- 1School of Agriculture, Forest and Food Sciences, Bern University of Applied Sciences, Bern, Switzerland
- 2Division of Conservation Biology, Institute of Ecology and Evolution, University of Bern, Bern, Switzerland
We need landscape-scale approaches to design and manage agro-ecosystems that can sustain both agricultural production and biodiversity conservation. In this study, yield figures provided by 299 farmers served to quantify the energy-equivalents of food production across different crops in 49 1-km2 landscapes. Our results show that the relationship between bird diversity and food energy production depends on the proportion of farmland within the landscape, with a negative correlation observed in agriculture dominated landscapes (≥ 64–74% farmland). In contrast, neither typical farmland birds nor butterflies showed any significant relationship with total food energy production. We conclude that in European temperate regions consisting of small-scale, mixed farming systems (arable and livestock production), productivity and biodiversity conservation may not be purely antagonistic, particularly when (semi-)natural habitats make up a large fraction of the landscape (≥ 20%).
1 Introduction
Global agriculture production has more than doubled in the last 50 years and demand for food and agricultural products is foreseen to further increase in the next decades (Tilman et al., 2011; Ritchie, 2022). Corollary, agricultural practices strongly intensified and natural areas have undergone continued conversion to farmland (FAO, 2017). Specifically, at the field scale, the increased use of agrochemicals (e.g., mineral fertilizers and pesticides), mechanisation, and the use of high-yielding crop varieties have increased productivity. While at landscape scale field sizes have increased over time, farms have specialized on few crops (or even monocultures), permanent grasslands have been converted to arable fields, fallow lands have disappeared and semi-natural habitats such as field boundaries and hedgerows have been destroyed (Tscharntke et al., 2005). These land-use changes, have reduced, not only the biodiversity of natural habitats and traditional low-intensity agroecosystems, but also the flora and fauna of intensively used agroecosystems (Tscharntke et al., 2005; Sutcliffe et al., 2015; Warren et al., 2021; Rigal et al., 2023). Ironically, biodiversity is an important component for a sustainable long-term food production, as it supports a wide range of ecosystem services such as soil fertility, natural pest control and pollination (Pywell et al., 2015; Orford et al., 2016; Dainese et al., 2019; Albrecht et al., 2020; Gaba et al., 2020). With the increasing awareness on the consequences of farmland biodiversity loss and, at the same time, the need to ensure food production, research on the agricultural productivity-biodiversity frontier has considerably increased in the last two decades, with the focus moving from local- to landscape-scale processes (Tscharntke et al., 2012; Batary et al., 2020; Scherber, 2022).
A trade-off between agricultural production and biodiversity at landscape scale has been repeatedly demonstrated in tropical regions, where agriculture activities generally have detrimental effects on species typical of natural, habitats such as pristine forests (Phalan et al., 2011; Macchi et al., 2020; Wenzel et al., 2024). In Europe however, agricultural landscapes have developed over centuries and typically hold species dependent upon open or semi-open landscapes and adapted to a given level of land-use intensity (Burgi et al., 2015; Van Swaay et al., 2019; Boch et al., 2020). In these temperate regions, negative relationships have been evidenced in intensively managed arable and livestock production systems (Dross et al., 2017, 2018). Although Europe is characterized by a wide range of farming systems, landscape-scale studies from mixed, small-scale production systems are still rare (Feniuk et al., 2019). So far, most studies have either focused on the extent of farmland, or on the per unit area productivity, ignoring possible interactions between the two (e.g., Dross et al., 2018). This is regrettable, as structurally complex agricultural landscapes favour spatial connectivity and provide additional resources for farmland species (Villemey et al., 2015; Grass et al., 2019). Even butterflies, which typically depend upon farmland habitats, show the highest overall diversity in landscapes with a combination of farmed and semi-natural areas (Ouin and Burel, 2002; Zingg et al., 2018). The same is valid for birds, as many species require different habitats and a diversity of resources to complete their life cycles (Vickery and Arlettaz, 2012; Teillard et al., 2014). Consequently, structurally complex farmlands may compensate for local high-intensity management, leading to the productivity-biodiversity relationship being dependent on the extent of farmland within the landscapes (Tscharntke et al., 2005).
In this study, we analysed the relationship between agricultural productivity, defined as food energy production, and bird and butterfly diversity in 49 temperate agricultural landscapes of 1 km2 each. In order to compare agricultural yields across grasslands and different arable crops, food energy, was used as a common metric of production per unit area (Dross et al., 2018; Feniuk et al., 2019). Contrary to most other studies, which use reference yield data from regional or national agricultural statistics, we collected actual yield data from 299 farmers, thus capturing the spatial and temporal heterogeneity of agricultural yields (Butsic et al., 2020). Birds and butterflies were selected as model taxa because they have been proven to be good bioindicators of farmland biodiversity, influenced by changes in agricultural management and landscape composition (Zingg et al., 2018). In addition, typical farmland species of both taxa have shown a dramatic decline in the last few decades (Gregory et al., 2019; Van Swaay et al., 2019).
We expected the productivity-biodiversity relationships for birds and butterflies to be predominantly negative. Negative relationships have been repeatedly shown at the field scale, for example when comparing yield and biodiversity of organic and conventional farming systems (Gong et al., 2022), as well at the landscape scale where agricultural intensification is generally associated with the decline of bird and butterfly populations (Warren et al., 2021; Rigal et al., 2023). However, in landscapes with a higher degree of heterogeneity, we anticipated that the negative effect of locally highly productive agriculture could be mitigated by the presence of semi-natural areas (Persson et al., 2010; Botham et al., 2015; Batary et al., 2020; Redhead et al., 2020). Conversely, in landscapes dominated by farmland, we anticipated that an increase in agricultural production would have a stronger negative effect on the diversity and abundance of birds and butterflies (Ekroos et al., 2010; Dross et al., 2017; Zingg et al., 2018; Rigal et al., 2023). As land is limited and the demand for food rising, the conflict between agriculture and biodiversity conservation is likely to increase further and calls for more research on the topic (see also Grass et al., 2021).
2 Materials and methods
2.1 Study sites
The study was conducted on the Swiss lowland Plateau, the most important agricultural area and densely populated region of Switzerland (426 inhabitants per km2). The Biodiversity Monitoring Switzerland (BDM) conducts repeated biodiversity surveys in 520 systematically distributed landscape grid cells of 1 × 1 km across Switzerland (BDM Coordination Office, 2014). For this study, 49 BDM landscapes located on the Swiss lowland Plateau (altitude ranging from 400 to 800 m), with less than 25 ha of water bodies and paved areas were selected (electronic Supplementary material S1). For each of the 49 landscape grid cells (hereafter called landscapes), digitized information on land use was provided by the Swiss cadastral survey in 2014. The supplied GIS polygon layers were controlled and completed where necessary, using satellite images in ArcGIS (Version 10.2.2). Crop cover maps were provided by the cantonal agricultural offices in 2014. Because such maps were not available for some regions (cantons of Aargau and Vaud), these landscapes (n = 16) were visited and crops were mapped in summer 2016.
The study landscapes had on average (mean ± SD) 68 ± 16 hectares of farmland (ranging from 27 to 94 hectares) and were characterised by relatively small agricultural fields (mean field size was 1.32 ± 1.68 hectares). Farmers cultivated in total 12 different crop categories, with cereals, intensively managed grasslands and silage maize being the most abundant ones in terms of area cultivated (Figure 1). The landscapes showed a high level of crop diversity, visually represented in Figure 2. On average, there were 7.4 crops (± 2) present in each landscape, and the crop diversity, measured using the Shannon index based on the total area per crop category, was 1.34 (± 0.4).
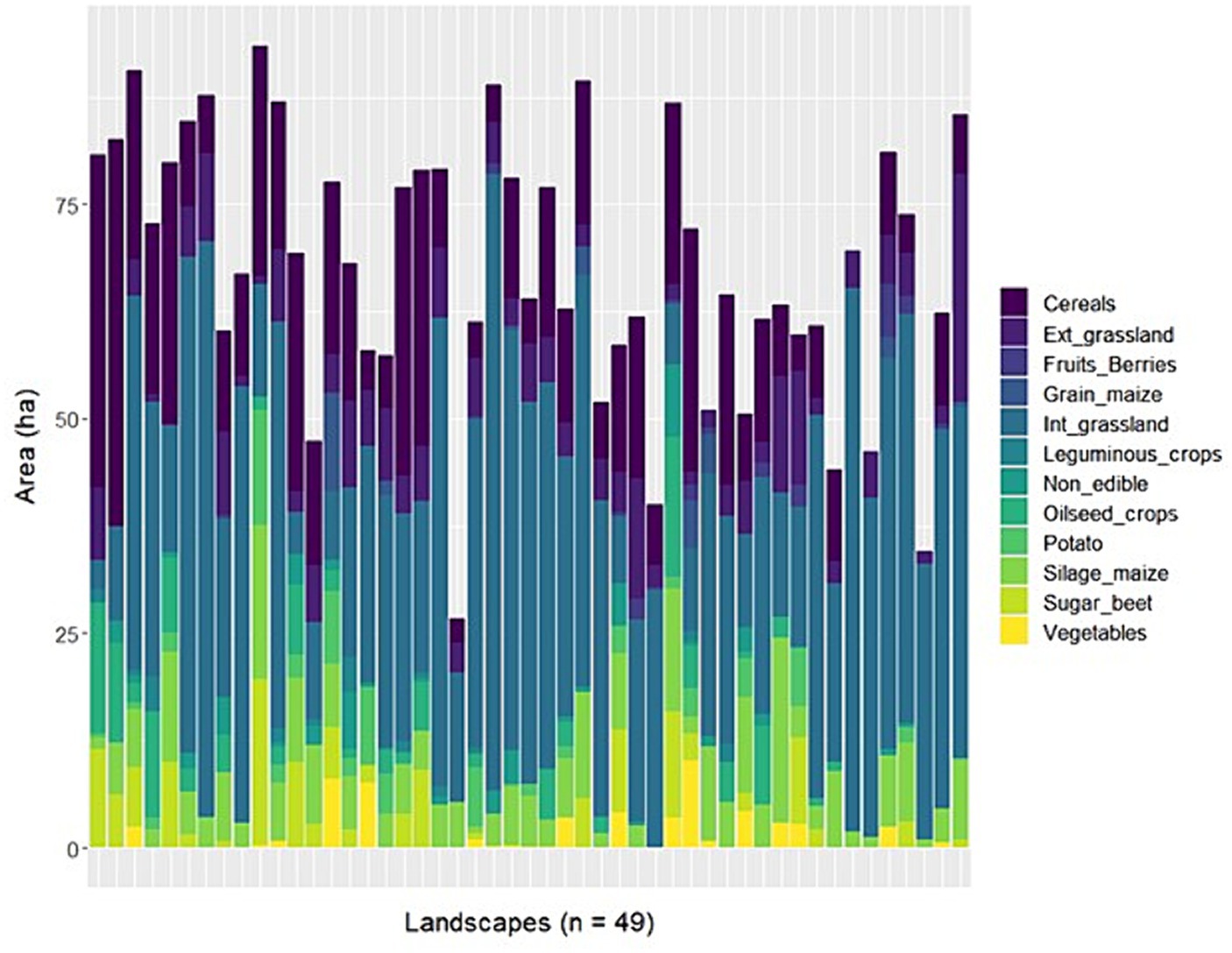
Figure 1. Composition of the 49 study landscapes showing the proportions (ha) of the different agricultural crops grouped in twelve categories. The non-farmed habitats (grey) consisted mainly of forests (mean ± SD = 15 ± 13 ha), impervious (e.g., buildings and streets, 8 ± 6 ha) and non-farmed vegetated areas (e.g., gardens, 3 ± 7 ha), and to a lesser extent of waterbodies, hedges and unvegetated areas (e.g., gravel, rock, sand). Ext., extensively managed; Int., intensively managed.
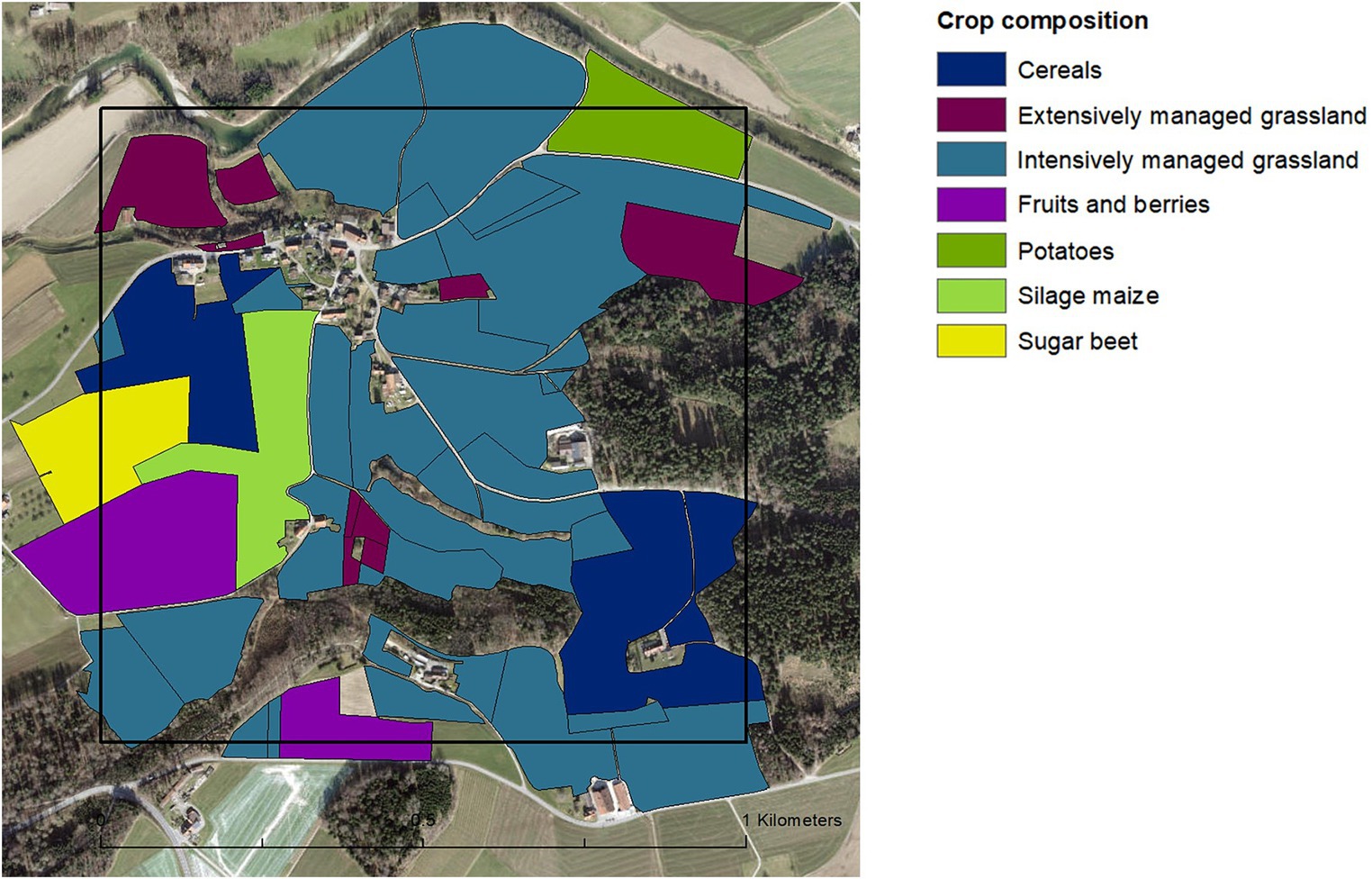
Figure 2. Example of a 1-km2 study landscape showing the variegated spatial agriculture configuration with relatively small fields and high crop diversity.
2.2 Biodiversity
Data on species richness and abundance of birds and butterflies were provided by the Swiss Biodiversity Monitoring and the Monitoring of common breeding birds. Repeated transect counts (seven times per sampling year for butterflies and three times for birds, conducted between April and September) were used to assess species presence in the landscapes. Surveys were conducted along transects of 2.5 km (BDM Coordination Office, 2014). For data analysis, birds and butterflies were classified into two groups: (1) all species pooled within the corresponding taxonomic group; and (2) typical farmland species. Complete species lists can be found in the Supplementary information (electronic Supplementary material S2). As total and farmland butterfly species richness and abundance were highly correlated (Pearson correlation coefficient > 0.9), results are only shown for total butterfly species richness and abundance.
2.3 Productivity
To estimate agricultural productivity, interviews with 299 farmers (in person or via questionnaires) were conducted. Farmers were asked to provide information on crop area, production system, yield (biomass), as well as the frequency of use (number of cuts and grazing events per year) for grasslands, over a three-year period (e.g., 2012–2014 or 2013–2015). Interviews led to a minimum of ten valid observations for yield and frequency of use per landscape.
2.3.1 Multiple imputation for missing yield values
Yield estimates were not available for all fields, either because farmers were not willing to participate in the survey (farmer participation ranged from 19 to 100% with an average of 68%, calculated as the percentage of agricultural area covered by the interview), or because yields were unknown (see electronic Supplementary material S3). Therefore, prior to the statistical analysis, we completed our yield dataset using Multiple Imputation (MI). As an advanced procedure for handling missing data, MI consists of estimating the missing data multiple times to create several complete versions of an incomplete dataset (van Buuren, 2012). We used predictive mean matching (PMM) from the R Package mice to impute the missing yield values and to create 50 completed datasets (van Buuren and Groothuis-Oudshoorn, 2011). The PMM procedure subsamples from the observed data and predicts the value of the target variable Y according to the specified imputation models:
i. Grassland yield = Grassland category + Frequency of use + Management + Year + Landscape + Region + Elevation
ii. Arable yield = Crop category + Management + Year + Landscape + Region + Elevation
The following predictors were included: grassland or crop category (the same as in Figure 1), the frequency of use for grasslands (number of cuts and grazing events per year), the management (organic, extensive or conventional) and the year (2012 to 2015). In addition, landscape (ID), elevation (meter above sea level), and the region (Swiss canton) were included. Because MI can generate implausible values (e.g., 200 dt/ha for wheat), we restricted the yield values after the imputation (post-processing), to the 1st and 3rd quartile of real yield values given by farmers. For more information on the missing yield values in general and the MI process see electronic Supplementary material S3.
2.3.2 Food energy-equivalent per landscape
For each of the 50 completed datasets, we calculated the mean crop yield per ha, averaging over all three sampling years and fields, within each landscape. Using this, we calculated the total food energy production P (in GJ of metabolizable energy ME per year), in each landscape for each imputed dataset k as follows:
Where, j refers to the study landscape and i to the crop category. X is the averaged crop yield (dt ha−1 year−1) from the imputed dataset, A the crop area (ha) from the agricultural survey or crop mapping, CF the conversion factor, which accounts for the losses during food processing or conversion (see electronic Supplementary material S4) and ME the content of metabolizable energy per unit weight of edible portion (GJ dt−1) from the Swiss Food Composition Database (FSVO, 2017). Non-edible crops, such as ornamental plants (e.g., Christmas trees), by-products such as straw, and landscape features such as wildflower strips, or hedgerows were attributed a food energy content of zero. In general, we accounted for one main crop per year (except on vegetable fields, where we accounted for two harvests per year), while catch crops covering the soil during winter were not included in the productivity estimates.
2.3.3 Crop-use scenarios
We calculated total food energy production per landscape for two scenarios. In scenario 1, we assumed that all crops would be converted in an edible form and directly consumed by humans, except for fodder crops (i.e., silage maize and grass) which were expressed as the energy-equivalent of edible meat (in GJ) produced per unit weight. In scenario 2 (a more realistic estimation of joules produced for human consumption), we accounted for the fact that some edible crops are also used as animal feed; in cereals, for example, a share of 42% is used as animal feed in Switzerland, mostly to produce meat (Bundesamt für Statistik, 2016). We included the two scenarios to consider the aspects of the feed/food debate and the influence this has on the ultimate human food production of agricultural landscapes (Mottet et al., 2017). Information on the use of the crops in the two scenarios and the energetic values of the products can be found in the electronic Supplementary material S4.
2.4 Statistical analysis
Species richness, abundance and Pielou’s evenness index of birds and butterflies were used as response variables. While models were run on total and farmland species richness and abundances, Pielou’s evenness was calculated for total birds and butterflies. Food energy production per landscape in gigajoule (GJ) and the amount of farmland in hectare (ha) were included as explanatory variables. We used the following generalized linear models (GLM) with Poisson (for species richness), negative binomial (for abundance) or Gaussian (for Pielou’s evenness) distributions:
The interaction was removed if not significant. The regression models were fitted to the n (= 50) imputed datasets and the model results were pooled using the R Package mitools (Lumley, 2015). Hereby, for logistic regression modelling in combination with MI, the pooled regression coefficients and standard errors were obtained by using Rubin’s Rule (Rubin, 1976). The pooled coefficient was derived by averaging the regression coefficient estimates from each complete data analysis result across the imputed datasets. The standard error was obtained by pooling the variance between as well as within imputations, which account for sampling and imputation uncertainty, respectively (Eekhout et al., 2017). The variability between the imputations reflects the uncertainty of the actual value (van Buuren, 2012).
Finally, in models where the interaction term between food energy production and proportion of farmland was significant, the threshold, i.e., when the trend changes sign due to the proportion of farmland in the landscape (or in other words when food energy production has no influence on the response variable) was computed using model outputs.
3 Results
Total food energy produced (given as metabolizable energy for human consumption) averaged to 2′344 GJ (± 1′958) per 1-km2 landscape and year for scenario 1 (all food energy production directly consumed by humans) and 1′921 GJ (± 1′713) for scenario 2 (part of the production used as animal feed to produce meat, electronic Supplementary material S5). Computed per hectare of farmland, food energy production averaged to 33 GJ/ha (± 24) for scenario 1 and 27 GJ/ha (± 21) for scenario 2. These food energy figures provide a landscape-scale measure of agricultural production reflecting the proportion of the landscape that is farmed, the types of crops grown within the landscape and the in-field yield of those crops. In other words, at the landscape scale the proportion of farmland, the share of highly productive crops (such as sugar beet or potatoes) correlated with the total food energy produced (see electronic Supplementary material S5). Note that the food energy figures given by scenarios 1 and 2 were highly correlated (r = 0.99).
3.1 Relationship between biodiversity and food energy
Results showed a significant interaction effect between food energy production and the extent of farmland. The nature of the interaction indicates that the relationship between productivity and overall bird richness, abundance and evenness varies depending on the amount of farmland within the landscapes (Table 1). Hereby, overall bird species richness and abundance decreased with food energy production in landscapes with high shares of farmland (i.e., ≥ 74 or ≥ 72 ha respectively), but increased in landscapes with lesser fractions of farmland (Figure 3). The same pattern was observed for total bird evenness, where the threshold at which the regression changed from positive to negative was at 64 ha of farmland per landscape. Farmland birds were analysed separately as a subgroup, however no significant effects on species richness or abundance were detected (Table 1). Further analyses revealed that although some farmland bird species such as the Eurasian Skylark (Alauda arvensis) responded positively to food energy production, most species had a neutral or slightly negative response (electronic Supplementary material S7).
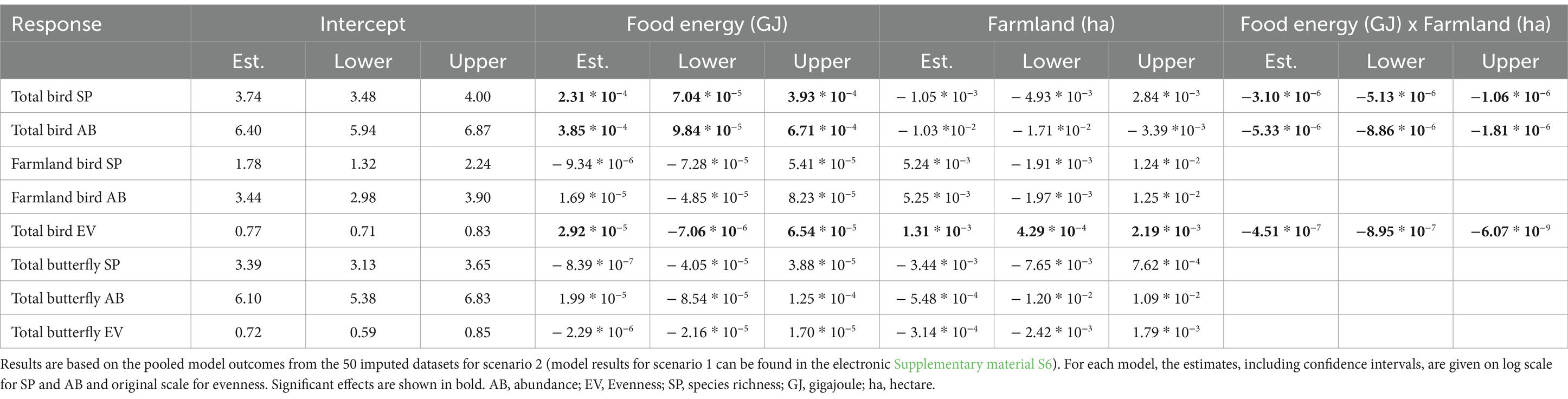
Table 1. Summary of the models showing the relationships between birds and butterflies and agricultural productivity given as total food energy produced.
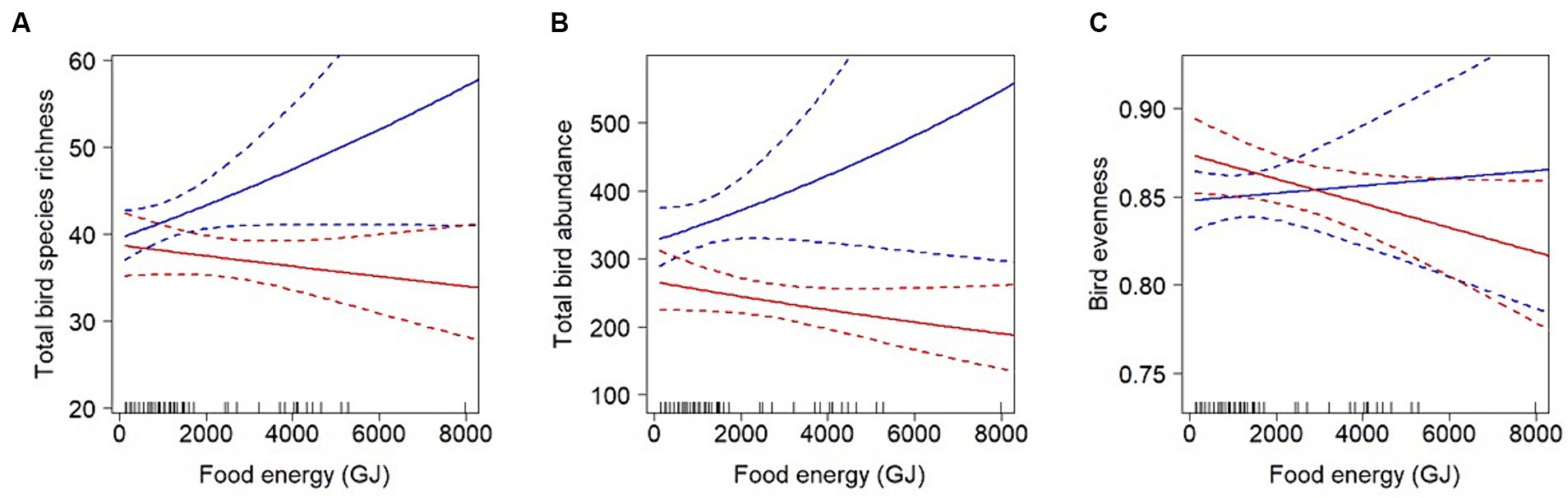
Figure 3. The relationship between bird species richness (A), abundance (B), evenness (C) and food energy-equivalents (given as metabolizable energy for human consumption, i.e., scenario 2) depends on the amount of farmland within the respective 1-km2 landscape (see Table 1 for the parameters of the linear models). The figure shows the predicted regression lines for landscapes with 60 ha (blue) and 80 ha of farmland (red) that fall below or above, respectively, the threshold where the regression line changes slope. Threshold values are at 74, 72 and 64 ha of farmland for total bird species richness, abundance and evenness, respectively. Shown are pooled predictions with 95% confidence intervals from the (n = 50) models. The means of the imputed food energy values in gigajoule (GJ) are shown as tick marks at the bottom. Relationships with butterflies were not significant and can be found in the electronic Supplementary material S6.
No significant relationship between butterfly diversity or abundance, and food energy production at landscape scale was found (electronic Supplementary material S6). Single species analyses confirmed that most farmland butterflies responded neutrally to food energy production with four exceptions; namely the Ringlet (Aphantopus hyperantus) the Queen of Spain Fritillary (Issoria lathonia) and the Large Skipper (Ochlodes sylvanus) that significantly decreased with food energy production, and the Essex skipper (Thymelicus lineola) that significantly increased with food energy production.
4 Discussion
So far agricultural productivity-biodiversity studies were mostly conducted at field scale. The novelty of the present study lies in the fact that it was conducted at the landscape scale (1 × 1 km plots, equivalent to 100 ha) and was based on real yield information. Contrary to our expectations, observed relationships between total food energy produced and biodiversity per landscape were not predominantly negative, indicating that in intensively managed but small-scale mixed farmland, food production and biodiversity conservation are not necessarily incompatible.
In our studied Swiss lowland landscapes, mean productivity (food energy production) averaged to 33 GJ/ha (± 24) for scenario 1 and 27 GJ/ha (± 21) for scenario 2. In comparison with other European studies, it represents intermediate agricultural systems, accounting neither for the very low-yield extensively managed grassland-based systems (as found in Poland, Feniuk et al., 2019) nor for the very high-productivity, industrialized, monocultural systems (as found in France, Dross et al., 2017). Thus, unsurprisingly, farmland sensitive species adapted to very low-productivity systems are absent from our datasets. Such species, like the corn crake (Crex crex) and the woodchat shrike (Lanius senator), gradually vanished from the Swiss lowland decades ago (Keller et al., 2010). The range of productivity levels in our study system is also limited: all our study sites contained at least 27% of farmland (as shown in Figure 1). Subsequently we do not discuss our results in the light of the land sparing-sharing model, because this would require data on the density of wild species across a range of agricultural yields, including 100% unfarmed, or natural landscapes (Phalan, 2018). Therefore, we emphasize that conclusions drawn from this study are mostly valid in currently farmed European temperate regions with intermediate agricultural productivity and similar agri-environmental policies as implemented in Switzerland (see related paragraph in the next subsection).
4.1 Relationship between biodiversity and food energy
While the aspects of agricultural productivity and the extent of farmland are in general separately analysed (Jeliazkov et al., 2016; Dross et al., 2017), we show here that there exists a significant interaction between these two aspects. In landscapes characterized by a high proportion of farmland (≥ 64–74%), we observed a negative relationship between overall bird richness, abundance, evenness, and food energy production. This can be attributed to the limited availability of habitat elements (e.g., nesting sites) in cleared, agriculture-dominated landscapes (Tscharntke et al., 2012; Batary et al., 2020). Moreover, when agricultural areas are intensively managed, the depletion of food resources (e.g., invertebrates) exacerbates the negative impact on bird biodiversity (Vickery and Arlettaz, 2012; Rigal et al., 2023).
Conversely, we found that an increase in food energy production positively correlated with bird biodiversity in landscapes with lower proportions of farmland (≤ 64–74%). This suggests that the replacement of some low-energy yield with high-energy yield crop types, or the transitioning from grassland-dominated landscapes to mixed grassland and cereal landscapes, can enhance habitat heterogeneity and resource availability for birds on farmland. In landscapes with lower shares of farmland, the presence of other semi-natural or man-made habitats, such as forests, hedges, or settlements, further contributes to habitat complexity. These structurally diverse landscapes not only promote local diversity in agroecosystems, particularly for mobile organisms (Zingg et al., 2018; Redhead et al., 2020; Kühne et al., 2022), but also potentially offset the negative effects of within field high-intensity management practices (Tscharntke et al., 2005; Batary et al., 2020).
The depicted relationships between bird evenness and food energy production indicate changes in species dominance when productivity increases, highlighting that there is no optimal land-use intensity and configuration that will maximize all species (Teillard et al., 2014).
We did not find a significant relationship between butterfly diversity or abundance, and food energy production at landscape scale. Neutral productivity relationships for butterflies were mostly described in tropical agroforestry systems, where crops such as vanilla, coffee or cacao are produced under shade trees in spatially combined and complex systems, which can provide both high yield and biodiversity (Clough et al., 2011; Wurz et al., 2022). In temperate agro-ecosystems, predominantly characterized by monocultures of grasslands and arable fields, productivity is maintained at high levels through agricultural inputs, which often reduce biodiversity (Kleijn et al., 2009; Gong et al., 2022). Nonetheless, in our system, several aspects may explain the observed neutral relationships between food energy production and butterfly biodiversity: (i) productivity in GJ does not equal agricultural intensity; (ii) current agroecological measures, including in as well as out of production agri-environment schemes, effectively maintain biodiversity; (iii) biodiversity supports productivity. The three points are described more in detail in the following paragraphs.
i. There is no doubt that agriculture has a strong influence on biodiversity. However, it is not agricultural productivity per se, but management practices (e.g., soil work, fertilizer and pesticide input), fields size, crop identity and crop diversity which mostly influence biodiversity (Kremen, 2015; Hass et al., 2018; Sirami et al., 2019). While in-field productivity strongly depends on management intensity (e.g., extensively vs. intensively managed grasslands, Kleijn et al., 2009; Boch et al., 2021), landscape productivity is strongly linked to the composition of the landscape. In our mixed agricultural landscapes, productivity increased with the share of farmland and of crops with high energetic values and high yields (i.e., sugar beets, potatoes and cereals, see electronic Supplementary material S5). Whereas high in-field productivity does imply high management intensity (e.g., higher cereal yield due to higher fertilizer and pesticide application), higher landscape-scale productivity cannot be directly linked to crop management practices.
ii. Agricultural policy in Switzerland follows the framework of environmental cross compliance (Aviron et al., 2009; Swiss Federal Council, 2013). Sustainable agricultural practices such as intercropping, crop rotations or reduced agrochemical use aim to reduce environmental impact and safeguard production. In addition, Swiss farmers have to dedicate at least 7% of their land to wildlife-friendly agri-environment schemes (at the time of the study, 13% of the Swiss lowland farmland was managed under such schemes). These schemes, which include, for example, extensively managed grasslands and wildflower strips, have been shown to promote farmland biodiversity, including butterflies, at local (Aviron et al., 2009; Bruppacher et al., 2016) and landscape scale (Zingg et al., 2019). Moreover, many farms in our study region still combine livestock and crop production, meaning that our landscapes all display a matrix combining grassland and arable fields (Figure 1). Although arable and grassland specialist species thrive in regions dominated by either production system, most species prefer mixed landscapes (Botham et al., 2015; Dross et al., 2018).
iii. It is intuitively assumed that the presence of natural or low-intensity managed areas promotes biodiversity at the cost of agricultural productivity because it excludes land from production and reduces local yield, respectively. However, there is more and more evidence of biodiversity-mediated benefits to agricultural production (Batary et al., 2020). For example, it was demonstrated in a UK field-scale study that wildlife-friendly habitats that promote pollinators and other beneficial organisms can increase yield per unit area, compensating for the land that was taken out of production (Pywell et al., 2015). Similarly, it has been shown that crop yield resilience is positively related to semi-natural habitats in the landscape (Redhead et al., 2020). At local scale, it has been known for a long time that phytomass production is higher and more stable in species-rich grasslands (Hautier et al., 2014). However, the reliance of modern agriculture on intensive management such as the prophylactic use of agrochemicals may mask (or even suppress) potential benefits from ecosystem services (Gagic et al., 2017), also in our system.
5 Conclusion
The main finding of this study is that in temperate mixed agricultural landscapes, high agricultural production, in terms of joules produced per 1-km2 landscape (100 ha), is not necessarily incompatible with high biodiversity. While total bird species richness, abundance and diversity were negatively correlated with agricultural production in landscapes dominated by farmland, we found no relationship in landscapes with a share of ≥30–40% of non-farmed habitats. In addition, and more surprisingly, neither farmland birds, nor butterflies were correlated with total food energy production. Although it is not possible to establish any causality from our analyses, non-farmed areas such as forest patches and hedges (semi-natural habitats represented usually ≥20% of the studied landscapes), small fields (field size averaged 1.32 ha), wildlife-friendly agri-environment schemes and high crop diversity, seem to mitigate the negative influence of intensive and highly productive in-field management practices (Konvicka et al., 2016; Grass et al., 2019; Sirami et al., 2019; Zingg et al., 2019; Batary et al., 2020). In such small scale, well connected heterogeneous landscapes, the productivity-biodiversity trade-off may be less pronounced or absent. In conclusion, as the main purpose of agriculture is to produce food for human consumption, it is promising to see that there are ways to design multi-functional agro-ecosystems that support both biodiversity and agricultural food production (Batary et al., 2020; Finch et al., 2020).
Data availability statement
The original contributions presented in the study are publicly available. This data can be found here: https://doi.org/10.5061/dryad.hmgqnk9nf.
Author contributions
SZ: Conceptualization, Data curation, Formal analysis, Funding acquisition, Investigation, Methodology, Project administration, Resources, Validation, Visualization, Writing – original draft, Writing – review & editing. JG: Conceptualization, Methodology, Writing – review & editing. J-YH: Conceptualization, Funding acquisition, Methodology, Project administration, Resources, Supervision, Validation, Visualization, Writing – review & editing.
Funding
The author(s) declare that financial support was received for the research, authorship, and/or publication of this article. We thank the foundations Sur-la-Croix and Temperatio as well as the Canton of Argovia for their financial support. Open access funding by University of Bern.
Acknowledgments
We thank the Swiss Ornithological Institute, the Swiss Biodiversity Monitoring, Hintermann & Weber and the cantonal offices of agriculture for data provision. Further thank goes to Annika Winzeler, Christian Dougoud, Michael Hüsler and Alan Storelli for help with data preparation, Julia Menk for support in database and Christoph Kopp for statistical advice. Special thanks goes to all the farmers taking part in our study.
Conflict of interest
The authors declare that the research was conducted in the absence of any commercial or financial relationships that could be construed as a potential conflict of interest.
Publisher’s note
All claims expressed in this article are solely those of the authors and do not necessarily represent those of their affiliated organizations, or those of the publisher, the editors and the reviewers. Any product that may be evaluated in this article, or claim that may be made by its manufacturer, is not guaranteed or endorsed by the publisher.
Supplementary material
The Supplementary material for this article can be found online at: https://www.frontiersin.org/articles/10.3389/fsufs.2024.1377369/full#supplementary-material
References
Albrecht, M., Kleijn, D., Williams, N. M., Tschumi, M., Blaauw, B. R., Bommarco, R., et al. (2020). The effectiveness of flower strips and hedgerows on pest control, pollination services and crop yield: a quantitative synthesis. Ecol. Lett. 23, 1488–1498. doi: 10.1111/ele.13576
Aviron, S., Nitsch, H., Jeanneret, P., Buholzer, S., Luka, H., Pfiffner, L., et al. (2009). Ecological cross compliance promotes farmland biodiversity in Switzerland. Front. Ecol. Environ. 7, 247–252. doi: 10.1890/070197
Batary, P., Baldi, A., Ekroos, J., Gallé, R., Grass, I., and Tscharntke, T. (2020). Biologia Futura: landscape perspectives on farmland biodiversity conservation. Biologia Futura 71, 9–18. doi: 10.1007/s42977-020-00015-7
BDM Coordination Office (2014). “Swiss biodiversity monitoring BDM. Description of methods and indicators” in Environmental studies (Bern, Switzerland: BDM Coordination Office).
Boch, S., Biurrun, I., and Rodwell, J. (2020). “Grasslands of western Europe” in Encyclopedia of the World's biomes. eds. M. I. Goldstein, D. A. DellaSala, and D. A. DiPaolo (Amsterdam: Elsevier).
Boch, S., Kurtogullari, Y., Allan, E., Lessard-Therrien, M., Rieder, N. S., Fischer, M., et al. (2021). Effects of fertilization and irrigation on vascular plant species richness, functional composition and yield in mountain grasslands. J. Environ. Manag. 279:111629. doi: 10.1016/j.jenvman.2020.111629
Botham, M. S., Fernandez-Ploquin, E. C., Brereton, T., Harrower, C. A., Roy, D. B., and Heard, M. S. (2015). Lepidoptera communities across an agricultural gradient: how important are habitat area and habitat diversity in supporting high diversity? J. Insect Conserv. 19, 403–420. doi: 10.1007/s10841-015-9760-y
Bruppacher, L., Pellet, J., Arlettaz, R., and Humbert, J.-Y. (2016). Simple modifications of mowing regime promote butterflies in extensively managed meadows: evidence from field-scale experiments. Biol. Conserv. 196, 196–202. doi: 10.1016/j.biocon.2016.02.018
Bundesamt für Statistik (2016). Landwirtschaft und Ernährung, Taschenstatistik 2016. Neuchâtel, Switzerland: Bundesamt für Statistik BFS.
Burgi, M., Salzmann, D., and Gimmi, U. (2015). 264 years of change and persistence in an agrarian landscape: a case study from the Swiss lowlands. Landsc. Ecol. 30, 1321–1333. doi: 10.1007/s10980-015-0189-1
Butsic, V., Kuemmerle, T., Pallud, L., Helmstedt, K. J., Macchi, L., and Potts, M. D. (2020). Aligning biodiversity conservation and agricultural production in heterogeneous landscapes. Ecol. Appl. 30:e02057. doi: 10.1002/eap.2057
Clough, Y., Barkmann, J., Juhrbandt, J., Kessler, M., Wanger, T. C., Anshary, A., et al. (2011). Combining high biodiversity with high yields in tropical agroforests. Proc. Natl. Acad. Sci. 108, 8311–8316. doi: 10.1073/pnas.1016799108
Dainese, M., Martin, E. A., Aizen, M. A., Albrecht, M., Bartomeus, I., Bommarco, R., et al. (2019). A global synthesis reveals biodiversity-mediated benefits for crop production. Science. Advances 5:eaax0121. doi: 10.1126/sciadv.aax0121
Dross, C., Jiguet, F., and Tichit, M. (2017). Concave trade-off curves between crop production and taxonomic, functional and phylogenetic diversity of birds. Ecol. Indic. 79, 83–90. doi: 10.1016/j.ecolind.2017.03.046
Dross, C., Princé, K., Jiguet, F., and Tichit, M. (2018). Contrasting bird communities along production gradients of crops and livestock in French farmlands. Agric. Ecosyst. Environ. 253, 55–61. doi: 10.1016/j.agee.2017.10.025
Eekhout, I., van de Wiel, M. A., and Heymans, M. W. (2017). Methods for significance testing of categorical covariates in logistic regression models after multiple imputation: power and applicability analysis. BMC Med. Res. Methodol. 17:129. doi: 10.1186/s12874-017-0404-7
Ekroos, J., Heliölä, J., and Kuussaari, M. (2010). Homogenization of lepidopteran communities in intensively cultivated agricultural landscapes. J. Appl. Ecol. 47, 459–467. doi: 10.1111/j.1365-2664.2009.01767.x
Feniuk, C., Balmford, A., and Green, R. E. (2019). Land sparing to make space for species dependent on natural habitats and high nature value farmland. Proc. R. Soc. B Biol. Sci. 286:20191483. doi: 10.1098/rspb.2019.1483
Finch, T., Green, R. E., Massimino, D., Peach, W. J., and Balmford, A. (2020). Optimising nature conservation outcomes for a given region-wide level of food production. J. Appl. Ecol. 57, 985–994. doi: 10.1111/1365-2664.13594
Gaba, S., Cheviron, N., Perrot, T., Piutti, S., Gautier, J.-L., and Bretagnolle, V. (2020). Weeds enhance multifunctionality in arable lands in south-west of France. Front. Sustain. Food Syst. 4:71. doi: 10.3389/fsufs.2020.00071
Gagic, V., Kleijn, D., Báldi, A., Boros, G., Jørgensen, H. B., Elek, Z., et al. (2017). Combined effects of agrochemicals and ecosystem services on crop yield across Europe. Ecol. Lett. 20, 1427–1436. doi: 10.1111/ele.12850
Gong, S., Hodgson, J. A., Tscharntke, T., Liu, Y., van der Werf, W., Batáry, P., et al. (2022). Biodiversity and yield trade-offs for organic farming. Ecol. Lett. 25, 1699–1710. doi: 10.1111/ele.14017
Grass, I., Batáry, P., and Tscharntke, T. (2021). “Chapter Six—Combining land-sparing and land-sharing in European landscapes” in Advances in ecological research, Eds. D. A. Bohan and A. J. Vanbergen. (New York: Academic Press), 251–303.
Grass, I., Loos, J., Baensch, S., Batary, P., Libran-Embid, F., Ficiciyan, A., et al. (2019). Land-sharing/−sparing connectivity landscapes for ecosystem services and biodiversity conservation. People Nat. 1, 262–272. doi: 10.1002/pan3.21
Gregory, R. D., Skorpilova, J., Vorisek, P., and Butler, S. (2019). An analysis of trends, uncertainty and species selection shows contrasting trends of widespread forest and farmland birds in Europe. Ecol. Indic. 103, 676–687. doi: 10.1016/j.ecolind.2019.04.064
Hass, A. L., Kormann, U. G., Tscharntke, T., Clough, Y., Baillod, A. B., Sirami, C., et al. (2018). Landscape configurational heterogeneity by small-scale agriculture, not crop diversity, maintains pollinators and plant reproduction in western Europe. Proc. R. Soc. B Biol. Sci. 285:20172242. doi: 10.1098/rspb.2017.2242
Hautier, Y., Seabloom, E. W., Borer, E. T., Adler, P. B., Harpole, W. S., Hillebrand, H., et al. (2014). Eutrophication weakens stabilizing effects of diversity in natural grasslands. Nature 508, 521–525. doi: 10.1038/nature13014
Jeliazkov, A., Mimet, A., Chargé, R., Jiguet, F., Devictor, V., and Chiron, F. (2016). Impacts of agricultural intensification on bird communities: new insights from a multi-level and multi-facet approach of biodiversity. Agric. Ecosyst. Environ. 216, 9–22. doi: 10.1016/j.agee.2015.09.017
Keller, V., Gerber, A., Schmid, H., Volet, B., and Zbinden, N. (2010). Rote Liste Brutvögel. Gefährdete Arten der Schweiz, Stand 2010. Bundesamt für Umwelt, Bern, and Schweizerische Vogelwarte, Sempach. Umwelt-Vollzug, 1019.
Kleijn, D., Kohler, F., Báldi, A., Batáry, P., Concepción, E., Clough, Y., et al. (2009). On the relationship between farmland biodiversity and land-use intensity in Europe. Proc. R. Soc. B Biol. Sci. 276, 903–909. doi: 10.1098/rspb.2008.1509
Konvicka, M., Benes, J., and Polakova, S. (2016). Smaller fields support more butterflies: comparing two neighbouring European countries with different socioeconomic heritage. J. Insect Conserv. 20, 1113–1118. doi: 10.1007/s10841-016-9940-4
Kremen, C. (2015). Reframing the land-sparing/land-sharing debate for biodiversity conservation. Ann. N. Y. Acad. Sci. 1355, 52–76. doi: 10.1111/nyas.12845
Kühne, I., Arlettaz, R., and Humbert, J. Y. (2022). Landscape woody features, local management and vegetation composition shape moth communities in extensively managed grasslands. Insect Conserv. Divers. 15, 739–751. doi: 10.1111/icad.12600
Lumley, T. (2015). “Mitools: tools for multiple imputation of missing data” in R Package. version 2.3 ed. Available at: https://CRAN.R-project.org/package=mitools
Macchi, L., Decarre, J., Goijman, A. P., Mastrangelo, M., Blendinger, P. G., Gavier-Pizarro, G. I., et al. (2020). Trade-offs between biodiversity and agriculture are moving targets in dynamic landscapes. J. Appl. Ecol. 57, 2054–2063. doi: 10.1111/1365-2664.13699
Mottet, A., de Haan, C., Falcucci, A., Tempio, G., Opio, C., and Gerber, P. (2017). Livestock: on our plates or eating at our table? A new analysis of the feed/food debate. Glob. Food Sec. 14, 1–8. doi: 10.1016/j.gfs.2017.01.001
Orford, K. A., Murray, P. J., Vaughan, I. P., and Memmott, J. (2016). Modest enhancements to conventional grassland diversity improve the provision of pollination services. J. Appl. Ecol. 53, 906–915. doi: 10.1111/1365-2664.12608
Ouin, A., and Burel, F. (2002). Influence of herbaceous elements on butterfly diversity in hedgerow agricultural landscapes. Agric. Ecosyst. Environ. 93, 45–53. doi: 10.1016/S0167-8809(02)00004-X
Persson, A. S., Olsson, O., Rundlof, M., and Smith, H. G. (2010). Land use intensity and landscape complexity-analysis of landscape characteristics in an agricultural region in southern Sweden. Agric. Ecosyst. Environ. 136, 169–176. doi: 10.1016/j.agee.2009.12.018
Phalan, B. T. (2018). What have we learned from the land sparing-sharing model? Sustain. For. 10:24. doi: 10.3390/su10061760
Phalan, B., Onial, M., Balmford, A., and Green, R. E. (2011). Reconciling food production and biodiversity conservation: land dharing and land dparing compared. Science 333, 1289–1291. doi: 10.1126/science.1208742
Pywell, R. F., Heard, M. S., Woodcock, B. A., Hinsley, S., Ridding, L., Nowakowski, M., et al. (2015). Wildlife-friendly farming increases crop yield: evidence for ecological intensification. Proc. R. Soc. B Biol. Sci. 282:20151740. doi: 10.1098/rspb.2015.1740
Redhead, J. W., Oliver, T. H., Woodcock, B. A., and Pywell, R. F. (2020). The influence of landscape composition and configuration on crop yield resilience. J. Appl. Ecol. 57, 2180–2190. doi: 10.1111/1365-2664.13722
Rigal, S., Dakos, V., Alonso, H., Aunins, A., Benko, Z., Brotons, L., et al. (2023). Farmland practices are driving bird population decline across Europe. Proc. Natl. Acad. Sci. USA 120:e2216573120. doi: 10.1073/pnas.2216573120
Ritchie, H. (2022). After millennia of agricultural expansion, the world has passed ‘peak agricultural land’ : Our World in Data. Available at: https://ourworldindata.org/peak-agriculture-land
Rubin, D. B. (1976). Inference and missing data. Biometrika 63, 581–592. doi: 10.1093/biomet/63.3.581
Scherber, C. (2022). Agroecology- reconciling biodiversity and production in farming systems. Basic Appl. Ecol. 65, 62–66. doi: 10.1016/j.baae.2022.10.002
Sirami, C., Gross, N., Baillod, A. B., Bertrand, C., Carrié, R., Hass, A., et al. (2019). Increasing crop heterogeneity enhances multitrophic diversity across agricultural regions. Proc. Natl. Acad. Sci. 116, 16442–16447. doi: 10.1073/pnas.1906419116
Sutcliffe, L., Batáry, P., Kormann, U., Báldi, A., Dicks, L. V., Herzon, I., et al. (2015). Harnessing the biodiversity value of central and eastern European farmland. Divers. Distrib. 21, 722–730. doi: 10.1111/ddi.12288
Swiss Federal Council (2013). Ordonnance sur les paiements directs versés dans l’agriculture. RS 910.13. Switzerland: Bern.
Teillard, F., Antoniucci, D., Jiguet, F., and Tichit, M. (2014). Contrasting distributions of grassland and arable birds in heterogenous farmlands: implications for conservation. Biol. Conserv. 176, 243–251. doi: 10.1016/j.biocon.2014.06.001
Tilman, D., Balzer, C., Hill, J., and Befort, B. L. (2011). Global food demand and the sustainable intensification of agriculture. Proc. Natl. Acad. Sci. 108, 20260–20264. doi: 10.1073/pnas.1116437108
Tscharntke, T., Klein, A. M., Kruess, A., Steffan-Dewenter, I., and Thies, C. (2005). Landscape perspectives on agricultural intensification and biodiversity - ecosystem service management. Ecol. Lett. 8, 857–874. doi: 10.1111/j.1461-0248.2005.00782.x
Tscharntke, T., Tylianakis, J. M., Rand, T. A., Didham, R. K., Fahrig, L., Batáry, P., et al. (2012). Landscape moderation of biodiversity patterns and processes - eight hypotheses. Biol. Rev. 87, 661–685. doi: 10.1111/j.1469-185X.2011.00216.x
van Buuren, S., and Groothuis-Oudshoorn, K. (2011). Mice: multivariate imputation by chained equations in R. J. Stat. Softw. 45, 1–67. doi: 10.18637/jss.v045.i03
Van Swaay, C., Dennis, E., Schmucki, R., Sevilleja, C., Balalaikins, M., Botham, M., et al. (2019). The EU butterfly Indicator for grassland species: 1990–2017: Technical report : Butterfly Conservation Europe & ABLE/eBMS. Available at: https://butterfly-monitoring.net/
Vickery, J. A., and Arlettaz, R. (2012). “The importance of habitat heterogeneity at multiple scales for birds in European agricultural landscapes” in Birds and habitat: Relationships in changing landscapes. ed. R. J. Fuller (Cambridge, UK: Cambridge University Press), 177–204.
Villemey, A., van Halder, I., Ouin, A., Barbaro, L., Chenot, J., Tessier, P., et al. (2015). Mosaic of grasslands and woodlands is more effective than habitat connectivity to conserve butterflies in French farmland. Biol. Conserv. 191, 206–215. doi: 10.1016/j.biocon.2015.06.030
Warren, M. S., Maes, D., van Swaay, C. A. M., Goffart, P., Van Dyck, H., Bourn, N. A. D., et al. (2021). The decline of butterflies in Europe: problems, significance, and possible solutions. Proc. Natl. Acad. Sci. USA 118:10. doi: 10.1073/pnas.2002551117
Wenzel, A., Westphal, C., Ballauff, J., Berkelmann, D., Brambach, F., Buchori, D., et al. (2024). Balancing economic and ecological functions in smallholder and industrial oil palm plantations. Proc. Natl. Acad. Sci. 121:e2307220121. doi: 10.1073/pnas.2307220121
Wurz, A., Tscharntke, T., Martin, D. A., Osen, K., Rakotomalala, A., Raveloaritiana, E., et al. (2022). Win-win opportunities combining high yields with high multi-taxa biodiversity in tropical agroforestry. Nat. Commun. 13:4127. doi: 10.1038/s41467-022-30866-8
Zingg, S., Grenz, J., and Humbert, J.-Y. (2018). Landscape-scale effects of land use intensity on birds and butterflies. Agric. Ecosyst. Environ. 267, 119–128. doi: 10.1016/j.agee.2018.08.014
Keywords: sustainable agriculture, birds, butterflies, farmland, landscape scale, conservation
Citation: Zingg S, Grenz J and Humbert J-Y (2024) Food production and biodiversity are not incompatible in temperate heterogeneous agricultural landscapes. Front. Sustain. Food Syst. 8:1377369. doi: 10.3389/fsufs.2024.1377369
Edited by:
Matteo Dainese, University of Verona, ItalyReviewed by:
Xiangjin Shen, Chinese Academy of Sciences (CAS), ChinaMauricio Gonzalez-Chang, Austral University of Chile, Chile
Copyright © 2024 Zingg, Grenz and Humbert. This is an open-access article distributed under the terms of the Creative Commons Attribution License (CC BY). The use, distribution or reproduction in other forums is permitted, provided the original author(s) and the copyright owner(s) are credited and that the original publication in this journal is cited, in accordance with accepted academic practice. No use, distribution or reproduction is permitted which does not comply with these terms.
*Correspondence: Jean-Yves Humbert, amVhbi15dmVzLmh1bWJlcnRAdW5pYmUuY2g=