- School of Marxism, Xian Jiaotong University, Shaanxi, China
Informatization, a vital element in economic development, plays a pivotal role in shaping rural areas by promoting efficient resource allocation and fostering the prosperity of high-income rural farmers. This research delves into the intricate relationship between informatization and the enhancement of farmers' income, revealing a variety of perspectives on the matter. The research explores three distinct angles that highlight the complexities of the relationship between informatization and the growth of income in rural areas. Through rigorous analysis, the study aims to provide insights into the interplay between informatization and farmers' income growth. It identifies challenges and opportunities in the process of rural digitalization, such as the incomplete industrial chain of agricultural digitalization and the need for enhanced agricultural market information platforms. The study also underscores the importance of increasing farmers' digital literacy as a means to broaden income growth avenues. In doing so, the research strives to contribute to a more balanced and equitable rural society in China. This investigation employs various research methodologies, including panel analysis and threshold effect analysis, to explore the multifaceted relationships between informatization and farmers' income growth. The findings of this study provide valuable insights for policymakers and stakeholders involved in the ongoing development of rural areas and in ensuring the equitable distribution of wealth in China.
1 Introduction
The 20th National Congress of the Communist Party of China (CPC) has highlighted the important aim of realizing shared prosperity by 2035. This goal is acknowledged as a vital duty for both the CPC and the entire nation. The Congress has identified that the primary hurdle to achieving this objective is centered around the development of rural areas, specifically in enhancing the living standards of the farming population. This recognition underscores the critical need to address rural development as a fundamental aspect in working toward the broader aspiration of shared prosperity (Jinping, 2022). Presently, the income of farmers in China is considerably lower than that of other segments of society, resulting in a significant wealth disparity between urban and rural areas. This situation is a matter of serious concern, not only for social stability but also for the realization of a consumer-oriented economy. To achieve a more balanced and equitable society, it is imperative to address this disparity. Jinping places great importance on the role of rural Information Technology (IT) in increasing farmers' incomes. He emphasizes the use of digital agriculture, the adoption of agricultural IT, and the establishment of rural e-commerce platforms as effective means to boost farmers' incomes (Jiang et al., 2020).
By 2035, the CPC, in the 20th National Congress, is determined to achieve “Shared Prosperity,” acknowledging the vital importance of enhancing rural areas, particularly in raising the living standards of the farming population. The disparity in income between urban and rural sectors poses a significant challenge to this objective, impacting social stability and economic growth. While substantial efforts have been made to integrate IT into rural areas and boost farmers' incomes, there are persisting challenges hindering the full realization of its potential. Issues such as incomplete agricultural digitalization, insufficient market information platforms, and limited digital literacy among farmers have restricted the efficacy of IT in rural development. Conflicting perspectives in academic research further complicate the understanding of the actual impact of informatization on farmers' incomes, leading to a “productivity paradox” in some instances while showing promising results in others.
Recent studies on the relationship between rural IT and the increase in farmers' income have primarily revealed three types of perspectives. The first viewpoint suggests that the actual efficiency of gains in increasing farmers' incomes through informatization is lower than expected, leading to a “productivity paradox” in informatization (Hongpeng et al., 2013; Aimin, 2015; Zhang et al., 2016; Qubo et al., 2019). The second perspective asserts that informatization has yielded substantial results in increasing farmers' income and has demonstrated a discernible impact (Hongpeng et al., 2013; Aimin, 2015). A third perspective underscores the significance of farmers' informatization literacy and the extent of rural IT as two pivotal factors influencing the effectiveness of informatization in elevating farmers' incomes. Furthermore, this perspective emphasizes that the effects of these factors may vary across different regions within China (Haibin and Li, 2015; Yang and Ziheng, 2018). These diverse perspectives reflect that academic research on the impact of IT on increasing farmers' incomes is still in the exploratory stage.
Pingda et al. (2022) illustrated that the application of science and technology in agricultural science boosted investment opportunities by up to 90% in 2019, signaling an upward trajectory in overall income (Pingda et al., 2022). Wei and Lu (2023) noted an increase in farmers' independence compared to the pre-internet era in China, coinciding with income growth (Wei and Lu, 2023). Jin et al. (2023) highlighted that while productivity advancements impact natural ecology due to population growth, the focus should be on adopting low-carbon and science-based agricultural practices rather than halting productivity. Research conducted in Shandong province by Kang et al. (2023) shed light on the impact of ICT utilization on the growth, performance, and delivery of vegetable farms. Additionally, Li et al. (2023) suggested that utilizing IT might help narrow the income gap between rural and urban areas. With the strong promotion of digital rural planning in 2019, the use of IT is a prerequisite for increasing agricultural production and increasing farmers' income in China's existing rural areas. For which the construction of rural networking infrastructure has been completed, and the hardware requirements for informatization1 have been met. According to the “50th Statistical Report on China's Internet Network Development,” by June 2022, a total of 1.854 million 5G base stations had been built and operationalized across the country, which has 5G coverage in counties and broadband connectivity in villages. The goal is to reach 58.8 percent rural Internet penetration, and 293 million rural Internet users (CINI Center, 2022). On the other hand, the introduction of policies such as the “14th Five-Year Plan to Promote Modernization of Agriculture and Rural Areas” and “Digital Rural Development Action Plan (2022–2025)”, party and government cadres in charge of the agricultural sector and agriculture-related enterprises attached great importance to IT. The use of relevant departments has increased the intensity of support for agricultural technology innovation, strengthening farmers' technical skills and software usage, which are prerequisites for the integration of IT in rural areas (Aimin, 2015).
However, it should also be noted that there are still many challenges in the ongoing process of rural digitalization. These include the incomplete industrial chain of agricultural digitalization, insufficient development of agricultural product market information platforms, and a deficiency in farmers' digital literacy. These factors have limited the channels for farmers to increase their income. Therefore, it is imperative to address these barriers to further enhance the role of IT in rural development and ensure equitable wealth distribution (Benqing and Hongzhi, 2022; Mingxian and Jiabin, 2023).
Furthermore, a complex relationship exists between the synergistic effects of multiple factors in the realm of digitalization, necessitating further exploration of its impacts. This paper employs a panel analysis, utilizing data from 31 Chinese provinces spanning 2010 to 2021, to comprehensively assess the real impact of Information Technology (IT) on farmers' income and delve into the factors influencing its effectiveness. Specifically, the study aims to: (1) Establish a theoretical model of Synergy Analysis (SA) to examine the effects of informatization on farmers' income growth, with a particular focus on rural informatization as a significant factor. (2) Develop a measurement index system for assessing rural informatization levels and conduct a fixed-effect model regression analysis to scrutinize the impact of digitalization on farmers' income growth. (3) Perform a regression analysis using the Spatial Durbin Model to evaluate the impact of IT on farmers' income growth, considering time and regional heterogeneity from 2010 to 2021. (4) Conduct a threshold effect test on control variables related to social environmental constraints, such as “urbanization rate,” “local government financial support for agriculture,” and “agricultural science and technology innovation research and Development (R&D) funds,” analyzing their non-linear impact.
The research identifies crucial gaps related to challenges in numerous rural areas, emphasizing constraints such as low literacy rates, insufficient familiarity with household technological tools, and the farmers' ability to leverage technological information. Additionally, disparities in financial support from local governments across different regions act as barriers to rural digitization, impeding income growth across the 31 provinces. The study delves into investigating the long-term sustainability and resilience of digital farming practices in enhancing farmers' income, aiming to comprehend the durability of income gains resulting from informatization over extended periods and changing market conditions. Furthermore, it involves evaluating existing policies and proposing potential interventions or frameworks to maximize the positive impact of informatization on farmers' income, conducting policy assessments at local, provincial, and national levels. Addressing these research gaps holds the potential to provide a more nuanced understanding of the intricate relationship between informatization, digital farming, and the income of rural farmers in China, ultimately facilitating the development of more effective strategies and policies for sustainable agricultural development.
2 Theoretical model
Continuously improving the level of rural IT is an important prerequisite for effectively boosting farmers' incomes through optimal utilization of agricultural resources and smooth operation of the entire agricultural value chain (Yuezhou and Junnan, 2015; Benqing and Hongzhi, 2022; Mingxian and Jiabin, 2023). In recent years, China's digital village strategy has boosted rural informatization, enabling IT to enhance agricultural productivity. This technology has improved the allocation of resources and information dissemination in agricultural markets, thereby increasing farmers' real income. Consequently, IT advancements have elevated Total Factor Productivity (TFP) in agriculture, closing the urban-rural income gap (Yuezhou and Junnan, 2015).
The rapid global development of IT and its pervasive role in various sectors necessitates the effective management of data collection, retrieval, processing, and storage to drive economic development (Kalinina, 2008). Following the principles of collaborative governance, in the era of informatization, the coordination of multiple elements is leveraged to enhance the overall efficiency of the governing system (Guisheng and Mingchen, 2022). The researchers discovered the effectiveness of the informatization model for cross-regional poverty alleviation cooperation (Xiaohui, 2020). A clear example of a comprehensive analysis involves examining the effectiveness of implementing IT in increasing farmers' income. Specifically, rural informatization stands out as the most significant factor influencing the rise in farmers' income.
The paramount factor influencing the growth of farmers' income is the degree of rural informatization. This enhancement is achieved through the effective utilization of IT in rural agriculture. The aim of increasing farmers' income and fostering integrated urban-rural development can be realized through the synchronized use of IT. This assertion finds robust support from multiple researchers, who contend that elevating the level of rural IT has the potential to substantially improve the agricultural sector. It can invigorate rural markets, facilitate cross-regional product circulation, and diminish the urban-rural income disparity. In the long term, these advancements can lead to a significant increase in total rural social productivity, potentially up to 51% (Hui et al., 2022).
In a 2022 study, Huang et al. analyzed 9 years of panel data from 30 provinces (2011–2020). They observed that the rise in rural informatization substantially enhances resource utilization and allocation to production factors like land, capital, and labor. This progress is linked to a narrowing income gap between urban and rural regions, driven by the “IT level → allocation → urban-rural integration and development” transmission mechanism (Yongchun et al., 2022).
In a distinct study, Ningze and Jing (2019) investigated the influence of the Internet on farmers' income through an analysis of data from the China General Social Survey (CGSS). Their findings revealed that the utilization of the Internet by farmers led to a significant increase of 41.2–51.1% in non-agricultural income (Ningze and Jing, 2019).
Wei (2022) conducted a study on Internet broadband access and mobile phone usage in 86 counties of the Inner Mongolia Autonomous Region from 2015 to 2019, examining its correlation with farmers' disposable income. The research revealed that for each 1% increase in Internet broadband access, farmers' income increased by 0.11%. Similarly, for each 1% increase in mobile phone usage, farmers' income increased by 0.07%. Additionally, for each 1% increase in the county's Internet broadband access rate, the total agricultural output value of the county increased by 0.14% (Wei, 2022).
Empirical evidence demonstrates that regions in rural China with higher levels of IT are more effective in elevating farmers' incomes through IT adoption. This, in turn, contributes to increased disposable incomes for farmers and a reduction in the income disparity between urban and rural areas. For instance, in 2021, Zhejiang Province ranked second in the country in terms of per capita disposable income, reaching 35,247 Yuan. Simultaneously, Zhejiang Province succeeded in narrowing the income gap between urban and rural areas. These findings suggest that enhancing IT can significantly boost the real income of farmers and decrease the urban-rural income gap.
3 Research hypothesis
H1, positing that the enhancement of rural IT significantly contributes to an increase in farmers' income.
H2, variations exist in the level of rural informatization among different regions or provinces.
H3, Hypotheses related to the impact of social environmental constraints on the relationship between informatization and the growth of farmers’ income include H31, H32, and H33.
H31: The urbanization rate significantly moderates the effect of informatization on farmers' income growth, with higher urbanization rates strengthening this relationship.
H32: The level of financial support provided by local governments for agriculture significantly moderates the impact of informatization on farmers' income growth, with increased financial support enhancing this relationship.
H33: R&D funds for agricultural technology innovation significantly moderate the effect of informatization on farmers' income growth, with higher R&D investment strengthening this relationship.
3.1 Regional disparity level of rural China in IT
The promotion of balanced economic development and the establishment of digital villages greatly hinges on rural informatization. In 2022, Beijing launched the “Beijing NongTong” pilot project, utilizing advanced technologies such as 5G, the Internet of Things, and remote sensing to facilitate smart agriculture through IT integration. Jiangxi Province has established comprehensive digital villages at various administrative levels and plans to create more in pilot counties. Shaanxi Province is actively developing the digital agriculture industry in 2022. It launched the “National Digital & Animal Husbandry Innovation” platform for arid areas and aims to build 50 provincial-level agricultural smart parks and 100 provincial-level agricultural Internet of Things applications by 20252.
There are still hurdles in the way of simultaneous rural informatization. Provincial governments have differing perspectives on its planning and implementation. There are differences among government cadres regarding its urgency, and information availability is also a concern. Relevant studies conducted by Zhichuan et al. (2018) used rural broadband access users as a measurement of the level of rural informatization in 31 provinces. The results show that the level of rural informatization in China is decreasing from eastern to central to western to northern to southwestern regions (Zhichuan et al., 2018).
Junjie (2022) employed the entropy-weight TOPSIS method and utilized “network coverage level” as the core indicator to measure the level of rural informatization in 30 provinces of China. The study found that the score of rural informatization in China was 0.009 in 2010, 0.012 in 2015, and 0.026 in 2020. This indicates that regional disparity based on informatization is decreasing over time (Junjie, 2022).
3.2 Impact of economic constraints on IT-led income growth of farmers
China's agricultural industry significantly influences its GDP, and in turn, the state of the economy impacts the agricultural sector. Economic constraints play a pivotal role in shaping the dynamics and evolution of the agricultural industry. In recent years, the widespread integration of IT in agriculture has transformed the sector from a traditional endeavor into a more intensive, large-scale, and industrialized business. Official statistics reveal that the agricultural sector, along with its related industries, contributed to a total value of 18.4 trillion yuan, accounting for ~16.05% of China's GDP in 2021.
The integration of agriculture with other industries necessitates IT support to overcome the confines of the traditional agricultural sector. The ongoing refinement of the agricultural industrial structure significantly influences the increase in farmers' income, and several empirical studies have substantiated the concept of technological integration with agriculture. Informatization can empower the high-quality development of agriculture through two mediating variables: industrial structure upgrading and industrial structure rationalization. In particular, the upgrading of industrial structure can yield a positive facilitating effect of 1.064%, while rationalizing industrial structure can contribute a 0.033% positive impact (Zhaoyang and Yutong, 2022).
A study conducted by Liangjiao (2018) examined the effectiveness of “Internet Plus Agriculture” in improving the agricultural industrial structure in 30 provinces over the period from 2012 to 2016 using the maximum order difference method. The study revealed that the western region exhibited the highest average growth rate contribution at 87.8%, followed by the central region at 80.9%, and the eastern region at 47.4% (Liangjiao, 2018).
This paper considers three indicators as social environmental constraints: the urbanization rate, local government financial support for agriculture, and agricultural R&D funds.
3.3 Impact of informatization on urbanization
Wenting and Zhibiao (2022) conducted a panel analysis on 106 cities in nine provinces in the Yangtze River Economic Belt from 2013 to 2018. They found that the level of rural informatization has a non-linear effect on farmers' income growth. Specifically, the urbanization rate indicator exhibits a threshold effect. When the urbanization rate exceeds 62.82%, it results in a 0.61% increase in farmers' income. In the range of 57.22–62.82% urbanization, it has a positive but statistically insignificant effect on farmers' income. However, when the urbanization rate falls below 57.22%, it has a negative impact on farmers' income growth (Wenting and Zhibiao, 2022). Wuke (2022) conducted an analysis of panel data from Henan Province covering the period from 1998 to 2017. Their findings indicate that for every 1% increase in the level of urbanization, the per capita income of rural residents would increase by 1.204% in the same year and by 1.1067% in the following year (Wuke, 2022).
3.4 Impact of government financial aid on agriculture and informatization
Considering that agriculture is a capital-intensive industry marked by low returns and high natural risks, the support provided by local governments through financial means plays a pivotal role in fostering rural economic development (Lipton, 1976; Ping and Zhong, 2006), enhancing farmers' income (Shen and Jincai, 2022), and alleviating absolute rural poverty (Akanfe et al., 2019).
Junyong and Lezhu (2022) assessed China's financial support for agriculture using the Data Envelopment Analysis (DEA) model from 2008 to 2019. The study revealed that local government financial support for agriculture had a substantial 10% impact on the growth of farmers' income (Junyong and Lezhu, 2022). Likewise, a study by Hongxia (2021) highlighted significant regional variations in the impact of local government financial support for agriculture. The Eastern region exhibited the highest agricultural industry productivity, the Central region demonstrated the most pronounced influence of financial support for agricultural digitization, and the Western region displayed the greatest level of financial support for agricultural technology innovation (Hongxia, 2021).
3.5 The impact of R&D its innovation on agriculture
Scientific and technological innovation is a crucial factor in driving the high-quality development of agriculture. Enhancing cereal seeds and agricultural production practices can effectively boost farmers' income. Agricultural science and technology innovation, as well as research and development (R&D) investment in agriculture, serve as indicators to assess the influence of agricultural science and technology innovation on farmers' income. Yang Yiwu (2018) examined the connection between “agricultural science and technology innovation and R&D investment” and farmers' per capita disposable income from 2002 to 2013. Their study revealed that agricultural science and technology innovation has a positive impact on increasing farmers' income. However, it exhibits a threshold effect based on geographical distance (Yang Yiwu, 2018). These findings emphasize the significance of farmers' proximity to sources of technological innovation as a determining factor in the effectiveness of such interventions for enhancing farmers' incomes. In a study conducted by Yang (2022), an analysis was performed on the correlation between the advancement of agricultural science and technology in China's potato industry, investment in scientific and technological R&D funds, and farmers' income spanning from 2011 to 2018. The study revealed the presence of a threshold effect between the “level of agricultural science and technology progress,” “R&D investment in science and technology,” and “farmer household income.” When the level of agricultural science and technology development is <0.094, an increase in R&D funds can positively impact farmers' income. When it ranges from 0.094 to 0.9970, an increase in R&D input has a dampening effect on farmers' income. However, when the level of agricultural science and technology development is >0.9970, an increase in R&D input can significantly boost farmers' income (Yang, 2022).
3.6 The effects of informatization on farmers' income growth: an overview
Farmers are the most important actors in agricultural production, and their skill levels are also crucial factors affecting their ability to utilize IT. These factors, in turn, determine the ultimate impact of rural IT on increasing farmers' incomes (Haibin and Li, 2015; Yang and Ziheng, 2018).
The use of IT in rural areas to increase farmers' income is a recent approach to agricultural development that depends on farmers' skills in using technology, which, in turn, predicts their level of human capital. Unfortunately, the current situation suggests that a substantial number of farmers in rural areas have limited awareness and skills in the use of IT, which hinders their ability to increase income and improve their economic status. The academic community commonly uses a composite index of educational attainment to measure the level of rural human capital (Haibin and Li, 2015).
Haibin and Li (2015) divided rural human capital into high, medium, and low levels according to the comprehensive index of educational attainment. The research found that high and medium levels of rural human capital can achieve the effect of increasing farmers' income through informatization, while low levels of rural human capital do not have a significant effect on farmers' income growth (Haibin and Li, 2015). Shenglong et al. (2021) analyzed 30,993 observations from the Peking University Social Survey Center's China Family Tracking Survey (CFPS) in 2010, 2014, and 2018 and found that Internet use in rural areas can increase farmers' disposable income by 0.7253. Farmers with <6 years of primary school education have the lowest return on income from Internet use compared to farmers with more than 12 years of education, such as high school education or above (Shenglong et al., 2021). The hypotheses H31, H32, and H33 suggest that social environmental factors like urbanization, financial backing, and technological innovation funding may impact the connection between informatization and the growth of farmers' income.
4 Research design
4.1 Model structuring
According to the theoretical model of convergence analysis synergy analysis (SA), the process of increasing farmers' income through informatization primarily involves information as a crucial production factor, leading to the development of rural knowledge and rural IT. Economic constraints, social environmental constraints, and individual farmers' constraints are being addressed. In the context of limited production capacity, farmers have an interactive effect on increasing overall agricultural productivity. Increasing capacity can lead to the goal of raising farmers' income.
By integrating the development of rural IT with the restrictive constraints of the economic environment, social environment, and farmers' individual ability, the total factor productivity of agriculture can be elevated, resulting in the growth of farmers' income. Therefore, the process of using IT to increase farmers' income involves a complex interaction between various environmental and individual factors to achieve the desired results. Based on the convergence theoretical analysis model and the SA theoretical analysis model of informatization, this paper constructs the following formula for constructing a model of farmers' income growth.
(1) Econometric statistical model (Equation 1)
Where Yt is the per capita disposable income of farmers in time period t, A0 is a constant independent variable, I is the level of rural informational technology, C is the economic environment constraint, i.e., “the share of agriculture in GDP”, K is the social environment constraint, i.e., “the urbanization rate”, “local government financial support to agriculture” and “R&D investment in agricultural science and technology innovation”, and L is the individual farmer capacity constraint, i.e., “the level of rural human capital”.
In order to reduce the error, we take the natural logarithm of both sides of Equation (2) to obtain an econometric model (Equation 2).
Enable Yt = InYt, A = InAO, Ct = InCt, Kt = InKt, Lt = InLt, It = InIt, brought into the model (Equation 2) to obtain model (Equation 3).
Considering the relationship to be measured for different years in 31 provinces, adding variable i to model (Equation 3) produces:
Model (Equation 4) is the econometric statistical model used in this paper, where A is a constant item, α, β, Y, and ∅ are, respectively the elasticity coefficients of the economic environmental constraint, social environmental constraint, the individual farmer's ability constraint and the level of rural IT, respectively.
5 Data sources and variables
5.1 Variable selection
5.1.1 Dependent variable
An important prerequisite for analyzing the impact of information on farmers' income growth is the selection of indicators that can accurately measure farmers' income growth. Some researchers have chosen farmers' per capita disposable income as an indicator to measure the increase in farmers' income. For example, Ru and Yewei (2022) used the per capita disposable income of farmers as the primary indicator to measure common prosperity among different regions in China. They discovered that the common prosperity index is relatively high in the eastern coastal areas, particularly in Shanghai, which stands out as the most prosperous with an index of 0.54. In contrast, the five northwestern provinces displayed a comparatively lower average index of 0.218. Additionally, rural informatization positively contributes to reducing the imbalance in development between regions (Ru and Yewei, 2022). Jing (2022) found that digital inclusive finance can significantly increase agricultural productivity. The proportion of agricultural product processing and e-commerce transactions also contributes to the per capita disposable income of farmers (Jing, 2022). Drawing on the above studies, this paper selects the per capita disposable income of farmers as dependent variable to measure the growth of farmers' income.
5.1.2 Independent variable
In this study, the level of rural informatization is the independent variable that significantly influences farmers' income growth. As demonstrated by researchers like Mingxian and Jiabin (2023), five indicators were selected (see Table 1), including the number of Internet broadband access per 100 households, the number of mobile phones per 100 households, per capita telecommunication business volume, cable TV household rate, and broadcast coverage. Principal component analysis was employed to generate a comprehensive index of rural informatization level, aiming to measure the effectiveness of informatization in driving farmers' income growth in Hunan Province from 2012 to 2020 (Mingxian and Jiabin, 2023).
Benqing and Hongzhi (2022) selected four indicators, including the number of rural broadband access users, the average annual mobile phone ownership per 100 rural households, the proportion of rural households' annual consumption expenditure on smart devices, the transaction volume of rural e-commerce, and applied factor analysis. The study revealed that an increase in each unit corresponds to an improvement in informatization, which contributes to the high-quality development of agriculture (Benqing and Hongzhi, 2022). Building on prior research, this paper uses the level of rural informatization as an independent variable to gauge farmers' income growth. Six indicators have been chosen to reflect rural IT infrastructure and residents' usage: radio program population coverage, rural TV program coverage, rural Internet broadband users, the ratio of color TV sets per 100 households (HH), computers per 100 HH, and mobile phones per 100 HH. To ensure an unbiased evaluation of rural informatization levels, we employ the Entropy Weighting Method (EWM) to eliminate human interference and account for dimensional differences (Zhu et al., 2020). To mitigate potential dimensional differences among various data indicators, we standardized the data from the aforementioned six indicators. Subsequently, we applied the Entropy Weighting Method (EWM) to compute the weight of each indicator, yielding the rural informatization levels for different provinces across the country (see Table 1). The following entropy method is used to calculate the Weight Model Equation 5:
In: Ej ≥ 0 like, Pij = 0, defined Ej = 0, Degree is Dj = 1 – Ej:
The Information redundancy is: Dj = 1 – Ej
The Coefficient calculation Equation (6) is:
5.1.3 Controlled variable
The ultimate impact of the informatization drive on increasing farmers' income growth is influenced by several environmental factors. This paper categorizes these factors into three types of controlled variables: economic constraints, social-environmental constraints (urbanization rate, local government financial support for agriculture, and agricultural science and technology innovation R&D funding), and farmers' individual capacity constraints (rural human capital level).”
1. Economic-environmental constraints, such as “the proportion of agriculture GDP.”
2. Socio-environmental constraints, including the urbanization rate, financial support for agriculture, and R&D funds for agricultural technology innovation.
3. Farmers' capacity, encompassing rural farmers' skills, the level of rural informatization, and these controlled variables, have created a linkage and integration effect that collectively influences the actual outcome of increasing farmers' income. This study constructs a theoretical analysis model based on the relationship between the above variables as shown in Figure 1 below.
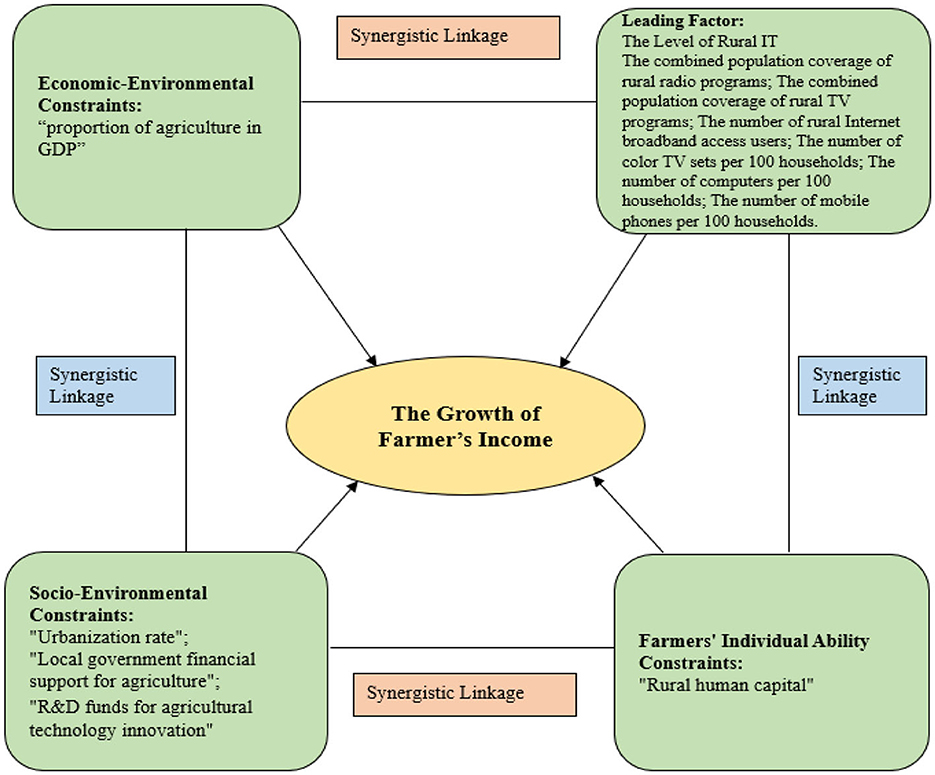
Figure 1. Synergistic analysis of the theoretical model of informatization driving farmers' income growth.
5.1.3.1 Explanation of SA model
The controlled variable representing the economic environment, “the proportion of agriculture in GDP,” is a commonly used indicator in academic circles. It reflects changes in China's economic structure, and this paper also utilizes this indicator for measurement. The controlled variable representing the social environment comprises three indicators: urbanization rate, local government financial support for agriculture, and agricultural science and technology innovation R&D funding input. Among these, the urbanization rate and agricultural science and technology innovation R&D funding input are commonly used indicators (Table 2). This study, like some scholars, uses the indicator of “expenditure on agriculture, forestry, and water management” to assess the influence of “local government financial support for agriculture” on local farmers' consumption (Xiao, 2022), the provincial poverty reduction ability (Yuqiang and Qun, 2020). Building on the composite index method (CI) employed by Haibin and Li (2015), the indicator for “Rural Human Capital,” representing the constraint on individual farmers' abilities, is calculated using the following formula: Rural Human Capital = (Number of individuals with no formal education × 0 + Number of individuals with primary school education × 6 + Number of individuals with middle school education × 9 + Number of individuals with high school or secondary school education × 12 + Number of individuals with college or undergraduate education or above × 16)/Total population aged 6 or older (Haibin and Li, 2015).
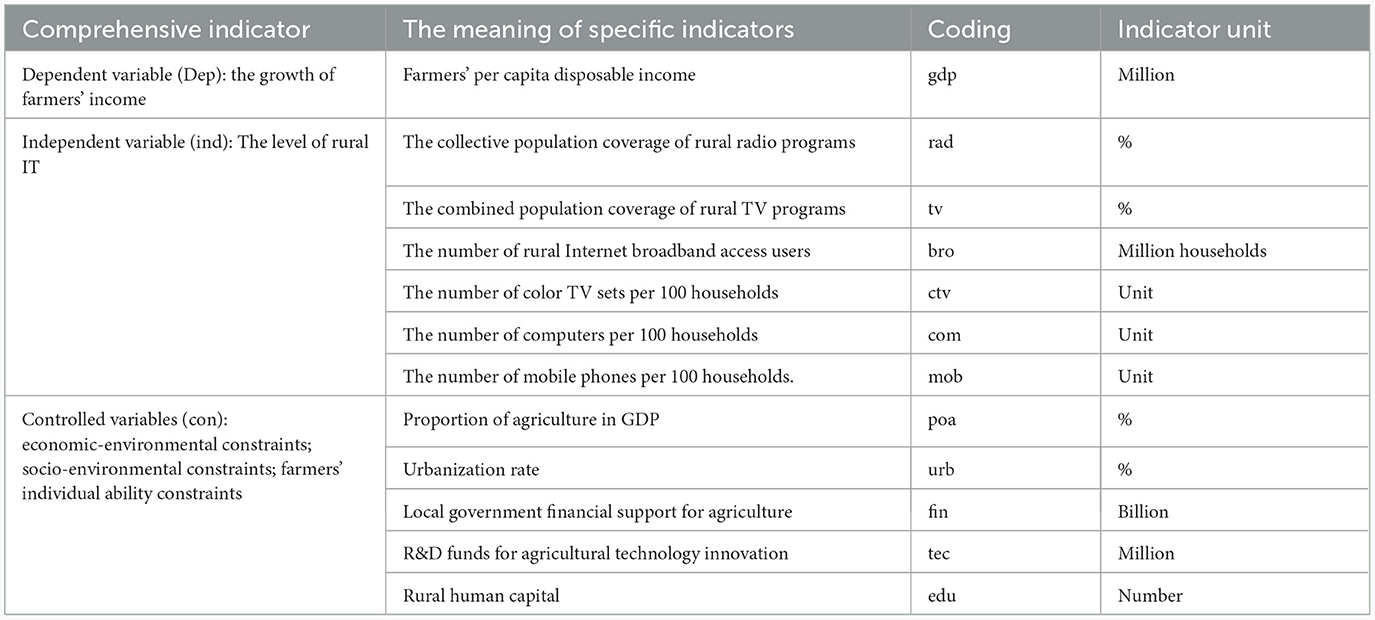
Table 2. Comprehensive index of variables: the specific indicator meanings and coding of the dependent, independent, and controlled variables in detail.
5.2 Data sources
Given the need for stable and available data, this paper establishes a time interval for the selection of dependent variables, independent variables, and control variables from 2010 to 2021. Panel data from 31 provinces (excluding Hong Kong, Macao, and Taiwan) has been sourced from publications such as the “China Statistical Yearbook,” “China Rural Statistical Yearbook,” “China Population and Employment Statistical Yearbook,” “China's Labor Statistical Yearbook,” and the National Bureau of Statistics.
5.3 ADF model, multicollinearity and endogeneity tests
5.3.1 Augmented Dickey-Fuller Test
To conduct the regression analysis of the benchmark model, an Augmented Dickey-Fuller (ADF) test was performed on the panel data of 31 provinces from 2010 to 2021 to assess time series robustness. The results, displayed in Table 3, reveal that all variables have ADF test p-values of <0.01, indicating strong confidence in rejecting the null hypothesis. Therefore, the selected panel data is robust and suitable for regression analysis.
5.3.2 Multicollinearity test
To ensure the accuracy of the regression analysis results and mitigate potential issues related to collinearity, this study conducted a variance inflation factor (VIF) test. The results, presented in Table 4 and Figure 2, reveal that all VIF values are below 3. This indicates the absence of multicollinearity among the variables and confirms the suitability of the statistical models for regression analysis.
5.3.3 Endogeneity Test
To assess the presence of potential causal relationships among the six selected variables, this study conducted a Durbin-Wu-Hausman (DWH) test using a random effect model. The findings, outlined in Table 5, highlighted the presence of endogeneity within the variables. However, considering that the endogeneity primarily arises from individual differences among the provinces, the study addressed this issue by using the individual fixed effect model for regression analysis on the sample data encompassing the 31 provinces from 2010 to 2021.
6 Results
6.1 Benchmark regression analysis results
To investigate the specific effects and influencing factors of rural informatization on driving the growth of farmers' income, this study utilized the individual fixed effect (FE) model for regression analysis of the indicator variables. The findings are showcased in Table 6, revealing a statistically significant relationship: an enhancement in rural informatization (IFO) notably correlates with an increase in the per capita disposable income of farmers. These results are significant at the 1% level, and the regression coefficient value is 1.05, which is greater than zero. This implies that rural informatization has a positive effect on increasing farmers' income, thus validating hypothesis H1. The variable “the proportion of agriculture in GDP” (poa) did not exhibit statistical significance, thus failing to establish research hypothesis H3. This suggests that alterations in the agricultural industrial structure do not significantly impact the outcome of rural informatization driving farmers' income growth. Furthermore, the variables “urbanization rate” (urb) and “agricultural science and technology innovation R&D investment (tec)” did not pass the significance test. However, the variable “local government financial support for agriculture” (fin) was statistically significant, indicating that an increase in local government financial expenditure on agricultural investment has a positive effect on increasing farmers' income. The comprehensive research hypotheses H31, H32, and H33 indicate that research hypothesis H3 is partly supported. This suggests that the relationship between social environmental constraints and the impact of rural informatization on farmers' income growth is not simply linear. Further research is needed to better understand the interactions among these three variables. The non-linear effect test revealed that there is a threshold effect. The regression coefficient of “rural human capital” (edu) is 0.204, which passes the significance test at the 1% level. This confirms the validity of research hypothesis H33, demonstrating that an improvement in the level of rural human capital significantly increases the per capita disposable income of farmers.
6.2 Spatio-temporal heterogeneity analysis of rural informatization
To assess the validity of research hypothesis H2, this study employs the spatio-temporal heterogeneity analysis approach in examining the coordinated development of rural informatization, as suggested by Yuxin et al. (2022), to examine the impact of rural informatization on the growth of farmers' income. For an accurate evaluation of rural informatization development, this paper initially standardized the pertinent data associated with rural informatization. Subsequently, it utilized SPSS 22.0 to analyze the main components influencing the scale of rural informatization across 31 provinces from 2010 to 2021. The selection process involved choosing indices with characteristic values exceeding 1 as the principal component factors of rural informatization. After conducting factor analysis from 2010 to 2021, it was observed that only the first factor had a characteristic value exceeding 1, while the second and third factors fell below this threshold. As a result, factor 1 was chosen for principal component analysis. Table 7 displays the data for the past 12 years, indicating that the characteristic values of factor 1 across the 31 provinces consistently exceeded 1, with high factor contribution rates and cumulative factor contribution rates exceeding 65%. Additionally, the KMO test value was above 0.6, and the data passed the Bartlett spherical significance test. Thus, this factor can effectively evaluate the level of rural informatization in China.
To further assess the variation in rural informatization among the 31 provinces between 2010 and 2021, this study applied data weighting methods to recalculate and categorize the rural informatization balance in these provinces. Consequently, it generated specific scores and rankings for each province in China. Due to space constraints, only the scores and rankings of rural informatization levels for the 5-year period from 2017 to 2021 are displayed in Figure 3.
Upon examining the data depicted in Figure 3, it is evident that the extent of rural informatization in all provinces has consistently increased each year. Simultaneously, there has been a reduction in the significant disparities in rural informatization, with the regional gap gradually narrowing from 4.54 to 3.65. These trends imply that regional disparities in rural informatization are gradually diminishing over the years. However, the scores of rural informatization in the 31 provinces still indicate the characteristics of imbalanced and uncoordinated regional development. The informatization level of Zhejiang, Jiangsu, Beijing, Shanghai, and Tianjin is high. Gansu, Guizhou, Xinjiang, Yunnan, Hainan, Tibet, and other six provinces the informatization is at a low level.
To investigate the temporal changes in the rural IT level across China, this study employs the fuzzy cluster analysis method as utilized by Suzhen et al. (2014) to cluster the IT levels of the 31 provinces from 2010 to 2021. By employing computer algorithms, the rural informatization levels in the 31 provinces are analyzed using two dimensions and six secondary indicators, focusing on the infrastructure development of rural informatization and the informatization of rural residents. This analysis generates a fuzzy equivalence relationship used for clustering and classifying, denoted as “R”. The obtained cluster classification fuzzy equivalence relationship value, “R~,” is employed to categorize the rural IT level of the 31 provinces into four regions: high level, higher level, medium level, and lower level regions. Table 8 illustrates the regional changes observed during the last 5 years (2017–2021).
From a spatial perspective, the regions with a high level of rural informatization are concentrated in the economically developed eastern areas. Provinces in this region have established informatization infrastructure and have essentially realized the goal of increasing farmers' income through informatization. These regions also exhibit high per capita disposable incomes for farmers, positioning them among the top in China. Meanwhile, areas with high levels of rural informatization are concentrated in some of the larger agricultural provinces in central China. The informatization infrastructure in these regions is comparatively well-established, and the fusion of informatization and modern agriculture is gaining momentum. The per capita disposable income of farmers in this region falls within the mid to upper range across the country. Exception for Heilongjiang Province, the provinces with a moderate level of rural informatization are primarily located in the central and western regions. The regional disparities have somewhat diminished due to financial investments in infrastructure development, and the per capita disposable income of farmers in these regions falls within the mid-level range nationwide. With the exception of Hainan Province, the provinces with lower levels of rural informatization are predominantly located in the northwest region. These areas face relatively challenging conditions in terms of rural information infrastructure, and the per capita disposable income of farmers in these regions ranks among the lowest in the country.
In order to further verify research hypothesis H2 and test for potential time series and regional differences in the impact of informatization on farmers' income growth, fixed effect regression analyses were conducted on panel data, considering different time intervals and levels of informatization across regions. The results are presented in Table 9 below. Analyzing the regression results in Table 9, concerning the temporal dimension, the time period from 2010 to 2013 did not yield significant results, while the time period from 2014 to 2021 did produce significant outcomes. When combined with Figure 2, we can discern the impact of informatization on farmers' income growth. The driving effect becomes more apparent after 2014, underscoring the significant role of China's implementation of the digital village strategy in increasing farmers' disposable income. Table 9 also provides regression test results for the four different areas of rural informatization levels. Table 10 indicates that the positive impact of increasing the level of rural informatization on farmers' income growth is observed in areas with high, middle, and low levels of rural informatization.
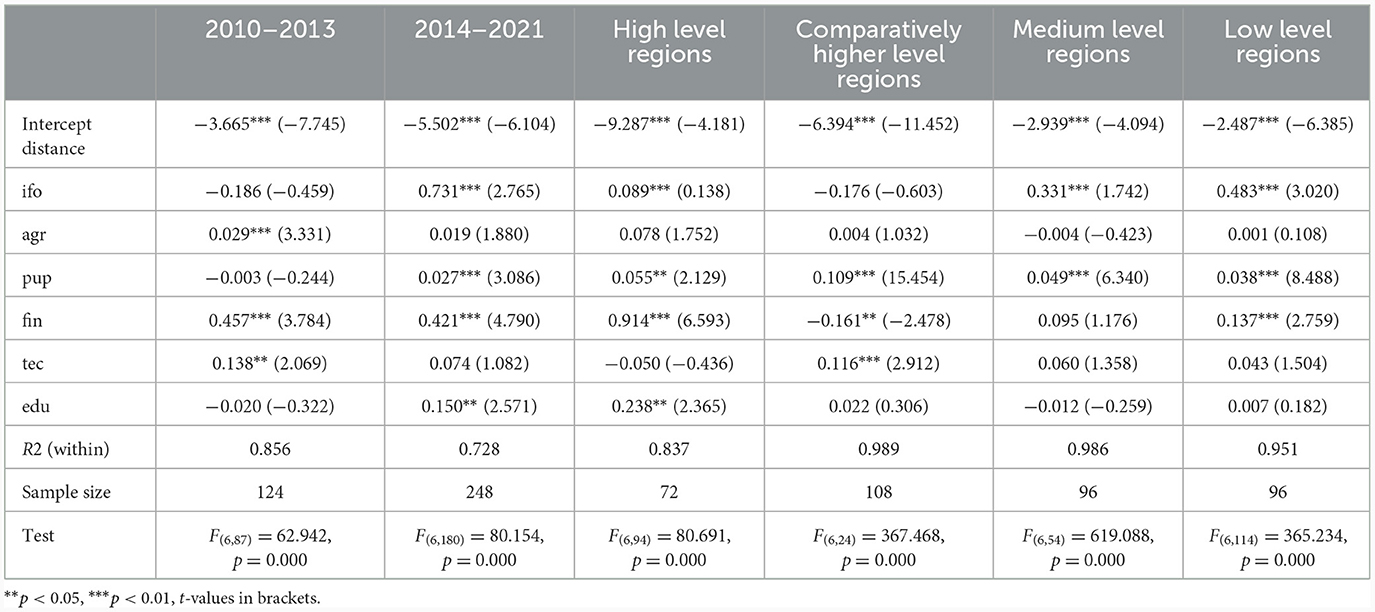
Table 9. Regression results of spatio-temporal heterogeneity of farmers' income growth driven by informatization.
The findings extracted from Table 9 indicate that the influence of elevating rural informatization levels on farmers' income growth is most pronounced in regions characterized by low rural informatization. This effect diminishes in medium-level areas and is least significant in high-level areas. In fact, high-level areas did not pass the significance test, and there is a negative correlation between rural informatization and farmers' income. These findings indicate that strengthening rural informatization development is not effective in increasing farmers' income in areas with high levels of informatization. Overall, the regression results for different time periods and horizontal areas of rural informatization support the research hypothesis H2 proposed in this paper.
6.3 Analysis of nonlinear effects
In order to investigate the non-linear effects of social environmental constraints and further test the validity of research hypotheses H3, H31, H32, and H33, this paper establishes single thresholds, double thresholds, and triple thresholds for the indicators “urbanization rate,” “local government financial support for agriculture,” and “agricultural science and technology innovation R&D funding.” These thresholds are used to test the existence of non-linear effects.
In this section, the study aims to assess the validity of research hypotheses H3, H31, H32, and H33 by examining the non-linear effects of socio-environmental constraint variables. To achieve this objective, single, double, and triple thresholds are defined to test the threshold effect on the “urbanization rate,” “local government financial support for agriculture,” and “R&D funds for agricultural technology innovation.” The threshold values for these three variables were estimated using the Bootstrap sampling method, and the results are presented in Table 11 below.
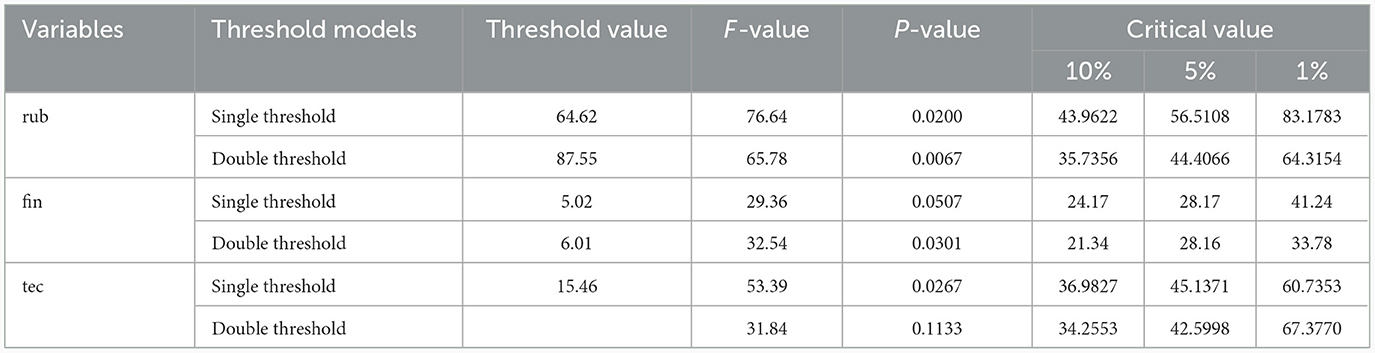
Table 11. Threshold test results of the controlled variables of rural informatization social environment (P-values and critical values are obtained by using Bootstrap repeated sampling 300 times).
Among these variables, “urbanization rate” and “local government fiscal support for agriculture” passed the double threshold test, while “agricultural technology innovation R&D funding” passed the single threshold test. The results indicate that among the controlled variables related to the social environment, “local government fiscal support for agriculture” significantly contributes to increasing farmers' income driven by informatization. However, there is an optimal range for the effects of “urbanization rate” and “agricultural science and technology innovation R&D funds.” These variables need to reach a certain threshold value to have a meaningful impact, confirming the establishment of the null hypotheses H31, H32, and H33.
The implementation of the national rural revitalization strategy, coupled with enhanced policy support and increased capital investment in rural industrial development, has improved the social environment for driving farmers' income growth through rural informatization. However, the social environment, as a complex controlled variable, exhibits a non-linear impact on the relationship between rural informatization and farmers' income growth. This study considered three indicators for regression analysis: “urbanization rate,” “local government financial support for agriculture ‘and' R&D funds for agricultural technology innovation.” The results of moderating variables (1), (2), and (3) in Table 10 reveal a positive non-linear relationship in the effect of informatization on farmers' income growth under the social environmental constraints of these threshold variables.
Analyzing the “urbanization rate” (urb) indicator, when the urbanization rate (urb) is below 64.62%, the regression coefficient for rural informatization's impact on farmers' income growth is 0.691. However, when the urbanization rate (urb) surpasses 64.62%, the regression coefficient increases to 1.446. As the urbanization rate (urb) continues to rise beyond 87.55%, the regression coefficient becomes −11.940. This suggests that moderate urbanization development can effectively increase farmers' disposable income, but an excessively high urbanization rate is not conducive to increasing farmers' income.
Regarding the “Local Government Fiscal Support to Agriculture” (fis) indicator, when the annual average local government financial support to agriculture (fis) is below 15.14 billion Yuan (the threshold value is based on the standardized processing of the original sample data), the impact of informatization on increasing farmers' income is not significant. However, when the expenditure (fis) exceeds 15.14 billion Yuan, the regression coefficient for informatization driving farmers' income growth rises to 0.243. As the expenditure (fis) continues to increase to 40.748 billion Yuan, the regression coefficient for informatization driving farmers' income growth further rises to 0.353. This indicates that “local government fiscal support for agriculture” exhibits characteristics of increasing marginal effects, and higher agricultural fiscal expenditure can effectively drive the growth of farmers' disposable income.
Regarding the “Agricultural Science and Technology Innovation R&D Expenditure” (tec) indicator, when the average R&D expenditure (tec) for the year is below 5.1784 million yuan (the threshold value was obtained after standardizing the original sample data and dimensionally restored here), the effect of informatization on increasing farmers' income is not significant. However, when it (tec) exceeds 5.1784 million yuan, the regression coefficient for informatization driving farmers' income growth rises to 1.018 (Table 10). This indicates that financial support for agricultural technology innovation can bring significant incremental marginal effects and effectively drive the growth of farmers' disposable income. The results of the non-linear effect analysis above show that the effect of informatization on increasing farmers' income will increase with the improvement of social environmental constraints, and present a non-linear feature of increasing marginal benefits. This shows that the local government's financial support and agricultural R&D investment should be further strengthened to create strong social environmental development conditions for the sustainable growth of farmers' income.
6.4 Robustness test
In order to ensure the stability of the above regression results, this paper has done the following robustness test. First, the core variables are subjected to a substitution test. Replace the dependent variable “per capita disposable income of farmers” with “per capita consumption expenditure of rural residents”; secondly, change the test period of the panel data. Replace 2010–2021 with 2014–2021 for inspection; again, perform shrinkage inspection on panel data. With reference to Jun and Xi's (2023) shrinking test method, the independent variable “rural informatization level” from 2010 to 2021 was shrinked at the 1% significance level, and regression analysis was performed on the remaining control variables (Jun and Xi, 2023). The robustness test results of these three methods are shown in Table 12. The significance of the main indicators and the sign of the coefficients in the regression results are consistent, which proves that the estimated regression results in this paper are robust.
6.5 Descriptive analysis of targeted provinces
To mitigate dimensional discrepancies among data indicators, this paper utilized logarithmic standardization for the relevant data. In analyzing regional disparities in rural informatization and its influence on farmers' income growth, the study classified the 31 provincial administrative units (excluding Hong Kong, Macao, and Taiwan) according to the economic region classification provided by the National Bureau of Statistics. To achieve this, the selected provinces were categorized into four regions: East, Central, West, and Northeast (see for results Figure 4). Figure 4 illustrates significant variations not only in the independent variable “rural informatization level” but also in the dependent variable “farmers” per capita disposable income' and the control variables “economic environment constraints, social environment constraints, and individual ability constraints” across the eastern, central, western, and northeastern regions. These disparities suggest regional differences in China, warranting further analysis of regional heterogeneity.
7 Conclusion
Since the 19th National Congress of the Communist Party of China, there has been a rapid acceleration in the digitalization of rural areas. Consequently, the level of informatization in China's rural areas has notably advanced. This progress has effectively promoted the optimal distribution of agricultural resources across urban and rural regions, facilitating the transmission and dissemination of agricultural market information across different times and locations. As a result, there has been a significant upsurge in the disposable income of farmers.
According to the results of this paper, the effect of rural informatization on increasing farmers' income is not a simple linear effect, but is affected by several controlled variables such as economic environment constraints, social environment constraints, and farmers' Individual capacity constraints are collective influencing factors. The Independent variable “rural informatization level” and the control variable of farmers' individual ability “rural human capital” will have a significant positive effect on increasing farmers' income, and the social environment control variables “urbanization rate,” “local government financial support for agriculture” and “Agricultural science and technology innovation R&D expenditure” has a threshold effect and shows the characteristics of increasing marginal effect, and the economic environment control variable “agriculture-to-GDP ratio” has a positive effect on farmers' income increase but has not passed the significance test.
First, the independent variable “rural informatization level” has a significant promotion effect on the dependent variable “farmers' per capita disposable income”, that is, for every 1 percentage point increase in the rural informatization level, the per capita disposable income of farmers will increase by 1.05 percentage points. Specifically, the economic environment constraint “the proportion of agriculture in GDP” did not pass the significance test, the three variables of the social environment constraint had nonlinear threshold effects, and the farmers' individual ability “rural human capital” had a significant positive effect. To promote the effect, every increase in the number of years of education received by farmers can increase the per capita disposable income of farmers by 0.204 percentage points.
Second, from 2010 to 2021, the results of farmers' income increase in China's 31 provinces showed heterogeneity. From the perspective of time series, the level of rural informatization plays a significant role in stimulating the growth of farmers' income from 2014 to 2021. From the perspective of the regional distribution of informatization level, the pulling effect of rural informatization level on farmers' income growth shows a significant difference of “low-level area > medium-level area > high-level area”, but the pulling effect of “higher-level area” is not obvious, and has a negative correlation effect on farmers' income.
Third, the controlled variables of the social environment have a threshold effect on the effect of informatization on farmers' income growth, and present a non-linear characteristic of increasing marginal benefits. Among them, “urbanization rate” and “local government financial support for agriculture” passed the double threshold test, and “agricultural science and technology innovation R&D funds” passed the single threshold test. When the “urbanization rate” is <64.62%, the regression coefficient of farmers' income growth driven by informatization is 0.691, and when the “urbanization rate” is >64.62%, the regression coefficient rises to 1.446. When the “local government fiscal support for agriculture” is <15.14 billion yuan per year, the effect of informatization driving farmers' income growth is not significant. When it is >15.14 billion yuan, the regression coefficient rises to 0.243, and when it continues to increase to more than 40.748 billion yuan, the regression coefficient continues to rise to 0.353. When the average annual “agricultural science and technology innovation R&D expenditure” is <5.1784 million yuan, the effect of informatization driving farmers' income growth is not significant. When it exceeds 5.1784 million yuan, farmers' income will increase significantly.
The overall contribution of the study outlined in the text lies in its multi-faceted approach to understanding and addressing the challenges impeding the successful integration of IT in rural China, specifically aimed at improving the livelihoods of the farming population. By conducting a comprehensive panel analysis utilizing data from various provinces over a substantial timeframe, the study endeavors to:
i) It aims to empirically assess the actual impact of IT on farmers' income. This empirical analysis, spanning multiple regions and years, will provide concrete evidence regarding the effectiveness of digitalization in rural areas.
ii) The study seeks to identify and analyze the factors that influence the efficacy of IT in rural development. This includes considering regional variations and understanding how different elements interact in the process of rural digitization.
iii) By acknowledging conflicting perspectives in existing academic research, the study aims to navigate the complexities surrounding the impact of informatization on farmers' incomes. This comprehensive analysis intends to offer clarity amidst the “productivity paradox” observed in some cases and promising outcomes in others.
iv) Ultimately, the study's findings are expected to yield valuable insights. These insights can inform policymakers, stakeholders, and development agencies about optimizing IT's role for equitable wealth distribution and sustainable rural development in China.
In essence, the study aspires to contribute substantial empirical evidence and nuanced understanding, shedding light on the intricate relationship between IT and rural income enhancement. Its findings have the potential to guide future strategies and initiatives aimed at bridging the rural-urban income gap, fostering social stability, and bolstering economic growth in China's agricultural regions.
7.1 Policy recommendations
Based on the above conclusions and the detailed findings outlined, here are some specific policy recommendations:
7.1.1 Investment in rural informatization
Encourage continued investment and efforts in the digitalization and informatization of rural areas. Prioritize enhancing the rural informatization level by deploying advanced technologies and infrastructure across agricultural sectors.
7.1.2 Focus on human capital development
Promote initiatives that focus on enhancing rural human capital, such as education and skill development programs for farmers. Increasing farmers' education levels can significantly boost per capita disposable income.
7.1.3 Strategic approach to social environment variables
Develop nuanced policies addressing social environment constraints. For instance, considering the thresholds identified in the study, tailor urbanization policies and increase financial support for agriculture, aligning with the identified inflection points to maximize the impact on farmers' income growth.
7.1.4 Optimizing agricultural innovation
Emphasize agricultural science and technology innovation through increased R&D expenditure. Ensure that the investment in innovation reaches a threshold level, identified in the study, to achieve significant growth in farmers' income.
7.1.5 Regional strategies for rural informatization
Acknowledge the regional disparities in the impact of rural informatization. Tailor strategies are based on regional differences, focusing on low and medium-level areas where the impact of informatization on income growth is more prominent.
7.1.6 Environmental considerations in productivity enhancement
Promote low-carbon agriculture and sustainable practices while enhancing productivity. Encourage the adoption of environmentally friendly agricultural methods to mitigate negative ecological impacts due to increased productivity.
7.1.7 Continuous monitoring and evaluation
Implement a system for ongoing monitoring and evaluation of the impact of rural informatization initiatives. This evaluation should consider the identified variables and their threshold effects to fine-tune policies for optimal results.
These policy recommendations aim to capitalize on the positive impact of rural informatization while addressing the nuanced variables influencing farmers' income growth in different contexts, thereby fostering sustainable and inclusive agricultural development across regions in China.
Data availability statement
The original contributions presented in the study are included in the article/supplementary material, further inquiries can be directed to the corresponding author.
Author contributions
DX: Conceptualization, Writing – original draft. ZJ: Data curation, Writing – original draft. GX: Investigation, Software, Writing – review & editing. MA: Writing – review & editing.
Funding
The author (s) declare that no financial support was received for the research, authorship, and/or publication of this article.
Conflict of interest
The authors declare that the research was conducted in the absence of any commercial or financial relationships that could be construed as a potential conflict of interest.
Publisher's note
All claims expressed in this article are solely those of the authors and do not necessarily represent those of their affiliated organizations, or those of the publisher, the editors and the reviewers. Any product that may be evaluated in this article, or claim that may be made by its manufacturer, is not guaranteed or endorsed by the publisher.
Footnotes
1. ^Informatization (INF) shares similarities with Industrialization and Civilization. It refers to the transformation of a geographical area's economy or society into an information-based entity, characterized by a growing information labor force. INF signifies a progression wherein emerging communication technologies are utilized to drive socio-economic development, leading a nation toward the evolution of an information society (Rogers, 2000).
2. ^Digital Countryside Promotion Route Map of 31 Provinces (2022). Available online at: https://www.163.com/dy/article/H9E6SV8J0511N341.html.
References
Aimin, W. (2015). Is there a “productivity paradox” in China's rural informatization? –A test based on a threshold panel regression model. China Soft Sci. 84:102318. doi: 10.1016/j.irfa.2022.102318
Akanfe, A. Y. S., Olusegun, A., Aderonke, N. I., and Modupe, O. (2019). Rural financing as a panacea for poverty alleviation: a study of selected micro and small enterprises in Ogun State. J. Bus. Econ. Dev. 4, 81–89. doi: 10.11648/j.jbed.20190403.12
Benqing, L., and Hongzhi, Y. (2022). Digital economy enables high-quality agricultural development: theoretical logic and empirical test. J. Jiangxi Univ. Finan. Econ. 2022, 95–107. doi: 10.13676/j.cnki.cn36-1224/f.2022.06.004
CINI Center (2022). China Internet Network Information Center Releases the 50th Statistical Report on the Development of the Internet in China. Available online at: https://kns.cnki.net/kcms2/article/abstract?v=3uoqIhG8C44YLTlOAiTRKibYlV5Vjs7iJTKGjg9uTdeTsOI_ra5_XTk1g17hd4CB3ajCZbwclLilmoxbziASVpqtmV6AlRsk&uniplatform=NZKPT (accessed June 15, 2022).
Guisheng, C., and Mingchen, X. (2022). Research on the collaborative construction of digital villages: an analysis based on the SFIC model. J. Party Sch. Fujian Prov. Committ. Commun. Party China 138–147. doi: 10.15993/j.cnki.cn35-1198/c.2022.01.016
Haibin, H., and Li, Z. (2015). Analysis of the threshold effect of agricultural informatization on total factor productivity growth in agriculture. China Rural Econ. 11–21. Available online at: https://kns.cnki.net/kcms2/article/abstract?v=3uoqIhG8C44YLTlOAiTRKibYlV5Vjs7ir5D84hng_y4D11vwp0rrtTmmr7EcXNeYBIshbWy5QFdjZDOPXEA99BpavYRE67JS&uniplatform=NZKPT
Hongpeng, D., Jie, L., and Endong, M. (2013). An empirical study on the contribution of informatization to agricultural economic growth–Liaoning Province as an example. Rural Econ. 99–101. Available online at: https://kns.cnki.net/kcms2/article/abstract?v=3uoqIhG8C44YLTlOAiTRKjw8pKedNdX5_mkCYmAjR9xJhoNEMBPSm6VuGP5tBJCnL5ksUsudrCh2FpbbJ0h2i11QqxpGHIjJ&uniplatform=NZKPT
Hongxia, L. (2021). Research on the synergy of financial and fiscal support to agriculture in the perspective of rural revitalization: based on DEA-Malmquist and Tobit models. Southwest Finan. 14–26. Available online at: https://kns.cnki.net/kcms2/article/abstract?v=3uoqIhG8C44YLTlOAiTRKibYlV5Vjs7iy_Rpms2pqwbFRRUtoUImHZl1QbF6btXzIkXgiFOEfGPSpglUt0Ffku4dytUXEHqI&uniplatform=NZKPT
Hui, Z., Ge, S., and Jalai, Z. (2022). Can the digital economy narrow the multidimensional gap between urban and rural areas? –A resource mismatch perspective. Mod. Finan. Econ. 42. doi: 10.19559/j.cnki.12-1387.2022.01.004
Jiang, L., Xinghua, Z., and Rui, C. (2020). A study on the threshold effect of digital economy to improve urban-rural income gap. Dev. Res. 105–113. doi: 10.13483/j.cnki.kfyj.2020.06.015
Jin, W., Wu, S., Zhang, Y., Zhou, G., Xu, L., and Xu, Y. (2023). Review on Chinese agricultural science and technology research from a low-carbon economy perspective: hotspots, evolution, and frontiers. Front. Environ. Sci. 11:1268432. doi: 10.3389/fenvs.2023.1268432
Jing, J. (2022). The impact of digital inclusive finance on rural industrial revitalization in the context of common wealth - an empirical evidence based on provincial panel data. Bus. Econ. Res. 177–180. Available online at: https://kns.cnki.net/kcms2/article/abstract?v=jBOcPZekd6NzEgwsTJZJZrwLqFsGyxcyxJCG5bLuvzJyN5WVTq-3g_V9b5iUU97aXYXaauTyqokIhELt8wThazea0Ui_0ow3KrkmPdjVjnmalgXK5r6_UEFcg8FAKndJ&uniplatform=NZKPT&language=gb
Jinping, X. (2022). Holding High the Great Banner of Socialism With Chinese Characteristics Uniting Struggles for the Comprehensive Construction of a Modern Socialist Country. Beijing: People's Daily.
Jun, W., and Xi, L. (2023). The inner mechanism and spatial spillover effect of digital economy on common wealth. Stat. Inform. Forum. 16–27. Available online at: https://kns.cnki.net/kcms2/article/abstract?v=3uoqIhG8C44YLTlOAiTRKibYlV5Vjs7ioT0BO4yQ4m_mOgeS2ml3UHL_aC2KtL3ut7gfOh8prMnIfwPcKcumYkRaAULHDk5e&uniplatform=NZKPT
Junjie, Z. (2022). Analysis of the spatial and temporal characteristics of China's agricultural and rural modernization development level and obstacle factors. Econ. Syst. Reform 87–94. Available online at: https://kns.cnki.net/kcms2/article/abstract?v=3uoqIhG8C44YLTlOAiTRKibYlV5Vjs7iJTKGjg9uTdeTsOI_ra5_XdFVn8lYnxeRXKo-jCkkqqIIhm0fqYIomaLiuBqN9yxt&uniplatform=NZKPT
Junyong, C., and Lezhu, Z. (2022). Fiscal and financial synergy in supporting rural industries: efficiency evaluation, empirical references and insights. Southwest Finan. 97–108. Available online at: https://kns.cnki.net/kcms2/article/abstract?v=3uoqIhG8C44YLTlOAiTRKibYlV5Vjs7iJTKGjg9uTdeTsOI_ra5_XUxnv8V6jDPOu7ADHTwy4Xl2_1i8sP54qbTqOtt_nAPX&uniplatform=NZKPT
Kalinina, A. E. (2008). Theoretical and methodological aspects in the development of information systems. Sci. Tech. Inf. Process. 35, 50–54. doi: 10.1007/s11984-008-1009-3
Kang, S., Ait Sidhoum, A., Frick, F., Sauer, J., and Zheng, S. (2023). The impact of information and communication technology on the technical efficiency of smallholder vegetable farms in Shandong of China. Q Open 3, qoad017. doi: 10.1093/qopen/qoad017
Li, H., Tian, H., and Rabbi, M. A. (2023). The impact of informatization on the urban-rural income gap: an empirical investigation of China. Inf. Dev. 35, 02666669231210839. doi: 10.1177/02666669231210839
Liangjiao, D. (2018). Research on the Development of “Internet+Agriculture” and Its Impact on Agricultural Structure Adjustment (Doctor). Zhejiang University of Technology and Business. Available online at: https://kns.cnki.net/kcms2/article/abstract?v=3uoqIhG8C475KOm_zrgu4lQARvep2SAkWfZcByc-RON98J6vxPv10Zl9yPZkOSReNBp_ssAgGcqg9SYCBp40ce3oY6jECesG&uniplatform=NZKPT (accessed June 15, 2022).
Lipton, M. (1976). Agricultural finance and rural credit in poor countries. World Dev. 4, 543–553. doi: 10.1016/0305-750X(76)90065-6
Mingxian, L., and Jiabin, H. (2023). Research on digital economy empowering high quality development of agriculture–analysis based on panel data of Hunan Province from 2012 to 2020. J. Hunan Agric. Univ. 24, 14–23. doi: 10.13331/j.cnki.jhau(ss).2023.01.003
Ningze, Y., and Jing, Z. (2019). Can Internet use contribute to the increase of farmers' non-farm income? – An empirical analysis based on China General Social Survey (CGSS) 2015 data. Econ. Surv. 41–48. doi: 10.15931/j.cnki.1006-1096.20190624.019
Ping, X., and Zhong, X. (2006). Public finance, financial support for agriculture and rural financial reform–an analysis of a survey based on Guizhou Province and its sample counties. Econ. Res. 106–114. Available online at: https://kns.cnki.net/kcms2/article/abstract?v=3uoqIhG8C44YLTlOAiTRKgchrJ08w1e7eWoVfj7plMwAGGnxmuiwW7VSUzgUMmziEeBAt-CyM4dsG8aXYAIJhVh0e1XuAJtB&uniplatform=NZKPT
Pingda, W., Wang, Z., and Zhong, H. (2022). The impact of agricultural information system on agricultural product trading efficiency using internet of things technology. Math. Prob. Eng. 2022:4061908. doi: 10.1155/2022/4061908
Qubo, Z., Junfei, B., Chao, P., and Chen, Z. (2019). Has information technology improved agricultural productivity? China Rural Econ. 22–40. Available online at: https://kns.cnki.net/kcms2/article/abstract?v=3uoqIhG8C44YLTlOAiTRKibYlV5Vjs7iLik5jEcCI09uHa3oBxtWoMrudmJ7cUEQer67TByCKHt1_49UAe5O89_RONN5_nXS&uniplatform=NZKPT
Rogers, E. M. (2000). Informatization, globalization, and privatization in the new Millenium. Asian J. Commun. 10, 71–92. doi: 10.1080/01292980009364785
Ru, L., and Yewei, Z. (2022). Digital economy and common prosperity-an empirical study based on spatial threshold effect. J. Southwest Univ. Nation. 90–99. Available online at: https://kns.cnki.net/kcms2/article/abstract?v=jBOcPZekd6Ng9qYHeyVN_1s7QOwgqrxori2z3fK1VgxpQggsJ1em1WjbMYMTrnD270I4efC6TqF8uwEPVlxnFmdDC80XpTXxNiixEqMnY2YxzTm4OR4i-P1ySdTxnawa&uniplatform=NZKPT&language=gb
Shen, L., and Jincai, Z. (2022). A study on the factors and pathways influencing the effectiveness of digital financial empowerment of rural entrepreneurship: a qualitative and comparative analysis based on 32 cases. Technol. Econ. 71–80. Available online at: https://kns.cnki.net/kcms2/article/abstract?v=3uoqIhG8C44YLTlOAiTRKibYlV5Vjs7iJTKGjg9uTdeTsOI_ra5_XQUPX74xNvY4B_qEE4TjZaxxSNDq5PUcTPwlxECfU407&uniplatform=NZKPT
Shenglong, L., Xiaoming, Z., and Zhusong, Y. (2021). The impact of internet use on the income of rural residents. Quant. Tech. Econ. Res. 103–119. doi: 10.13653/j.cnki.jqte.2021.04.006
Suzhen, W., Xia, Z., Haixia, R., and Yuwei, H. (2014). Research on fuzzy cluster analysis method and countermeasures for the current situation of informationization in new rural areas: the case of Hebei Province. Econ. Manage. 76–80. Available online at: https://kns.cnki.net/kcms/detail/13.1032.f.20141118.0943.014.html
Wei, S., and Lu, Y. (2023). How does internet use affect the farmers' trust in local government: evidence from China. Int. J. Environ. Res. Public Health 20:3489. doi: 10.3390/ijerph20043489
Wei, Z. (2022). The relationship between county informatization level and farmers' income growth under rural revitalization-based on panel data of 86 banner counties in Inner Mongolia. J. Inner Mongolia Univ. Finan. Econ. 48–55. doi: 10.13895/j.cnki.jimufe.2022.01.015
Wenting, S., and Zhibiao, L. (2022). Digital economy, urbanization and farmers' income increase - an empirical test based on the Yangtze River Economic Belt. Explor. Econ. Iss.
Wuke, G. (2022). Study on the relationship between new urbanization and farmers' income growth in Henan Province. China Agric. Resour. Zoning 42, 239–247. Available online at: https://kns.cnki.net/kcms2/article/abstract?v=f950eQlbaWlqCs__TqLYlPdvSDp6-QBIcJ3RVHV4z-0MZrYVcG4rEsFBKjN6LqGsITPEJCYmxpckiAuQLPWN09BjsgdrYCE4gnkTte65AWqxTtm7aTJuPslJCbK86BCas4-7hZj6PkOgLtWyWRVMIg==&uniplatform=NZKPT&language=CHS
Xiao, T. (2022). Research on the dynamic effect and threshold effect of financial support to agriculture on consumption upgrading of rural residents. Bus. Econ. Res. 41–45. Available online at: https://kns.cnki.net/kcms2/article/abstract?v=3uoqIhG8C44YLTlOAiTRKibYlV5Vjs7iJTKGjg9uTdeTsOI_ra5_XU4eSIAXbZLg3nYzdfcresIteeUM81MfpWTWVLvmPPn_&uniplatform=NZKPT
Xiaohui, J. (2020). How can poverty alleviation collaboration be optimized under cross-regional governance?–A changing picture based on Guangdong-Guizhou poverty alleviation collaboration. Lanzhou J. 153–167. Available online at: https://kns.cnki.net/kcms2/article/abstract?v=3uoqIhG8C44YLTlOAiTRKibYlV5Vjs7i8oRR1PAr7RxjuAJk4dHXotEq_999SYVq1T6WcJdD9at8TdmHlSqB1jezGkN13F2w&uniplatform=NZKPT
Yang Yiwu, L. W. (2018). Agricultural science and technology innovation, spatial linkages and farmers' income. Finan. Econ. Sci. 70–82. Available online at: https://kns.cnki.net/kcms2/article/abstract?v=Y7KVjSR9dMKNfmZTFdXHmq7TVRvDFc8XfgmWLZYLuyGc_wWoDcd01_CA7Tzpgpchs6hRzYzwuABZqtccu0lGw1lbcCkagKPWusObtL7ZXpC2dlWrAQ-BrawYLfLPz2nK&uniplatform=NZKPT&language=gb
Yang, G., and Ziheng, N. (2018). Agricultural informatization, spatial spillover effects and agricultural green total factor productivity-based on SBM-ML index method and spatial Durbin model. Stat. Inform. Forum 66–75. Available online at: https://kns.cnki.net/kcms2/article/abstract?v=jBOcPZekd6PBO_saWbdieC_Hfktd2J32J_xRX6hkGX7EajPuIfm7bkhSfDwKGBzTEFJmk6by7XVnb7z9kmDd0s7SayrrF51Zm1Kjd8RVe9XvMOujQv9h7mxUWO0_Ljsv&uniplatform=NZKPT&language=gb
Yang, S. (2022). Impact of agricultural science and technology progress on farmers' income-based on panel data in the main potato producing areas. J. Honghe Coll. 108–112. doi: 10.13963/j.cnki.hhuxb.2022.02.024
Yongchun, H., Shangjun, G., Chen, Z., Lin, J., and Zifei, X. (2022). Digital economy, factor allocation efficiency and urban-rural integration development. China Popul. Resour. Environ. 32, 77–87. Available online at: https://kns.cnki.net/kcms2/article/abstract?v=3uoqIhG8C44YLTlOAiTRKibYlV5Vjs7iJTKGjg9uTdeTsOI_ra5_XSjgKD7Wue3wKx5GFJlRmMrY1DvWvIYApUaDEwQnIYhd&uniplatform=NZKPT
Yuezhou, C., and Junnan, Z. (2015). The substitution and penetration effects of information and communication technologies on China's economic growth. Econ. Res. 12, 100–114. Available online at: https://kns.cnki.net/kcms2/article/abstract?v=3uoqIhG8C44YLTlOAiTRKibYlV5Vjs7ijP0rjQD-AVm8oHBO0FTadkumpNiFVFGCM_UEnCfZ7Ap6_TF-IraW4jTsPKrboZWp&uniplatform=NZKPT
Yuqiang, G., and Qun, S. (2020). Research on the poverty reduction efficiency of provincial financial support expenditure for agriculture in China. J. Central South Univ. For. Sci. Technol. 59–65, 100. doi: 10.14067/j.cnki.1673-9272.2020.01.010
Yuxin, X., Mijti, M., Ximing, Y., and Ruojie, G. (2022). Analysis of the spatial and temporal patterns of coordinated development of digital villages and the influencing factors. J. Lanzhou Univ. Finan. Econ. 87–94. Available online at: https://kns.cnki.net/kcms2/article/abstract?v=3uoqIhG8C44YLTlOAiTRKibYlV5Vjs7ioT0BO4yQ4m_mOgeS2ml3UC5dlgr1zfcTXGBTC9ZNOVD6RR1wtnCYL9u-HZjuc6df&uniplatform=NZKPT
Zhang, Y., Wang, L., and Duan, Y. (2016). Agricultural information dissemination using ICTs: a review and analysis of information dissemination models in China. Inf. Process. Agric. 3, 17–29. doi: 10.1016/j.inpa.2015.11.002
Zhaoyang, L., and Yutong, D. (2022). An empirical study of digital economy empowering high-quality agricultural development. China Circ. Econ. 36, 3–14. doi: 10.14089/j.cnki.cn11-3664/f.2022.11.001
Zhichuan, Z., Guochao, Z., Junyan, Z., and Xinxin, W. (2018). Research on the development level and spatial distribution differences of agricultural and rural modernization in China. Jiangsu Agric. Sci. 386–391. doi: 10.15889/j.issn.1002-1302.2018.19.096
Keywords: informatization, agriculture, digitalization, research and development, China, methodology
Citation: Xiaoyan D, Jiangnan Z, Xuelian G and Ali M (2024) The impact of informatization on agri-income of China's rural farmers: ways for digital farming. Front. Sustain. Food Syst. 8:1329674. doi: 10.3389/fsufs.2024.1329674
Received: 29 October 2023; Accepted: 22 January 2024;
Published: 07 February 2024.
Edited by:
Isabelle Piot-Lepetit, INRAE Occitanie Montpellier, FranceReviewed by:
Peng Jiquan, Jiangxi University of Finance and Economics, ChinaNawab Khan, Sichuan Agricultural University, China
Copyright © 2024 Xiaoyan, Jiangnan, Xuelian and Ali. This is an open-access article distributed under the terms of the Creative Commons Attribution License (CC BY). The use, distribution or reproduction in other forums is permitted, provided the original author(s) and the copyright owner(s) are credited and that the original publication in this journal is cited, in accordance with accepted academic practice. No use, distribution or reproduction is permitted which does not comply with these terms.
*Correspondence: Majid Ali, bWFqaWRrc2tAb3V0bG9vay5jb20=