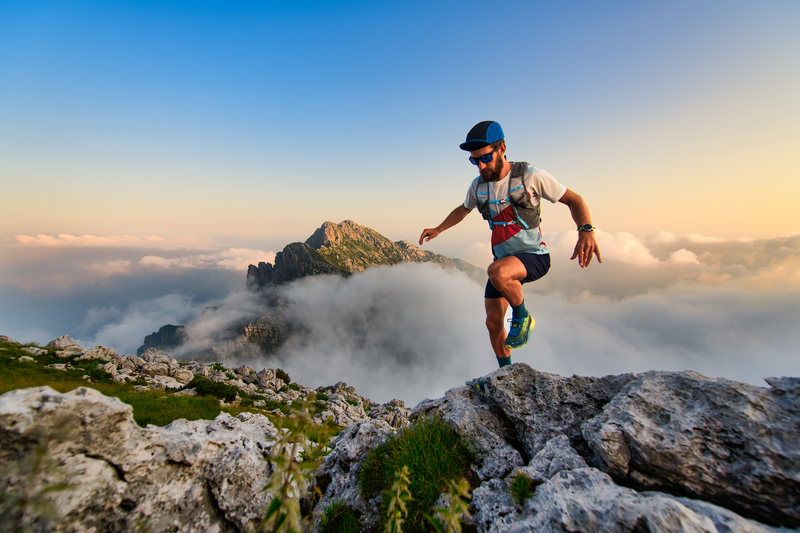
94% of researchers rate our articles as excellent or good
Learn more about the work of our research integrity team to safeguard the quality of each article we publish.
Find out more
REVIEW article
Front. Sustain. Food Syst. , 23 February 2024
Sec. Land, Livelihoods and Food Security
Volume 8 - 2024 | https://doi.org/10.3389/fsufs.2024.1331251
This article is part of the Research Topic Microalgae as Sustainable Food Resources: Prospects, Novel Species, Bioactive Compounds, Cultivation Process and Food Processing View all 7 articles
Microalgae are emerging as a sustainable source of bioproducts, including food, animal feed, nutraceuticals, and biofuels. This review emphasizes the need to carefully select suitable species and highlights the importance of strain optimization to enhance the feasibility of developing algae as a sustainable resource for food and biomaterial production. It discusses microalgal bioprospecting methods, different types of cultivation systems, microalgal biomass yields, and cultivation using wastewater. The paper highlights advances in artificial intelligence that can optimize algal productivity and overcome the limitations faced in current microalgal industries. Additionally, the potential of UV mutagenesis combined with high-throughput screening is examined as a strategy for generating improved strains without introducing foreign genetic material. The necessity of a multifaceted optimization approach for enhanced productivity is acknowledged. This review provides an overview of recent developments crucial for the commercial success of microalgal production.
Finding alternative sources to fulfill food and fuel security needs is imperative, especially in impoverished regions or those heavily relying on imports. For example, Middle Eastern and North African countries, dependent on food imports, were significantly affected during the COVID-19 pandemic. These regions are also predicted to face accelerated hardships, especially in regard to their food and fuel supplies, due to global conflicts (Ben Hassen and El Bilali, 2022). In a broader sense, alternative food and fuel sources are needed for the ever-growing human population, either to supplant or complement current resources, to prevent resource scarcity in the future.
Microalgae and their high-value bioproducts have the potential to address food and fuel security issues due to their advantages in commercial production. Microalgae are unicellular photosynthetic microbes that have been widely studied and used for the sustainable production of vitamins and carotenoids (Abo et al., 2019; Fu et al., 2019). They can grow photoautotrophically using carbon dioxide and minimal salts media or heterotrophically using a wide range of organic compounds as an energy source (Carino and Vital, 2022). Their faster growth rate, higher biomass productivity, lack of competition with crops for arable land and freshwater (Diaz et al., 2023), and capacity for sustainable growth using marginal resources make microalgae more favorable organisms than higher plants for mass production where land and water are limiting resources (Abo et al., 2019; Fu et al., 2019).
Microalgae produce up to 31 times higher lipid yield per hectare in comparison to other oil-producing crops (Udayan et al., 2022). They have a suitable size for ingestion, and their cell walls are highly digestible (Abo et al., 2019). These organisms also produce nutrients found in higher plants, such as proteins, nucleic acids, carbohydrates, fibers, starches, vitamins, antioxidants, and polyunsaturated fatty acids (Jareonsin and Pumas, 2021). Some microalgae species are promising for biodiesel production due to the high lipid content of 50–80% of their biomass (Khan et al., 2018). This sustainable energy source is eco-friendly, non-toxic, and presents a strong potential for CO2 reduction. Utilizing microalgae biomass to manufacture various biofuels adds new inputs to the CO2 sequestration process, which helps maintain a stable atmospheric CO2 level and a clean, safe environment (Jaiswal et al., 2022). Many countries across Asia, Europe, and America have started the industrialization of bioenergy derived from microalgae biomass (Khan et al., 2018). For instance, in 2019, America produced 1,551.1 peta joules (PJ) of biofuel, followed by Brazil with 992.2 PJ and Europe with 403.3 PJ (Khoo et al., 2023). The global market for microalgae-based products is estimated to increase from $32.60 billion in 2017 to $53.43 billion in 2026 (Rahman, 2020; Rafa et al., 2021). Governments around the globe aim to integrate biofuels into their transport fuel sectors, with the estimation that biofuels will constitute 20% of liquid fuels by 2050 (Khoo et al., 2023). However, despite these initiatives and clear advantages over land crops, the current state of the microalgal industry is insufficient to address wider issues in food and fuel security.
Advancements in microalgal technology focus on reducing production costs (Xie et al., 2022), as the processing stages of cultivation and harvesting are currently too costly to produce adequate profit margins (Khoo et al., 2023). Such technologies can be biological, for example, by the overexpression of carbon anhydrase (CA) to improve microalgae photosynthesis efficiency or through gene manipulation of fatty acid acyl thioesterase and acyl-CoA synthase (ACS) to produce extracellular fatty acids; additional approaches include producing fatty acid ethyl esters (biodiesel) through pyruvate decarboxylase, alcohol dehydrogenase and wax ester synthase, or through stearoyl-ACPΔ9 saturase, thioesterase, ACS and desaturase to produce high-quality biodiesel (Xie et al., 2022). These modifications ensure that growth and lipid productivity in the chosen microalgal strains are simultaneously optimized (Khoo et al., 2023). Subjecting microalgae to high salinity and nitrogen deprivation leads to overproducing lipids due to the algae's attempt to establish an equilibrium within the system (Russell et al., 2022). Nutrient starvation is a notable strategy to amplify lipid content in microalgae; although this often compromises biomass yield (Kim et al., 2023), new approaches for strain optimization can overcome this hurdle. Wider dissemination of targeted information, such as presented in this review, can assist strain selection and optimization efforts that can in turn alleviate high costs associated with genetic alterations limits imposed by proprietary technologies (Varela Villarreal et al., 2020). Inducing mutagenesis by physical methods such as UV rays has been an efficient technique for strain improvement and increased lipids in microalgae (Sivaramakrishnan and Incharoensakdi, 2023). Since this method is scalable and inexpensive, it may be the best strain optimization technique for broad application to improve microalgae production strains.
This review outlines several important steps to improve microalgal productivity to fulfill food and fuel security needs at a larger scale. It describes important steps for generating production-level strains, including bioprospecting and strain optimization. Specifically, the application of UV mutagenesis combined with a precise selection technique to successfully enhance microalgal lipids without affecting biomass productivity is highlighted.
Microalgal bioprospecting is an essential first step to working with a new biotechnology candidate strain. The inception involves collecting samples from diverse aquatic and terrestrial habitats. Freshwater, terrestrial, and marine environments, including soil (Leitner et al., 2022), lakes (Berges et al., 2021), rivers (Kumar et al., 2023), and coastal areas (Nelson et al., 2017), serve as rich sources for novel microalgal isolates; although, microalgae can be isolated from harsh environments including desert sand (Nelson et al., 2019), cryospheric (Nelson et al., 2013) or lithospheric (Häubner et al., 2006) surfaces, as shown in Table 1. In sample collection, exemplified in Figure 1, the documentation of each collection site's geographical coordinates, temperature, pH, and salinity provides essential context for downstream studies.
Figure 1. Field locations and laboratory methods for microalgal bioprospecting. Microalgae occur in a wide range of environments. Adaptations to these varying conditions can manifest the production of different metabolites suitable for different applications (top panel). After sampling, single colony (isogenic) and decontaminated (axenic) cultures can be obtained through several physical and chemical protocols. Once a suitable, isogenic, axenic strain has been obtained, downstream analyses and scale-up operations can be carried out (bottom panel). The search for algae that produce specific compounds of interest can be narrowed by considering that these compounds are generally environmentally-responsive. Abiotic or biotic factors can cajole an algal species into making commercially profitable bioproducts. For example, harmful algal blooms, which produce various toxins, can be a rich source of potent pharmaceuticals (Pradhan and Ki, 2022). Ultraviolet light also promotes the accumulation of some useful bioproducts. For example, the high-value pigment astaxanthin is more likely to accumulate in strains subjected to intense UV irradiation in their natural environment, and the production of astaxanthin increases with higher UV levels (Wu et al., 2010).
Algal lipids are highly diverse bioproducts whose accumulation and characteristics vary based on the strain's ancestry and environment. These differences in lipid types help maintain cell membrane stability under different conditions and represent a wide variety of bioprospecting opportunities. Species that can withstand cold temperatures, like those from polar, boreal, and alpine areas, tend to have abundant long-chain polyunsaturated acids. For example, the nutraceutical, very-long-chain fatty acids eicosapentaenoic acid (EPA, C20:5) and docosahexaenoic acid (DHA, C22:6) are more likely to be found in abundance in alpine (Nelson et al., 2013), polar, and cold temperate regions (Boelen et al., 2013). Species from warmer climates usually have shorter fatty acids. For example, we recently reported isolating high palmitic acid (C16:0) strains from desert regions. The green alga Chloroidium sp. was isolated from multiple locations in the United Arab Emirates (UAE) in a screen for lipid-producing algae (Sharma et al., 2015). We found that 41.8% of its total fatty acids consisted of palmitic acid (Nelson et al., 2017), a value close to the 44% palmitic acid found in the palm tree Elaeis guineensis (Mancini et al., 2015). The nearly equivalent lipid profiles in oil palm and the desert alga revealed the alga as a promising candidate for sustainable palm oil alternatives.
Microalgal strains are extracted from environmental samples using specialized microbiology techniques. Isolation techniques include direct plating, serial dilution, and single-cell isolation. Single-cell isolation can be done with microscopy, fluorescence-activated cell sorting (FACS), and generating aerosol clouds where individual droplets contain one cell of the target species (Nelson et al., 2013). In microscopy-based isolation, cells are observed under a microscope, and individual cells are picked using a micropipette (Lue et al., 2022). Sampled cells can be plated on solid media or inoculated into liquid media. These meticulous methods ensure the isolation of pure strains, removing possible xenic confounding factors from downstream projects. Several routes for the early culturing of isolates are available. Water or media-based samples are spread onto agar plates containing minimal salts medium (e.g., F/2; Lananan et al., 2013). Incubation conditions typically involve a 16:8 light-dark cycle at 25°C for several weeks until colonies emerge and can be picked for transfer.
Algal strains usually need to grow axenically for proper characterization and to make high-value products. Decontamination procedures include antibiotic treatments, repeated sub-culturing, and physical separation (Andersen, 2004). These methods ensure the purity of isolated strains for subsequent applications and research. To eliminate bacterial contaminants, cultures can be treated with antibiotics, commonly penicillin and streptomycin (50–100 μg/mL). This is most commonly done by adding antibiotics to solid media in agar-based Petri dishes, but antibiotics added to liquid media, such as in the serial dilution technique, are also effective. Monitoring bacterial growth is essential, and treatments may be repeated as necessary. The serial dilution technique is beneficial for samples with high microbial density or recalcitrance to solid media culture. Serial transfer involves transferring a portion of the culture to fresh media multiple times. It aids in gradually eliminating contaminants, ensuring a pure microalgal culture. Certain kinds of persistent contamination may require density gradient centrifugation (Okumura et al., 2015). Here, cells are separated from contaminants based on density, although maintaining cell viability is challenging in this technique.
Post-isolation, strains are identified and evaluated for commercial potential based on morphological characteristics using microscopy techniques (Deniset-Besseau et al., 2021; Sonmez et al., 2023). Identification through DNA barcoding, mainly 18S rRNA gene sequencing, is a precise and widely used method for strain determination (Darienko et al., 2015; Hadi et al., 2016; Zou et al., 2016). After identification, candidate bioactive compounds are extracted and subjected to various assays to ascertain their bioactivity (Uzlasir et al., 2023; Yang et al., 2023; Zhou et al., 2023). Recent bioprospecting endeavors have unveiled a plethora of microalgal strains with promising bioactivities. For instance, certain strains have demonstrated antioxidant (Yang et al., 2023), antimicrobial (Zhou et al., 2023), and anti-inflammatory properties (Leitner et al., 2022; Kurniawan et al., 2023; Manabe et al., 2023). Moreover, some strains are suited for biofuel production due to their high lipid content (Nelson et al., 2013, 2017; Deniset-Besseau et al., 2021). Microalgal bioprospecting stands at the forefront of discovering novel compounds with diverse applications. The techniques employed, from isolation to decontamination, play a pivotal role in ensuring the success of these endeavors. As research in this domain intensifies, refining these techniques and adopting innovative approaches will be instrumental in harnessing the full potential of microalgae.
Research areas for future studies on microalgal bioprospecting from diverse environments include increasing studies in Asia and Africa. For example, the exceptionally high marine biodiversity region of the Coral Triangle (CT; Tornabene et al., 2015; Williams et al., 2017; Ampou et al., 2018) consisting of the tropical waters by the Philippines, Malaysia, Papua New Guinea, Timor-Leste, the Solomon Islands, and Indonesia, would be a very rich source of algal isolates, as would each of the remote islands in that area of the Pacific Ocean. Intensifying bioprospecting efforts in less-studied regions will likely lead to valuable discoveries of new strains with commercial potential.
Most known algal isolates are held in culture centers, predominantly in the Americas and Europe. For example, the Culture Collection of Algae at the University of Texas (UTEX, https://utex.org/), the Scottish Association for Marine Science (SAMS, https://www.sams.ac.uk/), the Culture Collection of Cryogenic Algae (CCCryo, http://cccryo.fraunhofer.de/), and the Bigelow National Center for Marine Algae and Microbiota (NCMA, https://ncma.bigelow.org/) are a few prominent examples of large algal collections. Isolates from these collections are available with few restrictions to the greater scientific community and can be explored for their suitability for various applications.
Cultivating microalgae using various methods for commercial (Araújo et al., 2021) and economical high-value products requires mass cultivation, and it should be economically feasible (Shekh et al., 2022). It is estimated that algal biomass production costs about 11 USD per 1 kg in 1 acre of area, which is high as compared to biodiesel obtained from other plants (Khoo et al., 2023); therefore, it is desirable first to assess the suitability of a strain for mass cultivation in the laboratory setting.
Lab-scale culture systems that simulate light and temperature settings can test a strain's biomass productivity in outdoor ponds, speeding up strain characterization and lowering the costs, time, and effort involved in setting up and running ponds (Huesemann et al., 2017). Pond simulators such as the indoor climate simulation raceway developed by Pacific Northwest National Laboratory (PNNL), and the benchtop environmental photobioreactor (ePBR) manufactured by Phenometrics, Inc. (Lansing, MI, USA) are capable of mimicking the growth behavior of microalgae cultured in outdoor ponds to faithfully reproduce the microalgal growth performance of outdoor cultures exposed to varying levels of light intensity and water temperature (Huesemann et al., 2017). A study compared the biomass productivities of Chlorella sorokiniana DOE 1412 in PNNL climate-simulation ponds and outdoor ponds, showing no statistically significant difference (Huesemann et al., 2017; Skeffington and Scheffel, 2018).
The main cultivation systems employed in large-scale commercial biomass production are open and closed culture configurations that are used to obtain different chemical compounds, such as fatty acids, proteins, antioxidants, pigments, and animal feedstock (Alishah Aratboni et al., 2019). Table 2 presents a detailed comparison of both cultivation systems, highlighting the advantages and disadvantages of each system. These two cultivation systems support both suspension and attached cultures. However, attached microalgae cultivation for biofuel production has been more favorable than suspended cultivation, as shown in Table 3, because it requires less space, has a low process cost, and is simple to harvest cultivated culture.
The commercial cultivation of Arthrospira in open raceways or tubular photobioreactors (PBRs) in over twenty countries, and Chlorella in open ponds, cascades, enclosed tubular and circular bonds in Japan, China, Germany, and Portugal yields annual biomass of 10,000 and 5,000 metric tons, respectively (Qin et al., 2023). The total amount of microalgal biomass produced worldwide is now close to 20,000 metric tons (Ciani et al., 2021; Qin et al., 2023). Table 4 shows the biomass concentrations yielded from different microalgae species cultivated in various systems. PBRs have better control of cultivation conditions as well as higher biomass productivity of 2–8 mg/L as compared to open systems that can produce 0.1–0.2 mg/L biomass (Ahmad et al., 2021).
Bioremediation, where strains can clean up fouled lands resulting from industrial pollution, has also been a topic of interest (Martínez-Ruiz et al., 2022). Cultivating microalgae in various types of wastewater, such as municipal, industrial, or agricultural, can remove wastewater residues such as nitrogen, phosphorus, and carbon (Costa et al., 2019); these compounds support the growth of microalgae (Leong et al., 2021; Wang and Zhang, 2022; Sundaram et al., 2023). Different microalgae species are used for treating specific types of wastewater. For agricultural wastewater, species such as Leptolyngbya sp. are commonly used, while in the case of municipal wastewater, Euglena viridis is an effective (Satya et al., 2023). Some microalgal species are versatile in bioremediation industrial wastewater that contains high organic loads, as they are capable of performing chemohetetrotrophic or mixotrophic pathways (Leong et al., 2021). Such species include Geitlerinema sp., Galdieria sulphuraria, Eucheuma denticulatum, and Chlorella vulgaris (Satya et al., 2023).
The microalgal-bacterial system is a promising wastewater bioremediation alternative (Leong et al., 2020). Microalgae and bacteria can depend on each other symbiotically, where microalgae take up carbon dioxide and convert inorganic into organic while releasing oxygen, which bacteria consume along with the organics (Zhao et al., 2023). In this manner, a newly designed sequential flow baffled microalgal-bacterial photobioreactor was integrated to study the impact of growing the microalgal species, Chlorella vulgaris, with a bacterial culture, resulting in a maximum biomass production of 792 mg/L and nitrogen removal efficiency enhanced from 40.91 to 96.38% (Leong et al., 2021, 2022). Therefore, cultivating microalgae on wastewater offers a viable option for lowering the cost of microalgae while simultaneously producing algal biomass as a feedstock for the production of biofuels, fertilizers, animal and fish feed, and other bio-based compounds (Wang and Zhang, 2022; Wang et al., 2022).
Artificial Intelligence (AI) can substantially enhance the productivity of microalgal cultures. Through AI, the optimization of cultivation conditions becomes more precise and comprehensive, enabling higher accuracy in the identification, classification, and quantification of algal strains and their growth dynamics (Peter et al., 2023). This advancement paves the way for the development of automated cultivation systems. Such systems can potentially reduce the costs associated with the harvesting and extraction of bioproducts, increasing the efficiency and economic viability of microalgal biotechnology applications (Long et al., 2022). The use of AI in the microalgae industry is rising with the development of AI technology and the need for sustainable resources. Figure 2 shows the current challenges and AI-driven solutions in the algal industry. AI often involves the use of large datasets, digitalization, and automation for increased efficiency in producing bioactive ingredients (Chong et al., 2023). Algal oil is considered one of the main solutions for renewable energy, but its application faces limitations related to sunlight penetration, poor cultivation dynamics, relatively low yield, and the high cost of industrial harvesting (Chen et al., 2012; Milledge and Heaven, 2013; Slocombe et al., 2021).
Figure 2. Challenges and AI-driven solutions in the algal industry. The diagram illustrates the challenges faced in producing high-value bioactive products from algae (highlighted in gray). It also showcases the AI techniques employed to address these challenges (highlighted in blue) and the anticipated results of these interventions (highlighted in green).
Recently, a model was developed to overcome these limitations, where different microalgae were cultured, and their growth was monitored with a mobile spectral imager. By introducing absorbance spectra of the cultured microalgae and simulating pairwise mixtures of them, they trained and validated a one-dimensional convolution neural network. This approach minimized extensive sample processing and allowed for automation, facilitating the microalgal culture analysis (Otálora et al., 2023). Mutual shading is one of the main factors limiting large-scale algal cultivation due to exponential light attenuation along the optical depth gradient (Nwoba et al., 2019). Sustaining optimal growth and minimizing mutual shading can be achieved by using machine learning (ML) to inform the design of semi-continuous algal cultivation (SAC). Aggregation-based sedimentation (ABS) strategies can achieve low-cost biomass harvesting and economical SAC. Based on model predictions, the National Renewable Energy Laboratory (NREL) estimated that a minimum biomass selling price (MBSP) of 281$ compared to 1,227$ per ton for open pond algal cultivation in 2019 (Long et al., 2022). These AI-mediated advances may eventually prove to be the deciding factor in bringing microalgae to successful and sustainable commercialization.
Artificial neural networks (ANN) and support vector machines (SVMs) are ML algorithms used for classification and regression tasks. ANNs resemble biological neural networks in terms of performance, created as extensions of mathematical models of human neural biology. Neural networks have the capacity to process large datasets with adaptive learning, allowing pattern association, classification, clustering, and prediction (Thakur and Konde, 2021). ANN was used to predict the growth of polyculture microalgae in semi-continuous open ponds with different input parameters. The best combination of weight and bias was selected during the training process, resulting in effective and precise prediction of microalgae growth with high accuracy (R2 = 0.93). They identified solar irradiance and temperature as key variants to estimate microalgal growth (Noguchi et al., 2019). The powerful and flexible SVM ML model is capable of minimizing complexity and noise and enhancing the generalization performance of a network by defining the hyperplanes that best separate data into different classes (Elkiran et al., 2019). It has been used in hydro-environmental studies, more specifically for wastewater treatment plant performance analysis (Nourani et al., 2018; Elkiran et al., 2019). SVMs were also used for fucoxanthin extraction optimization and antioxidant activity quantification. Fucoxanthin is a carotenoid with various beneficial medicinal properties for human wellbeing (Chong et al., 2023). In addition, SVMs can predict the growth behavior of microalgae in outdoor cultivation. The Fraunhofer Institute of Interfacial Engineering and Biotechnology used the SVM-based model to predict the growth rate of Phaedactylum tricornutum with a correlation coefficient of 88%, a significant advancement toward superior predictive models for microalgae production (IGB, 2023).
Biochar is a carbon-rich product of biomass produced through pyrolysis from waste residues and can also be produced from microalgae (for example, Chlorella vulgaris), yielding a potential clean technology for carbon sequestration and microalgal biorefinery (Yu et al., 2018). Machine learning (ML) libraries based on the gradient boosting algorithms (eXtreme Gradient Boosting; XGB) were implemented to predict biochar yields from algae. By achieving a high regression coefficient (R2 = 0.84) between experimental and model predictive biochar yields, they showed that it could be effectively used to estimate biochar yield from biomass conversion. They determined the key parameters (H/C, N/C, Ash, pyrolysis temperature, and time) for deciding biochar yield from algal culture (Pathy et al., 2020). The XGB model evaluated 213 input combinations and identified key parameters (such as pyrolysis temperature, carbon, and ash content). The model can optimize production yield quantitatively and qualitatively (Pathy et al., 2020). XGBoost and other gradient-boosting models were used to predict harmful algal blooms (HABs). HABs are a serious threat to aquatic ecosystems and human health; therefore, managing and controlling their spread is crucial (Ahn et al., 2023).
Can a promising isolate be further improved? Random mutagenesis and genetic engineering are examples of useful techniques adopted for microalgal strain improvement (Carino and Vital, 2022). Random mutagenesis is a useful approach for creating more productive strains, with the advantage of not requiring extensive knowledge of microalgal genetics (Trovão et al., 2022). Microalgal enhanced through random mutagenesis could alter biomass generation, production of target compounds, and strain optimization that leads to increased tolerance of a wider range of environmental conditions.
The process of random mutagenesis involves subjecting microalgae cells to chemical or physical mutagens, leading to the generation of a diverse population of mutants with distinct genetic and phenotypic characteristics (Fathy et al., 2023). Examples of chemical mutagens are alkylating agents, Base Analogs (BAs), AntiMetabolites (AMs), and Intercalating Agents (IAs) while the physical mutagens include UV radiation, ionizing radiation, Atmospheric and Room Temperature Plasma (ARTP), and laser irradiation (Bleisch et al., 2022).
UV irradiation is a safer, more rapid, and more effective mutagenesis method in comparison to chemical mutagenesis methods (Sivaramakrishnan and Incharoensakdi, 2017; Carino and Vital, 2022). Based on its wavelengths, UV light is classified into low (UV-A, 320–420 nm), middle (UV-B, 280–320 nm), and high (UV-C, 180–280 nm) energy (Cao X. et al., 2021). UV-C is more efficient for microalgae compared to UV-A or B in terms of its mutagenesis effects (Sivaramakrishnan and Incharoensakdi, 2017). UV-A produces reactive oxygen species and causes indirect damage to the cells by damaging DNA, proteins, and lipids, while UV-B and UV-C cause direct damage to the DNA by forming pyrimidine dimers in adjacent base pairs (Sydney et al., 2018). UV-C irradiation is optimal for methods utilizing random mutations followed by selection, such as those used for microalgae (Fathy et al., 2023).
Mutations caused by UV-C radiation are related to the formation of cyclobutane pyrimidine dimers (CPDs) and pyrimidine (6–4) pyrimidinone dimers [(6–4) photoproducts] (Bleisch et al., 2022). During this process, it is essential to prevent light-induced (300–600 nm) DNA repair via photoreactivation (Ha and Bhagavan, 2022) by covering the UV-irradiated plates with foil immediately after exposure and incubating them in the dark (Carino and Vital, 2022) for 24–72 h, depending on the cell cycle period. Photoreactivation, which is mediated by photolyase, is thought to be the major DNA repair pathway for CPDs and (6–4) photoproducts in higher plants where photolyases bind specifically to these DNA lesions and remove them directly by absorbing light in the 300–600 nm range (Ha and Bhagavan, 2022).
Exposing microalgae to UV light increases the levels of saturated fatty acids and monosaturated fatty acids and forms antioxidants, which could be the reason for enhanced polyunsaturated fatty acids synthesis (Udayan et al., 2023). Random UV-C mutagenesis performed on Chlorella vulgaris resulted in two isolated mutants with a significantly enhanced total lipid content (75%, >1.3-fold increase; Carino and Vital, 2022). Studies employed UV radiation in Chlorella vulgaris and Scenedesmus obliquus achieved substantial 2.4- and 2.39-fold increases, respectively, in lipid content when compared to the control strains (Fathy et al., 2023). Table 5 shows other examples of applying UV radiation to enhance biomass and lipid productivity in different microalgae strains. Significantly, microalgal strains improved using the above random mutation methods are not labeled as genetically modified organisms (GMOs) since no foreign genetic material is introduced into their genomes (Trovão et al., 2022).
However, while non-targeted mutagenesis introduces random changes in the genome, the results are usually unpredictable, often leading to mutants with lower yields than the parental strain (Yi et al., 2018). Rare random mutations with positive effects can be isolated if a successful screening method is applied (Yi et al., 2018). Figure 3 illustrates combining classical random mutagenesis with high-throughput screening via FACS which has become the gold standard for generating strains with a high growth rate and productivity, stress tolerance, and resistance to common pests (Arora and Philippidis, 2021). FACS quantitatively measures the optical characteristics of each single cell passing through a focused light beam when coupled with fluorescent labeling; the technique allows for differentiating cell subpopulations in various physiological states (Sibanda and Buys, 2017).
Figure 3. Iterative cycles of UV mutagenesis combined with fluorescence-activated cell sorting (FACS). A diagram of the workflow for applying UV radiation to microalgae strains combined with live-cell sorting by FACS to improve strain productivity is shown. These steps can be repeated to obtain mutants with the desired phenotypes. Analysis of the cellular metabolites of the sorted algal populations can be done by ultra-high-pressure liquid chromatography coupled with quadrupole time-of-flight mass spectrometry (UPLC-QTOF-MS) or by confocal Raman microscopy.
Applying random mutagenesis prior to FACS has been reported to isolate lipid-rich mutants of several different microalgal species (Pereira et al., 2018). For instance, Yi et al. (2018) generated several mutants of the model diatom, Phaeodactylum tricornutum, with increased carotenoid accumulation using the chemical mutagens ethyl methanesulfonate (EMS) and N-methyl-N'-nitro-N-nitrosoguanidine (NTG) alkylating agents. They then screened ~1,000 strains using a fluorescence-based high-throughput method, and five selected mutants had 33% or higher total carotenoids than the wild-type (Yi et al., 2018). Similarly, Sharma et al. (2015) and Abdrabu et al. (2016) applied repetitive rounds of UV radiation on Chlamydomonas reinhardtii (CC-503) and selected high lipid accumulated mutants by FACS (Sharma et al., 2015; Abdrabu et al., 2016).
Microalgal compounds can be analyzed by ultra-high-pressure liquid chromatography coupled with quadrupole time-of-flight mass spectrometry (UHPLC-QTOF-MS; Lee et al., 2018). It is a rapid and sensitive analytical technique for detecting and identifying metabolites in biological samples (Cao X. C. et al., 2021). This technique has been successfully used to determine and identify intact polar and neutral lipid molecular species among lipidomes (Castro-Gómez et al., 2017). Confocal Raman microscopy (CRM) is a non-invasive method for locating and examining lipids, pigments, and carbohydrates in the algal research (Scalfi-Happ et al., 2011; Sharma et al., 2015). It allows real-time monitoring and examination of living cells without labeling; thus, Raman gives extensive chemical information without damaging or labeling samples (Zhang et al., 2021).
Microalgae have emerged as a promising group of organisms for pursuing sustainable solutions, specifically in bioproducts, biofuels, and consumer nutritional supplements. The recent data availability and advances in high-throughput techniques helped better understand and characterize the biology of microalgae (Kumar et al., 2020; Villanova and Spetea, 2021; Helmy et al., 2023).
Using systems biology approaches and artificial intelligence can revolutionize the industrial application of microalgae (e.g., algal oil and high-value bioactive products) by optimizing their growth and enhancing product quality. The application of artificial intelligence methods in microalgae research has enabled the definition of the influence of input parameters on cultivation conditions and variables, resulting in high prediction accuracy that significantly enhances the efficiency and optimization of algal cultivation systems and industry. While ANNs comprise the go-to algorithms for ML, they are often complemented by other approaches like SVMs and XGBs (Coşgun et al., 2023). At present, ML is an emerging field in algal cultivation. The mentioned methods allow the deciphering of complex patterns and interactions that can help to overcome limiting challenges and achieve sustainability.
With increased environmental and supply chain challenges, microalgae are expected to gain interest globally. In addition to their prospective applications, microalgae are great candidates for addressing pressing issues like wastewater treatment and carbon sequestration. The full potential of microalgae will likely be realized through multidisciplinary research to solve the most critical problems. Of these, advances in cultivation systems and strain engineering will be vital. Importantly, integrating multi-omics datasets through AI approaches can provide critical solutions to enhance productivity.
This review examined the aspects of microalgal isolation, cultivation, and strain optimization, focusing on mutagenesis screens and the use of artificial intelligence in optimizing cultivation. Biotechnological innovations, such as genome editing tools, will play a vital role when molecular-genetic tools are available for the species. Furthermore, comprehensive techno-economic analyses considering scalability and sustainability factors will be crucial in guiding the commercialization of microalgae. With concerted efforts, microalgae can significantly contribute to global sustainability initiatives for food, fuel, and nutraceuticals.
KS-A: Conceptualization, Funding acquisition, Investigation, Project administration, Supervision, Visualization, Writing – original draft, Writing – review & editing. AA: Conceptualization, Visualization, Writing – original draft, Writing – review & editing. SD: Conceptualization, Visualization, Writing – original draft, Writing – review & editing. DN: Conceptualization, Visualization, Writing – original draft, Writing – review & editing. DA-K: Conceptualization, Formal analysis, Visualization, Writing – review & editing. J-CT: Conceptualization, Writing – review & editing.
The author(s) declare financial support was received for the research, authorship, and/or publication of this article. This work was supported by NYUAD Faculty Research Funds (AD060).
Landscape images in Figure 1 were obtained from: https://neural.love under a CC0 (no rights reserved) license. Flask and tube clipart icons were obtained from: https://www.freeclipartnow.com. Icon images in Figures 2, 3 were obtained from: https://chat.openai.com (GPT-4) using the DALL-E 3 plugin. Claude-2 (https://www.anthropic.com/index/claude-2) was used to improve the flow and readability of the text, Claude-2, Quilbot (https://quillbot.com/), and Grammarly (https://www.grammarly.com/) were used as proofreading tools.
The authors declare that the research was conducted in the absence of any commercial or financial relationships that could be construed as a potential conflict of interest.
All claims expressed in this article are solely those of the authors and do not necessarily represent those of their affiliated organizations, or those of the publisher, the editors and the reviewers. Any product that may be evaluated in this article, or claim that may be made by its manufacturer, is not guaranteed or endorsed by the publisher.
Abdrabu, R., Sharma, S. K., Khraiwesh, B., Jijakli, K., Nelson, D. R., Alzahmi, A., et al. (2016). Single-cell characterization of microalgal lipid contents with confocal raman microscopy. Essent. Single Cell Anal. 14, 363–382. doi: 10.1007/978-3-662-49118-8_14
Abo, B. O., Odey, E. A., Bakayoko, M., and Kalakodio, L. (2019). Microalgae to biofuels production: a review on cultivation, application and renewable energy. Rev. Environ. Health 34, 91–99. doi: 10.1515/reveh-2018-0052
Ahmad, I., Abdullah, N., Koji, I., Yuzir, A., and Muhammad, S. E. (2021). “Evolution of photobioreactors: a review based on microalgal perspective,” in IOP Conference Series: Materials Science and Engineering (Bristol: IOP Publishing), e012004. doi: 10.1088/1757-899X/1142/1/012004
Ahn, J. M., Kim, J., and Kim, K. (2023). Ensemble machine learning of gradient boosting (XGBoost, LightGBM, CatBoost) and attention-based CNN-LSTM for harmful algal blooms forecasting. Toxins 15:608. doi: 10.3390/toxins15100608
Alishah Aratboni, H., Rafiei, N., Garcia-Granados, R., Alemzadeh, A., and Morones-Ramírez, J. R. (2019). Biomass and lipid induction strategies in microalgae for biofuel production and other applications. Microbial. Cell Factor. 18, 1–17. doi: 10.1186/s12934-019-1228-4
Ampou, E. E., Ouillon, S., and Andrefouet, S. (2018). Challenges in rendering Coral Triangle habitat richness in remotely sensed habitat maps: the case of Bunaken Island (Indonesia). Mar. Pollut. Bull. 131, 72–82. doi: 10.1016/j.marpolbul.2017.10.026
Araújo, R., Vázquez Calderón, F., Sánchez López, J., Azevedo, I. C., Bruhn, A., Fluch, S., et al. (2021). Current status of the algae production industry in Europe: an emerging sector of the blue bioeconomy. Front. Mar. Sci. 7:626389. doi: 10.3389/fmars.2020.626389
Arora, N., and Philippidis, G. P. (2021). Microalgae strain improvement strategies: random mutagenesis and adaptive laboratory evolution. Trends Plant Sci. 26, 1199–1200. doi: 10.1016/j.tplants.2021.06.005
Ben Hassen, T., and El Bilali, H. (2022). Impacts of the Russia-Ukraine war on global food security: towards more sustainable and resilient food systems? Foods 11:2301. doi: 10.3390/foods11152301
Berges, J. A., Driskill, A. M., Guinn, E. J., Pokrzywinski, K., Quinlan, J., von Korff, B., et al. (2021). Role of nearshore benthic algae in the Lake Michigan silica cycle. PLoS ONE 16:e0256838. doi: 10.1371/journal.pone.0256838
Bleisch, R., Freitag, L., Ihadjadene, Y., Sprenger, U., Steingröwer, J., Walther, T., et al. (2022). Strain development in microalgal biotechnology-random mutagenesis techniques. Life 12:961. doi: 10.3390/life12070961
Boelen, P., van Dijk, R., Sinninghe Damsté, J. S., Rijpstra, W. I. C., and Buma, A. G. (2013). On the potential application of polar and temperate marine microalgae for EPA and DHA production. AMB Expr. 3, 1–9. doi: 10.1186/2191-0855-3-26
Cao, X., Zhao, J., Wang, Z., and Xing, B. (2021). New insight into the photo-transformation mechanisms of graphene oxide under UV-A, UV-B and UV-C lights. J. Hazard. Mater. 403:123683. doi: 10.1016/j.jhazmat.2020.123683
Cao, X. C., Guo, M. F., Han, Y., Fan, Y. T., Zhu, J. H., Zhu, H., et al. (2021). Systematic metabolite profiling of N-acetyldopamine oligomers from Cicadae Periostracum in rats by ultra-high performance liquid chromatography coupled with quadrupole-time-of-flight mass spectrometry. J. Pharmaceut. Biomed. Anal. 192:113665. doi: 10.1016/j.jpba.2020.113665
Carino, J. D., and Vital, P. G. (2022). Characterization of isolated UV-C-irradiated mutants of microalga Chlorella vulgaris for future biofuel application. Environ. Dev. Sustainabil. 8, 1–18. doi: 10.1007/s10668-021-02091-8
Castro-Gómez, P., Montero, O., and Fontecha, J. (2017). In-depth lipidomic analysis of molecular species of triacylglycerides, diacylglycerides, glycerophospholipids, and sphingolipids of buttermilk by GC-MS/FID, HPLC-ELSD, and UPLC-QToF-MS. Int. J. Mol. Sci. 18:605. doi: 10.3390/ijms18030605
Chen, H. H., Xue, L. L., Liang, M. H., and Jiang, J. G. (2019). Effects of triethylamine on the expression patterns of two G3PDHs and lipid accumulation in Dunaliella tertiolecta. Enzyme Microb. Technol. 127, 17–21. doi: 10.1016/j.enzmictec.2019.04.004
Chen, L., Liu, T., Zhang, W., Chen, X., and Wang, J. (2012). Biodiesel production from algae oil high in free fatty acids by two-step catalytic conversion. Bioresour. Technol. 111, 208–214. doi: 10.1016/j.biortech.2012.02.033
Chong, J. W. R., Tang, D. Y. Y., Leong, H. Y., Khoo, K. S., Show, P. L., and Chew, K. W. (2023). Bridging artificial intelligence and fucoxanthin for the recovery and quantification from microalgae. Bioengineered 14:2244232. doi: 10.1080/21655979.2023.2244232
Chowdury, K. H., Nahar, N., and Deb, U. K. (2020). The growth factors involved in microalgae cultivation for biofuel production: a review. Comput. Water Energy Environ. Eng. 9, 185–215. doi: 10.4236/cweee.2020.94012
Ciani, M., Lippolis, A., Fava, F., Rodolfi, L., Niccolai, A., and Tredici, M. R. (2021). Microbes: food for the future. Foods 10:971. doi: 10.3390/foods10050971
Concordio-Reis, P., David, H., Reis, M. A. M., Amorim, A., and Freitas, F. (2023). Bioprospecting for new exopolysaccharide-producing microalgae of marine origin. Int. Microbiol. 26, 1123–1130. doi: 10.1007/s10123-023-00367-9
Coşgun, A., Günay, M. E., and Yildirim, R. (2023). Machine learning for algal biofuels: a critical review and perspective for future. Green Chem. 2023:D3GC00389B. doi: 10.1039/D3GC00389B
Costa, J. A. V., Freitas, B. C. B., Santos, T. D., Mitchell, B. G., and Morais, M. G. (2019). Open pond systems for microalgal culture. Biofuels Algae 3, 199–223. doi: 10.1016/B978-0-444-64192-2.00009-3
Darienko, T., Gustavs, L., Eggert, A., Wolf, W., and Proeschold, T. (2015). Evaluating the species boundaries of green microalgae (Coccomyxa, Trebouxiophyceae, Chlorophyta) using integrative taxonomy and DNA barcoding with further implications for the species identification in environmental samples. PLoS ONE 10:e0127838. doi: 10.1371/journal.pone.0127838
Darwesh, O. M., Mahmoud, R. H., Abdo, S. M., and Marrez, D. A. (2022). Isolation of Haematococcus lacustris as source of novel anti-multi-antibiotic resistant microbes agents; fractionation and identification of bioactive compounds. Biotechnol. Rep. 35:e00753. doi: 10.1016/j.btre.2022.e00753
Dasan, Y. K., Lam, M. K., Yusup, S., Lim, J. W., Show, P. L., Tan, I. S., et al. (2020). Cultivation of Chlorella vulgaris using sequential-flow bubble column photobioreactor: a stress-inducing strategy for lipid accumulation and carbon dioxide fixation. J. CO2 Util. 41:101226. doi: 10.1016/j.jcou.2020.101226
Deniset-Besseau, A., Coat, R., Moutel, B., Rebois, R., Mathurin, J., Grizeau, D., et al. (2021). Revealing lipid body formation and its subcellular reorganization in oleaginous microalgae using correlative optical microscopy and infrared nanospectroscopy. Appl. Spectrosc. 75, 1538–1547. doi: 10.1177/00037028211050659
Diaz, C. J., Douglas, K. J., Kang, K., Kolarik, A. L., Malinovski, R., Torres-Tiji, Y., et al. (2023). Developing algae as a sustainable food source. Front. Nutr. 9:3147. doi: 10.3389/fnut.2022.1029841
Elagoz, A. M., Ambrosino, L., and Lauritano, C. (2020). De novo transcriptome of the diatom Cylindrotheca closterium identifies genes involved in the metabolism of anti-inflammatory compounds. Sci. Rep. 10:4138. doi: 10.1038/s41598-020-61007-0
Elkiran, G., Nourani, V., and Abba, S. (2019). Multi-step ahead modelling of river water quality parameters using ensemble artificial intelligence-based approach. J. Hydrol. 577:123962. doi: 10.1016/j.jhydrol.2019.123962
Fathy, W. A., Techen, N., Elsayed, K., Essawy, E., Tawfik, E., Abdelhameed, M. S., et al. (2023). Insights into random mutagenesis techniques to enhance biomolecule production in microalgae: implications for economically viable bioprocesses. Int. Aquat. Res. 15, 85–102. doi: 10.22034/IAR.2023.1982761.1419
Fu, W., Nelson, D. R., Mystikou, A., Daakour, S., and Salehi-Ashtiani, K. (2019). Advances in microalgal research and engineering development. Curr. Opin. Biotechnol. 59, 157–164. doi: 10.1016/j.copbio.2019.05.013
Gong, Y., Wang, Q., Wei, L., Liang, W., Wang, L., Lv, N., et al. (2023). Genome-wide adenine N6-methylation map unveils epigenomic regulation of lipid accumulation in Nannochloropsis. Plant Commun. 2023:100773. doi: 10.1016/j.xplc.2023.100773
Hadi, S. I., Santana, H., Brunale, P. P., Gomes, T. G., Oliveira, M. D., Matthiensen, A., et al. (2016). DNA barcoding green microalgae isolated from neotropical inland waters. PLoS ONE 11:e0149284. doi: 10.1371/journal.pone.0149284
Häubner, N., Schumann, R., and Karsten, U. (2006). Aeroterrestrial microalgae growing in biofilms on facades-response to temperature and water stress. Microbial. Ecol. 51, 285–293. doi: 10.1007/s00248-006-9016-1
Helmy, M., Elhalis, H., Liu, Y., Chow, Y., and Selvarajoo, K. (2023). Perspective: multiomics and machine learning help unleash the alternative food potential of microalgae. Adv. Nutr. 14, 1–11. doi: 10.1016/j.advnut.2022.11.002
Huesemann, M., Dale, T., Chavis, A., Crowe, B., Twary, S., Barry, A., et al. (2017). Simulation of outdoor pond cultures using indoor LED-lighted and temperature-controlled raceway ponds and Phenometrics photobioreactors. Algal Res. 21, 178–190. doi: 10.1016/j.algal.2016.11.016
IGB (2023). Algae Cultivation. Available online at: https://www.igb.fraunhofer.de/en/about-us/spotlight/machine-learning-for-algae-cultivation.html (accessed October 17, 2023).
Jaiswal, K. K., Chowdhury, C. R., Yadav, D., Verma, R., Dutta, S., Jaiswal, K. S., et al. (2022). Renewable and sustainable clean energy development and impact on social, economic, and environmental health. Energy Nexus 7:100118. doi: 10.1016/j.nexus.2022.100118
Jareonsin, S., and Pumas, C. (2021). Advantages of heterotrophic microalgae as a host for phytochemicals production. Front. Bioeng. Biotechnol. 9:628597. doi: 10.3389/fbioe.2021.628597
Khan, M. I., Shin, J. H., and Kim, J. D. (2018). The promising future of microalgae: current status, challenges, and optimization of a sustainable and renewable industry for biofuels, feed, and other products. Microbial. Cell Factor. 17, 1–21. doi: 10.1186/s12934-018-0879-x
Khoo, K. S., Ahmad, I., Chew, K. W., Iwamoto, K., Bhatnagar, A., and Show, P. L. (2023). Enhanced microalgal lipid production for biofuel using different strategies including genetic modification of microalgae: a review. Progr. Energy Combust. Sci. 96:101071. doi: 10.1016/j.pecs.2023.101071
Kim, S., Kim, M., Chang, Y. K., and Kim, D. (2023). Lipid production under a nutrient-sufficient condition outperforms starvation conditions due to a natural polarization of lipid content in algal biofilm. Fuel 339:126902. doi: 10.1016/j.fuel.2022.126902
Kumar, D., Agrawal, S., and Sahoo, D. (2023). Assessment of the intrinsic bioremediation capacity of a complexly contaminated Yamuna River of India: a algae-specific approach. Int. J. Phytoremed. 2, 1–15. doi: 10.1080/15226514.2023.2200862
Kumar, G., Shekh, A., Jakhu, S., Sharma, Y., Kapoor, R., and Sharma, T. R. (2020). Bioengineering of microalgae: recent advances, perspectives, and regulatory challenges for industrial application. Front. Bioeng. Biotechnol. 8:914. doi: 10.3389/fbioe.2020.00914
Kurniawan, R., Nurkolis, F., Taslim, N. A., Subali, D., Surya, R., Gunawan, W. B., et al. (2023). Carotenoids composition of green algae Caulerpa racemosa and their antidiabetic, anti-obesity, antioxidant, and anti-inflammatory properties. Molecules 28:3267. doi: 10.3390/molecules28073267
Lacour, T., Robert, E., and Lavaud, J. (2023). Sustained xanthophyll pigments-related photoprotective NPQ is involved in photoinhibition in the haptophyte Tisochrysis lutea. Sci. Rep. 13:14694. doi: 10.1038/s41598-023-40298-z
Lananan, F., Jusoh, A., Lam, S. S., and Endut, A. (2013). Effect of conway medium and f/2 medium on the growth of six genera of South China Sea marine microalgae. Bioresour. Technol. 141, 75–82. doi: 10.1016/j.biortech.2013.03.006
Lee, B.-J., Zhou, Y., Lee, J. S., Shin, B. K., Seo, J.-A., Lee, D., et al. (2018). Discrimination and prediction of the origin of Chinese and Korean soybeans using Fourier transform infrared spectrometry (FT-IR) with multivariate statistical analysis. PLoS ONE 13:e0196315. doi: 10.1371/journal.pone.0196315
Leitner, P. D., Jakschitz, T., Gstir, R., Stuppner, S., Perkams, S., Kruus, M., et al. (2022). Anti-inflammatory extract from soil algae Chromochloris zofingiensis targeting TNFR/NF-κB signaling at different levels. Cells 11:1407. doi: 10.3390/cells11091407
Leong, W. H., Kiatkittipong, K., Kiatkittipong, W., Cheng, Y. W., Lam, M. K., Shamsuddin, R., et al. (2020). Comparative performances of microalgal-bacterial co-cultivation to bioremediate synthetic and municipal wastewaters whilst producing biodiesel sustainably. Processes 8:1427. doi: 10.3390/pr8111427
Leong, W. H., Kiatkittipong, W., Lam, M. K., Khoo, K. S., Show, P. L., Mohamad, M., et al. (2022). Dual nutrient heterogeneity modes in a continuous flow photobioreactor for optimum nitrogen assimilation to produce microalgal biodiesel. Renew. Energy 184, 443–451. doi: 10.1016/j.renene.2021.11.117
Leong, W. H., Lim, J. W., Lam, M. K., Lam, S. M., Sin, J. C., and Samson, A. (2021). Novel sequential flow baffled microalgal-bacterial photobioreactor for enhancing nitrogen assimilation into microalgal biomass whilst bioremediating nutrient-rich wastewater simultaneously. J. Hazard. Mater. 409:124455. doi: 10.1016/j.jhazmat.2020.124455
Lin-Lan, Z., Jing-Han, W., and Hong-Ying, H. (2018). Differences between attached and suspended microalgal cells in ssPBR from the perspective of physiological properties. J. Photochem. Photobiol. B 181, 164–169. doi: 10.1016/j.jphotobiol.2018.03.014
Liu, P. Y., Li, G., Lin, C. B., Wu, J. J., Jiang, S., Huang, F. H., et al. (2022). Modulating DHA-producing Schizochytrium sp. toward astaxanthin biosynthesis via a seamless genome editing system. ACS Synth. Biol 11, 4171–4183. doi: 10.1021/acssynbio.2c00490
Liu, S., Zhao, Y., Liu, L., Ao, X., Ma, L., Wu, M., et al. (2015). Improving cell growth and lipid accumulation in green microalgae Chlorella sp. via UV irradiation. Appl. Biochem. Biotechnol. 175, 3507–3518. doi: 10.1007/s12010-015-1521-6
Long, B., Fischer, B., Zeng, Y., Amerigian, Z., Li, Q., Bryant, H., et al. (2022). Machine learning-informed and synthetic biology-enabled semi-continuous algal cultivation to unleash renewable fuel productivity. Nat. Commun. 13:541. doi: 10.1038/s41467-021-27665-y
Lopez, A., Rico, M., Santana-Casiano, J. M., Gonzalez, A. G., and Gonzalez-Davila, M. (2015). Phenolic profile of Dunaliella tertiolecta growing under high levels of copper and iron. Environ. Sci. Pollut. Res. Int. 22, 14820–14828. doi: 10.1007/s11356-015-4717-y
Lue, L.-F., Walker, D. G., Beh, S. T., and Beach, T. G. (2022). Isolation of human microglia from neuropathologically diagnosed cases in the single-cell era. Alzheimer's Dis. 3, 43–62. doi: 10.1007/978-1-0716-2655-9_3
Manabe, Y., Takagi-Hayashi, S., Mohri, S., and Sugawara, T. (2023). Intestinal absorption and anti-inflammatory effects of siphonein, a siphonaxanthin fatty acid ester from green algae. J. Nutrit. Sci. Vitaminol. 69, 62–70. doi: 10.3177/jnsv.69.62
Mancini, A., Imperlini, E., Nigro, E., Montagnese, C., Daniele, A., Orrù, S., et al. (2015). Biological and nutritional properties of palm oil and palmitic acid: effects on health. Molecules 20, 17339–17361. doi: 10.3390/molecules200917339
Martínez-Ruiz, M., Molina-Vázquez, A., Santiesteban-Romero, B., Reyes-Pardo, H., Villaseñor-Zepeda, K. R., and Meléndez-Sánchez, E. R.. (2022). Micro-algae assisted green bioremediation of water pollutants rich leachate and source products recovery. Environ. Pollut. 306:119422. doi: 10.1016/j.envpol.2022.119422
Milledge, J. J., and Heaven, S. (2013). A review of the harvesting of micro-algae for biofuel production. Rev. Environ. Sci. Bio/Technol. 12, 165–178. doi: 10.1007/s11157-012-9301-z
Mohamadnia, S., Tavakoli, O., and Faramarzi, M. A. (2022). Production of fucoxanthin from the microalga Tisochrysis lutea in the bubble column photobioreactor applying mass transfer coefficient. J. Biotechnol. 348, 47–54. doi: 10.1016/j.jbiotec.2022.03.009
Moore, D. (2021). Saving the planet with appropriate biotechnology: 4. coccolithophore cultivation and deployment/Salvando el planeta con biotecnología apropiada: 4. Cultivo de cocolitóforos e implementación. Mex. J. Biotechnol. 6, 129–155. doi: 10.29267/mxjb.2021.6.1.129
Morales, M., Collet, P., Lardon, L., Hélias, A., Steyer, J.-P., and Bernard, O. (2019). Life-cycle assessment of microalgal-based biofuel. Biofuels Algae 2, 507–550. doi: 10.1016/B978-0-444-64192-2.00020-2
Nelson, D. R., Chaiboonchoe, A., Fu, W., Hazzouri, K. M., Huang, Z., Jaiswal, A., et al. (2019). Potential for heightened sulfur-metabolic capacity in coastal subtropical microalgae. Iscience 11, 450–465. doi: 10.1016/j.isci.2018.12.035
Nelson, D. R., Khraiwesh, B., Fu, W., Alseekh, S., Jaiswal, A., Chaiboonchoe, A., et al. (2017). The genome and phenome of the green alga Chloroidium sp. UTEX 3007 reveal adaptive traits for desert acclimatization. Elife 6:e25783. doi: 10.7554/eLife.25783
Nelson, D. R., Mengistu, S., Ranum, P., Celio, G., Mashek, M., Mashek, D., et al. (2013). New lipid-producing, cold-tolerant yellow-green alga isolated from the Rocky Mountains of Colorado. Biotechnol. Prog. 29, 853–861. doi: 10.1002/btpr.1755
Noguchi, R., Ahamed, T., Rani, D. S., Sakurai, K., Nasution, M. A., Wibawa, D. S., et al. (2019). Artificial neural networks model for estimating growth of polyculture microalgae in an open raceway pond. Biosyst. Eng. 177, 122–129. doi: 10.1016/j.biosystemseng.2018.10.002
Nourani, V., Elkiran, G., and Abba, S. (2018). Wastewater treatment plant performance analysis using artificial intelligence-an ensemble approach. Water Sci. Technol. 78, 2064–2076. doi: 10.2166/wst.2018.477
Nwoba, E. G., Parlevliet, D. A., Laird, D. W., Alameh, K., and Moheimani, N. R. (2019). Light management technologies for increasing algal photobioreactor efficiency. Algal Res. 39:101433. doi: 10.1016/j.algal.2019.101433
Okumura, N., Kusakabe, A., Hirano, H., Inoue, R., Okazaki, Y., Nakano, S., et al. (2015). Density-gradient centrifugation enables the purification of cultured corneal endothelial cells for cell therapy by eliminating senescent cells. Sci. Rep. 5:15005. doi: 10.1038/srep15005
Otálora, P., Guzmán, J., Acién, F., Berenguel, M., and Reul, A. (2023). An artificial intelligence approach for identification of microalgae cultures. N. Biotechnol. 77, 58–67. doi: 10.1016/j.nbt.2023.07.003
Pathy, A., and Meher, S. P. B. (2020). Predicting algal biochar yield using eXtreme Gradient Boosting (XGB) algorithm of machine learning methods. Algal Res. 50:102006. doi: 10.1016/j.algal.2020.102006
Pereira, H., Schulze, P. S., Schüler, L. M., Santos, T., Barreira, L., and Varela, J. (2018). Fluorescence activated cell-sorting principles and applications in microalgal biotechnology. Algal Res. 30, 113–120. doi: 10.1016/j.algal.2017.12.013
Peter, A. P., Chew, K. W., Pandey, A., Lau, S. Y., Rajendran, S., Ting, H. Y., et al. (2023). Artificial intelligence model for monitoring biomass growth in semi-batch Chlorella vulgaris cultivation. Fuel 333:126438. doi: 10.1016/j.fuel.2022.126438
Pradhan, B., and Ki, J. S. (2022). Phytoplankton toxins and their potential therapeutic applications: a journey toward the quest for potent pharmaceuticals. Mari. Drugs 20:271. doi: 10.3390/md20040271
Qi, F., Wu, D., Mu, R., Zhang, S., and Xu, X. (2018). Characterization of a microalgal UV mutant for CO2 biofixation and biomass production. Biomed. Res. Int. 2018:4375170. doi: 10.1155/2018/4375170
Qin, S., Wang, K., Gao, F., Ge, B., Cui, H., and Li, W. (2023). Biotechnologies for bulk production of microalgal biomass: from mass cultivation to dried biomass acquisition. Biotechnol. Biofuels Bioproduct. 16:131. doi: 10.1186/s13068-023-02382-4
Rachmayati, R., Agustriana, E., and Rahman, D. Y. (2020). UV mutagenesis as a strategy to enhance growth and lipid productivity of Chlorella sp. 042. J. Trop. Biodivers. Biotechnol. 5:218. doi: 10.22146/jtbb.56862
Rafa, N., Ahmed, S. F., Badruddin, I. A., Mofijur, M., and Kamangar, S. (2021). Strategies to produce cost-effective third-generation biofuel from microalgae. Front. Energy Res. 9:749968. doi: 10.3389/fenrg.2021.749968
Rahman, D., Rachmayati, R., Widyaningrum, D., and Susilaningsih, D. (2020). “Enhancement of lipid production of Chlorella sp. 042 by mutagenesis,” in IOP Conference Series: Earth and Environmental Science (Bristol: IOP Publishing), e012021. doi: 10.1088/1755-1315/439/1/012021
Rahman, K. M. (2020). Food and high value products from microalgae: market opportunities and challenges. Microalgae Biotechnol. Food Health High Value Prod. 1, 3–27. doi: 10.1007/978-981-15-0169-2_1
Russell, C., Rodriguez, C., and Yaseen, M. (2022). Microalgae for lipid production: cultivation, extraction & detection. Algal Res. 66:102765. doi: 10.1016/j.algal.2022.102765
Satya, A. D. M., Cheah, W. Y., Yazdi, S. K., Cheng, Y.-S., Khoo, K. S., Vo, D.-V. N., et al. (2023). Progress on microalgae cultivation in wastewater for bioremediation and circular bioeconomy. Environ. Res. 218:114948. doi: 10.1016/j.envres.2022.114948
Scalfi-Happ, C., Udart, M., Hauser, C., and Rück, A. (2011). Investigation of lipid bodies in a colon carcinoma cell line by confocal Raman microscopy. Med. Laser Appl. 26, 152–157. doi: 10.1016/j.mla.2011.08.002
Sharma, S. K., Nelson, D. R., Abdrabu, R., Khraiwesh, B., Jijakli, K., Arnoux, M., et al. (2015). An integrative Raman microscopy-based workflow for rapid in situ analysis of microalgal lipid bodies. Biotechnol. Biofuels 8, 1–14. doi: 10.1186/s13068-015-0349-1
Shekh, A., Sharma, A., Schenk, P. M., Kumar, G., and Mudliar, S. (2022). Microalgae cultivation: photobioreactors, CO2 utilization, and value-added products of industrial importance. J. Chem. Technol. Biotechnol. 97, 1064–1085. doi: 10.1002/jctb.6902
Sibanda, T., and Buys, E. M. (2017). Resuscitation and growth kinetics of sub-lethally injured Listeria monocytogenes strains following fluorescence activated cell sorting (FACS). Food Res. Int. 100, 150–158. doi: 10.1016/j.foodres.2017.08.020
Sivaramakrishnan, R., and Incharoensakdi, A. (2017). Enhancement of lipid production in Scenedesmus sp. by UV mutagenesis and hydrogen peroxide treatment. Bioresour. Technol. 235, 366–370. doi: 10.1016/j.biortech.2017.03.102
Sivaramakrishnan, R., and Incharoensakdi, A. (2023). UV mutagenesis followed by hydrogen peroxide treatment ameliorates lipid production and omega-3 fatty acids levels in Chlorella sp. Algal Res. 74:103195. doi: 10.1016/j.algal.2023.103195
Skeffington, A. W., and Scheffel, A. (2018). Exploiting algal mineralization for nanotechnology: bringing coccoliths to the fore. Curr. Opin. Biotechnol. 49, 57–63. doi: 10.1016/j.copbio.2017.07.013
Slocombe, S. P., Huete-Ortega, M., Kapoore, R. V., Okurowska, K., Mair, A., Day, J. G., et al. (2021). Enabling large-scale production of algal oil in continuous output mode. Iscience 24:102743. doi: 10.1016/j.isci.2021.102743
Sonmez, M. E., Altinsoy, B., Ozturk, B. Y., Gumus, N. E., and Eczacioglu, N. (2023). Deep learning-based classification of microalgae using light and scanning electron microscopy images. Micron 172:103506. doi: 10.1016/j.micron.2023.103506
Sundaram, T., Rajendran, S., Gnanasekaran, L., Rachmadona, N., Jiang, J.-J., Khoo, K. S., et al. (2023). Bioengineering strategies of microalgae biomass for biofuel production: recent advancement and insight. Bioengineered 14:2252228. doi: 10.1080/21655979.2023.2252228
Sydney, T., Marshall-Thompson, J.-A., Kapoore, R. V., Vaidyanathan, S., Pandhal, J., and Fairclough, J. P. A. (2018). The effect of high-intensity ultraviolet light to elicit microalgal cell lysis and enhance lipid extraction. Metabolites 8:65. doi: 10.3390/metabo8040065
Tan, J. S., Lee, S. Y., Chew, K. W., Lam, M. K., Lim, J. W., Ho, S.-H., et al. (2020). A review on microalgae cultivation and harvesting, and their biomass extraction processing using ionic liquids. Bioengineered 11, 116–129. doi: 10.1080/21655979.2020.1711626
Thakur, A., and Konde, A. (2021). Fundamentals of neural networks. Int. J. Res. Appl. Sci. Eng. Technol. 9, 407–426. doi: 10.22214/ijraset.2021.37362
Tornabene, L., Valdez, S., Erdmann, M., and Pezold, F. (2015). Support for a “Center of Origin” in the Coral Triangle: cryptic diversity, recent speciation, and local endemism in a diverse lineage of reef fishes (Gobiidae: Eviota). Mol. Phylogenet. Evol. 82, 200–210. doi: 10.1016/j.ympev.2014.09.012
Trovão, M., Schüler, L. M., Machado, A., Bombo, G., Navalho, S., Barros, A., et al. (2022). Random mutagenesis as a promising tool for microalgal strain improvement towards industrial production. Mar. Drugs 20:440. doi: 10.3390/md20070440
Udayan, A., Pandey, A. K., Sirohi, R., Sreekumar, N., Sang, B.-I., Sim, S. J., et al. (2023). Production of microalgae with high lipid content and their potential as sources of nutraceuticals. Phytochem. Rev. 22, 833–860. doi: 10.1007/s11101-021-09784-y
Udayan, A., Sirohi, R., Sreekumar, N., Sang, B.-I., and Sim, S. J. (2022). Mass cultivation and harvesting of microalgal biomass: current trends and future perspectives. Bioresour. Technol. 344:126406. doi: 10.1016/j.biortech.2021.126406
Uzlasir, T., Selli, S., and Kelebek, H. (2023). Effect of salt stress on the phenolic compounds, antioxidant capacity, microbial load, and in vitro bioaccessibility of two microalgae species (Phaeodactylum tricornutum and Spirulina platensis). Foods 12:3185. doi: 10.3390/foods12173185
Varela Villarreal, J., Burgués, C., and Rösch, C. (2020). Acceptability of genetically engineered algae biofuels in Europe: opinions of experts and stakeholders. Biotechnol. Biofuels 13, 1–21. doi: 10.1186/s13068-020-01730-y
Vigeolas, H., Duby, F., Kaymak, E., Niessen, G., Motte, P., Franck, F., et al. (2012). Isolation and partial characterization of mutants with elevated lipid content in Chlorella sorokiniana and Scenedesmus obliquus. J. Biotechnol. 162, 3–12. doi: 10.1016/j.jbiotec.2012.03.017
Villanova, V., and Spetea, C. (2021). Mixotrophy in diatoms: molecular mechanism and industrial potential. Physiol. Plant. 173, 603–611. doi: 10.1111/ppl.13471
Wan Afifudeen, C. L., Teh, K. Y., and Cha, T. S. (2022). Bioprospecting of microalgae metabolites against cytokine storm syndrome during COVID-19. Mol. Biol. Rep. 49, 1475–1490. doi: 10.1007/s11033-021-06903-y
Wang, L., and Zhang, B. (2022). “Cultivation of microalgae on agricultural wastewater for recycling energy, water, and fertilizer nutrients,” in Integrated Wastewater Management and Valorization Using Algal Cultures (Amsterdam: Elsevier), 235–264.
Wang, L. R., Zhang, Z. X., Nong, F. T., Li, J., Huang, P. W., Ma, W., et al. (2022). Engineering the xylose metabolism in Schizochytrium sp. to improve the utilization of lignocellulose. Biotechnol. Biofuels Bioprod. 15:114. doi: 10.1186/s13068-022-02215-w
Wang, S. B., Chen, F., Sommerfeld, M., and Hu, Q. (2005). Isolation and proteomic analysis [corrected] of cell wall-deficient Haematococcus pluvialis mutants. Proteomics 5, 4839–4851. doi: 10.1002/pmic.200400092
Williams, S. L., Ambo-Rappe, R., Sur, C., Abbott, J. M., and Limbong, S. R. (2017). Species richness accelerates marine ecosystem restoration in the Coral Triangle. Proc. Natl. Acad. Sci. U. S. A. 114, 11986–11991. doi: 10.1073/pnas.1707962114
Wu, Z., Chen, G., Chong, S., Mak, N., Chen, F., and Jiang, Y. (2010). Ultraviolet-B radiation improves astaxanthin accumulation in green microalga Haematococcus pluvialis. Biotechnol. Lett. 32, 1911–1914. doi: 10.1007/s10529-010-0371-0
Xie, Y., Khoo, K. S., Chew, K. W., Devadas, V. V., Phang, S. J., Lim, H. R., et al. (2022). Advancement of renewable energy technologies via artificial and microalgae photosynthesis. Bioresour. Technol. 363:127830. doi: 10.1016/j.biortech.2022.127830
Yang, N., Zhang, Q., Chen, J., Wu, S., Chen, R., Yao, L., et al. (2023). Study on bioactive compounds of microalgae as antioxidants in a bibliometric analysis and visualization perspective. Front. Plant Sci. 14:1144326. doi: 10.3389/fpls.2023.1144326
Yi, Z., Su, Y., Xu, M., Bergmann, A., Ingthorsson, S., Rolfsson, O., et al. (2018). Chemical mutagenesis and fluorescence-based high-throughput screening for enhanced accumulation of carotenoids in a model marine diatom Phaeodactylum tricornutum. Mar. Drugs 16:272. doi: 10.3390/md16080272
Yu, K. L., Show, P. L., Ong, H. C., Ling, T. C., Chen, W.-H., and Salleh, M. A. M. (2018). Biochar production from microalgae cultivation through pyrolysis as a sustainable carbon sequestration and biorefinery approach. Clean Technol. Environ. Pol. 20, 2047–2055. doi: 10.1007/s10098-018-1521-7
Zhang, L., Hu, T., Yao, S., Hu, C., Xing, H., Liu, K., et al. (2023). Enhancement of astaxanthin production, recovery, and bio-accessibility in Haematococcus pluvialis through taurine-mediated inhibition of secondary cell wall formation under high light conditions. Bioresour. Technol. 389:129802. doi: 10.1016/j.biortech.2023.129802
Zhang, R., Wu, H., Su, Y., Qiu, L., Ni, H., Xu, K.-M., et al. (2021). In-situ high-precision surface topographic and Raman mapping by divided-aperture differential confocal Raman microscopy. Appl. Surf. Sci. 546:149061. doi: 10.1016/j.apsusc.2021.149061
Zhao, D., Cheah, W. Y., Lai, S. H., Ng, E. P., Khoo, K. S., Show, P. L., et al. (2023). Symbiosis of microalgae and bacteria consortium for heavy metal remediation in wastewater. J. Environ. Chem. Eng. 2023:109943. doi: 10.1016/j.jece.2023.109943
Zhao, Q., and Huang, H. (2021). “Microalgae cultivation,” in Advances in Bioenergy (Amsterdam: Elsevier), 37–115.
Zhou, L., Duan, X., Li, K., Hill, D. R. A., Martin, G. J. O., and Suleria, H. A. R. (2023). Extraction and characterization of bioactive compounds from diverse marine microalgae and their potential antioxidant activities. Chem. Biodivers. 2023:e202300602. doi: 10.1002/cbdv.202300602
Keywords: microalgae, bioprospecting, ultraviolet (UV) mutagenesis, strain selection, artificial intelligence
Citation: Alzahmi AS, Daakour S, Nelson D, Al-Khairy D, Twizere J-C and Salehi-Ashtiani K (2024) Enhancing algal production strategies: strain selection, AI-informed cultivation, and mutagenesis. Front. Sustain. Food Syst. 8:1331251. doi: 10.3389/fsufs.2024.1331251
Received: 01 November 2023; Accepted: 31 January 2024;
Published: 23 February 2024.
Edited by:
Kuan Shiong Khoo, Yuan Ze University, TaiwanReviewed by:
Nova Rachmadona, Padjadjaran University, IndonesiaCopyright © 2024 Alzahmi, Daakour, Nelson, Al-Khairy, Twizere and Salehi-Ashtiani. This is an open-access article distributed under the terms of the Creative Commons Attribution License (CC BY). The use, distribution or reproduction in other forums is permitted, provided the original author(s) and the copyright owner(s) are credited and that the original publication in this journal is cited, in accordance with accepted academic practice. No use, distribution or reproduction is permitted which does not comply with these terms.
*Correspondence: Kourosh Salehi-Ashtiani, a3NhM0BueXUuZWR1
†These authors share senior authorship
Disclaimer: All claims expressed in this article are solely those of the authors and do not necessarily represent those of their affiliated organizations, or those of the publisher, the editors and the reviewers. Any product that may be evaluated in this article or claim that may be made by its manufacturer is not guaranteed or endorsed by the publisher.
Research integrity at Frontiers
Learn more about the work of our research integrity team to safeguard the quality of each article we publish.