- 1Ecotecma S.A.S, Popayán, Colombia
- 2Universidad del Cauca, Facultad de Ingeniería Electrónica y Telecomunicaciones, Popayán, Colombia
- 3Centro de Desarrollo Tecnológico Cluster Creatic, Popayán, Colombia
Introduction: Climate change and weather variability pose significant challenges to small-scale crop production systems, increasing the frequency and intensity of extreme weather events. In this context, data modeling becomes a crucial tool for risk management and promotes producer resilience during losses caused by adverse weather events, particularly within agricultural insurance. However, data modeling requires access to available data representing production system conditions and external risk factors. One of the main problems in the agricultural sector, especially in small-scale farming, is data scarcity, which acts as a barrier to effectively addressing these issues. Data scarcity limits understanding the local-level impacts of climate change and the design of adaptation or mitigation strategies to manage adverse events, directly impacting production system productivity. Integrating knowledge into data modeling is a proposed strategy to address the issue of data scarcity. However, despite different mechanisms for knowledge representation, a methodological framework to integrate knowledge into data modeling is lacking.
Methods: This paper proposes developing a methodological framework (MF) to guide the characterization, extraction, representation, and integration of knowledge into data modeling, supporting the application of data solutions for small farmers. The development of the MF encompasses three phases. The first phase involves identifying the information underlying the MF. To achieve this, elements such as the type of knowledge managed in agriculture, data structure types, knowledge extraction methods, and knowledge representation methods were identified using the systematic review framework proposed by Kitchemhan, considering their limitations and the tools employed. In the second phase of MF construction, the gathered information was utilized to design the process modeling of the MF using the Business Process Model and Notation (BPMN).Finally, in the third phase of MF development, an evaluation was conducted using the expert weighting method.
Results: As a result, it was possible to theoretically verify that the proposed MF facilitates the integration of knowledge into data models. The MF serves as a foundation for establishing adaptation and mitigation strategies against adverse events stemming from climate variability and change in small-scale production systems, especially under conditions of data scarcity.
Discussion: The developed MF provides a structured approach to managing data scarcity in small-scale farming by effectively integrating knowledge into data modeling processes. This integration enhances the capacity to design and implement robust adaptation and mitigation strategies, thereby improving the resilience and productivity of small-scale crop production systems in the face of climate variability and change. Future research could focus on the practical application of this MF and its impact on small-scale farming practices, further validating its effectiveness and scalability.
1 Introduction
The development of agricultural insurance requires access to comprehensive data that accurately represents the conditions within productive systems and accounts for external risk factors. Currently, insurers employ techniques based on statistical and actuarial concepts to assess the conditions of the granted insurance and fulfill their acquired commitments. In this process, deficiencies in the mechanisms for determining insurance determinants are evident, stemming from a lack of understanding of the risk factors associated with agricultural activity and the vulnerability conditions of producers (Carter et al., 2017). Additionally, the non-stationary spatiotemporal structure of the data used for risk assessment introduces high complexity when a non-linear relationship between events and crop yield is present. Therefore, traditional statistical methods or other models may not be appropriate (Ghahari et al., 2019). By this, it is of great importance to propose alternatives that support the design of agricultural insurance, considering factors of data accessibility and availability in the agriculture domain.
At the farm level, crop yield data are either scarce or unavailable, impeding the estimation of individual losses due to a lack of representation and selection bias due to the high scarcity and low credibility of data at the local scale. Data scarcity can arise from the phenology of the assessed crops, as some have an extended development period, mainly perennial crops, making it challenging to obtain a historical data series. Additionally, in some productive systems, crop intercropping or rotation occurs, resulting in inconsistencies in data recording (Porth et al., 2019). Meanwhile, low credibility can be attributed to the fact that past data may not be representative of the current state of the productive system, owing to changes in management practices such as the use of technologies, application of agricultural inputs, and production arrangement, among others (Porth et al., 2014, 2019). These issues lead to the design of insurance being formulated based on regional or municipal data rather than local or farm scales, resulting in an aggregation bias. This bias may increase idiosyncratic risk by underestimating or overestimating the anticipated risk compared to the actual individual risk (Finger, 2012; Lyubchich et al., 2019), a situation known as base risk, one of the primary challenges associated with the design of agricultural insurance.
Base risk discourages producers from showing a low willingness to pay for agricultural insurance, owing to a lack of confidence in determining policy payments. In this regard, studies (Berg et al., 2009; Ramasubramanian, 2012; Thompson, 2017) evaluated the payment capability of producers, finding that they encounter issues with the insurance design, considering that payment is made based on an index constructed with data at the municipal or regional scale. Additionally, there are difficulties in comprehending the mechanisms for determining insurance policy payments. Therefore, it is pertinent to evaluate analytical methods that enhance the relationship between the indices determining policy payments and individual losses and increase transparency and trust in the methods employed to determine the proposed indices to improve their acquisition by producers.
Techniques based on machine learning, statistics, mechanistic or empirical models, or the integration of expert knowledge have been proposed to address the issue of base risk. Independently, each of these techniques presents drawbacks in its application. Mechanistic or empirical models have a high capacity to represent the complex processes of the agricultural system; however, their conception requires a high degree of knowledge of the system’s processes, and their application necessitates specific input data for validation within new scenarios (Tartarini et al., 2021). Due to their high heterogeneity, statistical techniques have limitations when analyzing data with different structures, frequencies, and scales (Ghahari et al., 2019). On the other hand, machine learning techniques are constrained or yield inadequate results when insufficient data is available for training and validating the developed models or when their development or outcome lacks a rational explanation within the framework of natural laws or human regulation (Von Rueden et al., 2019; Roscher et al., 2020). Based on the preceding, there is a need to propose mechanisms that allow for mitigating the disadvantages presented by the individual application of techniques and to leverage the advantages each offers. Accordingly, this paper proposes a methodological framework (MF) to facilitate knowledge’s characterization, extraction, representation, and integration into data modeling. This framework serves as a tool to support agricultural insurance design, particularly under data scarcity scenarios. The paper is structured as follows, the initial phase entails identifying the foundational information of the MF, employing the systematic review framework proposed by Kitchemhan (Kitchenham et al., 2009). The second phase of MF construction involves utilizing the gathered information to design the process model of the MF using the Business Process Model and Notation (BPMN; Chinosi and Trombetta, 2012). The third phase, involving an evaluation, was conducted employing the expert weighting method.
2 Materials and methods
A Methodological Framework (MF) provides the structure, elements, rules, and methods required to implement a particular process or a series of processes (McMeekin et al., 2020). Constructing an MF necessitates identifying data and information that underpin its development. In this regard, McMeekin et al. (2020) consolidates three phases from a literature review on MF development. The first phase corresponds to identifying evidence to inform the MF, initially considering the identification of utilized MFs, which will serve as the foundation for constructing the new framework. Secondly, unused data and information that aid in contextualizing the MF are identified. The second phase involves the development of the MF; in this phase, elements, processes, and techniques found in the recognized frameworks are adapted, combined, or complemented to structure the new framework.
Additionally, critical data identified in the second instance of phase one are extracted. The extracted information must be analyzed, synthesized, grouped, or merged into categories that will support the new MF, following an iterative approach until consensus is reached with experts, which will serve as a basis for refining the proposed framework. Finally, the third phase corresponds to the process of evaluating the MF.
In this regard, a macro-process is proposed for constructing an MF to support the implementation of agricultural insurance under a data scarcity scenario within the informed data analytics framework (Figure 1). The MF will consider the integration of different methodologies, which will be adapted within the guidelines proposed by McMeekin et al. (2020). The schematization of diagrams follows the procedures offered by the American National Standards Institute—ANSI (Zabinski, 2021).
In McMeekin et al. (2020), three (Porth et al., 2019) phases are established. The first corresponds to the identification of evidence to inform the MF (MFI), the second corresponds to the development of the MF (MFD), and the third corresponds to the evaluation and refinement of the MF (MFV). In MFI, one (Carter et al., 2017) macro-process is considered. It involves identifying new information supporting the new MF’s development (P1). On the other hand, in MFD, one (Carter et al., 2017) macro-process is established, focused on the iterative development process of the MF (P2). Finally, in MFV, one (Carter et al., 2017) macro-process is found, oriented toward evaluating and refining the MF (P3).
2.1 Phase 1. Identification of new data to support the MF
To develop the macro-process (Figure 2), we consider the six steps for conducting a systematic review as established in the methodology proposed by Kitchenham (2004). The steps are the planning phase (SR-0), research identification (SR-1), primary study selection (SR-2), study quality assessment (SR-3), the relevant information is extracted from the preliminary studies (SR-4), and synthesis of the results found in the primary studies (SR-5). In the SR-0 phase, research questions and protocol design are established. In SR-1, the search strategy for the systematic review is generated, publication bias is identified, the bibliography management process is determined, and the search documentation mechanism is established. Additionally, in SR-2, inclusion and exclusion criteria are set for study selection. In SR-3, quality thresholds are defined, and instruments for their assessment are designed. In SR-4, relevant information is extracted from the primary studies; the formats established in the review planning are utilized to achieve this. Finally, in SR-5, a synthesis of the results found in the prior studies is carried out for a case study. The extracted information is tabulated in a way that consistently answers the research questions posed in the previous stages.
According to the review objectives, we present the plan to build that below.
2.1.1 PICOC
This study employs the PICOC framework (García-Peñalvo, 2022), with the population defined as the agriculture and knowledge domain. The review is specifically directed toward identifying the elements utilized in knowledge management within the agricultural sector. Furthermore, the primary emphasis lies in identifying techniques, methods, and tools employed for extracting and representing knowledge. The “Comparison” component has not been considered, as there is no requirement for a specific comparison of the results obtained by applying identified methods or techniques.
• Population: Knowledge, agriculture
• Intervention: Methods or techniques for knowledge management
• Outcome: Describe methods or procedures for knowledge management in the field of agriculture
• Context: Systematic Review of methods or techniques for knowledge management in the field of agriculture
2.1.2 Research questions
Four research questions have been formulated, which are related to identifying the type of knowledge and data structure managed in knowledge management processes and identifying methods or techniques for knowledge extraction and representation in agriculture. Additionally, the identification of the most used tools for knowledge representation and the limitations of each recognized knowledge representation method have been addressed.
R1. What methods or techniques have been used to extract the different types of knowledge in agriculture?
R2. What methods or techniques have been used to represent knowledge in agriculture?
R3. What are the most commonly used techniques for knowledge extraction and representation?
R4. What are the main limitations posed by knowledge representation methods?
2.1.3 Keywords and synonyms
Following the procedural steps, keywords were chosen for the proposed research questions. These keywords will be instrumental in formulating search equations within bibliographic sources. The selected keywords encompass all types of activities undertaken in a knowledge management process.
2.1.4 Search string
An exploratory search equation was formulated, incorporating the critical term “agriculture” alongside all words associated with knowledge management processes. The equation was devised to address the review’s posed questions. The search scope did not concentrate on the agricultural insurance domain, as a preliminary review indicated insufficient data retrieval to inform the Methodological Framework (MF; agricultur*) AND (“knowledge elicitation” OR “knowledge harvesting” OR “expertise extraction” OR “expertise elicitation” OR “knowledge discovery” OR “knowledge extraction” OR “knowledge acquisition” OR “knowledge gathering” OR “knowledge revelation” OR “knowledge representation” OR “knowledge integration”) ≥ 2013.
2.1.5 Sources
The bibliographic sources IEEE, Scopus, and Web of Science are selected for their outstanding reputation and extensive coverage of scientific articles. The IEEE source is pivotal as it focuses explicitly on papers related to the engineering and data analytics component, providing a solid foundation for research in this field. On the other hand, Scopus and Web of Science span all knowledge areas, ensuring a comprehensive and multidisciplinary view of research. It is crucial for contextualizing and enriching the work, enabling the identification of interdisciplinary connections and emerging trends that may significantly contribute to the study at hand.
• IEEE1
• Scopus2
• WoS3
2.1.6 Selection criteria
About the selection criteria, consideration is given to studies that introduce new methods or replicate existing methods for knowledge extraction and representation. Additionally, studies corresponding to systematic reviews of the proposed topics are included, as they can provide comparative analyses or facilitate the identification of studies not captured by the formulated search equation. As for exclusion criteria, articles inaccessible through available databases are excluded, as some databases may have partial accessibility. Studies lacking descriptions of knowledge extraction or representation methods, those outside the domain of agriculture, and those lacking a clearly defined methodological and formal process are also excluded, as they lack a scientific foundation conducive to replication.
Inclusion Criteria:
• We select articles presenting novel methods or techniques for knowledge extraction or replicating existing ones.
• We choose research with new methods or techniques for knowledge representation or replicating existing ones.
• We pick papers incorporating a review as part of the research or where the review is the main objective.
• Finally, we sort out the most current version of an article in case of duplication across multiple sources.
We exclude papers:
• That is not accessible in the available databases.
• Outside the field of agriculture.
• That does not describe the required methods or techniques.
• The informal literature does not have a clearly defined research process.
2.1.7 Quality assessment checklist
In the quality evaluation process, criteria are considered to ensure that articles contain the necessary elements for the data extraction process. In this regard, articles that describe the methods or techniques for knowledge management (characterization, extraction, representation, and integration) are selected. These methods should not be solely based on expert opinions but should also offer sufficient information about the methodological process for obtaining the proposed results. The selected articles should also demonstrate that the methods or techniques used have been replicated in other studies or subjected to a rigorous evaluation. Furthermore, studies should acknowledge the limitations of the evaluated methods or approaches.
The established criteria are evaluated on a categorical scale, determining whether they fully, partially, or do not meet the specified criteria. Articles scoring equal to or above 4.0 are then chosen and proceed to the data extraction stage.
Questions:
• Is there a description of the methods or techniques for knowledge management?
• Are the results based on research rather than expert opinions?
• Do the articles provide sufficient information about the methodology and data used to develop or adapt the methods?
• Are the knowledge management methods presented in a practical case?
• Do the articles clearly state the limitations of the evaluated methods?
Answers:
• Yes
• Partially
• No
2.1.8 Data extraction form
Finally, to address the guiding questions of the review, the extraction of general information from the articles is considered to characterize the studies, such as the publication year and the specific application area within agriculture. Regarding the detailed required data, the type of data used in the analysis is considered to identify the handling of structured, unstructured, or semi-structured data. The kind of knowledge managed (explicit or implicit), the methods or techniques for knowledge extraction and representation, the tools (languages, software) used to apply methods, and the limitations identified in their application are also considered.
• Year
• Specific area of application
• Type of data used.
• Type of knowledge
• Extraction method or technique
• Representation method or technique
• Tools, languages, software
• Limitations
2.2 Phase 2. Development of the MF
Considering the information extracted, the development of the MF is constructed following the Business Process Notation and Modeling - BPMN. The Bizagi software (Bizagi, 2020) is employed to achieve this.
2.3 Phase 3. Assessment and refinement of the MF
For the evaluation of the MF, the expert weighting method was employed, which considers the following steps under Ishizaka and Nemery (2013):
• Expert Identification: assembling a group of experts in knowledge application and its integration into data analytics processes, especially in agriculture.
• Definition of Evaluation Criteria: in this case, the following evaluation criteria were proposed, taking into consideration aspects of clarity and comprehensibility, relevance and pertinence, adaptability and flexibility, and feasibility of implementation:
o C1: Is the Methodological Framework (MF) formulated and easily understandable for users and experts in data modeling?
o C2: Does the MF adequately address challenges related to integrating knowledge in data modeling?
o C3: Can the framework be adapted and applied in various data modeling contexts and situations?
o C4: Is implementing and effectively implementing the MF in real-world settings feasible?
o C5: Does the MF demonstrate activities related to characterization, extraction, and representation of knowledge?
o C6: Are the potential advantages and benefits of applying the MF in the data modeling context identified?
o C7: Does the MF address potential challenges that may arise during the knowledge management process in data modeling?
o C8: Is it possible to consider adaptations or updates to the MF without compromising the overall proposed structure?
• Definition of the Evaluation Scale: a scale from 1 to 5 was used, where 1 indicates low acceptance, and 5 indicates high acceptance.
• Calculation of the Average: based on the evaluations provided by the experts, a total weighted score was determined for each criterion.
• Verification of Consensus: a review of significant discrepancies between the weights assigned by the experts was conducted. If substantial differences are found, reaching a consensus with the experts is necessary. For evaluating the consistency between experts, the Intraclass Correlation Coefficient (ICCa) and Spearman’s coefficient were used. For the ICCa, the ranges established by Hills and Fleiss (1987) were considered (low if ICC < 0.40; good if 0.41 < ICC < 0.75; very good if ICC > 0.75). For Spearman’s coefficient, the correlation between experts ranges from 0 to 1, with values close to 1 indicating higher correlation.
• Utilization of the Evaluation for Decision-Making: based on the conducted evaluation, a decision was made on whether the MF requires changes or if, on the contrary, it remains as initially established. It ensures an iterative process in the development of the MF.
3 Results
3.1 Identification of new data to support the MM
Applying the protocol outlined in Figure 2 and considering the elements established in the systematic review planning, articles about knowledge management in agriculture were assessed between 2013 and 2023. A total of 481 articles were initially identified, resulting in a final count of 37 articles after removing duplicates, applying the defined exclusion and inclusion criteria, and conducting a quality assessment of the studies (Figure 3). This structure conforms to the requirements for an indexed journal submission.
Following the data extraction process, various types of knowledge, extraction methods, representation methods, their limitations, central areas of application, and the tools employed were identified. Regarding knowledge representation methods, it was observed that 40.4% of the studies utilized knowledge graphs, followed by ontologies at 34.6% and production rules at 25% (Figure 4).
On the other hand, Table 1 identifies the techniques employed in the data extraction process, noting the utilization of manual procedures such as interviews or the application of surveys with experts, alongside Natural Language Processing (NLP) techniques oriented toward entity recognition and relation extraction in unstructured data. Some of the tools employed for knowledge extraction and representation were also identified. There was a notable prevalence of the “Web Ontology Language - OWL,” used for knowledge representation in the Semantic Web, and the rule-oriented programming language CLIPS or one of its adaptations, such as Jess Rule, for knowledge representation through rules. Furthermore, in knowledge graphs, the Resource Description Framework (RDF) was identified as the primary means of representation. Additionally, the query language SPARQL was highlighted as essential for accessing and extracting information from RDF datasets.
Additionally, the main areas of intervention within the field of agriculture were identified, with pest and disease management accounting for 53.8%, comprehensive crop management at 19.2%, and nutritional management at 11.5% (Figure 5).
Regarding the data structure, 75% of the articles contemplate using unstructured data, encompassing text, images, audio, and video. 39% consider semi-structured data, and 12% pertain to structured data. Furthermore, the two types of knowledge considered in the knowledge management process were identified, with explicit knowledge comprising 92% of the studies and tacit knowledge accounting for 36% (Figure 6).
Finally, Table 2 presents some of the limitations of knowledge representation methods. At a general level, limitations were identified, such as the size of the knowledge base, the impact of the quality of input data on the reliability of the represented knowledge, the specificity of knowledge, which constrains its scalability, and the high requirement of experts for the creation and updating of the knowledge base. In ontologies, resistance may arise from formalizing specific agricultural domain knowledge, highlighting the challenge of representing knowledge with spatiotemporal characteristics.
3.2 Development of the MF (P2)
Based on the information gathered during the systematic review process, the Knowledge Management Framework (MF) was proposed for subsequent integration into data analytics. Initially, the MF was proposed using the flow diagram standard, and subsequently, the refined process involved applying the Business Process Model and Notation (BPMN).
Knowledge Characterization and Knowledge Extraction (KC and KE): in the initial phase of the proposed MF, the characterization process of the data scarcity issue was considered, along with an assessment of the required knowledge type and the identification of available knowledge sources. These sources may contain either implicit or explicit knowledge. Therefore, a selection process was defined through a gate establishing an inclusive flow, meaning that both types may be found within the same knowledge source.
In cases where the source contains tacit knowledge, an elicitation process was outlined to extract unstructured data, which is subsequently stored in a data repository. Next, an activity was defined to extract implicit knowledge from the unstructured data, utilizing the identified extraction methods. These methods align with the Natural Language Processing techniques described in Table 1 and any others that may be placed. This process yields semi-structured or structured data. In the event of semi-structured data, a normalization and transformation process were established to convert it into structured data. Conversely, if the extraction yields structured data, it is directly stored in a data repository.
Finally, in cases where the assessed knowledge is explicit, the type of data structure to be processed was determined through an exclusive gate. Depending on the structure, the same processes defined earlier will be followed.
Knowledge Representation (KR): in the second phase, corresponding to the knowledge representation process, the selection of the knowledge representation method was defined. This decision was informed by the data repository containing the various representation methods and their respective limitations (Table 2). These limitations were identified for each method (Table 2). Subsequently, the representation method was implemented, considering the data object containing the available tools (Table 1). Following this, an exclusive gate was set up to evaluate the response of the knowledge representation method to the defined data scarcity issue. Should the implementation of the representation method appropriately address the problem, the represented knowledge is then stored in a data repository.
Knowledge Integration (KI): in the third phase of the MF, corresponding to the process of knowledge integration in data modeling, the task of integrating the knowledge represented in one or more phases of data modeling was established. This selection will depend on the model optimization objectives. This task makes use of the data warehouse containing the represented knowledge. Similarly, the knowledge integration process is defined by an inclusive gate, allowing the integration of knowledge in one or more phases simultaneously. In this sense, the represented knowledge can facilitate business or data understanding, support the data preparation process, optimize the modeling process, or support the evaluation process of the generated models. Following the model evaluation, compliance with the established requirements for the model was defined through an exclusive gate to proceed with its deployment or iterate the evaluation process of the integration phase(s).
Finally, in Figure 7, the Methodological Framework is presented, articulating the three phases that support the knowledge management process and its integration into data analytics models.
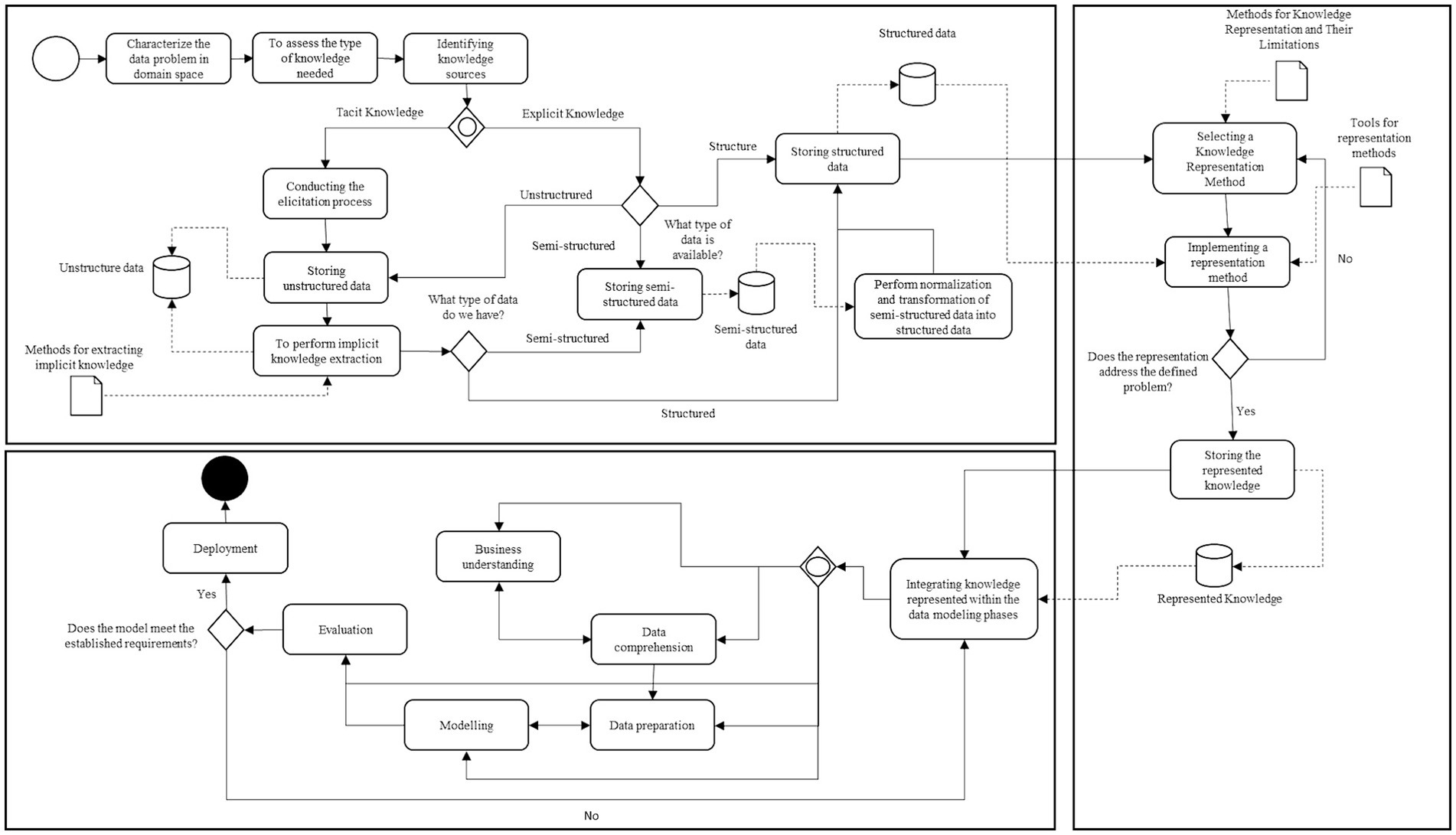
Figure 7. Methodological framework to support the integration of knowledge into data modeling under data scarcity scenarios.
3.3 Assessment and refinement of the MF
In this phase of the MF development process, the framework underwent evaluation and refinement conducted by four experts in the field of data analytics. Eight evaluation criteria were employed, encompassing aspects of clarity and comprehensibility, relevance and pertinence, adaptability and flexibility, and feasibility of implementation.
• C1: Is the Methodological Framework (MF) formulated and easily understandable for users and experts in data modeling?
• C2: Does the MF adequately address challenges related to integrating knowledge in data modeling?
• C3: Can the framework be adapted and applied in various data modeling contexts and situations?
• C4: Is implementing and effectively putting the MF into practice in real-world settings feasible?
• C5: Does the MF demonstrate activities related to characterization, extraction, and representation of knowledge?
• C6: Are the potential advantages and benefits of applying the MF in the data modeling context identified?
• C7: Does the MF address potential challenges that may arise during the knowledge management process in data modeling?
• C8: Is it possible to consider adaptations or updates to the MF without compromising the overall proposed structure?
Following the evaluation conducted by experts (Figure 8), the highest weighted scores were assigned to criteria 3 (Lyubchich et al., 2019), 4 (4.4), 5 (4.4), and 8 (4.4), reflecting aspects of adaptability, flexibility, relevance, and reliability in implementation. On the other hand, criteria 1 (3.4), 2 (3.4), 6 (3.2), and 7 (3.8) yielded lower averages, although not falling below the mean evaluation level. These criteria are associated with the clarity, comprehensibility, and relevance of the Methodological Framework (MF). The lowest weighted score was attributed to criterion 6, which pertains to identifying the advantages and benefits of applying MF in data analytics. Furthermore, considering the indicators used to evaluate the consistency among experts, the ICCa was satisfactory, with a value of 0.41. Additionally, the average Spearman coefficient among all experts was 0.85, indicating a high level of concordance.
Furthermore, in addition to the assigned rating for each established criterion, the experts provided recommendations to be considered in addressing the weaknesses identified in the MF (Table 3).
Based on the consolidated information, modifications were made to the MF (Figure 9):
• Specific conditions were established at each output of the inclusive gateway for knowledge integration in data modeling, defining the objectives sought through the implementation of knowledge in data modeling.
• A “data object” was added to describe various available methods for knowledge integration in data modeling.
• Stages of the knowledge management process were delimited and named using lanes.
• An activity was included to support the verification process of extracted tacit knowledge.
• The order for activities of knowledge characterization was reorganized.
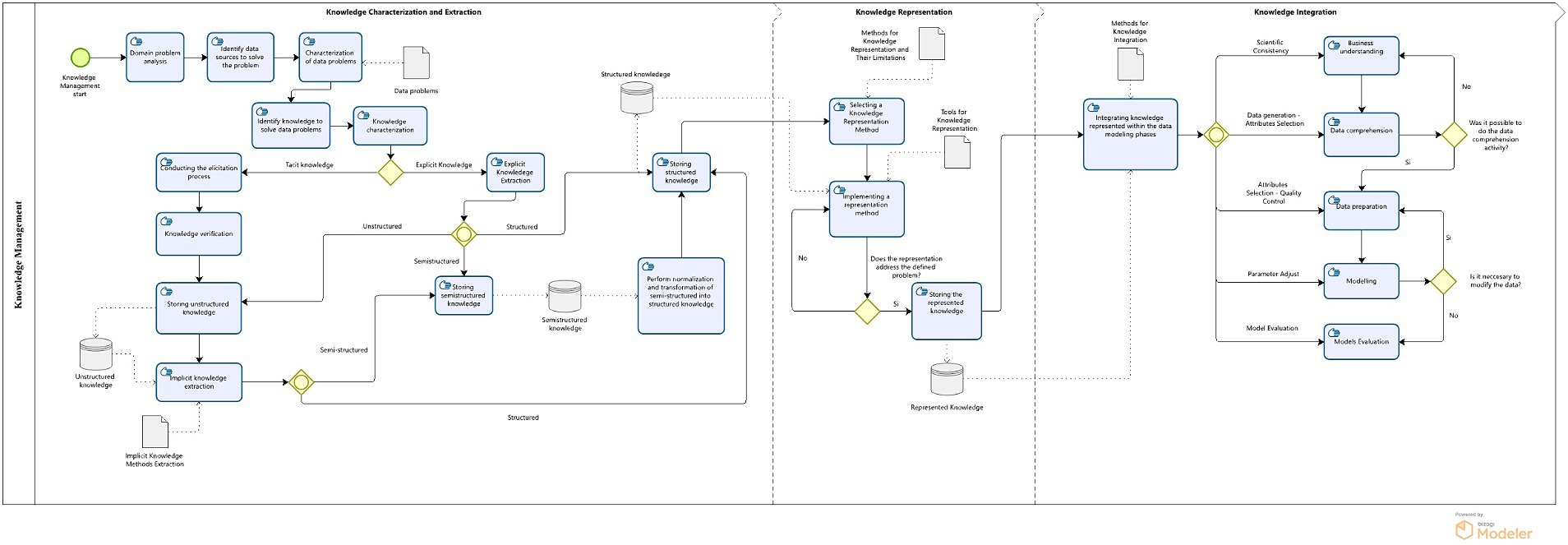
Figure 9. Methodological framework for knowledge characterization, extraction, representation and integration into data modeling.
4 Discussion
4.1 Knowledge characterization and extraction
The integration of knowledge into data modeling allows for a reduction in data dependence, an improvement in the precision and robustness of models, and, in some cases, confers physical meaning to the obtained results (Willard et al., 2020). Also, knowledge management strategies are critical for making decisions in climate change mitigations and adaptations to ensure better practices in small farming (Chisita and Fombad, 2020). In this context, some authors propose general frameworks for knowledge integration in data modeling, such as in Von Rueden et al. (2019), where the information flow in a process called informed machine learning is defined. This process generally involves problem identification and the search for a joint solution where data and prior knowledge are integrated, presenting some mechanisms for representing knowledge and its integration into data modeling. Similarly, in Roscher et al. (2020), an approach is proposed where the integration of domain knowledge is considered to improve the explainability of data models. Additionally, in Karpatne et al. (2017), despite not presenting a guide for knowledge management or its integration into data modeling, the paradigm of theory-guided data science is referred to, where the use of explicit and tacit knowledge is considered for refining the results of data models to be consistent with the understanding of physical phenomena.
Similarly, the proposed Methodological Framework (MF) is based on the general approach of integrating knowledge into data modeling. However, it delves into the processes by presenting specific activities to support the characterization, extraction, and representation of knowledge and its subsequent integration into data modeling. It considers the type of knowledge required, the type of data structure, and methods of knowledge extraction and representation, allowing for the support of the optimization of data models in their different development phases.
Regarding the characterization and extraction of knowledge, according to its origin and considering the types usually defined in the knowledge management area, it is classified as explicit and tacit knowledge (Hajric, 2018). Explicit knowledge, formalized and encoded, is called “Know-What.” This type of knowledge is found in the content of indexed journals, databases, public documents, reports, videos, and images, among others. Explicit knowledge is contained in files with different formats of structure, known as structured, semi-structured, or unstructured data, and treated by various methods to carry out the extraction process. For the extraction process of explicit knowledge, the MF considers the identification of the type of data structure where it is contained. Data extraction and direct storage are proposed when dealing with structured formats, thinking they possess a formal structure (Hajric, 2018). The performance of a normalization and transformation process from semi-structured to structured data is presented for semi-structured data. It is suggested by some authors who carry out the knowledge extraction process from HTML formats refined for the identification of concepts with the help of experts (Ahsan et al., 2014; Bonacin et al., 2016), the use of web crawlers to extract information directly from pages in HTML or XML formats (Baumgartner et al., 2005; Lin et al., 2018; Zhai et al., 2021) or the process of manual error correction, normalization, and standardization of semi-structured data to structured data suggested by Chenglin et al. (2018). This procedure is necessary to obtain data in a formal structure to be worked with using knowledge representation methods.
Similarly, tacit knowledge, known as “Know-How,” corresponds to that found in the minds of individuals and has not been quantified or represented in any accessible format. It is manifested through practices and experiences in the application domain (Rhem, 2005; Becerra-Fernandez and Sabherwal, 2014) and possesses defining characteristics such as difficulty in communication, practicality, experiential nature, unconsciousness, and personalization (Pérez-Fuillerat et al., 2019). In the MF, when the process of extracting tacit knowledge is carried out, knowledge elicitation methods are used, which involve storing extracted knowledge from experts in non-structured formats (Jakus et al., 2013). The use of elicitation methods depends on the characteristics of the users with whom the process will be developed. In the case of agriculture, some studies suggest the application of techniques such as knowledge harvesting (Frappaolo, 2008), storytelling (Whyte and Classen, 2012; Prasarnphanich et al., 2016; Zammit et al., 2018), interviews (Ferrari et al., 2016), and video sharing (Zammit et al., 2018).
Subsequently, when knowledge is contained in a non-structured data format, either through elicitation or explicit knowledge in this structure, the MF proposes a process of extracting knowledge considered implicit knowledge. It refers to patterns or relationships between data that are not evident to humans (Frappaolo, 2008). For this purpose, a tacit knowledge extraction task is established and supported by a data object containing extraction methods identified in the agriculture domain. Among the recognized methods, some studies report the use of manual tasks to carry out the extraction and categorization of data (Devraj and Deep, 2015; Bonacin et al., 2016; Goldstein et al., 2019; Admass, 2022). Similarly, some authors mention Natural Language Processing (NLP) techniques, such as the “Stanford Dependency Trees” structure used for extracting entities from the agricultural knowledge domain (Devi and Dua, 2017), Named Entity Recognition (NER) used for identifying and classifying entities from text into predefined categories (Chenglin et al., 2018), Predicate-Argument Structure (PAS) used to represent relationships between the predicate and its arguments (nouns, prepositional phrases, etc.) in a sentence (Chatterjee et al., 2019), Conditional Random Field (CRF), which corresponds to a probabilistic graphical model used for sequence labeling, and Syntactic Tree-based Relation Extraction, which uses syntactic trees to extract relationships between named entities in text (Xiaoxue et al., 2019). There are also tasks proposed by Gharibi et al. (2020), such as POS tagging, chunking, and Stanford Parser, which allow the identification of relevant words, their grouping into meaningful phrases, and the provision of a syntactic structure for understanding relationships between words. Finally, other authors mention the use of neural networks such as Lattice Long Short-Term Memory (LSTM), Structured Perceptron, or Bidirectional Gated Recurrent Init (bi-GRU; Kung et al., 2021; Zhu et al., 2021), used to process sequences of data in texts. These methods are necessary to identify patterns that are not explicit to humans and are present in the unstructured data used in the knowledge characterization process.
4.2 Knowledge representation
On the other hand, following Bergman (2018), knowledge representation is the description of an object through different elements. Knowledge representation comprises three main aspects: concepts as basic units of knowledge, associations or relationships between concepts, and a dynamic structure built by the concepts and their associations (Gutiérrez, 2012). Knowledge representation methods are applied to logical language resources, that is, formal and explicit language. Therefore, the Methodological Framework (MF) establishes a series of activities to extract and transform knowledge from unstructured and semi-structured data into a set of structured data that possess the required characteristics to implement representation methods (Staab and Studer, 2009). In this context, the MF delineates activities for selecting the knowledge representation method and its subsequent implementation. It is supported by data objects containing representation methods, their limitations, and the tools available to carry out the process.
The methods identified are production rules, generally used for procedural knowledge representation (Yingying et al., 2017), i.e., methods or processes for performing a task (Gutiérrez, 2012). In agriculture, it has been widely used, especially for supporting pest and disease management (Balleda et al., 2014; Devraj and Deep, 2015; Kalita et al., 2017; Yingying et al., 2017; Admass, 2022). In some cases, production rules are used with other knowledge representation methods, as in Yingying et al. (2017), where rules are combined with knowledge graphs to design an expression and reasoning model for diagnosing diseases in tomato cultivation. In Afzal and Kasi (2019), a knowledge model based on ontology was developed to support rice production, using rules to keep the reasoning process of the knowledge base created through ontology. Additionally, in Sottocornola et al. (2023), rules are employed to support the explanation process of diagnosis in treating diseases in apple cultivation.
However, the use of production rules in agriculture has limitations, such as the relatively small size of the constructed knowledge bases (Balleda et al., 2014); moreover, when working with semi-structured or unstructured data, metadata may be derived from unreliable sources due to incomplete or incorrect information (Gomez-Perez et al., 2017). Rule-based systems are limited to the dataset used for constructing the knowledge base, which may not represent all the dynamics of the addressed problem (Godara and Toshniwal, 2020). Similarly, rule-based models are limited by the quality of the rules and require extensive expert intervention in the domain for rule maintenance and updating. They also face challenges when attempting to scale to other problems, either within the same domain or outside of it (Nismi Mol and Santosh Kumar, 2023).
On the other hand, ontologies can be defined as a formal and explicit specification of a set of related concepts (Jakus et al., 2013). Some studies in agriculture have proposed the use of ontologies to improve semantic interoperability between developed systems and data sources (Bonacin et al., 2016; Stucky et al., 2018), design and build a knowledge base to support query systems (Devi and Dua, 2017; Aminu et al., 2019; Jearanaiwongkul et al., 2019), support the development of knowledge graphs serving as a design layer (Chenglin et al., 2018; Xiaoxue et al., 2019), provide lexical modeling and conceptualization to extracted knowledge (Yanchinda, 2019) or propose a semantic representation of IoT device data to reduce the need for human intervention (Afzal and Kasi, 2019). However, ontologies have limitations in their application, such as linguistic disambiguation. Expert keyword selection and query formulation may affect the quality of results, requiring a high availability of experts for any system scaling process. Many resources are needed for knowledge base maintenance.
Additionally, there is a low standardization of concepts in the agriculture domain, affecting ontology understanding and consistency, along with language barriers in which concepts used for ontology construction are found (Ahsan et al., 2014; Bonacin et al., 2016; Goldstein et al., 2019; Fahad et al., 2021; Kung et al., 2021; Malik et al., 2021). These limitations have led to the development of graphs as a novel mechanism for knowledge representation. It involves the extraction of entities, attributes, and their relationships, integrating knowledge through entity alignment and association with ontologies. Moreover, it facilitates the completion of the knowledge update and retrieval processes (Xiaoxue et al., 2019).
Like ontologies, knowledge graphs serve as a structured semantic knowledge base that describes concepts and their relationships in symbols (Xiaoxue et al., 2019). In this sense, graphs can be represented with varying levels of formalization, depending on whether one desires a lighter and more flexible representation or aims for knowledge representation with semantic consistency, integrating with an ontology that serves as a design layer for the knowledge graph (Chenglin et al., 2018). Under this, some authors have proposed the use of knowledge graphs with semantic support through ontologies to assess the impacts of agriculture and climate change on water resources (Bonacin et al., 2016), represent knowledge at a general level in the agricultural field (Ahsan et al., 2014; Devi and Dua, 2017; Chatterjee et al., 2019; Malik et al., 2021), automatically generate agrometeorological reports (Chenglin et al., 2018), address fertilization and soil management in corn cultivation (Aminu et al., 2019), support decision-making in pest and disease management (Goldstein et al., 2019; Jearanaiwongkul et al., 2021), and precision agriculture (Fahad et al., 2021).
Similarly, studies have been proposed to consider using knowledge graphs to support the wine sector, employing a lighter and more flexible representation, i.e., without being supported by an ontology (Abbal et al., 2016; Groumpos and Groumpos, 2016). Finally, like ontologies and production rules, knowledge graphs present similar limitations, such as low scalability to other knowledge domains and even to different areas within the same knowledge domain. The quality of the represented knowledge depends on the input data to the system (Chenglin et al., 2018), the need for labeled data for the application of machine learning models for entity and relationship extraction, and the necessity of domain knowledge experts for identifying or verifying meaningful semantic relationships among extracted concepts, which can consume significant resources (Chatterjee et al., 2019); moreover, graphs must undergo constant maintenance and updates, requiring a substantial allocation of resources due to the need for a high level of expertise in the knowledge domain (Xiaoxue et al., 2019). When selecting a knowledge representation method, the limitations of its application must be considered to address the problem appropriately.
4.3 Knowledge integration
Integrating knowledge into data modeling is of great interest, particularly in scenarios where data might be inaccessible, unavailable, or of low quality (Porth et al., 2019). Knowledge integration can occur at any phase of modeling (Von Rueden et al., 2023). Therefore, within the MF (Methodological Framework), a flow is established through an inclusive gate that allows the inclusion of knowledge represented in any data modeling phase. The conditions set in the inclusive gate include data generation (data understanding), model evaluation (model assessment), parameter adjustment (model development), scientific consistency (business understanding and model evaluation), and attribute selection (data preparation). In this regard, studies have been proposed related to knowledge integration in the data acquisition phase (Hain et al., 2011; Wang et al., 2017; Read et al., 2019; Yu et al., 2019; Clemens and Viechtbauer-Gruber, 2020; Downton et al., 2020; Zhao et al., 2020; Sepe et al., 2021; Yu et al., 2021; Raymond et al., 2022; Schröder et al., 2022), data preparation phase (Froehlich, 2020; Mudunuru and Karra, 2021; Bajracharya and Jain, 2022; Fuhg and Bouklas, 2022; Kohtz et al., 2022), optimization process of machine learning algorithms (Anoop Krishnan et al., 2018; Azari et al., 2020; Chadalawada et al., 2020; Huang et al., 2020; Qian et al., 2020; Sun et al., 2020; Tartakovsky et al., 2020; Jurj et al., 2021; Lu et al., 2021; Soriano et al., 2021; Kim et al., 2022), and as support for explaining data model results (MacInnes et al., 2010; Read et al., 2019).
Ontologies and knowledge graphs can support interoperability among knowledge domain datasets, verify the quality of extracted data, classify data, extract attributes or relationships, or facilitate working with heterogeneous data (Robinson and Haendel, 2020; Sahoo et al., 2022; Mummigatti et al., 2023). Furthermore, axioms established in an ontology can support constructing new ontologies by inducing the reuse of existing knowledge or verifying the consistency of the new ontology (Smith et al., 2007; Mungall et al., 2011). They can also expand or enrich the characteristics used in a machine learning model without finding relationships from the data, ensuring consistency or coherence through context rules (Kulmanov et al., 2021; Shrivastava and Deepak, 2023). Similarly, ontologies and graphs can be used for task prediction (Mazandu et al., 2017; Chen et al., 2021), text clustering (Wei et al., 2015; Ruas and Grosky, 2018; Mehta et al., 2021), or to support attribute reduction or selection (Garla and Brandt, 2012). These integrations are typically achieved through entity similarity or embedded entity methods (Deepa and Vigneshwari, 2019; Sun et al., 2020; Mežnar et al., 2022). Therefore, ontologies and knowledge graphs are highly useful in supporting the development of data models, especially in contexts such as small-scale agriculture, where historical data series are mostly unavailable, or the available data is of low quality.
On the other hand, in some cases, knowledge can be explicitly represented, allowing its integration into data modeling phases without any characterization or extraction process. In this regard, hybrid models that integrate results from mechanistic or empirical models have been developed, either for generating training data or for model evaluation data (Ji and Lu, 2018; Feng et al., 2019; Maya Gopal and Bhargavi, 2019; Saha et al., 2020; Sansana et al., 2021). Additionally, the integration of algebraic or differential equations has been proposed, which can be used to condition policy in learning, modify the error function, function parameterization, or as restrictive functions (Mangasarian and Wild, 2008; Karpatne et al., 2017; Lu et al., 2017; Muralidhar et al., 2019; Ramamurthy et al., 2019; Asvatourian et al., 2020; Gupta and Das, 2020; Meng et al., 2022). Similarly, invariance properties have been proposed to enhance the performance of machine learning models (Ling et al., 2016; Wu et al., 2018). Lastly, expert knowledge has been incorporated to ensure that results generated by machine learning models have scientific consistency (Brown et al., 2012; Choo et al., 2013; Spinner et al., 2020). Thus, knowledge integration depends on the improvement objectives sought concerning data models.
4.4 The methodological framework as a tool for risk management in small-scale farming
The increase in variability and climate change, diseases, and pests, among other problems, negatively impacts agriculture, particularly affecting small-scale producers who are highly vulnerable and have low resilience. Additionally, food security relies on the adaptive capacity of small-scale producers to address such events (Hatfield et al., 2020). A significant amount of research has proposed data methods to contribute to solving these problems (Xie, 2011; Ghahari et al., 2019) (Dalhaus et al., 2018; Wang et al., 2018; Mangani and Kousalya, 2019; Roznik et al., 2019; Shirsath et al., 2019; Boyd et al., 2020; Zhang et al., 2020). However, the information used has different temporal and spatial resolution, affecting its correct application at the local level. At the local level, farmers possess knowledge about practices and techniques; however, this local knowledge can vary from one agricultural region to another. In this context, knowledge extraction and representation can be useful for storing knowledge from heterogeneous sources and sharing it with farmers (Jearanaiwongkul et al., 2019; Haider et al., 2021). Furthermore, there is an exponential amount of data about farm management and system conditions, necessitating proper methods to represent and share this data to support farmers’ activities (Aminu et al., 2019; Goldstein et al., 2019; Bhuyan et al., 2022). For this reason, in the context of small-scale farming, it is necessary to complement data analysis with knowledge that can support model development, considering data scarcity and heterogeneity.
Another problem where the Framework can be useful is addressing the lack of financial data to support risk management in the context of financial inclusion. In this sense, knowledge about system conditions or agronomic management may be necessary for develop new instruments for improvement. The Methodological Framework (MF) can facilitate the extraction and representation of knowledge from various sources to build new tools, such as credit scoring, while considering the heterogeneity of diverse agricultural systems (Simumba et al., 2018; Bunnell et al., 2021).
In the context of agricultural insurance, the management and integration of knowledge in data modeling will enable the proposition of agricultural insurance design solutions, facilitating the reduction of aggregation bias by considering specific characteristics of the production system. These include crop phenology, access conditions or availability of primary resources and implementing techniques or practices that enhance or diminish producers’ adaptive capacity. Additionally, it may facilitate the integration of area-related knowledge, such as agroecological classifications or soil types. This adjustment would fine-tune the utilization of the proposed parametric index, consequently mitigating idiosyncratic risks. It also aims to minimize gaps in insurance acquisition stemming from poor design comprehension or a weak correlation between premium payments and individual-level losses (Berg et al., 2009; Ramasubramanian, 2012; Thompson, 2017; Fonta et al., 2018; Madaki et al., 2023).
The optimization of data models through knowledge can provide producers with more adaptive tools to enhance their resilience against variability and climate change events, diseases, pest control, and all agronomic management factors contributing to food security and economic growth in small-scale agriculture.
5 Conclusion and recommendations
The Methodological Framework (MF) is a tool designed to guide researchers in knowledge management. It defines techniques and methods for knowledge characterization, extraction, representation, and integration into data modeling to support data model development, particularly in risk management in small-scale agriculture. One of the main challenges in knowledge representation is that knowledge can be specific to one domain and might not apply to others. Therefore, it is essential to increase research on methods for data interoperability and knowledge sharing and evaluate reasoning characteristics.
Additionally, it is crucial to continue research on techniques for knowledge extraction, considering the significant amount of heterogeneous data and information sources (such as images, text, audio, and video) that can support development in the agricultural sector. Particular attention should be given to methods or techniques used for knowledge extraction from unstructured data.
It should be noted that the Methodological Framework (MF) was evaluated through an expert consensus. For this reason, it is considered a proposal, and it is crucial to apply the framework to address problems in small-scale farming, especially when there is a significant lack of consistent and high-quality data available. An example of such application is the design of agricultural insurance in small-scale farming, with an emphasis on the processes of index selection, data preparation, and determination of optimal triggers, exit thresholds, and premium calculation.
Data availability statement
The raw data supporting the conclusions of this article will be made available by the authors, without undue reservation.
Author contributions
JCA: Conceptualization, Methodology, Writing – original draft, Writing – review & editing. JCO: Conceptualization, Writing – review & editing.
Funding
The author(s) declare that financial support was received for the research, authorship, and/or publication of this article. This research was funded by the Department of Cauca, the Sistema General de Regalías de Colombia (SGR), and the Cluster CreaTIC project. Additionally, this work is part of the research project ‘Incremento de la Oferta de Prototipos Tecnológicos en Estado Pre-Comercial Derivados de Resultados de I + D Para el Fortalecimiento del Sector Agropecuario en el Departamento del Cauca’ (grant no: BPIN 2020000100098), funded by the Sistema General de Regalías (SGR) of Colombia.
Acknowledgments
The authors are grateful to the Telematics Engineering Group (GIT) of the University of Cauca, Cluster Creatic, and the Sistema General de Regalías de Colombia (SGR).
Conflict of interest
JCA was employed by the company Ecotecma S.A.S.
The author declares that the research was conducted in the absence of any commercial or financial relationships that could be construed as a potential conflict of interest.
Publisher’s note
All claims expressed in this article are solely those of the authors and do not necessarily represent those of their affiliated organizations, or those of the publisher, the editors and the reviewers. Any product that may be evaluated in this article, or claim that may be made by its manufacturer, is not guaranteed or endorsed by the publisher.
Footnotes
1. ^https://ieeexplore-ieee-org.ezproxy.unal.edu.co/
References
Abbal, P., Sablayrolles, J. M., Matzner-Lober, É., Boursiquot, J. M., Baudrit, C., and Carbonneau, A. (2016). A decision support system for vine growers based on a Bayesian network. J. Agric. Biol. Environ. Stat. 21, 131–151. doi: 10.1007/s13253-015-0233-2
Admass, W. S. (2022). Developing knowledge-based system for the diagnosis and treatment of mango pests using data mining techniques. Int. J. Inf. Technol. 14, 1495–1504. doi: 10.1007/s41870-022-00870-8
Afzal, H, and Kasi, MK. Ontology-based knowledge modeling for rice crop production. In: Proceedings - 2019 international conference on future internet of things and cloud, FiCloud 2019. (2019).
Agustina, E, Pratomo, I, Wibawa, AD, and Rahayu, S. Expert system for diagnosis pests and diseases of the rice plant using forward chaining and certainty factor method. In: 2017 international seminar on intelligent technology and its application: Strengthening the link between university research and industry to support ASEAN energy sector, ISITIA 2017 - proceeding. (2017).
Ahsan, M, Motla, YH, and Asim, M. Knowledge modeling fore-agriculture using ontology. In: ICOSST 2014–2014 international conference on open source systems and technologies, proceedings. (2014).
Aminu, EF, Oyefolahan, IO, Abdullahi, MB, and Salaudeen, MT. An OWL based ontology model for soils and fertilizations knowledge on maize crop farming: scenario for developing intelligent systems. In: 2019 15th international conference on electronics, Computer and Computation, ICECCO (2019). 2019.
Anoop Krishnan, N. M., Mangalathu, S., Smedskjaer, M. M., Tandia, A., Burton, H., and Bauchy, M. (2018). Predicting the dissolution kinetics of silicate glasses using machine learning. J. Non-Cryst. Solids 487, 37–45. doi: 10.1016/j.jnoncrysol.2018.02.023
Asvatourian, V., Leray, P., Michiels, S., and Lanoy, E. (2020). Integrating expert’s knowledge constraint of time dependent exposures in structure learning for Bayesian networks. Artif. Intell. Med. 107:101874. doi: 10.1016/j.artmed.2020.101874
Azari, A. R., Lockhart, J. W., Liemohn, M. W., and Jia, X. (2020). Incorporating physical knowledge into machine learning for planetary space physics. Front. Astronomy Space Sci. 7:7. doi: 10.3389/fspas.2020.00036
Bajracharya, P., and Jain, S. (2022). Hydrologic similarity based on width function and hypsometry: An unsupervised learning approach. Comput. Geosci. 163:105097. doi: 10.1016/j.cageo.2022.105097
Balleda, K, Satyanvesh, D, NVSSP, Sampath, KTN, Varma, and Baruah, PK. Agpest: An efficient rule-based expert system to prevent pest diseases of rice & wheat crops. In: 2014 IEEE 8th international conference on intelligent systems and control: Green challenges and smart solutions, ISCO 2014 - proceedings. (2014).
Ballot, R., Loyce, C., Jeuffroy, M. H., Ronceux, A., Gombert, J., Lesur-Dumoulin, C., et al. (2018). First cropping system model based on expert-knowledge parameterization. Agron. Sustain. Dev. 38:33. doi: 10.1007/s13593-018-0512-8
Baumgartner, R, Frölich, O, Gottlob, G, Herzog, M, and Lehmann, P. Integrating semi-structured data into business applications: a web intelligence example. In: Lecture notes in computer science (including subseries lecture notes in artificial intelligence and lecture notes in bioinformatics). (2005).
Becerra-Fernandez, I., and Sabherwal, R. (2014). Knowledge management: systems and processes. 2nd Edn, (New York: Routledge), p. 382.
Berg, A., Quirion, P., and Sultan, B. (2009). Weather-index drought insurance in Burkina-Faso: assessment of its potential interest to farmers. Weather, Climate, and Society. 1, 71–84. doi: 10.1175/2009WCAS1008.1
Bhuyan, B. P., Tomar, R., and Cherif, A. R. (2022). A systematic review of knowledge representation techniques in smart agriculture (urban). Sustainability (Switzerland). 14, 1049–1055. doi: 10.3390/su142215249
Bonacin, R., and Nabuco OFPierozzi, J. I. (2016). Ontology models of the impacts of agriculture and climate changes on water resources: scenarios on interoperability and information recovery. Futur. Gener. Comput. Syst. 54, 423–434. doi: 10.1016/j.future.2015.04.010
Boyd, M., Porth, B., Porth, L., Seng Tan, K., Wang, S., and Zhu, W. (2020). The Design of Weather Index Insurance Using Principal Component Regression and Partial Least Squares Regression: the case of forage crops. North American Actuarial J. 24, 355–369. doi: 10.1080/10920277.2019.1669055
Brown, ET, Liu, J, Brodley, CE, and Chang, R. Dis-function: learning distance functions interactively. In: IEEE conference on visual analytics science and technology 2012, VAST 2012 - proceedings. (2012).
Bunnell, L., Osei-Bryson, K. M., and Yoon, V. Y. (2021). Development of a consumer financial goals ontology for use with FinTech applications for improving financial capability. Expert Syst. Appl. 165:113843. doi: 10.1016/j.eswa.2020.113843
Carter, M., De Janvry, A., Sadoulet, E., and Sarris, A. (2017). Index Insurance for Developing Country Agriculture: a reassessment. Ann. Rev. Resour. Econ. 9, 421–438. doi: 10.1146/annurev-resource-100516-053352
Chadalawada, J., Herath, H. M. V. V., and Babovic, V. (2020). Hydrologically informed machine learning for rainfall-runoff modeling: a genetic programming-based toolkit for automatic model induction. Water Resour. Res. 56, 1430–1436. doi: 10.1029/2019WR026933
Chatterjee, N., Kaushik, N., and Bansal, B. (2019). Inter-subdomain relation extraction for agriculture domain. IETE Techn. Rev. (Institution of Electronics and Telecommun. Engineers, India). 36, 157–163. doi: 10.1080/02564602.2018.1435312
Chen, J., Althagafi, A., and Hoehndorf, R. (2021). Predicting candidate genes from phenotypes, functions and anatomical site of expression. Bioinformatics 37, 853–860. doi: 10.1093/bioinformatics/btaa879
Chenglin, Q, Qing, S, Pengzhou, Z, and Hui, Y. (2018). Cn-MAKG: China meteorology and agriculture knowledge graph construction based on semi-structured data. In: Proceedings - 17th IEEE/ACIS international conference on computer and information science, ICIS 2018.
Chinosi, M., and Trombetta, A. (2012). BPMN: An introduction to the standard. Comput Stand Interfaces. 34, 124–134. doi: 10.1016/j.csi.2011.06.002
Chisita, C. T., and Fombad, M. (2020). Knowledge Management for Climate Change Adaptation to enhance urban agriculture among selected Organisations in Zimbabwe. J. Inf. Knowl. Manag. 19:2050009. doi: 10.1142/S0219649220500094
Choo, J., Lee, C., Reddy, C. K., and Park, H. (2013). UTOPIAN: user-driven topic modeling based on interactive nonnegative matrix factorization. IEEE Trans. Vis. Comput. Graph. 19, 1992–2001. doi: 10.1109/TVCG.2013.212
Clemens, T., and Viechtbauer-Gruber, M. (2020). Impact of digitalization on the way of working and skills development in hydrocarbon production forecasting and project decision analysis. SPE Reserv. Eval. Eng. 23, 1358–1372. doi: 10.2118/200540-PA
Dalhaus, T., Musshoff, O., and Finger, R. (2018). Phenology information contributes to reduce temporal basis risk in agricultural weather index insurance. Sci. Rep. 8:46. doi: 10.1038/s41598-017-18656-5
Deepa, R., and Vigneshwari, S. (2019). A novel HNN-DOC for automated agricultural ontology construction on climate factors. Int. J. Recent Technol. Engineer. 8, 6040–6042. doi: 10.35940/ijrte.C5586.098319
Devi, M, and Dua, M. ADANS: An agriculture domain question answering system using ontologies. In: Proceeding - IEEE international conference on computing, Communication and Automation, ICCCA. (2017). 2017.
Devraj, Jain R, and Deep, V. Expert system for the management of insect-pests in pulse crops. In: 2015 international conference on computing for sustainable global development, INDIACom 2015. (2015).
Downton, J. E., Collet, O., Hampson, D. P., and Colwell, T. (2020). Theory-guided data science-based reservoir prediction of a North Sea oil field. Lead. Edge 39, 742–750. doi: 10.1190/tle39100742.1
Fahad, M., Javid, T., Beenish, H., Siddiqui, A. A., and Ahmed, G. (2021). Extending ONTAgri with service-oriented architecture towards precision farming application. Sustainability (Switzerland). 13:9801. doi: 10.3390/su13179801
Feng, P., Wang, B., Liu, D. L., Waters, C., and Yu, Q. (2019). Incorporating machine learning with biophysical model can improve the evaluation of climate extremes impacts on wheat yield in South-Eastern Australia. Agric. For. Meteorol. 275, 100–113. doi: 10.1016/j.agrformet.2019.05.018
Ferrari, A., Spoletini, P., and Gnesi, S. (2016). Ambiguity and tacit knowledge in requirements elicitation interviews. Requir. Eng. 21, 333–355. doi: 10.1007/s00766-016-0249-3
Finger, R. (2012). Biases in farm-level yield risk analysis due to data aggregation. German J. Agric. Econ. 61, 30–43.
Fonta, W. M., Sanfo, S., Kedir, A. M., and Thiam, D. R. (2018). Estimating farmers’ willingness to pay for weather index-based crop insurance uptake in West Africa: insight from a pilot initiative in southwestern Burkina Faso. Agric. Food Econ. 6, 1531–1532. doi: 10.1186/s40100-018-0104-6
Frappaolo, C. (2008). Implicit knowledge. Knowl. Manag. Res. Pract. 6, 23–25. doi: 10.1057/palgrave.kmrp.8500168
Froehlich, D. C. (2020). Neural network prediction of alluvial stream Bedforms. J. Hydraul. Eng. 146. doi: 10.1061/(ASCE)HY.1943-7900.0001831
Fuhg, J. N., and Bouklas, N. (2022). On physics-informed data-driven isotropic and anisotropic constitutive models through probabilistic machine learning and space-filling sampling. Comput Methods Appl Mech Eng 394:114915. doi: 10.48550/arXiv.2109.11028
Gaikwad, S, Asodekar, R, Gadia, S, and Attar, VZ. AGRI-QAS question-answering system for agriculture domain. In: 2015 international conference on advances in computing, communications and informatics, ICACCI 2015. (2015).
García-Peñalvo, F. J. (2022). Developing robust state-of-the-art reports: Systematic Literature Reviews. Educ. Knowledge Society. 23, 307–308. doi: 10.14201/eks.28139
Garla, V. N., and Brandt, C. (2012). Ontology-guided feature engineering for clinical text classification. J. Biomed. Inform. 45, 992–998. doi: 10.1016/j.jbi.2012.04.010
Ghahari, A., Newlands, N. K., Lyubchich, V., and Gel, Y. R. (2019). Deep learning at the Interface of agricultural insurance risk and Spatio-temporal uncertainty in weather extremes. N. Am. Actuar. J. 23, 535–550. doi: 10.1080/10920277.2019.1633928
Gharibi, M., Zachariah, A., and Rao, P. (2020). FoodKG: a tool to enrich knowledge graphs using machine learning techniques. Front Big Data. 3:3. doi: 10.3389/fdata.2020.00012
Godara, S., and Toshniwal, D. (2020). Sequential pattern mining combined multi-criteria decision-making for farmers’ queries characterization. Comput. Electron. Agric. 173:105448. doi: 10.1016/j.compag.2020.105448
Goldstein, A., Fink, L., Raphaeli, O., Hetzroni, A., and Ravid, G. (2019). Addressing the “tower of babel” of pesticide regulations: An ontology for supporting pest-control decisions. J. Agric. Sci. 157, 493–503. doi: 10.1017/S0021859619000820
Gomez-Perez, P, Phan, TN, and Kueng, J. Agricultural knowledge extraction from text sources using a distributed MapReduce cluster. In: Proceedings - international workshop on database and expert systems applications, DEXA. (2017).
Groumpos, PP, and Groumpos, VP. Modeling vineyards using fuzzy cognitive maps. In: 24th Mediterranean conference on control and automation, MED 2016. (2016).
Gupta, H, and Das, Sheng VS. A roadmap to domain knowledge integration in machine learning. In: Proceedings - 11th IEEE international conference on knowledge graph, ICKG 2020. (2020).
Haider, W., Rehman, A. U., Durrani, N. M., and Rehman, S. U. (2021). A generic approach for wheat disease classification and verification using expert opinion for knowledge-based decisions. IEEE Access. 9, 31104–31129. doi: 10.1109/ACCESS.2021.3058582
Hain, D. J., Wands, L., and Liehr, P. (2011). Approaches to resolve health challenges in a population of older adults undergoing hemodialysis. Res. Gerontol. Nurs. 4, 53–62. doi: 10.3928/19404921-20100330-01
Hajric, E. (2018). Knowledge management: system and practices a theoretical and practical guide for knowledge Management in Your Organization. Eur. Manag. J. 19.
Hatfield, J. L., Antle, J., Garrett, K. A., Izaurralde, R. C., Mader, T., Marshall, E., et al. (2020). Indicators of climate change in agricultural systems. Clim. Chang. 163, 1719–1732. doi: 10.1007/s10584-018-2222-2
Hills, M., and Fleiss, J. L. (1987). The design and analysis of clinical experiments. J. R. Stat. Soc. Ser. A 150:400. doi: 10.2307/2982050
Huang, Q., Wang, Y., Lyu, M., and Lin, W. (2020). Shape deviation generator-a convolution framework for learning and predicting 3-D printing shape accuracy. IEEE Trans. Autom. Sci. Eng. 17, 1–15. doi: 10.1109/TASE.2019.2959211
Ishizaka, A, and Nemery, P. (2013). Multi-criteria decision analysis: Methods and software. John Wiley & Sons.
Jakus, G., Milutinović, V., Omerović, S., and Tomažič, S. (2013). “Concepts BT - concepts, ontologies, and knowledge representation” in Concepts, ontologies, and knowledge representation. eds. G. Jakus, V. Milutinović, S. Omerović, and S. Tomažič (New York, NY: Springer New York), 5–27.
Jearanaiwongkul, W., Anutariya, C., and Andres, F. (2019). A semantic-based framework for Rice Plant disease management: identification, early warning, and treatment recommendation using multiple observations. N. Gener. Comput. 37, 499–523. doi: 10.1007/s00354-019-00072-0
Jearanaiwongkul, W., Anutariya, C., Racharak, T., and Andres, F. (2021). An ontology-based expert system for rice disease identification and control recommendation. App. Sci. (Switzerland). 11, 841–842. doi: 10.3390/app112110450
Ji, X., and Lu, J. (2018). Forecasting riverine total nitrogen loads using wavelet analysis and support vector regression combination model in an agricultural watershed. Environ. Sci. Pollut. Res. 25, 26405–26422. doi: 10.1007/s11356-018-2698-3
Jurj, S. L., Grundt, D., Werner, T., Borchers, P., Rothemann, K., and Möhlmann, E. (2021). Increasing the safety of adaptive cruise control using physics-guided reinforcement learning. Energies (Basel). 14:7572. doi: 10.3390/en14227572
Kalita, H, Sarma, SK, and Choudhury, RD. Expert system for diagnosis of diseases of rice plants: prototype design and implementation. In: International conference on automatic control and dynamic optimization techniques, ICACDOT 2016. (2017).
Karpatne, A., Atluri, G., Faghmous, J. H., Steinbach, M., Banerjee, A., Ganguly, A., et al. (2017). Theory-guided data science: a new paradigm for scientific discovery from data. IEEE Trans. Knowl. Data Eng. 29, 2318–2331. doi: 10.1109/TKDE.2017.2720168
Karpatne, A., Watkins, W., Read, J., and Kumar, V. (2017). Physics-guided neural networks (PGNN): An application in lake temperature modeling. arXiv.
Kim, K. M., Hurley, P., and Duarte, J. P. (2022). Physics-informed machine learning-aided framework for prediction of minimum film boiling temperature. Int. J. Heat Mass Transf. 191:122839. doi: 10.1016/j.ijheatmasstransfer.2022.122839
Kitchenham, B. (2004). Procedures for performing systematic reviews. UK and National ICT Australia: Keele University, 33.
Kitchenham, B., Pearl Brereton, O., Budgen, D., Turner, M., Bailey, J., and Linkman, S. (2009). Systematic literature reviews in software engineering - a systematic literature review. Inf. Softw. Technol. 51, 7–15. doi: 10.1016/j.infsof.2008.09.009
Kohtz, S., Xu, Y., Zheng, Z., and Wang, P. (2022). Physics-informed machine learning model for battery state of health prognostics using partial charging segments. Mech. Syst. Signal Process. 172, 172:109002. doi: 10.1016/j.ymssp.2022.109002
Kulmanov, M., Smaili, F. Z., Gao, X., and Hoehndorf, R. (2021). Semantic similarity and machine learning with ontologies. Brief. Bioinform. 22, 1448–1449. doi: 10.1093/bib/bbaa199
Kung, H. Y., Yu, R. W., Chen, C. H., Tsai, C. W., and Lin, C. Y. (2021). Intelligent pig-raising knowledge question-answering system based on neural network schemes. Agron. J. 113, 906–922. doi: 10.1002/agj2.20622
Lin, Y., Jun, Z., Hongyan, M., Zhongwei, Z., and Zhanfang, F. (2018). A method of extracting the semi-structured data implication rules. Procedia Computer Sci. 131, 706–716. doi: 10.1016/j.procs.2018.04.315
Ling, J., Kurzawski, A., and Templeton, J. (2016). Reynolds averaged turbulence modelling using deep neural networks with embedded invariance. J. Fluid Mech. 807, 155–166. doi: 10.1017/jfm.2016.615
Lu, D., Konapala, G., Painter, S. L., Kao, S. C., and Gangrade, S. (2021). Streamflow simulation in data-scarce basins using bayesian and physics-informed machine learning models. J. Hydrometeorol. 22, 1421–1438. doi: 10.1175/JHM-D-20-0082.1
Lu, Y., Rajora, M., Zou, P., and Liang, S. Y. (2017). Physics-embedded machine learning: case study with electrochemical micro-machining. Mach. Des. 5, 1470–1471. doi: 10.3390/machines5010004
Lyubchich, V., Newlands, N. K., Ghahari, A., Mahdi, T., and Gel, Y. R. (2019). Insurance risk assessment in the face of climate change: integrating data science and statistics. Wiley Interdis. Rev.: Computational Stat. 11:e1462. doi: 10.1002/wics.1462
MacInnes, J., Santosa, S., and Wright, W. (2010). Visual classification: expert knowledge guides machine learning. IEEE Comput. Graph. Appl. 30, 8–14. doi: 10.1109/MCG.2010.18
Madaki, M. Y., Kaechele, H., and Bavorova, M. (2023). Agricultural insurance as a climate risk adaptation strategy in developing countries: a case of Nigeria. Clim. Pol. 23, 747–762. doi: 10.1080/14693062.2023.2220672
Malik, N., Hijam, D., and Sharan, A. (2021). Ontology based knowledge representation: case study from agriculture domain. Int. J. Knowledge-Based and Intel. Engineer. Syst. 25, 97–108. doi: 10.3233/KES-210055
Mangani, K. P., and Kousalya, R. (2019). Designing weather based crop insurance payout estimation based on agro-meteorological data using machine learning techniques. Int. J. Recent Technol. Engineer. 8, 2953–2960. doi: 10.35940/ijrte.C4806.098319
Mangasarian, O. L., and Wild, E. W. (2008). Nonlinear knowledge-based classification. IEEE Trans. Neural Netw. 19, 1826–1832. doi: 10.1109/TNN.2008.2005188
Maya Gopal, P. S., and Bhargavi, R. (2019). A novel approach for efficient crop yield prediction. Comput. Electron. Agric. 165:104968. doi: 10.1016/j.compag.2019.104968
Mazandu, G. K., Chimusa, E. R., and Mulder, N. J. (2017). Gene ontology semantic similarity tools: survey on features and challenges for biological knowledge discovery. Brief. Bioinform. 18:bbw067. doi: 10.1093/bib/bbw067
McMeekin, N., Wu, O., Germeni, E., and Briggs, A. (2020). How methodological frameworks are being developed: evidence from a scoping review. BMC Med. Res. Methodol. 20:173. doi: 10.1186/s12874-020-01061-4
Mehta, V., Bawa, S., and Singh, J. (2021). Stamantic clustering: combining statistical and semantic features for clustering of large text datasets. Expert Syst. Appl. 174:114710. doi: 10.1016/j.eswa.2021.114710
Meng, H., An, X., and Xing, J. (2022). A data-driven Bayesian network model integrating physical knowledge for prioritization of risk influencing factors. Process. Saf. Environ. Prot. 160, 434–449. doi: 10.1016/j.psep.2022.02.010
Mežnar, S., Bevec, M., Lavrač, N., and Škrlj, B. (2022). Ontology completion with graph-based machine learning: a comprehensive evaluation. Mach Learn Knowl Extr. 4, 1107–1123. doi: 10.3390/make4040056
Mudunuru, M. K., and Karra, S. (2021). Physics-informed machine learning models for predicting the progress of reactive-mixing. Comput. Methods Appl. Mech. Eng. 374:113560. doi: 10.1016/j.cma.2020.113560
Mummigatti, K. V. K., Chandramouli, S. M., and Ramachandra, D. H. (2023). Deep neural network system using ontology to recommend organic fertilizers for a sustainable agriculture. Ingenierie des Systemes d’Information. 28, 461–467. doi: 10.18280/isi.280222
Mungall, C. J., Bada, M., Berardini, T. Z., Deegan, J., Ireland, A., Harris, M. A., et al. (2011). Cross-product extensions of the gene ontology. J. Biomed. Inform. 44, 80–86. doi: 10.1016/j.jbi.2010.02.002
Muralidhar, N, Islam, MR, Marwah, M, Karpatne, A, and Ramakrishnan, N. Incorporating prior domain knowledge into Deep neural networks. In: Proceedings - 2018 IEEE international conference on big data, big data 2018. (2019).
Nismi Mol, E. A., and Santosh Kumar, M. B. (2023). Review on knowledge extraction from text and scope in agriculture domain. Artif. Intell. Rev. 56, 4403–4445. doi: 10.1007/s10462-022-10239-9
Pérez-Fuillerat, N., Solano-Ruiz, M. C., and Amezcua, M. (2019). Conocimiento tácito: características en la práctica enfermera. Gac. Sanit. 33, 191–196. doi: 10.1016/j.gaceta.2017.11.002
Porth, L., Tan, K. S., and Zhu, W. (2019). A relational data matching model for enhancing individual loss experience: An example from crop insurance. N. Am. Actuar. J. 23, 551–572. doi: 10.1080/10920277.2019.1634595
Porth, L., Zhu, W., and Tan, K. S. (2014). A credibility-based Erlang mixture model for pricing crop reinsurance. Agricul. Finance Rev. 74, 162–187. doi: 10.1108/AFR-04-2014-0006
Prasarnphanich, P., Janz, B. D., and Patel, J. (2016). Towards a better understanding of system analysts’ tacit knowledge: a mixed method approach. Inf. Technol. People 29, 69–98. doi: 10.1108/ITP-06-2014-0123
Qian, E., Kramer, B., Peherstorfer, B., and Willcox, K. (2020). Lift & learn: physics-informed machine learning for large-scale nonlinear dynamical systems. Physica D. 406:132401. doi: 10.1016/j.physd.2020.132401
Ramamurthy, R, Bauckhage, C, Sifa, R, Schücker, J, and Wrobel, S. Leveraging domain knowledge for reinforcement learning using MMC architectures. In: Lecture notes in computer science (including subseries lecture notes in artificial intelligence and lecture notes in bioinformatics). (2019).
Ramasubramanian, J. A. (2012). Willingness to pay for index based crop insurance in India. USA: NEUDC 2012, Darmouth College.
Raymond, S. J., Cecchi, N. J., Alizadeh, H. V., Callan, A. A., Rice, E., Liu, Y., et al. (2022). Physics-informed machine learning improves detection of head impacts. Ann. Biomed. Eng. 50, 1534–1545. doi: 10.1007/s10439-022-02911-6
Read, J. S., Jia, X., Willard, J., Appling, A. P., Zwart, J. A., Oliver, S. K., et al. (2019). Process-guided Deep learning predictions of Lake water temperature. Water Resour. Res. 55, 9173–9190. doi: 10.1029/2019WR024922
Robinson, P. N., and Haendel, M. A. (2020). Ontologies, knowledge representation, and machine learning for translational research: recent contributions. Yearb. Med. Inform. 29, 159–162. doi: 10.1055/s-0040-1701991
Roscher, R., Bohn, B., Duarte, M. F., and Garcke, J. (2020). Explainable machine learning for scientific insights and discoveries. IEEE Access. 8, 42200–42216. doi: 10.1109/ACCESS.2020.2976199
Rousi, M., Sitokonstantinou, V., Meditskos, G., Papoutsis, I., Gialampoukidis, I., Koukos, A., et al. (2021). Semantically enriched crop type classification and linked earth observation data to support the common agricultural policy monitoring. IEEE J Sel Top Appl Earth Obs Remote Sens. 14, 529–552. doi: 10.1109/JSTARS.2020.3038152
Roznik, M., Brock Porth, C., Porth, L., Boyd, M., and Roznik, K. (2019). Improving agricultural microinsurance by applying universal kriging and generalised additive models for interpolation of mean daily temperature. Geneva Papers on Risk and Insurance: Issues Prac. 44, 446–480. doi: 10.1057/s41288-019-00127-9
Ruas, T, and Grosky, W. Semantic feature structure extraction from documents based on extended lexical chains. In: GWC 2018 - 9th global WordNet conference. (2018).
Saha, A., Singh, K. N., Ray, M., and Rathod, S. (2020). A hybrid spatio-temporal modelling: an application to space-time rainfall forecasting. Theor. Appl. Climatol. 142:1271. doi: 10.1007/s00704-020-03374-2
Sahoo, S. S., Kobow, K., Zhang, J., Buchhalter, J., Dayyani, M., Upadhyaya, D. P., et al. (2022). Ontology-based feature engineering in machine learning workflows for heterogeneous epilepsy patient records. Sci. Rep. 12:19430. doi: 10.1038/s41598-022-23101-3
Sansana, J., Joswiak, M. N., Castillo, I., Wang, Z., Rendall, R., Chiang, L. H., et al. (2021). Recent trends on hybrid modeling for industry 4.0. Comput. Chem. Eng. 151:107365. doi: 10.1016/j.compchemeng.2021.107365
Schröder, L., Dimitrov, N. K., Verelst, D. R., and Sørensen, J. A. (2022). Using transfer learning to build physics-informed machine learning models for improved wind farm monitoring. Energies (Basel). 15:558. doi: 10.3390/en15020558
Sepe, M., Graziano, A., Badora, M., di Stazio, A., Bellani, L., Compare, M., et al. (2021). A physics-informed machine learning framework for predictive maintenance applied to turbomachinery assets. J. Global Power and Propulsion Society 2021, 1–15. doi: 10.33737/jgpps/134845
Shirsath, P., Vyas, S., Aggarwal, P., and Rao, K. N. (2019). Designing weather index insurance of crops for the increased satisfaction of farmers, industry and the government. Clim. Risk Manag. 25:100189. doi: 10.1016/j.crm.2019.100189
Shrivastava, RR, and Deepak, G. BiCropRec: a bi-classifier approach for crop recommendation based on inclusion of semantic intelligence and topic modelling. In: Lecture notes in networks and systems. (2023).
Simumba, N, Okami, S, Kodaka, A, and Kohtake, N. Alternative scoring factors using non-financial data for credit decisions in agricultural microfinance. In: 4th IEEE international symposium on systems engineering, ISSE 2018 - proceedings. (2018).
Smith, B., Ashburner, M., Rosse, C., Bard, J., Bug, W., Ceusters, W., et al. (2007). The OBO foundry: coordinated evolution of ontologies to support biomedical data integration. Nat. Biotechnol. 25, 1251–1255. doi: 10.1038/nbt1346
Soriano, M. A., Siegel, H. G., Johnson, N. P., Gutchess, K. M., Xiong, B., Li, Y., et al. (2021). Assessment of groundwater well vulnerability to contamination through physics-informed machine learning. Environ. Res. Lett. 16:084013. doi: 10.1088/1748-9326/ac10e0
Sottocornola, G., Baric, S., Stella, F., and Zanker, M. (2023). Development of a knowledge-based expert system for diagnosing post-harvest diseases of apple. Agriculture (Switzerland). 13, 1295–1296. doi: 10.3390/agriculture13010177
Spinner, T., Schlegel, U., Schäfer, H., and El-Assady, M. (2020). ExplAIner: a visual analytics framework for interactive and explainable machine learning. IEEE Trans. Vis. Comput. Graph. 26:1. doi: 10.1109/TVCG.2019.2934629
Stucky, B. J., Guralnick, R., Deck, J., Denny, E. G., Bolmgren, K., and Walls, R. (2018). The plant phenology ontology: a new informatics resource for large-scale integration of plant phenology data. Front. Plant Sci. 9:9. doi: 10.3389/fpls.2018.00517
Sun, L., Gao, H., Pan, S., and Wang, J. X. (2020). Surrogate modeling for fluid flows based on physics-constrained deep learning without simulation data. Comput. Methods Appl. Mech. Eng. 361:112732. doi: 10.1016/j.cma.2019.112732
Sun, Z., Zhang, Q., Hu, W., Wang, C., Chen, M., Akrami, F., et al. (2020). A benchmarking study of embeddingbased entity alignment for knowledge graphs. Proceed. VLDB Endowment 13, 2326–2340. doi: 10.14778/3407790.3407828
Tartakovsky, A. M., Marrero, C. O., Perdikaris, P., Tartakovsky, G. D., and Barajas-Solano, D. (2020). Physics-informed Deep neural networks for learning parameters and constitutive relationships in subsurface flow problems. Water Resour. Res. 56:e2019WR026731. doi: 10.1029/2019WR026731
Tartarini, S., Vesely, F., Movedi, E., Radegonda, L., Pietrasanta, A., Recchi, G., et al. (2021). Biophysical models and meta-modelling to reduce the basis risk in index-based insurance: a case study on winter cereals in Italy. Agric. For. Meteorol. 300:108320. doi: 10.1016/j.agrformet.2021.108320
Thompson, M. (2017). Willingness to pay for agricultural risk insurance as a strategy to adapt climate change. Accounting & FinanceUNU-MERIT. 28.
Von Rueden, L., Mayer, S., Beckh, K., Georgiev, B., Giesselbach, S., Heese, R., et al. (2019). Informed machine learning - a taxonomy and survey of integrating knowledge into learning systems. arXiv. 3, 614–633.
Von Rueden, L., Mayer, S., Beckh, K., Georgiev, B., Giesselbach, S., Heese, R., et al. (2023). Informed machine learning - a taxonomy and survey of integrating prior knowledge into learning systems. IEEE Trans. Knowl. Data Eng. 35:1. doi: 10.1109/TKDE.2021.3079836
Wang, X. W., Du, M. Z., Wang, L., Li, M. X., Xu, Y. Y., Liu, X. Y., et al. (2018). Design of the continuous rainfall days index insurance of peanut in Henan Province. Chinese J. Ecol. 37, 3390–3395. doi: 10.48550/arXiv.1606.07987
Wang, J. X., Wu, J. L., and Xiao, H. (2017). Physics-informed machine learning approach for reconstructing Reynolds stress modeling discrepancies based on DNS data. Phys Rev Fluids. 2:034603. doi: 10.1103/PhysRevFluids.2.034603
Wei, T., Lu, Y., Chang, H., Zhou, Q., and Bao, X. (2015). A semantic approach for text clustering using WordNet and lexical chains. Expert Syst. Appl. 42, 2264–2275. doi: 10.1016/j.eswa.2014.10.023
Whyte, G., and Classen, S. (2012). Using storytelling to elicit tacit knowledge from SMEs. J. Knowl. Manag. 16, 950–962. doi: 10.1108/13673271211276218
Willard, J., Jia, X., Xu, S., Steinbach, M., and Kumar, V. (2020). Integrating Scientific Knowledge with Machine Learning for Engineering and Environmental Systems. ACM Comp Syst. 1, 1–35. doi: 10.48550/arXiv.2003.04919
Wu, J. L., Xiao, H., and Paterson, E. (2018). Physics-informed machine learning approach for augmenting turbulence models: a comprehensive framework. Phys Rev Fluids. 3, 1474–1475. doi: 10.1103/PhysRevFluids.3.074602
Xiaoxue, L., Xuesong, B., Longhe, W., Bingyuan, R., Shuhan, L., and Lin, L. (2019). Review and trend analysis of knowledge graphs for crop Pest and diseases, vol. 7: IEEE Access, 62251–62264.
Xie, Y. (2011). Values and limitations of statistical models. Res Soc Stratif Mobil. 29, 343–349. doi: 10.1016/j.rssm.2011.04.001
Yanchinda, J. Effective ontologies based on the STEM learning concept of organic Rice knowledge using knowledge engineering. In: International symposium on wireless personal multimedia communications, WPMC. (2019).
Yingying, L, Xiaoyan, Z, Xiaodong, Z, Shibin, L, Na, Z, and Min, S. Knowledge expression and reasoning model for tomato disease diagnosis. In: 2017 3rd international conference on information management, ICIM 2017. (2017).
Yu, T., Canales-Rodríguez, E. J., Pizzolato, M., Piredda, G. F., Hilbert, T., Fischi-Gomez, E., et al. (2021). Model-informed machine learning for multi-component T2 relaxometry. Med. Image Anal. 69:101940. doi: 10.1016/j.media.2020.101940
Yu, T., Li, Z., and Wu, D. (2019). Predictive modeling of material removal rate in chemical mechanical planarization with physics-informed machine learning. Wear 426-427, 1430–1438. doi: 10.1016/j.wear.2019.02.012
Zabinski, J. (2021). “American National Standards Institute (ANSI)” in Encyclopedia of computer science and technology. 3rd Edn. Eds. M. J. Bates and M. N. Maack.(CRC Press) p. 3.
Zammit, J., Gao, J., Evans, R., and Maropoulos, P. (2018). A knowledge capturing and sharing framework for improving the testing processes in global product development using storytelling and video sharing. Proc. Inst. Mech. Eng. B J. Eng. Manuf. 232, 2286–2296. doi: 10.1177/0954405417694062
Zhai, Z., Chen, X., Zhang, Y., and Zhou, R. (2021). Decision-making technology based on knowledge engineering and experiment on the intelligent water-fertilizer irrigation system. J. Comput. Methods Sci. Engineer. 21, 665–684. doi: 10.3233/JCM-215117
Zhang, J., Zhang, Z., and Tao, F. (2020). Rainfall-related weather indices for three Main crops in China. Int. J. Disaster Risk Sci. 11, 466–483. doi: 10.1007/s13753-020-00283-w
Zhao, X., Shirvan, K., Salko, R. K., and Guo, F. (2020). On the prediction of critical heat flux using a physics-informed machine learning-aided framework. Appl. Therm. Eng. 164:114540. doi: 10.1016/j.applthermaleng.2019.114540
Keywords: methodological framework, small-scale farming, risk management, knowledge management, data modelling
Citation: Casanova Olaya JF and Corrales JC (2024) A methodological framework proposal for managing risk in small-scale farming through the integration of knowledge and data analytics. Front. Sustain. Food Syst. 8:1363744. doi: 10.3389/fsufs.2024.1363744
Edited by:
Leida Y. Mercado, Centro Agronomico Tropical De Investigacion Y Ensenanza Catie, Costa RicaReviewed by:
Iván Darío López, TBBC, ColombiaGordana Radović, Institute of Agricultural Economics, Serbia
Copyright © 2024 Casanova Olaya and Corrales. This is an open-access article distributed under the terms of the Creative Commons Attribution License (CC BY). The use, distribution or reproduction in other forums is permitted, provided the original author(s) and the copyright owner(s) are credited and that the original publication in this journal is cited, in accordance with accepted academic practice. No use, distribution or reproduction is permitted which does not comply with these terms.
*Correspondence: Juan Fernando Casanova Olaya, juancasanova@unicauca.edu.co