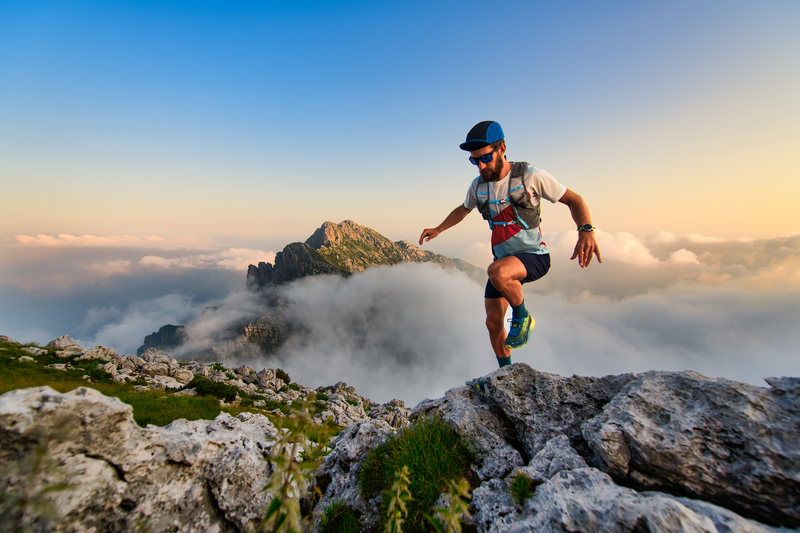
95% of researchers rate our articles as excellent or good
Learn more about the work of our research integrity team to safeguard the quality of each article we publish.
Find out more
ORIGINAL RESEARCH article
Front. Sustain. Food Syst. , 05 January 2024
Sec. Agricultural and Food Economics
Volume 7 - 2023 | https://doi.org/10.3389/fsufs.2023.1296922
Introduction: Rice production is the core component of the food security strategy in China, but it is also a major source of methane and nitrous oxide. Promoting low-carbon rice farming (LCRF) to increase the carbon sink, decrease carbon emissions, and achieve low-carbon, high-yield production is an inevitable “win-win” choice for achieving “double carbon” targets and guaranteeing national food security. This study contributes to the advancement of research on farmers’ adoption decisions and fills gaps in LCRF’s technical research on farmers’ decision-making behavior. The results also provide a basis for formulating policies to encourage LCRF and protect cultivated land.
Methods: This study conducted field research on 2,173 farming households in Jiangxi Province, a traditional agricultural province in China, and examined the effects of personal forces, local forces, and cultural forces on LCRF adoption behavior by introducing the distributed cognition theoretical framework based on a status quo analysis and employing the multivariate ordinal logistic model.
Results and discussion: The results of the study showed that: 1) the overall acceptance of LCRF behavior is currently not very high. In the 2173 questionnaires, the mean number of LCRF behavior items accepted by farmers was 3.10 items; 153 farmers did not adopt any LCRF behavior, and only 77 farmers adopted all LCRF behaviors. Most farmers (n = 535) adopted three LCRF behaviors. 2) In distributed cognition affecting LCRF behavior, acceptance was primarily affected by cultural forces, followed by local forces, while the effects of personal forces were limited. Therefore, it is recommended that training and promotion should be increased, policy subsidies should be increased, the land market should be improved, and LCRF demonstrations should be carried out to increase the acceptance of LCRF behavior among rice farmers.
In order to achieve a balance between anthropogenic emissions by sources and removals by sinks of greenhouse gases in the second half of this century as stated in the Paris Agreement, increasing numbers of countries have formulated strategies and have taken action (Klein et al., 2017; Millot and Maïzi, 2021). In September 2020, China announced that it aimed to achieve peak carbon dioxide emissions before 2030 and achieve carbon neutrality before 2060 (hereafter referred to as the “double carbon policy”) (Li et al., 2022; Xinfa and Xue, 2022). Carbon emissions in China accounted for 30% of the global total (Zheng et al., 2020). The proposal of China’s double carbon policy is an inevitable option and long-term strategy for solving resource environmental limitations and achieving sustainable development of the human society, and it is also a national responsibility and important measure for constructing a community of common destiny in China (He et al., 2022; Zhao et al., 2022). Agriculture is the second largest source of carbon emissions globally after industry. During production, greenhouse gases such as carbon dioxide (CO2), methane (CH4), and nitrous oxide (N2O) are produced, accounting for a large proportion of global emissions. Therefore, agriculture is a field that cannot be ignored in achieving “double carbon” goals. The carbon emissions generated by agriculture in China account for 17% of the total carbon emissions in China (Dong et al., 2008; Xu and Lin, 2017; Shan et al., 2018; Zhong et al., 2022), of which carbon emissions from rice cultivation account for 16% of the carbon dioxide emissions, and rice production is also an important source of methane and nitrous oxide. Methane is primarily derived from emissions from rice field soils, with a temperature-increasing effect 25 times that of carbon dioxide in the air, and nitrogen oxide is primarily derived from agricultural production activities, with a temperature-increasing effect 298 times that of carbon dioxide in the air (Yan et al., 2015; Huang et al., 2019). Since the year 2021, the Chinese Government has released several key policy documents, namely the Action Plan for Carbon Dioxide Peaking Before 2030, the Synergizing the Reduction of Pollution and Carbon Emissions Implementation Plan, and the Agricultural and Rural Carbon Emission Reduction and Sequestration Implementation Plan. These documents collectively underscore the imperative to expedite the realization of low-carbon green transformation in the agricultural sector, as well as to facilitate carbon sequestration and emission reduction in agricultural production. Therefore, it is urgent to reduce carbon emissions from rice cultivation.
Rice is an integral part of the national food security strategy. In 2020, the rice cultivation area in China was 30.07 million hm2, accounting for 25.76% of China’s food crop cultivation area. The total annual yield of rice was 211.86 million tonnes, accounting for 31.64% of the total food yield in China. Achieving “double carbon” goals, effective protection of cultivated land, safeguarding the rice cultivation area, and ensuring food security are practical issues that must be solved. Low-carbon rice farming (LCRF) is the umbrella term for rice carbon sink increasing, carbon emission decreasing, and low carbon, high-yield rice farming. The application of LCRF techniques to achieve high rice production efficiency, high yield, low carbon emissions, a high carbon sink, reduce pollution, and protect cultivated land are known as LCRF behaviors (Cao and Li, 2014; Chen et al., 2021). Similar to ecological farming and green production, these production behaviors can decrease damage to the environment and promote the sustainable development of the agriculture industry (Sá et al., 2017; Schoonhoven and Runhaar, 2018; Tan et al., 2022; Zhang et al., 2022a). As a branch of general low carbon farming, the major characteristics of LCRF are construction of a rice field farming system “that prioritizes increasing carbon sinks, decreases consumption, and focuses on reducing carbon emission and recycling” based on the principle of rice field ecosystem carbon cycling to promote low carbon and high yield rice production (Cao and Li, 2014; Chen et al., 2021). Therefore, LCRF is a “win-win” option for achieving “double carbon” goals and food security, and it engenders an intrinsic need for modern rice industry transformation (Chen et al., 2021; Wei et al., 2022).
Existing study findings primarily examine the effects of carbon emissions and carbon neutrality in rice fields from various types of rice cropping patterns, as well as the impact on rice yield. Although academics have undertaken thorough research on LCRF at the technical level, no scholars have yet examined the current state and influencing factors of LCRF adoption behavior from the farmers’ perspective. Moreover, a farmer household responsibility system is implemented in China’s agriculture industry. Even though various types of modern agriculture business entities have continuously emerged, small farmers are still the main business entity in China’s agriculture industry for the foreseeable future. Therefore, fully mobilizing farmers to carry out LCRF is an objective requirement for achieving “double carbon” goals and food security. Fully understanding the LCRF behavior patterns of farmers and their influencing factors are an important basis for formulating relevant policies to encourage farmers to adopt LCRF behavior. Farmers directly participate in rice cultivation, and decision acceptance is a complex cognitive process. Customers will weigh the pros and cons based on their cognition (Homburg and Stolberg, 2006; Yu et al., 2020).Cognitive psychologists believe that an individual’s cognition can determine their decisions and behaviors. Therefore, any form of cognitive behavior in actual production can change the cultivation decisions of farmers. The distributed cognition theory emphasizes that an individual’s cognition is affected by the interactions between individuals, the social environment, and their culture. This shows that the farmer’s adoption of certain behaviors is not caused by a single factor but rather is the outcome of multiple factors. Therefore, the use of distributed cognition theory to study the behavior of farmers is more in line with current logic (Cole and Engeström, 1993; Salomon, 1997; Shahangian et al., 2021).
The key issues to be addressed in this study are exploring the current situation of farmers’ adoption of LCRF based on a preliminary analysis of the intrinsic mechanism of LCRF, using Jiangxi Province as the study area, and analyzing the influence of each cognitive force level on farmers’ decision-making behavior through the lens of distributed cognitive theory. This study is expected to contribute to existing research in the following ways: (1) analyze the current situation of LCRF adoption by farmers from a micro perspective, and fill a research gap in the field of LCRF research on farmers’ decision-making behavior. (2) Using the Distributed Cognition Theory framework, examine how to encourage farmer adoption of LCRF with the aim of providing practical guidance for the formulation of relevant policies to encourage farmers. The study’s findings may also provide policy recommendations for China and other developing countries.
The remainder of this paper is structured as follows: The second section is titled “Theoretical Analysis,” and it is based on the preliminary analysis of the intrinsic mechanism of LCRF that the distributed cognition theory is introduced to analyze the influencing mechanism of farmer adoption behavior, and then the research hypothesis is proposed. “Materials and methods” is the third section, which introduces the selected study area, data sources, study variables, and methods. The empirical results are presented in the fourth section. The final section concludes with policy recommendations.
In contrast to the conventional model, which relies on heavy inputs and the irrational application of chemical fertilizers and pesticides to ensure rice production, LCRF is a high-yield, low-emission, and high-efficiency rice crop model (Neue, 1993; Guo and Zhou, 2007; Cao and Li, 2014; Chen et al., 2021). The majority of farmers in China employ the conventional approach of rice farming, which entails the utilization of traditional seeding and irrigation techniques, the selection of traditional rice varieties, and the application of conventional fertilizers and pesticides, et al. This approach is characterized by high labor and agricultural material costs. Simultaneously, its high carbon emissions and excessive pollution exert significant strain on the environment, which is not beneficial to sustainable agriculture development (Poulton et al., 2016; Liang et al., 2021; Gangopadhyay et al., 2022; Wu et al., 2022). Current academic research on LCRF technology focuses primarily on enhancing soil organic carbon accumulation (Xia et al., 2020; Wei et al., 2021; Tang et al., 2022; Zhang et al., 2022b), enhancing agricultural material utilization efficiency (Memon et al., 2018; Yadav et al., 2020; Zhang et al., 2020a, 2020b), and promoting carbon biocycling (Dai et al., 2022; Sultana et al., 2022; Gao et al., 2023). Based on a theoretical study of LCRF technology, this research lists the seven typical categories of LCRF behaviors based on regional rice farming features and empirical evidence. These typical LCRF can achieve low-carbon rice production by reducing agricultural consumption, reducing carbon emissions, promoting resource recycling, and increasing carbon sinks, such as soil formula fertilization, application of organic fertilizer, rational crop rotation, straw return to the field, agricultural film recycling, conservation tillage techniques, the eco-agriculture model, and other rice farming techniques (Figure 1).
Soil formula fertilization can develop fertilizer ratios based on the nutrients required for rice cultivation, control the amount of nitrogen applied to fertilizers, and reduce N2O emissions, thereby enhancing the quality of cultivated land and contributing to a high yield and reduction in emissions (Zhang et al., 2014, 2016; Tao et al., 2019; Li and Ju, 2020). The application of organic fertilizer can significantly increase the soil’s exogenous organic matter and enhance arable land’s productivity. Simultaneously, it can inhibit nitrification and mineralization of organic nitrogen in the soil, reduce soil N2O emissions, and contribute to soil carbon sequestration and emission reduction (Maillard and Angers, 2014; Jiang et al., 2018; Lin et al., 2019; Tang et al., 2022). Rational crop rotation can regulate soil structure and reduce the impact of rice cultivation on the soil carbon pool; it can also regulate soil fertility and enhance the quality of cultivated land, thereby increasing food production (Cha-un et al., 2017; Sun et al., 2019; Liu et al., 2021b; Yang et al., 2022). Straw is a renewable resource with multiple uses and a significant carbon sink for rice. Scientific straw return is beneficial to lowering the use of synthetic fertilizers, boosting soil fertility in rice ecosystems, enhancing soil organic carbon content, increasing arable land quality, and raising rice yields (Liu et al., 2014; Chen et al., 2017; Zhang et al., 2021). As a significant component of agricultural production material, agricultural film indirectly produces carbon emissions. By recycling agricultural films, we may cut greenhouse gas emissions and prevent soil pollution caused by film leftovers. Conservation tillage techniques can reduce CH4 emissions, minimize the integrated greenhouse effect of rice fields, and increase soil carbon fixation. It can also improve soil physical and chemical properties, reduce soil erosion and organic matter consumption in arable land, promote nutrient cycling, and help increase rice yields (Lal, 2015; Li et al., 2016; Kader et al., 2022; Xu, 2022). The eco-agriculture model enhances the economic, ecological, and social benefits of rice farming via a sustainable cycle involving rice-ducks, rice-fish, rice-crabs, and other breeding types (Zheng et al., 2019). In this model of compound farming, CH4 oxidation can be facilitated by animal activities, thereby reducing CH4 emissions, enhancing soil quality, and ensuring stable rice production (Ying et al., 2014; Nayak et al., 2015; Wan et al., 2019; Sun et al., 2021).
Distributed cognition refers to the processing of internal and external information (Moore and Rocklin, 1998; Herrero and Brown, 2010). Distributed cognition is an emerging perspective in theoretical cognitive science research and is an analytical theory that considers all factors participating in cognitive activity (Salomon, 1997; Liu et al., 2021a). This theory breaks away from the limitations of traditional cognitive concepts that focus on the individual and affirms the role of individual cognition. However, it emphasizes the interactions between culture, region, and society that are beyond specific scenarios during the cognitive process (Hatch and Gardner, 1993; Boland et al., 1994). There are many types of theoretical distributed cognition studies. In 1993, Hatch and Gardner used this cognitive activity theory to propose a concentric circle model of distributed cognition. In this model, there are three concentric circles. The outermost circle represents cultural forces and includes routines, activities, and evaluation; this level is beyond specific scenarios, and it indirectly affects individual cognitive activities. The middle circle represents local forces and includes resource endowment and material constraints and emphasizes the resources or individual in the local scenario. The innermost circle comprises personal forces and represents the characteristics, experiences, and inclination of an individual in a local scenario (Hatch and Gardner, 1993; Tan and Mei, 2018; Lai et al., 2019). The three cognitive layers are indispensable and rely on each other. A farmer’s cognition is affected by internal and external factors, and cognition “distribution” shows that various factors can play a role in decisions. Therefore, these factors can fully explain the complex cognitive activities of farmers (Tan and Mei, 2018). The differences in the distribution of cognitive activities also determine the differences in LCRF behavior decisions of farmers. These differences include individual, family, social, and cultural aspects such as gender and education level. This study incorporates the theory of distributed cognition and subsequently formulates the following research hypotheses (Figure 2).
Figure 2. Analytical framework of farmers’ LCRF adoption behavior based on distributed cognition theory.
H1: The adoption of LCRF behaviors by farmers can be enhanced through personal forces. The centrality of personal forces in distributed cognitive theory may determine whether individual farmers are capable of adopting LCRF during their adoption decision-making process. The influence of this force on farmers’ attitudes and behaviors toward LCRF is typically contingent upon the farmer’s educational attainment, employment status, and various other factors (Li and Li, 2023).
H2: The adoption of LCRF behaviors by farmers can be enhanced through local forces. Local forces are observed as distinct constraints on resources within a specific situation and scenario. These forces are notably apparent in the production and living conditions affecting individual farmers during the adoption decision-making process (Shi and Zhang, 2022). Therefore, farmers’ adoption of LCRF behaviors may be somewhat restricted by more restrictive local forces.
H3: The adoption of LCRF behaviors by farmers can be enhanced through cultural forces. The cultural forces hierarchy is located at the outermost level, where external factors such as activity, evaluation, and satisfaction are abstract and objective. These forces are commonly observed in the form of proactive actions and subjective experiences exhibited by farmers, among other factors (Rong and Hou, 2022). Farmers who possess a greater degree of cultural forces are more likely to exhibit a propensity toward adopting LCRF, primarily due to their rational decision-making processes.
Jiangxi Province is located at the southern bank of the lower and middle reaches of the Yangtze River, from latitudes 24°29′14″ N to 30°04′43″ N and longitudes of 113°34′18″ E to 118°28′56″. Jiangxi is an important province in southeastern China (Figure 3). Jiangxi Province is characterized by a subtropical monsoon climate, characterized by an average annual precipitation ranging from 1,600 to 1700 mm. The region experiences a warm climate with ample sunlight and abundant rainfall, resulting in a prolonged frost-free period. These favorable agro-climatic conditions create an advantageous environment for the cultivation and growth of crops. Jiangxi Province occupies a significant role as a conventional agricultural province. It is recognized as one of the thirteen principal grain-producing regions in the country and additionally serves as a significant double-season rice production zone. In 2020, the rice cultivation area in Jiangxi Province was 34.42 million hm2, accounting for 91.24% of the food crop cultivation area in Jiangxi Province. The total annual rice yield was 20.512 million tonnes, accounting for 94.79% of the total food yield in Jiangxi Province. Moreover, Jiangxi Province contributes significantly to agricultural greenhouse gas emissions. Specifically, the total carbon emissions resulting from rice production in Jiangxi constitute approximately 22.36% of the total emissions observed in the middle and lower reaches of the Yangtze River. This proportion surpasses other provinces in the surrounding provinces, including Hunan, Anhui, Hubei, and Jiangsu, among others (Chen et al., 2021). Therefore, the sampling of farmers in Jiangxi Province can better reflect the actual situation of LCRF behavior acceptance by farmers in China. The policy implications are also applicable to other rice-producing areas in China.
In order to ensure the validity of data collected using the survey questionnaires, the questionnaire design underwent repeated rounds of research, and experts were consulted. Finally, the main content of the questionnaire was determined. The questionnaire consists of three primary parts. The first part of the questionnaire includes basic information associated with the farmers, encompassing the individual characteristics such as gender, age, and educational attainment of the participants. Additionally, household particulars such as the overall population and annual income of the respondents are included. Furthermore, farming-related information, such as the contracted arable land area, actual farming area, and utilization of farming equipment by the farmers, is also incorporated. The second part is farmers’ perceptions and adoption of LCRF technology, including respondents’ cognition, willingness to adopt, and adoption behaviors or not. Due to the dispersed nature of respondents’ behavior in adopting a particular technology, this part specifically asks questions about cognition and adoption of each technology based on the supplemental explanation of the relevant concepts. The third part is about farmers’ perspectives on LCRF technology, including an assessment of farmers’ ability to access LCRF technology information and an assessment of efforts to publicize LCRF technology.
The survey was conducted in Jiangxi Province from January to March 2022. Farmers were selected by using stratified sampling for the survey. Stratification was carried out based on topography, economic development level, and proportion of the agriculture industry. Eight counties (cities, districts) were selected for each type, and 2–3 towns were randomly selected from the counties (cities, districts). One or two administrative villages were randomly selected from the towns, and 10–15 rice farmer households were randomly selected from each village. A total of 2,314 questionnaires were obtained through interviews and door-to-door visits. After removing repeated, blank, and contradictory questionnaires, there were 2,173 valid questionnaires for a validity rate of 93.91%.
The explained variables were set with reference to existing studies setting multiple categories of adoption behaviors (Zhang et al., 2022a). Conventional statistical study methods normally use dichotomous variables, i.e., the explained variables are separated into farmers’ adoption and non-adoption of LCRF behaviors. In reality, there are numerous sorts of LCRF behaviors employed by farmers, and classification into two broad categories is not consistent with reality. The adoption behavior is quantified for the purpose of this study by assigning a score to each adoption situation, with 1 point for adoption and 0 points for non-adoption, and then summing the scores of farmers to determine their LCRF behavior. The higher the score, the greater the level of adoption among farmers. After summing the adoption variables, the explained variables were divided into eight categories.
Core explanatory variables are factors that primarily affect farmer acceptance level. First, the aforementioned theory was used for marker selection, and the actual survey status of the study site was fully considered. Based on the three circles in the concentric circle model, personal forces, local forces, and cultural forces were used to set four markers each. Finally, 12 core explanatory variables were confirmed. The personal forces level included gender, age, education level, and employment; local forces included total family size, proportion of agriculture income, whether the subject’s family was a major household of the village, and whether large farming equipment was used for cultivation; cultural forces included cultivated land quality evaluation by the farmer, evaluation of LCRF advocacy, join the cooperative, and whether cultivated land was transferred out (Table 1).
In this study, the explained variables were divided into eight categories through accumulation. Each category represents the number of LCRF behavior items adopted by the farmer; the categories have significant sequential relationships and are multivariate ordinal variables. Conventional linear regression models commonly employ binary selection models and multiple selection models. However, it is important to note that these regression analyses may not accurately reflect causal relationships between the variables under study. Multivariate ordinal logistic modeling is an analytical method that can handle the type of multicategorical ordinal variable problems encountered in this study. Finally, a multivariate ordinal logistic model was used to explain the response relationships of explanatory variables toward explained variables (Freedman, 2009). The multivariate ordinal logistic model is presented as:
where P represents the probability of the explained variable Y = j, i.e., the probability of increase or decrease in the number of LCRF behaviors adopted by the farmer. When Y = 0, the farmer did not adopt any LCRF behavior; when Y = 1, the farmer adopted 1 LCRF behavior; and when Y = 7, the farmer adopted 7 LCRF behaviors. Xn is the influencing factor that affects LCRF behavior acceptance by the farmer; εj represents random error; β0 is the constant of the equation, and βn represents the coefficient to be estimated, i.e., the partial regression coefficient of logistic regression, and shows the effects of Xn on Logit(P).The Odds Ratio (OR) for the dominance ratio is determined by applying the aforementioned equation, which incorporates the intercept. The resulting OR quantifies the extent to which the risk of the outcome variable changes with each unit alteration in the independent variable.
A preliminary sociodemographic analysis of respondents was done in this study. In the 2173 samples, there were 1629 males, accounting for 74.97% of the total sample. The mean age of the farmers was 50.93 years, of which 40–60 year old farmers accounted for 68.8% of the total sample. The education level of 84.58% of farmers was junior high school and below, and education level was generally low. With regards to employment, full-time farmers or subjects whose main operation was farming accounted for most of the subjects (59.3%). The proportion of migrant farmers was the lowest, accounting for 14.1%. With regard to family status, the mean family size of the surveyed farmers was 5.55. The proportion of families with total size of 4–7 people was the highest (80.1%). The proportion of families with total size ≤3 was 8.6%. The mean annual total income was 83,100 RMB and farmers with total annual household income <60,000 RMB accounted for 52.65% of the total sample. This means that medium income families made up most of the study subjects, but there were also families that were relatively poor (Table 2).
Among the 2,173 valid questionnaires, with regard to the acceptance of LCRF behavior by farmers, the mean number of LCRF behavior items adopted was 3.10. A total of 153 farmers did not adopt any LCRF behavior, for a marginal percentage of 7.04%. Only 77 farmers adopted all LCRF behaviors, for a marginal percentage of 3.54%. Most farmers (n = 535) adopted three LCRF behaviors for a marginal percentage of 24.62%. The cumulative percentage of farmers who adopted three or fewer items was 60.06%. This shows that more than half of the farmers adopted three or fewer items, and the overall acceptance was not high (Figure 4).
Further analysis of different LCRF behaviors adopted by farmers found that the differences in acceptance were large (Figure 5). (1) There were 1,522 and 1,445 farmers who adopted the application of organic fertilizers and straw returning, respectively. These had the highest ratios amongst the seven types of LCRF behaviors, being 70.04 and 66.50%, respectively. This may be because organic fertilizer and straw are natural fertilizers that are easily obtained. In addition, the Chinese government has restrictions on straw burning. Hence, farmers usually do not waste these two types of natural resources. Therefore, the proportion of farmers who carried out straw returning and application of organic fertilizers was high. (2) There were 1,213 and 909 farmers who adopted rational crop rotation and agricultural film recycling, respectively. This may be because farmers are unsure about the benefits of crop rotation. In addition, most rice cultivation business entities are small farms, and it is difficult to implement agricultural film recycling for scattered farms. Therefore, the proportion of subjects who adopted rational crop rotation and agricultural film recycling was not high. (3) There were 724, 484, and 441 farmers who adopted conservation tillage techniques, soil formula fertilization, and eco-agriculture, respectively, accounting for less of the total sample. The percentages were 33.32, 22.27, and 20.29%, respectively. This was due to the local complexity in conservation tillage techniques at the technical level, as suitable local technical models must be selected. In addition, the farmer needs to purchase the proper equipment. Therefore, the farmers’ willingness to employ conservation tillage techniques was low. Secondly, soil formula fertilization may be affected by insufficient promotion, low awareness, and high technical requirements. This resulted in low farmer acceptance. The proportion of farmers who adopted eco-agriculture was the lowest. This was because most farmers in China have small and scattered rice cultivation fields, and establishing eco-agriculture requires high initial costs, and short-term returns are low. The rational choices of farmers caused the number of farmers who adopted eco-agriculture to be low.
The researchers employed SPSS 24 software (IBM Corp., Armonk, NY, USA) for the purposes of conducting model diagnostic testing and estimation. Before the model was used, multicollinearity between variables needed to be ruled out. In theory, multicollinearity will prevent the estimation of regression coefficients. Therefore, the presence of multicollinearity between the explanatory variables, X1, X2, …, Xn must be determined before deciding whether the multivariate ordinal logistic model can be used for regression. Under normal circumstances, the variance inflation factor (VIF) method and tolerance method are used for analysis and diagnosis. The VIF is a measure that quantifies the extent of multicollinearity between explanatory variables. It is calculated as the ratio of the variance in a model with multicollinearity to the variance in a model without multicollinearity. Conversely, the tolerance is the reciprocal of the variance inflation factor. Usually, multicollinearity is considered to be absent between explanatory variables when tolerance >0.1, and VIFi < 10. The two methods can be expressed as:
Here, VIFi is the variance inflation factor. is the linear relationship between explanatory variables. The variables of this study were included in a multicollinearity diagnosis. The results showed that minimum tolerance was 0.55 and maximum VIF was 1.802, values that satisfied the test conditions. This shows that severe collinearity was absent between explanatory variables and that adjustments to explanatory variables were not needed. At the same time, the model parallelism test results showed that p = 0.453 > 0.05, and the null hypothesis should not be rejected, i.e., there are no significant differences in positional parameters of the model in different explained variables and that the model is stable and reliable. SPSS 24 was used for fitting of the multivariate ordinal logistic model. The significance determines the correlation between the explanatory variable and the explained variable.
Based on the estimation results of the multivariate ordinal logistic regression model (Table 3), the effects of three cognitive levels on LCRF behavior in farmers were analyzed. The impact of cultural forces was the greatest in farmers, and the four markers all showed strong significance. This was followed by local forces in which three markers showed significant effects. The impact of personal forces was the lowest, as only two markers showed significant effects. Overall, the adoption of behaviors by farmers in the study site was mainly affected by activity routines, material constraints, and endowment. This showed that individual factors have limited effects on LCRF behaviors of farmers.
For personal forces, the gender and age of farmers had significant effects on adoption behavior (p < 0.05). With regard to gender, females were more likely to adopt LCRF behavior. It is generally believed that men are the main movers of agricultural labor, that they more often take the initiative in making decisions, participate more in rice cultivation activities, and have greater contact with LCRF techniques. However, the actual situation showed that LCRF can be conducive to promoting environmental protection. Women tend to exhibit higher environmental quality standards and display stronger pro-environmental intentions. Hence, more women adopted LCRF. The probability of adopting LCRF behavior decreased as farmer age increased. In fact, older farmers are affected by lower learning capacity and do not have high willingness to adopt LCRF techniques. This causes the probability of adopting LCRF behavior to decrease with age.
For local forces, total family size, whether the subject’s family was a major household of the village, and whether large farming equipment was used for cultivation significantly affected adoption of LCRF behavior by farmers. The probability of adopting LCRF behavior decreased with total family size (p < 0.01). This was because a larger family means a heavier family burden experienced by the farmer. Farmers do not adopt LCRF behavior due to family livelihood concerns and insufficient awareness of LCRF. If the farmer’s family was a major household, the probability of adopting LCRF behavior was higher (p < 0.01). In fact, in China’s rural society where blood ties form a network, if the farmer’s family is a major household, this means that he/she has richer social networks in the village and more frequent agricultural production exchanges. Farmers tend to be exposed to LCRF techniques. Therefore, such farmers tend to adopt LCRF behaviors. If the farmer had large farming equipment, the possibility of adopting LCRF behavior was higher (p < 0.1). The use of large farming equipment can increase rice cultivation efficiency, and the farmer will pay more attention to low-carbon methods. The probability of adopting LCRF behavior in cultivation is also greater.
For cultural forces, cultivated land quality evaluation (p < 0.01), join the cooperative (p < 0.01), whether cultivated land was transferred out (p < 0.01), and evaluation of LCRF advocacy (p < 0.1) were all significant. Among these factors, the greater the farmer’s evaluation of cultivated land quality, the higher the probability that the farmer will adopt LCRF behavior to obtain more stable and high yield, continuously maintain or improve cultivated land quality, and decrease carbon emissions. This forms a virtuous cycle of cultivated land protection. Cooperatives provide examples for technical learning and play a role in farming demonstrations. Therefore, farmers who joined cooperatives tended to learn LCRF techniques. At the same time, farmers who joined cooperatives tended to have more advantages and vitality than traditional business entities; they could shoulder some risk, and they had greater acceptance of new techniques. Therefore, they were more likely to adopt LCRF behavior. Farmers who transferred out cultivated land were more likely to adopt LCRF behavior. This is because the cultivated land may be transferred to family farms, cooperatives, and agricultural companies, and farmers can learn LCRF techniques from professional technical staff. Hence, such farmers tended to adopt LCRF behaviors. The higher the evaluation of LCRF advocacy by farmers, the greater the possibility of adopting LCRF behavior. The greater the advocacy, the higher the probability of encountering LCRF and the possibility of adoption is greater. This conforms to the technological diffusion theory.
This study was based on onsite survey data of 2,173 farmers in Jiangxi Province. (1) The overall acceptance of LCRF behaviors by farmers was not very high, and there were significant differences in the types of behavior adopted. Among the seven LCRF behaviors, the mean number adopted was 3.10, and 153 farmers did not adopt any LCRF behaviors. This means that the acceptance was not high in Jiangxi Province, where is with a strong agricultural base. Then there is room for the government to implement policies to promote adoption of LCRF behaviors. (2) There were significant differences in the types of LCRF behaviors adopted by farmers. The number of farmers who adopted application of organic fertilizers was the highest, followed by straw returning. The number of farmers who adopted eco-agriculture was the lowest. (3) Under the framework of distributed cognitive theory, acceptance of LCRF behaviors by farmers was affected by cultural forces and local forces, while personal forces had limited effects. At the personal force level, female farmers were more active in adopting LCRF behaviors. The learning and acceptance capacities of younger farmers were stronger, and they preferred to adopt LCRF behaviors. At the local forces level, farmers with smaller total family size, those whose family was a major household in the village, and those who possessed large farming equipment tended to adopt LCRF behaviors. At the cultural forces level, farmers who transferred cultivated land, had a higher evaluation of LCRF advocacy. Farmers who had higher evaluation of the quality of the cultivated land and joined cooperatives, tended to adopt LCRF behaviors.
Based on the above study results, we recommend that the following three measures can be used to increase acceptance of LCRF behavior in farmers:
a. Expanding training and advocacy. With regard to the current status of LCRF behavior acceptance in farmers, the government needs to promulgate policies to promote LCRF techniques, improve technical training, and strengthen knowledge popularization. The government should actively lead in technical advocacy and training. On the one hand, individuals such as agricultural technique extension staff, village cadres, and other trusted members of the rural community should engage in the dissemination of information and distribute informational brochures. On the other hand, the government can provide technical training through traditional offline and online courses, new media live broadcasts, and other institutional platforms. And it can organize periodic training for professionals in agriculture, water conservancy, land, and other fields through cooperatives, agricultural research institutes, county vocational education centers, and other institutional platforms in order to maximize the impact of technical training (Wang et al., 2021). At the same time, active efforts to change the current status of aging in rice cultivation should be made to attract and guide young people to become farmers. This will promote LCRF.
b. Increasing policy subsidies. In view of the effects of local forces markers on acceptance of LCRF behavior, governments should take full account of differential subsidies for different types of farmers who accept LCRF behavior, such as gender, age, and family size, in order to accurately meet the needs of diverse farmers (Zhao et al., 2021). Subsidies can be disbursed through both direct and indirect means. Direct subsidies involve the provision of funds or resources directly to farmers, such as subsidies for agricultural machinery purchases. Indirect subsidies encompass increased subsidies for agriculture technical staffs and the implementation of preferential policies to encourage technology adoption among farmers. These measures can lessen the financial burden experienced by farmers.
c. Improving land markets, demonstrating LCRF. Cultural forces were the cognitive level that had the greatest influence in this study, and this aspect requires more attention from the government. On the one hand, the government should actively manage the land transfer, minimize the wastage of arable land resources, enhance utilization efficiency, and further achieve large-scale operations (Lu et al., 2019). On the other hand, China’s rural society is a human sentiment society, wherein farmers maintain deeper links and engage in frequent encounters. This type of farming community facilitates the effective dissemination of technical information among farmers. On this basis, the government can actively foster agricultural socialization services and the development of new agricultural management entities, such as cooperative farmers (Yang et al., 2021). Agricultural socialization services facilitate communication and learning among farmers, enabling them to access pertinent agricultural technique guidance, which helps to reduce the cognitive differences caused by technical information constraints and, ultimately, boosts farmer behavior in adopting LCRF.
The original contributions presented in the study are included in the article/supplementary material, further inquiries can be directed to the corresponding author.
Ethical review and approval was not required for the study on human participants in accordance with the local legislation and institutional requirements. Written informed consent from the [patients/ participants OR patients/participants legal guardian/next of kin] was not required to participate in this study in accordance with the national legislation and the institutional requirements.
QH: Data curation, Software, Writing – original draft, Visualization. MC: Conceptualization, Formal Analysis, Funding acquisition, Supervision, Writing – review & editing. TZ: Methodology, Supervision, Writing – review & editing. FZ: Investigation, Resources, Validation, Writing – review & editing. JZ: Investigation, Resources, Validation, Writing – review & editing.
The author(s) declare financial support was received for the research, authorship, and/or publication of this article. This research study is financially supported by the National Natural Science Foundation of China (grant nos. 71964016, 72004084, and 72364017).
We would like to extend our heartfelt gratitude to all the members of Research Center on Rural Land Resources Use and Protection who actively contributed to the field investigation, preparation of questionnaires, and data input processes.
JZ was employed by Jiangxi Hangke Survey and Planning Co., Ltd.
The remaining authors declare that the research was conducted in the absence of any commercial or financial relationships that could be construed as a potential conflict of interest.
All claims expressed in this article are solely those of the authors and do not necessarily represent those of their affiliated organizations, or those of the publisher, the editors and the reviewers. Any product that may be evaluated in this article, or claim that may be made by its manufacturer, is not guaranteed or endorsed by the publisher.
Boland, R., Tenkasi, R., and Teeni, D. (1994). Designing information technology to support distributed cognition. Organ. Sci. 5, 456–475. doi: 10.1287/orsc.5.3.456
Cao, C. G., and Li, C. F. (2014). The theory and practice of low carbon rice farming. Beijing: Science Press.
Cha-un, N., Chidthaisong, A., Yagi, K., Sudo, S., and Towprayoon, S. (2017). Greenhouse gas emissions, soil carbon sequestration and crop yields in a rain-fed rice field with crop rotation management. Agric. Ecosyst. Environ. 237, 109–120. doi: 10.1016/j.agee.2016.12.025
Chen, S. W., Liu, T. Q., Cao, C. G., Ling, L., and Wang, B. (2021). Situation of carbon neutrality in rice production and techniques for low-carbon rice farming. J. Huazhong Agricult. Univ. 40, 3–12. doi: 10.13300/j.cnki.hnlkxb.2021.03.002
Chen, Z., Wang, H., Liu, X., Zhao, X., Lu, D., Zhou, J., et al. (2017). Changes in soil microbial community and organic carbon fractions under short-term straw return in a rice-wheat cropping system. Soil Tillage Res. 165, 121–127. doi: 10.1016/j.still.2016.07.018
Cole, M., and Engeström, Y. (1993, 1993). “A cultural-historical approach to distributed cognition” in Distributed cognitions: psychological and educational considerations. ed. G. Salomon (New York: Cambridge University Press), 1–46.
Dai, R. X., Zhao, L. F., Tang, J. J., Zhang, T. J., Guo, L., Luo, Q. Y., et al. (2022). Characteristics of carbon sequestration and methane emission in rice-fish system. Chin. J. Eco-Agric. 30, 616–629. doi: 10.12357/cjea.20210811
Dong, H., Li, Y., Tao, X., Peng, X., Li, N., Zhu, Z., et al. (2008). China greenhouse gas emissions from agricultural activities and its mitigation strategy. Transact. Chin. Soc. Agricult. Eng. 24, 269–273. doi: 10.3321/j.issn:1002-6819.2008.10.055
Freedman, D. A. (2009). Statistical models: theory and practice. 1st. New York: Cambridge University Press
Gangopadhyay, S., Banerjee, R., Batabyal, S., Das, N., Mondal, A., Pal, S. C., et al. (2022). Carbon sequestration and greenhouse gas emissions for different rice cultivation practices. Sustain. Product. Consumpt. 34, 90–104. doi: 10.1016/j.spc.2022.09.001
Gao, H., Dai, L., Xu, Q., Gao, P., and Dou, Z. (2023). Transforming agrifood systems in a win-win for health and environment: evidence from organic rice-duck coculture. J. Sci. Food Agric. 103, 968–975. doi: 10.1002/jsfa.12282
Guo, J., and Zhou, C. (2007). Greenhouse gas emissions and mitigation measures in Chinese agroecosystems. Agric. For. Meteorol. 142, 270–277. doi: 10.1016/j.agrformet.2006.03.029
Hatch, T., and Gardner, H. (1993). “Finding cognition in the classroom: an expanded view of human intelligence” in Distributed cognitions. Psychological and educational considerations. ed. G. Salomon (Cambridge: Cambridge University Press)
He, J., Li, Z., Zhang, X., Wang, H., Dong, W., Du, E., et al. (2022). Towards carbon neutrality: a study on China’s long-term low-carbon transition pathways and strategies. Env. Sci. Ecotechnol. 9:100134. doi: 10.1016/j.ese.2021.100134
Herrero, C., and Brown, M. (2010). Distributed cognition in community-based education. Rev. Psicodidact. 15, 253–268.
Homburg, A., and Stolberg, A. (2006). Explaining pro-environmental behavior with a cognitive theory of stress. J. Environ. Psychol. 26, 1–14. doi: 10.1016/j.jenvp.2006.03.003
Huang, X., Xu, X., Wang, Q., Zhang, L., Gao, X., and Chen, L. (2019). Assessment of agricultural carbon emissions and their spatiotemporal changes in China, 1997–2016. Int. J. Environ. Res. Public Health 16:3105. doi: 10.3390/ijerph16173105
Jiang, G., Zhang, W., Xu, M., Kuzyakov, Y., Zhang, X., Wang, J., et al. (2018). Manure and mineral fertilizer effects on crop yield and soil carbon sequestration: a meta-analysis and modeling across China. Glob. Biogeochem. Cycle 32, 1659–1672. doi: 10.1029/2018GB005960
Kader, M. A., Jahangir, M. M. R., Islam, M. R., Begum, R., Nasreen, S. S., Islam, M. R., et al. (2022). Long-term conservation agriculture increases nitrogen use efficiency by crops, land equivalent ratio and soil carbon stock in a subtropical rice-based cropping system. Field Crop Res. 287:108636. doi: 10.1016/j.fcr.2022.108636
Klein, D. R., Carazo Ortiz, M. P., Doelle, M., Bulmer, J., and Higham, A. eds. (2017). The Paris agreement on climate change: analysis and commentary. Oxford, United Kingdom: Oxford University Press.
Lai, Z. H., Chen, M. Q., Kuang, F. Y., Fu, C. Y., and Zhang, Y. Q. (2019). Influencing factors of farm households’willingness to ecological farming based on the distributed cognition theory. J. South. Agricult. 50, 905–912. doi: 10.3969/j.issn.2095-1191.2019.04.31
Lal, R. (2015). Sequestering carbon and increasing productivity by conservation agriculture. J. Soil Water Conserv. 70, 55A–62A. doi: 10.2489/jswc.70.3.55A
Li, S., Dong, C., Yang, L., Gao, X., Wei, W., Zhao, M., et al. (2022). Research on evolutionary game strategy selection and simulation research of carbon emission reduction of government and enterprises under the “dual carbon” goal. Sustainability 14:12647. doi: 10.3390/su141912647
Li, H., He, J., Bharucha, Z. P., Lal, R., and Pretty, J. (2016). Improving China’s food and environmental security with conservation agriculture. Int. J. Agric. Sustain. 14, 377–391. doi: 10.1080/14735903.2016.1170330
Li, Y., and Ju, X. T. (2020). Rational nitrogen application is the key to mitigate agricultural nitrous oxide emission. J. Agro Environ. Sci. 39, 842–851. doi: 10.11654/jaes.2020-0245
Li, K., and Li, Q. (2023). Towards more efficient low-carbon agricultural technology extension in China: identifying lead smallholder farmers and their behavioral determinants. Environ. Sci. Pollut. Res. 30, 27833–27845. doi: 10.1007/s11356-022-24159-2
Liang, D., Lu, X., Zhuang, M., Shi, G., Hu, C., Wang, S., et al. (2021). China’s greenhouse gas emissions for cropping systems from 1978–2016. Sci. Data 8, 171–110. doi: 10.1038/s41597-021-00960-5
Lin, Y., Ye, G., Kuzyakov, Y., Liu, D., Fan, J., and Ding, W. (2019). Long-term manure application increases soil organic matter and aggregation, and alters microbial community structure and keystone taxa. Soil Biol. Biochem. 134, 187–196. doi: 10.1016/j.soilbio.2019.03.030
Liu, T., Guo, L., Cao, C., Tan, W., and Li, C. (2021b). Long-term rice-oilseed rape rotation increases soil organic carbon by improving functional groups of soil organic matter. Agric. Ecosyst. Environ. 319:107548. doi: 10.1016/j.agee.2021.107548
Liu, C., Lu, M., Cui, J., Li, B., and Fang, C. (2014). Effects of straw carbon input on carbon dynamics in agricultural soils: a meta-analysis. Glob. Change Biol. 20, 1366–1381. doi: 10.1111/gcb.12517
Liu, H. B., Wu, M. Y., Ma, X. L., Liu, X. H., Gao, J. J., and Wu, Y. (2021a). Research on influencing factors of farmers’ adoption behavior of conservation tillage technology based on distributed cognition theory. China Land Sci. 35, 75–84. doi: 10.11994/zgtdkx.20210930.124356
Lu, H., Zhang, P., Hu, H., Xie, H., Yu, Z., and Chen, S. (2019). Effect of the grain-growing purpose and farm size on the ability of stable land property rights to encourage farmers to apply organic fertilizers. J. Environ. Manag. 251:109621. doi: 10.1016/j.jenvman.2019.109621
Maillard, E., and Angers, D. A. (2014). Animal manure application and soil organic carbon stocks: a meta-analysis. Glob. Change Biol. 20, 666–679. doi: 10.1111/gcb.12438
Memon, M. S., Guo, J., Tagar, A. A., Perveen, N., Ji, C., Memon, S. A., et al. (2018). The effects of tillage and straw incorporation on soil organic carbon status, rice crop productivity, and sustainability in the rice-wheat cropping system of eastern China. Sustainability 10:961. doi: 10.3390/su10040961
Millot, A., and Maïzi, N. (2021). From open-loop energy revolutions to closed-loop transition: what drives carbon neutrality? Technol. Forecast. Soc. Chang. 172:121003. doi: 10.1016/j.techfore.2021.121003
Moore, J. L., and Rocklin, T. R. (1998). The distribution of distributed cognition: multiple interpretations and uses. Educ. Psychol. Rev. 10, 97–113. doi: 10.1023/A:1022862215910
Nayak, D., Saetnan, E., Cheng, K., Wang, W., Koslowski, F., Cheng, Y.-F., et al. (2015). Management opportunities to mitigate greenhouse gas emissions from Chinese agriculture. Agric. Ecosyst. Environ. 209, 108–124. doi: 10.1016/j.agee.2015.04.035
Poulton, P. L., Dalgliesh, N. P., Vang, S., and Roth, C. H. (2016). Resilience of Cambodian lowland rice farming systems to future climate uncertainty. Field Crop Res. 198, 160–170. doi: 10.1016/j.fcr.2016.09.008
Rong, Y., and Hou, Y. (2022). Farmers’ willingness to participate in voluntary field water management greenhouse gas emission reduction projects based on a context–attitude–behavior framework. Sustainability 14:15698. doi: 10.3390/su142315698
Sá, J. C., Lal, R., Cerri, C. C., Lorenz, K., Hungria, M., and de Faccio Carvalho, P. C. (2017). Low-carbon agriculture in South America to mitigate global climate change and advance food security. Environ. Int. 98, 102–112. doi: 10.1016/j.envint.2016.10.020
Salomon, G. ed. (1997). Distributed cognitions: Psychological and educational considerations 1st pbk. ed. Cambridge: Cambridge University Press.
Schoonhoven, Y., and Runhaar, H. (2018). Conditions for the adoption of agro-ecological farming practices: a holistic framework illustrated with the case of almond farming in Andalusia. Int. J. Agric. Sustain. 16, 442–454. doi: 10.1080/14735903.2018.1537664
Shahangian, S. A., Tabesh, M., and Yazdanpanah, M. (2021). Psychosocial determinants of household adoption of water-efficiency behaviors in Tehran capital, Iran: application of the social cognitive theory. Urban Clim. 39:100935. doi: 10.1016/j.uclim.2021.100935
Shan, Y., Guan, D., Zheng, H., Ou, J., Li, Y., Meng, J., et al. (2018). China CO2 emission accounts 1997–2015. Sci Data 5:170201. doi: 10.1038/sdata.2017.201
Shi, Z. H., and Zhang, K. X. (2022). Re-examination of farmers’ green production “strong willings and weak behaviors”: based on the perspective of resource constraints and social norms—personal norms as an intermediary variable. Chin. J. Agricult. Res. Reg. Plann. 43, 43–54. doi: 10.7621/cjarrp.1005-9121.20220805
Sultana, N., Zhao, J., Cai, Y., Rahman, G. K. M. M., Alam, M. S., Faheem, M., et al. (2022). Methanotrophy-driven accumulation of organic carbon in four paddy soils of Bangladesh. Pedosphere 32, 348–358. doi: 10.1016/S1002-0160(20)60030-3
Sun, G., Sun, M., Du, L., Zhang, Z., Wang, Z., Zhang, G., et al. (2021). Ecological rice-cropping systems mitigate global warming-a meta-analysis. Sci. Total Environ. 789:147900. doi: 10.1016/j.scitotenv.2021.147900
Sun, M., Zhan, M., Zhao, M., Tang, L. L., Qin, M. G., Cao, C. G., et al. (2019). Maize and rice double cropping benefits carbon footprint and soil carbon budget in paddy field. Field Crop Res. 243:107620. doi: 10.1016/j.fcr.2019.107620
Tan, Y. H., and Mei, Y. (2018). Analysis on influencing factors of farmers’ land transfer behavior from distributed cognition perspective——based on a survey in typical areas of Wuhan City circle. Res. Develop. Market 34, 547–553. doi: 10.3969/j.issn.1005-8141.2018.04.016
Tan, S., Xie, D., Ni, J., Chen, F., Ni, C., Shao, J., et al. (2022). Characteristics and influencing factors of chemical fertilizer and pesticide applications by farmers in hilly and mountainous areas of Southwest, China. Ecol. Indicat. 143:109346. doi: 10.1016/j.ecolind.2022.109346
Tang, H., Cheng, K., Shi, L., Li, C., Wen, L., Li, W., et al. (2022). Effects of long-term organic matter application on soil carbon accumulation and nitrogen use efficiency in a double-cropping rice field. Environ. Res. 213:113700. doi: 10.1016/j.envres.2022.113700
Tao, F., Palosuo, T., Valkama, E., and Makipaa, R. (2019). Cropland soils in China have a large potential for carbon sequestration based it on literature survey. Soil Tillage Res. 186, 70–78. doi: 10.1016/j.still.2018.10.009
Wan, N., Li, S., Li, T., Cavalieri, A., Weiner, J., Zheng, X., et al. (2019). Ecological intensification of rice production through rice-fish co-culture. J. Clean. Prod. 234, 1002–1012. doi: 10.1016/j.jclepro.2019.06.238
Wang, J., Hu, C. H., and Hu, E. H. (2021). Study on training mode and influencing factors of China’s ecological agriculture under background of rural revitalization strategy—based on grounded approach. Jiangsu Agricult. Sci. 49, 22–28. doi: 10.15889/j.issn.1002-1302.2021.23.004
Wei, W., Cui, Q., and Sheng, Y. (2022). Dual carbon goals and the impact on future agricultural development in China: a general equilibrium analysis. China Agric. Econ. Rev. 14, 664–685. doi: 10.1108/CAER-02-2022-0020
Wei, L., Ge, T., Zhu, Z., Luo, Y., Yang, Y., Xiao, M., et al. (2021). Comparing carbon and nitrogen stocks in paddy and upland soils: accumulation, stabilization mechanisms, and environmental drivers. Geoderma 398:115121. doi: 10.1016/j.geoderma.2021.115121
Wu, H. P., Qin, H. J., He, B., You, Y., Chen, J. F., Zou, C. P., et al. (2022). A brief discussion on the development trend of the agricultural non-point source pollution control model based on carbon neutrality. Ecol. Environ. Sci. 31, 1919–1926. doi: 10.16258/j.cnki.1674-5906.2022.09.023
Xia, L. L., Yan, X. Y., and Cai, Z. C. (2020). Research progress and prospect of greenhouse gas mitigation and soil carbon sequestration in croplands of China. J. Agro Environ. Sci. 39, 834–841. doi: 10.11654/jaes.2020-0108
Xinfa, T., and Xue, L. (2022). Research on energy policies of Jiangxi province under the dual-carbon constraints. Front. Environ. Sci. 10:986385. doi: 10.3389/fenvs.2022.986385
Xu, Y. D. (2022). Conservation agriculture-mediated soil carbon sequestration: a review. Chin. J. Eco-Agric. 30, 658–670. doi: 10.12357/cjea.20210889
Xu, B., and Lin, B. (2017). Factors affecting CO2 emissions in China’s agriculture sector: evidence from geographically weighted regression model. Energy Policy 104, 404–414. doi: 10.1016/j.enpol.2017.02.011
Yadav, G. S., Babu, S., Das, A., Mohapatra, K. P., Singh, R., Avasthe, R. K., et al. (2020). No-till and mulching enhance energy use efficiency and reduce carbon footprint of a direct-seeded upland rice production system. J. Clean. Prod. 271:122700. doi: 10.1016/j.jclepro.2020.122700
Yan, M., Luo, T., Bian, R., Cheng, K., Pan, G., and Rees, R. (2015). A comparative study on carbon footprint of rice production between household and aggregated farms from Jiangxi, China. Environ. Monit. Assess. 187:332. doi: 10.1007/s10661-015-4572-9
Yang, B. J., Li, X. M., Hu, Q. L., Liu, N., and Huang, G. Q. (2022). Effects of rotation and fallow patterns on organic carbon and its components in soil of paddy fields. J. Huazhong Agricult. Univ. 41, 51–58. doi: 10.13300/j.cnki.hnlkxb.2022.06.006
Yang, X. J., Qi, Z. H., Yang, C. Y., and Liu, Z. (2021). Can the new type of agricultural management promote the promotion of ecological agricultural technology: take Rice and shrimp co-cultivation technology as an example. Resour. Environ. Yangtze Basin 30, 2545–2556. doi: 10.11870/cjlyzyyhj202110022
Ying, Y., Ming-da, L., Dan, Y., Wei, Z., Hui, A., Yao-jing, W., et al. (2014). Effect of different rice-crab coculture modes on soil carbohydrates. J. Integr. Agric. 13, 641–647. doi: 10.1016/S2095-3119(13)60722-4
Yu, L., Chen, C., and Gao, Y. (2020). Confucian values, trust, and family farm adoption of green control techniques. Environ. Sci. Pollut. Res. 27, 35099–35111. doi: 10.1007/s11356-020-09724-x
Zhang, J., Chen, M., Huang, C., and Lai, Z. (2022a). Labor endowment, cultivated land fragmentation, and ecological farming adoption strategies among farmers in Jiangxi Province, China. Land 11:679. doi: 10.3390/land11050679
Zhang, J., Deng, A. X., Shang, Z. Y., Tang, Z. W., Yan, S. J., and Zhang, W. J. (2021). Innovative rice cropping for higher yield and less CH4 emission under crop straw incorporation. Crops 37, 230–235. doi: 10.16035/j.issn.1001-7283.2021.06.037
Zhang, Z., Du, L., Xiao, Z., Li, C., Wang, Z., Zhou, P., et al. (2022b). Rice-crayfish farming increases soil organic carbon. Agric. Ecosyst. Environ. 329:107857. doi: 10.1016/j.agee.2022.107857
Zhang, X., Fang, Q., Zhang, T., Ma, W., Velthof, G. L., Hou, Y., et al. (2020b). Benefits and trade-offs of replacing synthetic fertilizers by animal manures in crop production in China: a meta-analysis. Glob. Change Biol. 26, 888–900. doi: 10.1111/gcb.14826
Zhang, Q., Song, Y., Wu, Z., Yan, X., Gunina, A., Kuzyakov, Y., et al. (2020a). Effects of six-year biochar amendment on soil aggregation, crop growth, and nitrogen and phosphorus use efficiencies in a rice-wheat rotation. J. Clean. Prod. 242:118435. doi: 10.1016/j.jclepro.2019.118435
Zhang, C. Q., Wang, L., Hua, C. L., Jin, S. Q., and Liu, P. T. (2016). Potentialities of fertilizer reduction for grain produce and effects on carbon emissions. Resour. Sci. 38, 790–797. doi: 10.18402/resci.2016.04.20
Zhang, X., Yin, S., Li, Y., Zhuang, H., Li, C., and Liu, C. (2014). Comparison of greenhouse gas emissions from rice paddy fields under different nitrogen fertilization loads in Chongming Island, Eastern China. Sci. Total Environ. 472, 381–388. doi: 10.1016/j.scitotenv.2013.11.014
Zhao, C. J., Li, J., and Feng, X. (2021). Development strategy of smart agriculture for 2035 in China. Eng. Sci. 23:1. doi: 10.15302/J-SSCAE-2021.04.001
Zhao, X., Ma, X., Chen, B., Shang, Y., and Song, M. (2022). Challenges toward carbon neutrality in China: strategies and countermeasures. Resour. Conserv. Recycl. 176:105959. doi: 10.1016/j.resconrec.2021.105959
Zheng, X., Lu, Y., Yuan, J., Baninla, Y., Zhang, S., Stenseth, N. C., et al. (2020). Drivers of change in China’s energy-related CO2 emissions. Proc. Natl. Acad. Sci. U. S. A. 117, 29–36. doi: 10.1073/pnas.1908513117
Zheng, Z. Y., Wang, W. C., Li, Z. J., Sun, Y., Hu, A. S., Xiao, D. D., et al. (2019). A typical ecological agriculture pattern-planting and breeding in rice field: a review. Jiangsu Agricult. Sci. 47, 11–16. doi: 10.15889/j.issn.1002-1302.2019.04.003
Keywords: low-carbon rice farming (LCRF), distributed cognition, cultivated land protection, “double carbon”, food security
Citation: Huang Q, Chen M, Zhang T, Zhang F and Zhang J (2024) Analysis of low-carbon rice farming behavior and its influencing factors in farmers under the distributed cognition perspective—empirical study based on 2,173 farmers in Jiangxi Province. Front. Sustain. Food Syst. 7:1296922. doi: 10.3389/fsufs.2023.1296922
Received: 03 October 2023; Accepted: 07 December 2023;
Published: 05 January 2024.
Edited by:
Debasis Mitra, National Rice Research Institute (ICAR), IndiaReviewed by:
Shankarappa Sridhara, University of Agricultural and Horticultural Sciences, Shivamogga, IndiaCopyright © 2024 Huang, Chen, Zhang, Zhang and Zhang. This is an open-access article distributed under the terms of the Creative Commons Attribution License (CC BY). The use, distribution or reproduction in other forums is permitted, provided the original author(s) and the copyright owner(s) are credited and that the original publication in this journal is cited, in accordance with accepted academic practice. No use, distribution or reproduction is permitted which does not comply with these terms.
*Correspondence: Meiqiu Chen, Y21xMTJAanhhdS5lZHUuY24=
Disclaimer: All claims expressed in this article are solely those of the authors and do not necessarily represent those of their affiliated organizations, or those of the publisher, the editors and the reviewers. Any product that may be evaluated in this article or claim that may be made by its manufacturer is not guaranteed or endorsed by the publisher.
Research integrity at Frontiers
Learn more about the work of our research integrity team to safeguard the quality of each article we publish.