of agricultural crops and soil properties in semi-arid region of India. Int. J. Curr. Microbiol. App. Sci. 6, 2059–2065. doi: 10.20546/ijcmas.2017.610.245
Binkley, D., Stape, J. L., and Ryan, M. G. (2004). Thinking about efficiency of resource use in forests. For. Ecol. Manage. 193, 5–16. doi: 10.1016/j.foreco.2004.01.019
Bray, R. H., and Kurtz, L. T. (1945). Determination of total, organic, and available forms of phosphorus in soils. Soil Sci. 59, 39–46. doi: 10.1097/00010694-194501000-00006
Chaudhari, S. K., Kumar, P., Mishra, A. K., Singh, K., Rai, P., Singh, R., et al. (2015). Labile carbon fractions build-up and dynamics under vertical stratification of Populus deltoides and Eucalyptus tereticornis based agroforestry systems in trans-Gangetic Plains of India. Ann. Agric. Res. New Series. 6, 1–9.
Chavan, S. B., and Dhillon, R. S. (2019). Doubling farmers’ income through Populus deltoides-based agroforestry systems in northwestern India. Current Science. 117, 219–226.
Chavan, S. B., Dhillon, R. S., Ajit, R., Rizvi, R. H., Sirohi, C., Handa, A. K., et al. (2022a). Estimating biomass production and carbon sequestration of poplar-based agroforestry systems in India. Environ. Dev. Sustain. 24, 13493–13521. doi: 10.1007/s10668-021-01996-8
Chavan, S. B., Dhillon, R. S., Sirohi, C., Keerthika, A., Kumari, S., Bharadwaj, K. K., et al. (2022b). Enhancing farm income through boundary plantation of poplar (Populus deltoides): an economic analysis. Sustain. For. 14:8663. doi: 10.3390/su14148663
Chavan, S. B., Dhillon, R. S., Sirohi, C., Uthappa, A. R., Jinger, D., Jatav, H. S., et al. (2023). Carbon sequestration potential of commercial agroforestry Systems in Indo-Gangetic Plains of India: poplar and Eucalyptus-based agroforestry systems. Forests 14:559. doi: 10.3390/f14030559
Chave, J., Davies, S. J., Phillips, O. L., Lewis, S. L., Sist, P., Schepaschenko, D., et al. (2019). Ground data are essential for biomass remote sensing missions. Surv. Geophys. 40, 863–880. doi: 10.1007/s10712-019-09528-w
Chave, J., Réjou-Méchain, M., Búrquez, A., Chidumayo, E., Colgan, M. S., Delitti, W. B., et al. (2014). Improved allometric models to estimate the aboveground biomass of tropical trees. Glob. Chang. Biol. 20, 3177–3190. doi: 10.1111/gcb.12629
Chave, J., Riéra, B., and Dubois, M. A. (2001). Estimation of biomass in a neotropical forest of French Guiana: spatial and temporal variability. J. Trop. Ecol. 17, 79–96. doi: 10.1017/S0266467401001055
Cresswell, H. P., and Hamilton, G. J. (2002). “Particle size analysis” in Soil Physical Measrement and Interpretation for Land Evalution, eds. N. McKenzie, K. Coughlan, and H. Cresswell (Collingwood, Victoria: CSIRO Publishing) 224–239.
de Gier, A. (2003). A new approach to woody biomass assessment in woodlands and shrublands. Geoinformatics Trop. Ecosyst., 161–198. Available at: https://www.cabidigitallibrary.org/doi/full/10.5555/20053032584
De Oliveira, I. R., Chagas, M. P., Bouillet, J.-P., Longui, E. L., De Lima, I. L., Bordron, B., et al. (2018). Effect of tree spacing on growth and wood density of 38-year-old Cariniana legalis trees in Brazil. South. For 80, 311–318. doi: 10.2989/20702620.2017.1393741
Deo, R. K. (2008). Modelling and mapping of above-ground biomass and carbon sequestration in the cool temperate forest of north-East China. The Netherlands: ITC Enschede.
Deshmukh, H., Dobriyal, M., Tandel, M. B., Gunaga, R., Sharma, O. P., Garde, Y. A., et al. (2023). Development and standardization of an innovative scale for measuring the socio-economic status of agroforestry farmers in South Gujarat, India. Sustainability 15:2691. doi: 10.3390/su15032691
Dhanda, R. S., and Singh, R. P. (1990). Volume and biomass tables for Eucalyptus hybrid (E. tereticornis Sm) from Kandi area of Punjab. J. Res. PAU 27, 428–433,
Dhillon, R. S., Chavan, S. B., Bangarwa, K. S., Bharadwaj, K. K., Kumari, S., and Sirohi, C. (2018). Eucalyptus-based agroforestry system under semi-arid condition in North-Western India: an economic analysis. Indian J. Ecol. 45, 470–474,
Dhillon, G. S., Grewal, S. S., and Atwal, A. S. (1979). Developing Agri-silvicultural practices. 1. Effect of farm trees (eucalyptus) on the adjoining crops. J. Ecol. 6, 88–97.
Dhillon, G. S., Singh, S., Dhillon, M. S., and Atwal, A. S. (1982). Developing Agri-silvicultural practices: studies on the shading effect of Eucalyptus on the yield of adjoining crops. Indian J. Ecol. 6, 228–236,
Dhyani, S. K., Ram, A., and Dev, I. (2016). Potential of agroforestry systems in carbon sequestration in India. Indian J. Agric. Sci. 86, 1103–1112. doi: 10.56093/ijas.v86i9.61348
Dhyani, S. K., and Tripathi, R. S. (1998). Tree growth and crop yield under agrisilvicultural practices in north-East India. Agr. Syst. 44, 1–12. doi: 10.1023/A:1006176303162
Du, H., Zeng, F., Peng, W., Wang, K., Zhang, H., Liu, L., et al. (2015). Carbon storage in a Eucalyptus plantation chronosequence in southern China. Forests 6, 1763–1778. doi: 10.3390/f6061763
Dwivedi, R. P., Kareemulla, K., Singh, R., Rizvi, R. H., and Chauhan, J. (2016). Socio-economic analysis of agroforestry systems in Western Uttar Pradesh. Indian Res. J. Ext. Educ. 7, 18–22,
Eamus, D., Burrows, W., and McGuinness, K. (2000). Review of allometric relationships for estimating woody biomass for Queensland, the Northern Territory and Western Australia. Australian Greenhouse Office. Australia: National Carbon Accounting System technical report.
Fahad, S., Chavan, S. B., Chichaghare, A. R., Uthappa, A. R., Kumar, M., Kakade, V., et al. (2022). Agroforestry systems for soil health improvement and maintenance. Sustain. For. 14:14877. doi: 10.3390/su142214877
Food, A. N. (2010). Global Forest resources assessment 2010: Main report. United Nations: Food Agric. Organ.
Forrester, D. I., Wiedemann, J. C., Forrester, R. I., and Baker, T. G. (2013). Effects of planting density and site quality on mean tree size and total stand growth of Eucalyptus globulus plantations. Can. J. For. Res. 43, 846–851. doi: 10.1139/cjfr-2013-0137
Garrity, D. (1994). Reforestation through agroforestry: market driven small-holder timber production on the frontier. Mark. Multipurp. Tree Prod. Asia, 265–268,
George, M. (1982). Litter production and nutrient return in Eucalyptus hybrid plantations. Indian For. 108, 252–260,
Gill, R. I. S., Singh, B., Kaur, N., and Sangha, K. S. (2016). Agroforestry-A viable option for crop diversification in Punjab. Punjab, India: Department of Forests and Wildlife Preservation. 1–22.
Guo, Z., Zhang, Y., Deegen, P., and Uibrig, H. (2006). Economic analyses of rubber and tea plantations and rubber-tea intercropping in Hainan, China. Agr. Syst. 66, 117–127. doi: 10.1007/s10457-005-4676-2
Gupta, M. K., and Sharma, S. D. (2008). Effect of tree plantation on soil properties, profile morphology and productivity index I Poplar in Uttarakhand. Ann. For. 17, 43–70,
Halli, H. M., Govindasamy, P., Choudhary, M., Srinivasan, R., Prasad, M., Wasnik, V. K., et al. (2022). Range grasses to improve soil properties, carbon sustainability, and fodder security in degraded lands of semi-arid regions. Sci. Total Environ. 851:158211. doi: 10.1016/j.scitotenv.2022.158211
Hong, S., Piao, S., Chen, A., Liu, Y., Liu, L., Peng, S., et al. (2018). Afforestation neutralizes soil pH. Nat. Commun. 9, 1–7. doi: 10.1038/s41467-018-02970-1
Houghton, R. A., and Nassikas, A. A. (2017). Global and regional fluxes of carbon from land use and land cover change 1850–2015. Global Biogeochem. Cycles 31, 456–472. doi: 10.1002/2016GB005546
IPCC (2021). “Summary for Policymakers” in Climate Change: The Physical Science Basis. Contribution of Working Group I to the Sixth Assessment Report of the Intergovernmental Panel on Climate Change. eds. V. Masson-Delmotte, P. Zhai, A. Pirani, S. L. Connors, C. Péan, and S. Berger Cambridge University Press. (In press).
Jackson, M. L. (2005). Soil chemical analysis: Advanced course. USA: UW-Madison Libraries parallel press.
Jhariya, M. K., Meena, R. S., Banerjee, A., Kumar, S., and Raj, A. (2024). Agroforestry for carbon and ecosystem management: an overview. Agrofor Carbon Ecosyst Manage, 3–16. doi: 10.1016/B978-0-323-95393-1.00031-2
Jhariya, M. K., Meena, R. S., Banerjee, A., and Meena, S. N. (2021). Natural resources conservation and advances for sustainability. New Delhi, India: Elsevier, Academic Press.
Jinger, D., Kaushal, R., Kumar, R., Paramesh, V., Verma, A., Shukla, M., et al. (2023). Degraded land rehabilitation through agroforestry in India: achievements, current understanding, and future prospectives. Front. Ecol. Evol. 11:1088796. doi: 10.3389/fevo.2023.1088796
Jinger, D., Kumar, R., Kakade, V., Dinesh, D., Singh, G., Pande, V. C., et al. (2022). Agroforestry system for controlling soil erosion and enhancing system productivity in ravine lands of Western India under climate change scenarios. Environ. Monit. Assess. 194:267. doi: 10.1007/s10661-022-09910-z
Johar, V. (2017). Effect of nitrogen levels on wheat varieties under Agri-silvi-horticultural system. PhD diss., Forestry, CCSHAU, Hisar, India
Juhari, A. (2017). Eucalyptus plantation: Socio-economic and environmental impact. New Delhi, India: Indian Paper Manufacturers Association.
Keerthika, A., Parthiban, K. T., Chavan, S. B., Shukla, A. K., Gupta, D. K., and Venkatesh, V. (2024). Leaf litter decomposition in different tree species of multifunctional agroforestry: Decay constant and initial litter chemistry : Environment, Development and Sustainability, 1–23.
Khan, G. S., and Chaudhry, A. K. (2007). Effect of spacing and plant density on the growth of poplar (Populus deltoides) trees under agro-forestry system. Pak. J. Agri. Sci 44, 321–327.
Kidanu, S., Mamo, T., and Stroosnijder, L. (2005). Biomass production of eucalyptus boundary plantations and their effect on crop productivity on Ethiopian highland Vertisols. Agrofor Syst. 63:281290.
Kim, D.-G., Kirschbaum, M. U. F., and Beedy, T. L. (2016). Carbon sequestration and net emissions of CH4 and N2O under agroforestry: synthesizing available data and suggestions for future studies. Agric. Ecosyst. Environ. 226, 65–78. doi: 10.1016/j.agee.2016.04.011
Klemperer, W. D. (2003). Forest resource economics and finance. Blacksburg, VA, USA: Tech Bookstore, 1–551.
Kumar, A., Kumar, M., Nandal, D. P. S., and Kaushik, N. (2013). Performance of wheat and mustard under Eucalyptus tereticornis based agrisilviculture system. Range Manag. Agrofor. 34, 192–195,
Kumar, P., Mishra, A. K., Chaudhari, S. K., Basak, N., Rai, P., Singh, K., et al. (2018). Carbon pools and nutrient dynamics under Eucalyptus-based agroforestry system in semi-arid region of north-West India. J. Indian Soc. Soil Sci 66, 188–199. doi: 10.5958/0974-0228.2018.00024.5
Kumar, P., Uthappa, A. R., Chavan, S. B., Chichaghare, A. R., Debta, H., Bhat, S., et al. (2023). “Achieving biodiversity conservation, livelihood security and sustainable development goals through agroforestry in coastal and island regions of India and Southeast Asia” in Agroforestry for sustainable intensification of agriculture in Asia and Africa (Springer Nature Singapore: Singapore), 429–486.
Kuyah, S., Dietz, J., Muthuri, C., Jamnadass, R., Mwangi, P., Coe, R., et al. (2012). Allometric equations for estimating biomass in agricultural landscapes: II Belowground biomass. Agric. Ecosyst. Environ. 158, 225–234. doi: 10.1016/j.agee.2012.05.010
Kuyah, S., Dietz, J., Muthuri, C., van Noordwijk, M., and Neufeldt, H. (2013). Allometry and partitioning of above-and below-ground biomass in farmed eucalyptus species dominant in Western Kenyan agricultural landscapes. Biomass Bioenergy 55, 276–284. doi: 10.1016/j.biombioe.2013.02.011
Kuyah, S., Mbow, C., Sileshi, G. W., van Noordwijk, M., Tully, K. L., and Rosenstock, T. S. (2016). Quantifying tree biomass carbon stocks and fluxes in agricultural landscapes. Methods Meas. Greenh. Gas Balanc. Eval. Mitig. Options Smallhold. Agric., 119–134. doi: 10.1007/978-3-319-29794-1_6
Lal, R. (2005). Forest soils and carbon sequestration. For. Ecol. Manage. 220, 242–258. doi: 10.1016/j.foreco.2005.08.015
Lorenz, K., and Lal, R. (2014). Biochar application to soil for climate change mitigation by soil organic carbon sequestration. J. Plant Nutr. Soil Sci. 177, 651–670. doi: 10.1002/jpln.201400058
Luna, R. K., Thakur, N. S., Gunaga, R. P., and Kumar, V. (2016). Biomass, carbon stock and carbon dioxide removal across different girth classes of Eucalyptus species in Punjab: implication for Eucalyptus plantations. J. Tree Sci. 35, 13–20,
Luna, R. K., Thakur, N. S., and Kumar, V. (2014). Prediction models for biomass, volume, carbon stock and carbon dioxide removal for Eucalyptus hybrid plantations in Punjab. J. Tree Sci. 33, 49–53,
Luna, R. K., Thakur, N. S., and Vijay, K. (2009). Performance of clonal Eucalyptus in different agroclimatic zones of Punjab, India. Indian For. 135, 1455–1464,
Lynch, J. (2019). Availability of disaggregated greenhouse gas emissions from beef cattle production: A systematic review. Environ. Impact Assess. Rev. 76, 69–78. doi: 10.1016/j.eiar.2019.02.003
Masson-Delmotte, V., Zhai, P., Pirani, A., Connors, S. L., Péan, C., Berger, S., et al. (2021). Climate change 2021: The physical science basis. The Working Group I contribution to the Sixth Assessment Report addresses the most up-to-date physical understanding of the climate system and climate change, bringing together the latest advances in climate science. 2.
Nair, P. R., Nair, V. D., Kumar, B. M., and Showalter, J. M. (2010). Carbon sequestration in agroforestry systems. Adv. Agron. 108, 237–307. doi: 10.1016/S0065-2113(10)08005-3
Newaj, R., Dhyani, S. K., Chavan, S. B., Rizvi, R. H., and Prasad, R. (2014). Methodologies for assessing biomass, carbon stock and carbon sequestration in agroforestry systems. Jhansi, India: National Research Centre for agroforestry.
Nyaga, J., Muthuri, C. W., Barrios, E., Öborn, I., and Sinclair, F. L. (2019). Enhancing maize productivity in agroforestry systems through managing competition: lessons from smallholders’ farms, rift valley, Kenya. Agr. Syst. 93, 715–730. doi: 10.1007/s10457-017-0169-3
Ounban, W., Puangchit, L., and Diloksumpun, S. (2016). Development of general biomass allometric equations for Tectona grandis Linn. f. and Eucalyptus camaldulensis Dehnh. Plantations in Thailand. Agric. Nat. Resour. 50, 48–53. doi: 10.1016/j.anres.2015.08.001
Pande, M. C., Tandon, V. N., and Rawat, H. S. (1987). Organic matter production and distribution of nutrients in Eucalyptus hybrid plantation ecosystems in Karnataka. Indian For. 114, 713–724,
Pandey, D. N. (2002). Carbon sequestration in agroforestry systems. Clim. Pol. 2, 367–377. doi: 10.3763/cpol.2002.0240
Panse, V. G., and Sukhatme, P. V. (1954). Statistical methods for agricultural workers. 4th Edn. New Delhi, India: ICAR Publication.
Pathak, H., Srinivasarao, C. H., and Jat, M. L. (2021). Conservation agriculture for climate change adaptation and mitigation in India. J Agric Phys 21, 182–196,
Prasad, J., Korwar, G. R., Rao, K. V., Mandal, U. K., Rao, C. A. R., Rao, G. R., et al. (2010). Tree row spacing affected agronomic and economic performance of Eucalyptus-based agroforestry in Andhra Pradesh, southern India. Agr. Syst. 78, 253–267. doi: 10.1007/s10457-009-9275-1
Prasad, J., Srinivas, K., Rao, C. S., Ramesh, C., Venkatravamma, K., and Venkateswarlu, B. (2012). Biomass productivity and carbon stocks of farm forestry and agroforestry systems of leucaena and eucalyptus in Andhra Pradesh, India. Curr. Sci. 103, 536–540,
Ramesh, K. R., Deshmukh, H. K., Sivakumar, K., Guleria, V., Umedsinh, R. D., Krishnakumar, N., et al. (2023). Influence of eucalyptus agroforestry on crop yields, soil properties, and system economics in southern regions of India. Sustain. For. 15:3797. doi: 10.3390/su15043797
Rathore, A. C., Singh, C., Jayaprakash, J., Gupta, A. K., Doharey, V. K., Jinger, D., et al. (2023). Impact of conservation practices on soil quality and ecosystem services under diverse horticulture land use system. Front. For. Glob. Change 6:1289325. doi: 10.3389/ffgc.2023.1289325
Ribeiro, S. C., Soares, C. P. B., Fehrmann, L., Jacovine, L. A. G., and von Gadow, K. (2015). Biomassa acima e abaixo do solo e estimativas de carbono para um plantio clonal de eucalipto no sudeste do brasiL. Rev. Árvore 39, 353–363. doi: 10.1590/0100-67622015000200015
Rojo Alboreca, A., García Villabrille, J. D., and Pérez Rodríguez, F. (2015). EucaTool®, a cloud computing application for estimating the growth and production of Eucalyptus globulus Labill. plantations in Galicia (NW Spain). For Syst 24:06. doi: 10.5424/fs/2015243-07865
Salunkhe, S., Nandgude, S., Tiwari, M., Bhange, H., and Chavan, S. B. (2023). Land suitability planning for sustainable mango production in vulnerable region using geospatial multi-criteria decision model. Sustain. For. 15:2619. doi: 10.3390/su15032619
Sheoran, O. P., Tonk, D. S., Kaushik, L. S., Hasija, R. C., and Pannu, R. S. (1998). “Statistical software package for agricultural research workers” in Recent advances in information theory, statistics & computer applications. eds. D. S. Hooda and R. C. Hasija (CCS HAU, Hisar: Department of Mathematics Statistics), 139–143.
Singh, R. P. (1975). Studies of annual net production, mineral standing state and PhD thesis, Meerut University. Energy accumulation pattern of Eucalyptus tereticornis Smith in Western Uttar Pradesh.
Singh, D., and Kohli, R. K. (1992). Impact of Eucalyptus tereticornis Sm. Shelterbelts on crops. Agr. Syst. 20, 253–266. doi: 10.1007/BF00053143
Singh, H., and Mavi, H. K. (2016). Economic analysis of poplar based agroforestry system under riparian wet land conditions of Punjab. Indian J. Econ. Dev. 12, 191–196. doi: 10.5958/2322-0430.2016.00023.8
Singh, K. P., Singh, B., Jhariya, M. K., Khemraj, R., Banerjee, A., and Thakur, D. S. (2023b). Soil properties and carbon dynamics under coffee-based agroforestry system in Bastar region of Chhattisgarh, India. Environ Dev Sustain 26, 13411–13428. doi: 10.1007/s10668-023-04230-9
Singh, K. P., Singh, B., Patil, S. K., Rahangdale, C. P., Banerjee, A., Shukla, R., et al. (2023a). Biomass, carbon stock, CO2 mitigation and carbon credits of coffee-based multitier cropping model in Central India. Environ. Monit. Assess. 195:1250. doi: 10.1007/s10661-023-11892-5
Singh, M., Sridhar, K. B., Kumar, D., Uthappa, A. R., Dwivedi, R. P., Dev, I., et al. (2017). Doubling farmers’ income through agroforestry in North-Western India: Apolicy perspective. Indian J. Agrofor. 19, 90–95,
Sirohi, C., Dhillon, R. S., Chavan, S. B., Handa, A. K., Balyan, P., Bhardwaj, K. K., et al. (2022). Development of poplar-based alley crop system for fodder production and soil improvements in semi-arid tropics. Agr. Syst. 96, 731–745. doi: 10.1007/s10457-022-00735-w
Snowdon, P., Raison, J., Keith, H., Ritson, P., Grierson, P., Adams, M., et al., (2002). Protocol for sampling tree and stand biomass. National carbon accounting system technical report no. 31. Australian Greenhouse Office, Canberra.
Stewart, B. A. (1993). Managing crop residues for the retention of carbon. Water Air Soil Pollut. 70, 373–380. doi: 10.1007/BF01105008
Subbiah, B. V., and Asija, G. L. (1956). A rapid method for the estimation of nitrogen in soil. Curr. Sci. 26, 259–260,
Sultan, M. T., Mahmud, U., and Khan, M. Z. (2023). Addressing soil salinity for sustainable agriculture and food security: innovations and challenges in coastal regions of Bangladesh. Fut Foods 8:100260. doi: 10.1016/j.fufo.2023.100260
Tandon, V. N., Pande, M. C., and Singh, R. (1988). Biomass estimation and distribution of nutrients in five different aged Eucalyptus grandis plantation ecosystems in Kerala state. Indian For. 114, 184–199,
Tewari, V. P. (2016). Volume and biomass functions for trees grown under arid conditions in India. Indian For. 142, 23–30,
Thumbar, P. D., Behera, L. K., Gunaga, R. P., Mehta, A. A., Huse, S. A., Dholariya, C. A., et al. (2023). Eucalyptus based agroforestry Systems for Wood Production and Higher Economic Return. E-J Appl For Ecology 11, 17–22,
Tian, G., Kang, B. T., Kolawole, G. O., Idinoba, P., and Salako, F. K. (2005). Long-term effects of fallow systems and lengths on crop production and soil fertility maintenance in West Africa. Nutr. Cycl. Agroecosyst. 71, 139–150. doi: 10.1007/s10705-004-1927-y
Toky, O. P., Kumar, P., and Khosla, P. K. (1989). Structure and function of traditional agroforestry systems in the western Himalaya II. Nutrient cycling. Agr. Syst. 9, 71–89. doi: 10.1007/BF00120156
Uthappa, A. R., Bana, O. P. S., Kumar, M., and Kanwal, M. (2015). Soil physico-bio-chemical properties as influenced by varying tree densities in poplar (Populus deltoides Bartr. Ex marsh.) based agroforestry system. Indian J. Agrofor. 17, 81–90,
Uthappa, A. R., Devakumar, A. S., das, B., Mahajan, G. R., Chavan, S. B., Jinger, D., et al. (2024). Comparative analysis of soil quality indexing techniques for various tree based land use systems in semi-arid India. Front For Glob Change. 6:1322660. doi: 10.3389/ffgc.2023.1322660
Walkley, A., and Black, I. A. (1934). An examination of the Degtjareff method for determining soil organic matter, and a proposed modification of the chromic acid titration method. Soil Sci. 37, 29–38. doi: 10.1097/00010694-193401000-00003
Watson, R. T., Noble, I. R., Bolin, B., Ravindranath, N. H., Verardo, D. J., and Dokken, D. J. (2000). Land use, land-use change and forestry: A special report of the intergovernmental panel on climate change. Washington, DC, USA: Cambridge University Press.
Yadav, G. S., Kandpal, B. K., Das, A., Babu, S., Mohapatra, K. P., Devi, A. G., et al. (2021). Impact of 28 year old agroforestry systems on soil carbon dynamics in eastern Himalayas. J. Environ. Manage. 283:111978. doi: 10.1016/j.jenvman.2021.111978
Yadava, A. K. (2010). Biomass production and carbon sequestration in different agroforestry systems in Tarai region of central Himalaya. Ind For. 136, 234–244,
Yue, C., Ciais, P., Houghton, R. A., and Nassikas, A. A. (2020). Contribution of land use to the interannual variability of the land carbon cycle. Nat. Commun. 11:3170. doi: 10.1038/s41467-020-16953-8
Zewdie, M., Olsson, M., and Verwijst, T. (2009). Above-ground biomass production and allometric relations of Eucalyptus globulus Labill. coppice plantations along a chronosequence in the central highlands of Ethiopia. Biomass Bioenergy 33, 421–428. doi: 10.1016/j.biombioe.2008.08.007
Zhou, X., Wen, Y., Goodale, U. M., Zuo, H., Zhu, H., Li, X., et al. (2017). Optimal rotation length for carbon sequestration in Eucalyptus plantations in subtropical China. New For. 48, 609–627. doi: 10.1007/s11056-017-9588-2
Keywords: biomass equations, validation, destructive sampling, agroforestry, biomass productivity, carbon stock
Citation: Chavan SB, Dhillon RS, Sirohi C, Saleh IA, Uthappa AR, Keerthika A, Jinger D, Halli HM, Pradhan A, Kakade V, Morade A, Chichaghare AR, Rawale GB, Okla MK, Alaraidh IA, AbdElgawad H, Fahad S, Nandgude S and Singh R (2024) Optimizing planting geometries in eucalyptus-based food production systems for enhanced yield and carbon sequestration. Front. Sustain. Food Syst. 8:1386035. doi: 10.3389/fsufs.2024.1386035
Edited by:
Prabhu Govindasamy, National Research Centre for Banana (ICAR), IndiaReviewed by:
Sanjay Singh Rathore, Indian Agricultural Research Institute (ICAR), IndiaSyam Viswanath, Kerala Forest Research Institute, India
Manoj Kumar Jhariya, Sant Gahira Guru Vishwavidyalaya, India
Copyright © 2024 Chavan, Dhillon, Sirohi, Saleh, Uthappa, Keerthika, Jinger, Halli, Pradhan, Kakade, Morade, Chichaghare, Rawale, Okla, Alaraidh, AbdElgawad, Fahad, Nandgude and Singh. This is an open-access article distributed under the terms of the Creative Commons Attribution License (CC BY). The use, distribution or reproduction in other forums is permitted, provided the original author(s) and the copyright owner(s) are credited and that the original publication in this journal is cited, in accordance with accepted academic practice. No use, distribution or reproduction is permitted which does not comply with these terms.
*Correspondence: A. R. Uthappa, YXJ1dGhhcHBhQGdtYWlsLmNvbQ==; Shah Fahad, c2hhaGZhaGFkQGF3a3VtLmVkdS5waw==