- 1Department of Environmental Science, Policy, and Management, University of California, Berkeley, Berkeley, CA, United States
- 2Department of Entomology, University of Kentucky, Lexington, KY, United States
- 3The Organic Center, Washington, DC, United States
- 4Department of Corporate Responsibility, Handsome Brook Farms, New York, NY, United States
- 5Department of Zoology, Biodiversity Research Centre, Institute for Resources, Environment, and Sustainability, University of British Columbia, Vancouver, BC, Canada
Sustainable pest management requires growers and regional land managers to consider the relationships among pest management practices, pest and natural enemy communities, crop loss, and multi-scalar habitat complexity. However, the causal links among these variables, in particular potential interactions between landscape and local-scale habitat complexity, remain underexplored. In the context of organically managed strawberry crops in California’s Central Coast, we tested the independent effects of landscape and local habitat complexity gradients on arthropod communities and crop loss using a piecewise structural equation model (PSEM). We found that landscape-scale woody habitat proportion indirectly decreased crop loss through its positive effect on natural enemy abundance, while grassland proportion had the opposite effect due to its association with an important strawberry pest (Lygus spp.). We detected a pattern suggesting that on-farm diversification practices are most effective at reducing crop loss at an intermediate level (26%) of woody habitat proportion. Both organic-compliant insecticide application and tractor vacuuming negatively impacted natural enemies, and therefore had qualified effects on crop loss. Our study shows the key roles of native woodlands and natural enemy communities in reducing crop loss and highlights the importance of managing habitat complexity at both landscape and local scales.
1 Introduction
Maintaining and restoring habitat complexity in agricultural landscapes to promote pest control services can lead to significant economic, ecological, and public health benefits (Kremen and Miles, 2012). Broadly, habitat complexity can be measured at different spatial scales and encompasses numerous land cover characteristics, including land cover diversity, crop and non-crop plant diversity, (semi)natural habitat proportion, and configuration. Habitat complexity in agricultural landscapes is generally associated with healthier (in terms of abundance and diversity) communities of beneficial insects, including pollinators and natural enemies of agricultural pests (Dainese et al., 2019; Kremen et al., 2007; Sánchez et al., 2022; Sirami et al., 2019). Natural enemies provide pest control ecosystem services and theoretically reduce pest-induced crop loss in a process known as top-down control or the enemies hypothesis (Root, 1973). In contrast, landscapes dominated by crops, and especially monocultures, theoretically provide abundant resources for pests and increase pest pressure via the resource concentration hypothesis, also known as bottom-up effects (Gurr et al., 2017; Root, 1973). In practice, however, habitat complexity studies have shown highly variable effects on pest control and crop loss due to differences in spatial scale, types of habitat, crop types, arthropod traits, and farm management practices, among other factors (Karp et al., 2018; Tscharntke et al., 2016). In order to design landscapes and manage farms that balance short-term productivity with long-term sustainability, policymakers and growers need to know the environmental conditions at landscape and farm scales that are most likely to enhance natural pest control and reduce pest-induced crop loss. Toward this end, we present a study to test the network of direct and indirect causal links among: (a) orthogonal (i.e., independent) gradients of landscape composition and local (farm-scale) diversification practices; (b) local pest management practices; (c) arthropod communities (pests and their natural enemies); and (d) crop loss, in a strawberry growing region of California’s Central Coast.
Complexity at both local and landscape scales influences pest control ecosystem services. Several meta-analyses have concluded that local-scale plant diversity positively affects natural enemy communities, levels of pest control, and crop yield (Beillouin et al., 2021; Judt et al., 2023; Letourneau et al., 2011; Tamburini et al., 2020), but others show that these effects are inconsistent under different landscape contexts (Boetzl et al., 2020; Dassou and Tixier, 2016; Tscharntke et al., 2012). At the landscape scale, meta-analysis reveals that complexity generally increases natural enemy abundance (Chaplin-Kramer et al., 2011b; Duarte et al., 2018), but effects on pest control services remain inconsistent and controversial (Karp et al., 2018; Rusch et al., 2016). A dominant source of variability among studies may be the interaction between different scales of complexity. For example, in landscapes with high proportions of natural habitat, colonization by low-mobility natural enemies may require local-scale features such as hedgerows to improve connectivity (Martin et al., 2016, 2019). Conversely, the effectiveness of local-scale diversification may also depend on landscape-scale complexity. Under the “intermediate landscape complexity hypothesis,” simplified landscapes may contain insufficient habitat to sustain the natural enemy meta-populations needed to provide effective pest control even with ample local diversification, while in highly complex landscapes, pest control services may already be robust and thus adding local complexity may be redundant (Tscharntke et al., 2012). Therefore, local diversification may have the highest impact at intermediate levels of landscape complexity. Despite the logical appeal of this hypothesis, few empirical studies have uncovered evidence of its operation (but see Beaumelle et al., 2021; Jonsson et al., 2015; Sánchez et al., 2022; Wilson et al., 2017).
Representing local-scale complexity is another challenge in multi-scale studies. Some studies investigating the interaction between habitat complexity at landscape and local scales have relied on the organic/conventional dichotomy as a proxy for local diversification (Martin et al., 2016; Saqib et al., 2020). Although organic management can increase biodiversity relative to conventional management (Hole et al., 2005) and often relies on diversification practices to provide critical inputs to farming (Kremen and Miles, 2012), organically managed farms also include monocultures that employ ecologically disruptive pest control methods like organic-compliant insecticides and arthropod vacuuming (Guthman, 2000; Lu et al., 2022; Swezey et al., 2007; Tscharntke et al., 2021). Thus, organic management does not reliably signify high on-farm complexity.
In Europe, where government-supported agri-environment schemes (AES) encourage growers to deploy specific non-crop diversification features such as flower strips, hedgerows, and fallow fields (Batáry et al., 2015), pest control researchers have studied multi-scale interactions by representing local complexity with presence/absence of an AES-compliant feature or comparisons between feature types (Beaumelle et al., 2021; Dainese et al., 2017). In the United States, pest control studies on specific feature types are less common (but see Wilson et al., 2017), perhaps because government incentives for local diversification are less accessible and consistent than in Europe, leading growers to use a patchwork of different diversification practices. A composite, continuous local diversification score comprising various practices is therefore a practical way to represent local complexity in our study region. A composite score also aligns with growing evidence that simultaneously deploying multiple diversification strategies, rather than individual ones alone, is more likely to generate positive social and environmental outcomes (Rasmussen et al., 2024). Despite the advantages of doing so, few studies have used continuous, orthogonal gradients to explore how interactions between multi-scale habitat complexity contribute to pest control services (but see Garcia et al., 2023).
Even fewer studies have measured crop yield (or loss), which is a crucial gap because it embodies the economic bottom line for growers (Chaplin-Kramer et al., 2011b; Kleijn et al., 2019), and it often does not correlate with measured levels of pest control, or even measured levels of herbivory (Iverson et al., 2014). Factors other than pest control can strongly affect yield, including management history, abiotic conditions, and soil quality (Liere et al., 2015; Schellhorn et al., 2015). The handful of studies that have investigated the interaction effect between landscape and local habitat complexity on crop yield have not revealed a consistent pattern. Some found evidence of an interaction effect with respect to pest control but not yield (Midega et al., 2014; Wilson et al., 2017), others have found no interaction effect on either (Albrecht et al., 2020), while others have found an effect on both (Gagic et al., 2021; Jonsson et al., 2015). All of these prior studies represent local diversification with an organic/conventional dichotomy or the presence of specific AES features. To our knowledge, this is the first study to explore the crop loss effect of a landscape by local-scale interaction using a composite continuous local diversification score.
Two additional hypotheses regarding the inconsistent effect of habitat complexity on pest control are the contrasting effects of different types of non-crop habitat (Karp et al., 2018), and the potentially confounding effect of local pest management practices such as insecticide application (Tscharntke et al., 2016). We investigate non-crop differences by separating woody habitats from grasslands, which we expect to support different arthropod groups. We investigate local pest management practices by including covariates for two commonly used pest control practices in this region: frequency of tractor-mounted arthropod vacuuming and intensity of insecticide application.
We collected data for this study in the strawberry growing region of California’s Central Coast centered around Monterey Bay. Our study focused on the lygus bug, an economically important strawberry pest complex in this region, and its predator community. Absent pest management, an estimated 26–54% of strawberries grown in this region show signs of lygus-induced malformation resulting in crop losses (Pickel et al., 1995). Unlike crops whose yield is defined solely by harvested biomass, crop loss in strawberries can be estimated from crop damage surveys because yield is tracked by both weight and number of harvested (i.e., marketable) berries (Bolda et al., 2024). While the woody habitats in this region are generally perennial, native, and biodiverse, and are expected to provide resources for natural enemies, the grasslands are unmanaged and dominated by exotic grasses and annuals, many known to be lygus bug hosts (Fye, 1982; Scott, 1977). We sampled at sites located along orthogonal gradients of landscape composition and local diversification practices. At each site, we collected lygus bugs and natural enemies and surveyed strawberries to estimate lygus-caused crop loss. Our study addresses the following questions: (1) How do landscape-scale and local-scale habitat complexity interact to alter arthropod communities and crop loss, and how are those impacts influenced by pest management practices; and (2) What are the direct and indirect causal links among multi-scale habitat complexity, arthropod communities, and crop loss (Figure 1)?
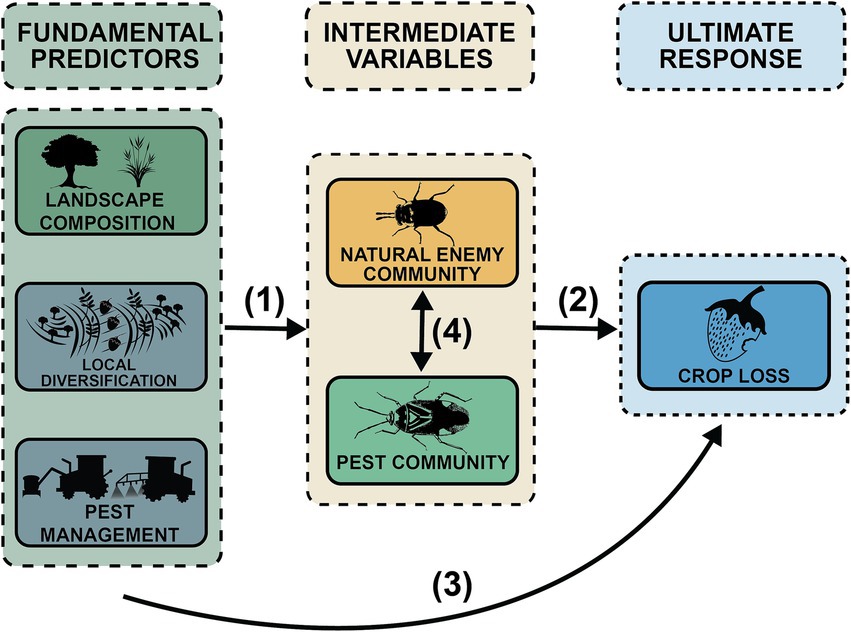
Figure 1. The PSEM tests the causal relationships between (1) the Fundamental Predictors and Intermediate Variables, (2) the Intermediate Variables and the Ultimate Response, (3) the Fundamental Predictors and the Ultimate Response, and (4) the correlated error between Intermediate Variables.
2 Methods
2.1 Study system and site selection
The study was carried out in the Santa Cruz-Watsonville-Salinas area of California’s Central Coast, a region characterized by a mosaic landscape of natural habitat mixed with high agricultural productivity, heavy pesticide use, and unique biodiversity (Karp et al., 2016). The mean annual high and low daily temperatures are 20.1 and 8.9°C, respectively, and annual precipitation averages 319.5 mm. During the peak strawberry harvest season of May–August when we sampled, the mean high and low daily temperatures are 21.3 and 11.9°C, respectively, and precipitation during those months averages 10.6 mm, of which 9.7 mm falls in May (NOAA, 2024a). The elevation at the Salinas Municipal Airport weather station is 22.3 m (NOAA, 2024b). To document the impact of local and landscape diversification on strawberry pests, their natural enemies, and crop damage, we sampled 27 organic strawberry farms from May–August in 2015 and 2016. Given that many organic strawberry farmers lease multiple spatially independent farms to accommodate crop rotation, some study sites were different between years. Thus, 17 sites were sampled in both years, 10 were unique to 2015, and another 10 were unique to 2016. Sites were selected along orthogonal gradients of landscape composition and local diversification practices, as described below. All sites were organically-managed farms containing strawberry as either the sole crop or as one of many types of crops, and were defined as contiguous cultivated areas under the same manager. Sites ranged in size from 0.88 to 100.3 hectares.
2.2 Local diversification score
A continuous, composite diversification score (hereafter, “diversification score”) was created by tallying on-farm diversification practices including four crop-related (crop diversity, crop rotational length, perennial crop structure, and cover crops) and three non-crop related elements (non-flowering non-crop plants, flowering non-crop plants, and water features). Water features were included in the score because they are accompanied by non-crop vegetation, increasing in diversity from sparsely vegetated sediment retention ponds to highly diverse riparian areas. After taking on-site observations, we scaled the observation states for each element at each site (unweighted) and then used Principal Components Analysis (PCA) to reduce the number of variables and create a composite score based on a site by score matrix (Supplementary Table S1). We used the function prcomp in the R package stats to perform the PCA. The first axis of the PCA, which accounted for 37% of the variance in our data, was used as a continuous variable representing local diversification (for further details, see Lu et al., 2022).
2.3 Landscape variables
The United States Geological Survey’s National Agriculture Imagery Program (NAIP) data was used to digitize landscape cover within 1,000 m buffers around each site (Earth Resources Observation and Science (EROS) Center, 2017). NAIP data is collected by aircraft at one meter spatial resolution. We used 2014 NAIP data and confirmed that the land cover in our buffers had not changed in the 2015 and 2016 data. Land cover categories were based on the Salinas-Pajaro Generalized Land Use / Land Cover Mapping and the Anderson Level II hierarchy (Karp et al., 2015). We reclassified the default land cover categories by placing native/riparian forest and woodland, exotic tree patches, chaparral and scrubland in the woody habitat category; and herbaceous vegetation, Mediterranean grasslands and forbs, and pasturelands in the grassland category. The grasslands in this region are not natural habitat; they are unmanaged or used as pasture, and are dominated by exotic annual grasses and forbs. Proportions of woody habitat and grasslands were not correlated (ρ = 0.11, p = 0.45), largely due to a high proportion of cropland in the study region.
The most predictive buffer sizes for each response variable were determined by first regressing the response variable against each landscape proportion at buffer sizes ranging from 500 m to 1,000 m and then selecting the top-ranked (by AICc) buffer size for each response-landscape type combination (Jackson and Fahrig, 2012, 2015, Supplementary material). Neither of the landscape types was correlated with local diversification (|ρ| < 0.21; p > 0.12) at any of the selected buffer sizes, confirming the independence of the gradients (Supplementary Figure S1).
2.4 Pest management variables
While the premise of organic agriculture is fundamentally similar across the globe, variations in certification standards exist across countries. The growers in our study were certified to the standards set by the USDA National Organic Program (NOP) (National Organic Program, 2000) whose standards are outlined for growers in the NOP Handbook (USDA, 2024b). The USDA NOP Guidance on Natural Resources and Biodiversity Conservation adopted language in 2016 stating that “the [organic] producer must initiate practices to support biodiversity and avoid, to the extent practicable, any activities that would diminish it,” directing growers to use organic compliant pesticides only as a last resort (USDA, 2024a). The National List of Allowed and Prohibited Substances outlines the categories of substances in organic production (USDA, 2024c) and the Organic Materials Review Institute (OMRI) provides a list of USDA Organic compliant pesticides approved for use on our study sites (OMRI, 2024).
USDA organic compliant pesticides that are commonly applied in the study area were classified into one of three categories: targeting lepidopteran larvae (Bacillus thuringiensis-based (Bt)), broad spectrum (spinosad, pyrethrin, or Chromobacterium subtsugae-based), and targeting soft-bodied pests such as aphids, thrips, and whiteflies (Beauvaria bassiana or azadirachtin-based). Insecticide intensity and tractor vacuum frequency were included as predictor variables to test their effects on arthropod communities and crop damage. In our study, insecticide intensity was assessed as an index from 0 to 3 indicating the number of insecticide categories applied; application frequency was not included in the index because farm managers seldom reported this information. The insecticide intensity variable is a proxy for the level of chemical management and does not directly link chemical modes of action with berry damage. For example, we do not expect Bt application to directly affect lygus damage; however, Bt may indirectly affect pest control and lygus damage by reducing alternative prey or imposing sub-lethal effects on natural enemies (De Bortoli et al., 2017). Tractor vacuum frequency ranged from 0 to 2 and indicates the number of times per week that the site was vacuumed during the growing season, as reported by farm managers.
2.5 Arthropod sampling
At each site, we sampled arthropod communities at three sampling stations located within the strawberry crop. Plants within an area of approximately 10 m by 15 m were sampled at each site. To assess natural enemy and pest abundance and diversity, we sampled arthropods on 150 strawberry plants (50 per station) using a Stihl BG55 leaf blower in vacuum mode, directing the vacuum tube over each plant and holding for 1 s (method modified from Swezey et al., 2007). To reduce the effect of arthropods fleeing the sound of the vacuum, we sampled one of every five plants, skipping four plants between samples. Arthropods were trapped by a filter fabric bag attached to the vacuum tube entrance. To reduce bias caused by differences in arthropod activity under varying weather conditions, sampling occurred only under the following conditions: ambient temperature 20–28° C, average wind speed ≤3.0 m/s, and no fog or precipitation.
Natural enemy specimens were sorted into the following categories, all of which are known predators of lygus bugs: Geocoris spp. (Hemiptera), Orius spp. (Hemiptera), Coccinellidae (Coleoptera), Nabis spp. (Hemiptera), Neuroptera, Formicidae (Hymenoptera), and Araneae (Bolda et al., 2019; Hagler, 2011). The categories represent functional groups identified by previous lygus bug predation studies (Hagler et al., 2020a,b; Leigh and Gonzalez, 1976; Perkins and Watson, 1972; Wilson and Gutierrez, 1980; Zink and Rosenheim, 2008). The lygus bug complex in this region primarily comprises Lygus hesperus, and also includes small proportions of L. elisus and L. lineolaris (Hagler et al., 2020b). Individuals of all Lygus species were included in the lygus bug category.
Abundance measures are counts of individual specimens belonging to each order or finer classification. Shannon’s diversity index H′ was used to calculate natural enemy diversity (Magurran, 2013).
2.6 Strawberry damage survey
At each site, we surveyed 50 strawberry plants at each sampling station in 2015 (150 per site) and 20 strawberry plants at each station in 2016 (60 per site). Sampling effort in the second year was reduced following a power analysis indicating that the reduced sampling would suffice for this study’s statistical analysis. For each plant, surveyors recorded the number of unripe (≥20% white surface color) berries and ripe (<20% white surface color) berries. Green berries were not included in the analysis because they are too small to consistently detect damage. All unripe and ripe berries were then classified according to the damage categories in Table 1. We performed a greenhouse study that compared the appearances of berries subjected to (1) lygus damage in the flower stage, (2) lygus damage in the berry stage, and (3) insufficient pollination (Sciligo et al., 2022) to determine characteristics of damage unequivocally attributed to lygus bugs. We found that berries subjected to lygus damage at the berry stage could be distinguished based on the formation of fully formed achenes within the puckered region. However, insufficient pollination alone could not be reliably distinguished from berries subjected to lygus damage at the flower stage (Figure 2). To isolate the effect of lygus bugs on crop damage, we included in this analysis only berries that, according to visual inspection, were damaged by lygus bugs at the berry stage and excluded those whose damage may have been caused by insufficient pollination. To estimate the crop loss, we categorized both unripe and ripe berries with damage levels 2 and 3 as damaged, representing berries that would be culled at harvest (Table 1).
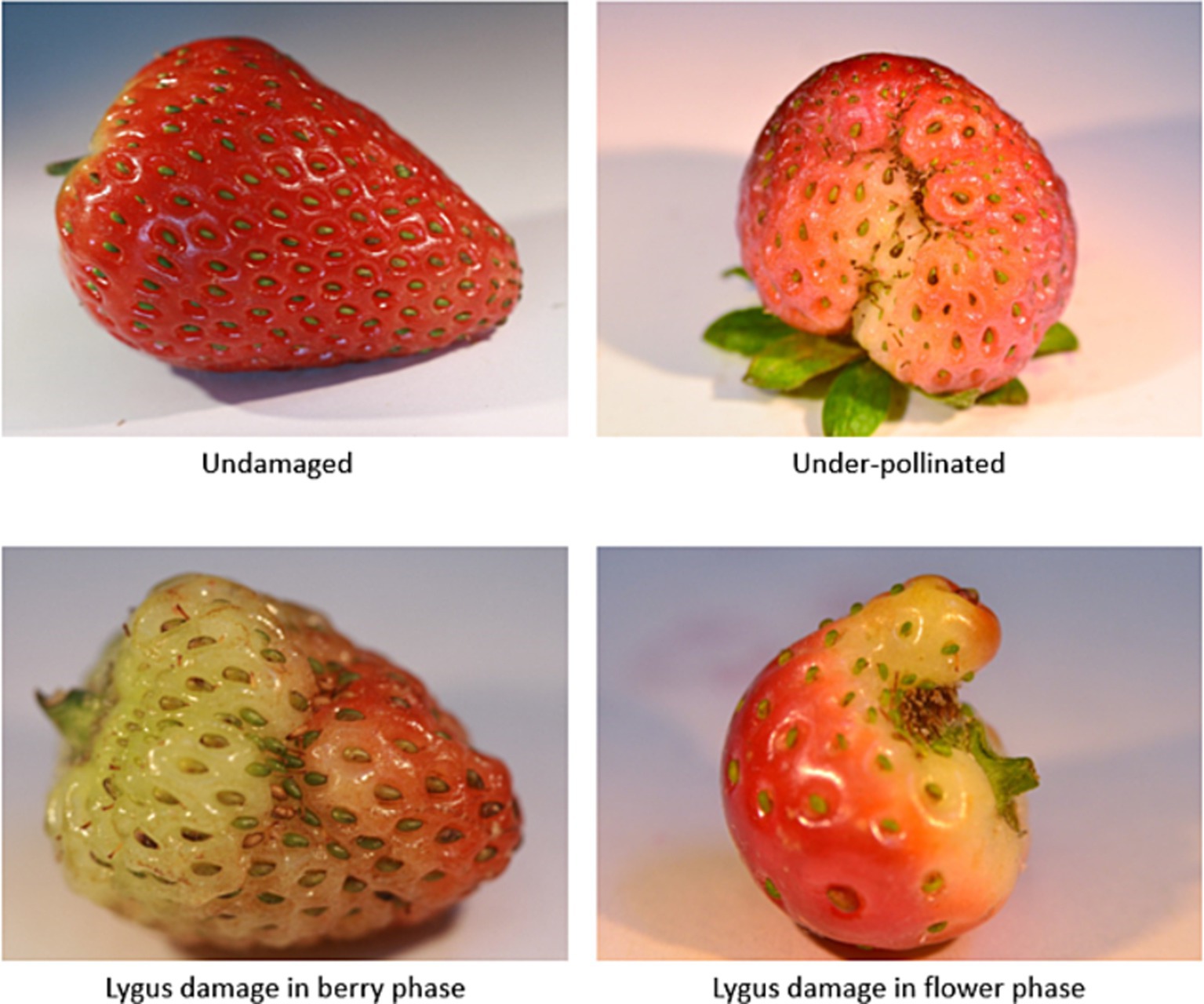
Figure 2. Representative strawberries showing the visual characteristics of different malformation types. Under-pollination and lygus damage in the flower phase show both puckered flesh (cat-facing) and under-developed achenes, while lygus damage in the berry phase shows puckered flesh with fully developed achenes. These characteristics were used to limit the category of damaged berries in this study to those that were damaged by lygus in the berry phase.
2.7 Statistical methods
Statistical analyses were performed using the open source statistical programming language R (R Core Team, 2019). Piecewise structural equation modeling (PSEM) tests hypothesized causal links among predictor variables, response variables, and intermediate variables (variables acting as both predictors and responses) (J. S. Lefcheck, 2016). PSEM differs from traditional structural equation modeling (SEM) in that it represents a path diagram as a set of individually evaluated linear equations (i.e., pieces), whereas traditional SEM relies on global estimation from the observed variance–covariance matrix of the entire model. The piecewise approach enables the PSEM to fit hierarchical data with non-normally distributed errors, which is typical of ecological data.
The predictor variables, response variables, and intermediate variables in the PSEM are depicted in the path diagram (Figure 1). The terminal response variable is crop damage odds (the ratio of the probabilities of a berry being damaged versus undamaged). Landscape composition, local diversification and pest management practices, interaction effects between landscape factors and local diversification, and interaction effects between landscape factors and time (days since rain, discussed below) are the hypothesized fundamental predictor variables. Lygus abundance, natural enemy abundance, and natural enemy diversity are the hypothesized intermediate variables. To test for parabolic changes in the effect of local diversification at different values of landscape composition (i.e., the intermediate landscape complexity hypothesis), we included linear and quadratic terms of both landscape composition factors (woody and grassland) in their respective interaction terms with local diversification.
The R package piecewiseSEM accepts pieces in the form of linear equations based on the causal paths represented in Figure 1 (Lefcheck et al., 2023). A multi-model inference process based on AICc ranking was used to select these linear models (Symonds and Moussalli, 2011; Supplementary material). The PSEM consists of three pieces – one equation for each of the response variables (crop damage odds, natural enemy abundance, and lygus abundance). We hypothesized natural enemy diversity as a predictor variable of crop damage odds, but it was rejected in the model selection process and therefore not included in the PSEM. All three pieces were generalized linear mixed models. The crop damage odds response variable was modeled using the binomial error distribution with R package lme4 and the two abundance response variables were modeled using the quadratic parameterization of the negative binomial error distribution with R package glmmTMB (Bates et al., 2015; Brooks et al., 2017). For each response variable, we began with a full model including all hypothesized predictors, as well as the covariates of site size in hectares, days since rain (DSR), and DSR2. DSR was used in lieu of Julian date (day of year), which is often included as a covariate to account for phenological changes in arthropod communities. A rain day is defined by the United States Geological Survey as 1 mm of rain within a 24-h period (United States Geological Survey, 2007). We opted to use DSR because it is a more accurate proxy of phenology than Julian date in our study area’s Mediterranean climate. We included DSR2 to account for potential non-linear patterns in arthropod phenology which have been detected across seasons in California (Leong et al., 2016). A random effect of site-year was included in all models to avoid pseudo-replication. The site-year random effect, rather than separate site and year effects, was used because a subset of sites (10 per year) was not sampled in both years. Based on the full model structures for each response variable, model structures containing all combinations of fixed effect terms were generated and ranked by AICc using the R package MuMIn (Barton, 2024). Three model structures corresponding to the three response variables (Table 2) were then chosen from the top-ranked structures (Supplementary Tables S3–S5) and entered into the PSEM.
Because we expected that natural enemy abundance and lygus abundance would be correlated, but we had no hypothesis regarding the causal relationship between them, we ran three versions of the PSEM. The first included a correlated error between natural enemy and lygus abundance, representing the hypothesis that their correlation is driven by additional, unmeasured predictor variables (Shipley, 2000). The second and third versions represented the hypotheses that natural enemy abundance drove lygus abundance and vice versa. We then selected the model with the best fit according to the Fisher’s C statistic (Lefcheck, 2016; Shipley, 2009, 2013).
As commonly found with count data, the natural enemy and lygus abundance data were overdispersed; the negative binomial error distribution was used to account for overdispersion (Brooks et al., 2017; Hardin and Hilbe, 2007). No significant spatial autocorrelation was found in the residuals of the linear model pieces according to the Moran’s I test in R package ape (Paradis and Schliep, 2019). We calculated variance inflation factors (VIF) for each linear model to confirm the absence of multicollinearity (all VIFs ≤5.0). Overdispersion, uniformity, outliers, and zero inflation for each of the linear models were tested using R package DHARMa (Hartig and Lohse, 2022).
3 Results
We collected 5,071 natural enemy specimens (total individuals across all natural enemy categories) and 1,347 lygus bugs. We surveyed a total of 32,706 berries, of which 3,518 (~11%) had level 2 or 3 lygus bug damage. The PSEM confirmed that landscape-scale habitats had both direct and indirect effects on crop damage odds (Figure 3; Supplementary material). At α = 0.05, grassland proportion directly influenced berry damage odds (linear component p = 0.03 and quadratic component p < 0.001; Figure 4A). Woody proportion had a direct positive effect on natural enemy abundance (0.7 ± 0.3%, p = 0.05, Figure 4B), and grassland proportion had a similar, nearly significant effect (1.2 ± 0.7%, p = 0.07). The interaction between proportion grassland and the quadratic term of days since rain significantly influenced lygus abundance (p = 0.01, Figure 5A).
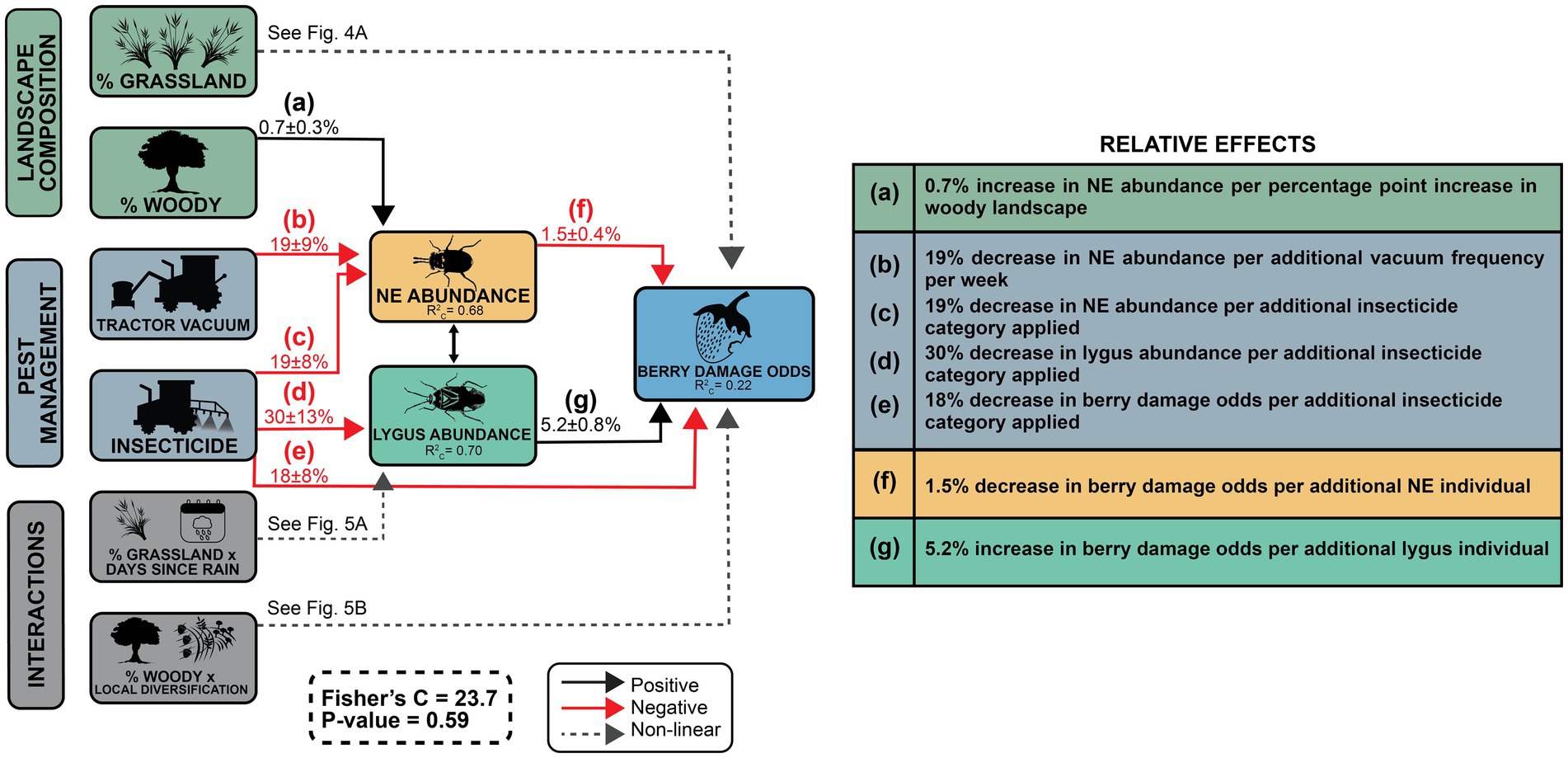
Figure 3. PSEM results. Global goodness-of-fit is represented by Fisher’s C = 23.7 (p = 0.59), indicating the model is well-fit. Conditional R2 (R2C) values indicate the variance attributed to both fixed and random effects (Nakagawa and Schielzeth, 2013).
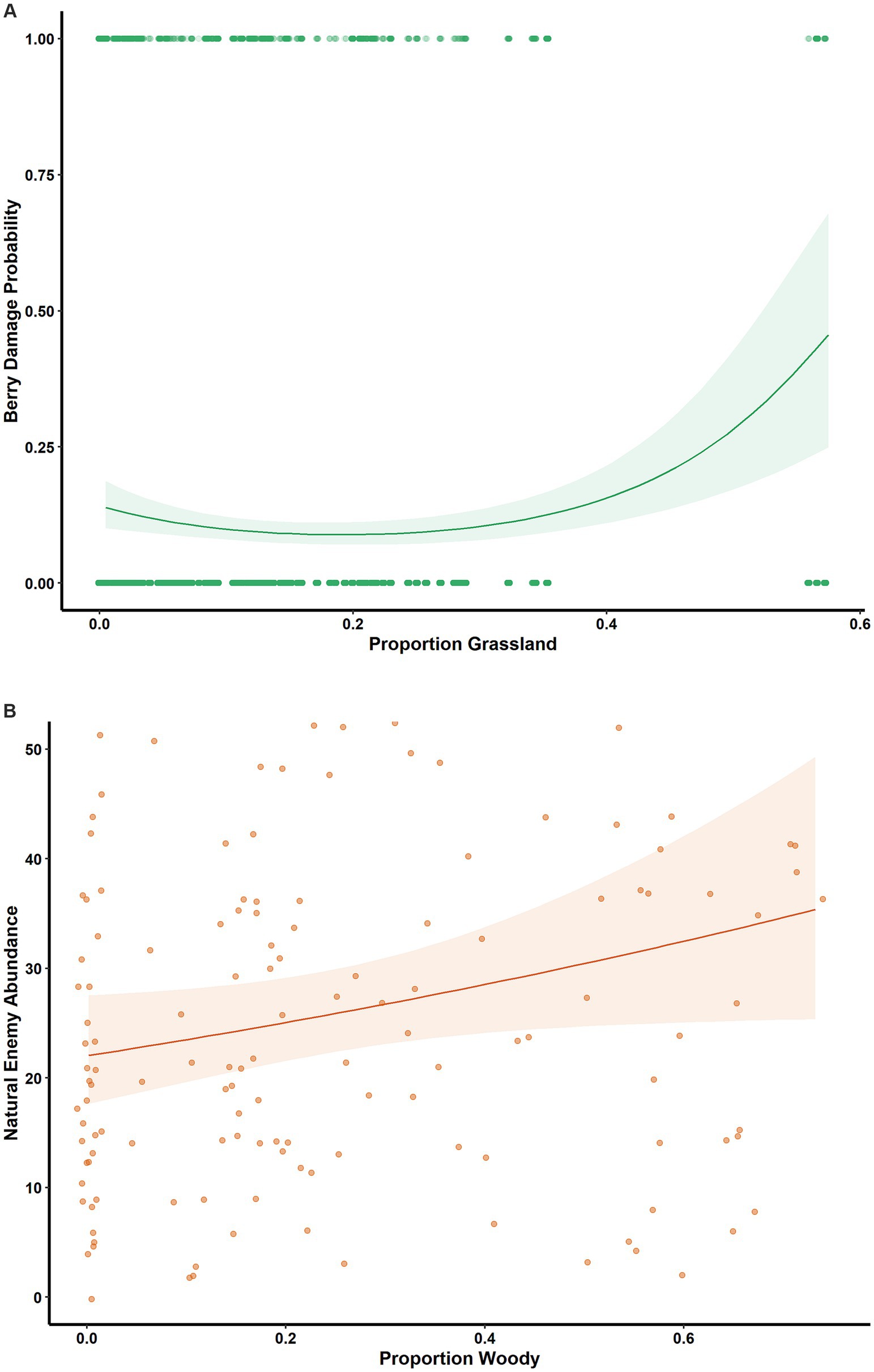
Figure 4. Landscape-scale effects. (A) Estimated effect of grassland proportion on berry damage probability (plinear = 0.03; pquadratic <0.001); generalized linear mixed model with binomial error distribution; (B) Estimated effect of woody proportion on natural enemy abundance (0.7 ± 0.3%, p = 0.05); generalized linear mixed model with negative binomial error distribution.
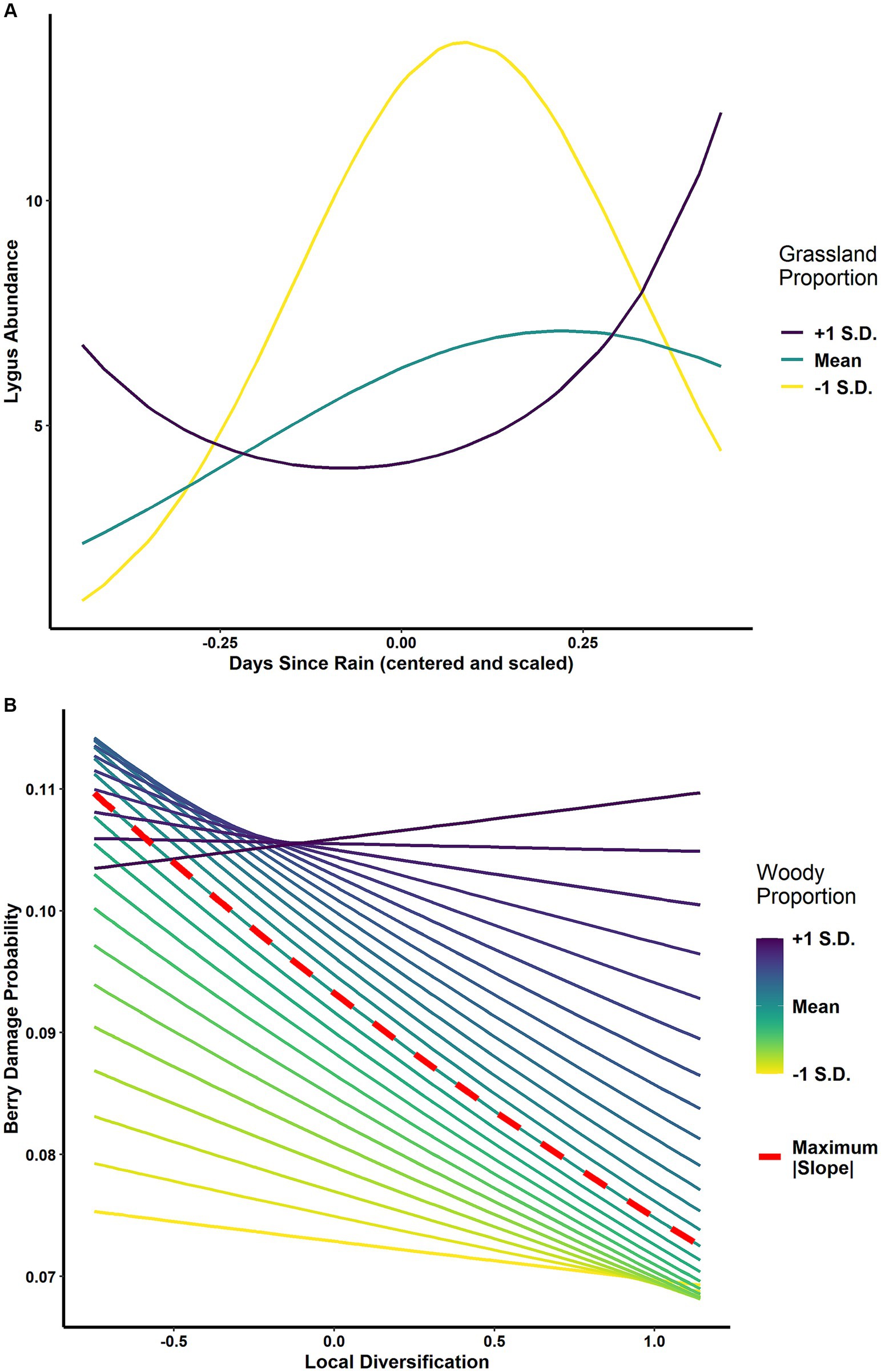
Figure 5. Landscape-scale interaction effects. (A) Estimated interaction effect between grassland proportion and days since rain on lygus abundance (pquadratic = 0.01), visualized as the effect of days since rain on lygus abundance at +/- 1 S.D. and mean values of grassland proportion; generalized linear mixed model with negative binomial error distribution; (B) Estimated interaction effect between woody proportion and local diversification on berry damage probability (pquadratic = 0.04); generalized linear mixed model with binomial error distribution. Lines represent simple slopes (effect of local diversification on berry damage) at values of woody proportion from +1 S.D. to -1 S.D.; the dashed red line indicates the highest magnitude simple slope (β = –0.24 ± 0.16, p = 0.13), which occurs at woody proportion = 0.26; generalized linear mixed model with binomial error distribution.
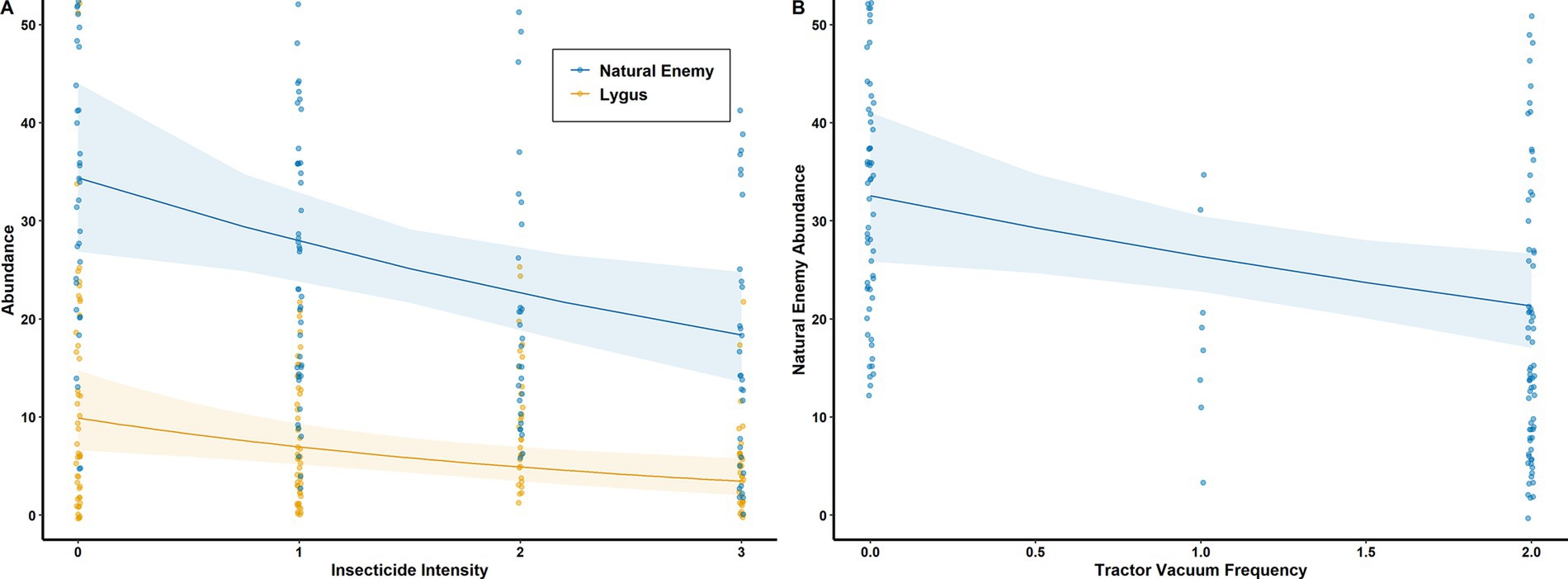
Figure 6. (A) Estimated effects of insecticide intensity on natural enemy (-19 ± 8%, p = 0.007) and lygus (-30±13%, p = 0.003) abundance; (B) Estimated effect of tractor vacuum frequency on natural enemy abundance (-19 ± 9%, p = 0.02). Generalized linear mixed models with negative binomial error distributions.
We detected a direct effect of the interaction between local diversification and woody proportion on berry damage odds (p = 0.04). Logistic regression requires a transformation of the original response variable (expressed in probability or odds) into logit space (log odds) in order to linearize the regression equation. A consequence of this transformation is that significant interactions in logit space may not always reflect significant effects in probability space. The significance of interaction effects in probability space are calculated using simple slopes, which are the slopes of the predictor variable (here, local diversification) at specified values of the moderator variable (here, woody proportion). When berry damage odds are expressed as probabilities, the simple slopes of local diversification at ±1 S.D. of woody proportion are not significantly different from zero (β = 0.04 ± 0.22, p = 0.87 at +1 S.D.; β = −0.05 ± 0.20, p = 0.82 at −1 S.D.). The simple slope of local diversification at mean woody proportion was also not significant (β = −0.24 ± 0.16, p = 0.13), but we detected a pattern showing that the slope of local diversification is highest in magnitude at an intermediate value (26%) of woody proportion (Figure 5B).
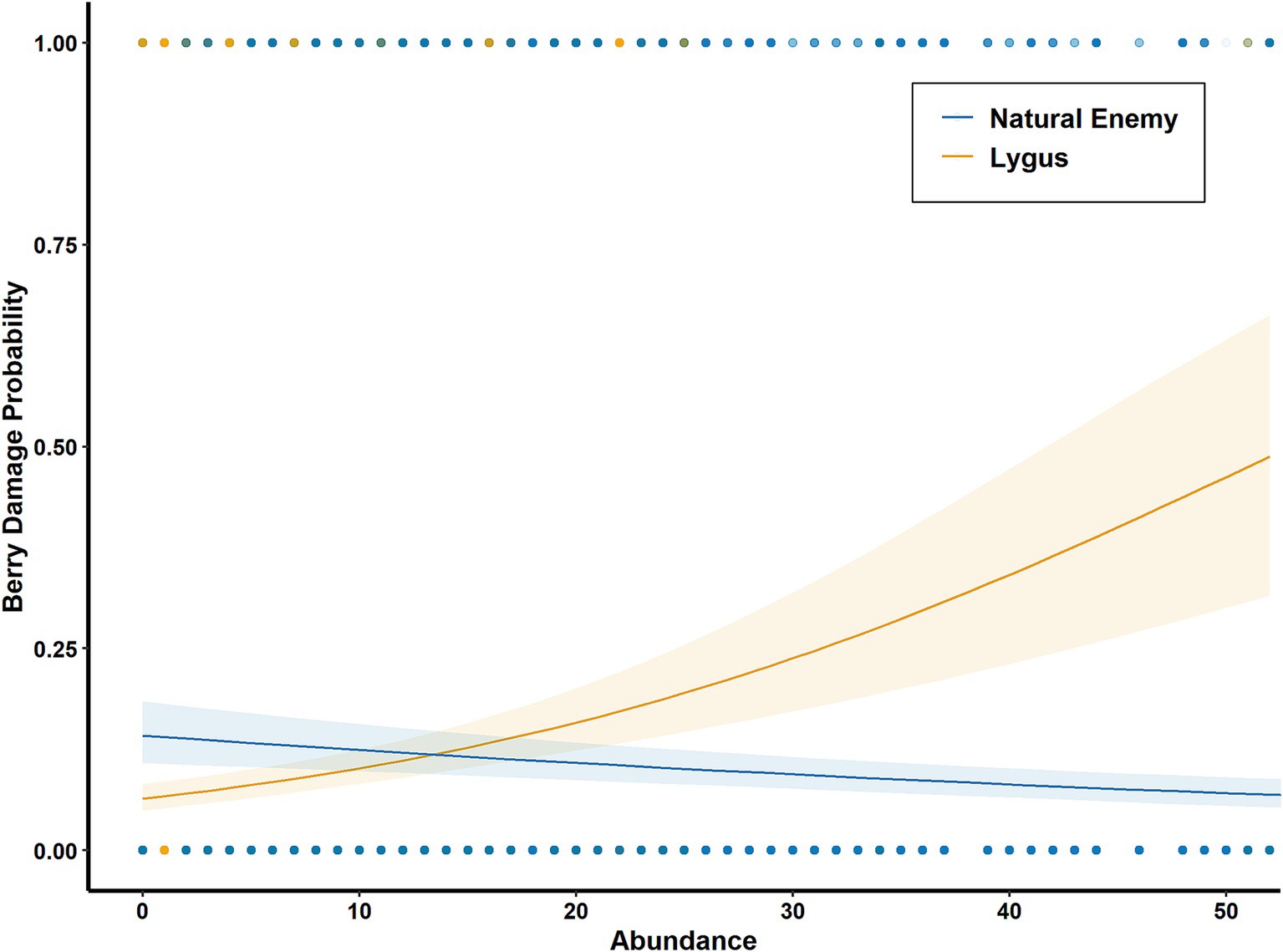
Figure 7. Estimated effects of natural enemy (-1.5 ± 0.4%, p < 0.001) and lygus (5.2 ± 0.8%, p < 0.001) abundance on berry damage probability; generalized linear mixed models with binomial error distributions.
Farm-scale pest management influenced berry damage odds directly as well as indirectly through its effects on arthropod communities. Both natural enemy (–19 ± 8%, p = 0.007) and lygus (–30 ± 13%, p = 0.003) abundance decreased with insecticide intensity (Figure 6A). Tractor vacuum frequency decreased natural enemy abundance (–19 ± 9%, p = 0.028, Figure 6B), but had no effect on lygus abundance. Since higher lygus abundance directly increased berry damage odds (5.2 ± 0.8%, p < 0.001), while natural enemy abundance had the opposite effect (–1.5 ± 0.4%, p < 0.001, Figure 7), pest management practices indirectly affected berry damage via effects on these arthropod groups. Insecticide intensity also directly reduced berry damage odds (–18 ± 8%, p = 0.008).
Of the three PSEM models, the correlated error model (Figure 3; Supplementary material) had the best fit, with Fisher’s C = 23.7 with p-value = 0.59, indicating that unmeasured factors drive both natural enemy abundance and lygus abundance more than they drive each other.
4 Discussion
Our results reveal that multi-scalar habitat complexity and local management practices influence crop loss directly as well as indirectly through their direct effects on arthropod communities. We found that natural enemy abundance decreased crop damage odds while lygus abundance had the opposite effect (Figure 7), evidence in support of the enemies hypothesis (top down control). Because our definition of berry damage aligns with the level of damage at which berries are culled at harvest, berry damage probability (Figures 4A, 5B, 7) can be interpreted as the estimated rate of crop loss. As we expected based on the contrasting plant communities between woody habitat and grasslands, natural enemy abundance, but not lygus abundance, increased with woody proportion in the landscape (Figure 4B). A significant interaction effect with days since rain shows that varying levels of grassland proportion influence lygus abundance differently as the growing season progresses (Figure 5A).We uncovered evidence for the intermediate landscape complexity hypothesis in the form of an interaction effect between woody proportion and local diversification on crop loss, showing that the effect of local diversification on crop loss has highest magnitude at an intermediate value of woodland proportion (Figure 5B). Regarding pest management practices, insecticides decrease natural enemy and lygus abundance, while tractor vacuuming decreases only natural enemy abundance (Figure 6). These results illustrate the benefits of habitat complexity at landscape and local scales on pest control services, highlighting the importance of preserving and restoring native woody vegetation, managing exotic weeds, utilizing farm diversification practices and accounting for the ecological costs of pest management.
Based on our knowledge of lygus damage in strawberries and the focus among extension researchers and growers on lygus bugs as the primary cause of berry malformation in this region, we had expected environmental effects on crop loss to be mediated entirely by lygus and natural enemy abundance. However, we found a direct effect of grassland proportion on crop loss (Figure 4A). We have two hypotheses regarding this unexpected direct effect. First, despite our best efforts, we may have included under-pollinated berries in our data. The results of the greenhouse experiment informed our protocol for visually distinguishing between malformation caused by under-pollination and lygus damage. Although we intended to limit our analysis to berries that were only lygus-damaged, our visual inspection could not have been perfect. Therefore, the changes in crop loss associated with grassland proportion may result in part from differences in pollination services. Prior work in this region has shown positive effects of landscape-scale forests and chaparral (i.e., woody habitat) on pollination services in crops, but these studies did not investigate the effects of pastures and non-native grasslands (Klein et al., 2012; Kremen et al., 2004). Studies on the interactions between pollinators, native plants, and invasive plants have shown that pollination of native plant species can be compromised in areas of high invasive density due to decreased flower visits and increased heterospecific pollen deposition (Bartomeus et al., 2008; Bruckman and Campbell, 2016; King and Sargent, 2012). Furthermore, species sensitive to pollen limitation may be at higher risk of negative impacts from invasive plants (Dietzsch et al., 2011). In strawberry crops, excluding pollinators has been found to double the probability of berry malformation (Sciligo et al., 2022), and insect pollination can increase the average commercial value of marketable fruits by 92% over self-pollination (Wietzke et al., 2018). Future work in this region targeting the effect of non-native grasslands on native pollinators and pollination services may confirm that under-pollination decreases strawberry quality in landscapes dominated by exotic weeds and grasses.
Second, the direct effect of grasslands on berry damage odds may indicate that non-lygus species induce visually similar berry malformation. Researchers recently found that an “emerging pest” called the strawberry seed bug (Neopamera bilobata) causes cat-facing in Florida-grown strawberries, (Talton et al., 2020). Furthermore, the thrips species Frankliniella occidentalis, present in California, has recently been observed to cause similar malformations (Strzyzewski et al., 2021), a finding contrary to conventional wisdom in the Central Coast region (Allen and Douglas, 1974; Bolda, 2022). These studies imply that the economic importance and damage characteristics of pest species remain controversial and that relationships between arthropod pests and crops are dynamic. The direct effect of insecticide on berry damage odds lends further support to this hypothesis, suggesting that insecticides negatively impact non-lygus pests that cause visually similar damage. While researchers and growers have historically focused on lygus bugs as the primary cause of berry malformation in this region, further experiments that expose strawberry flowers and fruit to understudied pest species may reveal additional economically important strawberry pests.
Grasslands in this region are dominated by exotic forbs and grasses known to be lygus hosts and were hypothesized to increase lygus abundance in nearby fields via bottom-up effects (i.e., the resource concentration hypothesis). Furthermore, we expected these effects to strengthen as weeds desiccated during the dry season, inducing more lygus bugs to seek the greener pastures of irrigated strawberry crops. The interaction between days since rain and grassland on lygus abundance supports the hypothesis that high grassland proportion promotes early lygus colonization of strawberry fields and feeds population growth throughout peak harvest season (Figure 5A). In contrast, at mean and low levels of grassland proportion, lygus abundance starts low but increases through mid-season before decreasing into late season.
The different effects of high and low grassland proportion in mid-season may be related to the role of grasslands as sources of natural enemies and the dynamic interactions between natural enemies and lygus. We detected a relatively strong, nearly significant positive effect of grassland proportion on natural enemy abundance, consistent with a previous study suggesting that grasslands may be a source of natural enemies as well as lygus bugs (Nieto et al., 2023). In general, natural enemies are attracted to sites with high food resources, commonly resulting in positive correlations between natural enemy and pest abundance (Eubanks, 2001; Lundgren et al., 2015). Here, natural enemies may follow lygus bugs into crop fields and eventually suppress lygus abundance where grassland proportion is relatively low. However, at high levels of grassland, where early-season lygus abundance is high and substantial lygus migration into crops continues as weeds desiccate, natural enemy suppression may never overcome lygus population growth. In agreement with previous studies in this region (Morandin et al., 2014; Nieto et al., 2023), our results reinforce the benefits of preserving and restoring native woods and shrubs while controlling invasive grasses and flowering weeds; relative to each other, woody habitats tend to support beneficial insects while grasslands tend to be sources of pests.
We wish to highlight here the importance of pest and natural enemy population dynamics in the investigation of pest control services (Costamagna et al., 2015; Gardiner et al., 2009). In particular, future work should examine the environmental factors and grower actions that would delay pest establishment and facilitate earlier colonization of crops by natural enemies, reducing the risk of lygus outbreaks (Le Gal et al., 2020). Studying the arthropod population dynamics associated with native plant species that support natural enemies in this region could be a fruitful starting point (Lundin et al., 2019).
In addition to the effects of landscape composition, we aimed to explore the relative contribution of local diversification to changes in arthropod communities and crop loss. The interaction between local diversification and woodland proportion exhibits evidence of the intermediate landscape complexity hypothesis with respect to crop loss (Figure 5B). Recently, systematic reviews and meta-analyses have found that it is critical to maintain 20–25% or more of natural and semi-natural habitats within agricultural working landscapes to maintain a wide variety of ecosystem services, including, but not limited to pest control (Garibaldi et al., 2021; Mohamed et al., 2024). Interestingly, our finding that diversification practices enhanced pest control at approximately the same proportion of natural/semi-natural habitat provides additional evidence for multi-scalar synergies (Estrada-Carmona et al., 2022) and reinforces both the policy goal of retaining or restoring ~20% of natural habitat within working lands and promoting on-farm diversification practices.
Despite the interaction effect between woody landscape and local diversification, we found no main effect of local diversification on either natural enemy or lygus abundance. These effects may have been obscured by the effect of small-scale weedy refuges, such as ditches, field edges, and roadside vegetation common in the region. These features are largely populated by exotic annual plants such as wild mustard (Brassica spp.), wild radish (Raphanus raphanistrum), and shepherd’s purse (Capsella bursa-pastoris), all known to be favored hosts of lygus bugs as well as other hemipteran pests (Fye, 1982; Malcolm, 1953). In some cases, these plants even provide enemy-free spaces for certain pest species (Chaplin-Kramer et al., 2011a). Although their surface area is miniscule, the ubiquity and linear configuration of these weedy strips may create a highly connected habitat across the landscape for certain organisms, generating outsized impacts on arthropod abundance and diversity that obscure the effects of local diversification (Haan et al., 2020; Thiele et al., 2018). Landscape configuration is relatively understudied in comparison to landscape composition, but recent studies have shown its influence on pest control services, and future work in this region controlling for linear weedy features may uncover relationships between local diversification and arthropod communities (Ali et al., 2023; Martin et al., 2016, 2019).
Local pest management techniques (insecticide application and tractor vacuuming) were included as covariates to address our primary research questions, but they also produced notable effects on arthropods (Figure 6) and berry damage odds (Figure 3). Despite the putative efficacy of insecticides in reducing crop loss, the goal of pest management is not merely to reduce pest abundance but to enhance pest control, and the effectiveness of insecticide application must be qualified by its negative impact on natural enemy communities. Insecticide use may have depressed natural enemy populations over a long time period, reducing their current contributions to pest control. Even when toxicology studies indicate similar insecticide impacts on natural enemy and pest species, natural enemies are generally more severely impacted due to population characteristics such as longer generation times and lower fecundity (Roubos et al., 2014; Stark et al., 2004). Sub-lethal effects of insecticides, including behavioral changes, can also impose greater harm on natural enemy populations than toxicological results suggest (Desneux et al., 2007). The contributions of insecticides to pest control must therefore be qualified by the possibility of past, cumulative impacts on natural enemy populations. These ecological costs must not be neglected when calculating the total cost of organic-compliant insecticide application.
We found no evidence that tractor vacuuming decreases lygus bug abundance or crop loss, but its negative effect on natural enemies is clear (Figure 6B). Organic strawberry growers may resist the idea that tractor vacuuming does more harm than good because it is touted as one of their few tools against lygus bugs (Wells et al., 2020). According to a number of studies from the preceding decades, the efficacy of tractor vacuuming is limited at best, and has never been shown to conclusively reduce lygus damage under an economically acceptable level (Joseph and Bolda, 2018; Pickel et al., 1994; Rancourt et al., 2003; Vincent and Lachance, 1993). Although we cannot unequivocally state that tractor vacuuming is ineffective, our results reinforce the importance of accounting for negative ecological impacts such as removal of natural enemies and pollinators (Chiasson et al., 1997) in the costs of tractor vacuuming, along with the economic costs of fuel, labor, maintenance ($1,351 per acre per year) and equipment ($12,937 per tractor per year) (Bolda et al., 2024). To put these costs in context, organic strawberry growers in this region earn an average of $8,555 in profit per acre per year, on $112,000 of revenue (Supplementary material).
5 Conclusion
Our results offer many considerations for pest management in strawberry production. First, habitat complexity in the farming landscape, which is out of most individual growers’ control, has a large influence on crop loss. In our study system, woody habitats and grasslands had opposite effects on crop loss. Second, local diversification, within the purview of some growers, may reduce crop loss, but this effect is highest near intermediate amounts of woody habitat, which are beneficial in their own right for maintaining a wide variety of critical ecosystem services. Third, on-farm pest management practices that are commonly used in this study system such as organic-compliant insecticides and tractor vacuuming may cause more harm than good, particularly over the long term, due to their negative impacts on natural enemies.
For regional land managers, our study provides evidence that preserving and promoting native woodlands, shrubs, and chaparral can be beneficial for natural pest control. Because they tend to be sources of pests, grasslands dominated by exotic annuals may be fertile ground for replacement with native flora.
As of 2016, organic certification in the United States charges growers with specific mandates to conserve and maintain natural resources, including biodiversity. The USDA National Organic Program sets rules for organic certification compliance and requires use of pest control methods that maintain or improve on-farm biodiversity to the greatest extent possible. Our results show that growers can adhere more closely to these rules by implementing pest control strategies that focus on building natural enemy populations rather than single-mindedly targeting pests. We hope that our results will advance advocacy for enhancing the U.S. Farm Bill’s conservation programs, including the Environmental Quality Incentives Program, The Regional Conservation and Partnership Program, and the Conservation Reserve Program. These programs help growers and regional land managers maintain and restore multi-scalar natural habitats and biodiversity that will bolster our efforts in creating a healthy, sustainable, and productive agricultural system.
Data availability statement
The raw data supporting the conclusions of this article will be made available by the authors, without undue reservation.
Ethics statement
The manuscript presents research on animals that do not require ethical approval for their study.
Author contributions
AL: Conceptualization, Data curation, Formal analysis, Funding acquisition, Investigation, Methodology, Supervision, Validation, Visualization, Writing – original draft. DG: Conceptualization, Data curation, Funding acquisition, Investigation, Methodology, Project administration, Supervision, Writing – review & editing. AS: Conceptualization, Data curation, Funding acquisition, Investigation, Methodology, Project administration, Supervision, Writing – review & editing. KG: Data curation, Investigation, Methodology, Visualization, Writing – review & editing. TC: Data curation, Investigation, Methodology, Writing – review & editing. GJ: Investigation, Writing – review & editing. CK: Conceptualization, Funding acquisition, Methodology, Project administration, Resources, Supervision, Writing – review & editing.
Funding
The author(s) declare that financial support was received for the research, authorship, and/or publication of this article. The United States Department of Agriculture (NIFA Project # 2015–67019-23147/ 1005662), the CS Fund, the Robert and Peggy van den Bosch Memorial Scholarship, the Berkeley Fellowship for Graduate Study, and the Margaret C. Walker Fund provided funding for this study.
Acknowledgments
Erin Berg, Maggie Deng, David Hung, Elizabeth Ordeman, Shuhan Song, and Candace Yee contributed their efforts toward categorizing, counting, and storing specimens. Sara Winsemius deserves special thanks for her multiple roles as lab manager, keeper of specimens, and invaluable field team member. We appreciate Michael Seagraves, Ryan Kennedy, and Diego Nieto at Driscoll’s in Watsonville, California for their help in contacting growers and providing comments on preliminary results. Finally, we express our sincere gratitude to all of the growers in the Santa Cruz -Watsonville-Salinas area who shared their expertise, allowed our field teams to collect data on their farms, and offered their time to coordinate our field visits. Their participation and dedication to science made this work possible.
Conflict of interest
The authors declare that the research was conducted in the absence of any commercial or financial relationships that could be construed as a potential conflict of interest.
The author(s) declared that they were an editorial board member of Frontiers, at the time of submission. This had no impact on the peer review process and the final decision.
Publisher’s note
All claims expressed in this article are solely those of the authors and do not necessarily represent those of their affiliated organizations, or those of the publisher, the editors and the reviewers. Any product that may be evaluated in this article, or claim that may be made by its manufacturer, is not guaranteed or endorsed by the publisher.
Supplementary material
The Supplementary material for this article can be found online at: https://www.frontiersin.org/articles/10.3389/fsufs.2024.1336888/full#supplementary-material
References
Albrecht, M., Kleijn, D., Williams, N. M., Tschumi, M., Blaauw, B. R., Bommarco, R., et al. (2020). The effectiveness of flower strips and hedgerows on pest control, pollination services and crop yield: a quantitative synthesis. Ecol. Lett. 23, 1488–1498. doi: 10.1111/ele.13576
Ali, M. P., Clemente-Orta, G., Kabir, M. M. M., Haque, S. S., Biswas, M., and Landis, D. A. (2023). Landscape structure influences natural pest suppression in a rice agroecosystem. Sci. Rep. 13:15726. doi: 10.1038/s41598-023-41786-y
Allen, W. W., and Douglas, M. B. (1974). Lygus bugs, Thrips, and Catfaced strawberries. Los Angeles, CA: California Strawberry Advisory Board.
Bartomeus, I., Vilà, M., and Santamaría, L. (2008). Contrasting effects of invasive plants in plant–pollinator networks. Oecologia 155, 761–770. doi: 10.1007/s00442-007-0946-1
Barton, K. (2024). MuMIn: Multi-Model Inference (Version 1.48.4) [Computer software]. Available at: https://cran.r-project.org/web/packages/MuMIn/index.html (Accessed October 3, 2024).
Batáry, P., Dicks, L. V., Kleijn, D., and Sutherland, W. J. (2015). The role of Agri-environment schemes in conservation and environmental management. Conserv. Biol. 29, 1006–1016. doi: 10.1111/cobi.12536
Bates, D., Maechler, M., Bolker, B., and Walker, S. (2015). Fitting linear mixed-effects models using {lme4}. J. Stat. Softw. 67:i01. doi: 10.18637/jss.v067.i01
Beaumelle, L., Auriol, A., Grasset, M., Pavy, A., Thiéry, D., and Rusch, A. (2021). Benefits of increased cover crop diversity for predators and biological pest control depend on the landscape context. Ecol. Solut. Evid. 2:e12086. doi: 10.1002/2688-8319.12086
Beillouin, D., Ben-Ari, T., Malézieux, E., Seufert, V., and Makowski, D. (2021). Positive but variable effects of crop diversification on biodiversity and ecosystem services. Glob. Chang. Biol. 27, 4697–4710. doi: 10.1111/gcb.15747
Boetzl, F. A., Schuele, M., Krauss, J., and Steffan-Dewenter, I. (2020). Pest control potential of adjacent Agri-environment schemes varies with crop type and is shaped by landscape context and within-field position. J. Appl. Ecol. 57, 1482–1493. doi: 10.1111/1365-2664.13653
Bolda, M. (2022). 1974 strawberry news bulletin—Back to basics on Lygus. ANR Blogs: Starwberries and Caneberries. Available at: https://ucanr.edu/blogs/blogcore/postdetail.cfm?postnum=52126 (Accessed April 27, 2022).
Bolda, M., Dara, S., Daugovish, O., Koike, S., Ploeg, A., and Browne, G. (2019). UC IPM Pest management guidelines strawberry. Available at: https://www2.ipm.ucanr.edu/agriculture/strawberry/ (Accessed October 3, 2024).
Bolda, M., Tourte, L., Murdock, J., and Goodrich, B. (2024). 2024 sample costs to produce and harvest organic strawberries—Fresh market – Central Coast Region, Santa Cruz, Monterey, and San Benito Counties. University of California Agriculture and Natural Resources Cooperative Extension.
Brooks, M. E., Kristensen, K., Benthem, K. J., Magnusson, A., Berg, C. W., and Nielsen, A. (2017). glmmTMB balances speed and flexibility among packages for zero-inflated generalized linear mixed modeling. R J. 9, 378–400. doi: 10.32614/RJ-2017-066
Bruckman, D., and Campbell, D. R. (2016). Pollination of a native plant changes with distance and density of invasive plants in a simulated biological invasion. Am. J. Bot. 103, 1458–1465. doi: 10.3732/ajb.1600153
Chaplin-Kramer, R., Kliebenstein, D. J., Chiem, A., Morrill, E., Mills, N. J., and Kremen, C. (2011a). Chemically mediated tritrophic interactions: opposing effects of glucosinolates on a specialist herbivore and its predators. J. Appl. Ecol. 48, 880–887. doi: 10.1111/j.1365-2664.2011.01990.x
Chaplin-Kramer, R., O’Rourke, M. E., Blitzer, E. J., and Kremen, C. (2011b). A meta-analysis of crop pest and natural enemy response to landscape complexity. Ecol. Lett. 14, 922–932. doi: 10.1111/j.1461-0248.2011.01642.x
Chiasson, H., Vincent, C., and Oliveira, D. (1997). Effect of an insect vacuum device on strawberry pollinators. Acta Hortic. 437, 373–378. doi: 10.17660/ActaHortic.1997.437.47
Costamagna, A. C., Venables, W. N., and Schellhorn, N. A. (2015). Landscape-scale pest suppression is mediated by timing of predator arrival. Ecol. Appl. 25, 1114–1130. doi: 10.1890/14-1008.1
Dainese, M., Martin, E. A., Aizen, M. A., Albrecht, M., Bartomeus, I., Bommarco, R., et al. (2019). A global synthesis reveals biodiversity-mediated benefits for crop production. Science. Advances 5:eaax0121. doi: 10.1126/sciadv.aax0121
Dainese, M., Montecchiari, S., Sitzia, T., Sigura, M., and Marini, L. (2017). High cover of hedgerows in the landscape supports multiple ecosystem services in Mediterranean cereal fields. J. Appl. Ecol. 54, 380–388. doi: 10.1111/1365-2664.12747
Dassou, A. G., and Tixier, P. (2016). Response of pest control by generalist predators to local-scale plant diversity: a meta-analysis. Ecol. Evol. 6, 1143–1153. doi: 10.1002/ece3.1917
De Bortoli, S. A., Vacari, A. M., Polanczyk, R. A., Veiga, A. C. P., and Goulart, R. M. (2017). “Effect of Bacillus thuringiensis on parasitoids and predators” in Bacillus thuringiensis and Lysinibacillus sphaericus: Characterization and use in the field of biocontrol. eds. L. M. Fiuza, R. A. Polanczyk, and N. Crickmore (Cham: Springer International Publishing), 67–77.
Desneux, N., Decourtye, A., and Delpuech, J.-M. (2007). The sublethal effects of pesticides on beneficial arthropods. Annu. Rev. Entomol. 52, 81–106. doi: 10.1146/annurev.ento.52.110405.091440
Dietzsch, A. C., Stanley, D. A., and Stout, J. C. (2011). Relative abundance of an invasive alien plant affects native pollination processes. Oecologia 167, 469–479. doi: 10.1007/s00442-011-1987-z
Duarte, G. T., Santos, P. M., Cornelissen, T. G., Ribeiro, M. C., and Paglia, A. P. (2018). The effects of landscape patterns on ecosystem services: Meta-analyses of landscape services. Landsc. Ecol. 33, 1247–1257. doi: 10.1007/s10980-018-0673-5
Earth Resources Observation and Science (EROS) Center (2017). National Agriculture Imagery Program (NAIP). New York, NY: U.S. Geological Survey.
Estrada-Carmona, N., Sánchez, A. C., Remans, R., and Jones, S. K. (2022). Complex agricultural landscapes host more biodiversity than simple ones: a global meta-analysis. Proc. Natl. Acad. Sci. 119:e2203385119. doi: 10.1073/pnas.2203385119
Eubanks, M. D. (2001). Estimates of the direct and indirect effects of red imported fire ants on biological control in field crops. Biol. Control 21, 35–43. doi: 10.1006/bcon.2001.0923
Fye, R. E. (1982). Weed hosts of the Lygus (Heteroptera: Miridae) bug complex in Central Washington. J. Econ. Entomol. 75, 724–727. doi: 10.1093/jee/75.4.724
Gagic, V., Holding, M., Venables, W. N., Hulthen, A. D., and Schellhorn, N. A. (2021). Better outcomes for pest pressure, insecticide use, and yield in less intensive agricultural landscapes. Proc. Natl. Acad. Sci. 118:e2018100118. doi: 10.1073/pnas.2018100118
Garcia, K., Olimpi, E. M., M’Gonigle, L., Karp, D. S., Wilson-Rankin, E. E., Kremen, C., et al. (2023). Semi-natural habitats on organic strawberry farms and in surrounding landscapes promote bird biodiversity and pest control potential. Agric. Ecosyst. Environ. 347:108353. doi: 10.1016/j.agee.2023.108353
Gardiner, M. M., Landis, D. A., Gratton, C., DiFonzo, C. D., O’Neal, M., Chacon, J. M., et al. (2009). Landscape diversity enhances biological control of an introduced crop pest in the north-Central USA. Ecol. Appl. 19, 143–154. doi: 10.1890/07-1265.1
Garibaldi, L. A., Oddi, F. J., Miguez, F. E., Bartomeus, I., Orr, M. C., Jobbágy, E. G., et al. (2021). Working landscapes need at least 20% native habitat. Conserv. Lett. 14:e12773. doi: 10.1111/conl.12773
Gurr, G. M., Landis, D. A., and You, M. (2017). Review of habitat management to suppress Pest populations: Progress and prospects. Ann. Rev. Entomol. 62, 91–109. doi: 10.1146/annurev-ento-031616-035050
Guthman, J. (2000). Raising organic: an agro-ecological assessment of grower practices in California. Agric. Hum. Values 17, 257–266. doi: 10.1023/A:1007688216321
Haan, N. L., Zhang, Y., and Landis, D. A. (2020). Predicting landscape configuration effects on agricultural Pest suppression. Trends Ecol. Evol. 35, 175–186. doi: 10.1016/j.tree.2019.10.003
Hagler, J. R. (2011). An immunological approach to quantify consumption of protein-tagged Lygus hesperus by the entire cotton predator assemblage. Biol. Control 58, 337–345. doi: 10.1016/j.biocontrol.2011.05.015
Hagler, J. R., Casey, M. T., Hull, A. M., and Machtley, S. A. (2020a). A molecular approach for detecting stage-specific predation on Lygus hesperus (Hemiptera: Miridae). J. Insect. Sci. 20:Article 35. doi: 10.1093/jisesa/ieaa136
Hagler, J. R., Nieto, D. J., Machtley, S. A., and Swezey, S. L. (2020b). Predator demographics and dispersal in alfalfa trap-cropped strawberry. Entomologia Experimentalis et Applicata 168:Article 1. doi: 10.1111/eea.12864
Hardin, J. W., and Hilbe, J. M. (2007). Generalized linear models and extensions. 2nd Edn. College Station, TX: Stata Press.
Hartig, F., and Lohse, L. (2022). DHARMa: Residual Diagnostics for Hierarchical (Multi-Level / Mixed) Regression Models (Version 0.4.6) [Computer software]. Available at: https://cran.r-project.org/web/packages/DHARMa/index.html (Accessed October 3, 2024).
Hole, D. G., Perkins, A. J., Wilson, J. D., Alexander, I. H., Grice, P. V., and Evans, A. D. (2005). Does organic farming benefit biodiversity? Biol. Conserv. 122, 113–130. doi: 10.1016/j.biocon.2004.07.018
Iverson, A. L., Marín, L. E., Ennis, K. K., Gonthier, D. J., Connor-Barrie, B. T., Remfert, J. L., et al. (2014). REVIEW: do polycultures promote win-wins or trade-offs in agricultural ecosystem services? A meta-analysis. J. Appl. Ecol. 51, 1593–1602. doi: 10.1111/1365-2664.12334
Jackson, H. B., and Fahrig, L. (2012). What size is a biologically relevant landscape? Landsc. Ecol. 27, 929–941. doi: 10.1007/s10980-012-9757-9
Jackson, H. B., and Fahrig, L. (2015). Are ecologists conducting research at the optimal scale? Glob. Ecol. Biogeogr. 24, 52–63. doi: 10.1111/geb.12233
Jonsson, M., Straub, C. S., Didham, R. K., Buckley, H. L., Case, B. S., Hale, R. J., et al. (2015). Experimental evidence that the effectiveness of conservation biological control depends on landscape complexity. J. Appl. Ecol. 52:5. doi: 10.1111/1365-2664.12489
Joseph, S. V., and Bolda, M. (2018). Evaluating the potential utility of an electrostatic sprayer and a tractor-mounted vacuum machine for Lygus hesperus (Hemiptera: Miridae) management in California’s coastal strawberry. Crop Prot. 113, 104–111. doi: 10.1016/j.cropro.2018.08.005
Judt, C., Korányi, D., Zaller, J. G., and Batáry, P. (2023). Floral resources and ground covers promote natural enemies but not pest insects in apple orchards: a global meta-analysis. Sci. Total Environ. 903:166139. doi: 10.1016/j.scitotenv.2023.166139
Karp, D. S., Chaplin-Kramer, R., Meehan, T. D., Martin, E. A., DeClerck, F., Grab, H., et al. (2018). Crop pests and predators exhibit inconsistent responses to surrounding landscape composition. Proc. Natl. Acad. Sci. 115, E7863–E7870. doi: 10.1073/pnas.1800042115
Karp, D. S., Gennet, S., Kilonzo, C., Partyka, M., Chaumont, N., Atwill, E. R., et al. (2015). Comanaging fresh produce for nature conservation and food safety. Proc. Natl. Acad. Sci. 112, 11126–11131. doi: 10.1073/pnas.1508435112
Karp, D. S., Moses, R., Gennet, S., Jones, M. S., Joseph, S., M’Gonigle, L. K., et al. (2016). Agricultural practices for food safety threaten pest control services for fresh produce. J. Appl. Ecol. 53, 1402–1412. doi: 10.1111/1365-2664.12707
King, V. M., and Sargent, R. D. (2012). Presence of an invasive plant species alters pollinator visitation to a native. Biol. Invasions 14, 1809–1818. doi: 10.1007/s10530-012-0191-3
Kleijn, D., Bommarco, R., Fijen, T. P. M., Garibaldi, L. A., Potts, S. G., and Putten, W. H. (2019). Ecological intensification: bridging the gap between science and practice. Trends Ecol. Evol. 34, 154–166. doi: 10.1016/j.tree.2018.11.002
Klein, A.-M., Brittain, C., Hendrix, S. D., Thorp, R., Williams, N., and Kremen, C. (2012). Wild pollination services to California almond rely on semi-natural habitat. J. Appl. Ecol. 49, 723–732. doi: 10.1111/j.1365-2664.2012.02144.x
Kremen, C., and Miles, A. (2012). Ecosystem services in biologically diversified versus conventional farming systems: benefits, externalities, and trade-offs. Ecol. Soc. 17:4. doi: 10.5751/ES-05035-170440
Kremen, C., Williams, N. M., Aizen, M. A., Gemmill-Herren, B., LeBuhn, G., Minckley, R., et al. (2007). Pollination and other ecosystem services produced by mobile organisms: a conceptual framework for the effects of land-use change. Ecol. Lett. 10, 299–314. doi: 10.1111/j.1461-0248.2007.01018.x
Kremen, C., Williams, N. M., Bugg, R. L., Fay, J. P., and Thorp, R. W. (2004). The area requirements of an ecosystem service: crop pollination by native bee communities in California. Ecol. Lett. 7, 1109–1119. doi: 10.1111/j.1461-0248.2004.00662.x
Le Gal, A., Robert, C., Accatino, F., Claessen, D., and Lecomte, J. (2020). Modelling the interactions between landscape structure and spatio-temporal dynamics of pest natural enemies: implications for conservation biological control. Ecol. Model. 420:108912. doi: 10.1016/j.ecolmodel.2019.108912
Lefcheck, J. S. (2016). piecewiseSEM: piecewise structural equation modelling in R for ecology, evolution, and systematics. Methods Ecol. Evol. 7, 573–579. doi: 10.1111/2041-210X.12512
Lefcheck, J., Byrnes, J., and Grace, J. (2023). Piecewise SEM: piecewise structural equation modeling (version 2.3.0) [computer software]. Available at: https://cran.r-project.org/web/packages/piecewiseSEM/ (Accessed October 3, 2024).
Leigh, T. F., and Gonzalez, D. (1976). Field cage evaluation of predators for control of Lygus hesperus knight on cotton. Environ. Entomol. 5:Article 5. doi: 10.1093/ee/5.5.948
Leong, M., Ponisio, L. C., Kremen, C., Thorp, R. W., and Roderick, G. K. (2016). Temporal dynamics influenced by global change: bee community phenology in urban, agricultural, and natural landscapes. Glob. Chang. Biol. 22, 1046–1053. doi: 10.1111/gcb.13141
Letourneau, D. K., Armbrecht, I., Rivera, B. S., Lerma, J. M., Carmona, E. J., Daza, M. C., et al. (2011). Does plant diversity benefit agroecosystems? A synthetic review. Ecol. Appl. 21, 9–21. doi: 10.1890/09-2026.1
Liere, H., Kim, T. N., Werling, B. P., Meehan, T. D., Landis, D. A., and Gratton, C. (2015). Trophic cascades in agricultural landscapes: indirect effects of landscape composition on crop yield. Ecol. Appl. 25, 652–661. doi: 10.1890/14-0570.1
Lu, A., Gonthier, D. J., Sciligo, A. R., Garcia, K., Chiba, T., Juárez, G., et al. (2022). Changes in arthropod communities mediate the effects of landscape composition and farm management on pest control ecosystem services in organically managed strawberry crops. J. Appl. Ecol. 59, 585–597. doi: 10.1111/1365-2664.14076
Lundgren, J. G., McDonald, T., Rand, T. A., and Fausti, S. W. (2015). Spatial and numerical relationships of arthropod communities associated with key pests of maize. J. Appl. Entomol. 139, 446–456. doi: 10.1111/jen.12215
Lundin, O., Ward, K. L., and Williams, N. M. (2019). Identifying native plants for coordinated habitat management of arthropod pollinators, herbivores and natural enemies. J. Appl. Ecol. 56, 665–676. doi: 10.1111/1365-2664.13304
Malcolm, D. R. (1953). Host relationship studies of Lygus in south Central Washington. J. Econ. Entomol. 46, 485–488. doi: 10.1093/jee/46.3.485
Martin, E. A., Dainese, M., Clough, Y., Báldi, A., Bommarco, R., Gagic, V., et al. (2019). The interplay of landscape composition and configuration: new pathways to manage functional biodiversity and agroecosystem services across Europe. Ecol. Lett. 22, 1083–1094. doi: 10.1111/ele.13265
Martin, E. A., Seo, B., Park, C.-R., Reineking, B., and Steffan-Dewenter, I. (2016). Scale-dependent effects of landscape composition and configuration on natural enemy diversity, crop herbivory, and yields. Ecol. Appl. 26, 448–462. doi: 10.1890/15-0856
Midega, C. A. O., Jonsson, M., Khan, Z. R., and Ekbom, B. (2014). Effects of landscape complexity and habitat management on stemborer colonization, parasitism and damage to maize. Agric. Ecosyst. Environ. 188, 289–293. doi: 10.1016/j.agee.2014.02.028
Mohamed, A., DeClerck, F., Verburg, P. H., Obura, D., Abrams, J. F., Zafra-Calvo, N., et al. (2024). Securing nature’s contributions to people requires at least 20–25% (semi-)natural habitat in human-modified landscapes. One Earth 7, 59–71. doi: 10.1016/j.oneear.2023.12.008
Morandin, L. A., Long, R. F., and Kremen, C. (2014). Hedgerows enhance beneficial insects on adjacent tomato fields in an intensive agricultural landscape. Agric. Ecosyst. Environ. 189, 164–170. doi: 10.1016/j.agee.2014.03.030
Nakagawa, S., and Schielzeth, H. (2013). A general and simple method for obtaining R2 from generalized linear mixed-effects models. Methods Ecol. Evol. 133–142. doi: 10.1111/j.2041-210x.2012.00261.x
National Organic Program . (2000). Pub. L. No. 7 C.F.R. Part 205. Available at: https://www.ecfr.gov/current/title-7/part-205 (Accessed October 3, 2024).
Nieto, D. J., Hagler, J. R., Swezey, S. L., Machtley, S. A., and Bryer, J. A. (2023). Immigration of Lygus spp. (Hemiptera: Miridae) and predaceous natural enemies to trap-cropped organic strawberry. Environ. Entomol. 52, 824–831. doi: 10.1093/ee/nvad085 (Accessed May 20, 2024)
NOAA . (2024a). NOAA NCEI U.S. climate Normals Quick Access. Available at: https://www.ncei.noaa.gov/access/us-climate-normals/#dataset=normals-annualseasonal&timeframe=30&station=USW00023233 (Accessed March 14, 2024).
NOAA . (2024b). Normals Annual/Seasonal Station Details: SALINAS AIRPORT, CA US, GHCND:USW00023233 | Climate Data Online (CDO) | National Climatic Data Center (NCDC). Available at: https://www.ncdc.noaa.gov/cdo-web/datasets/NORMAL_ANN/stations/GHCND:USW00023233/detail (Accessed May 30, 2024).
Paradis, E., and Schliep, K. (2019). Ape 5.0: an environment for modern phylogenetics and evolutionary analyses in R. Bioinformatics 35, 526–528. doi: 10.1093/bioinformatics/bty633
Perkins, P. V., and Watson, T. F. (1972). Nabis alternatus as a Predator of Lygus hesperus. Ann. Entomol. Soc. Am. 65:Article 3. doi: 10.1093/aesa/65.3.625
Pickel, C., Zalom, F. G., Walsh, D. B., and Welch, N. C. (1994). Efficacy of vacuum machines for Lygus hesperus (Hemiptera: Miridae) control in coastal California strawberries. J. Econ. Entomol. 87, 1636–1640. doi: 10.1093/jee/87.6.1636
Pickel, C., Zalom, F. G., Walsh, D. B., and Welch, N. C. (1995). Vacuums provide limited Lygus control in strawberries. Calif. Agric. 49, 19–22. doi: 10.3733/ca.v049n02p19
R Core Team (2019). R: A language and environment for statistical computing [computer software]. Vienna: R Foundation for Statistical Computing.
Rancourt, B., Vincent, C., and De Oliveira, D. (2003). Field evaluation of efficacy and persistence of an insect vacuum device against the tarnished plant bug (Hemiptera: Miridae) in a day-neutral strawberry field. J. Econ. Entomol. 96, 401–406. doi: 10.1093/jee/96.2.401
Rasmussen, L. V., Grass, I., Mehrabi, Z., Smith, O. M., Bezner-Kerr, R., Blesh, J., et al. (2024). Joint environmental and social benefits from diversified agriculture. Science 384, 87–93. doi: 10.1126/science.adj1914
Root, R. B. (1973). Organization of a plant-arthropod association in simple and diverse habitats: the fauna of collards (Brassica Oleracea). Ecol. Monogr. 43, 95–124. doi: 10.2307/1942161
Roubos, C. R., Rodriguez-Saona, C., and Isaacs, R. (2014). Mitigating the effects of insecticides on arthropod biological control at field and landscape scales. Biol. Control 75, 28–38. doi: 10.1016/j.biocontrol.2014.01.006
Rusch, A., Chaplin-Kramer, R., Gardiner, M. M., Hawro, V., Holland, J., Landis, D., et al. (2016). Agricultural landscape simplification reduces natural pest control: a quantitative synthesis. Agric. Ecosyst. Environ. 221, 198–204. doi: 10.1016/j.agee.2016.01.039
Sánchez, A. C., Jones, S. K., Purvis, A., Estrada-Carmona, N., and De Palma, A. (2022). Landscape complexity and functional groups moderate the effect of diversified farming on biodiversity: a global meta-analysis. Agric. Ecosyst. Environ. 332:107933. doi: 10.1016/j.agee.2022.107933
Saqib, H. S. A., Chen, J., Chen, W., Pozsgai, G., Akutse, K. S., Ashraf, M. F., et al. (2020). Local management and landscape structure determine the assemblage patterns of spiders in vegetable fields. Sci. Rep. 10:15130. doi: 10.1038/s41598-020-71888-w
Schellhorn, N. A., Parry, H. R., Macfadyen, S., Wang, Y., and Zalucki, M. P. (2015). Connecting scales: achieving in-field pest control from area wide and landscape ecology studies. Insect Sci. 22, 35–51. doi: 10.1111/1744-7917.12161
Sciligo, A. R., M’Gonigle, L. K., and Kremen, C. (2022). Local diversification enhances pollinator visitation to strawberry and may improve pollination and marketability. Front. Sust. Food Syst. 6:941840. doi: 10.3389/fsufs.2022.941840
Scott, D. R. (1977). An annotated listing of host plants of Lygus hesperus knight. Bullet. Entomol. Soc. Am. 23, 19–22. doi: 10.1093/besa/23.1.19
Shipley, B. (2000). A new inferential test for path models based on directed acyclic graphs. Struct. Equ. Model. Multidiscip. J. 7, 206–218. doi: 10.1207/S15328007SEM0702_4
Shipley, B. (2009). Confirmatory path analysis in a generalized multilevel context. Ecology 90, 363–368. doi: 10.1890/08-1034.1
Shipley, B. (2013). The AIC model selection method applied to path analytic models compared using a d-separation test. Ecology 94, 560–564. doi: 10.1890/12-0976.1
Sirami, C., Gross, N., Baillod, A. B., Bertrand, C., Carrié, R., Hass, A., et al. (2019). Increasing crop heterogeneity enhances multitrophic diversity across agricultural regions. Proc. Natl. Acad. Sci. 116, 16442–16447. doi: 10.1073/pnas.1906419116
Stark, J. D., Banks, J. E., and Acheampong, S. (2004). Estimating susceptibility of biological control agents to pesticides: influence of life history strategies and population structure. Biol. Control 29, 392–398. doi: 10.1016/j.biocontrol.2003.07.003
Strzyzewski, I. L., Funderburk, J. E., Renkema, J. M., and Smith, H. A. (2021). Characterization of Frankliniella occidentalis and Frankliniella bispinosa (Thysanoptera: Thripidae) injury to strawberry. J. Econ. Entomol. 114, 794–800. doi: 10.1093/jee/toaa311
Swezey, S. L., Nieto, D. J., and Bryer, J. A. (2007). Control of western tarnished plant bug Lygus hesperus knight (Hemiptera: Miridae) in California organic strawberries using alfalfa trap crops and tractor-mounted vacuums. Environ. Entomol. 36, 1457–1465. doi: 10.1603/0046-225X(2007)36[1457:COWTPB]2.0.CO;2
Symonds, M. R., and Moussalli, A. (2011). A brief guide to model selection, multimodel inference and model averaging in behavioural ecology using Akaike’s information criterion. Behav. Ecol. Sociobiol. 65, 13–21. doi: 10.1007/s00265-010-1037-6
Talton, H. R., Rhodes, E. M., Chase, C. A., Swisher, M. E., Renkema, J. M., and Liburd, O. E. (2020). Effect of cultural practices on Neopamera bilobata in relation to fruit injury and marketable yields in organic strawberries. Insects 11:843. doi: 10.3390/insects11120843
Tamburini, G., Bommarco, R., Wanger, T. C., Kremen, C., van der Heijden, M. G. A., Liebman, M., et al. (2020). Agricultural diversification promotes multiple ecosystem services without compromising yield. Sci. Adv. 6:eaba1715. doi: 10.1126/sciadv.aba1715
Thiele, J., Kellner, S., Buchholz, S., and Schirmel, J. (2018). Connectivity or area: what drives plant species richness in habitat corridors? Landsc. Ecol. 33, 173–181. doi: 10.1007/s10980-017-0606-8
Tscharntke, T., Grass, I., Wanger, T. C., Westphal, C., and Batáry, P. (2021). Beyond organic farming – harnessing biodiversity-friendly landscapes. Trends Ecol. Evol. 36, 919–930. doi: 10.1016/j.tree.2021.06.010
Tscharntke, T., Karp, D. S., Chaplin-Kramer, R., Batáry, P., DeClerck, F., Gratton, C., et al. (2016). When natural habitat fails to enhance biological pest control – five hypotheses. Biol. Conserv. 204, 449–458. doi: 10.1016/j.biocon.2016.10.001
Tscharntke, T., Tylianakis, J. M., Rand, T. A., Didham, R. K., Fahrig, L., Batáry, P., et al. (2012). Landscape moderation of biodiversity patterns and processes—eight hypotheses. Biol. Rev. 87, 661–685. doi: 10.1111/j.1469-185X.2011.00216.x
United States Geological Survey . (2007). US Rain/Dry Days Readme | Early Warning and Environmental Monitoring Program. Available at: https://earlywarning.usgs.gov/usraindry/rdreadme.php (Accessed October 03, 2024).
USDA . (2024a). NOP 5020 Natural Resources and Biodiversity Conservation. Available at: https://www.ams.usda.gov/sites/default/files/media/NOP%205020%20Biodiversity%20Guidance%20Rev01%20%28Final%29.pdf (Accessed April 7, 2024).
USDA . (2024b). NOP Handbook | Agricultural Marketing Service. Available at: https://www.ams.usda.gov/rules-regulations/organic/handbook (Accessed April 7, 2024).
USDA . (2024c). The National List | Agricultural Marketing Service. Available at: https://www.ams.usda.gov/rules-regulations/organic/national-list (Accessed March 14, 2024).
Vincent, C., and Lachance, P. (1993). Evaluation of a tractor-propelled vacuum device for Management of Tarnished Plant bug (Heteroptera: Miridae) populations in strawberry plantations. Environ. Entomol. 22, 1103–1107. doi: 10.1093/ee/22.5.1103
Wells, J., Fink, C., Edsall, M., Olivier, D., and Lin, J. (2020). Prototype Lygus Spp. vacuum provides improved pest management in California strawberries. Int. J. Fruit Sci. 20, 1019–1028. doi: 10.1080/15538362.2020.1820261
Wietzke, A., Westphal, C., Gras, P., Kraft, M., Pfohl, K., Karlovsky, P., et al. (2018). Insect pollination as a key factor for strawberry physiology and marketable fruit quality. Agric. Ecosyst. Environ. 258, 197–204. doi: 10.1016/j.agee.2018.01.036
Wilson, L., and Gutierrez, A. (1980). Within-plant distribution of predators on cotton: Comments on sampling and predator efficiencies. Hilgardia 48:Article 2.
Wilson, H., Miles, A. F., Daane, K. M., and Altieri, M. A. (2017). Landscape diversity and crop vigor outweigh influence of local diversification on biological control of a vineyard pest. Ecosphere 8:1736. doi: 10.1002/ecs2.1736
Keywords: habitat complexity, landscape composition, local diversification practices, crop diversity, pest management, pest control ecosystem services, crop loss, diversified agriculture
Citation: Lu A, Gonthier D, Sciligo A, Garcia K, Chiba T, Juárez G and Kremen C (2024) Arthropod arbiters: natural enemy communities mediate the effects of landscape and local-scale complexity on Lygus-induced crop loss in organic strawberries. Front. Sustain. Food Syst. 8:1336888. doi: 10.3389/fsufs.2024.1336888
Edited by:
Johann G. Zaller, University of Natural Resources and Life Sciences, Vienna, AustriaReviewed by:
Gemma Clemente-Orta, Universitat de Lleida, SpainPedro Togni, University of Brasilia, Brazil
Copyright © 2024 Lu, Gonthier, Sciligo, Garcia, Chiba, Juárez and Kremen. This is an open-access article distributed under the terms of the Creative Commons Attribution License (CC BY). The use, distribution or reproduction in other forums is permitted, provided the original author(s) and the copyright owner(s) are credited and that the original publication in this journal is cited, in accordance with accepted academic practice. No use, distribution or reproduction is permitted which does not comply with these terms.
*Correspondence: Adrian Lu, adhlu@berkeley.edu