- 1Centro I + D Agroecología, Curicó, Chile
- 2Laboratorio de Evolución y Ecología Teórica, Facultad de Ciencias, Instituto de Zoología y Ecología Tropical, Universidad Central de Venezuela, Caracas, Venezuela
Regenerative agriculture offers important solutions to the enormous challenges that the climate crisis poses on food production. However, there are doubts about the possibility of implementing many of these solutions in a particularly important sector: the large scale. This paper addresses the issue, presenting examples of large-scale vineyard soil microbiome manipulation in Chile. The South American country has strongly faced the effects of climate change during the last decade and the organic viticulture sector is actively seeking strategies to adapt to the new climatic reality. Here the results of 4 experiments under real production conditions are shown. The experiments were designed to assess the effects of adding various microbial consortia to the soil on key agronomic parameters. Successful as well as unsuccessful cases are presented, allowing discussion of some conditions under which the microbiome manipulation can be expected to have positive effects. It was found that under good management conditions, incorporating effective microorganisms has positive effects on important production parameters (yield, root and vegetative growth). However, when fields yields are trending downward for prolonged periods, the incorporation of effective microbial consortia (e.g., antagonistic fungi, nutrient-fixing and nutrient-solubilizing bacteria) does not have a positive effect on the vineyard trend immediately. Similarly, even in favorable conditions the positive effects cannot be expected to be expressed in the short term (i.e., in just a few months). Therefore, its use should be conceived as a long-term strategy, not as an immediate solution to urgent management problems.
1 Introduction
To see a world in a grain of sand and a heaven in a wild flower, hold infinity in the palm of your hand and eternity in an hour. Auguries of Innocence by Blake W. (1988).
Extreme temperatures have become a daily occurrence, and it is not uncommon to see a new record set somewhere on the planet (Witze, 2022). Undoubtedly, we are living the beginning of a serious climate crisis at a planetary level and it is necessary to adapt to this context (Lovelock, 2007; Archer and Rahmstorf, 2010; Shen et al., 2018; Chakrabarty, 2021). For example, in Chile, a climate emergency was declared in 2021 due to the intense drought suffered in the last decade (Aparicio, 2021). This has meant significant challenges for its viticulture sector, especially for the large-scale and export-oriented subsector (Crowley, 2000; Hadarits et al., 2010; Mills-Novoa et al., 2016; Haddad et al., 2020). Climate change poses a major threat to grapevine cultivation (Coombe, 1987; Moutinho-Pereira et al., 2004; Greer et al., 2010, 2013; Fraga et al., 2020; Jones et al., 2022) and this may have mayor economic repercussions worldwide (FAO/OIV, 2021). In the emerging organic wine sector, the situation becomes even more complex, due to restrictions impose on crop management by the different certifications and the increase in manufacturing cost, especially in systems with high dependence on external inputs (Pino, 2013; Migliorini and Wezel, 2017; Pekdemir, 2018).
Climate forecasts anticipate a global decrease in water availability in most wine-producing regions (Santillán et al., 2019). This issue has sparked significant concern within the viticulture industry, prompting a considerable number of scientific papers to delve into the subject (Fraga et al., 2012; Xu et al., 2012; Mosedale et al., 2016; Storchmann, 2016; Ollat et al., 2017; van Leeuwen et al., 2019). For instance, it is expected that the increase in aridity in the future will result in a widespread loss of suitability for viticulture in the mediterranean climate zones of southern Europe (Droulia and Charalampopoulos, 2021), region responsible for 54% of the world’s wine exports (and 61% in terms of value) (Šajn, 2023). It is also important to consider that the feasibility of wine production is based both on yield and the quality of the grapes, as the latter can have a significant impact on the quality of the resulting wine and the prices consumers are willing to pay. In fact, wine prices, depending on their quality, can vary by a factor of up to 1,000, while yields usually fluctuate by a factor close to 10 (van Leeuwen et al., 2019).
The increase in temperatures and the reduction in rainfall, linked to climate change, can greatly affect the quality of the fruit and the yield of the crops in the vineyard (van Leeuwen et al., 2019). Among other aspects, climate change can impact the composition of the grape, its physiology, its phenology, and the quality of the wine. For example, high temperatures between veraison and harvest can result in an unbalanced fruit composition (due to the desynchronization in the development of sugars, acids, and other berry components) (van Leeuwen et al., 2019; Morales-Castilla et al., 2020). This can generate excessively high sugar levels, too low acidity, and an aromatic expression dominated by cooked fruit aromas, resulting in wines that lack freshness and aromatic complexity (Mira de Orduna, 2010; van Leeuwen et al., 2019; Morales-Castilla et al., 2020; Santos et al., 2020).
Among the techniques that can be used in organic agriculture to adapt agroecosystems to the new climatic context, is the manipulation of the microorganism community associated with plants, especially those present in the soil (Toro and Andrade, 2020; Chouhan et al., 2021; Antoszewski et al., 2022; Sandrini et al., 2022). This strategy can be underappreciated when the complexity of this component is underestimated (Vandermeer and Perfecto, 2018). However, soil is home to 59% of the planet’s biodiversity (Anthony et al., 2023) and is a complex web of ecological interactions (Wall and Moore, 1999; Reynolds et al., 2003).
In fact, the well-being and overall health of plants is highly dependent on these ecological interactions (Barrow et al., 2008, Chouhan et al., 2021; Antoszewski et al., 2022; Sandrini et al., 2022). Particularly important are those between the microorganisms associated with them, whether inside, outside or in the immediate vicinity of their bodies (Barrow et al., 2008; Qiao et al., 2023), which can help plants withstand important stress conditions (Barrow et al., 2008; Albornoz et al., 2022). The organisms involved in these interactions are known as the plant microbiome in the scientific literature (Whipps et al., 1988; Lederberg and McCray, 2001; Marchesi and Ravel, 2015; Berg et al., 2020) and are generally referred to as efficient microorganisms in the ecological agriculture milieu (Singh et al., 2011; de Araujo Avila et al., 2021). These microorganisms are related to health, well-being and tolerance to different forms of stress in plants (Mesa-Marín et al., 2019; Redondo-Gómez et al., 2022), for example, through the production of phytohormones, such as indole acetic acid, cytokinin, abscisic acid and ethylene reduction (Martínez-Viveros et al., 2010; Basu et al., 2021; Gupta et al., 2022; Notununu et al., 2022; Carreiras et al., 2023).
The microbiome can aid in the adaptation of crops to climate change through various mechanisms. For example, one expected impact of climate change is a significant reduction in rainfall and water availability for agriculture (Malek et al., 2018; Arora, 2019; Malhi et al., 2021), a situation already present in Chilean agriculture (del Pozo et al., 2019; Fernández et al., 2019; Vicuña et al., 2021). In such circumstances, introducing efficient microorganisms into the soil can promote root development (Lareen et al., 2016; Mhlongo et al., 2018; Pascale et al., 2020; Molefe et al., 2023), enabling more thorough soil exploration for water and enhancing plant vigor under harsh conditions (Agler et al., 2016; Tao et al., 2019; Arif et al., 2020; Singh et al., 2020; Trivedi et al., 2020; Gupta et al., 2021).
To some extent, plant health can be conceived as a possible state that emerges from interactions in its microbiome. Thus, the manipulation of this community offers important opportunities for the ecological management of agroecosystem in general (Chouhan et al., 2021) and to adapt these systems to the new climatic conditions in particular (Barrow et al., 2008; Albornoz et al., 2022). In fact, efficient microorganisms can be a valuable tool to adapt vineyards to new climatic conditions (Aguilera et al., 2022; Carreiras et al., 2023). However, agroecological practices are typically associated with small-scale farming, it is therefore necessary to demonstrate that they work at larger scales (Dalgaard et al., 2003; Nicol, 2020; Petit et al., 2020; Mayer et al., 2022), as is the case of Chilean export viticulture (Crowley, 2000).
The objective of this paper is to present a set of results on the manipulation of the microbiome in large-scale viticultural systems, in the context of the climate change that Chilean agriculture is currently facing. For this, we will present: 1—Results on the effect of incorporating or not, functional microorganisms in organic vineyards, 2—An experiment where the different treatments consist in adding (at consecutive times) different functional microorganisms in organic vineyards, 3—Results, at nursery level, on the short-term effect of inoculating grapevine plants with mycorrhiza-forming fungi, and 4—An experiment in which the effect of applying effective microorganisms in conjunction with a sugar source is evaluated in organic vineyards. In summary, this research aimed to evaluate the efficacy of various microbiome manipulation strategies in real-world field conditions, in an agricultural setting that is experiencing substantial impacts from climate change (Young et al., 2010; Roco et al., 2014, 2017). Consequently, the findings of this study offer practical value to farmers engaged in organic viticulture, as they search for feasible strategies to adapt to the challenging realities imposed by climate conditions.
2 Materials and methods
This article shows data obtained under real commercial field management conditions. All trials were conducted in commercially active fields, the harvests of which were taken to vinification. For this same reason, when the response variables involve destruction or damage to plants, the sample sizes conform to the minimum suggested for conducting efficacy trials (Kalamarakis and Markellou, 2007; EPPO, 2012a,b). The treatments used in the different experiments consist of bacterial and/or fungal plant growth-promoting consortia, based on evidence showing that this strategy is superior to the use of monospecific treatments (Carreiras et al., 2023). This is because the use of a single strain of microorganism does not allow benefiting from the synergistic effects offered by consortia, thanks to the activation of different growth-promoting mechanisms and the interaction between them (Mesa-Marín et al., 2019; Redondo-Gómez et al., 2022).
For the reasons set forth above, the following criteria were used in conducting the experiments: 1—The trials were carried out in actively producing crop fields, which have similar characteristics (in terms of soil, area, planting density, and varieties used) to the production units characteristic of the regions in which the experiments were conducted. 2—The microbial consortia used are readily available in commercial formulations, and the species included are of recognized utility in organic farming. 3—The response variables evaluated in the experiments are of easy agronomic interpretation and are routinely measured and used in commercial vineyards to make management decisions. These variables were measured using the techniques routinely used in the vineyards. This set of criteria aims to encourage the use of the results of this work by farmers.
The characteristics of four experiments are presented below. In all cases the microorganisms were used under the hypothesis that their incorporation into the system would improve the performance of plants under climatic stress conditions (Barrow et al., 2008; Aguilera et al., 2022; Carreiras et al., 2023), due to high solar radiation, high temperatures, low relative humidity and reduced rainfall levels. The microorganisms used in the experiments are part of commercial formulations available in Chile, approved for use in organic agriculture according to the USDA-NOP and EU-Chilean 20.089 standards. In all cases, the extra ingredients found in the treatments are either part of the commercial formulations used in the experiments (i.e., co-formulants, humic acid and seaweed extracts) or are incorporated to evaluate their effect in conjunction with the microorganisms (i.e., natural nanoparticles, composted hyacinth extract and sugar). In all cases, multiple modeling techniques were used to analyze the data (conventional analysis of variance, non-parametric analysis by ranks, generalized linear models, and permutation analysis of variance). The details of the analyses used in each case are presented after the description of the treatments for each experiment.
2.1 Experiment 1
This experiment was conducted during the 2020–2021 season in Santa María commune (Valparaiso Region, Chile) on a total area of 8 ha planted with Cabernet Sauvignon variety (established in 2012). The vineyard has a planting distance of 2.2 m between-rows by 1.2 m in-rows, using a simple trellis system, under a controlled drip irrigation system. The site has a loam soil, with 1.8% of organic matter and pH 7.5. Daily values of air temperature (average), accumulated precipitation, and solar radiation during the experiment are shown in the Supplementary Table S1. A completely randomized one-way classification design was used (Montgomery, 2004), to evaluate the effect of incorporating into the soil (via fertigation) efficient microorganisms, in contrast to a control. Treatments are identified as: T1—Control, T2—Incorporation of effective microorganisms. Fifteen replicates of each treatment were carried out. Each experimental unit consisted of 18.180 linear meters. In total, each treatment occupied an area of 4 ha. In treatment 2, microorganisms were applied on two dates (see details in Table 1).
The variables evaluated are associated with root development (root weight) and vigor expression (pruning weight). Both are important parameters in commercial wine production. To calculate the weight of the roots, trial pits were made using the modified monolith method (Böhm, 1979). For this purpose, a block of soil (60 cm wide x 60 cm deep x 240 cm long) was extracted from the west side of the plants and the roots were obtained from it. The roots were washed and weighed in the laboratory. To determine the pruning weight, the commercial pruning of a portion of the vineyard called “claro” or “entreposte” (a 6-meter portion of a row with 5 vine plants) was carried out. In other words, after winter pruning of vineyard, the weight of plant material removed was quantified.
The following analyses were performed on the data obtained (Montgomery, 2004): 1—Kolmogorov–Smirnov normality test, 2—Levene’s test for homogeneity of variances. Where these assumptions were met, the variables were evaluated using T-tests. In cases where non-normality and/or heteroscedasticity deviations occurred, which could not be corrected using Box-Cox transformations (Dag and Ilk, 2017), variables were also evaluated using the nonparametric Mann–Whitney rank test (Montgomery, 2004). Generalized linear models and permutation analysis of variance (Permanova) were also performed for each response variable (Anderson, 2001; Bolker et al., 2009). All analyses were performed in the R programming environment (R Core Team, 2021), according to the protocols outlined in Bates et al. (2015), Lawson (2015) and Oksanen et al. (2020) for the indicated analyses.
2.2 Experiment 2
The experiment was conducted during the 2020–2021 season in Chimbarongo Commune (Libertador General Bernardo O’Higgins Region, Chile) on a total area of 10 ha planted with Cabernet Sauvignon variety (established in 2005). The vineyard has a planting distance of 1.8 m between-rows by 1 m in-rows, using a simple trellis system, under a controlled drip irrigation system. The site has a loam soil, with 2.4% of organic matter and pH 6.1. Daily values of air temperature (average), accumulated precipitation, and solar radiation during the experiment are shown in the Supplementary Table S2. A completely randomized one-way classification design was used (Montgomery, 2004), to evaluate the effect of incorporating into the soil (via fertigation) different combinations and application times of efficient microorganisms, plus a control. The different treatments were (see details in Table 2): T1—Control, T2—Application (at a single point in time) of a set of microorganisms, T3—Sequential application of different sets of microorganisms and T4—Sequential application of different sets of microorganisms (same as those used in T3) plus natural nanoparticles. Treatments 2, 3 and 4 were established by crop experts and bioinput suppliers’ recommendations. There were 10 replicates of each treatment, each experimental unit consisted of 95 rows. The same variables already described in Experiment 1 were evaluated (using the same methodologies).
The following analyses were performed on the data obtained (Montgomery, 2004): 1—Kolmogorov–Smirnov normality test, 2—Levene’s test for homogeneity of variances. Where these assumptions were met, the variables were evaluated using ANOVAs. In cases where non-normality and/or heteroscedasticity deviations occurred, which could not be corrected using Box-Cox transformations (Dag and Ilk, 2017), variables were also evaluated using the Kruskal–Wallis rank tests (Montgomery, 2004). Generalized linear models and permutation analysis of variance (Permanova) were also performed for each response variable (Anderson, 2001, Bolker et al., 2009). All analyses were performed in the R programming environment (R Core Team, 2021), according to the protocols outlined in Bates et al. (2015), Lawson (2015) and Oksanen et al. (2020) for the indicated analyses.
2.3 Experiment 3
The experiment was conducted during the 2021–2022 season in Chimbarongo Commune (Libertador General Bernardo O’Higgins Region, Chile), in a nursery (use to obtain plants for replanting). A completely randomized one-way classification design (Montgomery, 2004) was used to evaluate the effect, in Sauvignon Blanc grapevines planted in nursery, of different forms of mycorrhiza-forming fungi application, plus a control. Specifically, three treatments were evaluated (see details in Table 3): T1—Control, T2—Application via drenching of 2 g of mycorrhizae in a 250 mL solution with non-chlorinated water, applied with a pitcher on the substrate in which the vines were planted (i.e., post-planting) and T3—Immersion of roots for 10 min in a 200 lt solution containing mycorrhizae (1 g of mycorrhizae: 125 mL of non-chlorinated water) (i.e., prior to planting).
In all cases the plants were planted on standard substrate (see Table 4) of grapevine nursery, in plastic bags (5 L). Daily values of air temperature (average), accumulated precipitation, and solar radiation during the experiment are shown in the Supplementary Table S3. The effect of treatments on root weight was evaluated. For this purpose, the plants were extracted from the bags and the substrate adhered to the roots was removed with a pressure washer. The roots were cut and taken to the laboratory, where they were weighed. Measurements were made at two different times: 180 days after application and 240 days after application. The two measurements were made on different plants. At each measurement time, 20 plants per treatment were evaluated. The data were analyzed using the same methodology described in experiment 2.
2.4 Experiment 4
The experiment was conducted during the 2022–2023 season in the Santa María Commune (Valparaiso Region, Chile) on a total area of 6 ha planted with Cabernet Sauvignon variety (established in 2010). The vineyard has a planting distance of 2.2 m between-rows by 1.2 m in-rows, using a simple trellis system, under a drip irrigation system. The site has a loam soil, with 1.8% of organic matter and pH 7.5. Daily values of air temperature (average), accumulated precipitation, and solar radiation during the experiment are shown in the Supplementary Table S4. A completely randomized two-way classification design (Montgomery, 2004) was used to evaluate the effect of two factors (each with two levels). The factors evaluated were: Factor 1—incorporation or not of efficient microorganisms to the soil, and Factor 2—application or not of a sugar source to the crop (jointly to leaves and soil). A full factorial design (Montgomery, 2004) was used to evaluate the effect of the different combinations of factor levels in the bunch weight at harvest. A total of 810 bunch measurements were made in each of the combinations of the two factors (called treatments and named: T1, T2, T3 and T4).
The sugar source used in this trial was organic cane sugar (28% total sugars, 46% organic matter), in conjunction with a composted hyacinth plant extract (enriched with willow bark). The efficient microorganisms used were: consortium 3, consortium 4 and Trichoderma harzianum (i.e., the microorganisms used in the treatment 3 of experiment 2). The characteristics of the different combinations of inputs evaluated in each treatment are described below:
T1—Control. Neither sugars nor efficient microorganisms were applied.
T2—A sugar source (plus hyacinth) was applied to the foliage and soil as described in Tables 5, 6.
T3—Different efficient microorganisms were applied to the soil as described in Table 7.
T4—A sugar source (plus hyacinth) and soil efficient microorganisms were applied as described in Tables 8, 9.
The following analyses were performed on the response variable (Montgomery, 2004): 1—Kolmogórov-Smirnov normality test, 2—Levene’s test for homogeneity of variances. Since the response variable presented deviations from normality and heteroscedasticity, which could not be corrected using Box-Cox transformations (Dag and Ilk, 2017), it was evaluated by means of an ANOVA performed on the data transformed using the Aligned Rank Transform (ART) procedure (Wobbrock et al., 2011; Elkin et al., 2021). It was also analyzed using a generalized linear model with a gamma-type error distribution (Bolker et al., 2009). Finally, permutation analysis of variance (Permanova) was also performed to the response variable (Anderson, 2001). All analyses were performed in the R programming environment (R Core Team, 2021), according to the protocols outlined in Bates et al. (2015), Lawson (2015) and de Mendiburu and Yaseen (2020), Oksanen et al. (2020) and Kay et al. (2021) for the indicated analyses.
3 Results
Levins (1966) rightly stated that “all models leave out a lot and are in that sense false, incomplete, inadequate,” and Box (1979) in the same vein said that “all models are wrong, some are useful.” For this reason, caution should be exercised in interpreting their results. One way to do this, without renouncing the clear advantages of their use in the interpretation of nature, is through the use of the concept of robustness proposed by Levins (1966). According to this, if multiple representations of reality (models), operating under different assumptions coincide, we are in the presence of a robust result.
In Levins’ (1966) own words: “our truth is the intersection of independent lies.” It is for this reason that here are used multiple approaches to data modeling. In this regard, it is important to mention that the results obtained from these methodologies (conventional analysis of variance, nonparametric analysis of variance by ranks, generalized linear models and analysis of variance by permutations) in the majority of cases coincided, which is an indication of their robustness (in the sense mentioned above).
In all cases the results are summarized with boxplots. The mean value is indicated by a black cross. Multiple comparison results are shown using the compact letter display. In all cases, the multiple comparison tests were performed with an alpha value equal to 0.05 (with Bonferroni correction). In addition, the average value (for each treatment) is presented with numbers and the standard deviation in parentheses.
3.1 Results experiment 1
The root weight variable fits well to a normal distribution (Kolmogorov–Smirnov test statistic D = 0.12939, p-value = 0.2251) and has no significant heteroscedasticity issues (Levene test statistic = 3.2433, p-value = 0.0825). The variable pruning weight does not fit well to a normal distribution (Kolmogorov–Smirnov test statistic D = 0.16511, p-value = 0.03605) and has no significant heteroscedasticity issues (Levene test statistic = 3.7787, p-value = 0.06202). For the latter variable, normality issues could be solved by a Box-Cox transformation with a lambda hat value of −0.32 (Kolmogorov–Smirnov test values on the transformed data: D = 0.08486, p-value = 0.8406).
Incorporating efficient microorganisms was found to have effects (see Figure 1C) on both root growth and pruning weight. This is likely to be achieved through the interaction of microorganisms with roots, fixation atmospheric nitrogen and facilitation of phosphorus uptake, that may result in the stimulation of root and leaf growth (see Figures 1A,B). In both cases the effects are in the desired direction (considering that the plants are subjected to strong stress due to solar radiation and drought, which limits their productive potential and oenological quality). Specifically, in the treatment involving microorganisms is observed: 1—Roots have a higher development (see Figure 1A), which suggests a greater capacity of plants to take advantage of moisture and capture nutrients in the soil, as well as to increase root exploration. 2—In the case vegetative development, there is a higher pruning weight (see Figure 1B), which is associated with greater plant vigor, higher capacity to accumulate photoassimilates and therefore greater productive potential.
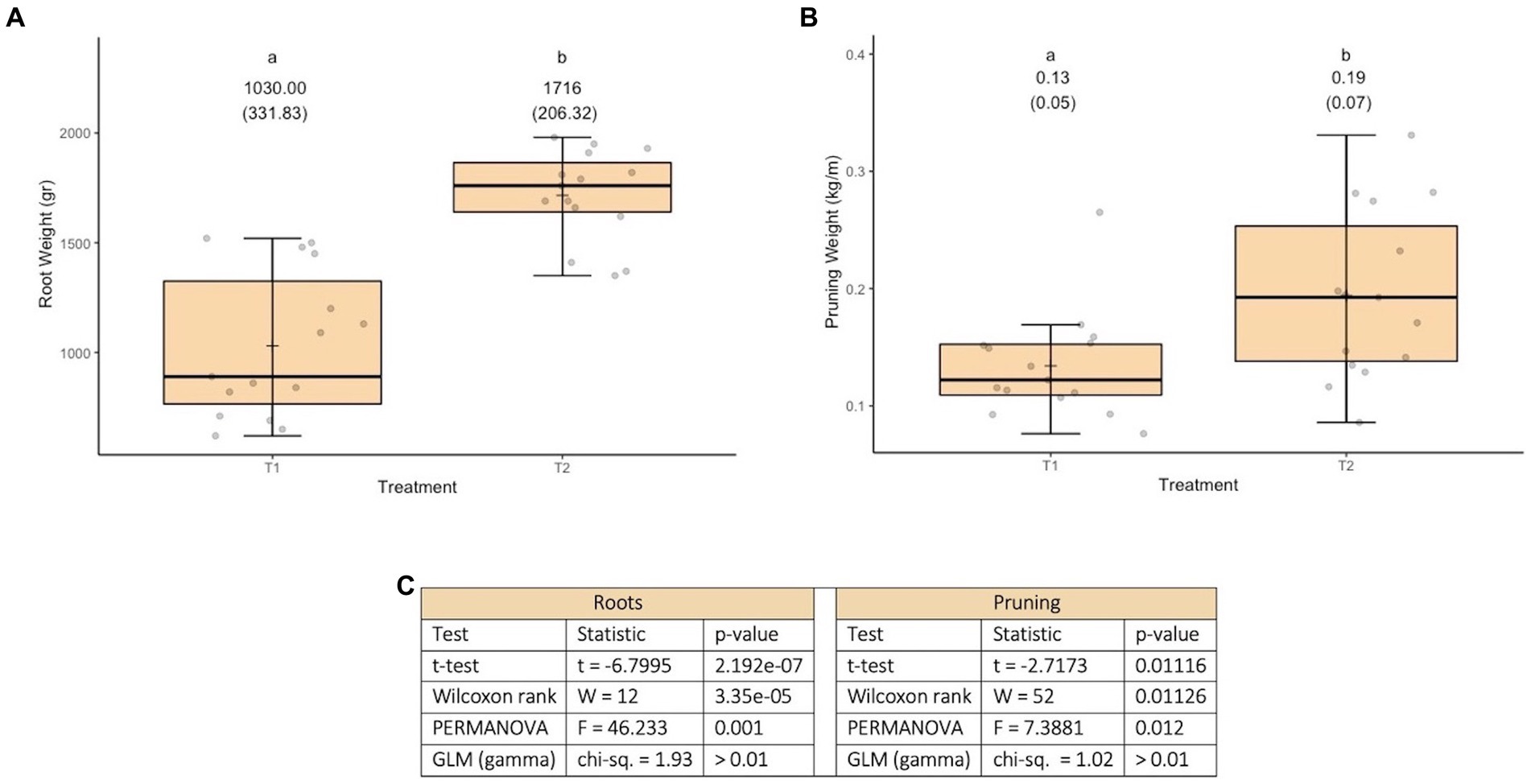
Figure 1. Results of experiment 1. (A) Results of the root weight variable. (B) Results of the pruning weight variable. (C) Values obtained in the tests performed. T1, control; T2, application of microorganisms presents in consortia 1 and 2. GLM, generalized linear model (a gamma type error distribution was used in the GLMs).
3.2 Results experiment 2
The variable root weight fits well to a normal distribution (Kolmogorov–Smirnov test statistic D = 0.12202, p-value = 0.14) and has no issues of heteroscedasticity (Levene test statistic = 0.55928, p-value = 0.6453). The variable pruning weight fits well to a normal distribution (Kolmogorov–Smirnov test statistic D = 0.077778, p-value = 0.7851) and has no heteroscedasticity issues (Levene test statistic = 0.81566, p-value = 0.4937). No effect of treatments was found on the response variables evaluated (see Figure 2C). In terms of the responses to the treatments, it is worth commenting that for root weight a less uniform response is observed, than what was observed for pruning weight (see Figures 2A,B). This possibly has to do with the fact that the roots are directly in the medium in which the microorganisms were incorporated, so their action could be manifested there first.
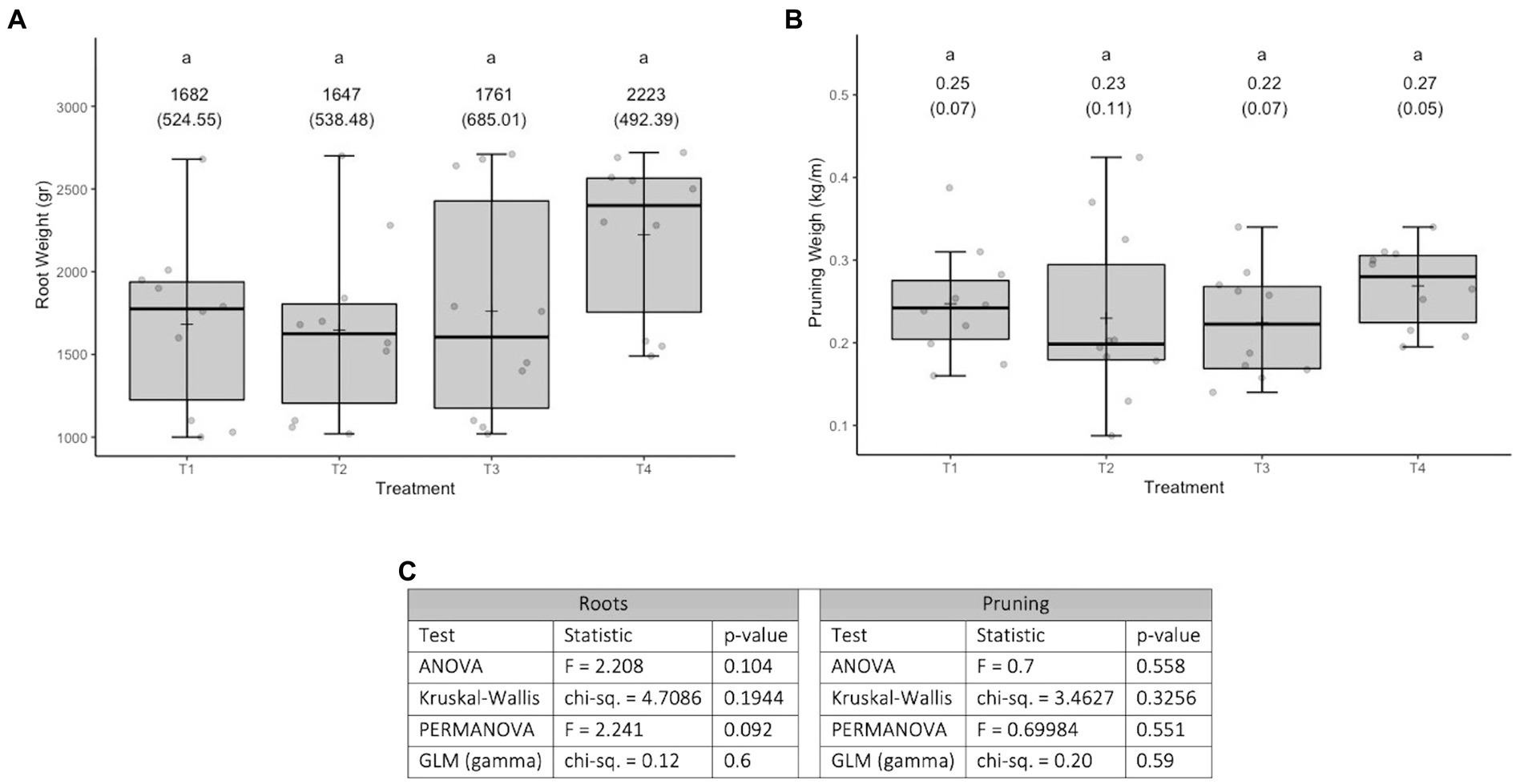
Figure 2. Results of experiment 2. (A) Results of the root weight variable. (B) Results of the pruning weight variable. (C) Values obtained in the tests carried out. T1, Control; T2, Application (in a single moment) of the set of organisms present in consortium 4; T3, Application in sequence of the set of microorganisms present in consortia 3, 4 and 5 (plus Trichoderma harzianum); T4, Application in sequence of the set of microorganisms used in T3, plus natural nanoparticles. GLM: generalized linear model (a gamma type error distribution was used in the GLMs).
3.3 Results experiment 3
The variable root weight in the first measurement (Figure 3A) fits well to a normal distribution (Kolmogorov–Smirnov test statistic D = 0.10192, p-value = 0.126) and has no heteroscedasticity issues (Levene test statistic = 0.81789, p-value = 0.4465). The variable root weight in the second measurement (Figure 3B) fits well to a normal distribution (Kolmogorov–Smirnov test statistic D = 0.065306, p-value = 0.7577) and has no heteroscedasticity issues (Levene test statistic = 0.67022, p-value = 0.5156). The overall relationship between the different treatments is similar for the two measurement times (see Figures 3A,B). Figure 3C shows that, for both measurement moments, the p-values obtained are close to the historical significance threshold of 0.05. However, the p-values obtained on the second date are lower than those obtained on the first date. For the second date, according to the Tukey multiple comparisons test (α =0.05 and Bonferroni adjustment), only the difference between T1 and T2 presents a 95% confidence interval that does not include 0 (see Figure 3D). It is worth mentioning (in term of effect sizes) that in the treatments that involve fungi, the roots weights (in average) at least 20 gr more than the control.
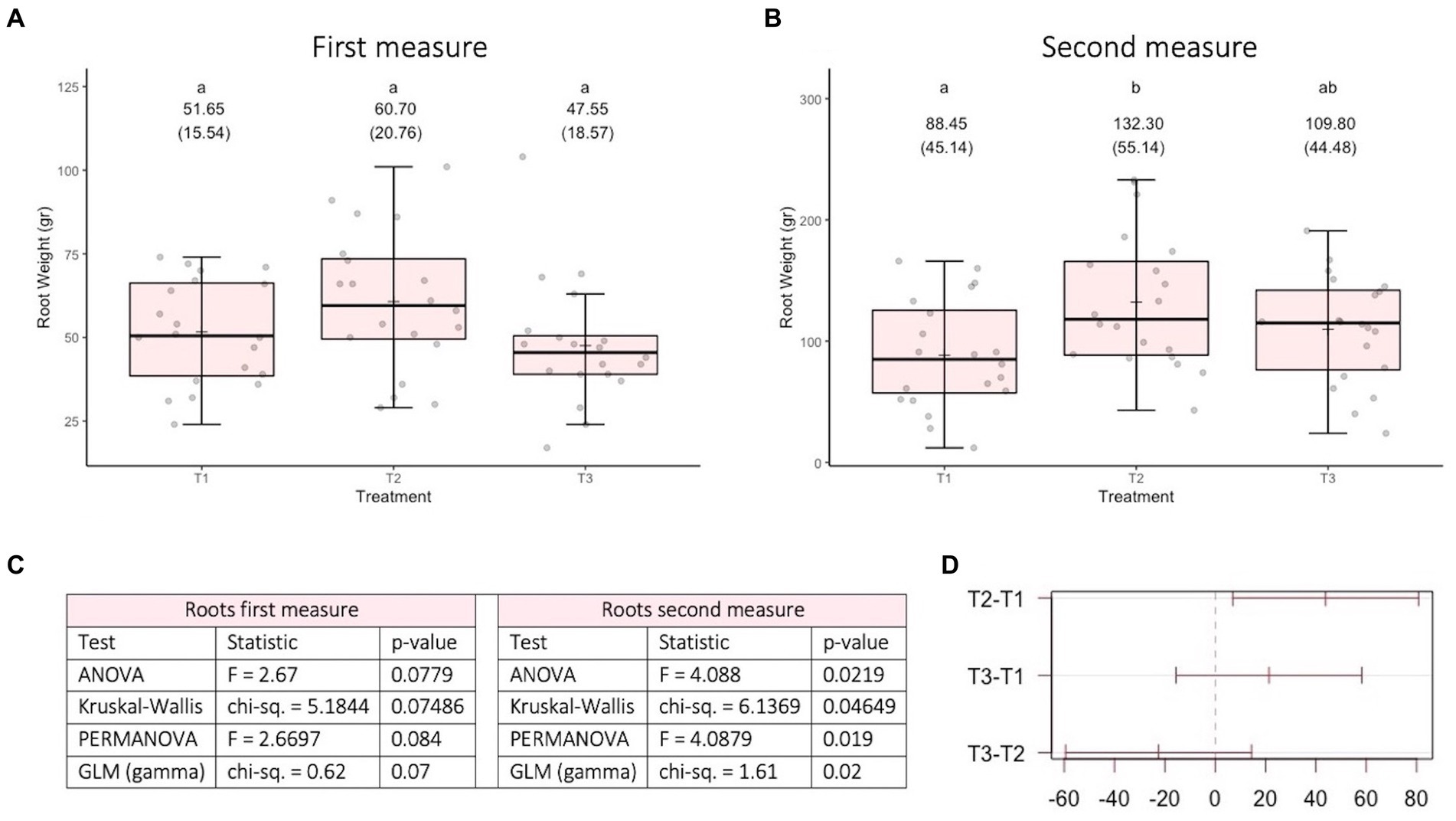
Figure 3. Results of experiment 3. (A) Results of the root weight variable in the first measurement. (B) Results of the root weight variable in the second measurement. (C) Values obtained in the tests performed. (D) 95% confidence interval of the Tukey multiple comparisons test for the second date. T1: Control, T2: Application of the set of organisms present in consortium 6 via drenching; T3, Application of the set of organisms present in consortium 6 via immersion; GLM, generalized linear model (a gamma type error distribution was used in the GLMs).
3.4 Results experiment 4
The response variable of the experiment does not fit well to a normal distribution (Kolmogorov–Smirnov test statistic D = 0.12437, p-value < 2.2e-16) and have significant heteroscedasticity issues (Levene test statistic = 28.848, p-value < 2.2e-1). The variable distribution is skewed to the right and the data fit better to a model with a gamma error distribution (AIC = 32293.04) than to one with a normal distribution (AIC = 33757.9). The issues of non-normality and heteroscedasticity could not be solved by Box-Cox transformation and the data do not meet the requirements of the aligned rank transform procedure (i.e., not all column of the aligned responses sum to zero). Therefore, in this case the best alternatives are a Pernanova and a GLM with a gamma error distribution.
Figure 4 shows that there is a treatment effect, with the best result (higher bunch weight) obtained with treatment 4 (groups created using pairwise Permanovas, with α = 0.05 and a Bonferroni adjustment), which consists of the joint application of efficient microorganisms and a sugar source (plus hyacinth) (see Figure 4A). In fact, the joint application has a positive synergistic effect on bunch weight (see the different slopes in the lines of Figure 4B). It is worth noting that both, the application of microorganisms and sugar separately (i.e., main effects), have positive effects on the response variable. As can be seen in Figures 4E,F for Permanova, both the main effects and their interaction are significant at 0.05 threshold level.
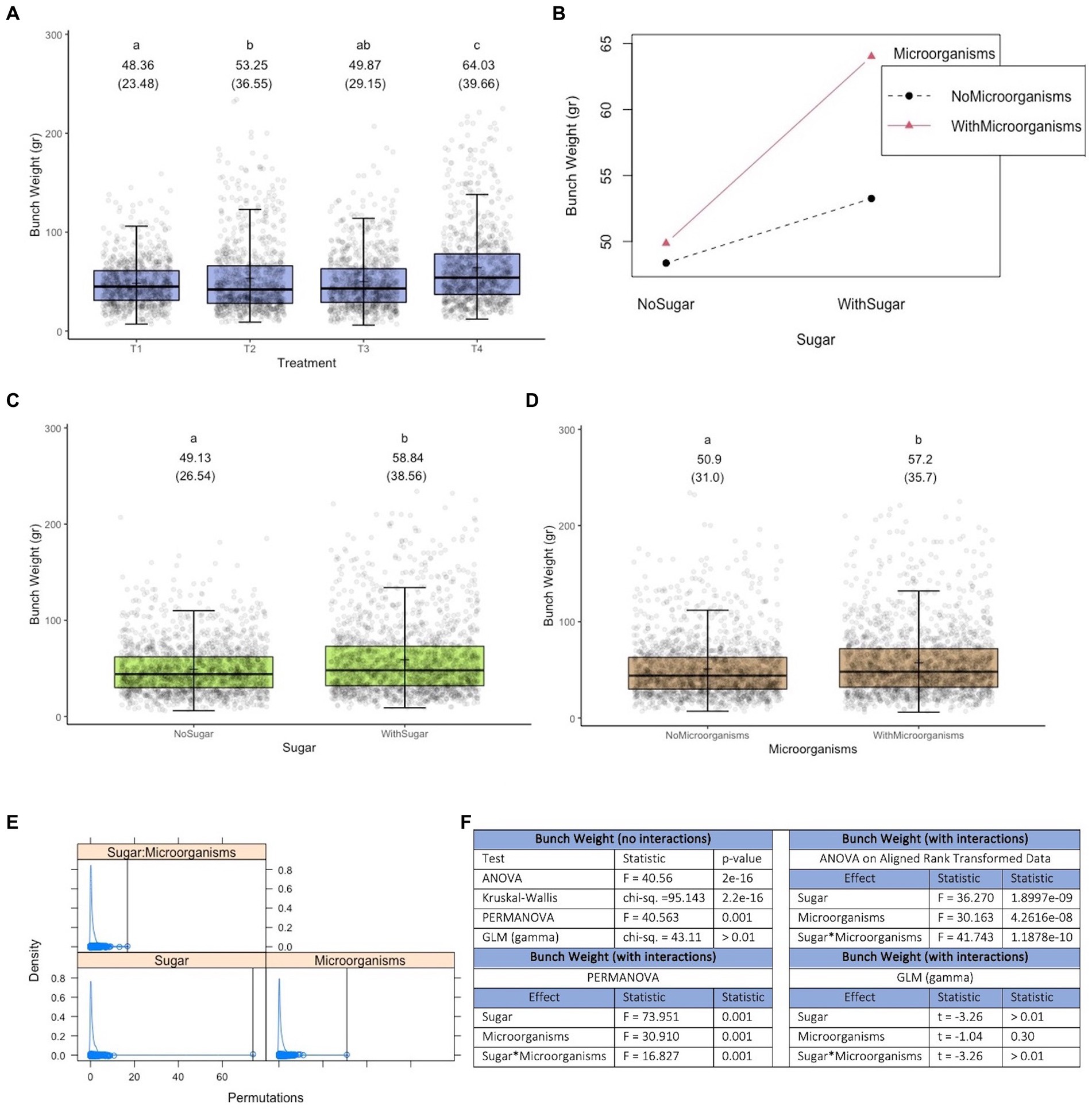
Figure 4. Results of experiment 4. (A) Results of the variable bunch weight. (B) Interaction diagram between the factors microorganisms and sugar. (C) Main effect of the sugar factor. (D) Main effect of the microorganisms factor. (E) Density plot of the results obtained in Permanova [the black vertical lines represent the values of the pseudo-F statistic; its specific values and the associated p-values are presented in (F)]. (F) Values obtained in the tests performed. T1, Control; T2, Application of a sugar source; T3, Application of the set of microorganisms presented in consortia 3, 4 and 5 (plus Trichoderma harzianum); T4, Application of the microorganisms used in T3 and sugar; GLM, generalized linear model (a gamma type error distribution was used in the GLMs).
4 Discussion
Results of experiments 1 and 4 support published evidence on the benefits of efficient microorganisms to agroecosystems (Martínez-Viveros et al., 2010; Schütz et al., 2018; Basu et al., 2021; Ferreira et al., 2021; Antoszewski et al., 2022; Gupta et al., 2022; Notununu et al., 2022). While experiments 2 and 3 results show some elements that should be taken into account when carrying out microbiome manipulations in ecological farming.
With respect to experiment 2, in order to make a fair assessment of its results, it is good to take into account the historical behavior of the vineyard area where it was carried out. Figure 5 shows that the vineyard sector in which it was implemented, has a clear downward trend in its harvests. This area has been under organic management for more than 12 years and shows clear signs of decline. This is a completely different situation from that found in the areas where experiments 1 and 4 were conducted (data not shown).
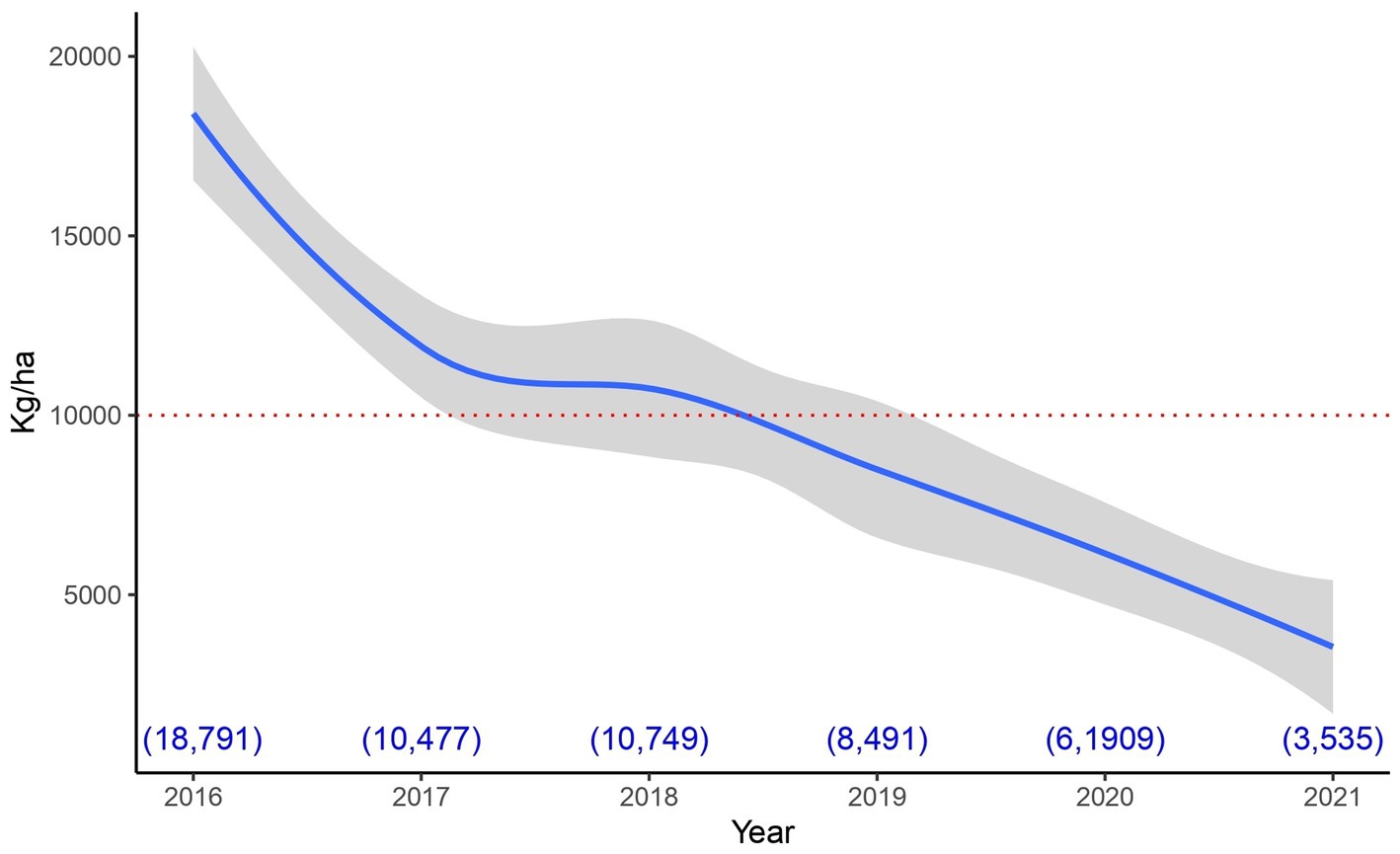
Figure 5. Historical production in the area where experiment 3 was carried out. The average production of the area is presented (with its 95% confidence interval). This was done using the smoothed conditional means procedure (Wickham, 2016). The red dotted line represents the minimum acceptable production according to the vineyard standards. The blue numbers in parentheses at the bottom of the graph represent the average production value per hectare for each year.
It should be noted that, for the vineyard where experiment 2 was conducted, a harvest below 10.000 kg/ha is considered deficient (good values are between: 12.000–14.000 kg/ha). This threshold was not achieved in the 2 years prior to the development of the experiment (see Figure 5), for this reason the different treatments were elaborated by a team of experts to try to address the production deficit. Even treatments inspired by the ecological succession process were tested, these involved the incorporation of different groups of microorganisms at different times (specifically treatments 3 and 4).
The low production associated with the sector where the experiment was carried out is possibly related to a number of causes: 1—adverse effect of weather, 2—excessive level of production before 2017 (well above the threshold of 14.000 kg/ha) and 3—mechanical damage caused (over the years) during weed control (because the distance between the rows is too short for the implement used for weeding). Thus, it is possible that the context in which efficient microorganisms were incorporated limited their effect. It should be noted that, given the historical performance of the sector, the vineyard manager decided to replant the vineyard after 2021 harvest (the year in which the experiment was completed).
It is fundamental to take into account that any manipulation of the microbiome is governed by ecological processes (at population and community levels) and that these take time. In a sense, microbiome interventions in agroecosystems are similar to augmentative approaches to biological control (Horn, 1988). In other words, the population density of certain organisms is artificially increased in order to make them perform an action desired by humans. However, changes in population densities are not immediately effective (Eisenhauer et al., 2010). In the case of soil microorganisms, it must be taken into account that in their action, important density dependent mechanisms intervene, for example, quorum sensing (Duddy and Bassler, 2021). While other mechanisms depend also on interactions between species (Qiao et al., 2023) and the nature of these interactions can change over time depending on various conditions such as, for example, the density of participating species (Bronstein, 1994; Griffon and Hernandez, 2019; Hernandez, 2021; Hanusch et al., 2023). Thus, important ecological phenomena in the soil influence the establishment and colonization of the environment by introduced microorganisms. Phenomena that, depending on different factors, may take different times, but certainly do not act immediately.
The times associated with the ecological phenomena possibly explain the results obtained in experiment 3 (particularly in the first measurement). Here it is worth commenting on the differences between the application modes of treatments 2 and 3 in experiment 3. In the case of treatment 3, the roots were in contact with the microorganism solution for 10 min, while in treatment 2 this solution was incorporated into the plant’s growing substrate. Therefore, in treatment 2, a greater number of microorganisms are incorporated into the medium, which could lead to greater symbiosis with the roots. For this reason, it is likely that this method of application can achieve the population densities necessary to exert an effect on the plants in a shorter time.
The time required for the growth of microorganism populations may also be associated with the synergistic effect observed in experiment 4. Because the sugar addition to the medium can create a favorable context for the rapid growth of microorganism populations. In addition, on previous experiences we have found that sugar has a positive effect on plants under climatic stress conditions. In this sense, it is important to mention that, in experiments not presented here (not involving efficient microorganisms), only applications of sugar to soil and leaves (together) were found to have positive effects on bunch size. The physiological explanation of this result in terms of fine mechanisms is unknown to us. It is an adaptation strategy inspired by a similar practice used in the management of avocado trees under stress due to climate change in Peru. These results may indicate that the effects of sugar extend to the microbiome found in the plant shoots. It is important to note that the consortia used in this experiment are the same as those used in the treatment 3 of experiment 2, which points out the importance of incorporating efficient microorganisms in a favorable environment.
Recently, there has been renewed interest in the holobiont concept, originally proposed by Lynn Margulis (1991). It accounts for the combination of the host (in this case the plant) with its microbiome. In other words, a holobiont is a composite entity, consisting of a host together with its microbiome (Roughgarden, 2020). It is important to mention that this controversial proposal already has an eco-evolutionary biomathematical theory that supports it (Roughgarden, 2023). In the context of ecological farming, what is really important is that selection on the holobiont, causes evolutionary changes in the traits of the holobiont itself (Roughgarden, 2020; Mesny et al., 2023; Wolfgang et al., 2023). This is particularly important for evolutionary breeding, which is a breeding strategy that really makes sense for ecological farming (Ceccarelli and Grando, 2020).
Evolutionary breeding is based on Fisher’s fundamental theorem of natural selection (Fisher, 1999), which states that the action of natural selection increases the average fitness of populations (as long as they present genetic variation). This theorem can be extended to a context of species interactions (León and Charlesworth, 1978). Thus, the objective of evolutionary breeding is that the forces of evolution act on the agroecosystem as a whole (Ceccarelli et al., 2022). In this context, the co-evolution of the microbiome with the rest of the system is fundamental. Now, for this to be possible, this component must be explicitly included in the breeding programs with an evolutionary approach. However, this promising research (and field management) program should not be taken as an invitation to introduce exotic microorganisms into agroecosystem soils, as there is a long history of failed introductions with disastrous consequences (Ladau et al., 2023). On the contrary, these programs should be based on the use of indigenous organisms.
It is also important to assess, albeit on a subjective note, the impression that this set of experiments left on the people who manage these agricultural systems. In this regard, in all cases efficient microorganisms were incorporated into the vineyard management schemes. This means an area of 450 ha under regenerative soil management. It is worth noting that the current trend in ecological soil management seems to be towards a regenerative type of management, which not only involves fixing atmospheric carbon in the soil and incorporating rhizobacteria and mycorrhizal fungi, but also seeks to incorporate microorganisms such as predatory nematodes, amoebae, protozoa and aerobic fungi, thus increasing the complexity of the system (Ingham, 2000; Pane et al., 2012; St. Martin, 2014; Johns, 2017; St. Martin et al., 2020; White, 2020; Lazarova et al., 2021; Curadelli et al., 2023; Eon et al., 2023; Mishra et al., 2023). Therefore, the characterization and understanding of the ecological interaction network of soils is a promising research program, that can provide valuable results for regenerative agriculture in the near future.
It is also important to highlight that the results presented here correspond to exploratory experiments. These motivate and suggest other questions to be addressed. For example, it is interesting to evaluate if there is a threshold density at which microorganisms begin to have a positive effect on plants. Hence, a subsequent step could involve conducting experiments in which different concentrations of microorganisms are evaluated. Similarly, it is worthwhile to study if there is an optimal concentration for the applications. This last question could be explored using the response surface methodology (Montgomery, 2004). Also, it is compelling to study if there is an optimal structure (e.g., in terms of species richness) for the applied microbial consortia, this question could be explored using treatments of increasing complexity.
Finally, it is useful to draw comparisons with other studies on the subject, to highlight the particularities of our study, specifically regarding our experimental setups. For instance, Carreiras et al. (2023) elegantly demonstrated the potential of marine plant growth-promoting rhizobacteria consortia as an eco-friendly solution for mitigating heatwave stress in vineyards. Their research was conducted under greenhouse conditions, with plants potted and treated with microorganism consortia prepared and applied under controlled conditions, with light and temperature also controlled and the experiment was relatively small-scale (treatments consisted of 5 replicate plants). Such experimental setups are crucial for advancing knowledge in the field. However, they significantly differ from the conditions experienced during fieldwork on commercial farms, which can hinder their adoption by farmers. For this reason, our study was specifically designed to mirror real field management conditions, making it more relatable for farmers.
Naturally, our approach comes with certain trade-offs in accuracy (particularly in controlling sources of variation). Factors such as temperature, rainfall, and sunlight (which are beyond our control in the experiments) influence grape growth. Nevertheless, our study was conceived with the understanding that these factors represent the real-world variability that agriculture must adapt to. We believe that developing viable adaptation strategies requires both types of research (those conducted under clear-cut controlled conditions and those under real field conditions), because these approaches are complementary.
From a long-term perspective, soil microbiome manipulation can be used as an adaptation strategy to the new climatic conditions facing agriculture. Through an adequate configuration of stimuli, which could be partially incentivized by public policies, these microorganisms could be multiplied in the farms themselves. This can be achieved through simple techniques, such as the production of compost tea (Ingham, 2003), and thus help reduce the costs that affect the economic viability of the sector (Reganold and Wachter, 2016; Meemken and Qaim, 2018; Łuczka and Kalinowski, 2020).
5 Conclusion
Manipulation of the microbiome in large-scale organic farming as a climate change adaptation strategy is feasible. But in its execution, it must be taken into account that this promising management strategy is governed by ecological processes that take time. This is why these manipulations cannot be expected to have effect automatically. Similarly, in agroecosystems subjected to different forms of stress associated with poor overall condition, it is possible that the microorganisms fail to establish themselves and thus exert positive effects on the system. In short, manipulation of the microbiome is a regenerative soil management strategy that has great potential, but is by no means a panacea.
Data availability statement
The raw data supporting the conclusions of this article will be made available by the authors, without undue reservation.
Author contributions
CP: Conceptualization, Data curation, Formal analysis, Funding acquisition, Investigation, Methodology, Project administration, Resources, Software, Supervision, Validation, Visualization, Writing – original draft, Writing – review & editing. DG: Conceptualization, Data curation, Formal analysis, Funding acquisition, Investigation, Methodology, Project administration, Resources, Software, Supervision, Validation, Visualization, Writing – original draft, Writing – review & editing.
Funding
The author(s) declare that no financial support was received for the research, authorship, and/or publication of this article.
Acknowledgments
Our gratitude to Natalie Moreno, Isolina Aguilar, Gabriela Rodríguez, Oscar Valenzuela, Pedro Moreno, Arnaldo Rodríguez, Ricardo Ríos, Pilar Browne, Maximiliano Muñoz, INIA Chile, and Cono Sur Vineyards & Winery.
Conflict of interest
The authors declare that the research was conducted in the absence of any commercial or financial relationships that could be construed as a potential conflict of interest.
Publisher’s note
All claims expressed in this article are solely those of the authors and do not necessarily represent those of their affiliated organizations, or those of the publisher, the editors and the reviewers. Any product that may be evaluated in this article, or claim that may be made by its manufacturer, is not guaranteed or endorsed by the publisher.
Supplementary material
The Supplementary material for this article can be found online at: https://www.frontiersin.org/articles/10.3389/fsufs.2024.1285981/full#supplementary-material
References
Agler, M. T., Ruhe, J., Kroll, S., Morhenn, C., Kim, S. T., Weigel, D., et al. (2016). Microbial hub taxa link host and abiotic factors to plant microbiome variation. PLoS Biol. 14:e1002352. doi: 10.1371/journal.pbio.1002352
Aguilera, P., Ortiz, N., Becerra, N., Turrini, A., Gaínza-Cortés, F., and Silva-Flores, P. (2022). Application of arbuscular mycorrhizal fungi in vineyards: water and biotic stress under a climate change scenario: new challenge for Chilean grapevine crop. Front. Microbiol. 13:826571. doi: 10.3389/fmicb.2022.826571
Albornoz, F. E., Prober, S. M., Ryan, M. H., and Standish, R. J. (2022). Ecological interactions among microbial functional guilds in the plant-soil system and implications for ecosystem function. Plant Soil 476, 301–313. doi: 10.1007/s11104-022-05479-1
Anderson, M. J. (2001). A new method for non-parametric multivariate analysis of variance. Austral Ecol. 26, 32–46. doi: 10.1111/j.1442-9993.2001.01070.pp.x
Anthony, M. A., Bender, S. F., and van der Heijden, M. G. A. (2023). Enumerating soil biodiversity. PNAS 120:e2304663120. doi: 10.1073/pnas.2304663120
Antoszewski, M., Mierek-Adamska, A., and Dąbrowska, G. B. (2022). The importance of microorganisms for sustainable agriculture – a review. Metabolites. 12:1100. doi: 10.3390/metabo12111100
Aparicio, E. (2021). Emergencia Climática por sequía: medidas que requieren de facultades extraordinarias que no existen. El Mostrador. Available at: https://www.elmostrador.cl/cultura/2021/08/18/emergencia-climatica-por-sequia-medidas-que-requieren-de-facultades-extraordinarias-que-no-existen/ (Accessed August 23, 2023).
Archer, D., and Rahmstorf, S. (2010). The climate crisis: An introductory guide to climate change. Cambridge: Cambridge University Press.
Arif, I., Batool, M., and Schenk, P. M. (2020). Plant microbiome engineering: expected benefits for improved crop growth and resilience. Trends Biotechnol. 38, 1385–1396. doi: 10.1016/j.tibtech.2020.04.015
Arora, N. K. (2019). Impact of climate change on agriculture production and its sustainable solutions. Environ. Sustain. 2, 95–96. doi: 10.1007/s42398-019-00078-w
Barrow, J. R., Lucero, M. E., Reyes-Vera, I., and Havstad, K. M. (2008). Do symbiotic microbes have a role in regulating plant performance and response to stress? Commun. Integr. Biol. 1, 69–73. doi: 10.4161/cib.1.1.6238
Basu, A., Prasad, P., Das, S. N., Kalam, S., Sayyed, R. Z., Reddy, M. S., et al. (2021). Plant growth promoting Rhizobacteria (PGPR) as green bioinoculants: recent developments, constraints, and prospects. Sustain. For. 13:1140. doi: 10.3390/su13031140
Bates, D., Mächler, M., Bolker, B., and Walker, S. (2015). Fitting linear mixed-effects models using lme4. J. Stat. Softw. 67, 1–48. doi: 10.18637/jss.v067.i01
Berg, G., Rybakova, D., Fischer, D., Cernava, T., Vergès, M., Charles, T., et al. (2020). Microbiome definition re-visited: old concepts and new challenges. Microbiome. 8, 103–122. doi: 10.1186/s40168-020-00875-0
Böhm, W. (ed.) (1979). “Monolith methods” in Methods of studying root systems. Ecological studies, vol. 33 (Berlin, Heidelberg: Springer).
Bolker, B. M., Brooks, M. E., Clark, C. J., Geange, S. W., Poulsen, J. R., Stevens, H. H., et al. (2009). Generalized linear mixed models: a practical guide for ecology and evolution. Trends Ecol. Evol. 24, 127–135. doi: 10.1016/j.tree.2008.10.008
Box, G. E. P. (1979). “Robustness in the strategy of scientific model building” in Robustness in statistics. eds. R. L. Launer and G. N. Wilkinson (Cambridge, MA: Academic Press), 201–236.
Bronstein, J. L. (1994). Conditional outcomes in mutualistic interactions. Trends Ecol. Evol. 9, 214–217. doi: 10.1016/0169-5347(94)90246-1
Carreiras, J., Cruz-Silva, A., Fonseca, B., Carvalho, R. C., Cunha, J. P., Proença Pereira, J., et al. (2023). Improving grapevine heat stress resilience with marine plant growth-promoting Rhizobacteria consortia. Microorganisms. 11:856. doi: 10.3390/microorganisms11040856
Ceccarelli, S., and Grando, S. (2020). Evolutionary plant breeding as a response to the complexity of climate change. iScience 23:101815. doi: 10.1016/j.isci.2020.101815
Ceccarelli, S., Grando, S., Salimi, M., and Razavi, K. (2022). “Evolutionary populations for sustainable food security and food sovereignty” in Seeds for diversity and inclusion. eds. Y. Nishikawa and M. Pimbert (Cham: Palgrave Macmillan).
Chakrabarty, D. (2021). The climate of history in a planetary age. Chicago: University of Chicago Press.
Chouhan, G. K., Verma, J. P., Jaiswal, D. K., Mukherjee, A., Singh, S., de Araujo Pereira, A., et al. (2021). Phytomicrobiome for promoting sustainable agriculture and food security: opportunities, challenges, and solutions. Microbiol. Res. 248:126763. doi: 10.1016/j.micres.2021.126763
Coombe, B. G. (1987). Influence of temperature on composition and quality of grapes. Acta Hortic. 206, 23–36. doi: 10.17660/ActaHortic.1987.206.1
Crowley, W. K. (2000). Chile’s wine industry: historical character and changing geography. In Yearbook. Conference of Latin Americanist Geographers, Vol. 26, pp. 87–101. Available at: http://www.jstor.org/stable/25765889 (Accessed August 23, 2023).
Curadelli, F., Alberto, M., Uliarte, E. M., Combina, M., and Funes-Pinter, I. (2023). Meta-analysis of yields of crops fertilized with compost tea and anaerobic Digestate. Sustain. For. 15:1357. doi: 10.3390/su15021357
Dag, O., and Ilk, O. (2017). An algorithm for estimating Box-cox transformation parameter in ANOVA. Commun. Stat. B: Simul. Comput. 46, 6424–6435. doi: 10.1080/03610918.2016.1204458
Dalgaard, T., Hutchings, N. R., and Porter, J. J. (2003). Agroecology, scaling and interdisciplinarity. Agric. Ecosyst. Environ. 100, 39–51. doi: 10.1016/S0167-8809(03)00152-X
de Araujo Avila, G. M., Gabardo, G., Clock, D. C., and de Lima Junior, O. S. (2021). Use of efficient microorganisms in agriculture. Res., Soc. Dev. 10:e40610817515. doi: 10.33448/rsd-v10i8.17515
de Mendiburu, F., and Yaseen, M. (2020). Agricolae: Statistical procedures for agricultural research. Available at: https://cran.r-project.org/package=agricolae (Accessed August 23, 2023).
del Pozo, A., Brunel-Saldias, N., Engler, A., Ortega-Farias, S., Acevedo-Opazo, C., Lobos, G. A., et al. (2019). Climate change impacts and adaptation strategies of agriculture in Mediterranean-climate regions (MCRs). Sustain. For. 11:2769. doi: 10.3390/su11102769
Droulia, F., and Charalampopoulos, I. (2021). Future climate change impacts on European viticulture: a review on recent scientific advances. Atmosphere 12:495. doi: 10.3390/atmos12040495
Duddy, O. P., and Bassler, B. L. (2021). Quorum sensing across bacterial and viral domains. PLoS Pathog. 17:e1009074. doi: 10.1371/journal.ppat.1009074
Eisenhauer, N., Beßler, H., Engels, C., Gleixner, G., Habekost, M., Milcu, A., et al. (2010). Plant diversity effects on soil microorganisms support the singular hypothesis. Ecology 91, 485–496. doi: 10.1890/08-2338.1
Elkin, L. A., Kay, M., Higgins, J. J., and Wobbrock, J. O. (2021). An aligned rank transform procedure for multifactor contrast tests. Proceedings of the 34th Annual ACM Symposium on User Interface Software and Technology. doi: 10.1145/3472749.3474784
Eon, P., Deogratias, J. M., Robert, T., Coriou, C., Bussiere, S., Sappin-Didier, V., et al. (2023). Ability of aerated compost tea to increase the mobility and phytoextraction of copper in vineyard soil. J. Environ. Manag. 325:116560:116560. doi: 10.1016/j.jenvman.2022.116560
EPPO (2012a). Introduction to the efficacy evaluation of plant protection products. EPPO Bull. 42, 398–402. doi: 10.1111/epp.2585
EPPO (2012b). Design and analysis of efficacy evaluation trials. EPPO Bull. 42, 367–381. doi: 10.1111/epp.2610
FAO/OIV . (2021). Annual assessment of the world vine and wine sector in 2021 international organisation of vine and wine intergovernmental organisation. Available at: https://www.oiv.int/sites/default/files/documents/OIV_Annual_Assessment_of_the_World_Vine_and_Wine_Sector_in_2021.pdf (Accessed August 23, 2023).
Fernández, F. J., Blanco, M., Ponce, R. D., Vásquez-Lavín, F., and Roco, L. (2019). Implications of climate change for semi-arid dualistic agriculture: a case study in Central Chile. Reg. Environ. Chang. 19, 89–100. doi: 10.1007/s10113-018-1380-0
Ferreira, M. J., Cunha, A., Figueiredo, S., Faustino, P., Patinha, C., Silva, H., et al. (2021). The root microbiome of Salicornia Ramosissima as a seedbank for plant-growth promoting halotolerant bacteria. Appl. Sci. 11:2233. doi: 10.3390/app11052233
Fisher, R. A. (1999). The genetical theory of natural selection. Oxford: Oxford University Press (originally published in 1930).
Fraga, H., Malheiro, A.-C., Moutinho-Perreira, J., and Santos, J. (2012). An overview of climate change impacts on European viticulture. Food Energy Secur. 1, 94–110. doi: 10.1002/fes3.14
Fraga, H., Molitor, D., Leolini, L., and Santos, J. A. (2020). What is the impact of heatwaves on European viticulture? A modelling assessment. Appl. Sci. 10:3030. doi: 10.3390/app10093030
Greer, D. H., Mark, M., and Weedon, M. M. (2013). The impact of high temperatures on Vitis vinifera cv. Semillon grapevine performance and berry ripening. Front. Plant Sci 4:491. doi: 10.3389/fpls.2013.00491
Greer, D. H., Weston, C., Greer, D. H., and Weston, C. (2010). Heat stress affects flowering, berry growth, sugar accumulation and photosynthesis of Vitis Vinifera cv. Semillon grapevines grown in a controlled environment. Funct. Plant Biol. 37, 206–214. doi: 10.1071/FP09209
Griffon, D., and Hernandez, M. J. (2019). Some theoretical notes on agrobiodiversity: spatial heterogeneity and population interactions. Agroecol. Sustain. Food Syst. 44, 795–823. doi: 10.1080/21683565.2019.1649781
Gupta, R., Anand, G., Gaur, R., and Yadav, D. (2021). Plant-microbiome interactions for sustainable agriculture: a review. Physiol. Mol. Biol. Plants 27, 165–179. doi: 10.1007/s12298-021-00927-1
Gupta, A., Mishra, R., Rai, S., Bano, A., Pathak, N., Fujita, M., et al. (2022). Mechanistic insights of plant growth promoting bacteria mediated drought and salt stress tolerance in plants for sustainable agriculture. Int. J. Mol. Sci. 23:3741. doi: 10.3390/ijms23073741
Hadarits, M., Smit, B., and Diaz, H. (2010). Adaptation in viticulture: a case study of producers in the Maule region of Chile. J. Wine Res. 21, 167–178. doi: 10.1080/09571264.2010.530109
Haddad, E., Aroca, P., Jano, P., Rocha, A., and Pimenta, B. (2020). A bad year? Climate variability and the wine industry in Chile. Wine Econ. Policy 9, 23–35. doi: 10.36253/web-7665
Hanusch, M., He, X., Janssen, S., Selke, J., Trutschnig, W., and Junker, R. R. (2023). Exploring the frequency and distribution of ecological non-monotonicity in associations among ecosystem constituents. Ecosystems 26, 1819–1840. doi: 10.1007/s10021-023-00867-9
Hernandez, M. J. (2021). The big ifs in the outcomes of species interactions: review and insights from the interaction function (IF) model. Rev. Mod. Mat. Sist. Biol. 1, 73–90.
Horn, D. J. (1988). Ecological approach to pest management. Elsevier Applied Science Publishers Ltd., London.
Johns, C. (2017). Living soils: the role of microorganisms in soil health. Fut Direct Intl. Available at: https://apo.org.au/node/96931 (Accessed August 23, 2023).
Jones, G. V., Edwards, E. J., Bonada, M., Sadras, V. O., Krstic, M. P., and Herderich, M. J. (2022). Climate change and its consequences for viticulture. Manag. Wine Qual. 1, 727–778. doi: 10.1016/B978-0-08-102067-8.00015-4
Kalamarakis, A. E., and Markellou, E. (2007). Efficacy evaluation of plant protection products at EU level: data requirements and evaluation principles. J. Pestic. Sci. 32, 1–9. doi: 10.1584/jpestics.K06-13
Kay, M., Elkin, L. A., Higgins, J. J., and Wobbrock, J. O. (2021). ARTool: aligned rank transform for nonparametric factorial ANOVAs. R package version 0.11.1. Available at: https://github.com/mjskay/ARTool
Ladau, J., Fahimipour, A. K., Newcomer, M. E., Brown, J. B., Vora, G. J., Melby, M. K., et al. (2023). Microbial invasions and inoculants: a call to action. Ecoevorxiv [Preprint]. Available at: https://ecoevorxiv.org/repository/view/5702/ (Accessed August 23, 2023).
Lareen, A., Burton, F., and Schäfer, P. (2016). Plant root-microbe communication in shaping root microbiomes. Plant Mol. Biol. 90, 575–587. doi: 10.1007/s11103-015-0417-8
Lazarova, S., Coyne, D., Rodríguez, M., Peteira, B., and Ciancio, A. (2021). Functional diversity of soil nematodes in relation to the impact of agriculture—a review. MDPI 13:64. doi: 10.3390/d13020064
Lederberg, J., and McCray, A. T. (2001). Ome Sweet'Omics—a genealogical treasury of words. Scientist 15:8.
León, J. A., and Charlesworth, B. (1978). Ecological versions of Fisher's fundamental theorem of natural selection. Ecology 59, 457–464. doi: 10.2307/1936575
Levins, R. (1966). The strategy of model building in population biology. Am. Sci. 54, 421–431. Available at: https://www.jstor.org/stable/27836590
Lovelock, J. (2007). The revenge of Gaia: earth's climate crisis & the fate of humanity. New York City: Basic Books.
Łuczka, W., and Kalinowski, S. (2020). Barriers to the development of organic farming: a polish case study. Agriculture 10:536. doi: 10.3390/agriculture10110536
Malek, K., Adam, J. C., Stöckle, C. O., and Peters, R. T. (2018). Climate change reduces water availability for agriculture by decreasing non-evaporative irrigation losses. J. Hydrol. 561, 444–460. doi: 10.1016/j.jhydrol.2017.11.046
Malhi, G. S., Kaur, M., and Kaushik, P. (2021). Impact of climate change on agriculture and its mitigation strategies: a review. Sustainability 13:1318. doi: 10.3390/su13031318
Marchesi, J. R., and Ravel, J. (2015). The vocabulary of microbiome research: a proposal. Microbiome 3:31. doi: 10.1186/s40168-015-0094-5
Margulis, L. (1991). “Symbiosis as a source of evolutionary innovation: speciation and morphogenesis” in Symbiogenesis and symbionticism. eds. L. Margulis and R. Fester (Cambridge, MA: MIT Press), 1–14.
Martínez-Viveros, O., Jorquera, M. A., Crowley, D. E., Gajardo, G., and Mora, M. L. (2010). Mechanisms and practical considerations involved in plant growth promotion by rhizobacteria. J. Soil Sci. Plant Nutr. 10, 293–319. doi: 10.4067/S0718-95162010000100006
Mayer, G., Kalt, L., Kaufmann, E., Röös, A., Muller, R., Weisshaidinger, A., et al. (2022). Impacts of scaling up agroecology on the sustainability of European agriculture in 2050. EuroChoices 21, 27–36. doi: 10.1111/1746-692X.12373
Meemken, E.-M., and Qaim, M. (2018). Organic agriculture, food security, and the environment. Annu. Rev. Resour. Econ. 10, 39–63. doi: 10.1146/annurev-resource-100517-023252
Mesa-Marín, J., Mateos-Naranjo, E., Rodríguez-Llorente, I. D., Pajuelo, E., and Redondo-Gómez, S. (2019). “Synergic effects of Rhizobacteria: increasing use of halophytes in a changing world” in Halophytes and climate change: adaptive mechanisms and potential uses, vol. 1. Eds. H. Mirza, S. Sergey and F. Masayuki (Wallingford UK: CABI), 240–254.
Mesny, F., Hacquard, S., and Thomma, B. P. (2023). Co-evolution within the plant holobiont drives host performance. EMBO Rep. 24:e57455. doi: 10.15252/embr.202357455
Mhlongo, M. I., Piater, L. A., Madala, N. E., Labuschagne, N., and Dubery, I. A. (2018). The chemistry of plant-microbe interactions in the rhizosphere and the potential for metabolomics to reveal signaling related to defense priming and induced systemic resistance. Front. Plant Sci. 9:112. doi: 10.3389/fpls.2018.00112
Migliorini, P., and Wezel, A. (2017). Converging and diverging principles and practices of organic agriculture regulations and agroecology. A review. Agron. Sustain. Dev. 37:63. doi: 10.1007/s13593-017-0472-4
Mills-Novoa, M., Pszczólkowski, P., and Meza, F. (2016). The impact of climate change on the viticultural suitability of Maipo Valley, Chile. Prof. Geogr. 68, 561–573. doi: 10.1080/00330124.2015.1124788
Mira de Orduna, R. (2010). Climate change associated effects on wine quality and production. Food Res. Int. 43, 1844–1855. doi: 10.1016/j.foodres.2010.05.001
Mishra, A., Singh, L., and Singh, D. (2023). Unboxing the black box—one step forward to understand the soil microbiome: a systematic review. Microb. Ecol. 85, 669–683. doi: 10.1007/s00248-022-01962-5
Molefe, R. R., Amoo, A. E., and Babalola, O. (2023). Communication between plant roots and the soil microbiome; involvement in plant growth and development. Symbiosis 90, 231–239. doi: 10.1007/s13199-023-00941-9
Morales-Castilla, I., García de Cortázar-Atauri, I., Cook, B. I., Lacombe, T., Parker, A., van Leeuwen, C., et al. (2020). Diversity buffers winegrowing regions from climate change losses. Proc. Natl. Acad. Sci. USA 117:2864–2869. doi: 10.1073/pnas.1906731117
Mosedale, J. R., Abernethy, K. E., Smart, R. E., Wilson, R. J., and Maclean, I. M. (2016). Climate change impacts and adaptive strategies: lessons from the grapevine. Glob. Chang. Biol. 22, 3814–3828. doi: 10.1111/gcb.13406
Moutinho-Pereira, J. M., Correia, C. M., Gonçalves, B. M., Bacelar, E. A., and Torres-Pereira, J. M. (2004). Leaf gas exchange and water relations of grapevines grown in three different conditions. Photosynthetica 42, 81–86. doi: 10.1023/B:PHOT.0000040573.09614.1d
Nicol, P. (2020). Pathways to scaling agroecology in the City region: scaling out, scaling up and scaling deep through community-led. Sustain. For. 12:7842. doi: 10.3390/su12197842
Notununu, I., Moleleki, L., Roopnarain, A., and Adeleke, R. (2022). Effects of plant growth-promoting Rhizobacteria on the molecular responses of maize under drought and heat stresses: A review. Pedosphere 32, 90–106. doi: 10.1016/S1002-0160(21)60051-6
Oksanen, J., Simpson, G. L., Blanchet, F. G., Kindt, R., Legendre, P., Minchin, P. R., et al. (2020). Vegan: community ecology package. R package Version 2.4-3. Available at: https://CRAN.R-project.org/package=vegan (Accessed August 23, 2023).
Ollat, N., van Leeuwen, C., Garcia de Cortazar, I., and Touzard, J.-M. (2017). The challenging issue of climate change for sustainable grape and wine production. OENO One. 51, 59–60. doi: 10.20870/oeno-one.2017.51.2.1872
Pane, C., Celano, G., Villecco, D., and Zaccardelli, M. (2012). Control of Botrytis cinerea, Alternaria alternata and Pyrenochaeta lycopersici on tomato with whey compost-tea applications. Crop Prot. 38, 80–86. doi: 10.1016/j.cropro.2012.03.012
Pascale, A., Proietti, S., Pantelides, I. S., and Stringlis, I. A. (2020). Modulation of the root microbiome by plant molecules: the basis for targeted disease suppression and plant growth promotion. Front. Plant Sci. 10:1741. doi: 10.3389/fpls.2019.01741
Pekdemir, C. (2018). On the regulatory potential of regional organic standards: towards harmonization, equivalence, and trade? Glob. Environ. Chang. 50, 289–302. doi: 10.1016/j.gloenvcha.2018.04.010
Petit, S., Muneret, L., Carbonne, B., Hannachi, M., Ricci, B., Rusch, A., et al. (2020). “Chapter one – landscape-scale expansion of agroecology to enhance natural pest control: a systematic review” in Advances in ecological research. eds. D. A. Bohan and A. J. Vanbergen, vol. 63 (Cambridge, MA: Academic Press), 1–48.
Qiao, S. A., Gao, Z., and Roth, R. (2023). A perspective on cross-kingdom RNA interference in mutualistic symbioses. New Phytol. 240, 68–79. doi: 10.1111/nph.19122
R Core Team . (2021). R: A language and environment for statistical computing. R Foundation for Statistical Computing, Austria. Available at: https://www.R-project.org/ (Accessed August 23, 2023).
Redondo-Gómez, S., García-López, J. V., Mesa-Marín, J., Pajuelo, E., Rodriguez-Llorente, I. D., and Mateos-Naranjo, E. (2022). Synergistic effect of plant-growth-promoting Rhizobacteria ImprovesStrawberry growth and flowering with soil salinization and increased atmospheric CO2 levels and temperature conditions. Agronomy 12:2082. doi: 10.3390/agronomy12092082
Reganold, J., and Wachter, J. (2016). Organic agriculture in the twenty-first century. Nature Plants 2:15221. doi: 10.1038/nplants.2015.2
Reynolds, H. L., Packer, A., Bever, J. D., and Clay, K. (2003). Grassroots ecology: plant–microbe–soil interactions as drivers of plant community structure and dynamics. Ecology 84, 2281–2291. doi: 10.1890/02-0298C
Roco, L., Bravo-Ureta, B., Engler, A., and Jara-Rojas, R. (2017). The impact of climatic change adaptation on agricultural productivity in Central Chile: a stochastic production frontier approach. Sustain. For. 9:1648. doi: 10.3390/su9091648
Roco, L., Engler, A., Bravo-Ureta, B., and Jara-Rojas, R. (2014). Farm level adaptation decisions to face climatic change and variability: evidence from Central Chile. Environ. Sci. Pol. 44, 86–96. doi: 10.1016/j.envsci.2014.07.008
Roughgarden, J. (2020). Holobiont evolution: mathematical model with vertical vs. horizontal microbiome transmission. Philosophy, Theory, and Practice in Biology. 12.
Roughgarden, J. (2023). Holobiont evolution: population theory for the hologenome. The American Naturalist. 201. doi: 10.1086/723782
Šajn, N. (2023). The EU wine sector. European Parliament Briefing. Available at: https://www.europarl.europa.eu/RegData/etudes/BRIE/2023/751399/EPRS_BRI(2023)751399_EN.pdf (Accessed January 22, 2024).
Sandrini, M., Nerva, L., Sillo, F., Balestrini, R., Chitarra, W., and Zampieri, E. (2022). Abiotic stress and belowground microbiome: the potential of omics approaches. Int. J. Mol. Sci. 23:1091. doi: 10.3390/ijms23031091
Santillán, D., Iglesias, A., La Jeunesse, I., Garrote, L., and Sotes, V. (2019). Vineyards in transition: a global assessment of the adaptation needs of grape producing regions under climate change. Sci. Total Environ. 657, 839–852. doi: 10.1016/j.scitotenv.2018.12.079
Santos, J. A., Fraga, H., Malheiro, A. C., Moutinho-Pereira, J., Dinis, L.-T., Correia, C., et al. (2020). A review of the potential climate change impacts and adaptation options for European viticulture. Appl. Sci. 10:3092. doi: 10.3390/app10093092
Schütz, L., Gattinger, A., Meier, M., Müller, A., Boller, T., Mäder, P., et al. (2018). Improving crop yield and nutrient use efficiency via biofertilization—a global meta-analysis. Front. Plant Sci. 8. doi: 10.3389/fpls.2017.02204
Shen, X., Liu, B., and Lu, X. (2018). Weak cooling of cold extremes versus continued warming of hot extremes in China during the recent global surface warming hiatus. J. Geophys. Res. Atmos. 123, 4073–4087. doi: 10.1002/2017JD027819
Singh, A., Kumar, M., Verma, S., Choudhary, P., and Chakdar, H. P. (2020). “Plant microbiome: trends and prospects for sustainable agriculture” in Plant microbe symbiosis. eds. A. Varma, S. Tripathi, and R. Prasad (Cham: Springer), 129–151.
Singh, J. S., Pandey, V. C., and Singh, D. P. (2011). Efficient soil microorganisms: A new dimension for sustainable agriculture and environmental development. Agric. Ecosyst. Environ. 140, 339–353. doi: 10.1016/j.agee.2011.01.017
St. Martin, C. C. G. (2014). Potential of compost tea for suppressing plant diseases. CABI Rev. 10, 1–38. doi: 10.1079/PAVSNNR20149032
St. Martin, C. C. G., Rouse-Miller, J., Barry, G. T., and Vilpigue, P. (2020). “Compost and compost tea microbiology: the “-omics” era” in Biology of composts. Soil biology, vol. 58. eds. M. Meghvansi and A. Varma (Cham: Springer)
Storchmann, K. (2016). Introduction to the special issue devoted to wine and climate change. J. Wine Econ. 11, 1–4. doi: 10.1017/jwe.2016.11
Tao, K., Kelly, S., and Radutoiu, S. (2019). Microbial associations enabling nitrogen acquisition in plants. Curr. Opin. Microbiol. 49, 83–89. doi: 10.1016/j.mib.2019.10.005
Toro, M., and Andrade, G. (2020). “Arbuscular mycorrhizae, beneficial microorganisms for sustainable agriculture” in Life on land. Encyclopedia of the UN sustainable development goals. eds. F. W. Leal, A. Azul, L. Brandli, S. A. Lange, and T. Wall (Cham: Springer).
Trivedi, P., Leach, J. E., Tringe, S. G., Sa, T., and Singh, B. K. (2020). Plant–microbiome interactions: from community assembly to plant health. Natl. Rev. Microbiol. 18, 607–621. doi: 10.1038/s41579-020-0412-1
van Leeuwen, C., Destrac-Irvine, A., Dubernet, M., Duchêne, E., Gowdy, M., Marguerit, E., et al. (2019). An update on the impact of climate change in viticulture and potential adaptations. Agronomy 9:514. doi: 10.3390/agronomy9090514
Vicuña, S., Vargas, X., Boisier, J. P., Mendoza, P. A., Gómez, T., Vásquez, N., et al. (2021). “Impacts of climate change on water resources in Chile” in Water Resources of Chile. World Water Resources, vol 8. eds. B. Fernández and J. Gironás (Cham: Springer).
Wall, D. H. C., and Moore, J. (1999). Interactions underground: soil biodiversity, mutualism, and ecosystem processes. Bioscience 49, 109–117. doi: 10.2307/1313536
Whipps, J., Lewis, K., and Cooke, R. (1988). “Mycoparasitism and plant disease control” in Fungi in biological control systems. ed. M. Burge (Manchester: Manchester University Press), 161–187.
White, C. (2020). Why regenerative agriculture? Am. J. Econ. Sociol. 79, 79, 799–812. doi: 10.1111/ajes.12334
Witze, A. (2022). Extreme heatwaves: surprising lessons from the record warmth. Nature 608, 464–465. doi: 10.1038/d41586-022-02114-y
Wobbrock, J. O., Findlater, L., Gergle, D., and Higgins, J. J. (2011). The aligned rank transform for nonparametric factorial analyses using only Anova procedures. Proceedings of the SIGCHI Conference on Human Factors in Computing Systems. doi: 10.1145/1978942.1978963
Wolfgang, A., Tack, A. J. M., Berg, G., and Abdelfattah, A. (2023). Reciprocal influence of soil, phyllosphere, and aphid microbiomes. Environ. Microbiome 18:63. doi: 10.1186/s40793-023-00515-8 for more details
Xu, Y., Castel, T., Richard, Y., Cuccia, C., and Bois, B. (2012). Burgundy regional climate change and its potential impact on grapevines. Clim. Dyn. 39, 1613–1626. doi: 10.1007/s00382-011-1284-x
Keywords: regenerative agriculture, ecological soil management, large-scale agriculture, plant microbiome, efficient microorganisms
Citation: Pino C and Griffon D (2024) Scaling up: microbiome manipulation for climate change adaptation in large organic vineyards. Front. Sustain. Food Syst. 8:1285981. doi: 10.3389/fsufs.2024.1285981
Edited by:
Pankaj Kumar Arora, M. J. P. Rohilkhand University, IndiaReviewed by:
Xiangjin Shen, Chinese Academy of Sciences (CAS), ChinaNilhari Neupane, International Water Management Institute, Nepal
Copyright © 2024 Pino and Griffon. This is an open-access article distributed under the terms of the Creative Commons Attribution License (CC BY). The use, distribution or reproduction in other forums is permitted, provided the original author(s) and the copyright owner(s) are credited and that the original publication in this journal is cited, in accordance with accepted academic practice. No use, distribution or reproduction is permitted which does not comply with these terms.
*Correspondence: Diego Griffon, Z3JpZmZvbmRpZWdvQGdtYWlsLmNvbQ==