- 1ICAR Research Complex for Eastern Region, Patna, Bihar, India
- 2ICAR Indian Institute of Soil & Water Conservation, Research Centre, Koraput, Odisha, India
- 3ICAR Research Complex for NEH Region, Umiam, Meghalaya, India
- 4ICAR Central Citrus Research Institute, Nagpur, Maharashtra, India
- 5ICAR Directorate of Weed Research, Jabalpur, Madhya Pradesh, India
- 6ICAR Natural Resource Management, New Delhi, India
- 7ICAR Indian Institute of Farming Systems Research, Modipuram, India
- 8ICAR Indian Agricultural Research Institute, New Delhi, India
- 9ICAR Central Institute of Agricultural Engineering, Bhopal, India
- 10KVK Aurangabad, Bihar Agricultural University, Bhagalpur, India
Integrated farming system (IFS) aims to diversify the agricultural landscapes by incorporating different components to meet the multifarious needs of the burgeoning population. The present study was undertaken to understand the impact of different cropping systems on soil organic carbon (SOC) stock, aggregate distribution, and aggregate associated organic carbon (AAOC) in 2-IFS models of varying sizes (0.4 and 0.8 ha) established during 2008–2009. After 10 years of the study, the fodder system registered the greatest TOC and carbon stocks across IFS models, with surface soil (0–15 cm) accumulating 17 and 13% higher TOC and C stock, respectively, in 0.4 and 0.8 ha models. In 0–15 cm, macroaggregates (Ma) represented the highest proportion (75–76%) in both models. Among cropping systems, the fodder system recorded the highest large macroaggregates in both IFS models. Within 0–30 cm depth, small macroaggregates are mostly found in the perennial system (fodder, guava+turmeric, and lemon intercropping system), indicating the potential to improve the aggregate stability over the seasonal (shorter duration) system. In general, micro aggregate (Mi) fraction was pre-dominant in sub-surface soil (17.35%). The maximum AAOC was found in Ma compared to Mi fractions, with approximately 67 and 63% of total carbon associated with Ma in 0.4 and 0.8 ha IFS models, respectively. Interestingly, the 0.8 ha IFS model had higher TOC (~11%) and carbon stock (~12%) than the 0.4 ha model, but AAOC did not show a similar result, indicating the influence of cropping systems on AAOC. The study indicated that the fodder-based production system had better performance in terms of soil physical health and increased aggregate stability and content of soil carbon. This is indicative of the advantages of perennial-based systems over seasonal- or annual-based cropping systems for soil sustainability in Eastern Indo-Gangetic Plains.
1 Introduction
Farming system research represents an agricultural research and development methodology that perceives the entire farm as a holistic system. It emphasizes understanding the interconnectedness among various components managed by household members and the intricate interactions between these elements, considering physical, biological, and socioeconomic factors (Shaner et al., 1982; Fatima et al., 2023). The Indian economy is primarily rural and agriculture-centric, with marginal and small farmers comprising 76.2% of the farming population. These farmers often face challenges posed by unpredictable monsoons, leading them to diversify their agricultural activities. They judiciously integrate enterprises such as dairy farming, poultry, pigeon rearing, sericulture, and apiculture, tailored to their specific agro-climatic and socioeconomic circumstances. The eastern region of India comprises 83% rural households, 40% of the population is below the poverty line, and approximately 86% of the population of this region have marginal landholdings, as against 67% of the national average, which is fragmented in nature (Bhagat et al., 2024). The average operational land holding of farmers in this region has declined to 1.16 ha from 2.28 ha from 1970–71 to 2010–11 (Kumar et al., 2018). This region, however, has a higher livestock population density (224 nos./sq. km) compared to the national average (115 nos./sq. km) (Bhatt and Mishra, 2016). Furthermore, the region has very low water productivity (0.54 kg grain/m3), and ~ 14% of soils are problematic in nature (Bhatt et al., 2011).
Addressing the pressing demand to mitigate the environmental footprint of food production and enhance its resilience to forthcoming climate shifts is imperative. Ensuring food security amidst these challenges is paramount. The agricultural domain stands particularly vulnerable to the ramifications of global climate shifts, which are anticipated to alter productivity levels, management practices, and the geographical distribution of crops. During the current COVID-19 pandemic, the eastern region receives higher reverse migration across states. Thus, it is necessary to change the pattern of agriculture using management practices that favor the positive balance of physical, chemical, and biological soil characteristics, such as improvement in carbon, nitrogen, moisture retention, and reduction of soil loss by erosion and leaching. Integrating various components of IFS, viz. field crops, horticultural crops, livestock, poultry, timber, and fishery, helps to increase space and time utilization (Paramesh et al., 2022). Integration of agroforestry in IFS provides a robust approach for carbon sequestration by the accumulation of biomass and organic matter within the soil matrix (Sunderland, 2011; Meera et al., 2019). In the farming systems, C sequestration is substantial due to the crucial role of tree/orchards, extensive use of organic manures and livestock by-products, and reduced fertilizer use. Salton et al. (2014) reported that net greenhouse gas emissions were positive in conventional systems, whereas integrated farming systems show a net negative due to the C sequestration potential within the IFS. Higher total carbon, nitrogen, and water-stable aggregates and microbial biomass C were observed by Maughan et al. (2009) in IFS when compared to continuous corn incorporation. The addition of crop residues and organic manures not only improves nutrient availability but also positively controls the soil quality, having a beneficial effect on crops (Dhaliwal et al., 2019). Several studies reported that the IFS approach would create a synergistic effect among crops, forestry, orchards, fishery, poultry, and apiary, in a way that leftover by-product of one component is used as input for others, ensuring the nutrient recycling and increasing resource use efficiency (Gill et al., 2009; Walia et al., 2019). There are practically no studies available on the impact of IFS on soil aggregation and its associated carbon since experiments related to aggregation in management systems integrated with agri-horti crops and livestock are not only scant but also evaluate mostly yield and economics of the systems. Studies on the effect of agri-horti-livestock systems on soil aggregation in an IFS are scarce in India. This indicates the need to verify the effects of integrated components on soil aggregation, aggregate-associated carbon, and, consequently, on physical protection of SOC, given the direct relationship between aggregation and carbon accumulation in soil. With this background, we hypothesized that soil aggregation and carbon stocks in different resilient cropping systems will be affected by the adoption of integrated farming practices. To test this hypothesis, a study was conducted to assess the effects of long-term IFS on soil carbon stock and soil aggregation patterns and the impact of different crop components on carbon sequestration potential.
2 Materials and methods
2.1 Study site
Two integrated farming system (IFS) models (0.4 ha and 0.8 ha) were initiated during the rainy season of 2008–2009 at the experimental farm of ICAR Research Complex for Eastern Region, Patna, India (25°59’ N latitude, 85°08′ E longitude, 53.5 above MSL). The experimental area falls under the middle Indo-Gangetic Plains (MIGPs) of India and is characterized by a subtropical humid climate. The soil of the experimental site was old alluvium having clay loam texture (29.4% sand, 39.4% silt, and 31% clay) (Bouyoucos, 1962), pH 6.6 (Jackson, 1973), organic carbon 0.6% (Walkley and Black, 1934), available N 95.1 ppm (Subbiah and Asija, 1956), Olsen’s phosphorus 8.48 ppm (Olsen et al., 1954), and available potassium 92.8 ppm (Hanway and Heidel, 1952). All basic soil properties were analyzed following standard laboratory methods. The farm consists of various interrelated components and a flow of farm components within and outside the system. One acre farm (0.4 ha) was divided into rice-based cropping system (rice-wheat-fodder, rice-maize-fodder, rice-wheat-lentil, rice-mustard-wheat, and rice-fodder-fodder), mushroom unit, horticultural crops (vegetable block-cauliflower, cowpea, okra, onion, and tomato; fruit trees-guava having turmeric as an intercrop and lemon), goat unit (20 no. and a buck), poultry (100 no. in each batch), and a vermicomposting unit. The 2-acre farm (0.8 ha) was divided into rice-based cropping system (rice-wheat-fodder, rice-winter maize-fodder, rice-wheat/lentil, rice-mustard/wheat, and rice-lentil-fodder), fish-duck integration, livestock (2 cows and 2 calves), horticultural crops (vegetable block- cauliflower, cowpea, okra, onion and tomato; fruit trees-guava and lemon having turmeric as intercrop), and a vermi-composting unit. The crops grown in the kharif, rabi, and summer seasons are mentioned in Table 1. The appropriate package of practices followed as per the crop requirement for rice, wheat, maize, mustard, lentil, fodder, and horticultural crops. Fertilizers were applied based on the standard recommended doses of particular crops. Some of the components, viz. animal feed, fertilizers, etc., were imported into the system, and some of the components, viz. crop products (grains, fruits, and vegetables) and animal products (meat, eggs, fish, milk, etc.) were exported out of the system (Supplementary Table S1). Detailed components of the 0.4 ha and 0.8 ha IFS systems are provided in Table 1.
2.2 Soil sampling and processing
Soil samples were collected 10 years after the establishment of the experiment. Samples were collected after harvesting rabi season crops. Soil samples from each treatment were collected from 0 to 15 and 15 to 30 cm depths of soil in four replicates with the help of an auger and spade. The soil samples were air-dried and sieved to analyze total organic carbon. Undisturbed soil samples collected using a spade were dried for aggregate analysis. The samples were then broken into their natural plane and utilized for aggregate analysis. For bulk density, a core sampler was used to collect the samples. The collected samples were oven-dried at 105°C and weighted to calculate bulk density.
2.3 Soil analysis
2.3.1 Total organic carbon
Using the tube digestion method, the total organic carbon in the soil sample was determined (Haenes, 1984). In summary, a 100 ml digestion tube containing 1.0 g of finely powdered (< 0.15 mm) soil sample was heated at 135°C for 30 min after adding 10 mL of 1 N K2Cr2O7 and 20 mL of concentrated H2SO4. The TOC of soil samples was determined as Cr+3 concentration in diluted soil digest by taking absorbance reading at 600 nm with calibration against similarly treated sucrose standard solution.
2.3.2 Soil organic carbon stock
The size of organic carbon stock was computed by multiplying organic carbon oxidized by 24 N H2SO4 with bulk density values of each plot and soil depth (0–15 cm). The following is the formula used (Samal et al., 2020):
Where C is the concentration expressed in g kg−1 soil, BD in Mg m−3, depth in m, and C stock in Mg ha−1.
2.3.3 Carbon flows
The farm design model (Groot et al., 2012) was used to quantify the carbon flows in 0.4 and 0.8 ha farming system models. The input data contain the data of various farm resources. The input database includes various components, viz. biophysical environment (soil physic-chemical properties, climate, and potential soil erosion rate), crops (agronomic practices, labor requirement, prices, and subsidies), crop products (crop production, bedding material, green manures, animal feed, home consumption, etc.), crop rotations, animals, and animal products (management, production, labor requirement, animal feeds, product price, and home consumption), fertilizers, and imported manures. Based on crop productivity, animal and crop product destinations (and imports and exports), animal feed balance, and the fertilizer, manure, and organic matter turnover, carbon flow between the systems was estimated.
2.3.4 Aggregate fractionation
Representative sub-samples of approximately 50 g of soil underwent a gentle process of sieving through a 10 mm mesh to break soil along its natural planes of weakness. After the soil was allowed to air dry, it was separated into four groups using Elliott’s (1986) wet sieving technique: (a) large macroaggregates (LM; >2000 μm), (b) small macroaggregates (SM; 250–2000 μm), (c) microaggregates (Mi; 53–250 μm), and (d) silt and clay-sized particles (SC; ≤53 μm). Subsequently, about 50 g of air-dried soil was evenly spread onto a 2 mm sieve submerged in deionized water and allowed to slake. The sieve was manually agitated for 2 min after a 5 min duration. This procedure was repeated with sieves measuring 250 μm and 53 μm. The soil aggregates extracted from each sieve were carefully cleaned, oven-dried for 48 h at 60°C, weighed, and stored in preparation for carbon analysis. The volume of the subsample and the total suspension volume were utilized to calculate the silt and clay-sized particles (SC).
The mean weight diameter (MWD) and geometric mean diameter (GMD) of water-stable aggregates (Kemper and Roseneau, 1986) were calculated using the following equations:
where n is the number of fractions and Xi is the mean diameter (mm) of the sieve used.
2.4 Statistical analysis
The data obtained underwent analysis of variance (ANOVA) to determine significant differences among the treatments. For multiple comparisons among the treatments at a significance level of p < 0.05, Duncan’s Multiple Range Test (DMRT) was employed. The statistical analysis was conducted using the Indian NARS Statistical Computing Portal, which is available at http://stat.iasri.res.in/sscnarsportal.
3 Results
3.1 Total organic carbon and C stock
After 10 years of establishment, significant (p < 0.05) differences in TOC and C stock in both 0.4 and 0.8 ha IFS models were reported (Table 2). In the 0.4 ha IFS model, the fodder system showed significantly higher TOC (10.5 g kg−1 soil) followed by guava orchard intercropped with turmeric (9.22 g kg−1 soil) in the 0–15 cm soil layer, and a similar trend was also observed in the 15–30 cm depth. Owing to higher TOC in the fodder system, this system contributed the greatest C stock in both depths compared to other components. In the 0.8 ha IFS model as well, the fodder system achieved significantly higher TOC (11.37 g kg−1) and C stock (26.09 Mg m−3) in the 0–15 cm depth. The rice-based system had significantly lower TOC (7.50 g kg−1 soil) in 0–15 cm depth. At 15–30 cm depth, the fodder system showed significantly higher C stock (9.95 g kg−1 soil) and TOC (24.74 Mg m−3) contents, followed by the vegetable-based production system (22.83 Mg m−3). Considering all the treatments, the surface layer contained 15 and 11% higher TOC and C stock than the 15–30 cm layer in the 0.4 ha model. In the 0.8 ha model, the impact was greater as it showed 19 and 15% higher TOC and C stock over the lower layer. Among the two models, the 0.8 ha model possessed more carbon stock (~11%) than the 0.4 ha model in the 0–30 cm soil depth.
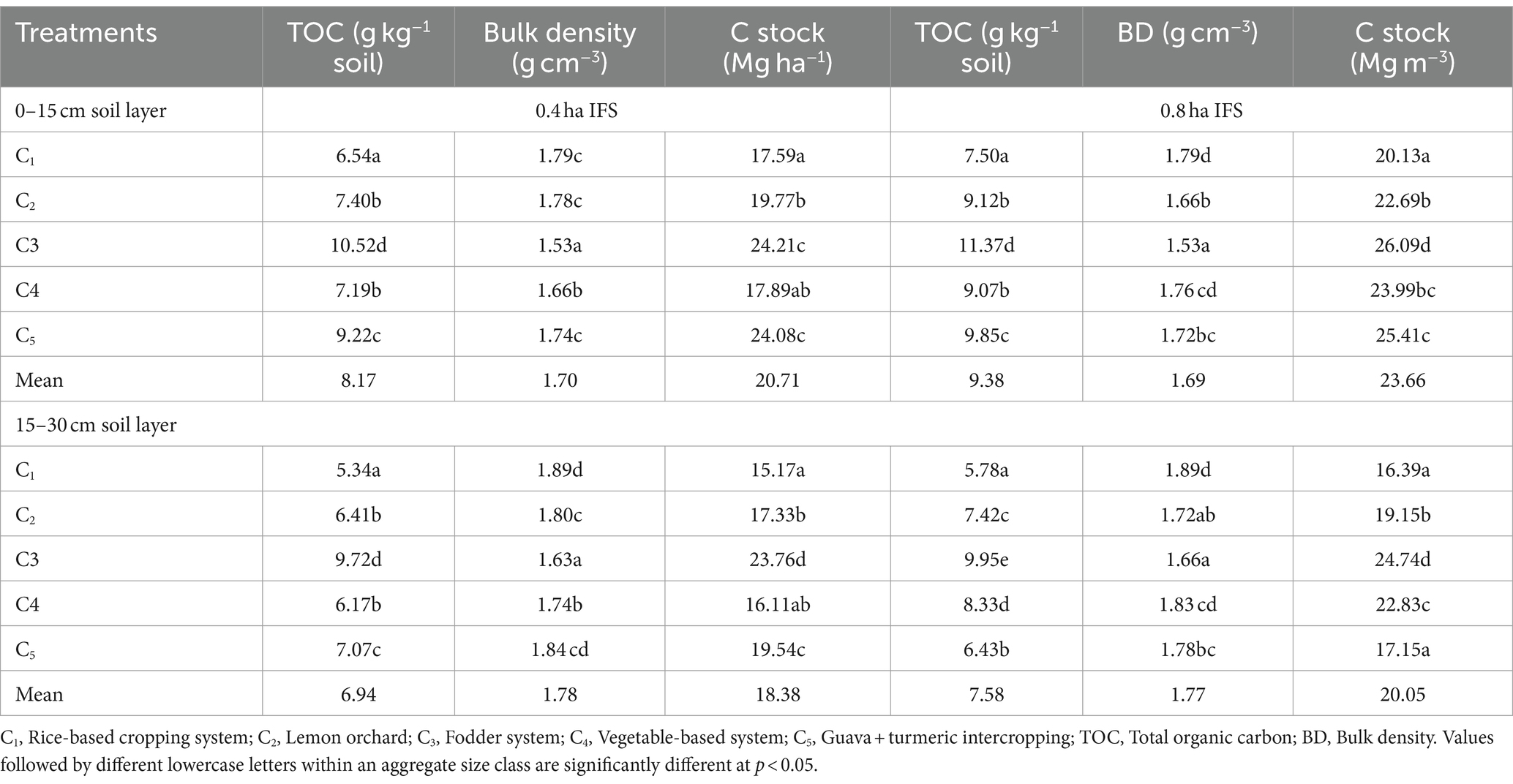
Table 2. Total organic carbon (TOC), bulk density, and C stock as influenced by the different farming system models.
In both 0.4 ha and 0.8 ha models, bulk density was significantly (p < 0.05) influenced by the cropping system. In both cases, the fodder-based system registered the lowest bulk density, while the rice-based system recorded the highest value. Irrespective of the cropping system, bulk density increased by 4.5 and 4.52% in the sub-surface (15–30 cm) over the surface layer (0–15) for the 0.4 ha and 0.8 ha models, respectively. The difference in bulk density across soil depths was less in the lemon intercropping system in both models.
3.2 Carbon flows
Carbon flows in the 0.4 ha and 0.8 ha IFS models are illustrated in Figures 1A,B. In the 0.4 ha model, the addition of poultry concentrate, farmyard manure, vermicompost, and mushroom left out added ~1,500 kg of C to the soil every year, whereas litter fall from orchard/crop residue added 392 kg carbon to the soil. Approximately 5 tonnes of carbon were exported out of the farm as different crop products and 456 kg of carbon was lost in the form of animal products. In addition, 884 kg of carbon was lost from the system during degradation of manures. Overall, the 0.4 ha system added 1890 kg of carbon in the soil, which either accumulated or lost from the system based on the management practices. In the case of the 0.8 ha model, 690 kg and 61 kg of carbon were added to the soil from manures and crops. Different crops assimilated ~9,672 kg carbon, and 2,626 kg C was imported to the system by various means. Of this total accumulated C, 4955 kg was exported out of the system as crop products, and 4,524 kg was utilized by livestock in the form of feed and bedding material. The livestock unit contributed 539 kg in the form of manure and 3,716 kg lost during the respiration process. In the 0.8 ha model, a net amount of 752 kg of soil organic carbon annually remained in the soil.
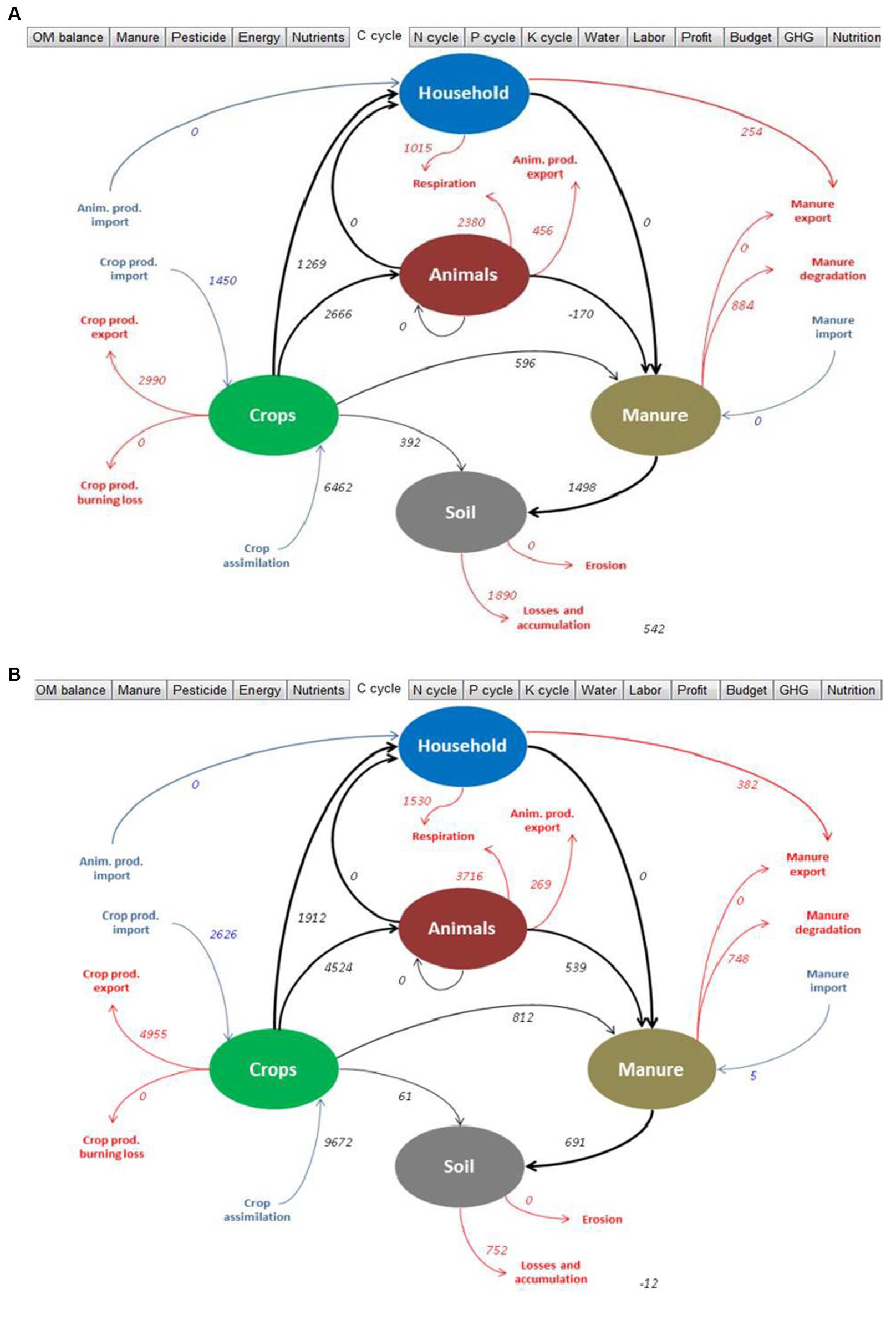
Figure 1. Schematic representation of farm design model showing carbon flows between different components of 0.4 (3a) and 0.8 ha (3b) IFS models. The black arrows represent C-flow within the farming system. The blue arrows represent inflows, and the red arrows represent outflow/losses (all values in kg/ha/year).
3.3 Soil aggregate fractionation
The results indicated that there was a significant effect of cropping systems on aggregate size distribution in both farming system models (Tables 3, 4). In the 0.4 and 0.8 ha models, macroaggregates accounted for approximately 75% of total aggregates and were the most dominant water-stable aggregate size class in the surface layer. In the top layer (0–15 cm) of the 0.4 ha IFS model, the fodder system showed a significantly higher LM fraction (17.25 g 50 g soil−1) followed by rice-based cropping system (15.35 g of 50 g soil−1), whereas vegetable system showed the lowest LM (11.17 g 50 g soil−1). The vegetable system shows significantly higher SM (22.23 g 50 g soil−1) followed by guava + turmeric intercropping (20.86 g 50 g soil−1) and lemon-based system (20.61 g 50 g soil−1). The rice system showed a significantly higher microaggregate fraction (14.87 g 50 g soil−1), while the fodder system recorded the lowest value (6.01 g 50 g soil−1). Guava + turmeric intercropping (3.67 g 50 g soil−1) showed a significantly lower silt + clay (SC) fraction, which was at par with the fodder system, whereas the fodder system showed a significantly higher SC fraction (8.12 g 50 g soil−1).
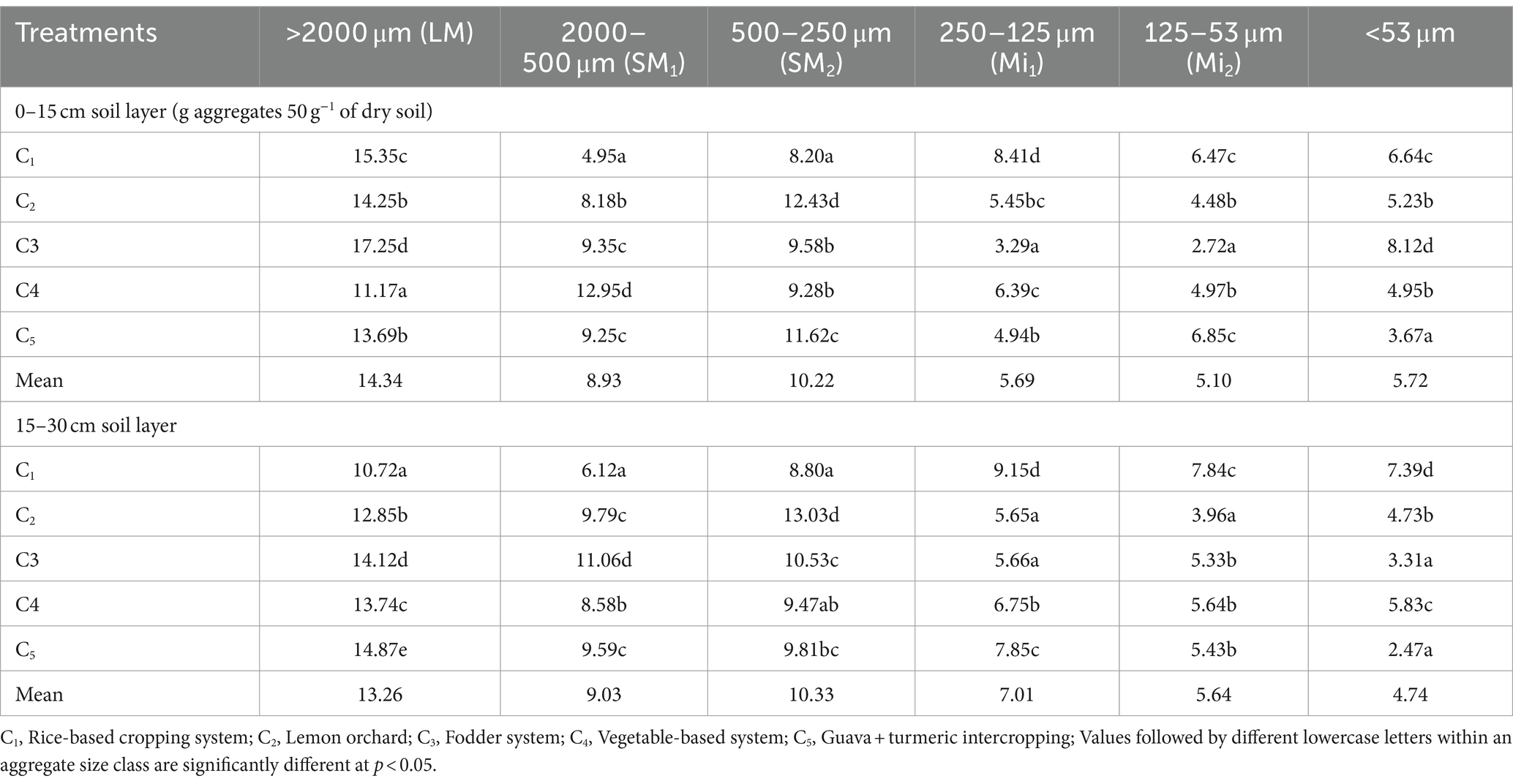
Table 3. Distribution of aggregates of different size classes and aggregation indices as influenced by different farming systems in 0.4 ha IFS models.
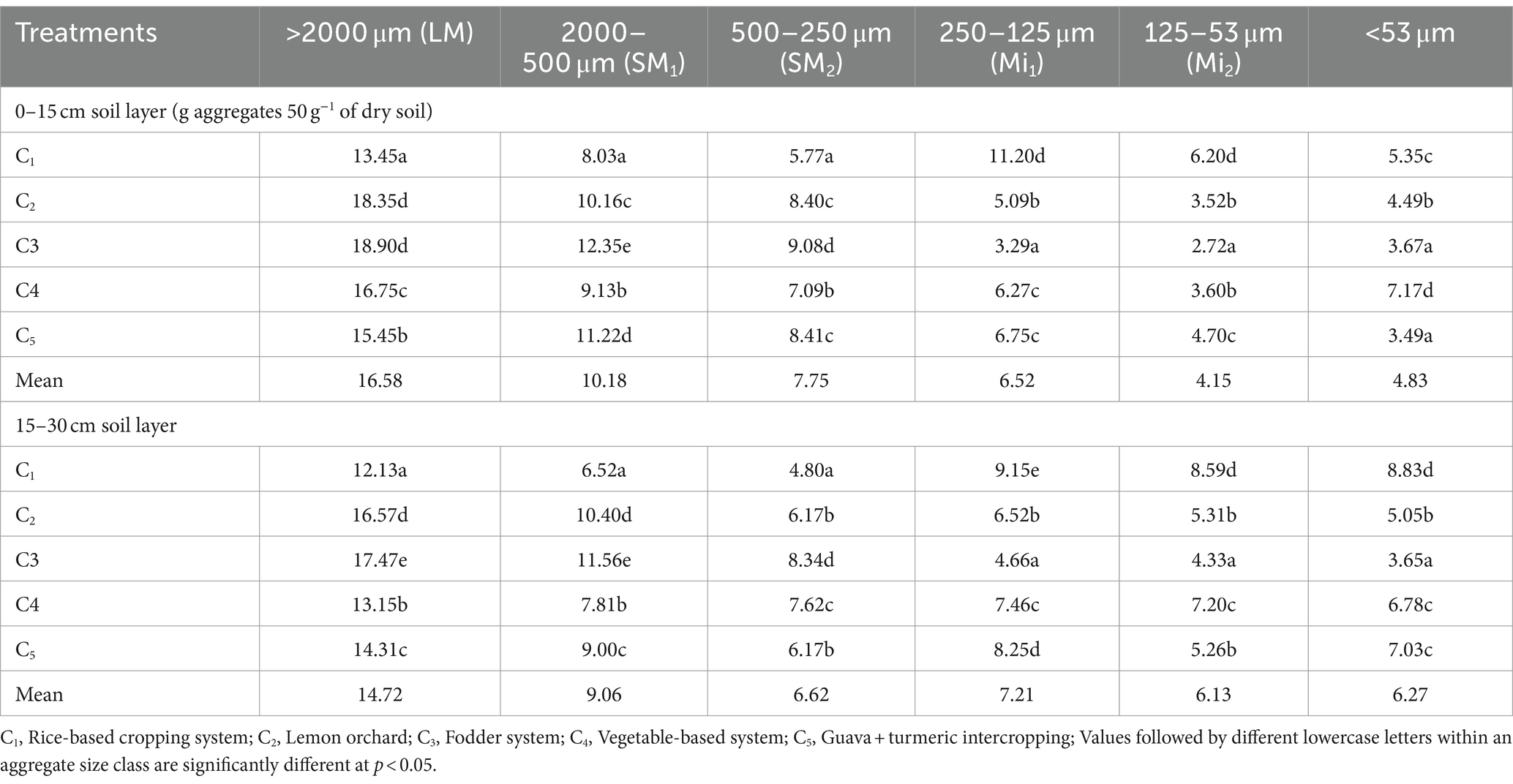
Table 4. Distribution of aggregates of different size classes and aggregation indices as influenced by different farming systems in the 0.8 ha IFS models.
The proportion of macroaggregates (Figure 2) present in the sub-surface layer was slightly lower (~65%) compared to the surface layer of soil (~67%). However, it was also observed that the vegetable-based cropping system and the guava + turmeric intercropping system had higher macroaggregates at lower depths than the surface layer. In 15–30 cm soil depth, rice showed significantly the lowest SM (14.92 g 50 g soil−1). Lemon orchards showed significantly higher SM (22.81 g 50 g soil−1) but were at par with the fodder system. It was observed that lower depth had slightly (2%) higher SM over the surface layer of soil irrespective of cropping systems. In contrast, the rice-based cropping and guava + turmeric intercropping systems showed higher Mi. Overall, in the 0.4 ha model, the 15–30 cm layer contained 14.7% more microaggregates than 0–15 cm. Irrespective of cropping systems, the surface layer showed a 3% increase in LM. The reduction in macroaggregate in lower depth might be due to lesser root activity. However, the proportion of SM was similar in both soil depths, while the distribution of silt and clay particles (aggregates) was higher (17%) in the 15–30 cm soil depth than in the surface layer (0–15 cm). It was also found that practices of different cropping systems had a significant influence on MWD and GMD (Figure 3). The fodder-based systems registered significantly higher MWD (1.63) and GMD (0.87) in the 0–15 cm depth.

Figure 2. Depth-wise macro and microaggregate distribution in 0.4 and 0.8 ha IFS models. Small MacAg, small macro aggregates; MicAg, micro aggregates. Values followed by different lowercase letters within an aggregate size class are significantly different at p < 0.05.
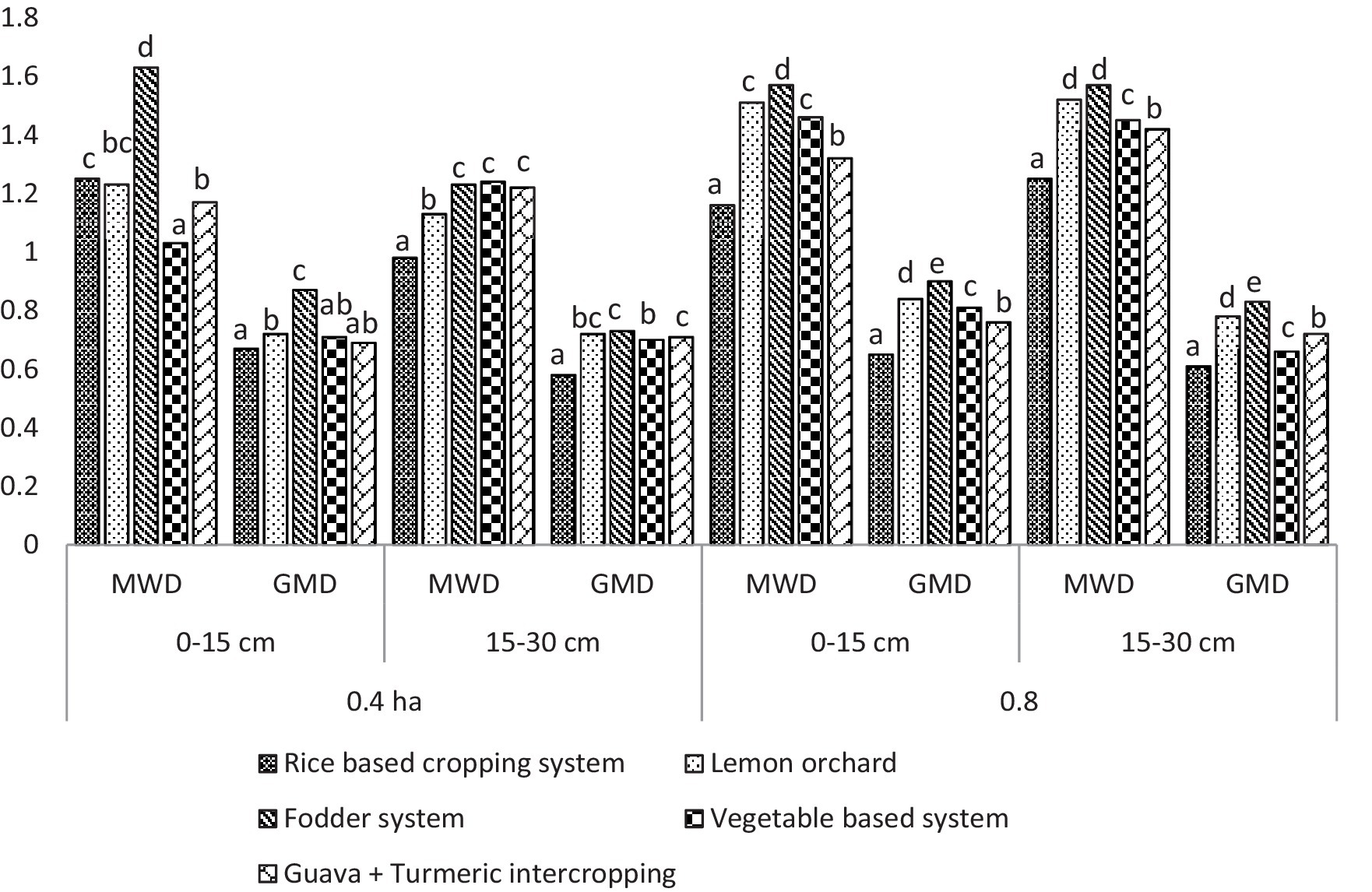
Figure 3. Depth-wise aggregation indices in 0.4 and 0.8 ha IFS models. MWD, mean weight diameter; GMD, geometric mean diameter. Values followed by different lowercase letters within an aggregate size class are significantly different at p < 0.05.
In the 0.8 ha IFS model, the rice-based cropping system showed significantly higher Mi (17.40 g 50 g soil−1) and lower SM (13.80 g 50 g soil−1) in topsoil (0–15 cm). The lemon-based system showed significantly lower Mi (8.61 g 50 g soil−1) and guava+turmeric had the highest SM (19.63 g 50 g soil−1). The distribution of microaggregates was found higher in 15–30 cm than in 0–15 in both models except guava + turmeric intercropping in the 0.4 ha model and rice-based cropping system in the 0.8 ha model, which showed a decreasing trend of microaggregates distribution with increasing soil depth. In the case of LM, the fodder system had the highest value (18.9 g/50 g) but was at par with the lemon system (18.35 g/50 g). The fodder system (1.57), followed by lemon (1.51) along with the vegetable system (1.46), showed a significantly higher mean diameter of aggregates. A similar trend was also observed in geometric mean diameter.
3.4 Aggregate-associated organic carbon
Data pertaining to aggregate-associated organic carbon revealed that maccoaggregates (Ma) had a higher potential to accumulate a higher amount of carbon content on their particles compared to microaggregate (Mi) fractions (Table 5). Macroaggregates contributed ~67% of the total organic carbon associated as compared to the rest of the aggregate fractions. Among macroaggregates, the SM category represented ~64% irrespective of the cropping system in the 0.4 ha model. Overall, in the 0–15 cm depth, the fodder system outweighed the rest of the cropping system, and the rice-based system showed the least carbon content except in SM, where the vegetable system prevailed. Comparatively, there was a 17% decrease in aggregate-associated organic carbon content in the 15–30 cm soil depth compared to the 0–15 cm depth. At 15–30 cm depth, the fodder-based system accumulated the highest organic carbon and the vegetable system represented the minimum carbon content.
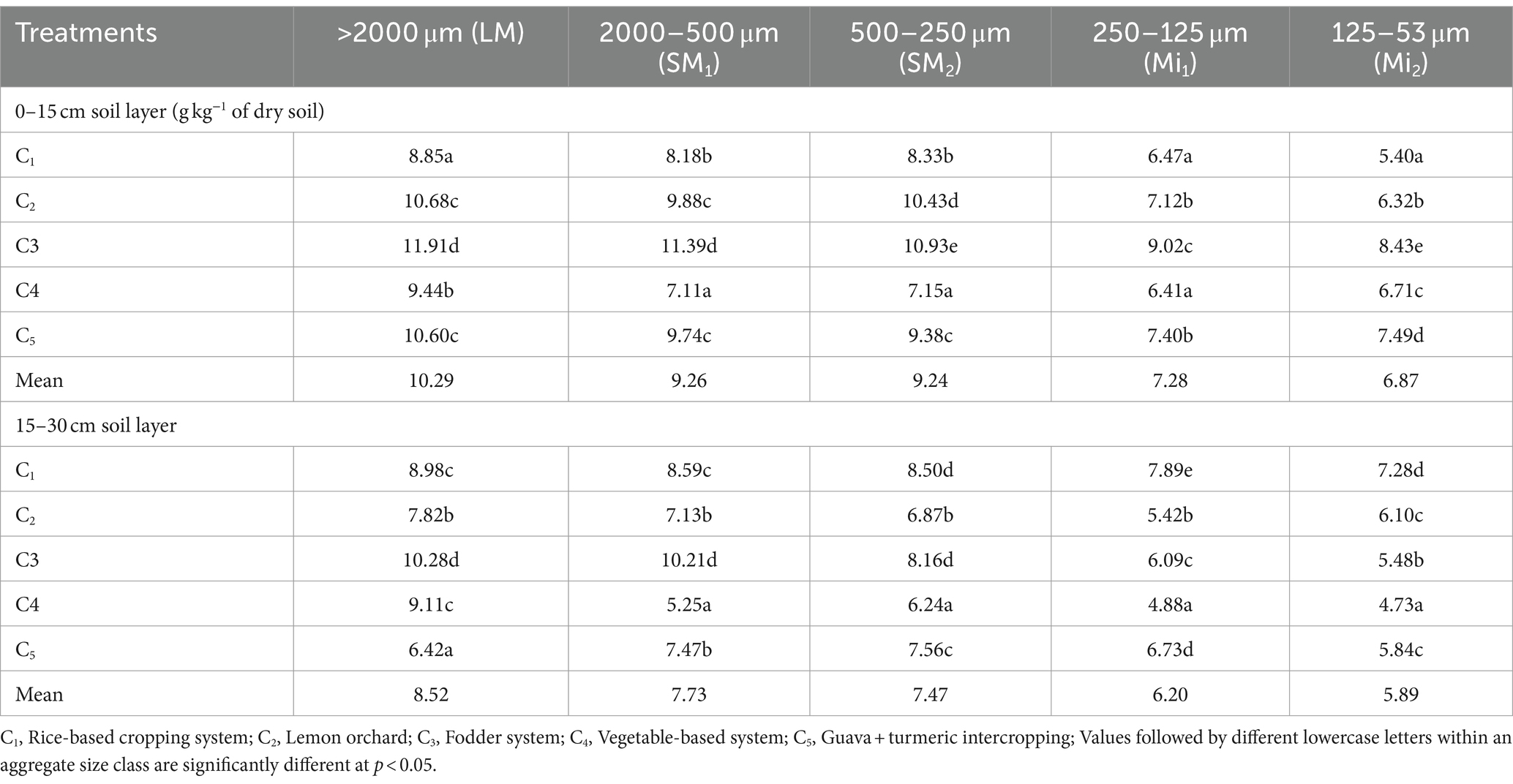
Table 5. Aggregate-associated organic carbon (AAOC) in different aggregate size classes as influenced by different farming systems in the 0.4 ha IFS system model.
In the 0.8 ha model as well, macroaggregates contribute significantly to total organic carbon (63%) compared to other aggregate fractions; however, this value is slightly lower than that of the 0.4 ha model (67%) in the 0–15 cm depth (Table 6). Among the cropping systems, the fodder system consistently performed the best, whereas the rice-based system had the lowest carbon content. In 15–30 cm depth as well, the fodder-based system showed better performance while the vegetable/lemon-based system contained the least aggregate-associated organic carbon in macroaggregate and microaggregate fractions, respectively. Comparatively, the 0.8 ha model had 8% less aggregate associated organic carbon than 0.4 ha. There was an increase of ~3% aggregate-associated organic carbon in 15–30 over 0–15 cm soil depth due to increased microaggregate fraction.
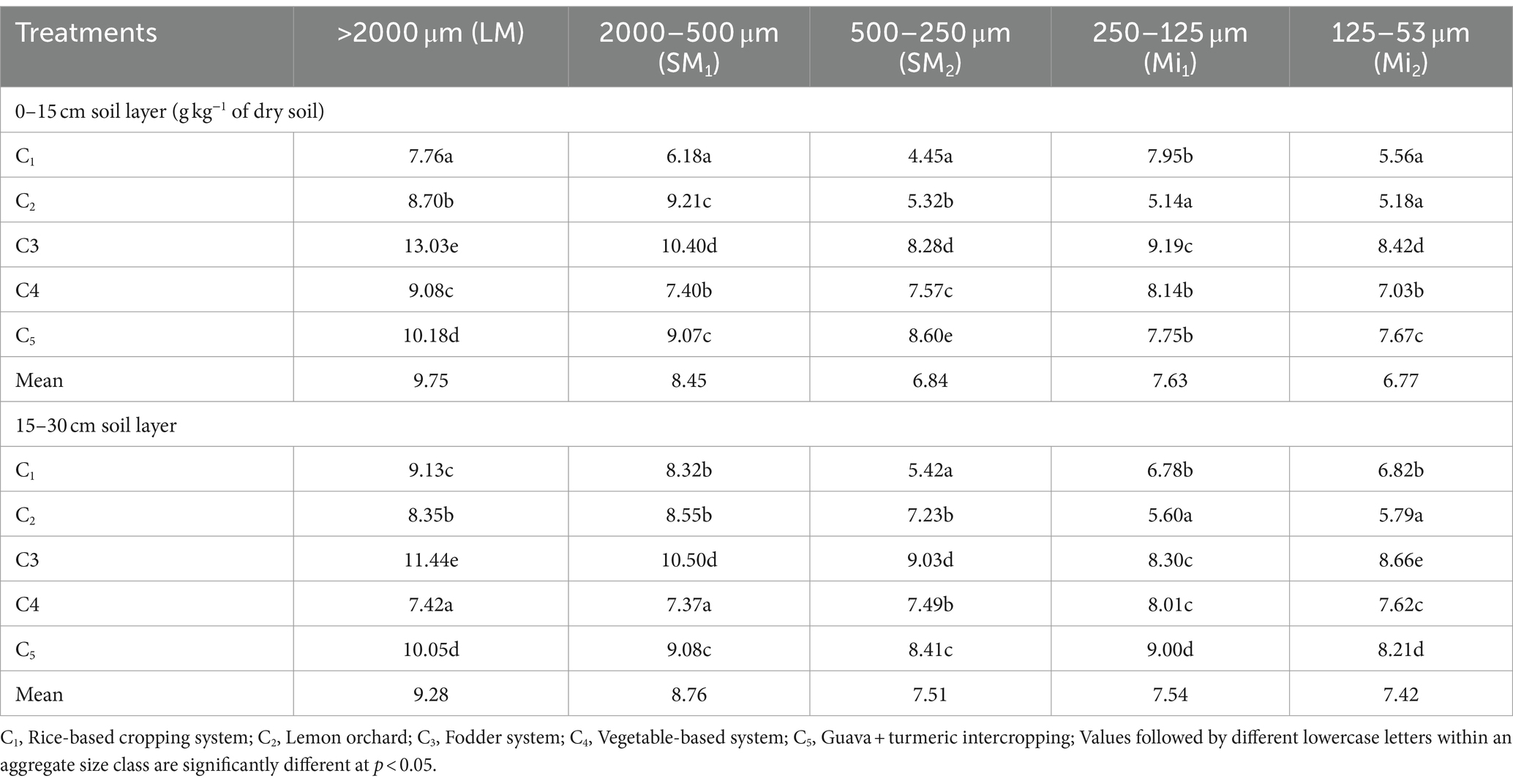
Table 6. Aggregate-associated organic carbon (AAOC) in different aggregate size classes as influenced by various farming systems in the 0.8 ha IFS model.
4 Discussion
The extensive and fibrous root nature of perennial grasses has more advantages than annual crops, such as rice, wheat, and vegetable crops. In the fodder-based cropping system, the higher TOC content of soil can be attributed to the continuous addition of organic matter in soil via degeneration of root and limited mineralization of organic matter owing to less disturbance of soil which can eventually maximize C-accumulation in soil (Mcsherry and Ritchie, 2013; Krüger et al., 2015; Das et al., 2016; Li et al., 2018). It is worth mentioning that horticultural crops such as guava- and lemon-based intercropping systems also had lower carbon accumulation than forage systems. This may be partly attributed to the soil tillage operation performed before planting intercrops in such production systems. A higher amount of carbon content in surface soil than in sub-surface soil has been reported by several authors, including Lenka et al. (2012).
Soil organic content is markedly influenced by management practices associated with a change in the cropping system, which eventually affects the soil structural behavior via soil compactness level. This corroborates the results of our study, where the fodder-based system achieved the lowest bulk density. Most seasonal cropping systems generated greater bulk density owing to more tillage or cultivation operation than perennial fodder grasses. It is widely accepted that soil organic matter has a direct influence on soil bulk density and vice versa (Leifeld et al., 2015; Gajda et al., 2016).
The adoption of a diverse cropping system has exerted a significant influence on the distribution of soil aggregate, and this is mainly attributed to the life span of the crop as well as management practices (tillage frequency) (Broersma et al., 1997; Mondal et al., 2021). The presence of a higher proportion of macroaggregates under a fodder-based system has largely contributed to increased root activity (Gelaw et al., 2013) as well as the continuous addition of litter in soil (Kautz et al., 2010). In this context, Kautz et al. (2010) reported an improvement in soil structure due to the binding potential of perennial fodder systems by their increased root activity. The role of microbial activity through the expansion of hyphal networking in this condition cannot be ruled out (Hallett et al., 2009; Sale et al., 2021). In this context, the release of root exudates such as phenolics (Martens, 2000) and glucosamine (Chantigny et al., 1997) during decomposition has also been responsible for the improvement of soil aggregate stability in the perennial fodder-based system. Mostly in the vegetable system, soils are tilled through conventional practices, and breakage of macroaggregates could lead to the formation of SM and to some extent microaggregates (Grandy and Robertson, 2007). Higher microaggregate fraction in rice-based systems shows that seasonal or annual crop-based cropping systems have a higher amount of microaggregates than perennial-based systems. This is in accordance with the results by Cates et al. (2016). Seasonal cropping systems such as rice and vegetable-based cropping systems generally possess fibrous or fine root systems, which leads to the production of microaggregates compared to perennial systems such as the fodder-based system (Chu et al., 2016). Interestingly, the production of SM was most prominent under the perennial system, especially in lemon and guava intercropping, indicating that the inclusion of horticultural fruit trees in the system has the potential to improve soil aggregate stability.
Under the fodder system, the soil was less affected due to the lesser extent of tillage operation, thereby preventing the destruction of soil particles. In this contention, Arshad et al. (2004) confirmed a higher MWD under no-tillage than under conventionally tilled soil mostly occupied by annual crops. Lenka et al. (2012) also found improvement in MWD where perennial grasses (agroforestry) were grown under sloping land conditions, indicating continuous addition of organic matter, which helped in the formation of clay humus complexes. While in 15–30 cm depth, the vegetable system recorded maximum MWD, which was statistically at par with the fodder system and guava + turmeric intercropping. The GMD showed a similar trend to that of MWD. Comparatively, the surface layer had higher MWD and GMD than the sub-surface layer. Interestingly, there was a similarity of macroaggregates distribution in the surface layer of soil in both the IFS model, 0.4 ha (75%) and 0.8 ha (76%). Macroaggregates are the dominant fraction in the soil, and the results are in conformity with previous studies by Lenka et al. (2012) and Gelaw et al. (2013). In general, the presence of microaggregates was slightly higher in the 0.8 ha than in the 0.4 ha model. This is possibly accredited to higher tillage operation since the rice fodder of the 0.4 ha model was replaced by the rice-lentil-fodder system in the 0.8 ha model, where the rest of the cropping system remained the same in both IFS models.
From the present study, it is clear that MWD has a significant positive relationship with organic carbon content. In other words, MWD showed an increasing tendency with the rise in organic C content in soil across cropping systems in both IFS models. This result is consistent with the study by Yousefi et al. (2008). Most significantly, we observed that aggregate stability was linked with the organic carbon content across cropping systems in both IFS models. The climatic and edaphic conditions of the two study sites were similar in nature and were validated in our study. Nonetheless, this is not always true for every situation where climatic and edaphic conditions across the sites are varied (Haynes et al., 1991; Tete-Mensah et al., 1999). In 15–30 cm depth, both LM and SM fractions were significantly higher in the fodder-based system and least in the rice-based cropping system. At the same time, a reverse trend was observed for Mi. As in 0–15 cm, 15–30 cm depth also had significantly higher GMD and MWD in the fodder system and least in the rice-based system.
Overall, aggregate-associated organic carbon is more in macroaggregates than microaggregates. Across IFS models, the fodder system noted the highest aggregate-associated organic carbon than the rest of the cropping systems. This is an indication of the occurrence of decomposition of root and hyphal networks inside macroaggregates (Gelaw et al., 2013). Rao et al. (2022) also claimed that the predominance of aggregate-associated organic carbon was higher in macroaggregates than in microaggregrates in their studies. It was also suggested that microbial activity played a major role in protecting macroaggregates, thereby improving organic carbon within macroaggregates. Although comparatively, the 0.8 ha model had more total organic carbon than the 0.4 ha model, but in aggregate-associated organic carbon, the same trend was not observed. This might be due to the effect of the cropping pattern/system on carbon storage potential in different fractions of the soil aggregates.
5 Conclusion
The sustainability of farming system models is largely linked with the soil productive capacity of the site. In other words, system productivity depends on nutrient recycling of systems involved in the buildup of soil organic matter. Soil organic matter and aggregate distribution could be varied with the management intensity of systems such as tillage and cultural operations associated with distinct cropping systems.
• In this study, SOC, aggregate distribution, and aggregate-associated organic carbon were markedly influenced by cropping systems adopted across the IFS model.
• The study indicated that fodder-based systems had a better performance than other cropping systems in terms of soil physical health and soil organic carbon. This is indicative of the advantages of perennial-based systems over seasonal or annual-based cropping systems for soil sustainability in Eastern Indo-Gangetic plains. Nevertheless, the extent of soil disturbance by tillage cannot be ruled out.
Additional research on various cropping patterns and crop product incorporation on nutrient dynamics and greenhouse gas emissions would provide valuable information. In addition, the contribution of various by-products, viz. goat manure, poultry manure, vermin wash, and crop residues on soil microbial population, will be important.
Data availability statement
The raw data supporting the conclusions of this article will be made available by the authors, without undue reservation.
Author contributions
KR: Conceptualization, Data curation, Formal analysis, Investigation, Methodology, Project administration, Supervision, Validation, Writing – original draft, Writing – review & editing. SS: Conceptualization, Data curation, Formal analysis, Investigation, Writing – original draft, Writing – review & editing. SanjK: Conceptualization, Data curation, Formal analysis, Investigation, Methodology, Writing – original draft, Writing – review & editing. NS: Formal analysis, Writing – original draft, Writing – review & editing. RK: Data curation, Formal analysis, Supervision, Writing – original draft, Writing – review & editing. SM: Conceptualization, Data curation, Formal analysis, Writing – original draft, Writing – review & editing. SantK: Conceptualization, Data curation, Formal analysis, Writing – original draft, Writing – review & editing. JM: Conceptualization, Data curation, Formal analysis, Methodology, Supervision, Writing – original draft, Writing – review & editing. BB: Conceptualization, Data curation, Formal analysis, Investigation, Software, Writing – original draft, Writing – review & editing. NR: Formal analysis, Writing – original draft, Writing – review & editing, Data curation. SuK: Data curation, Formal analysis, Writing – original draft, Writing – review & editing. PU: Data curation, Formal analysis, Writing – original draft, Writing – review & editing. SJ: Data curation, Formal analysis, Writing – original draft, Writing – review & editing. AC: Data curation, Formal analysis, Writing – original draft, Writing – review & editing.
Funding
The author(s) declare that no financial support was received for the research, authorship, and/or publication of this article.
Acknowledgments
The authors express their gratitude to the Indian Council of Agricultural Research for the financial support provided under the All India Co-ordinated Research Project on Integrated Farming Systems Research Programme (AICRP on IFS).
Conflict of interest
The authors declare that the research was conducted in the absence of any commercial or financial relationships that could be construed as a potential conflict of interest.
The reviewer RP declared a shared affiliation with the author AC to the handling editor at the time of review.
Publisher’s note
All claims expressed in this article are solely those of the authors and do not necessarily represent those of their affiliated organizations, or those of the publisher, the editors and the reviewers. Any product that may be evaluated in this article, or claim that may be made by its manufacturer, is not guaranteed or endorsed by the publisher.
Supplementary material
The Supplementary material for this article can be found online at: https://www.frontiersin.org/articles/10.3389/fsufs.2024.1384082/full#supplementary-material
References
Arshad, M. A., Franzluebbers, A. J., and Azooz, R. H. (2004). Surface-soil structural properties under grass and cereal production on a MollicCyroboralf in Canada. Soil Tillage Res. 77, 15–23. doi: 10.1016/j.still.2003.10.004
Bhagat, R., Walia, S. S., Sharma, K., Singh, R., Singh, G., and Hossain, A. (2024). The integrated farming system is an environmentally friendly and cost-effective approach to the sustainability of Agri-food systems in the modern era of the changing climate: a comprehensive review. Food Energy Secur. 13:e534. doi: 10.1002/fes3.534
Bhatt, B. P., Haris, A., Islam, A., Dey, A., Mukherjee, J., Barari, S. K., et al. (2011). Agriculture in eastern states: opportunities and challenges. Technical bulletin no. R-31/PAT-20.
Bhatt, B. P., and Mishra, J. S. (2016). Production & technological gaps in middle indo-Gangetic Plains. ICAR Research Complex for Eastern Region, Patna, Bihar, India: Policy document.
Bouyoucos, G. J. (1962). Hydrometer method improved for making particle size analyses of soils. Agron. J. 54, 464–465. doi: 10.2134/agronj1962.00021962005400050028x
Broersma, K., Robertson, J. A., and Chanasyk, D. S. (1997). The effects of diverse cropping systems on aggregation of a Luvisolic soil in the Peace River region. Can. J. Soil Sci. 77, 323–329. doi: 10.4141/S96-013
Cates, A. M., Ruark, M. D., Hedtcke, J. L., and Posner, J. L. (2016). Long-term tillage, rotation and perennialization effects on particulate and aggregate soil organic matter. Soil Tillage Res. 155, 371–380. doi: 10.1016/j.still.2015.09.008
Chantigny, M. H., Angers, D. A., Prévost, D., Vézina, L. P., and Chalifour, F. P. (1997). Soil aggregation and fungal and bacterial biomass under annual and perennial cropping systems. Soil Sci. Soc. Am. J. 61, 262–267. doi: 10.2136/sssaj1997.03615995006100010037x
Chu, J., Zhang, T., Chang, W., Zhang, D., Zulfiqar, S., Fu, A., et al. (2016). Impacts of cropping systems on aggregates associated organic carbon and nitrogen in a semiarid highland agroecosystem. PLoS One 11:e0165018. doi: 10.1371/journal.pone.0165018
Das, A., Patel, D. P., Lal, R., Kumar, M., Ramkrushna, G. I., Layek, J., et al. (2016). Impact of fodder grasses and organic amendments on productivity and soil and crop quality in a subtropical region of eastern Himalayas, India. Agric. Ecosyst. Environ. 216, 274–282. doi: 10.1016/j.agee.2015.10.011
Dhaliwal, S. S., Naresh, R. K., Mandal, A., Singh, R., and Dhaliwal, M. K. (2019). Dynamics and transformations of micronutrients in agricultural soils as influenced by organic matter build-up: A review. Environ. Sustain. Indic. 1–2:100007. doi: 10.1016/j.indic.2019.100007
Elliott, E. (1986). Aggregate Structure and Carbon, Nitrogen, and Phosphorus in Native and Cultivated Soils. Soil Science Society of America Journal, 50, 627–633. doi: 10.2136/sssaj1986.03615995005000030017x
Fatima, A., Singh, V. K., Babu, S., Singh, R. K., Upadhyay, P. K., Rathore, S. S., et al. (2023). Food production potential and environmental sustainability of dierent integrated farming system models in Northwest India. Front. Sustain. Food Syst. 7:959464. doi: 10.3389/fsufs.2023.959464
Gajda, A. M., Czyz, E. A., and Dexter, A. R. (2016). Effects of long-term use of different farming systems on some physical, chemical and microbiological parameters of soil quality. Int. Agrophys. 30, 165–172. doi: 10.1515/intag-2015-0081
Gelaw, A. M., Singh, B. R., and Lal, R. (2013). Organic carbon and nitrogen associated with soil aggregates and particle sizes under different land uses in Tigray, Northern Ethiopia. Land Degrad. Dev. 26, 690–700. doi: 10.1002/ldr.2261
Gill, M. S., Singh, J. P., and Gangwa, K. (2009). Integrated farming system and agriculture sustainability. Indian J. Agron. 54, 128–139. doi: 10.59797/ija.v54i2.4790
Grandy, A. S., and Robertson, G. P. (2007). Land-use intensity effects on soil organic carbon accumulation rates and mechanisms. Ecosystems 10, 59–74. doi: 10.1007/s10021-006-9010-y
Groot, J. C., Oomen, G. J., and Rossing, W. A. (2012). Multi-objective optimization and design of farming systems. Agric. Syst. 110, 63–77. doi: 10.1016/j.agsy.2012.03.012
Haenes, D. L. (1984). Determination of total organic C in soils by an improved chromic acid digestion and spectrophotometric procedure. Commun. Soil Sci. Plant Anal. 15, 1191–1213. doi: 10.1080/00103628409367551
Hallett, P. D., Feeney, D. S., Bengough, A. G., Rillig, M. C., Scrimgeour, C. M., and Young, I. M. (2009). Disentangling the impact of AM fungi versus roots on soil structure and water transport. Plant Soil 314, 183–196. doi: 10.1007/s11104-008-9717-y
Hanway, J. J., and Heidel, H. (1952). Soil analysis methods as used in Iowa state college soil testing laboratory. Iowa Agric. 57, 1–31,
Haynes, R. J., Swift, R. S., and Stephen, R. C. (1991). Influence of mixed cropping rotations (pasture-arable) on organic matter content, water stable aggregation and clod porosity in a group of soils. Soil Tillage Res. 19, 77–87. doi: 10.1016/0167-1987(91)90111-A
Kautz, T., Stumm, C., Kösters, R., and Köpke, U. (2010). Effects of perennial fodder crops on soil structure in agricultural headlands. J. Plant Nutr. Soil Sci. 173, 490–501. doi: 10.1002/jpln.200900216
Kemper, W. D., and Roseneau, R. C. (1986). “Aggregate stability and size distribution” in “Methods of soil analysis. Part I. Physical and mineralogical methods”. Soil properties and rice establishment method soil research 1005 agronomy monograph No. 9. ed. A. Klute (Madison, WI, USA: Soil Science Society of America Inc), 425–442.
Krüger, J. P., Leifeld, J., Glatzel, S., Szidat, S., and Alewell, C. (2015). Biogeochemical indicators of peatland degradation-a case study of a temperate bog in northern Germany. Biogeosciences 12, 2861–2871. doi: 10.5194/bg-12-2861-2015
Kumar, S., Bhatt, B. P., Dey, A., Shivani, S., Kumar, U., Idris, M. D., et al. (2018). Integrated farming system in India: current status, scope and future prospects in changing agricultural scenario. Indian J. Agric. Sci. 88, 1661–1675. doi: 10.56093/ijas.v88i11.84880
Leifeld, J., Heiling, M., and Hajdas, I. (2015). Age and Thermal Stability of Particulate Organic Matter Fractions Indicate the Presence of Black Carbon in Soil. Radiocarbon, 57, 99–107. doi: 10.2458/azu_rc.57.17964
Lenka, N. K., Choudhury, P. R., Sudhishri, S., Dass, A., and Patnaik, U. S. (2012). Soil aggregation, carbon buildup and root zone soil moisture in degraded sloping lands under selected agroforestry-based rehabilitation systems in eastern India. Agric. Ecosyst. Environ. 150, 54–62. doi: 10.1016/j.agee.2012.01.003
Li, J., Ramirez, G. H., Kiani, M., Quideau, S., Smith, E., Janzen, H., et al. (2018). Soil organic matter dynamics in long-term temperate agroecosystems: rotation and nutrient addition effects. Can. J. Soil Sci. 98, 232–245. doi: 10.1139/cjss-2017-0127
Martens, D. A. (2000). Management and crop residue influence soil aggregate stability. J. Environ. Qual. 29, 723–727. doi: 10.2134/jeq2000.00472425002900030006x
Maughan, M. W., Flores, J. P. C., Anghinoni, I., Bollero, G., Fernández, F. G., and Tracy, B. F. (2009). Soil quality and corn yield under crop–livestock integration in Illinois. Agron. J. 101, 1503–1510. doi: 10.2134/agronj2009.0068
McSherry, M. E., and Ritchie, M. E. (2013). Effects of grazing on grassland soil carbon: a global review. Glob. Change Biol. 19, 1347–1357. doi: 10.1111/gcb.12144
Meera, A. V., John, J., Sudha, B., Sajeena, A., Jacob, D., and Bindhu, J. S. (2019). Greenhouse gas emission from integrated farming system models: A comparative study. Green Farming 10, 696–701. doi: 10.37322/GreenFarming/10.6.2019.696-701
Mondal, S., Mishra, J. S., Poonia, S. P., Kumar, R., Dubey, R., Kumar, S., et al. (2021). Can yield, soil C and aggregation be improved under long-term conservation agriculture in the eastern I ndo-G angetic plain of India? Eur. J. Soil Sci. 72, 1742–1761. doi: 10.1111/ejss.13092
Olsen, S. R., Cole, C. V., Watanabe, F. S., and Dean, L. A. (1954). Estimation of available phosphorus in soils by extraction with sodium bicarbonate. USDA Circular 939. Washington, DC: U.S. Government Printing Office.
Paramesh, V., Ravisankar, N., Behera, U., Arunachalam, V., Kumar, P., Solomon Rajkumar, R., et al. (2022). Integrated farming system approaches to achieve food and nutritional security for enhancing profitability, employment, and climate resilience in India. Food Energy Secur. 11:e321. doi: 10.1002/fes3.321
Rao, K. K., Samal, S. K., Poonia, S. P., Kumar, R., Mishra, J. S., Bhatt, B. P., et al. (2022). Conservation agriculture improves soil physical properties and crop productivity: a long-term study in middle indo-Gangetic Plains of India. Soil Res. 60, 442–454. doi: 10.1071/SR20329
Sale, P., Tavakkoli, E., Armstrong, R., Wilhelm, N., Tang, C., Desbiolles, J., et al. (2021). Ameliorating dense clay subsoils to increase the yield of rain-fed crops. Adv. Agron. 165, 249–300. doi: 10.1016/bs.agron.2020.08.003
Salton, J. C., Mercante, F. M., Tomazi, M., Zanatta, J. A., Concenço, G., Silva, W. M., et al. (2014). Integrated crop-livestock system in tropical Brazil: toward a sustainable production system. Agric. Ecosyst. Environ. 190, 70–79. doi: 10.1016/j.agee.2013.09.023
Samal, S. K., Dwivedi, S. K., Rao, K. K., Choubey, A. K., Prakash, V., Kumar, S., et al. (2020). Five years’ exposure of elevated atmospheric CO2 and temperature enriched recalcitrant carbon in soil of subtropical humid climate. Soil Tillage Res. 203, 104707–104708. doi: 10.1016/j.still.2020.104707
Shaner, W. W., Philipp, P. F., and Schmehl, W. R. (1982). Farming systems research and development: guidelines for developing countries. Boulder: Westview.
Subbiah, B. V., and Asija, G. L. (1956). A rapid method for the estimation of available nitrogen in soils. Curr. Sci. 25, 259–260,
Sunderland, T. C. (2011). Food security: why is biodiversity important? Int. For. Rev. 13, 265–274. doi: 10.1505/146554811798293908
Tete-Mensah, I., Dowuona, G. N., Adiku, S. G. K., and Bonsu, M. (1999). Evaluation of the effects of some agroforestry practices on selected properties of soils in a sub-humid zone of Ghana. Ghana J. Agric. Sci. 32, 153–158. doi: 10.4314/gjas.v32i2.1896
Walia, S. S., Dhawan, V., Dhawan, A. K., and Ravisankar, N. (2019). Integrated Farming System: Enhancing Income Source for Marginal and Small Farmers. In: Peshin, R., Dhawan, A. (eds) Natural Resource Management: Ecological Perspectives. Sustainability in Plant and Crop Protection. Springer, Cham. doi: 10.1007/978-3-319-99768-1_5
Walkley, A., and Black, I. A. (1934). An examination of the Degtjareff method for determining soil organic matter and a proposed modification of the chromic acid titration method. Soil Sci. 37, 29–38. doi: 10.1097/00010694-193401000-00003
Keywords: IFS, crop rotation, carbon stock, aggregation indices, aggregate-associated carbon
Citation: Rao KK, Samal SK, Kumar S, Singh NR, Kumar R, Mondal S, Kumar S, Mishra JS, Bhatt BP, Ravisankar N, Kumar S, Upadhyay PK, Jadhav SK and Choubey AK (2024) Decade-long effects of integrated farming systems on soil aggregation and carbon dynamics in sub-tropical Eastern Indo-Gangetic plains. Front. Sustain. Food Syst. 8:1384082. doi: 10.3389/fsufs.2024.1384082
Edited by:
Mohamed Shokr, Tanta University, EgyptReviewed by:
Rajeev Padbhushan, Bihar Agricultural University, IndiaKailash Prajapat, Central Soil Salinity Research Institute (ICAR) Karnal, India
Ritesh Saha, Indian Council of Agricultural Research, Kolkata, India
Copyright © 2024 Rao, Samal, Kumar, Singh, Kumar, Mondal, Kumar, Mishra, Bhatt, Ravisankar, Kumar, Upadhyay, Jadhav and Choubey. This is an open-access article distributed under the terms of the Creative Commons Attribution License (CC BY). The use, distribution or reproduction in other forums is permitted, provided the original author(s) and the copyright owner(s) are credited and that the original publication in this journal is cited, in accordance with accepted academic practice. No use, distribution or reproduction is permitted which does not comply with these terms.
*Correspondence: Pravin Kumar Upadhyay, cHJhdmluLm5kdUBnbWFpbC5jb20=; Rakesh Kumar, cmFrZXNoYmh1MDhAZ21haWwuY29t