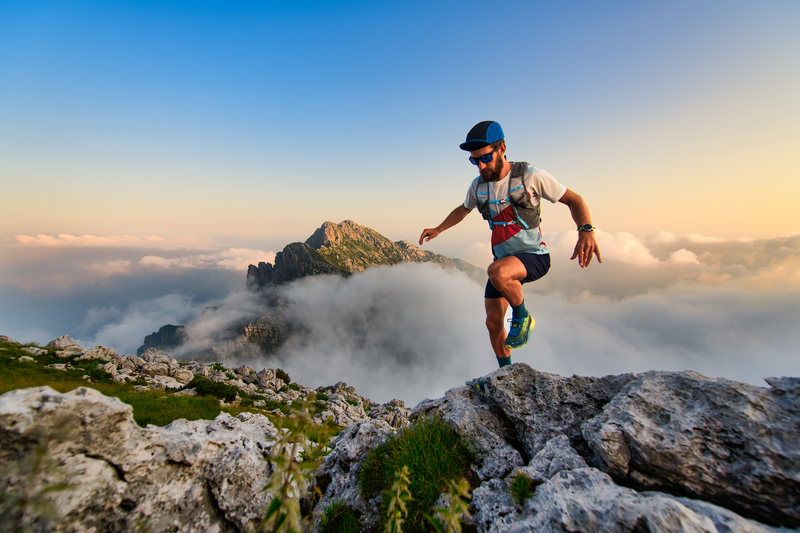
94% of researchers rate our articles as excellent or good
Learn more about the work of our research integrity team to safeguard the quality of each article we publish.
Find out more
ORIGINAL RESEARCH article
Front. Sustain. Food Syst. , 09 August 2024
Sec. Agricultural and Food Economics
Volume 8 - 2024 | https://doi.org/10.3389/fsufs.2024.1365536
The analysis of herding households’ agricultural insurance purchasing behavior under climate risk can help improve the agricultural insurance system in pastoral areas in China.By constructing an analytical framework of “climate change, risk perception, and herding households’ agricultural insurance purchase decisions,” this study matched the survey data from 764 herding households across different types of grasslands in Inner Mongolia with meteorological data from 2019 to 2023. Based on Probit model regression and mediation effect tests, the following conclusions were drawn: Firstly, climate change, especially abnormal fluctuations in precipitation, triggers yield losses and induces loss aversion among herding households, which translates into agricultural insurance purchasing behavior. Secondly, as herding households are divided into production herding households and subsistence herding households, the impact of climate risk on production herding households’ agricultural insurance purchase decisions is more pronounced than that of subsistence herding households. Thirdly, from the perspective of the mechanism of climate change on the agricultural insurance purchasing behavior of production-oriented herding households, climate risk mainly influences herding households’ agricultural insurance purchase through their risk perception. Therefore, with the intensification of global climate change, while further stimulating the agricultural insurance purchasing demand of living herding households through differentiated premium subsidy policies in the future, it is necessary to further enhance the risk perception level of herding households by strengthening the construction of weather forecasting and agricultural and livestock risk data sharing platform, so as to transform the external changes in climate risk into the intrinsic motivation of herding households’ agricultural insurance purchasing behaviors, and to enhance the herding households’ Climate risk coping capacity of herdsmen.
Climate risk, broadly speaking, refers to the uncertainty of economic and financial activities caused by climate factors such as climate change, extreme weather events, and society’s transition to sustainable development, including physical and transition risks (Task Force on Climate-related Financial Disclosures (TCFD),Recommendations of the Task Force on Climate-related Financial Disclosures, International Financial Stability Board, 2017). As climate change has occurred in recent years, global temperatures and precipitation have undergone subtle changes, and extreme weather events have occurred more frequently, causing significant damage to agricultural and livestock production. In 2018, the United Nations Intergovernmental Panel on Climate Change (IPCC) released its Special Report on Global Warming of 1.5°C, stating that since the 1950s, while the extreme low-temperature events have been declining, the frequency and intensity of extreme high-temperature events have been increasing in the vast majority of the globe, and warming has pushed up the evaporation potential of the atmosphere, thus affecting the amount of net water resources available to a region and leading to the prevalence of agricultural and ecological droughts around the globe (Masson-Delmotte et al., 2018). Meanwhile, according to the Food and Agriculture Organization of the United Nations (FAO), the current annual frequency of natural disasters is more than three times as high as that of the 1970s, and extreme weather events are the most important factor causing economic losses, while the agricultural sector suffers from related losses of up to 37 per cent (FAO, 2023). As an important branch of agriculture, grassland animal husbandry is an important pillar of the grassland region’s economy, the main source of income for the majority of herders’ families, and plays an important role in the regional national economy (Li et al., 2013), however, the characteristics of the dependence on the natural environment, so that the production process embodies the composite functioning of natural and economic ecosystems, which is extremely fragile (Shan, 2000), taking Inner Mongolia as an example, in which only the In 2021, Inner Mongolia grassland suffered disaster losses of 11.59 billion yuan, excluding all kinds of government aid and social fundraising, the loss of herders still reached 11.21 billion yuan, accounting for 97% of the proportion of all the losses (Qin et al., 2023), in the background of huge losses, there is an urgent need for effective risk management tools to diversify the risk.
Agricultural insurance, as an effective means of dispersing natural risks, is an effective means of coping with climate risks. Take Inner Mongolia mutton sheep weather index insurance as an example, 2021 mutton sheep weather index insurance for 817,800 head of mutton sheep to provide 153,337,500 yuan of risk protection, the year for 17,041 affected households of 347,000 head of sheep to provide 5,219,000 yuan of risk loss compensation; 2022 for 1,128,300 head of mutton sheep to provide 397,370,700 yuan of risk protection. The level of risk protection was doubled, and 13,443,600 yuan of loss compensation funds were provided for 963,000 head of sheep of 4,823 affected herdsmen throughout the year, which effectively promoted the recovery of pastoralists’ livestock production and operation, stabilizing their income (Gong, 2021).
Against the background of increasing climate risk, theoretically herders should be more willing to purchase agricultural insurance to diversify the risk of animal husbandry, but the problem of insufficient purchase of agricultural insurance has always been a concern of academics. Some scholars have pointed out that “since 2007, China has been promoting the pilot policy-based agricultural insurance, the government has taken out a large amount of money to subsidize agricultural insurance every year, but 32.1% of farmers and herdsmen have not purchased agricultural insurance, and this percentage will be as high as 54.5% if we exclude the farmers who are forced to purchase agricultural insurance (including those who unknowingly purchased the insurance from village committees or township governments; Guo and Tan, 2019), and this phenomenon is even more serious in grassland areas, such as mutton goat weather index insurance, for example, the current insurance rate in different grassland areas of Inner Mongolia has serious differences, according to the data that we have collected through symposiums on the overall participation in field research in the flag counties of the insurance pilot areas, the participation rate of Abaga Banner reaches 84.3 per cent, while the insurance participation rate in Wulagai Administrative Region is only 1.8 per cent. Due to the single production and income structure and the lack of effective protection mechanism, the current natural risk has become the dominant factor in the decline of herdsmen’s income. So why are herders instead reluctant to buy agricultural insurance as climate risks continue to intensify? Therefore, accurately identifying the climate risk on the insurance purchasing behavior of herding households will help to improve the policy design of agricultural insurance, better play the role of agricultural insurance on risk management in grassland areas where natural risks are frequent and risk management means are single, and construct a risk management system of firewall and safety net of agricultural insurance in pastoral areas in the context of rural revitalization.
As far as the demand for agricultural insurance is concerned, domestic and foreign scholars have conducted a large number of relevant studies. In terms of internal influencing factors, on the one hand, farmers’ personal characteristics such as age, education, production experience, risk perception, risk attitude, gender, knowledge of insurance products, and other personal characteristics have an important impact on the purchase demand for agricultural insurance (Sherrick et al., 2004; Hill et al., 2013; Akter et al., 2016; Shang et al., 2020); on the other hand, family business characteristics of farmers such as farming income, planting scale, and whether they join cooperatives have an important impact on the purchase demand for agricultural insurance (Zhang et al., 2017). In terms of external factors, mainly the risk characteristics faced by farmers (Shaik et al., 2008), government premium subsidies (Goodwin and Smith, 1995; Knight et al., 1997; Glauber, 2004; Sherrick et al., 2004), and the degree of conformity of insurance products with actual demand, etc. have an important impact on the demand for agricultural insurance (Fu et al., 2021). However, current research focuses more on purchasing studies of farmers’ agricultural insurance needs, while relatively little research has been done on the insurance purchasing behavior of ranchers. At the same time, there are even fewer studies on the agricultural insurance purchasing behavior of herding households from the perspective of climate risk changes.
The main contributions of this paper are as follows: first, it focuses on the study of herding households’ agricultural insurance purchasing behavior under the scenario of increasing climate risks. Since herders have more natural risks in pastoral areas than farmers, and their means of managing natural risks are more sole, accurate identification of herders’ agricultural insurance purchasing behavior will help the government to better formulate agricultural insurance development policies and promote the construction of agricultural insurance risk management systems in pastoral areas. Secondly, the use of meteorological data and other macro data combined with microdata from field research in pastoral areas, to more accurately identify the impact of climate risk on the agricultural insurance purchasing behavior of herdsmen. Compared with previous scholars who mostly used indirect means such as the number of livestock sheds to measure climate risk (Gong, 2021), the use of daily weather data in this paper is more direct, accurate, and scientific in climate risk measurement, which helps to make up for the previous micro-individual agricultural insurance purchasing behavior in the measurement of climate risk. Third, the use of daily weather data at the banner-county level helps to more accurately combine with micro-individual research data of herdsmen to accurately identify the impact of climate risk on herdsmen’s agricultural insurance purchasing behavior.
The key to the study on the impact of climate change on herders’ agricultural insurance purchasing behavior is to clarify the impact of climate change on herders’ agricultural output. Current research on the impact of grassland ecosystems in Inner Mongolia focuses on the impact of climatic factors such as temperature and precipitation on grassland productivity and ecological environment, which leads to inconsistent findings based on different research regions, data sources, and analysis methods, but the basic impact paths are analyzed in two direct and indirect paths:
The direct effect of climate risk on agricultural output and the indirect effect on the farming economy through increased levels of natural hazards coexist. At the earliest, the relationship between temperature and per capita income has been of interest to scholars in the 14th century (Gates, 1967; Dell et al., 2009). A 1°C increase in average annual temperature would lead to a 2.5% decrease in economic output (Hsiang, 2010; Welch et al., 2010; Zhou and Zhu, 2010; Butler and Huybers, 2013). On one hand, climate change is one of the main factors determining the high quality of forage production (Wang and Yang, 2010), in which the five main rates of change of climate change showed high correlations for each growth period of forage, i.e., the correlation coefficients of temperature, precipitation, relative humidity, wind speed, and sunshine with forage reached 0.858, 0.841, 0.723, 0.035, and 0.406, respectively (Zhang, 2017), but there is more agreement in the current study that the temperature increase in climate change usually have less impact on grassland productivity, and precipitation is the main influence factor (Gao et al., 2020). On the other hand, climate risks can have important impacts on livestock impacts: for example, affecting the development and reproduction of livestock and reducing production (Amundson et al., 2006; Bryant et al., 2007). Low temperatures lead to problems such as slow metabolism and tissue frostbite in crops and livestock (Shannon and Motha, 2015).
In addition to its direct impact on agricultural output, climate risk affects agricultural output and increases the marginal cost of production through its impact on factors of production such as land use, capital stock, labor quantity, and efficiency. For example, the reduction of water resources affects agricultural production and the storage of agricultural products (Piao et al., 2010); the reduction of labor hours in industries that are more affected by climate affects the efficiency of labor (Zivin et al., 2015), while some scholars have pointed out that the loss due to reduced labor efficiency in agricultural manufacturing caused by climate risk is nearly 3.95–4.73% of the total manufacturing output (Seppänen et al., 2003; Shakoor et al., 2011; Heal and Park, 2016; Limskul, 2018), and this effect varies regionally depending on local resource endowments and agricultural production conditions (Chen et al., 2016). High temperatures trigger a shift of labor between sectors, with high temperatures in some regions leading to a shift of labor from agriculture to industry and services (Kjellstrom et al., 2009).
In addition, climate risk acts directly on economic output in agriculture and livestock by increasing the severity of natural disasters. Rising climate risk leads to an increase in the probability and severity of natural disasters: disaster economics models suggest that the mean, variance, and correlation of the distribution of natural disasters will be positively correlated with climate change (Zhang et al., 2015; Stott, 2016; Bellprat et al., 2019), while according to the neoclassical economic growth model pointed out that it can destroy the production output, such as causing damage to crops and death of livestock (Gao et al., 2013; Felbermayr and Groschl, 2014), in the supply and demand model of the agricultural sector, the loss of agricultural supply output caused by natural disasters will lead to the supply and demand relationship in the agricultural sector supply curve in the agricultural sector to shift to the left, which eventually leads to a reduction in equilibrium output and growth rate, resulting in a negative impact on the economy (Bertrand, 1993).
Climate risk acts on agricultural output in direct and indirect ways (as shown in Figure 1). Agricultural insurance can diversify climate risk in time and space and restore agricultural reproduction through the function of loss compensation (Ye, 2018), thus generating agricultural insurance purchasing behavior. Based on the above discussion, this study proposes the following hypothesis:
Hypothesis 1: Climate risk is positively related to herders’ agricultural insurance purchasing behavior, i.e., the greater the climate risk, the greater the likelihood that herders will purchase agricultural insurance.
Figure 1. Analytical framework of climate risk on pastoralists’ agricultural insurance purchasing behavior.
With the development of industrialization and urbanization, more and more farming and herding households have left the farming and herding areas, and there has been a differentiation of farming and herding households. One part of the population shifts to non-agricultural industries based on their strong non-agricultural employability, leaving older people and women behind for rural residency, forming subsistence farming and herding households; while another part of farming and herding households form larger-scale productive farming and herding households that rely on farming and herding for income through land (grassland) transfer (Hu and Zheng, 2021). Based on the differences in the livelihood strategies of different types of farmers and herders, there are some differences in the allocation of production factors in farming and herding production, which leads to some differences in the demand for agricultural insurance to reduce the level of risk exposure. Based on this, this paper proposes a second hypothesis:
Hypothesis 2: There is a difference in the impact of climate risk on productive and subsistence herding households: the impact on subsistence herding households’ agricultural insurance purchase decisions is unclear, and there is a positive impact on productive herding households’ agricultural insurance purchases.
In the context of climate risk causing differential impacts on pastoralists’ agricultural insurance purchasing behavior in different contexts, risk perception is the starting point of the agricultural insurance decision-making process of farmers and pastoralists (Cheng et al., 2018), and existing foreign literature found that weather risk perception is a key influence on farmers’ adaptation decision adoption behavior (Below et al., 2012), the basis of climate change adaptation behavior, and the agricultural production main driver of adoption of transfer risk (Chen and Hu, 2023; Figure 2). Therefore, based on the above hypothesis, this paper further proposes that the extrinsic risk of climate change is transformed into the intrinsic starting point of herding households’ agricultural insurance purchase decisions by acting on the risk perception of productive herding households, and through the risk perception, rational economic people become loss averse and actively seek risk management tools that reduce the level of risk exposure and the probability of risk occurrence to diversify and transfer, thus proposing the following hypothesis:
Hypothesis 3: The effect of climate risk on the agricultural insurance purchasing behavior of productive pastoralists is internalized through the mediating role of risk perception.
Figure 2. Grassland availability in Inner Mongolia by banner and county Data source: Inner Mongolia Nature Museum No. GS (2022) 1873 map has not been modified.
This paper takes Inner Mongolia as an example, mainly based on the following reasons: firstly, Inner Mongolia as an important part of the Eurasian continental steppe, and at the same time as an important ecological security barrier and agricultural and livestock products production base in northern China, the grassland is both an ecological resource and an important production resource for farmers and herdsmen, which is mainly manifested in the following ways: on the one hand, as the largest grassland pastoral area in China, the grassland area accounts for 22% of the national grassland area and 74% of the area of the whole region, with a natural grassland area of 1.32 billion mu, and there are five major categories of grassland types from east to west, namely, temperate meadow grassland, temperate typical grassland, temperate desert grassland, temperate steppe desert, and temperate desert (Inner Mongolia Autonomous Region Forestry and Grassland Bureau, Grassland resources, 2024), and the region with higher utilization rate of grassland resources is mainly concentrated in the east-central part of Inner Mongolia (shown in Figure 2), so that the grassland area and grassland types are typical and representative of the region. On the other hand, Inner Mongolia, as an important “meat can” and “milk bank” in China, had a total meat output of 2.672 million tonnes in 2018, an increase of 0.8% compared with the previous year, and a milk output of 5.656 million tonnes, an increase of 2.3% compared with the previous year, with the production of beef and lamb ranking first in the country (Data source).1 Secondly, at the same time of the rapid development of grassland animal husbandry, the current grassland animal husbandry exists the problem of fragile ecological environment and the occurrence of natural disasters, for example, in 2018, by the impact of persistent drought, as of June of that year, the affected area of the region’s pasture reached 550 million acres, accounting for more than 40 per cent of the total area of the grassland, and the affected livestock amounted to 35,432,000 head (only; Data source).2 This is mainly due to natural climatic conditions: Inner Mongolia, which is in the middle and high latitudes, is dominated by temperate continental monsoon climate, far from the ocean, and it is difficult for the warm and humid airflow to reach the daily and annual difference of temperature, the closer to the center of the continent, the greater the annual and daily difference of temperature, the less precipitation, the more arid the climate, therefore, aridity is the main feature of the climate in the northern grassland areas of China, with high climate sensitivity and ecological vulnerability. The specific manifestations are that the temperature distribution increases from east to west, the winter is long and cold, up to about 200 days; the summer is hot and short, generally 90–100 days; the temperature in spring is 7°C and 6–8°C higher in April than in March and May than in April respectively, and the temperature increases suddenly; the temperature in autumn drops suddenly, and the monthly cooling can reach 6–10°C; meanwhile, the annual and daily differences of temperature are large, the annual difference can reach 33–34°C, and the annual average daily difference can reach 12–16°C. In terms of precipitation, precipitation decreases from southeast to northwest, and the overall precipitation is low. The annual precipitation in the easternmost mountainous area of Daxinganling and the southern mountainous area of the Xiliaohe River basin, which receives more precipitation, can reach 450 mm, while the annual precipitation in Bayannur-Alashan high plain is only about 100 mm, and the westernmost is less than 50 mm, with a large difference between east and west. Meanwhile, under the influence of monsoon climate, the precipitation in winter and spring is low, and the summer precipitation is concentrated from June to August, and the precipitation in most areas can generally reach 150-200 mm, accounting for 60–75% of the annual precipitation; the precipitation variation is large, gradually increasing from east to west, and the quarterly rate of change in precipitation in the west is 2–6 times of that in the east, and the interannual variation of precipitation in months and seasons can reach 10 of times. The guaranteed rate of natural pasture and crop growth is low; while the annual evaporation gradually increases from east to west, and the annual evaporation in the western region maybe 10 times of precipitation. For example, the annual evaporation in the northern Bayannur and western Ordos regions can reach 2,400 ~ 3,400 mm, and the difference of evaporation and precipitation is large (Gao et al., 2020). For this reason, in the context of higher climate sensitivity and ecological vulnerability, policy agricultural insurance such as weather index insurance for mutton sheep and grassland insurance began to be promoted in grassland areas on a pilot basis in 2019 to enhance the management capacity of herders to cope with climate risks.
First, in response to the long-term absence of weather risk management tools in grassland pastoral areas, the nation’s first weather index insurance for mutton sheep targeting the grassland livestock industry was implemented in Xilingol League and Hulunbeier City in Inner Mongolia in 2019, with specific insurance clause highlights shown in the Table 1.
Second, in 2019, Inner Mongolia proposed for the first time to develop grassland insurance, and in 2021, it began a large-scale pilot program, mainly in 13 banner counties in eight allied cities, with a trial period of 3 years (Table 2).
The microdata sources: Since the grassland in Inner Mongolia stretches for more than 1,000 km and spans three climatic zones, namely temperate semi-humid zone, semi-arid, and arid zone from east to west, based on considering the representativeness of grassland types, the final selection of the more comprehensive grassland types Xilin Gol league, the central region of Ulanqab city and the western region of Erdos city. Shepherds’ data were obtained from the research team’s four separate studies on the weather index for mutton sheep and the current status of grassland insurance implementation during the period 2019–2023, including studies on the weather index for mutton sheep in Xilingol League in 2019 and 2020, grassland insurance in Siziwangqi, Ulanqab city, in 2022, and grassland insurance in Wushen Banner and Etuoke Banner, Erdos City, in the early part of 2023.
Based on the research method, considering the policy agricultural insurance pilot implementation areas, multi-stage random sampling was adopted, that is, each research first randomly selected 1 to 4 banner counties, then randomly selected 2 to 3 soum townships in each banner county, each soum township randomly selected 1 to 3 villages, and finally selected a specific number of samples in each village. In order to ensure the authenticity and validity of the questionnaire data, the team researcher needed the preliminary questionnaire training. Accompanied by local staff proficient in both Mongolian and Chinese languages, each researcher was taken individually to visit pastoral households for one-on-one questionnaire surveys. Upon entering the household, the purpose of our visit was first explained by staff proficient in both Mongolian and Chinese languages. If the herders were willing to cooperate, the next step of the survey was conducted. If they were not cooperative, a new sampling was carried out in the village. The interviewer asked questions according to a pre-designed questionnaire, the staff translated, and the herders provided answers.
Finally, 764 herding households’ survey data were obtained from 3 leagues and 11 banner municipalities. The 11 banner municipalities are shown in Table 3. The contents of the questionnaire mainly involved the personal and family characteristics of herding households, and their disaster situations, perception of climate change risks, awareness and satisfaction of agricultural insurance.
Table 3. Distribution of surveyed herding households and purchase of agricultural insurance in each area (unit: households).
The macroscopic data sources were mainly: the daily precipitation data of the sample areas (banners and counties) from January 2015to December 2022 were obtained from the Central Weather Station agro-meteorological data.
Dependent variable: “whether herding households purchase agricultural insurance” was adopted as the measure of agricultural insurance, and based on the microdata derived from multiple field household surveys over different periods, different points in time were chosen to examine agricultural insurance purchasing behavior to ensure that, as far as possible, the research was fresh in the memory of the herdsmen and that the data were more accurate. The year 2019 was chosen as the point of insurance purchase for the weather index insurance for mutton sheep, 2021 for the grassland insurance in Siziwang Banner of Ulanqab City, and 2022 for Wushen Banner and Etuoke Banner of EOrdos City, and this variable mainly examined whether herders purchased the corresponding insurance at the above points.
Core explanatory variables: climate risk mainly refers to changes in temperature and precipitation that deviate from long-term average trends, as manifested by extreme climate events such as droughts and floods. In terms of research methodology, previous scholars have mainly used indicators such as temperature levels, temperature ranges, and temperature extremes when measuring climate warming risk (Deschênes and Greenstone, 2007; Dell et al., 2012), and the vast majority of the literature uses temperature anomalies (or standard temperatures) to measure climate warming risk (Hong et al., 2019; Choi et al., 2020; Ding and Sun, 2022), and also the quantile of standardized temperature to measure climate risk (Hong et al., 2019; Choi et al., 2020), such as using areas where the standardized temperature is greater than the 80% quantile of the current year’s sample defined annually as high quantile areas, and areas below the 20% quantile defined as low quantile areas, serving as a dummy variable (Ding and Sun, 2022). In examining the impact of climate risk on the economy, fixed effects models are mostly used to remove the effects of geographic environmental factors (Deschênes and Greenstone, 2007; Dell et al., 2012).
Drought is the main feature of the climate in the northern grassland regions of China, coupled with the fact that there is more consensus in current research that temperature increases in climate change usually have less impact on grassland productivity and precipitation is the main impact factor (Gao et al., 2020). Therefore, the use of precipitation variability and extreme weather events as a measure of climate risk in grasslands is a common practice among scholars at home and abroad. For the study of the effect of precipitation changes on the behavior of micro-individuals, the absolute value of the temperature deviation for 3–5 years is currently used as a measurement term (Deschênes and Greenstone, 2007). In this paper, based on other scholars’ formulas for calculating precipitation bias (Wang et al., 2014), and in line with the purpose of the study, the average daily rainfall in the year in which agricultural insurance was purchased minus the average daily rainfall over the past 4 years was used as a measure of climate risk for each flag county. In addition, “frequency of extreme weather events in the past three years” was adopted as the replacement term for climate risk. This variable mainly comes from the questionnaire that asks herders “In the past three years, what do you think was the level of natural risk to livestock production? Pastoralists are asked to make choices based on a Likert Scale approach. The description and statistical description of each variable are shown in Table 4.
Control variables: Referring to the above studies on factors affecting agricultural insurance purchasing behavior, six aspects of household head characteristics, family characteristics, herder production and business characteristics, social capital, risk attitudes, and regional characteristics are selected, the details are as follows:
(1) Household head characteristics. It mainly includes age, years of education, and agricultural insurance perception. According to previous studies in related literature (Sherrick et al., 2004; Hill et al., 2013; Guo and Tan, 2019; Gong, 2021), the older the age, the higher the risk aversion of herding households and the more inclined they are to diversify climate risks by purchasing agricultural insurance; the more educated the herding households are, the higher their cognition of agricultural insurance may be, which in turn enhances the demand for agricultural insurance.
(2) Household characteristics. The main characteristics include pure herding income and family members’ employment status (Akter et al., 2016). Among them, the higher the pure herding income is, the more likely it is to be affected by climate risk and the higher the demand for agricultural insurance purchase; whether any member of the family has held a public position, and the higher the probability of agricultural insurance purchase for herding households who have held a public position.
(3) Herding households’ production and operation characteristics. It mainly includes the pasture area. Among them, the larger the grassland area is, the greater the risk that herding households need to bear, and the more inclined they are to transfer the risk by purchasing agricultural insurance.
(4) Social capital. It includes whether they have joined cooperatives and whether their neighbors have purchased. Since the grassland area is sparsely populated and herding households are constrained by limited rationality, it is difficult for them to obtain all information about risk events, so social capital may have an important influence on their agricultural insurance purchasing behavior. At the same time, whether herding households have joined cooperatives, and if so, whether they have dispersed the impact of climate risk through cooperatives, may reduce their agricultural insurance purchasing behavior.
(5) Risk attitude. Including the number of other commercial insurance purchased. Risk attitude is an important influencing factor for herding households’ agricultural insurance purchases (Shang et al., 2020). Risk-averse herding households tend to purchase insurance to transfer risks, so the number of commercial insurance purchased by the respondent households was asked to measure herding households’ risk attitude more objectively.
(6) Regional characteristics. Including the distance to the township (Sumu).
From the calculated annual average daily precipitation deviation of single-day measured precipitation, the flag with larger precipitation deviation is Xiwuzhumuqin banner, reaching the average annual precipitation deviation of the past 4 years amounting to 0.426 mm, followed by the banners with larger deviation of precipitation in order Zhenglan Banner, Etuoke Banner, Siziwang Banner, Xilinhot, Wushen Banner, Abaga Banner, Dongwuzhumuqin Banner, which annual average daily precipitation all showed a decline of greater than 0.1 mm, and the climate risk was relatively large. The areas with smaller relative precipitation deviations are Wulagai Management Area, Suniteyou Banner and Xianghuang Banner (Figure 3).
Figure 3. Relative changes in annual average daily precipitation. Data source: Calculated according to the daily precipitation data of the National Meteorological Observator.
In addition, from the relationship between herders’ agricultural insurance purchase and the mean value of daily precipitation deviation, the mean value of precipitation deviation in the Banner (County) of herders who purchased agricultural insurance reached 0.190 mm, while the mean value of precipitation deviation in the Banner (County) of herders who did not purchase agricultural insurance was 0.165. The precipitation deviation in the banner (county) of herders who purchased agricultural insurance was significantly higher than the precipitation deviation in the Banner (County) of herders who did not purchase agricultural insurance. Therefore, from the characteristic factual relationship between the two, the climate risk caused by the deviation may have a certain effect on the herding households’ purchase of agricultural insurance, and there may be a certain correlation between the two, which needs to be further tested empirically.
Based on the fact that the dependent variable are binary discrete variables, and the core explanatory variable essentially follows a normal distribution. Therefore, the Probit model is proposed to be used in this paper for the empirical analysis.
In the regression model formula (1) described above: P(yi) represents whether herding household i purchases agricultural insurance. Riski represents herding household i faced with climate risk. Xi represents the control variables. β1i and β2i represents the regression coefficients for the core explanatory variable and the dependent variable. ε represents the random error term. Furthermore, the above variables were found to have a VIF mean of 1.14 (<5) through multicollinearity tests, indicating that there is no multicollinearity among the variables.
The estimation results in Table 5 show that the greater the climate risk, the greater the probability of herding households purchasing agricultural insurance at the 1% statistical significance level, in which the probability of herding households purchasing agricultural insurance will increase by 0.399% for each unit increase in climate risk. The reason for this is that climate risks are frequent in grassland areas, the income source is single, and the abnormal climate changes act on herding households’ agricultural production through both direct and indirect channels, which may lead to farmers’ risk loss aversion and active search for risk management tools to reduce risk losses, and then produce agricultural insurance purchasing behavior.
Table 5. Baseline regression results of the effect of climate risk on pastoralists’ agricultural insurance purchasing behavior.
In addition, from the model regression results, age, education level, and agricultural insurance cognition among herding households’ characteristics have significant positive effects on agricultural insurance purchasing behavior, especially agricultural insurance cognition has an impact on herding households’ agricultural insurance purchasing behavior, among which, each unit increase in agricultural insurer’s cognition will increase herding households’ agricultural insurance purchasing probability by 0.105%. Concerning household characteristics, the influence of public employment on herding households’ agricultural insurance purchasing behavior was not significant, indicating that the “de-administration” of agricultural insurance purchasing was more obvious. Among the production and operation characteristics of herding households, the pasture area has a greater influence on the purchase of agricultural insurance, indicating that the larger the pasture area, the higher the climatic risk faced by herding households, and the more likely they are to purchase agricultural insurance. In terms of social capital, whether or not neighbors purchase agricultural insurance has a positive and significant effect on the purchase behavior of herding households, indicating that herding households have difficulty obtaining all the information about the risk of climatic events due to the sparseness of the area, information and transportation, and the purchase of agricultural insurance is more likely to be influenced by the purchase behavior of neighbors, and a “herd effect” occurs.
In order to test the reliability of the conclusions obtained from the model, a robustness test of the conclusions is required. The robustness test of climate risk on pastoralist participation in agricultural insurance may be performed because of measurement error and sample selection problems that lead to the unreliability of the results.
To avoid the unreliability of the findings due to the measurement of climate risk by precipitation anomalies, the climate risk measured by rainfall variability in Table 5 was replaced by the frequency of extreme weather events to measure climate risk, and the Probit model was used again for robustness testing. In general, the risk perception level of farmers matches the actual occurrence of risk (Huang and Qian, 2023), so the frequency of extreme weather events was measured by asking herders “How much risk do you think the livestock production faced in the past three years,” and the Likert scale was used to measure the frequency of extreme weather events. The results showed that for each 1-unit increase in climate risk measured by the probability of extreme weather events at the 10% statistical significance level, the probability of herding households to purchase agricultural insurance increased by 0.025%, i.e., climate risk significantly promoted herding households’ agricultural insurance purchase behavior. The core explanatory variable climate risk was also subjected to a 5% upper and lower tailing process, and the regression test was conducted on the tailed data to verify the robustness of the above findings, and the results yielded similar results to the regressions in the above paper, which proved that the regression results of this paper are somewhat robust (Table 6).
Based on the theory of herding household differentiation, the results of model (1) and model (2) in Table 7 report the effects of climate risk on the agricultural insurance purchasing behavior of herding households with different behavioral abilities, respectively. Among them, the results of model (1) show that climate risk has a positive but insignificant effect on the agricultural insurance purchasing decision of lifestyle herding households, indicating that the effect of climate risk on the agricultural insurance purchasing decision of lifestyle herding households is uncertain. The results of model (2) show that the influence of climate risk on the agricultural insurance purchasing decision of herding households shows a positive and significant influence with a regression coefficient of 1.634, and it is significant at the 1% statistical level, and the probability of agricultural insurance purchasing behavior of production-oriented herding households will increase by 0.380% for every 1 unit increase in the water deviation of climate risk decline. Hypothesis 2 that there is a difference in the effect of climate risk on productive and subsistence herding households is verified, i.e., the effect of climate risk on subsistence herding households’ agricultural insurance purchase decisions is unclear, and there is a positive effect on productive herding households’ agricultural insurance purchases.
Table 7. Impact of climate risk on herders’ decision to purchase agricultural insurance: discussion based on herders’ differentiation.
In order to further analyze the influence mechanism of climate risk on the agricultural insurance purchase decision of productive pastoralists, this paper draws on the mediating effect model test proposed by Wen et al. (2004) and refers to the test of the mediating effect of the Porbit model with binary variables by Sun et al. (2020) to set the influence of climate risk on the agricultural insurance purchase decision of productive pastoralists as the following model formula (2)–(4), with the specific expressions:
Where Y represents herders’ agricultural insurance purchase, X is climate risk, and M is herders’ risk perception; X is the effect on Y through M, i.e., the mediating effect.
The regression results in Step 2 show (as in Table 8) that, given constant control variables, climate risk has a positive effect on farmers’ risk perception and is significant at the 5% statistical level. Step 3 After climate risk and risk perception are added to the agricultural insurance purchase decision of production pastoralists, the greater the change in climate risk, the greater the likelihood of production-type pastoralists purchasing agricultural insurance at the 1% statistical level; and At the 10% statistical level, an increase in risk perception increases the likelihood of production-type herding households to purchase agricultural insurance. Step 3 The coefficient of 1.586 for the effect of climate risk on production-type herding households’ agricultural insurance purchase decision is smaller than the coefficient of 1.634 for Step 1 and is significant, indicating the existence of a mediating effect of risk perception in the effect of climate risk on production-type herding households’ agricultural insurance purchase decision, i.e., Hypothesis 3 is supported.
To ensure the robustness of the above results, the test is based on the mediating effect product stepwise method for category variables proposed by Fang et al. (2017). The 95% confidence interval for Za × Zb obtained from the RMediation package of the R software is [0.340, 1.092], which does not contain 0. Therefore, the mediation effect is significant.
This paper constructs an analytical framework of “climate risk, risk perception and herding households’ agricultural insurance purchasing behavior.” We find that climate change positively affects the purchasing behavior of herding households, and that the effects on individual herding households of different natures are heterogeneous, which is consistent with Zheng et al. (2021) and Hu and Zheng (2021), revealing the purchasing behavior of herding households in agricultural insurance under climate change. However, unlike Zheng et al. (2021) and Hu and Zheng (2021), which focus on farmers, this paper finds that climate change, especially abnormal fluctuations in precipitation, is more likely to affect pastoralists, and the results of the probit model show that for every unit increase in climate risk, the probability that a pastoralist purchases agricultural insurance increases by 0.399 per cent, and that after substituting the risk measure variables and shrinking the tails. This finding remains robust. In contrast, Zheng et al. (2021) find that for every unit increase in climate risk, the probability of a farmer purchasing agricultural insurance increases by 0.182 per cent. The above study comparison indicates that the impact of climate risk on herding households’ agricultural insurance purchasing behavior is higher than its impact on farming households, which is also more in line with reality. This is because herding households have a relatively single livelihood compared to farming households, weaker risk resilience, and climate change will have a greater effect on their corresponding impacts.
In addition, in terms of the mechanism of climate change on the agricultural insurance purchasing behavior of productive herders, this paper argues that climate risk is transformed from an external factor to an internal purchasing incentive for farmers mainly through the risk perception of herders, which is also different from Zheng et al.’s findings (Zheng et al., 2021).
Admittedly, the article still has many shortcomings. Firstly, although there are only two types of policy grassland-type insurance in Inner Mongolia (as shown in Tables 1, 2), ignoring these differences may have an impact on the study’s conclusions, as two different types of insurance are involved and different time periods are involved. Secondly, due to the limitation of time and capital cost, in terms of the scope of the research, this paper lacks research on the Hulunbeier grassland area in Inner Mongolia, which leads to the failure of the scope of the research to cover all the grassland area in Inner Mongolia. In the future, the in-depth exploration of this issue can be further enhanced.
In the critical year of high-quality development of agricultural insurance and comprehensive promotion of rural revitalization, the agricultural insurance system in pastoral areas in China should be improved from the following aspects: First, the differentiated premium subsidy policy should be optimized to stimulate the agricultural insurance demand of herding households based on household consumption. Although scholars point out that it is difficult for farmers to generate sufficient incentive to participate in insurance without premium subsidies from the perspective of “institutional induction” (Xie and Pu, 2003), based on the different basic conditions of agricultural production practices in different parts of China, and against the background of increasing climate risks, it is important to increase the demand for agricultural insurance in pastoral areas with a single source of income and large risk exposure. In the context of increasing climate risk, it is important to increase the agricultural insurance subsidies for pastoral areas with a single source of income and a large risk exposure to stimulate the agricultural insurance purchase demand of consumer pastoral households and enhance the disaster resilience of pastoralists. Second, strengthen the construction of risk-formation assessment and early warning systems in pastoral areas, increase the construction of a big data sharing platform for agricultural risks, and improve the level of risk prediction and perception of herding households. On the one hand, effective climate forecasting can improve ex-ante risk management, i.e., risk minimization, because agricultural producers can reduce input sources and risk exposure in advance (Carriquiry and Osgood, 2012; Li et al., 2022). Accurate and effective weather forecasting can effectively enhance the ex-ante risk management ability of pastoralists and improve their disaster prevention ability. On the other hand, based on the characteristics of the vast and sparsely populated pastoral areas and closed information, the construction of an agricultural data-sharing platform for risk assessment and early warning in pastoral areas, supplemented by regular training and education of agricultural risk management knowledge by dedicated personnel, can effectively improve the risk perception ability to herd households, transform external changes in climate risk into intrinsic motivation for herding households to purchase agricultural insurance, and effectively improve the response-ability to herd households to changes in climate risk. This can effectively enhance the ability to herd households to cope with changes in climate risks and play the role of agricultural insurance “safety net” and “firewall.”
The original contributions presented in the study are included in the article/Supplementary material, further inquiries can be directed to the corresponding author.
Ethical review and approval was not required for the study on human participants in accordance with the local legislation and institutional requirements. Written informed consent from the patients/participants or patients/participants’ legal guardian/next of kin was not required to participate in this study in accordance with the national legislation and the institutional requirements.
Y-fZ: Conceptualization, Funding acquisition, Investigation, Resources, Writing – review & editing. X-lC: Methodology, Software, Visualization, Writing – original draft.
The author(s) declare that financial support was received for the research, authorship, and/or publication of this article. This research was funded by National Natural Science Foundation of China, grant number 72173069. Project of Basic Scientific Research Business Funds for Universities directly under Inner Mongolia Autonomous Region: Agricultural and Animal Husbandry Insurance Operation Mechanism and Product Innovation, grant number BR221316. Key Research Base of Humanities and Social Sciences in Higher Education Institutions of Inner Mongolia Autonomous Region: Research Base of Risk Management in Agriculture and Animal Husbandry. The funding for the above three funds is all attributed to Zhao Yuanfeng.
The authors declare that the research was conducted in the absence of any commercial or financial relationships that could be construed as a potential conflict of interest.
All claims expressed in this article are solely those of the authors and do not necessarily represent those of their affiliated organizations, or those of the publisher, the editors and the reviewers. Any product that may be evaluated in this article, or claim that may be made by its manufacturer, is not guaranteed or endorsed by the publisher.
The Supplementary material for this article can be found online at: https://www.frontiersin.org/articles/10.3389/fsufs.2024.1365536/full#supplementary-material
1. ^http://nmt.nmg.gov.cn/gk/zfxxgk/fdzdgknr/tjsj/201903/t20190314_1681293.html
2. ^http://www.xinhuanet.com/politics/2018-06/22/c_129898977.htm
Akter, S., Krupnik, T. J., Rossi, F., and Khanam, F. (2016). The influence of gender and product design on farmers' preferences for weather—indexed crop insurance. Glob. Environ. Chang. 38, 217–229. doi: 10.1016/j.gloenvcha.2016.03.010
Amundson, J. L., Mader, T. L., Rasby, R. J., and Hu, Q. S. (2006). Environmental effects on pregnancy rate in beef cattle. J. Anim. Sci. 84, 3415–3420. doi: 10.2527/jas.2005-611
Bellprat, O., Guemas, V., Reyes, F. D., and Donat, M. G. (2019). Towards reliable extreme weather and climate event attribution, nature. Communications 10, 1–7. doi: 10.1038/s41467-019-09729-2
Below, T. B., Mutabazi, K. D., Kirschke, D., Franke, C., Sieber, S., Siebert, R., et al. (2012). Can farmers’ adaptation to climate change be explained by socio-economic household-level variables? Glob. Environ. Chang. 22, 223–235. doi: 10.1016/j.gloenvcha.2011.11.012
Bertrand, J. M. A. (1993). Natural disaster situations and growth: a macroeconomic model for sudden disaster impacts. World Dev. 21, 1417–1434. doi: 10.1016/0305-750X(93)90122-P
Bryant, J. R., López-Villalobos, N., Pryce, J. E., Holmes, C. W., and Johnson, D. L. (2007). Quantifying the effect of thermal environment on production traits in three breeds of dairy cattle in New Zealand. N. Z. J. Agric. Res. 50, 327–338. doi: 10.1080/00288230709510301
Butler, E. E., and Huybers, P. (2013). Adaptation of US maize to temperature variations. Nat. Clim. Chang. 3, 68–72. doi: 10.1038/nclimate1585
Carriquiry, M. A., and Osgood, D. E. (2012). Index insurance, probabilistic climate forecasts, and production. J. Risk and Insurance 79, 287–300. doi: 10.1111/j.1539-6975.2011.01422.x
Chen, Z. H., and Hu, H. (2023). Influence of farmers' perception of weather risk on agricultural insurance selection behavior: an example of peach farmers. J. Nanjing Agricul. University (Soc. Sci. Edition) 23, 178–190. doi: 10.19714/j.cnki.1671-7465.20221208.001
Chen, S., Xu, J. T., and Zhang, H. P. (2016). The impact of climate change on grain production in China--an empirical analysis based on county-level panel data. China Rural Econ. 5, 2–15.
Cheng, J., Liu, F., and Tao, J. P. (2018). Risk perception, risk management and demand for agricultural insurance-an empirical study based on behavioral finance perspective. J. Nanjing Agricul. University (Soc. Sci. Edition) 18, 133–156.
Choi, D., Gao, Z., and Jiang, W. (2020). Attention to global warming. Rev. Financ. Stud. 33, 1112–1145. doi: 10.1093/rfs/hhz086
Dell, M., Jones, B. F., and Olken, B. A. (2009). Temperature and income: reconciling new cross-sectional and panel estimates. Am. Econ. Rev. 99, 198–204. doi: 10.1257/aer.99.2.198
Dell, M., Jones, B. F., and Olken, B. A. (2012). Temperature shocks and economic growth: evidence from the last half century. Am. Econ. J. Macroecon. 4, 66–95. doi: 10.1257/mac.4.3.66
Deschênes, O., and Greenstone, M. (2007). The economic impacts of climate change: evidence from agricultural output and random fluctuations in weather. Am. Econ. Rev. 97, 354–385. doi: 10.1257/aer.97.1.354
Ding, Y. G., and Sun, Q. X. (2022). Impact of climate risk on China's agricultural economic development - a heterogeneity and mechanism analysis. Financial Res. 507, 111–131.
Fang, J., Wen, Z. L., and Zhang, M. Q. (2017). Mediation effect analysis of categorical variables. Psychol. Sci. 40, 471–477. doi: 10.16719/j.cnki.1671-6981.20220326
FAO (2023). The impact of disasters on agriculture and food Security2023-avoiding and reducing losses through investment in resilience. Rome. 2023, 1–5. doi: 10.4060/cc7900en
Felbermayr, G., and Groschl, J. K. (2014). Naturally negative: the growth effects of natural disasters. J. Dev. Econ. 111, 92–106. doi: 10.1016/j.jdeveco.2014.07.004
Fu, H., Zhang, Y. H., et al. (2021). Subjective and objective risk perceptions and the willingness to pay for agricultural insurance: evidence from an in the field choice experiment in rural China. Geneva Risk Insur. Rev. 46, 1–24. doi: 10.1057/s10713-021-00071-6
Gao, Q. Z., Wan, Z. Q., and Liang, C. Z. (2020). Research on key technologies for adaptation of grassland animal husbandry to climate change in Inner Mongolia. Beijing: Sci. Press 9, 15–26.
Gao, Y., Zhan, H. L., Chen, W. Z., and Jiao, J. (2013). Study on the impact of natural disasters on China's agriculture. Disasters 3, 79–84. doi: 10.3969/j.issn.1000-811X.2013.03.015
Gates, W. E. (1967). The spread of Ibn Khaldûn's ideas on climate and culture. J. Hist. Ideas 28, 415–422. doi: 10.2307/2708627
Glauber, J. W. (2004). Crop insurance reconsidered. Am. J. Agric. Econ. 86, 1179–1195. doi: 10.1111/j.0002-9092.2004.00663.x
Gong, Y. F. (2021). Weather index insurance for grassland mutton sheep. Inner Mongolia Agricul. University 2021, 79–80. doi: 10.27229/d.cnki.gnmnu.2021.000079
Goodwin, B. K., and Smith, V. H. (1995). The economic of crop insurance and disaster aid. Washington D.C.: the AEI Press.
Guo, J., and Tan, S. (2019). Regional differences in farmers' agricultural insurance rejection: insufficient supply or insufficient demand--a study based on plantation insurance in 12 counties of 6 northern provinces. Agricul. Technol. Econ. 2, 85–98. doi: 10.13246/j.cnki.jae.2019.02.007
Heal, G., and Park, J. (2016). Reflections-temperature stress and the direct impact of climate change: a review of an emerging literature. Rev. Environ. Econ. Policy 10, 347–362. doi: 10.1093/reep/rew007
Hill, R. V., Hoddinott, J., and Kumar, N. (2013). Adoption of weather—index insurance: learning from wllingness to pay among a panel of households in rural Ethiopia. Agric. Econ. 44, 385–398. doi: 10.1111/agec.12023
Hong, H., Li, F. W., and Xu, J. (2019). Climate risks and market efficiency. J. Econ. 208, 265–281. doi: 10.1016/j.jeconom.2018.09.015
Hsiang, S. M. (2010). Temperatures and cyclones strongly associated with economic production in the Caribbean and Central America. Proc. Natl. Acad. Sci. USA 107, 15367–15372. doi: 10.1073/pnas.1009510107
Hu, X. Y., and Zheng, W. L. (2021). Climate change, agricultural risk and farmers' agricultural insurance purchasing behavior. J. Soc. Sci. Hunan Normal University 50, 95–104. doi: 10.19503/j.cnki.1000-2529.2021.02.010
Huang, H. C., and Qian, C. (2023). The influence of risk perception on farmers' rice income insurance purchase decision--a moderating role based on risk management behavior. J. Jiangxi University of Finance Econ. 1, 76–89. doi: 10.13676/j.cnki.cn36-1224/f.2023.01.008
Inner Mongolia Autonomous Region Forestry and Grassland Bureau. Grassland resources. (2024). Available at: https://lcj.nmg.gov.cn/lcgk_1/
Kjellstrom, T., Kovats, R. S., Lloyd, S. J., Holt, T., and Tol, R. S. J. (2009). The direct impact of climate change on regional labor productivity. Arch. Environ. Occup. Health 64, 217–227. doi: 10.1080/19338240903352776
Knight, T. O., and Coble, K. H.Survey of U.S. (1997). Multiple Peril Crop Insurance Since 1980. Rev. Agric. Econ. 19, 128–156. doi: 10.2307/1349683
Li, G. P., Li, J. L., and Xu, R. G. (2013). Development state of grassland animal husbandry in northern China and the preliminary study of the grassland ecological animal husbandry. J. Inner Mongolia University (Natural Sci. Edition) 44, 435–440. doi: 10.11702/nmgdxxbzk20130418
Li, L. L., Liu, Z. Q., Chen, J. Y., Wu, Y. C., and Li, H. (2022). Enhanced agriculture insurance with climate forecast. Sustain. For. 14:10617. doi: 10.3390/su141710617
Limskul, K. (2018). Climate change impact on labor productivity in thai manufacture. Asia Pac. J. Reg. Sci. 2, 195–209. doi: 10.1007/s41685-018-0073-5
Masson-Delmotte, V., Zhai, P., Pörtner, H.-O., Roberts, D., Skea, J., Shukla, P. R., et al. (2018). Global Warming of 1.5°C. Cambridge, UK and New York, NY, USA: Cambridge University Press. 2018, 1–2. doi: 10.1017/9781009157940
Piao, S., Ciais, P., Huang, Y., Shen, Z., Peng, S., Li, J., et al. (2010). The impacts of climate change on water resources and agriculture in China. Nature 467, 43–51. doi: 10.1038/nature09364
Qin, T., Zhu, C. X., Wang, J. H., and Zhang, J. (2023). Grassland insurance development mode, practical difficulties and system construction in China. J. Grassland Industry 32, 226–246. doi: 10.11686/cyxb2022471
Seppänen, O., Fisk, W. J., and Faulkner, D. (2003). Cost benefit analysis of the night-time ventilative cooling in office building, healthy. Buildings 27, 890–899. doi: 10.3901/CJME.2014.0618.113
Shaik, S., Coble, K. H., Knight, T. O., Baquet, A. E., and Patrick, G. F. (2008). Crop revenue and yield insurance demand: a subjective probability approach. J. Agric. Appl. Econ. 40, 757–766. doi: 10.1017/S1074070800002303
Shakoor, U., Saboor, A., Ali, I., and Mohsin, A. Q. (2011). Impact of climate change on agriculture: empirical evidence from arid region. Pak. J. Agric. Sci. 54, 331–352. doi: 10.1080/00288233.2011.613403
Shan, H. (2000). Characteristics of livestock production in inner Mongolian grassland pastoral areas. Inner Mongolia Sci. Economy 2000, 6–7.
Shang, Y., Xiong, T., and Li, C. G. (2020). Risk perception, risk attitude and Farmers' willingness to adopt risk management tools--the case of agricultural insurance and "insurance + futures". China Rural Observation 5, 52–72.
Shannon, H. D., and Motha, R. P. (2015). Managing weather and climate risks to agriculture in North America, Central America and the Caribbean, weather and climate. Extremes 10, 50–56. doi: 10.1016/j.wace.2015.10.006
Sherrick, B. J., Barry, P. J., Ellinger, P. N., and Schnitkey, G. D. (2004). Factors influencing farmers' crop insurance decisions. Am. J. Agric. Econ. 86, 103–114. doi: 10.1111/j.0092-5853.2004.00565.x
Stott, P. (2016). How climate change affects extreme weather events. Science 352, 1517–1518. doi: 10.1126/science.aaf7271
Sun, J. G., Han, K. Y., and Hu, J. Y. (2020). Does digital finance alleviate relative poverty? --an empirical study based on CHFS data. Finance Econ. Series 12, 50–60. doi: 10.13762/j.cnki.cjlc.2020.12.006
Task Force on Climate-related Financial Disclosures (TCFD). Recommendations of the Task Force on Climate-related Financial Disclosures, International Financial Stability Board. (2017). 5–6. Available at: https://assets.bbhub.io/company/sites/60/2020/10/FINAL-2017-TCFD-Report-11052018.pdf.
Wang, J. X., Huang, J. K., et al. (2014). Lmpacts of climate change on net crop revenue in north and South China. China Agricul. Econ. Rev. 6, 358–378. doi: 10.1108/CAER-12-2012-0138
Wang, J. B., and Yang, W. J. (2010). Climate change and effects on forage growth in the northeastern slope zone of the Tibetan plateau. Anhui Agricul. Sci. 38, 14535–14537. doi: 10.13989/j.cnki.0517-6611.2010.26.122
Welch, J. R., Vincent, J. R., Auffhammer, M., and Dawe, D. (2010). Rice yields in tropical / subtropical Asia exhibit large but opposing sensitivities to minimum and maximum temperatures. Proc. Natl. Acad. Sci. 107, 14562–14567. doi: 10.1073/pnas.1001222107
Wen, Z. L., Zhang, L., Hou, J. T., and Liu, H. Y. (2004). Mediation effect test procedure and its application. Aust. J. Psychol. 5, 614–620.
Xie, J. Z., and Pu, L. C. (2003). Research on government-induced agricultural insurance development model, insurance research 11, 42–44.
Ye, Z. H. (2018). Thoughts on improving China's agricultural insurance system. J. Financial Stud. 12, 174–188.
Zhang, Z. L. (2017). Research on the impact of climate change on pasture production in Qingnan area, Shanxi. Agric. Econ. 19, 85–86. doi: 10.16675/j.cnki.cn14-1065/f.2017.19.054
Zhang, Q., Han, L. Y., Hao, S. C., Han, T., Jia, J. Y., and Lin, J. (2015). Impact of climate change on drought loss rate in China and its regional variability from north to south. J. Meteorol. 6, 1092–1103. doi: 10.11676/qxxb2015.083
Zhang, Y. Y., Zhan, J. T., and Chen, C. (2017). Effects of specialization and awareness on farmers' demand for hog price index insurance. China Rural Econ. 2, 70–83.
Zheng, W. I., Hu, X. Y., and Luo, B. L. (2021). Effects of climate change on farmers’ agricultural insurance decision and its heterogeneity analysis. J. Statistics Info. 36, 66–74.
Zhou, S. D., and Zhu, H. G. (2010). Economic impact of climate change on rice yield in southern China and its adaptation strategies. China Population Resources Environ. 10, 152–157. doi: 10.3969/j.issn.1002-2104.2010.10.026
Keywords: climate risk, risk perception, risk management, agricultural insurance, herding behavior
Citation: Chen X-l and Zhao Y-f (2024) Study on the impact of climate risk on the agricultural insurance purchasing behavior of herding households—an empirical analysis based on Inner Mongolia. Front. Sustain. Food Syst. 8:1365536. doi: 10.3389/fsufs.2024.1365536
Received: 15 January 2024; Accepted: 17 July 2024;
Published: 09 August 2024.
Edited by:
Sanzidur Rahman, University of Reading, United KingdomReviewed by:
Chrysovalantis Malesios, Agricultural University of Athens, GreeceCopyright © 2024 Chen and Zhao. This is an open-access article distributed under the terms of the Creative Commons Attribution License (CC BY). The use, distribution or reproduction in other forums is permitted, provided the original author(s) and the copyright owner(s) are credited and that the original publication in this journal is cited, in accordance with accepted academic practice. No use, distribution or reproduction is permitted which does not comply with these terms.
*Correspondence: Yuan-feng Zhao, emhhb3lmQDI2My5uZXQ=
Disclaimer: All claims expressed in this article are solely those of the authors and do not necessarily represent those of their affiliated organizations, or those of the publisher, the editors and the reviewers. Any product that may be evaluated in this article or claim that may be made by its manufacturer is not guaranteed or endorsed by the publisher.
Research integrity at Frontiers
Learn more about the work of our research integrity team to safeguard the quality of each article we publish.