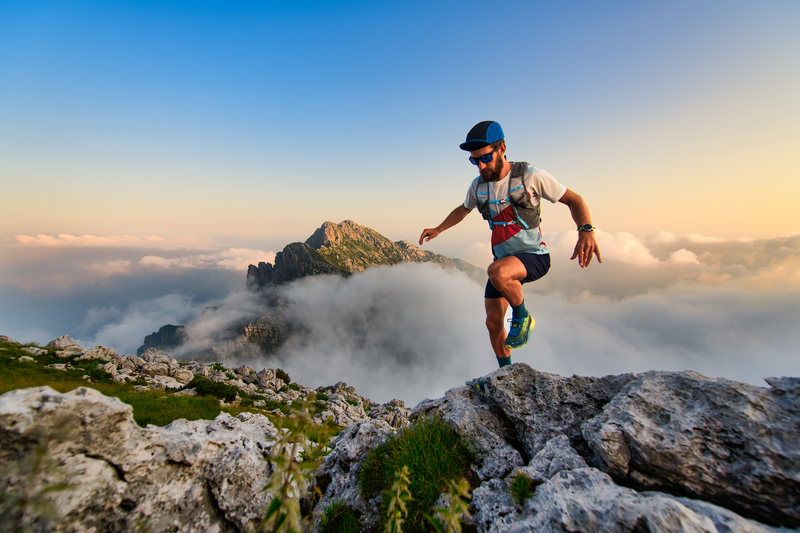
95% of researchers rate our articles as excellent or good
Learn more about the work of our research integrity team to safeguard the quality of each article we publish.
Find out more
ORIGINAL RESEARCH article
Front. Sustain. Food Syst. , 11 September 2024
Sec. Agro-Food Safety
Volume 8 - 2024 | https://doi.org/10.3389/fsufs.2024.1308394
Introduction: Enhancing the efficacy of online food safety supervision requires thoughtful selection and efficient application of regulatory measures.
Methods: This study examined the current state of online food safety supervision in the important food producing city in central China of Zhengzhou. The effectiveness of supervision frequency and penalty on food safety governance of online catering was examined through model construction based on the optimal law enforcement theory. The efficacy of monitoring was evaluated using real supervision and punishment data from the online catering sector in a Chinese new first-tier city.
Results and discussion: Results showed that although high-frequency and high-penalty supervision are two common methods of online food safety governance, the deterrent effect of high-frequency supervision on online food businesses is more significant for improving the supervision efficiency of the online catering market. Simultaneously, bolstering the education of caterers and food operators as well as raising their degree of training are also efficient ways to raise the efficacy of government oversight. The application of law enforcement economics is broadened in this study, which also has implication for the advancement of credit rating and classification supervision in the area of food safety for online catering.
Even though China’s regulatory bodies have tightened their grip on online food safety in recent years, government agencies still find it extremely challenging to monitor the commercial practices of online catering businesses because of the opaque nature of the Internet. The Chinese government has released pertinent documents in recent years to encourage front-line food safety regulators to restrict illegal activities of online catering enterprises through random checks, penalties, and other means. The goal is to ensure accurate and efficient supervision by government departments and foster a favorable market environment. However, there is no unanimity on the criteria and efficacy of supervision and punishment, and the limited regulatory resources frequently cause governance issues for government regulators.
Because there are no common standards for food safety oversight and enforcement among regulators, the one-size-fits-all nature of the regulatory process is frequently observed. A more common example of undue punishment is the case of a self-employed individual who was penalized 66,000 RMB in 2022 for selling a small bundle of celery in Yulin City, China. Furthermore, because government departments have limited regulatory resources, their oversight of food safety in online catering tends to concentrate on a certain cycle. Around significant holidays, regulators frequently decide to conduct extra food safety rectification. In addition, when the concentrated rectification period ends and there are not enough ongoing regulatory restrictions in place, some online catering businesses in the market may be tempted to violate unsafe business practices. A report by China Central Television about unlicensed workshops on Eleme’s platform that were fined and then covertly reopened is an example of a similar occurrence.
The preponderance of extant literature on online food safety governance centers on the discourse surrounding online food safety governance issues among government regulatory bodies, third-party platforms, the general public, and social organizations. Food safety oversight by many governments has changed from single authority to multi-center coordination, with a shift in focus from quantity, health, quality, and nutrition safety to multi-center coordination. Coordinated food safety management agencies, such as the European Food Safety Administration, the Japanese National Food Safety Commission, and the US President’s Food Safety Council, are typically located in countries or regions with more advanced regulatory frameworks (Edge and Meyer, 2019; Abou Ghaida et al., 2014). The online food safety supervision model in China is currently under the jurisdiction of the National Food Safety Commission. It was created through the stages of single supervision, segmented supervision, and unified supervision (Chen and Wu, 2019). In response to the online economy’s quick integration with the food market, government departments are still standardizing online food safety governance. The government departments’ increasing regulatory willingness to provide institutional supplies for online food safety governance is demonstrated by the promulgation of policies such as Measures for the Investigation and Punishment of Illegal Acts of Online Food Safety and Measures for the Supervision and Administration of Online Transactions (Yan and Ting, 2018). Nevertheless, given the need for additional development of the pertinent system now in place and the limitations of government law enforcement due to cost input, relying just on departmental monitoring from the government is insufficient to construct effective Internet food safety governance (Pouliot and Wang, 2018).
Relying solely on government monitoring is not as efficient as transforming government operations, coordinating the interests of several stakeholders, setting goals for food safety, and developing a diversified collaborative governance pattern for online food safety (Kang, 2019; Nakamura et al., 2021). Online food businesses are directly managed by the third-party trade platform, whose governance is characterized by high efficiency, flexibility, and autonomy. The platform not only assumes joint and several liabilities but also manages the online food enterprise’s production and operation qualification review, oversees sales and operation behavior, mediates disputes between buyers and sellers, builds the platform governance system, and carries out other duties. According to Liu and Li (2018), the majority of prior research has focused on data sharing, legal economy, private interest preference, and the distribution of power and responsibility in third-party platform self-regulation (Shen et al., 2023; Chen and Li, 2019). By helping to prevent and resolve online food safety risks through complaints, evaluations, publicity, and other channels, the public is an essential supervisory force for online food safety and plays a significant role in the modernization of food safety governance (Pan and Huang, 2023). The public’s willingness to engage in online food safety governance will be influenced by a variety of factors, varying in degree. When there is a dearth of reliable, publicly available information on food safety, the public is at a disadvantage when it comes to searching and sharing information and is more easily influenced by rumors. As a result, people are less inclined to take part in online food safety forgiveness and governance. Furthermore, factors such as the waiting period, the burden of proof, and the cost of defending the rights of online food safety affect the public’s enthusiasm and incentive to participate in the governance of online food safety (Zhu et al., 2022; Shen et al., 2021; Haji et al., 2022). The news media’s ability to shape public opinion is demonstrated by its rapid dissemination, wide-ranging impact, comprehensive disclosure, swift elimination, etc. It is a major factor behind the successful promotion of online food safety supervision, and food companies and government authorities rely heavily on the influence and reliability of its reports when making strategic decisions. The government’s conventional regulatory penalties and the media’s subjective evaluation of their role in social co-governance will impact the media’s involvement in online food safety governance. Online food businesses can be successfully deterred from engaging in illegal activities by pressing the government to closely monitor them and by the full coverage, authoritative supervisory system that the new media has built via its ongoing, in-depth reporting (Zhu et al., 2019; Sun et al., 2022). In addition, by using their informational advantages to monitor illegal activity within the industry and balance and coordinate public and private power in the online food market, industry associations of civil power subjects can achieve structural balance and functional complementarity of total social power (Liu et al., 2019).
Another group of academics suggests that issues in food safety governance can be examined based on the levels of supervision and severity of penalties. This research mostly relies on the notion of law enforcement economics. In the late 18th century, Bentham contended that the purpose of penalties should be to enhance the wellbeing of society rather than to seek retribution against the offender. During the latter half of the 20th century, Western law enforcement economics introduced the classic debate on the effectiveness of penalties in law enforcement. Becker (1968) and Posner (1985) claim that severe penalties are beneficial in law enforcement, while Stigler (1970), Harrington (1988), and Tsebelis (1990), among other scholars, suggest that imposing high penalties could worsen social issues. Not all harsh penalties lead to the desired level of compliance. Furthermore, several scholars believe that the probability of facing consequences affects illegal conduct. Increasing the frequency of spot inspections can offset the negative aspects of severe penalties, and raising the probability of penalties can enhance the incremental deterrent effect of law enforcement (Polinsky and Shavell, 1991; Polinsky, 1992).
There is still much discussion over the effectiveness of using supervision intensity and penalty intensity in the supervisory process (Wang, 2019; Golembeski et al., 2020). One way is to validate the legitimacy of a harsh penalty. Advocates argue that severe penalties for unsafe business operators in the food market can enhance the efficacy of food market safety oversight, while critics contend that harsh penalties to maintain order might reduce the willingness of online food vendors to engage in the market. Government regulation provides a better environment for market incentives, and it is necessary to create a synergistic effect of government regulation and market incentives to regulate the behavior of food enterprises (Zhao et al., 2018). The second goal is to examine the effectiveness of regular supervision. Studies on coal safety, financial markets, carbon emissions, and regulatory scenarios have shown that higher government regulation frequency leads to a decrease in illegal behavior among coal miners (Luo et al., 2018) and an enhancement in the quality of information disclosure by enterprises (Pan and Guo, 2018). Carbon emission companies find it challenging to have a motivation to reduce emissions (Wei et al., 2020). Theoretically, a high likelihood of severe penalties can enhance the deterrent effect of law enforcement. However, in actual supervision studies, the practice of merging the two types of supervision is uncommon. The above occurrences demonstrate the need to comprehend the supervision strength and penalty scale of online food safety monitoring. Currently, in the process of supervision, government departments frequently adopt two methods to regulate market subjects: increasing the intensity of surveillance or the severity of penalties, so as to limit the incidence of illegal behaviors. Improving supervision intensity indicates that government agencies manage the operation behavior of food businesses by raising the chance of sampling inspections, the frequency of inspections, and the scope of sampling inspections. To raise the severity of penalties is to instill in operators a dread of illegal behavior through harsher penalties, in an effort to warn other illegal groups. Intuitively, it is evidently more effective to simultaneously raise the intensity of supervision and penalties. The actual investigation of market supervision departments reveals, however, that to ensure the sustainable and stable development of the online food market, front-line law enforcement personnel do not typically employ both of the aforementioned supervision methods concurrently during the supervision process. However, in the existing research on food safety governance, there are few studies on optimal supervision and punishment.
According to the overview, previous foreign researchers placed greater emphasis on penalty deterrence in law enforcement. As the efficiency of supervision has increased, supervision intensity has steadily drawn the interest of scholars. In recent years, Chinese scholars have paid more attention to the application of regulatory effectiveness in a typical regulatory situation, but there have been few complete studies of supervision intensity and penalty intensity in the context of online food safety governance. Despite the fact that several research studies have evaluated the techniques of online food safety regulators by building game models (Jin et al., 2021), there are no empirical assessments based on realistic monitoring and penalty data. As Delaney et al. (2018) and Andrew et al. (2017) found many academic studies on food safety governance are mostly based on cases and theories, but lack analysis of empirical research data, it is essential to explore the strategy of food safety supervision through the data of supervision practice. The following four elements primarily represent the contribution made by this research. First, regarding the optimal intensity of law enforcement economics based on related research and regulatory penalties in a regulatory means to investigate the effectiveness of regulatory problems, this article focuses on the comparison between the effectiveness of the supervision strength and the regulatory penalty. The second is increasing the study’s research depth. Existing research on the economics of optimal law enforcement is primarily concerned with the efficacy of oversight in the financial market, environmental protection, and other areas. By focusing on online food safety supervision, this study makes the optimal law enforcement theory more useful. Third, existing studies on the economics of optimal law enforcement have either built an economic model of optimal law enforcement or a qualitative analysis of law enforcement economics from the perspectives of law and politics. This study introduces two key variables of supervision intensity and punishment intensity into the effectiveness model of online food safety supervision and further empirically tests the model through actual supervision and punishment data. The method of mathematical modeling and empirical analysis is comprehensively used to solve the practical problems of online food safety supervision. Fourth, the study’s conclusions, which are based on mathematical modeling and real-world testing, can help with the implementation of online food safety credit rating classification oversight.
The objective of this study is to broaden the application scenario of optimal law enforcement theory in online food safety governance. This research broadens the application scenarios of optimal law enforcement and introduces the supervision intensity and penalty intensity as two important regulatory tool variables in the effectiveness research on online food safety governance. Moreover, it further empirically tests and analyzes the changes in behavioral strategies of food enterprises in different regulatory intensities and penalties. In addition to serving as a useful reference for food safety officials in China’s first-tier cities, this study on the efficacy of online catering food safety supervision is anticipated to offer some broadly applicable theoretical groundwork for enhancing the efficacy of online food safety governance.
This study builds an online catering food safety supervision model for market supervision departments based on the theory of optimal law enforcement. In the study, the intensity of supervision and the intensity of penalty, which market regulators pay most attention to in the process of online food safety supervision, are described as two basic variables endogenous to the research model. The intensity of supervision is mainly reflected in the possibility of finding or the frequency of supervision of illegal business activities of online food. The intensity of punishment is mainly measured by the size of the penalty amount of online food operators. Taking the real regulatory data related to online catering as an example, the effectiveness of the regulatory mechanism is evaluated. To evaluate the efficacy of monitoring, real supervision data for online catering were gathered in Zhengzhou, a recently established first-tier Chinese city.
Such an online food economic system is considered, in which other key subjects have developed behaviors and the primary game interaction involves food businesses and regulators. It is anticipated that dangerous food businesses will be penalized by regulators once they are identified. The occurrence of unsafe operating behavior among food businesses in a network not only directly results in the loss of their own business but may also jeopardize the health of consumers and have bad social consequences. To limit the likelihood of dangerous accidents and the likelihood of being penalized by the regulator, enterprise food companies typically boost their safety investments in addition to their production and operation investments.
Under standardized production and operation, it is believed that the entire input of online food firms in the operation process is , which includes general operational input and safety input . As shown in Equation 1.
Assume that the per-order sales revenue of the online food business is and that the order quantity is . In general, the more input an online food firm invests, the more food it sells under standardized production and operations, and the greater its revenue. It is expected that order quantity and operating input have a positive change relationship. When the online food business has only operational inputs , its anticipated operational income is (as shown in Equation 2).
Security input can limit the likelihood of dangerous situations with online food businesses to some level. The greater the safety input of online food businesses, the lower the likelihood of unsafe accidents; correspondingly, the lower the likelihood of detection by government regulatory authorities, the greater the likelihood that they will be immune from penalty. Assume is the likelihood of dangerous events occurring when the safety input of the online food enterprise is , and is the probability that the government supervision department discovers the unsafe operating behavior of the online food enterprise when the safety input is . As increases, the probability of food safety events lowers ; likewise, the probability of dangerous operating behavior among the online food businesses under investigation falls . Clearly, the likelihood of risky accidents in online food businesses is positively connected with the likelihood of being identified by government oversight offices. In addition, it is considered that is the amount of penalty once the regulator discovers the dangerous operating behavior of online food businesses and that is the social damage caused by online food safety accidents to online food businesses in terms of reputation damage and customer loss. At this point, it is possible to draw the conclusion that the total expected return function of online food businesses with safety input is (as shown in Equation 3).
Substitute Equation 2 into Equation 3 to obtain Equation 4.
The main parameters of model construction are shown in Table 1.
According to Equation 4, is the subtraction function of the penalty amount or the chance of discovery ; therefore, assuming all other variables remain constant, will decline continuously with the increase of or until it equals 0. Therefore, according to Equation 4, the optimal supervision intensity or the optimal penalty intensity should at least make the total expected income of online food businesses penalized for hazardous operation equal to zero, so as to deter online food businesses from engaging in dangerous operation. When equals 0, the transformation results in Equation 5
The greater the frequency with which market regulatory bodies monitor online food businesses, the lower the probability of unsafe accidents and the more effective the supervision of online food safety, assuming all other variables remain constant. If all other factors stay the same, the less likely unsafe accidents are and the more effective online food safety supervision is, the harsher the penalty the market supervision department gives to online food businesses that do dangerous things.
To empirically test the efficacy of regulatory tools, it is necessary to examine how market regulators employ regulatory tools. The research focused on the supervision of two districts which are located in an important first-tier city called Zhengzhou and have a comparable rate of online food supervision intensity.
First, from the perspective of supervision intensity, there is a difference in the number of indicators of front-line law enforcement personnel investigating online catering enterprises in the district, although there is little difference in the number of spot checks conducted by front-line law enforcement personnel responsible for food safety, online restaurants within the jurisdiction, and online catering enterprises within the jurisdiction in J and E. Each law enforcement officer in district J conducts two investigations each month, but each officer in district E conducts one investigation every 2 months. In the online food safety management process, the difference in investigation indicators directly reflects the difference in annual supervision intensity between J and E. Due to the fact that the actual process of investigation and penalty did not conclude by the end of the month, the month index will only be communicated verbally. Consequently, the actual law enforcement investigation and penalty of unsafe business behavior may take slightly less time than the index specifies. To ensure the healthy development of the online catering market, the same online catering firms are not typically inspected again in the same year, although the supervision records of food safety in districts J and E are identical. The study found that there is a significant difference in the investigation frequency of unsafe operation behaviors of online catering enterprises between District J and District E in 2019. This is despite the fact that the number of front-line law enforcement personnel, the number of online restaurants in the jurisdiction, and the frequency of random inspections of online catering enterprises in the jurisdiction are generally the same. Of them, District J investigated online catering businesses with risky operation practices 638 times annually, while District E investigated them 82 times annually (see Table 2).
There are also distinctions between district J and district E in terms of the severity of penalties. According to the big penalty threshold of 1,000 RMB for individual small businesses in Zhengzhou, it has been determined that law enforcement agents in the two districts choose different penalties for online food businesses. The number of penalties with a single amount of large penalty standard or above accounted for only 28.7% of the 638 penalties in district J in 2019, the number of penalties with an amount of less than 1,000 RMB accounted for 71.3% of the 638 penalties in district J in 2019, and the majority of the penalties were less than 500 RMB (accounting for 63.3%). The average fine was only 1,916.21 RMB. However, among the 82 penalties issued in District E in 2019, those with a severe penalty standard or higher accounted for 90.2% of the total number of yearly penalties, and the average penalty amount was 13,991.62 RMB (see Table 3).
Comparing the selection of online food safety supervision tools in district J and district E reveals that the market supervision department in district J deals with unsafe business behaviors of online catering enterprises frequently, that is, the supervision intensity is high, but the single penalty amount is relatively low, and it tends to adopt frequent supervision. Although the frequency of investigations and penalties for risky business conduct in the E district is low, that is, the monitoring intensity is low, the single penalty amount is extremely large, and the district tends to impose harsh penalties.
According to the aforementioned Equation 5, the ideal supervision intensity and penalty amount of the market supervision department for the unsafe operation behavior of online food businesses may be determined, as shown in Equations 6, 7 below.
According to the survey, district J and district E are two adjacent administrative districts of Zhengzhou that have experienced significant economic growth, and the number of online eateries in the two districts is comparable. In 2019, there were 2,986 online catering businesses in the J District and 3,178 in the E District. Throughout the course of the year, the supervisory departments of district J and district E performed 3,376 and 3,241 random inspections, respectively, of online catering businesses operating within their respective districts. The number of catering businesses in the two districts and the total number of spot inspections by regulatory agencies were likewise comparable. In Table 3, column (4) indicates the average amount of fines assigned to each online catering business within the jurisdiction, and column (5) indicates the likelihood of online catering businesses within the jurisdiction discovering risky business practices. The average annual amount of illegal fines can be calculated by multiplying the two columns together. It is easy to determine that the likelihood of an annual examination of online catering businesses in the two districts is low, and hence, the probability of unsafe accidents in online food businesses is equally low.
In the past 5 years, the survey revealed that there were no serious food safety incidents in districts J and E; thus, the probability of food safety incidents occurring in online food firms under the safety input was extremely low. Although the market supervision department investigates and punishes some online catering businesses for hazardous business practices, the harm caused by these unsafe business practices, particularly the enterprise’s social harm , is little. Hence, is also very small and approaches zero. In the meantime, the ideal online food safety supervision tool model for J District and E District in Zhengzhou can be represented as follows, based on survey data from J District and E District and simplified processing of Equations 6, 7 (as shown in Equations 8 and 9):
In addition, it was determined that even if district E adopted a heavy penalty mode, the average amount of fines would reach 13,991.62 RMB. However, because the probability of getting caught is so low, the expected yearly penalty of online catering businesses is only 353.92 RMB. As the same year will typically not appear twice on an online catering business that has been inspected and dealt with, a penalty in the current year can effectively lessen the likelihood of being investigated; for individual online food enterprises, the real perceived deterrence may be smaller.
Based on the average wage of tertiary industry employees and the average number of online small restaurants in Zhengzhou in 2019, the average annual income of online small restaurants in Zhengzhou is predicted to be 132,045 RMB. Equation 8, 9, and survey data may be used to determine the ideal supervision intensity and optimal penalty intensity for online catering food safety governance in Zhengzhou.
In the first step, the optimal supervision intensity of online catering businesses in Zhengzhou is determined, and the actual supervision intensity of the two districts is compared. According to Equation 11, the ideal monitoring intensity for online catering businesses can be computed based on a specified fine amount. Using Henan Province’s administrative penalty on individual merchants as a standard definition of “big fine” of 1,000 RMB, the best annual supervision intensity of online catering firms within a jurisdiction should be (1/1000)*132,045 = 132, or 11 times per month on average. In fact, however, the frequency of supervision in district E is fewer than seven times per month, whereas it might exceed 53 times per month in district J. From the standpoint of supervision intensity as a tool for governance, the supervision intensity in district J can meet the optimal frequency of inquiry 11 times, whereas the supervision intensity in district E cannot meet the optimal frequency of investigation 11 times. Therefore, the actual supervision intensity tool in district J is superior to its counterpart in district E.
Second, the appropriate penalty amount for online catering businesses in Zhengzhou is then determined, and the actual penalty intensity of the two districts is compared. According to Equation 9, the optimal penalty for district E can be determined as (1/0.0253)*132,045 = 5219169.9 RMB. Although the average fine in the district is 13,991.62 RMB and the maximum fine might reach 130,000 RMB, the likelihood of the eatery being deemed illegal is quite low. This is below the 5.2 million threshold required for effective deterrence. The best penalty amount for district J can be computed as (1/0.1890)*132,045 = 69,8650.80 RMB. The average penalty amount in this district is 1,916.21 RMB, and the maximum penalty can reach 16,278 RMB, which is less than the effective deterrent requirement of 690,000 RMB. Through the calculation of the optimal penalty amount and the comparison of the actual penalty intensity between the two districts, it can be determined that the average and maximum penalty amounts of J district and E district do not meet the optimal penalty amount’s requirements, but the average penalty amount of J district is closer to the optimal penalty amount than that of E district. Consequently, the actual penalty severity tool in district J is similarly superior to that in district E.
The analysis and comparison of regulators in J and E districts online catering enterprises regulatory tool usage revealed that the district J uses relatively high investigate frequency and relatively low penalty, whereas the district E uses relatively low investigate frequency and relatively high penalty. In addition, in terms of the advantages and drawbacks of using regulatory instruments in the two districts, the penalties in both districts fall short of the optimal penalty amount, but district J’s penalty amount is closer to the optimal penalty amount than district E’s penalty amount. In addition, the level of supervision in District J meets the requirements for the ideal level of supervision in Zhengzhou. The regulatory tools in district J can approach or be closer to the optimal regulatory tools than those in district E, and the actual regulatory tools in district J are superior than those in district E. The test results provided above are only inferential. Also essential is a direct examination of the effectiveness of regulatory tools.
Whether or not the monitoring tool is efficient is mostly determined by whether or not it can alter the risky operation practices of online catering businesses. Whether the online catering businesses investigated and fined in the two districts are investigated and punished again by the government in the subsequent period is considered as an observation indicator. If it is determined that online catering businesses are penalized again for unsafe operations by the government supervision department, the employment of supervisory tools in this district is deemed ineffective. On the other hand, the use of regulatory instruments is deemed effective.
In this research, the online catering businesses that were sanctioned in 2019 serve as the observational objects, and the penalty records of 2 consecutive years, 2019 and 2020, are used to determine whether online catering businesses will be sanctioned again and investigated for unsafe business practices. In the survey, pertinent penalty data of online catering businesses in districts J and E were collected, and the regression model empirical test was used to examine the efficiency of the two supervisory instruments on online food safety governance.
The research obtained secondary data regarding the regulations and penalties pertaining to the online catering business from the Market Supervision Administration in Zhengzhou. Because the penalty data are private, we only used it for analysis in this study. Regression analysis is supported by the data, and the overall number of our samples is appropriate. We gathered 720 samples in total from the Market Supervision Administration’s inquiry, and we further separated them into high-frequency supervision and high-penalty groups based on the frequency and severity of supervision. Our data originated from Zhengzhou, a province capital city in central China. The data pertained to the supervision and enforcement actions taken by the market supervision and administration department of Zhengzhou in the online catering food market. Zhengzhou, representing China’s emerging first-tier cities, possesses a well-structured urban layout, with a population of 12.8 million and a GDP of 1.29 trillion RMB in 2022. Zhengzhou is expected to be designated as one of China’s 15 new first-tier cities in 2023. The city’s online catering business has experienced growth in recent years. In 2023, the online catering sector in Zhengzhou generated an annual revenue of $13 billion, representing one-third of the market share in the province. Zhengzhou is a typical food safety management excellent demonstration city in China, with advanced food safety management experience and excellent food safety government supervision team. It can serve as a valuable benchmark for online food safety inspection in other cities in China’s catering industry.
Assume that the market supervision department has a standard for the investigation and penalty of online catering businesses’ risky operating behavior. If the actual investigation and penalty are effective, online catering businesses that have been investigated and punished will alter their unsafe business practices. On the other hand, the unsafe business practices of online restaurants will not be punished, and they will continue to engage in unsafe business practices while being investigated. It can be observed through the results and standing of online eateries following an investigation and sanction. Since the explanatory variables are binary variables of type 0 and 1, a binary logistic regression model can be used to estimate the relationship between penalty and various regulatory mechanisms as shown in Equation 10.
The likelihood that the online catering company would not engage in risky business practices again following a sanction can be represented as Equation 11
The principal model variables and their respective measurements are as follows:
The value of the variable Y, which represents the effect of penalty, is either 0 or 1. Y = 0 indicates that the unsafe operation behavior of the online restaurant is not found in the subsequent penalty record and that the penalty is effective; Y = 1 indicates that the unsafe operation behavior of the online restaurant is found in the subsequent penalty record and that the penalty is ineffective.
It is defined as the actual amount of fine levied by government supervision departments when online catering firms are inspected and handled with. The variable of fine quantity is a specialized numerical variable.
It is defined as the percentage of the total amount of fines received by online catering businesses to their monthly income. To properly quantify the real perceived deterrent brought by the amount of penalties to online catering businesses, the proportion of fines is introduced as an alternative variable to the amount of fines. This is because the income of online catering businesses varies. Introducing the variable of penalty ratio can not only evaluate the model’s stability more effectively but also demonstrate the validity of penalty intensity in terms of the relative penalty amount.
Since the inspection times and investigation frequency of online catering businesses under the jurisdiction of the government market supervision department in the same year are fixed values, the probability of discovering unsafe business practices of online catering businesses in the two districts is also fixed, and the frequency of supervision can be replaced with the number of spot checks of online catering businesses in the same year. When the chance of discovering unsafe business behavior of online catering companies is certain, the greater the frequency of spot inspections of an online food business indicates that it is simpler to detect unsafe business conduct of online catering companies as the supervision intensity increases.
To explore the interpretation of the model following the inclusion of control variables, the operator’s educational background, physical store space, years of operation, and shop operating income are chosen as control variables. The square footage of the store and the manager’s educational background are dichotomous variables; however, the operational income and years in business of the store are numerical factors. Regarding the control variable of physical store area, it is divided by the Henan Province’s 100-m2 norm for food stores and catering. The number 0 represents less than 100 m2 of storage space, whereas the number 1 represents more than 100 m2 of storage space. Regarding the control variable of education level, it is further segmented according to the managers’ degree of education. Managers who have completed postsecondary education are listed as 1, and those who have not are listed as 0. Rather than the number of years the store has been open, the operator’s age is chosen as a control variable to assess the model’s resilience. Furthermore, to ascertain the impact of this variable on the variable under explanation, the region was selected as the control variable for the regression test.
The Hosmer test was employed in this investigation to confirm the binary logistic regression analysis data fitting index. Following Hosmer’s analysis of 720 data sets containing regulatory fines pertaining to the food safety of online catering, it is discovered that the significance level, p = 0.790, is higher than 0.05. It demonstrates that using the current data, we construct a binary logistic regression model, and the model fits the actual data quite well. Put another way, the outcomes of a subsequent study of the binary logistic regression model can accurately and consistently represent the true connection between the initial variables. The results of Hosmer’s test are shown in Table 4.
From the total supervision and penalty data of the two districts, the influence of regulatory tools on the subsequent safe operation behavior of online catering businesses is evaluated (see Table 5). The results indicate the following: (1) From the overall penalty data of the two districts, the regulatory frequency has a significant impact on the follow-up safe business behavior of standardized online catering enterprises, while the amount of penalty has no significant impact on the follow-up safe business behavior of standardized online catering enterprises; whether the operator has a higher education has a significant impact on standardizing the follow-up safe operation; and whether the operator has a higher education has a significant impact on standardizing the follow-up safe operation. (2) Variable REGION has a considerable impact on the subsequent risky business practices of online catering companies following a penalty, demonstrating that the efficacy of regulatory measures differs significantly between administrative areas. Using Model 1 as an example, the likelihood that risky operating behavior of online catering businesses will be investigated again following the penalty is 9.05 percentage points higher in district E than in district J (95% CI, 0.271, 1.910).
J and E data on supervision and penalty were analyzed using regression. The main focus of the analysis is how the frequency, amount, and proportion of penalties affect the risky business practices of food and catering enterprises operating online in the two districts. Tables 6, 7 detail the effectiveness analysis and robustness test of supervision tools.
According to the penalties influence in J and E, there was no significant difference in the amount of fine and the proportion of fine imposed for the online catering business’s subsequent risky behavior. The influence of penalties indicated that the regulatory tools for the two districts’ online food safety management behavior cannot be effectively controlled during operation. In contrast, the frequency of supervision in district J and district E can greatly lower (by 1% test) the recurrence of risky business practices among online food businesses. Using Model 5 as an example, in district J, the probability of online food enterprises violating the law again will be reduced by 6.9% (95% CI: −1.575, −0.564). Using Model 11 as an example, the probability of online food enterprises violating the law again will be reduced by approximately 52.0% (95% CI: −2.658, −0.381) with each increase in the frequency of super violations. It is evident that increasing the level of supervision can successfully prevent the occurrence of following risky business practices among online catering companies within the jurisdiction.
The test results based on sample data indicate that high-frequency supervision in districts J and E can successfully constrain following unsafe business practices; however, heavy penalties cannot effectively constrain the safe business practices of online catering businesses within the jurisdiction. Increasing the frequency of spot checks in supervision has a substantial impact on reducing the incidence of online catering firms’ dangerous business practices. In terms of supervision efficiency, frequent supervision is preferable to a harsh penalty.
Among the control variables, store operating revenue (SALARY), operating years (YEAR), and physical store area (AREA) have no influence on the future dangerous business behaviors of online catering businesses. There is no correlation between an operator’s risky operation behavior and the store’s revenue, number of years in business, or size. However, the education level of operators (EDU) has a substantial effect on the dangerous business practices of online eateries in the two districts (passing the 1 percent test). After being investigated and punished by regulatory authorities, operators with a higher level of education in J and E districts will be more effective at regulating their subsequent unsafe business behaviors, and the phenomenon of unsafe business behaviors being investigated and punished again will be significantly reduced. After controlling for other variables, operators of online catering businesses in district J (Model 7) without higher education are 2.28 times more likely to be investigated by market supervision authorities than those with a higher education (95% confidence interval: −2.892, −1.666), and 3.66 times more likely to be investigated in district E (Model 13) (95% CI: −6.000, −1.338). To verify the validity of the research results, the operator’s age was substituted for the number of years of operation in the robustness test. The connection and level of significance of the primary explanatory variables FINE, PERCENT, and FREQ did not differ between the two locations.
District E employs the high-penalty supervision tool, but in this district, increasing the penalty amount cannot significantly improve the safety operation of the online food market, nor do the key explanatory variables FINE and PERCENT have a significant effect on the compliance operation behavior of online catering businesses in this district. Even though the amount of penalty for unsafe business practices of online catering companies in district J is not high after being investigated and dealt with, the unsafe business practices of online catering companies can be effectively improved by increasing the frequency of investigation and penalty due to the high-frequency supervision. In general, the adoption of high-frequency supervision technologies makes online catering food safety supervision in district J more effective than in district E, where severe penalties are in place. This is consistent with indirect tests of regulatory instrument effectiveness.
This research constructs an efficacy model for online food safety supervision. By introducing two regulatory tool variables, namely, supervision intensity and penalty intensity, and utilizing actual survey data of online catering safety supervision and penalty in two districts of Zhengzhou, the effectiveness of supervision tools was analyzed and evaluated, and new findings were obtained. Increasing the sample frequency of market monitoring departments, for instance, can effectively supervise the safe operation of food businesses in the online food market. Increasing the severity of penalties, for instance, is insufficient for properly regulating the safety of food businesses in the online food market. In addition, food businesses with a greater level of education demonstrate a clearer commitment to safety management. The following are the managerial implications of these findings for market regulating authorities.
Based on selective law enforcement in law enforcement economics, this study extends the optimal law enforcement theory to the realm of online food safety supervision and expands law enforcement economics’ application possibilities. First, the frequency of spot checks on food enterprises can be raised by increasing the intensity of supervision throughout the process of developing standards for supervision and punishment for unsafe business practices of online catering firms. If there are circumstances that allow for reduced penalties, catering businesses that do not purposefully produce and operate unhealthy food can be deterred by frequent supervision coupled with light penalties. Second, by adjusting the frequency of spot checks on food businesses entering the Internet, the market supervision department is able to carry out risk credit rating and classification supervision in the online food safety governance, thereby improving the market regulator’s supervision efficiency. Third, the government should routinely conduct training and safety instruction for food operators as part of the supervisory process, as well as support and direct the safe operation behavior of food firms in the Internet. Due to objective constraints, this study only examined the penalty data of two typical administrative districts in Zhengzhou. Future research can increase regional restrictions and investigate the usefulness of inter-city supervision methods.
Our research findings also have reference value for the online catering market in other cities in China or other countries. Our research indicates that high-frequency supervision of the online catering market, rather than relying solely on hefty penalties, can enhance the market supervision department’s categorization and supervision mechanisms in the online catering and food market. Supervising the classification is beneficial for developing the credit assessment system for digital governance of online food safety. By utilizing online catering data and food safety regulations, a dynamic credit rating system can be established for food businesses entering the online network. This system can be used to supervise and classify food enterprises based on their credit status, enhancing the digital governance of online food safety with scientific precision and effectiveness. This holds significant reference value and practical importance for governing food safety in the online catering industry worldwide.
The original contributions presented in the study are included in the article/supplementary material, further inquiries can be directed to the corresponding authors.
Ethical review and approval were not required for the study in accordance with the local legislation and institutional requirements.
CS: Data curation, Formal analysis, Funding acquisition, Methodology, Software, Visualization, Writing – original draft, Writing – review & editing. MW: Conceptualization, Methodology, Writing – review & editing. CL: Data curation, Methodology, Writing – review & editing. XH: Data curation, Software, Writing – review & editing. LW: Data curation, Funding acquisition, Methodology, Supervision, Writing – review & editing.
The author(s) declare that financial support was received for the research, authorship, and/or publication of this article. This research was financially supported by the project of Philosophy and Social Science Planning Project of Henan Province (2022CZZ017), the Soft Science Research Project of Henan Province (Grant No. 242400410257), Research Fund for High-level Talents of Henan University of Technology (Grant No. 2022BS010), the National Social Science Fund of China (Grant No. 21CGL032), and the National Key Project of Philosophy and Social Sciences (Grant No. 23AGL010).
The authors appreciate the reviewers’ insights.
The authors declare that the research was conducted in the absence of any commercial or financial relationships that could be construed as a potential conflict of interest.
All claims expressed in this article are solely those of the authors and do not necessarily represent those of their affiliated organizations, or those of the publisher, the editors and the reviewers. Any product that may be evaluated in this article, or claim that may be made by its manufacturer, is not guaranteed or endorsed by the publisher.
Abou Ghaida, T., Spinnler, H. E., Soyeux, Y., Hamieh, T., and Medawar, S. (2014). Risk-based food safety and quality governance at the international law, EU, USA, Canada and France: effective system for Lebanon as for the WTO accession. Food Control 44, 267–282. doi: 10.1016/j.foodcont.2014.03.023
Andrew, J., Ismail, N. W., and Djama, M. (2017). An overview of genetically modified crop governance, issues and challenges in Malaysia. J. Sci. Food Agric. 98, 12–17. doi: 10.1002/jsfa.8666
Chen, J., and Li, W. (2019). Construction and perfection of the responsibility of third-party platform providers of the online food trade in China. Biotechnol. Law Rep. 38, 294–302. doi: 10.1089/blr.2019.29138.wl
Chen, X. J., and Wu, L. H. (2019). Psychological capital in food safety social co-governance. Front. Psychol. 10:1387. doi: 10.3389/fpsyg.2019.01387
Delaney, B., Goodman, R. E., and Ladics, G. S. (2018). Food and feed safety of genetically engineered food crops. Toxicol. Sci. 162, 361–371. doi: 10.1093/toxsci/kfx249
Edge, S., and Meyer, S. B. (2019). Pursuing dignified food security through novel collaborative governance initiatives: perceived benefits, tensions and lessons learned. Soc. Sci. Med. 232, 77–85. doi: 10.1016/j.socscimed.2019.04.038
Golembeski, C. A., Irfan, A., and Dong, K. R. (2020). Food insecurity and collateral consequences of punishment amidst the COVID-19 pandemic. World Med. Health Policy 12, 357–373. doi: 10.1002/wmh3.378
Haji, M., Kerbache, L., and Al-Ansari, (2022). Food quality, drug safety, and increasing public health measures in supply chain management. PRO 10:1715. doi: 10.3390/pr10091715
Harrington, W. (1988). Enforcement leverage when penalties are restricted. J. Public Econ. 37, 29–53. doi: 10.1016/0047-2727(88)90003-5
Jin, C. Y., Levi, R., Liang, Q., Renegar, N., and Zhou, J. H. (2021). Food safety inspection and the adoption of traceability in aquatic wholesale markets: a game-theoretic model and empirical evidence. J. Integr. Agric. 20, 2807–2819. doi: 10.1016/S2095-3119(21)63624-9
Kang, Y. (2019). Food safety governance in China: change and continuity. Food Control 106:106752. doi: 10.1016/j.foodcont.2019.106752
Liu, P., and Li, W. (2018). Food safety regulation of online meal ordering: a study from the perspective of smart regulation. J. Centr. Chin. Norm. Univ. 64, 1–9. doi: 10.3969/j.issn.1000-2456.2018.01.001
Liu, Z., Mutukumira, A. N., and Chen, H. J. (2019). Food safety governance in China: from supervision to coregulation. Food Sci. Nutr. 7, 4127–4139. doi: 10.1002/fsn3.1281
Luo, P., Qian, Y. K., and Xu, J. B. (2018). “High fine” or “high frequency”: research on the selection and effectiveness penalty for violation of coalmine in China. Bus. Manag. J. 40, 92–106.
Nakamura, A., Kondo, A., Takahashi, H., Keeratipibul, S., Kuda, T., and Kimura, B. (2021). Microbiological safety and microbiota of Kapi, Thai traditional fermented shrimp paste, from different sources. LWT Food Sci. Technol. 154:112763. doi: 10.1016/j.lwt.2021.112763
Pan, A. E., and Guo, Q. S. (2018). Government regulation and the disclosure of enterprise environmental information: based on the role of environmental protection awareness of executives. Soft Sci. 32, 84–87.
Pan, N., and Huang, W. Y. (2023). Trust, technology acceptance and digital engagement: an empirical study on influencing factors of public participation in food traceability system. Chin. Publ. Administr. 39, 99–110.
Polinsky, A. (1992). Enforcement costs and the optimal magnitude and probability of fines. J. Law Econ. 35, 133–148. doi: 10.1086/467247
Polinsky, A., and Shavell, S. (1991). A note on optimal fines when wealth varies among individuals. Am. Econ. Rev. 81, 618–621.
Posner, R. A. (1985). An economic of theory of the criminal law. Columbia Law Rev. 85, 1193–1467. doi: 10.2307/1122392
Pouliot, S., and Wang, H. H. (2018). Information, incentives, and government intervention for food safety. Ann. Rev. Resour. Econ. 10, 83–103. doi: 10.1146/annurev-resource-100516-053346
Shen, C., Lei, B., Lu, C., and Zhou, F. (2023). Research on the effectiveness of online food safety supervision under the existence of settled enterprises’ myopic cognitive bias. Heliyon 9:e12784. doi: 10.1016/j.heliyon.2022.e12784
Shen, C., Wei, M. X., and Sheng, Y. L. (2021). A bibliometric analysis of food safety governance research from 1999 to 2019. Food Sci. Nutr. 9, 2316–2334. doi: 10.1002/fsn3.2220
Stigler, G. (1970). The optimum enforcement of laws. J. Polit. Econ. 78, 526–536. doi: 10.1086/259646
Sun, S. L., Ge, X. W., Wen, X. W., Barrio, F., Zhu, Y., and Liu, J. L. (2022). The moderation of human characteristics in the control mechanisms of rumours in social media: the case of food rumours in China. Front. Psychol. 12:782313. doi: 10.3389/fpsyg.2021.782313
Tsebelis, G. (1990). Penalty has no impact on crime: a game-theoretic analysis. Ration. Soc. 2, 255–286. doi: 10.1177/1043463190002003002
Wang, Y. G. (2019). Review and prospect of selective law enforcement research. Hebei Law Sci. 37, 122–136.
Wei, Q., Zhou, H. W., and Li, L. J. (2020). Experimental study on the default behavior of the carbon emission trading under different supervision intensity. Clim. Change Res. 16, 345–354.
Yan, H. N., and Ting, Y. (2018). The effectiveness of online citizen evaluation of government performance: a study of the perceptions of local bureaucrats in China. Public Pers. Manag. 47, 419–444. doi: 10.1177/0091026018767475
Zhao, L., Wang, C. W., Gu, H. Y., and Yue, C. Y. (2018). Market incentive, government regulation and the behavior of pesticide application of vegetable farmers in China. Food Control 85, 308–317. doi: 10.1016/j.foodcont.2017.09.016
Zhu, X. Y., Huang, I. Y., and Manning, L. (2019). The role of media reporting in food safety governance in China: a dairy case study. Food Control 96, 165–179. doi: 10.1016/j.foodcont.2018.08.027
Keywords: online catering food, frequent supervision, strict penalty, supervision efficacy, food safety governance
Citation: Shen C, Wei M, Li C, Hao X and Wang L (2024) An investigation into China’s online catering food safety governance efficacy based on the strategies of frequent supervision and strict penalty. Front. Sustain. Food Syst. 8:1308394. doi: 10.3389/fsufs.2024.1308394
Received: 22 November 2023; Accepted: 19 August 2024;
Published: 11 September 2024.
Edited by:
Joshua B. Gurtler, Agricultural Research Service (USDA), United StatesReviewed by:
Vesna V. Janković, Institute of Meat Hygiene and Technology-INMES, SerbiaCopyright © 2024 Shen, Wei, Li, Hao and Wang. This is an open-access article distributed under the terms of the Creative Commons Attribution License (CC BY). The use, distribution or reproduction in other forums is permitted, provided the original author(s) and the copyright owner(s) are credited and that the original publication in this journal is cited, in accordance with accepted academic practice. No use, distribution or reproduction is permitted which does not comply with these terms.
*Correspondence: Lin Wang, d2FuZ2xpbl9oYXV0QDE2My5jb20=
Disclaimer: All claims expressed in this article are solely those of the authors and do not necessarily represent those of their affiliated organizations, or those of the publisher, the editors and the reviewers. Any product that may be evaluated in this article or claim that may be made by its manufacturer is not guaranteed or endorsed by the publisher.
Research integrity at Frontiers
Learn more about the work of our research integrity team to safeguard the quality of each article we publish.