- 1Department of Agricultural Extension and Education, Agricultural Sciences and Natural Resources University of Khuzestan, Mollasni, Ahvaz, Iran
- 2Department of Agricultural Extension and Education, Agricultural Sciences and Natural Resources University of Khuzestan & Visiting Researcher Member of Mohaghegh Ardabili University, Ardabil, Iran
- 3Dezab Consulting Engineering Company, Khuzestan, Ahvaz, Iran
Today, chemical inputs play an important and crucial role in increasing the production rate of agricultural products and the income of farmers in Iran. However, in parallel with this beneficial effect, the widespread and indiscriminate use of such inputs is considered to be one of the most significant barriers to environmental sustainability in Iran. The main objective of this research is to model the acceptance of the use of biological inputs among farmers living in the southwest of Iran. The research study was conducted using the cross-sectional survey method. The statistical population included all farmers of Meidavoud district located in Baghmalek county, southwest of Iran (N = 1,400). A sample of 302 individuals was selected according to Morgan’s sample size table and random sampling method. A questionnaire was used to collect the data, the validity of which was confirmed by a panel of experts, and its reliability was approved by various coefficients. The data were analyzed using SPSSV26, Smart PLS3, and AMOS software. The results of the structural equations showed that among the variables influencing farmers’ behavior toward biological inputs, the attitude and willingness variables could predict 80% of the variation in farmers’ behavior. In addition, attitude, ease of use, and usefulness were identified as effective factors in farmers’ willingness to use biological inputs. According to the results of the path analysis, the variables perceived usefulness and ease of use could predict 67% of the changes in farmers’ attitudes. To convince farmers and promote desirable attitudes that, in turn, influence their willingness to use biological inputs, some persuasive interventions seem to be necessary. Farmers need to be externally or internally motivated if they adopt environmentally friendly practices such as use of biological inputs.
1 Introduction
An increase in the world’s population has created a significant need for agricultural products in terms of quality and quantity (Lahlali et al., 2022); hence, the effort to ensure food security against population growth has increased the demand for food (Hawkins et al., 2019), leading to exceeding use of chemical fertilizers and pesticides along with the significant increase in crop yields in the 20th century (Bagheri et al., 2021). The dark side of this movement was applied dramatically to polluted soil, water, and environment, leading to ecological and environmental problems and human health risks (Rodrigues et al., 2022; Sehrawat et al., 2022). Residues of chemical compounds in the environment have caused resistance of weeds, pest, and disease species to chemical toxic materials, severe soil destruction and erosion, water pollution, and threat to human health (in terms of skin diseases, different cancer types, neurological diseases, diabetes, respiratory diseases, fetal insufficiency and diseases, congenital malformation, fertility problems, and genetic problems; Recena et al., 2006; Fianko et al., 2011; Chen et al., 2013; Fan et al., 2015; García-García et al., 2016; Jallow et al., 2017; Bondori et al., 2019, 2021, 2023; Bagheri et al., 2019b, 2021, 2022; Imani et al., 2021; Asante et al., 2023; Bawa, 2023; Dias et al., 2023; Imani et al., 2023; Terfe et al., 2023). However, in recent decades, consumers have become more aware and concerned about the side effects of agrochemicals, which have been highlighted in various studies [Lahlali et al. (2022) on fungicides; Carvalho (2017); Damalas and Eleftherohorinos (2011) on pesticides; Govindasamy et al. (1997) on pesticides, herbicides, fungicides, and insecticides; Önder et al. (2011); Bishnoi (2018) on chemical fertilizers; and Zhang et al. (2018) on chemical inputs used in agriculture]. As public concerns about the impact of chemicals on food safety and environmental protection increased, environmentally friendly management methods, such as biological control and biofertilizers, emerged (Dukare et al., 2019; Wang et al., 2022). As noted by Goulet (2021), the category of biological inputs is broader than the definition of biocontrol; biological inputs include both biocontrol agents and biofertilizers.
From the perspective of ecologists, biological control is known as the best alternative to pesticides (Wu et al., 2005; Bagheri et al., 2016) and one of the key components of integrated pest management strategies (Abdollahzadeh et al., 2015). Biological control means using natural enemies of external origin for permanent pest control (Shafiee et al., 2018; Gholifar et al., 2019). This strategy strengthens the ability and willingness of farmers to control all types of pests ensuring the effectiveness of reducing the population of harmful living organisms and increasing the safety of plants (Sharifzadeh et al., 2017). The most obvious benefit of this type of control is related to food security, human health, and environmental protection by controlling the population of agricultural pests, disease vectors, and invasive species (Rodrigues et al., 2022). Wheeler (2008) and Bagheri et al. (2021) stated that substances used in biological control do not produce residues or create toxic hazards. Therefore, they are not harmful to the environment and health. Moreover, biological control does not affect other organisms (non-target species) that are profitable for the agro-environment. In other words, it can be claimed that biological control has become a useful tool to reach sustainability in the agricultural sector (Rodrigues et al., 2022). While this newly replaced method for pest control can bring many benefits, it has not been successful in practice and sufficiently accepted by producers (Abdollahzadeh et al., 2015); still, many farmers are reluctant to use this method. Usually, they prefer chemical control operations instead of biological control (Safa et al., 2019).
No doubtfully, chemical inputs play an important and determining role in increasing the production rate of agricultural products and farmers’ income in Iran. However, in parallel with this beneficial effect, the widespread and indiscriminate use of such inputs is considered one of the most important barriers to Iran’s environmental sustainability. According to the latest report of the environmental performance index published in 2022, Iran has been ranked 133 showing a score of 34.50 among the 180 countries in the world, and more specifically, it ranked 45, 31, and 113 (worst performance) in agricultural sector, pesticide usage, and sustainable nitrogen management index, respectively, which indicates inappropriate environmental performance in this area (Wolf et al., 2022). As such, today, the fundamental challenge is how to change the behaviors and attitudes of farmers in using chemical inputs in such a way that could provide human health and, furthermore, prevent environmental pollution.
The gradual integration of new methods, considering the agricultural production system, requires not only the environmental dimension but also the social one (Purvis et al., 2019). Therefore, a deep and accurate understanding of farmers’ behavior is essential to accept biological control (Abdollahzadeh et al., 2015; Sharifzadeh et al., 2017). The success of any intervention in terms of activities and programs related to sustainable agriculture (like acceptance of biological control) depends on the use of social-psychological patterns and models (Safa et al., 2019). This study, therefore, was aimed to investigate the factors affecting farmers’ behavior and willingness to accept biological control. Many studies have been conducted on the acceptance of integrated pest management strategies, in general (Habibzadeh Shojaei and Sharifzade, 2014; Sabzian et al., 2015; Bagheri et al., 2019b; Imani et al., 2021), and biological control methods, in particular (Niyaki et al., 2010; Gangadhar et al., 2012; Abdollahzadeh et al., 2015; Ashoori et al., 2015; Shahani, 2020; Bagheri et al., 2021). The main framework of research in these studies was based on the behavioral models, in which the willingness of individuals to accept a particular behavior through different perceptual and cognitive-social processes is explained. Among a variant of environmental psychology theories, which are often used to investigate the factors affecting environmental performance, the Norm Activation Model (developed by Schwartz, 1977), Theory of Planned Behavior (developed by Ajzen, 1991), and Value-Belief-Norm theory (introduced by Stern, 2000) are more popular. Although these theories have been applied to predict the intention toward engaging in environmental behaviors, previous empirical studies have revealed that the technology acceptance model (TAM), in comparison with the rational action or planned behavior model, can account for a significant proportion of the variance (typically approximately 40%) in explaining the adoption behavior (Jokar et al., 2017).
The technology acceptance model (TAM) has been used in several studies, including those investigating biological inputs and organic farmers. Bagheri et al. (2021) used the technology acceptance model (TAM) to measure the acceptance and use of biological inputs by cereal farmers and found that attitudes and perceived usefulness were influential variables in their intention and behavior. In another study, Bagheri et al. (2016) also investigated the use of TAM in the adoption of biological control in the case of rice stem borer and found a positive correlation between perceived usefulness and perceived ease of use with farmers’ willingness to adopt biological control. Using the TAM, Silva et al. (2017) also investigated the intention of bean growers for integrated production in the central region of Brazil and showed that perceived usefulness had a positive effect on attitude and attitude influenced behavioral intentions. In the study of soil conservation behavior among cereal farmers, based on the extended TAM, Ahmadi Firouzjaie et al. (2023) found that perceived usefulness had a direct effect on perceived ease of use. The results also showed that behavioral intention is the most important factor explaining soil conservation behavior. Farmers’ perceived ease of use is recognized as one of the most important factors influencing intention. Naspetti et al. (2017) applied an extended version of TAM to examine attitudes and intentions toward sustainable production strategies among dairy farmers and found that perceived usefulness was the significant determinant of behavioral intention. Hendrawan et al. (2023) used TAM to explain smallholder farmers’ behavior in relation to the use of information and communication technology. Their results indicated that perceived usefulness was more important than perceived ease of use in farmers’ daily activities. Hannus and Sauer (2021), in their research study, used the conceptual framework of TAM to investigate farmers’ intentions to use sustainability standards and came to the conclusion that ease of use was the most effective factor in influencing farmers’ intentions. Chen et al. (2024), in their TAM-based study of farmers’ intentions to adopt live-streaming e-commerce, showed that TAM is quite capable of assessing farmers’ intentions to adopt live-streaming e-commerce. In addition, their results suggested that government support positively influenced perceived usefulness, social learning, and improved perceived ease of use and platform support positively influenced both perceived ease of use and usefulness. McCormack et al. (2022) investigated the ability of TAM to predict farmers’ adoption of an online nutrient management plan. They proposed that perceived usefulness and perceived ease of use were positively and significantly related to farmers’ intention to adopt and use the technology in the future. However, perceived usefulness, which captures the perceived benefits in terms of usefulness, was the main driver of technology adoption. Accordingly, in our research, the important factors affecting the acceptance of biological inputs have been investigated using the TAM model, which measures farmers’ behavior and willingness to accept the use of biological inputs at the same time and, in this respect, differs from the same research study.
2 Materials and methods
2.1 Conceptual background
The technology acceptance model (TAM) developed by Davis (1989) deals with the issue of how users accept and use a technology (Davis, 1989). The main components of this model are perceived ease, perceived usefulness, attitude toward use, and behavioral willingness or intention (Mohr and Kühl, 2021). In this model, the actual behavior can be better predicted by the intention or behavioral willingness (Popa et al., 2019). The intention variable, which means the desire and decision of a person to perform a certain behavior (Sánchez et al., 2018), is recognized as a very good predictor of real environmental behaviors (Holt et al., 2021). In other words, it is a reflection of the motivation level, readiness, and tendency of a person to adopt a behavior. Therefore, the state of behavioral willingness is of great importance to analyze and predict people’s behaviors (Bagheri et al., 2019a; Safi Sis et al., 2020). TAM also shows that an individual’s attitude toward technology determines how to behave with respect to this (Bagheri et al., 2021). Attitude toward a behavior refers to the degree to which a person represents a favorable or unfavorable evaluation of that behavior (Sánchez et al., 2018; Ullah et al., 2021). For example, a study carried out by Abdollahzadeh et al. (2015) on the attitude and intention of citrus producers to apply biological control methods in Iran showed that although most of the producers had a positive attitude toward using these methods, a few of them actually intended to manage pest by these methods in the future. Another influencing variable in the TAM framework is perceived usefulness that refers to the degree to which a person believes that using technology will improve his/her performance (Mohr and Kühl, 2021). This variable directly affects the attitude toward using technology and desire to use it Martínez-García et al. (2013). Perceived ease, as another variable of the model, from an individual’s perspective, is the level at which a person believes that working with a particular technology is possible without much effort (Tobbin, 2012). It also means how much the user believes in a certain technology and how comfortable and easy it is to use (Kim et al., 2008). It is expected that the perceived ease of use will have an impact on the perceived usefulness and the behavioral intention of people. Some research studies, for example, studies by Bagheri et al. (2016) and Lee et al. (2011), have revealed the effect of perceived ease of use on people’s behavioral intention, either directly or indirectly through its effect on perceived usefulness.
Therefore, this research seeks to investigate the factors influencing the acceptance behavior of farmers in using biological inputs. Hence, some relationships between different factors were examined by analyzing and structuring acceptance behavior through an SEM model. The construction of an SEM model was preceded by a theoretical model based on the literature review, which is graphically presented by Figure 1. In line with the study goal, the following hypotheses were formulated based on the theoretical framework of the research (Figure 1):
1. There is a relationship between the usefulness of biological inputs and the attitude of farmers toward using them.
2. There is a relationship between the ease of using biological inputs and the attitude of farmers toward using them.
3. There is a relationship between the ease of using and the usefulness of biological inputs from the farmers’ viewpoint.
4. There is a relationship between the usefulness of biological inputs and the intention (willingness) of farmers to use.
5. There is a relationship between the attitude of farmers regarding the use of biological inputs and their intention (willingness) to use.
6. There is a relationship between the ease of using biological inputs and the intention (willingness) of farmers to use.
7. There is a relationship between the intention and behavior of farmers to use biological inputs.
8. There is a relationship between the attitude and behavior of farmers to use biological inputs.
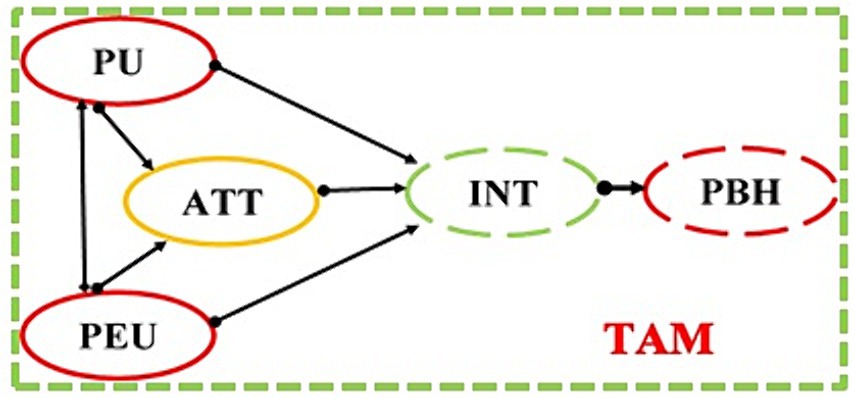
Figure 1. Conceptual framework of the study, in which PU, Perceived the Usefulness; PEU, Perceived Ease of Use; ATT, Attitude; INT, Intention; PBH, Perceived Behavior [TAM, adjusted from Davis (1989) and Venkatesh and Davis (1996)].
2.2 The area of study
The present research study was conducted among the farmers of Meidavoud District of Baghmalek County located in Khuzestan Province, southwest Iran. Baghmalek, which is located in the east of Khuzestan province, surrounded an area of 86134.58 hectares (Abiyat et al., 2021; Figure 2). The average annual rainfall of this region is 514 millimeters, according to meteorological statistics. Meidavoud district, in the southwest of this region, is known as one of the important agricultural hubs in Khuzestan province; the main crops are grown include rice, wheat, barley, and onions and summer crops are grown such as cucumber, tomato, and watermelon. Of the total 9,400 hectares of arable land in this region, more than 2,000 hectares are under rice cultivation (Agricultural Jihad Organization of Khuzestan, 2023). Recently, biological pesticides to control Chilo suppressalis in rice farms and biofertilizers have been introduced to farmers by extension agents and some private input suppliers. Despite the long history of rice cultivation in the region, there is little or no evidence of farmers changing their pest management practices, prompting the researchers to investigate the factors that make farmers reluctant to use biological inputs.
2.3 Sample selection
The statistical population of interest is composed of all the farmers living in the study area (N = 1,400). They were all involved in rice cultivation. A sample of 302 farmers was determined according to Morgan’s sample size table and was randomly selected.
2.4 Data collection
In the context of the present study, a quantitative research style was followed to design the study. For the purposes of the project, a survey study was conducted. A questionnaire was administered, as the study instrument, to collect data. It was basically designed on the basis of the TAM framework, where each component of the model was considered as a construct variable. Each of these constructs was measured with several items taken from different studies using this model. The questionnaire included 6 sections to study the demographic characteristics and the 5 research constructs including perceived ease of using biological inputs (7 statements), perceived usefulness of biological inputs (6 statements), attitude toward using biological inputs (6 statements), willingness to use biological inputs (6 statements), and the behavior of using biological inputs (5 statements). All these variables were measured by employing a five-point Likert scale (I completely disagree = 1 to I completely agree = 5). To collect data from farmers, face-to-face interviews were administered.
2.5 Data analysis
After collecting needed data, they were subjected to statistical analysis by applying SPSSv26, Smart PLS3, and AMOS software. Data were analyzed in terms of descriptive and inferential statistics. To test the study hypothesis, single and multi-group structural equation modeling was used. While various demographic characteristics were considered as a categorization based on the multi-group structural equation model, gender was not included in this type of analysis due to the relatively unequal number of male and female farmers. The reliability of the model was approved using both Cronbach’s alpha coefficients and combined reliability index (CR), in which values higher than 0.7 indicate the intensity of measurement error control in the structural equation model. To determine construct validity, the average variance extracted index (AVE) was calculated, and according to Fornell and Larcker (1981), a standard score above 0.5 was considered suitable value (Table 1). This index shows what percentage of the component’s variance has been influenced by its indicators. However, MacKenzie et al. (2005) reported a value of 0.4 or higher to be acceptable for AVE. The homogeneity reliability index (Rho) is also used to measure the internal reliability of structures by showing a value of higher than 0.7 (Mehmetoglu, 2012). Rho coefficient, which is occasionally called Dillon–Goldstein coefficient, is more reliable than Cronbach’s alpha. Moreover, Henseler et al. (2015) presented a new index called heterotrait–monotrait (HTMT) ratio to evaluate divergent validity. The HTMT index has been reported as an alternative to the Fornell–Larcker method. This criterion clearly performs better than the Fornell–Larker coefficient and cross-loadings, which are mostly unable to detect the lack of divergent validity. The threshold limit of HTMT is 0.85 to 0.9, i.e., values less than 0.9 are acceptable for divergent validity. In total, the analysis of data showed that for all the model variables, this coefficient value was lower than the mentioned threshold, so divergent validity of the model was confirmed (Table 2).
To classify the attitude and intention (willingness) of farmers toward using biological inputs, an Interval of Standard Deviation from the Mean (ISDM) was developed. Following this method, data were divided into four levels as below:
Unfavorable: Min ≤ A < Mean-SD.
Relatively unfavorable: Mean-SD ≤ B < Mean.
Relatively favorable: Mean ≤ C < Mean + SD.
Favorable: Mean + SD ≤ D < Max.
3 Results
The results of the research, as shown in Table 3, indicate that only 18.2% of the respondents were female, 81.8% were male, and 52.6% of the respondents were married. While the majority of the respondents were younger than 45 years of age, the average age of the respondents was close to 32 years. The results also showed that the highest frequency of respondents was among those with less than 10 years of farming experience (53.6%) and the lowest among those with more than 20 years of farming experience (13.3%). In terms of educational level, the data showed a range starting with illiterate respondents (7.3%), those with primary education (14.6%) and secondary and post-secondary education (045.6%) and is over with higher educated respondents (32.5%).
Below is a description of the model variables (Table 4). It should be noted that all the statements used to measure each variable have been prioritized based on their ranking mean.
As shown in Table 4, regarding the ease of using biological inputs, the statements “Consuming manure in the fields is preferable due to creating pores in the soil and making plowing easy,” “Access to biological inputs is easy,” and “Learning how to use biological inputs is easy” were prioritized with an average of 4.10, 4.06, and 3.70, respectively. In total, farmers’ viewpoint regarding the ease of using biological inputs was relatively moderate (x̅=3.61). Examination of the farmers’ perception regarding the usefulness of biological inputs remarked that the statements “Using biological methods is useful for nature and human health” by an average of 4.00, “Using biological control methods is economically beneficial” by an average of 3.90, and “Using biological methods prevents entering pesticides/herbicides to human food chain” by an average of 3.89 were prioritized over the others. The ranking mean value of perceived usefulness of biological inputs is estimated to be 3.8 indicating a moderate value. As shown in Table 4, the attitude of farmers regarding the use of biological inputs was relatively moderate (x̅=3.85). Detailed examination of the statements composed of this variable revealed that the statements “In my opinion, it is wise to use biological methods in pest control,” “I do not believe that herbicides and fertilizers to control weeds are harmless because they diminish the land’s fertility over the years “, and “I pay attention to conserve the environment for the future generations during performing farming operations” ranked first by the average of 4.20, 4.11, and 4.02, respectively. The intention of respondents regarding the use of biological inputs was relatively moderate (x̅=3.73). Among the items administered to measure this variable, the statements “I intend to help conserve the environment by using organic manure and biological fertilizers” by an average of 3.76, “I intend to use fully decomposed animal manure to increase the health of agricultural crops” by an average of 3.75, and “I intend to reduce the cost of purchasing pesticides/herbicides and chemical fertilizers by substituting organic and biological fertilizers” showing an average of 3.73 were mostly agreed by farmers. The findings also showed that the respondents have a tendency to use biological inputs, including fully decomposed animal manures and resources that are usually found in the farm, such as straw and stubble, dry fodder, leaves, and crop residues. Furthermore, they have a willingness to reduce the cost of purchasing chemical fertilizers and pesticides/herbicides by replacing them with biological inputs. Prioritized viewpoints of farmers regarding the behavior to use biological inputs revealed that the statements of “I use organic inputs (green manure, animal manure, and compost) in my farm,” “I perform manual weeding to control weeds in my farm instead of using pesticides/herbicides,” and “I use green manure to prevent soil erosion in my farm” ranked first among the others by an average of 4.01, 3.84, and 3.83, respectively. The total average of the farmers’ behavior using biological inputs showed a moderate value (x̅=3.81), which is in tune with the other model constructs.
3.1 Classifying the studied variables
To gain a better understanding of the factors influencing the acceptance of biological inputs among our respondents, a classification was developed using the ISDM method. As shown in Figure 3, more than half of the respondents fell into relatively to completely favorable groups in terms of attitude (54.6%), willingness (61.3%), and behavior (54.3%) toward the use of biological inputs.
3.2 Correlation analysis of factors influencing the acceptance behavior
According to the result of the correlation analysis, as shown in Table 5, there was a positive and significant relationship between perceived ease of use and the variables of perceived usefulness, attitude, intention, and behavior at the level of 0.01%. While the relationship between perceived ease of use and respondents’ attitude (r = 0.717), intention (r = 0.646), and behavior (r = 0.689) was very strong or strong, the relationship with the variable of perceived usefulness was moderate (r = 0.412). There was also a strong positive and significant association between perceived usefulness and the three variables of attitude (r = 0.523), intention (r = 0.583), and behavior (r = 0.559). As shown in Table 5, the three variables of attitude, intention, and behavior were highly intercorrelated. In other words, there was strong support for structural equation modeling.
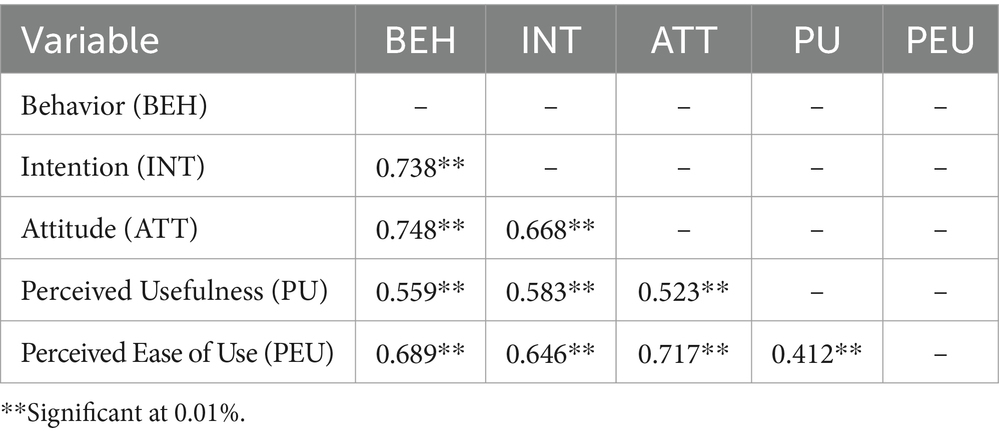
Table 5. Correlation between the factors affecting the acceptance behavior of biological inputs among the farmers (n = 302).
3.3 Factors affecting the acceptance behavior of farmers regarding biological inputs
Finally, to identify the causal relationship between factors influencing the acceptance of biological inputs among respondents using the TAM model, structural equation modeling was performed. Structural equation modeling is a method that is used to show, estimate, and test hypotheses about the causal relationship between observed and latent variables (Adrian et al., 2005; Imani et al., 2021). Once the structural equation model is used, an important part of the analysis is to observe how well is the fitness of the model hypothesis with the observed data. Researchers usually use goodness of fit indices to evaluate this feature (Table 6). Chi-square (χ
3.4 Examining research hypotheses
The SEM model represents the relationships between the latent variables through acceptable R2 values. Table 7 shows the regression weights for predicting the latent variables from the observed variables.
3.4.1 Hypothesis #1: relationship between the usefulness of biological inputs and the attitude of farmers toward using them
Based on the results of equation modeling, as shown in Table 7, the path coefficient or standardized beta of the effect of farmers’ perceived usefulness on attitude toward the use of biological inputs was estimated to be 0.40. The value of t for this parameter was calculated to be more than 1.96 (t = 8.01); accordingly, the null hypothesis was rejected at 99% confidence interval. This suggests that one of the factors that was statistically substantiated in the results and positively influenced farmers’ attitudes was farmers’ understanding of the benefits of organic inputs. According to the coefficient of this relationship, it can be claimed that the better the farmers’ understanding of the usefulness of biological inputs is, the more favorable is their attitude, which increases in the form of a linear function.
According to the results of some separate multi-group analyses, this hypothesis was confirmed among different groups of farmers, including young, non-young (middle-aged, old, and elderly), single, married, low-educated (illiterate and primary education), educated (secondary and post-secondary), university educated farmers, less experienced, and high experienced farmers in farming (Tables 8–11). While the existence of a causal relationship between the usefulness of biological inputs and attitude is independent of how farmers are categorized, the contribution of usefulness as a predictor variable is completely different in each group of farmers.
3.4.2 Hypothesis #2: relationship between the ease of using biological inputs and the attitude of farmers toward using them
As shown in Table 7, the path coefficient between ease of use of biological inputs and farmers’ attitudes toward these inputs was estimated to be 0.56, meaning that if ease of use changes by one standard deviation, farmers’ attitudes change by 0.56 standard deviations. The value of t for this parameter (t = 9.30) indicated that the null hypothesis was rejected at 99% confidence interval. Thus, farmers’ understanding of the ease of use of biological inputs can be interpreted as one of the significant factors, positively influencing farmers’ attitudes toward these inputs. This relationship still follows a linear pattern.
As presented in Tables 8–11, the results of the multi-group analyses show that similar results were observed among farmers characterized as young, non-young, single, married, low-educated, educated, university-educated, less experienced, and highly experienced farmers in farming. For each group of farmers, the impact of ease of use as a predictor variable is quite different.
3.4.3 Hypothesis #3: relationship between the ease of use and the usefulness of biological inputs from the farmers’ perspective
Looking at the values obtained from the model (Table 7), it can be observed that the path coefficient between the ease of use of biological inputs and the usefulness of biological inputs from the farmers’ point of view was estimated to be 0.44. The value of t for this parameter was calculated to be greater than 1.96 (t = 6.83), which confirms the rejection of the null hypothesis. Considering the size and significant level of this coefficient, it can be stated that ease of use is statistically one of the effective factors that positively influence the farmers’ perception of the usefulness of biological inputs.
Further examination of the data using multi-group analyses showed that this hypothesis was confirmed when farmers were grouped according to different characteristics. Farmers that grouped into each of the categories of young, married, poorly educated, educated, university educated, less experienced, and highly experienced in farming showed similar results (Tables 8–11). For farmers characterized as not young or those who are single, the null hypothesis was accepted, meaning that there is no causal relationship between the ease of use and the usefulness of biological inputs from the perspective of farmers belonging to each of these groups (Tables 8, 10).
3.4.4 Hypothesis #4: relationship between the usefulness of biological inputs and the intention (willingness) of farmers to use
As shown in Table 7, the estimated coefficients indicate that the causal path between the usefulness of biological inputs and farmers’ intention (willingness) to adopt biological inputs, which is depicted in the model, is defined with a path coefficient of 0.32. Since the value of t is greater than 1.96 (t = 5.41), indicating a statistically significant result, the null hypothesis was rejected. This means that the perceived usefulness of organic inputs was recognized as an effective factor in farmers’ willingness to adopt organic inputs. The positive relationship means that as farmers’ perceptions of the usefulness of biological inputs improve, their willingness to use biological inputs increases. This relationship also follows a linear pattern.
Examination of the SEM model by grouping farmers showed that this hypothesis was also confirmed for categorized farmers. Farmers belonging to the different categories of young, non-young, single, married, educated, university educated, less experienced, and highly experienced in farming showed similar results (Tables 8–11). For the category of poorly educated farmers, the null hypothesis was not rejected, meaning that among this group of farmers, the perceived usefulness of biological inputs could not be estimated as a predictor of the intention (willingness) to use (Table 9).
3.4.5 Hypothesis #5: relationship between the attitude of farmers regarding the use of biological inputs and their intention (willingness) to use
The estimated SEM model also confirmed the positive relationship between farmers’ attitudes and their intention (willingness) to adopt organic inputs. The path coefficient of this relationship was 0.37, indicating that if farmers’ attitude changes by one standard deviation, their willingness to accept changes by 0.37 standard deviations. Examination of the result of the t-test also showed statistical significance (t = 4.44, which is greater than the 1.96 level), which means that the null hypothesis must be rejected. In other words, the latent variable of farmers’ attitude toward the use of biological inputs directly influenced their willingness to accept (Table 7).
The multi-group analyses showed that there is a causal relationship between the attitudes and intentions of farmers belonging to different groups regarding the use of biological inputs on their farms. While different groups of farmers including young, non-young, single, married, poorly educated, educated, university educated, less experienced, and highly experienced in farming showed similar results, the contribution of attitude as a predictor variable varied (Tables 8–11).
3.4.6 Hypothesis #6: relationship between the ease of using biological inputs and the intention (willingness) of farmers to use
As shown in Table 7, there is a dependent interaction between the ease of use of biological inputs and the farmers’ willingness to accept them, with the smallest effect of 0.23. The value of t for this parameter was calculated to be greater than 1.96 (t = 3.43), which led to the rejection of the null hypothesis. Thus, farmers’ perceived ease of use had a positive impact on their willingness to adopt biological inputs. Following a linear function, as farmers’ understanding of the ease of use of biological inputs improves, their willingness to accept and use them increases.
Structural modeling of the data using multi-group analysis revealed that there is a positive interaction between the ease of use of biological inputs and the willingness to adopt them among farmers characterized by different demographic features. Farmers belonging to each of the categories including non-young, single, married, poorly educated, educated, university educated, and highly experienced in farming showed similar results (Tables 8–11). For farmers categorized as either young, married, or less experienced in farming, the null hypothesis was accepted indicating that for each of these groups of farmers, no causal relationship could be found between the ease of using biological inputs and farmers’ intention (willingness) to use them (Tables 8, 10, 11).
3.4.7 Hypothesis #7: relationship between the intention and behavior of farmers to use biological inputs
Based on the estimated research model, the path coefficient of the effect of intention to use biological inputs on the farmers’ use behavior was estimated to be 0.48. Considering the significance of the t-value (t = 9.98 greater than 1.96) for this parameter, it can be concluded that the null hypothesis is rejected. Therefore, the intention to use is found to be an effective factor, which positively influences the acceptance of the behavior by the farmers. Knowing that the pattern of a linear function still prevails, it can be stated that as the farmers’ intention (willingness) to use biological inputs improves, the use of such products becomes more acceptable (Table 7).
Considering different groups of farmers, the estimation of the model showed that there is a causal relationship between the intention to use biological inputs and the behavior of using them. Farmers who grouped into different categories such as young, non-young, single, married, poorly educated, educated, university educated, less experienced, and highly experienced in farming separately showed similar results (Tables 8–11). The contribution made by farmers’ intentions in predicting behavior is quite different for each group.
3.4.8 Hypothesis #8: relationship between the attitude and behavior of farmers to use biological inputs
Finally, examining the relationship between farmers’ attitudes and their behavior in accepting organic inputs revealed a beta coefficient of 0.47, which indicates the magnitude of change in the standard deviation of behavior due to one standard deviation change in attitude. As expected, the value of t for this parameter was also significant at the 99% confidence level (t = 7.67 greater than 1.96), leading to the rejection of the null hypothesis. This reflects that farmers’ attitudes toward the use of organic inputs contribute as an important predictor of adoption behavior, and this association is also positive.
The results of the multi-group analysis also showed that similar results were observed among farmers separately characterized as young, not young, single, married, low educated, educated, university educated, less experienced, and highly experienced in farming (Tables 8–11). The contribution of farmers’ attitude as a behavioral predictor varies considerably between groups.
According to the findings of the present study, farmers’ intention (willingness) and attitude toward the use of biological inputs were found to be the most important variables affecting farmers’ acceptance behavior. They have the ability to explain the variations of the behavior variable up to 80%. Similarly, according to the findings of Table 7, the most important variable affecting the behavioral intention (willingness) of farmers to use biological inputs was the attitude variable, followed by perceived usefulness, and perceived ease of use. These variables were able to explain intention’s variations up to 62%. Among the variables of perceived usefulness and perceived ease of use, the latter was stronger in determining the farmers’ attitude regarding the use of biological inputs. These two variables are also capable to predict and explain 67% of the variance in the attitude. Similarly, the findings showed that only 19% of the variation in variable of perceived ease of use could be justified directly by perceived usefulness of biological inputs by farmers. An adjusted structure of path analysis is shown in Figure 4.
4 Discussion
Today, more than 300 dangerous chemical inputs, including various fertilizers with the purpose of soil fertility and different types of toxic compounds, are used in farms to control pests, diseases, and weeds (Mansourian et al., 2023). While near less than 1% of the consumed volume of pesticides reaches pests, 99% is released into the environment, causing soil and water pollution (Shah Pasand et al., 2021). The adverse impacts of fertilizers and pesticides on environment, food security, and human health have led to more attention to apply alternative methods, emphasizing safe and chemical-free products (Imani et al., 2021). The per capita consumption of toxic compounds as the ingredients of fertilizers is reported to be 400 grams per person in Iran; moreover, the cancer disease incidence statistics, as an index of unhealthy farming style, are higher in areas, where farming practices depend on a higher level of using chemical pesticides (Khosravi and Tohidfar, 2015; Bondori et al., 2020). Usage of fertilizers and pesticides is a topic, which has a longer tradition of research, while topics such as usage of biological control methods are areas of more recent interest. It is clear that farmers are at the forefront of agricultural practices, and the choice of farming style depends on their decision, which is, in turn, a function of different factors. Therefore, the present survey research sought to determine if factors affect farmers’ behavior and their willingness to accept consuming biological inputs; and if so, what mechanisms explain the relationship between them. Therefore, the research model was developed based on the technology acceptance model (TAM). The results presented that the average of attitude, intention, and behavior of farmers regarding the use of biological inputs was relatively moderate, which is in tune with the results of the study by Bagheri et al. (2021). Referring to the correlation findings, a positive and significant relationship between the perceived ease of use and the perceived usefulness of biological inputs was obtained. The higher the perceived ease of use, the more likely the farmers perceive the biological inputs that are beneficial and profitable. This result is in line with the findings by Salarvand (2014), Shahani (2020), and Bagheri et al. (2016, 2021). A positive and significant association was found between the variables of perceived ease of use, the perceived usefulness of biological inputs, and the attitude to use. These results are in agreement with the findings by Salarvand (2014) and Flett et al. (2004). Between the factors of perceived usefulness, perceived ease of use, attitude, and intention (willingness) to use, positive and significant correlations were found which appear to be in line with the studies by, Salarvand (2014), García-García et al. (2016), Shahani (2020) and Bagheri et al. (2016, 2021). The model also highlighted a direct and significant relationship between perceived usefulness, attitude, intention (willingness), and the behavior of accepting biological inputs, confirming the studies by Salehi et al. (2010), Salarvand (2014), and Bagheri et al. (2016, 2021). Moreover, the results that were obtained approved all the hypotheses aforementioned in previous sections. The findings indicated that behavioral intention (willingness) and attitude were recognized as the most important variables affecting farmers’ behavior in accepting biological inputs. Perceived usefulness and perceived ease of use had a direct, positive, and significant effect on the attitude regarding the use of biological inputs. These two factors could predict the attitude of farmers toward using biological inputs. This is consistent with the study by Salarvand (2014), Bagheri et al. (2016, 2021), and Shahani (2020). In addition, the SEM model also suggests that intention and attitude showed a direct, positive, and significant influence on the farmers’ behavior in accepting biological inputs. Totally, these two variables could predict 80% of the behavior variations. When farmers are inclined to use technology and hold a desirable attitude toward that, they are more likely to accept and use it. Referring to the results of the structural equations, it was observed that the perceived usefulness, perceived ease of use, and attitude had a direct, positive, and significant effect on the tendency of farmers to use biological inputs. Therefore, as improvement occurs in the understanding of farmers regarding the usefulness and ease of using biological inputs or in their attitude over time, there will be desirable changes in the extent and acceptance of these inputs in their farming. Similar results were observed in the studies by Salarvand (2014), Momvandi et al. (2018), Valizadeh et al. (2020), and Bagheri et al. (2021), in which the factors influencing the adoption of biological inputs have explained by the TAM model.
5 Conclusion
The recent drought in Iran has led the government to ban rice cultivation in many areas and impose many restrictions. Under these circumstances, the strategy of farmers to maintain a certain level of income has led to a higher yield per hectare, which is associated with the intensive use of pesticides and chemical inputs to ensure profitability and, usually, in exchange for the loss of environmental safety.
In general, this study not only described the behavior, intention, attitude, and perception of farmers regarding the ease and usefulness of biological inputs but also examined a model which has introduced important factors affecting the acceptance of biological inputs. In addition, in the presence of different categorization variables, the causal relationships between different constructs of the model were examined. In conclusion, the TAM model has the power to predict farmers’ behavior and willingness to accept biological inputs so that attitude and willingness variables could predict behavior, and in turn, willingness could be predicted by attitude, perceived usefulness, and ease of use variables. While the existence of a causal relationship between variables is independent of how farmers are categorized, the contribution of model variables as the predictors of each path is quite different for each group of farmers.
As far as the adoption of new technologies is concerned, the suggestion to be drawn from our analysis is that the most effective means of encouraging farmers to accept the use of safe and healthy inputs is to plan and implement education and extension programs that will lead to a more desirable attitude and willingness to use such products among farmers rather than their continued reluctance. Other actions include training in biological control for agricultural advisors and input suppliers. In addition, in the context of a transition to biological inputs (including biocontrol agents and biofertilizers), it is crucial to promote the coexistence of chemical and biological inputs and work toward a gradual substitution. Meanwhile, significant attempts should be made by agricultural service centers to change farmers’ attitudes, as farmers are reluctant to use biological inputs. Other, more desirable, actions can encourage the adoption and use of biological inputs through subsidies, insurance, and information campaigns that network farmers’ groups. Government initiatives to develop monitoring and control tools to manage the public health aspects of rice produced by farmers are of great importance to enforce their acceptance. At the same time, promoting the benefits of using biological inputs for crop health can make farmers more receptive to using biological inputs on their farms. Strictness should be applied to the health of agricultural products, and at the same time, emphasis should be placed on the benefits of using biological inputs for the health of products in order to influence farmers’ attitudes. In addition to these proposals, which are essentially instrumental, it is important to emphasize interactive interventions, which focus on involving farmers in extension programs to change their farming system in favor of producing healthy crops and protecting the environment. An example of this type of activity can be promoted in demonstration farms, where the usefulness and ease of use of biological inputs are made visible.
6 Limitations and future studies
This study had some limitations that should be addressed in future research. The first limitation was related to the lack of generalizability of the results. The study was conducted among a sample of farmers engaged in rice production in one district; therefore, the representation of farmers’ behavior in using biological inputs is limited. Given the differences among farmers living in different regions as well as a large research area of the country, a comprehensive study should be developed to investigate farmers’ behavior and willingness to use biological inputs in agricultural practices. Second, due to the lack of long-term perspective in using cross-sectional data, which do not reflect the real and dynamic nature of farmers’ behavior, future research should maintain a longitudinal perspective in the study. Third, the categorization of farmers was not been considered as a study objective in this study, so it is highly recommended that future studies focus on the study problems, dealing with structural equation and modeling in the presence of at least one categorizing factor to concentrate on a specific group of farmers.
Data availability statement
The data analyzed in this study is subjected to the following licenses/restrictions: the study respondents were assured about the confidentiality of collected data. Requests to access these datasets should be directed to MF, bS5mb3JvdXphbmlAYXNucnVraC5hYy5pcg==.
Ethics statement
Ethical approval was not required for the study involving humans in accordance with the local legislation and institutional requirements. Written informed consent to participate in this study was not required from the participants or the participants’ legal guardians/next of kin in accordance with the national legislation and the institutional requirements.
Author contributions
MF: Conceptualization, Formal analysis, Methodology, Project administration, Supervision, Writing – original draft. AB: Data curation, Formal analysis, Investigation, Software, Writing – original draft. AM: Conceptualization, Data curation, Writing – review & editing.
Funding
The author(s) declare that no financial support was received for the research, authorship, and/or publication of this article. This research was conducted as a research project (No. 1402/11) which is financially supported by the Agricultural Sciences and Natural Resources University of Khuzestan.
Acknowledgments
The authors wish to gratefully thank all the farmers who kindly agreed to participate in this study and also would like to thank the editor and referee whose precious comments greatly improved the quality and credibility of the study.
Conflict of interest
AM was employed by the Dezab Consulting Engineering Company.
The remaining authors declare that the research was conducted in the absence of any commercial or financial relationships that could be construed as a potential conflict of interest.
Publisher’s note
All claims expressed in this article are solely those of the authors and do not necessarily represent those of their affiliated organizations, or those of the publisher, the editors and the reviewers. Any product that may be evaluated in this article, or claim that may be made by its manufacturer, is not guaranteed or endorsed by the publisher.
References
Abdollahzadeh, G., Sharifzadeh, M. S., and Damalas, C. A. (2015). Perceptions of the beneficial and harmful effects of pesticides among Iranian rice farmers influence the adoption of biological control. Crop Prot. 75, 124–131. doi: 10.1016/j.cropro.2015.05.018
Abiyat, M., Abiyat, M., and Abiyat, M. (2021). Investigation of land-use changes and their impacts on soil erosion in Baghmalek basin using artificial neural network and RUSLE model. J. Environ. Stud. 47, 89–110. doi: 10.22059/JES.2021.324250.1008180
Abzari, M., Shaemi Barzaki, A., and Abbasi, R. (2009). “Investigation the knowledge sharing behavior among employees of agricultural Bank branches of Shiraz County using the planned behavior model” in ed. Shiraz The first National Management Conference (Shiraz: Management Scientific Association of Shiraz University), 1–18.
Adrian, A. M., Norwood, S. H., and Mask, P. L. (2005). Producers’ perceptions and attitudes toward precision agriculture technologies. Comput. Electron. Agric. 48, 256–271. doi: 10.1016/j.compag.2005.04.004
Agricultural Jihad organization of Khuzestan (2023). Agriculture Yearbook. Ministry of Agriculture-Jahad, Tehran, Iran.
Ahmadi Firouzjaie, A., Ahmadi, L., and Khalaj, H. (2023). The role of technical knowledge and technology accessibility in explaining grain farmers’ soil conservation behavior: the use of technology acceptance model. Environ. Educ. Sustain. Develop. 11, 41–59. doi: 10.30473/ee.2023.64105.2526
Ajzen, I. (1991). The theory of planned behavior. Organizational behavior and human decision processes, 50, 179–211. doi: 10.1016/0749-5978(91)90020-T
Asante, I. K., Ocran, J. K., and Inkoom, E. W. (2023). Modeling pesticide use behavior among farmers in the upper east region of Ghana: an empirical application of the theory of planned behavior. Environ. Protection Res. 3, 130–149. doi: 10.37256/epr.3120232074
Ashoori, D., Noorhosseini, A., Allahyari, M., and Bagheri, A. (2015). Investigating the factors affecting adoption of biological control of Rice stem borer (using Trichogramma wasps) using logit models; Paddy farmer of EastGuilan Province. Agricul. Extension Educ. Res. 8, 63–78.
Bagheri, A., Allahyari, M. S., and Ashouri, D. (2016). Interpretation on biological control adoption of the rice stem borer, Chilo Suppressalis (Walker) in north part of Iran: application for technology acceptance model (TAM). Egypt. J. Biol. Pest Control 26, 27–33.
Bagheri, A., Bondori, A., Allahyari, M. S., and Damalas, C. A. (2019b). Modeling farmers’ intention to use pesticides: an expanded version of the theory of planned behavior. J. Environ. Manag. 248:109291. doi: 10.1016/j.jenvman.2019.109291
Bagheri, A., Bondori, A., Allahyari, M. S., and Surujlal, J. (2021). Use of biologic inputs among cereal farmers: application of technology acceptance model. Environ. Dev. Sustain. 23, 5165–5181. doi: 10.1007/s10668-020-00808-9
Bagheri, A., Bondori, A., and Damalas, C. A. (2019a). Modeling cereal farmers’ intended and actual adoption of integrated crop management (ICM) practices. J. Rural. Stud. 70, 58–65. doi: 10.1016/j.jrurstud.2019.05.009
Bagheri, A., Shirzadi, Z., Sookhtanlou, M., Shokohian, A., Bondori, A., and Damalas, C. (2022). Occupational exposure to pesticides and safety behavior among Iranian greenhouse farmers, 1–16. doi: 10.21203/rs.3.rs-1561171/v1
Baumgartner, H., and Homburg, C. (1996). Applications of structural equation modeling in marketing and consumer research: a review. Int. J. Res. Mark. 13, 139–161. doi: 10.1016/0167-8116(95)00038-0
Bawa, U. (2023). Heavy metals concentration in food crops irrigated with pesticides and their associated human health risks in Paki, Kaduna state. Nigeria. Cogent Food Agricul. 9:2191889. doi: 10.1080/23311932.2023.2191889
Bishnoi, U. (2018). Agriculture and the dark side of chemical fertilizers. Environ. Analysis Ecol. Stud. 3:552. doi: 10.31031/EAES.2018.03.000552
Bondori, A., Bagheri, A., and Damalas, C. A. (2023). Protective behavior in chemical spraying among farmers of northern Iran. Environ. Dev. Sustain., 1–13. doi: 10.1007/s10668-023-03355-1
Bondori, A., Bagheri, A., and Sookhtanlou, M. (2019). Analysis of Moghan plain farmers’ health-safety behavior towards using chemical pesticides. Iranian Agricul. Extension Educ. J. 14, 161–183. doi: 20.1001.1.20081758.1397.14.2.10.7
Bondori, A., Bagheri, A., Sookhtanlou, M., and Damalas, C. A. (2021). Modeling farmers’ intention for safe pesticide use: the role of risk perception and use of information sources. Environ. Sci. Pollut. Res. 28, 66677–66686. doi: 10.1007/s11356-021-15266-7
Bondori, A., Bagheri, A., Sookhtanlou, M., Jamshidi, O., and Norozi, A. (2020). Assessing farmers' knowledge of Moghan plain about the consequences of chemical pesticides for community health, the environment, and food security. Iranian J. Health Environ. 12, 621–638.
Carvalho, F. P. (2017). Pesticides, environment, and food safety. Food Energy Security 6, 48–60. doi: 10.1002/fes3.108
Chen, R., Huang, J., and Qiao, F. (2013). Farmers' knowledge on pest management and pesticide use in Bt cotton production in China. China Econ. Rev. 27, 15–24. doi: 10.1016/j.chieco.2013.07.004
Chen, X., Zhang, X. E., and Chen, J. (2024). TAM-based study of farmers’ live streaming e-commerce adoption intentions. Agriculture 14:518. doi: 10.3390/agriculture14040518
Damalas, C. A., and Eleftherohorinos, I. G. (2011). Pesticide exposure, safety issues, and risk assessment indicators. Int. J. Environ. Res. Public Health 8, 1402–1419. doi: 10.3390/ijerph8051402
Davis, F. D. (1989). Perceived usefulness, perceived ease of use, and user acceptance of information technology. MIS Q. 13, 319–340. doi: 10.2307/249008
Dias, A. C., Silva, L. S., Cardoso, S. A., and Pinheiro, T. M. M. (2023). Knowledge and risk perception of rural workers exposed to pesticides in Teixeiras/MG: A cross-sectional study. Rev Med Minas Gerais. 33:e-33105. doi: 10.5935/2238-3182.2022e33105
Dukare, A. S., Paul, S., Nambi, V. E., Gupta, R. K., Singh, R., Sharma, K., et al. (2019). Exploitation of microbial antagonists for the control of postharvest diseases of fruits: a review. Crit. Rev. Food Sci. Nutr. 59, 1498–1513. doi: 10.1080/10408398.2017.1417235
Fan, L., Niu, H., Yang, X., Qin, W., Bento, C. P., Ritsema, C. J., et al. (2015). Factors affecting farmers' behaviour in pesticide use: insights from a field study in northern China. Sci. Total Environ. 537, 360–368. doi: 10.1016/j.scitotenv.2015.07.150
Fianko, J. R., Donkor, A., Lowor, S. T., and Yeboah, P. O. (2011). Agrochemicals and the Ghanaian environment, a review. J. Environ. Prot. 2, 221–230. doi: 10.4236/jep.2011.23026
Flett, R., Alpass, F., Humphries, S., Massey, C., Morriss, S., and Long, N. (2004). The technology acceptance model and use of technology in New Zealand dairy farming. Agric. Syst. 80, 199–211. doi: 10.1016/j.agsy.2003.08.002
Fornell, C., and Larcker, D. (1981). Evaluating structural equation models with unobservable variables and measurement error. J. Mark. Res. 3, 75–98.
Gangadhar, B., Kumaresan, P., Somaprakash, D. S., and Qadri, S. M. H. (2012). Adoption of biocontrol methods for the control of mealy bug and uzifly in sericulture. J. Biopest. 5:199.
García-García, C. R., Parrón, T., Requena, M., Alarcón, R., Tsatsakis, A. M., and Hernández, A. F. (2016). Occupational pesticide exposure and adverse health effects at the clinical, hematological and biochemical level. Life Sci. 145, 274–283. doi: 10.1016/j.lfs.2015.10.013
Gholifar, E., Mirshekari, A., Lavaei Adaryani, R., and Gholami, H. (2019). The impacts of perceived innovation characteristics on intention to use bio-fertilizers among farmers: evidence from Yengijeh Village, Zanjan County. Agricul. Extension Educ. Res. 12, 54–64.
Goulet, F. (2021). Biological inputs and agricultural policies in South America: between disruptive innovation and continuity. Perspective 55, 1–4. doi: 10.19182/perspective/36383
Govindasamy, R., Italia, J., and Liptak, C. (1997). Quality of agricultural produce: consumer preferences and perceptions. US: New Jersey agricultural Experiment Station, Rutgers university.
Habibzadeh Shojaei, S., and Sharifzade, M. S. (2014). Socio-economic factors influencing farmers' attitudes towards integrated pest management in Mashhad. Iranian J. Agricul. Econ. Develop. Res. 45, 739–746. doi: 10.22059/IJAEDR.2014.53847
Hannus, V., and Sauer, J. (2021). Understanding farmers’ intention to use a sustainability standard: the role of economic rewards, knowledge, and ease of use. Sustain. For. 13:10788. doi: 10.3390/su131910788
Hawkins, N. J., Bass, C., Dixon, A., and Neve, P. (2019). The evolutionary origins of pesticide resistance. Biol. Rev. 94, 135–155. doi: 10.1111/brv.12440
Hendrawan, S. A., Trihandoyo, A., and Saroso, D. S. (2023). Implementing technology acceptance model to measure ICT usage by smallholder farmers. SINERGI 27, 123–132. doi: 10.22441/sinergi.2023.1.014
Henseler, J., Ringle, C. M., and Sarstedt, M. (2015). A new criterion for assessing discriminant validity in variance-based structural equation modeling. J. Acad. Mark. Sci. 43, 115–135. doi: 10.1007/s11747-014-0403-8
Holt, J. R., Butler, B. J., Borsuk, M. E., Markowski-Lindsay, M., MacLean, M. G., and Thompson, J. R. (2021). Using the theory of planned behavior to understand family Forest owners’ intended responses to invasive Forest insects. Soc. Nat. Resour. 34, 1001–1018. doi: 10.1080/08941920.2021.1924330
Imani, B., Allahyari, M. S., Bondori, A., Surujlal, J., and Sawicka, B. (2021). Determinants of organic food purchases intention: the application of an extended theory of planned behavior, future of food. J. Food Agricul. Society, 9, 1–12. doi: 10.17170/kobra-202011192216
Imani, B., Bondori, A., and Jamshidi, O. (2023). Determinants of safety and health behaviors of wheat farmers towards the use of chemical pesticides in Qorveh County. Int. J. Agricul. Manag. Develop. 13, 227–240. doi: 10.22004/ag.econ.342871
Jallow, M. F., Awadh, D. G., Albaho, M. S., Devi, V. Y., and Thomas, B. M. (2017). Pesticide knowledge and safety practices among farm workers in Kuwait: results of a survey. Int. J. Environ. Res. Public Health 14:340. doi: 10.3390/ijerph14040340
Jokar, N. K., Noorhosseini, S. A., Allahyari, M. S., and Damalas, C. A. (2017). Consumers' acceptance of medicinal herbs: an application of the technology acceptance model (TAM). J. Ethnopharmacol. 207, 203–210. doi: 10.1016/j.jep.2017.06.017
Jöreskog, K. G., and Sörbom, D. (1996). LISREL 8: User's reference guide. Mooresville: Scientific Software.
Khosravi, S., and Tohidfar, M. (2015). Reduction of applied pesticides and cancer with the cultivation of transgenic crops. Genet Eng Biosaf J 4, 1–10.
Kim, D. J., Ferrin, D. L., and Rao, H. R. (2008). A trust-based consumer decision-making model in electronic commerce: the role of trust, perceived risk, and their antecedents. Decis. Support. Syst. 44, 544–564. doi: 10.1016/j.dss.2007.07.001
Lahlali, R., Ezrari, S., Radouane, N., Kenfaoui, J., Esmaeel, Q., El Hamss, H., et al. (2022). Biological control of plant pathogens: a global perspective. Microorganisms 10:596. doi: 10.3390/microorganisms10030596
Lee, Y. H., Hsieh, Y. C., and Hsu, C. N. (2011). Adding innovation diffusion theory to the technology acceptance model: supporting employees' intentions to use e-learning systems. J. Educ. Technol. Soc. 14, 124–137.
MacCallum, R. C., Browne, M. W., and Sugawara, H. M. (1996). Power analysis and determination of sample size for covariance structure modeling. Psychol. Methods 1, 130–149. doi: 10.1037/1082-989X.1.2.130
MacKenzie, S. B., Podsakoff, P. M., and Jarvis, C. B. (2005). The problem of measurement model misspecification in behavioral and organizational research and some recommended solutions. J. Appl. Psychol. 90, 710–730. doi: 10.1037/0021-9010.90.4.710
Mansourian, A., Jalali, M., and Eskandari, F. (2023). Investigating factors affecting the behavior of chemical fertilizer consumption: a study of corn growers in Daroodfaraman village, Kermanshah city. Agricul. Extension Educ. Res. 15, 25–47. doi: 10.30495/jaeer.2023.70100.10948
Martínez-García, C. G., Dorward, P., and Rehman, T. (2013). Factors influencing adoption of improved grassland management by small-scale dairy farmers in Central Mexico and the implications for future research on smallholder adoption in developing countries. Livest. Sci. 152, 228–238. doi: 10.1016/j.livsci.2012.10.007
McCormack, M., Buckley, C., and Kelly, E. (2022). Using a technology acceptance model to investigate what factors influence farmer adoption of a nutrient management plan. Irish J. Agricul. Food Res. 60, 142–151. doi: 10.15212/ijafr-2020-0134
Mehmetoglu, M. (2012). Partial least squares approach to structural equation modeling for tourism research. Advan. Hospital. Leisure 8, 43–61. doi: 10.1108/S1745-3542(2012)0000008007
Mohr, S., and Kühl, R. (2021). Acceptance of artificial intelligence in German agriculture: an application of the technology acceptance model and the theory of planned behavior. Precis. Agric. 22, 1816–1844. doi: 10.1007/s11119-021-09814-x
Momvandi, A., Omidi Najafabadi, M., Hosseini, J. F., and Lashgarara, F. (2018). The identification of factors affecting the use of pressurized irrigation systems by farmers in Iran. WaterSA 10:1532. doi: 10.3390/w10111532
Nadi, M. A., Mushfaqi, N., and Siadat, S. A. (2014). A structural equation modeling study of the relationship between the dimensions of organizational justice, trust, continuous and affective commitment with Teacher’s perceived self-efficacy. J. New Approach. Educ. Admin. 4, 19–44. doi: 20.1001.1.20086369.1392.4.16.2.2
Naspetti, S., Mandolesi, S., Buysse, J., Latvala, T., Nicholas, P., Padel, S., et al. (2017). Determinants of the acceptance of sustainable production strategies among dairy farmers: development and testing of a modified technology acceptance model. Sustain. For. 9:1805. doi: 10.3390/su9101805
Niyaki, A., Radjabi, R., and Allahyari, M. S. (2010). Social factors critical for adoption of biological control agents Trichogramma spp. egg parasitoid of rice stem borer Chilo suppressalis in north of Iran. J. Agricul. Environ. Sci. 9, 133–139.
Önder, M., Ceyhan, E., and Kahraman, A. (2011). “Effects of agricultural practices on environment” in International conference on biology, environment and chemistry IPCBEE, vol. 24 (Singapore: IACSIT Press), 28–32.
Ping, J. R. A. (2004). On assuring valid measures for theoretical models using survey data. J. Bus. Res. 57, 125–141. doi: 10.1016/S0148-2963(01)00297-1
Popa, B., Niță, M. D., and Hălălișan, A. F. (2019). Intentions to engage in forest law enforcement in Romania: an application of the theory of planned behavior. Forest Policy Econ. 100, 33–43. doi: 10.1016/j.forpol.2018.11.005
Purvis, B., Mao, Y., and Robinson, D. (2019). Three pillars of sustainability: in search of conceptual origins. Sustain. Sci. 14, 681–695. doi: 10.1007/s11625-018-0627-5
Recena, M. C. P., Caldas, E. D., Pires, D. X., and Pontes, E. R. J. (2006). Pesticides exposure in Culturama, Brazil—knowledge, attitudes, and practices. Environ. Res. 102, 230–236. doi: 10.1016/j.envres.2006.01.007
Rodrigues, L. C. C., Fortini, R. M., and Neves, M. C. R. (2022). Impacts of the use of biological pest control on the technical efficiency of the Brazilian agricultural sector. Int. J. Environ. Sci. Technol. 20, 1–16. doi: 10.1007/s13762-022-04032-y
Sabzian, M. K., Ajili, A., Mohammadzade, S., Yazdanpanah, M., and Forouzani, M. (2015). Examine the attitudes and behaviors of farmers to apply integrated Pest management by using the developed theory of planned behavior. Agricul. Extension Educ. Res. 8, 57–70.
Safa, L., Rezaei, R., Salahi Moghadam, N., and Karbasioun, M. (2019). Factors affecting farmers’ intention to use Habrobracon Hebetor to control tomato budworm (Heliothis spp.) in Tarom County. Iranian Agricul. Extension Educ. J. 15, 205–222. doi: 10.22034/IAEEJ.2019.96423
Safi Sis, Y., Joodi Damirchi, M., and Maleki, M. (2020). Analysis of factors affecting the behavioral intentions of organic crops technology from the viewpoint of agricultural experts of East Azarbaijan Province. Agricul. Extension Educ. Res. 13, 1–14.
Salarvand, Z. (2014). Identification of factors effecting adoption of biologic inputs among wheat farmers in Dorood County. M.Sc. thesis, Ramin University of agriculture and natural Resources (Khuzestan), p. 184.
Salehi, S., Rezaei-Moghaddam, K., and Fathi, G. H. (2010). Analysisi of structural model of factors affecting agricultural specialists’ intention to use Variable Rate Technologies in Seeding J. Agricul. Knowledge 20, 144–155.
Sánchez, M., López-Mosquera, N., Lera-López, F., and Faulin, J. (2018). An extended planned behavior model to explain the willingness to pay to reduce noise pollution in road transportation. J. Clean. Prod. 177, 144–154. doi: 10.1016/j.jclepro.2017.12.210
Schwartz, S. H. (1977). Normative influences on altruism. In Advances in experimental social psychology, 10, 221–279. doi: 10.1016/S0065-2601(08)60358-5
Sehrawat, A., Sindhu, S. S., and Glick, B. R. (2022). Hydrogen cyanide production by soil bacteria: biological control of pests and promotion of plant growth in sustainable agriculture. Pedosphere 32, 15–38. doi: 10.1016/S1002-0160(21)60058-9
Shafiee, F., Rezvanfar, A., and Sadat Mirtorabi, M. (2018). Investigation of farmers’ environmental behavior components about bio-fertilizers application (the case of Alborz Province). J. Environ. Sci. Technol. 20, 105–118. doi: 10.22034/jest.2018.12483
Shah Pasand, M., Bandari, A., Emami, N., Norozi, A., and Ghasemi, J. (2021). Explanation of effective factors on life environmental behavior of gardeners in Meshkinshahr county. Iranian J. Agricul. Econ. Develop. Res. 52, 663–678. doi: 10.22059/IJAEDR.2021.293647.668853
Shahani, A. (2020). Investigating the effective factors on the acceptance of using biological control by Citrus growers against Mediterranean Fly Pest in Sari City. Agricul. Extension Educ. Res. 13, 47–60.
Sharifzadeh, M. S., Damalas, C. A., Abdollahzadeh, G., and Ahmadi-Gorgi, H. (2017). Predicting adoption of biological control among Iranian rice farmers: an application of the extended technology acceptance model (TAM2). Crop Prot. 96, 88–96. doi: 10.1016/j.cropro.2017.01.014
Silva, A. G., Canavari, M., and Sidali, K. L. (2017). A technology acceptance model of common bean growers’ intention to adopt integrated production in the Brazilian central region. Die Bodenkultur J. Land Manag. Food Environ. 68, 131–143. doi: 10.1515/boku-2017-0012
Stern, P. C. (2000). Toward a coherent theory of environmentally significant behavior. J. Soc. Issues, 56, 407–424. doi: 10.1111/0022-4537.00175
Terfe, A., Mekonen, S., and Jemal, T. (2023). Pesticide residues and effect of household processing in commonly consumed vegetables in Jimma zone, Southwest Ethiopia. J. Environ. Public Health 2023, 1–12. doi: 10.1155/2023/7503426
Tobbin, P. (2012). Towards a model of adoption in mobile banking by the unbanked: a qualitative study. Info 14, 74–88. doi: 10.1108/14636691211256313
Ullah, S., Abid, A., Aslam, W., Noor, R. S., Waqas, M. M., and Gang, T. (2021). Predicting behavioral intention of rural inhabitants toward economic incentive for deforestation in Gilgit-Baltistan. Pakistan. Sustainability 13:617. doi: 10.3390/su13020617
Valizadeh, N., Rezaei-Moghaddam, K., and Hayati, D. (2020). Analyzing Iranian farmers' behavioral intention towards acceptance of drip irrigation using extended technology acceptance model. J. Agric. Sci. Technol. 22, 1177–1190. doi: 20.1001.1.16807073.2020.22.5.15.9
Venkatesh, V., and Davis, F. D. (1996). A model of the antecedents of perceived ease of use: Development and test. Decision sciences, 2, 451–481. doi: 10.1111/j.1540-5915.1996.tb00860.x
Wang, Z., Sui, Y., Li, J., Tian, X., and Wang, Q. (2022). Biological control of postharvest fungal decays in citrus: a review. Crit. Rev. Food Sci. Nutr. 62, 861–870. doi: 10.1080/10408398.2020.1829542
Wheeler, S. A. (2008). What influences agricultural professionals' views towards organic agriculture? Ecol. Econ. 65, 145–154. doi: 10.1016/j.ecolecon.2007.05.014
Wolf, M. J., Emerson, J. W., Esty, D. C., de Sherbinin, A., and Wendling, Z. A. (2022). Environmental performance index. New Haven, CT: Yale Center for Environmental Law & Policy. epi.yale.edu, 1–206.
Wu, S. C., Cao, Z. H., Li, Z. G., Cheung, K. C., and Wong, M. H. (2005). Effects of biofertilizer containing N-fixer, P and K solubilizers and AM fungi on maize growth: a greenhouse trial. Geoderma 125, 155–166. doi: 10.1016/j.geoderma.2004.07.003
Keywords: willingness, behavior, technology acceptance model, biological inputs, food safety
Citation: Forouzani M, Bondori A and Mombini AS (2024) Why are farmers reluctant to accept biological inputs? a structural equation model of technology adoption. Front. Sustain. Food Syst. 8:1360887. doi: 10.3389/fsufs.2024.1360887
Edited by:
Christian Schader, Research Institute of Organic Agriculture (FiBL), SwitzerlandReviewed by:
Christian Grovermann, Research Institute of Organic Agriculture (FiBL), SwitzerlandZhong Ren, Shandong Normal University, China
Copyright © 2024 Forouzani, Bondori and Mombini. This is an open-access article distributed under the terms of the Creative Commons Attribution License (CC BY). The use, distribution or reproduction in other forums is permitted, provided the original author(s) and the copyright owner(s) are credited and that the original publication in this journal is cited, in accordance with accepted academic practice. No use, distribution or reproduction is permitted which does not comply with these terms.
*Correspondence: Masoumeh Forouzani, bS5mb3JvdXphbmlAYXNucnVraC5hYy5pcg==