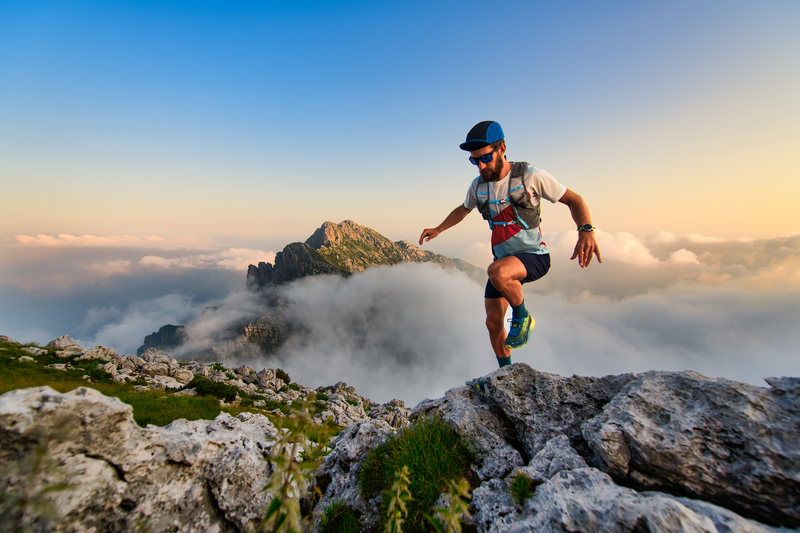
94% of researchers rate our articles as excellent or good
Learn more about the work of our research integrity team to safeguard the quality of each article we publish.
Find out more
ORIGINAL RESEARCH article
Front. Sustain. Food Syst. , 04 January 2024
Sec. Social Movements, Institutions and Governance
Volume 7 - 2023 | https://doi.org/10.3389/fsufs.2023.1284880
Agriculture is significantly impacted by the variability in weather patterns, imposing substantial constraints on farmers’ ability to make informed tactical and strategic decisions regarding their crops. Seasonal climate projections have shown potential for informing agricultural decisions, but the actual adoption of climate information by farmers has been relatively slow and limited. The present study was conducted with the objective of investigating the characteristics of adopters, the communication network, and the level of farmer’s satisfaction concerning the adoption and continued use of Agromet Advisories Services (AAS). Two semi-arid districts, namely Kurnool and Anantapur in Andhra Pradesh, were purposively selected, wherein 280 farmers constituted the sampling frame. In this study, social network analysis (SNA) was conducted to examine the peer-to-peer communication patterns, while importance-performance analysis (IPA) was employed to evaluate farmer’s satisfaction, contributing to the continued adoption of AAS. The findings revealed that with regard to personality and communication characteristics, farmers were in the low category for their ability to cope with uncertainties and risk and even for their information-seeking behavior. Furthermore, the results showed farmers to be highly satisfied with the overall adoption of AAS. However, the IPA matrix revealed that among the nine attributes, the credibility of the forecasts needed refinement to promote sustained adoption. Excessive emphasis was placed on attributes such as the frequency of forecasts, which could be channeled into other initiatives. Peer-to-peer communication emerged as a crucial strategy in the adoption of AAS. Governments, non-governmental organizations, and extension functionaries should make a concerted effort to enhance the continued adoption of AAS by involving local stakeholders in sharing and participating in climate information production, forming farmer’s groups, and focusing on farmers’ literacy toward AAS.
The impact of climate change in the form of increased extreme weather events and variability has been observed in India over the last decade. Specifically, there has been a noticeable shift in rainfall patterns in semi-arid tropical regions, where a substantial 80% of the total annual rainfall occurs within a concentrated 3 to 4 month period (Mitra et al., 2013). Extreme weather events such as floods and droughts, which are outcomes of climate change, have detrimental effects on livelihoods, particularly in rainfed regions. In India, the majority of farmers are small-scale and marginal, with limited access to technologies and resources, rendering them highly vulnerable to climate change. The provision of weather-based information, coupled with recommendations for agricultural practices, can assist farmers in preparing for various weather anomalies (Webster et al., 2010; Haigh et al., 2015). While farming communities worldwide have historically adapted to a wide range of weather and climatic conditions, the increasingly erratic climate variability and the rapid pace of other transformative forces are now overwhelming indigenous knowledge and traditional coping practices (Chattopadhyay and Chandras, 2018).
An Agromet Advisory Service (AAS) relies on scientific insights from meteorology in conjunction with agricultural information. This comprehensive package of information is then disseminated to farmers with the objective of “empowering farmers to plan their agricultural activities strategically to minimize crop damage during adverse weather conditions” (Tall et al., 2014). Weather forecasts followed by corresponding advisories can make a significant difference in proactively managing the adverse effects of weather on farming. This is primarily because the costs associated with reducing weather-related risks beforehand are substantially lower than the expenses incurred in managing losses after they have already occurred. In India, a diverse array of multi-channel systems is employed to disseminate agrometeorological advisories to farmers. These channels encompass All India Radio (AIR), Doordarshan television and radio networks, newspapers, the internet, Short Message Service (SMS), and Interactive Voice Response (IVR) technology. Numerous organizations are actively engaged in disseminating agrometeorological advisories in the form of SMS and IVR through public–private partnership (PPP) arrangements with the agricultural community. As of the latest available data, this service has directly benefited 21.69 million farmers (Chattopadhyay and Chandras, 2018). Advisories are issued in the local language at least twice a week in the summer months and more frequently during the agricultural season, as required, thus alerting farmers and giving them enough time to implement suggested measures. The advisories are disseminated through SMSs to mobile phones, wallpapers that are put up at prominent places in the project villages, and by word-of-mouth. Another source for receiving agro-met information was through membership in a “WhatsApp group.”
Adapting to climate change necessitates a sustained commitment, involving not only the initial adoption of climate-resilient agricultural practices by farmers but also their continued implementation without discontinuation in the short-to-medium term. AAS adoption is not a point event; rather, it is a continuous process throughout the growing season. Hence, farmers must consistently embrace Agromet Advisory Services (AAS) and implement them in their fields to mitigate the effects of weather aberrations. Worldwide, prior research on drivers of adoption and its continuation mainly focused on farmers’ socio-economic and structural factors. However, recent studies addressed farmers’ attitudes and motivations (Franks and Emery, 2013; Price and Leviston, 2014; Li et al., 2019), the role of human and social capital, e.g., of farmers’ knowledge, personality traits, information availability, and peers’ influence (Moschitz et al., 2015; Pagliacci et al., 2020) and satisfaction (Han et al., 2018). Studies have also highlighted that farmer satisfaction is considered to be an important indicator of the continued adoption or sustainability of a technology/program (Elias et al., 2016). A high level of satisfaction with the innovation could lead to continued and intense use, thereby increasing loyalty to the innovation and the institutions behind it (de Oca et al., 2021). Consultation with fellow farmers also reinforces the adoption of climate-resilient agricultural practices and reduces the chances of discontinuation (Tran et al., 2020; Ferrer et al., 2023). While there is extensive literature focused largely on the factors that influence farmers to adopt climate-resilient agricultural practices, studies addressing the issue of the continuation of farmers’ adoption of climate-resilient agricultural practice is still in the nascent stage in India. In this context, the present study aims to analyze the communication characteristics, patterns, and satisfaction of farmers regarding the adoption of Agromet Advisory Services (AAS) in selected areas as the drivers of continuous adoption.
Apart from researching highly climatically vulnerable landscapes, the present study makes other contributions to the adoption and continuation of AAS literature as well. First, it studies the satisfaction of each AAS attribute along with overall satisfaction using the IPA matrix. Second, knowledge about AAS and the role of peer-to-peer social networks are examined explicitly. Third, the study also emphasized the socio-psycho-communication characteristics of the adopters. Finally, constraints in the adoption of AAS are also investigated to provide the necessary suggestions for enhanced adoption. The research findings will help policymakers, field-level extension agents, private organizations, and NGOs to plan and implement more efficient and effective agricultural extension services for the uptake of weather-based advisories.
Managing the risk associated with climate variability or vagaries of weather is integral to any comprehensive strategy for adapting agriculture and food systems to a changing climate (Chattopadhyay and Chandras, 2018; Mittal and Hariharan, 2018). Climate services are receiving increasing attention globally as an important component in making agriculture resilient to the impacts of climate variability and change (Hansen et al., 2019). Climate information services (CIS), which involve the production, translation, transfer, and use of climate information for individual and societal decision-making, play an important role in managing agricultural systems productively, profitably, and with reduced risk (Hansen et al., 2019; Carr et al., 2020).
In India, the National Centre for Medium Range Weather Forecasting (NCMRWF), India Meteorological Department (IMD), Indian Council of Agricultural Research (ICAR), and State Agricultural Universities (SAUs) work together to provide farmers with weather forecasts and Agrometeorological Advisory Services (AAS) advice on how to manage their crops accordingly. Medium-range weather forecasts, which predict weather conditions for the next 2 to 3 weeks, are used to create location-specific forecasts for each agroclimatic zone and district. This helps farmers to get the most relevant information for their area (Chaubey et al., 2018). Impact studies suggest that farmers can increase production by 10% if they have access to good Agromet advisories (Maini and Rathore, 2011; Craufurd and Balaji, 2014). There are three phases of the climatic information value chain, namely forecast production, translation, and integration and application (Ofoegbu and New, 2022). In most countries, past works on climate services were mainly focused on managing extreme events through early warning systems aimed at better preparedness and reacting in a way that reduces losses (Pulwarty and Sivakumar, 2014). The application part of the climate service value chain has not been much explored. Hence, the present study deals with the application research of Agromet advisories. Furthermore, it is believed that mobile-phone-enabled agro-advisory services have the potential to reduce information gaps and generate awareness about improved technologies, which leads to improved adoption of technology (Mittal and Hariharan, 2018). It has been observed that seasonal climate projections have shown potential for informing agricultural decisions, but the actual adoption of climate information by farmers has been relatively slow and limited (Goddard et al., 2010; Lemos and Rood, 2010). Furthermore, studies have revealed that low adoption of weather-based advisories is also interlinked with the limited uptake of climate-resilient agricultural technologies (Ziro et al., 2023).
Communication and knowledge sharing are vital in the adoption and sustainability of any agricultural innovations (Babu et al., 2012; Ashraf et al., 2015). Social networks are crucial in technology adoption, diffusion, and innovation decisions (Rampersad et al., 2012), helping to transfer knowledge within and between organizations (Massaro et al., 2019; Marchiori and Franco, 2020). Social network analysis (SNA) enables the identification of stakeholders within a network and the understanding of the relationships and reciprocity between actors and their associated influence (Valujeva et al., 2023). Communication networks play a role in shaping an individual’s knowledge perspective because there is an interaction happening among people in one community. A network consists of a group of individual people who organize themselves together and have a basic rule willingly to exchange information, material, team work, and empowerment.
Research on adoption and sustained use has shown that a wide range of factors influences farmers’ choices, including environmental factors, technology, policy design features, farm structure, farmer socio-economic characteristics, attitudes and motivations, and social aspects (Page and Bellotti, 2015; Luo et al., 2016). Past studies have highlighted that farmers’ satisfaction with the services they receive increases the likelihood of achieving agricultural development outcomes (Ragasa and Mazunda, 2018). Additionally, farmers’ satisfaction with agricultural information is also influenced by socio-economic and institutional factors, although they differ significantly from the choice of agricultural information channels (Wale and Mkuna, 2023). Client satisfaction is defined in terms of how products and services supplied meet or surpass customer expectations (Yaqub and Halim, 2018). Satisfaction with services can be seen from two different perspectives, as a ‘process’ or ‘outcome’ measure (Kassem et al., 2021). Concerning extension services, Buadi et al. (2013) noted that overall satisfaction with a service depends on high levels of desired quality of all attributes, as one limiting attribute can render a service of little value. Studies on farmer satisfaction have mainly concentrated on satisfaction with agricultural extension services (Elias et al., 2016; Kassem et al., 2021). Few published studies have investigated the overall satisfaction of farmers in adopting AAS (Dupdal et al., 2020; Arpitha et al., 2022). There is a significant gap in the research pertaining to satisfaction with various attributes of AAS.
According to the survey and assessment of the National Innovation in Climate-Resilient Agriculture-Indian Council of Agricultural Research (NICRA-ICAR), a total of 121 districts are climate-vulnerable districts in the country. Of these, five districts, namely Anantapur, Chittoor, Kurnool, Srikakulam, and West Godavari, are located in Andhra Pradesh (Rama Rao et al., 2019). Under the aegis of NICRA, Agromet advisories are being provided to the farmers through its Krishi Vigyan Kendra located in India in these climatically vulnerable districts. The present study was conducted in two semi-arid districts, namely Kurnool and Anantapur of Andhra Pradesh, selected purposively where Agromet advisories (AAS) were given to farmers through the KVK in 2011 and 2015, respectively. These districts are ideal for this type of assessment because their cropping season rainfall is low and highly variable (cv. 50%), making farmers highly vulnerable to climate change (Ramaraj et al., 2023). Additionally, farmers in these districts have been aware of AAS for a long time, making them suitable for studying continued adoption. Long association with technology leads to the development of positive attitudes over time. These districts are neighboring districts and experience tropical climates. The temperature in Kurnool and Anantapur districts ranges from 26°C to 45°C during summer and 12°C to 31°C in winter. The average annual rainfall of Kurnool district is about 671 mm, and for Anantapur district, it is about 553 mm. Kurnool district lies between latitude 14°-54’ North to 16°-11′ and longitude 76°-56′ East to 78°-25′ East. The major soil type of the area is black (61%) soil, followed by red (33%) soil. Agriculture is mostly rainfed, and major crops in these areas are cotton, paddy, groundnut, sorghum, pigeonpea, chickpea, maize castor, and sunflower. Anantapur district is situated between 76° 47′ and 78° 26′E of the eastern longitudes and 13° 41′ and 15° 14’N of northern latitudes and is the driest district of Andhra Pradesh. The soil types are predominantly red (78%) followed by black (20%), and prominent crops include groundnut, pigeonpea, pearl millet, castor, paddy, chickpea, and chilies (Figure 1).
Three villages in Kurnool and two villages in Anantapur, where agrometeorological advisories were regularly distributed, were purposively selected as the study sites. A sampling frame was constructed using the list of farmers who were consistently receiving and adopting the Agromet Advisory Services (AAS) provided by the Krishi Vigyan Kendra (KVK) in the respective villages. To create a representative sample, farmers were chosen from each village using a proportionate random sampling method. The total sample size for this study comprised 280 farmers. Data collection was conducted through face-to-face interviews with the help of a semi-structured questionnaire from November 2019 to March 2020. Subsequently, due to the COVID-19 pandemic, telephonic interviews were conducted until July 2020.
The questionnaire developed in this study comprised two distinct sections. The first section encompassed inquiries related to demographic information, personality and communication patterns, and network characteristics, while the second part contained questions aimed at assessing satisfaction levels and constraints. Personality and communication characteristic variables were adapted and modified from the study by Fry et al. (2018). To investigate the communication networks of the farmers, respondents were requested to enumerate the names of individuals, along with their respective relationships, from whom they acquired information regarding Agromet Advisory Services (AAS) and its practical application in the field. Furthermore, they were asked to specify to whom they provided advice concerning AAS (Albizua et al., 2021). For the purpose of network visualization and computation of centrality measures, we employed UCINET 6.747 (Borgatti et al., 2002) in its trial version and NetDraw 2.176 as an open-source tool. To assess the satisfaction of farmers with various attributes of AAS that contribute to its sustained adoption, importance-performance analysis (I.P.A.) was employed. Initially introduced by Martilla and James (1977), I.P.A. generates a 2-dimensional graph that measures the importance and satisfaction levels of different attributes, thereby offering insights into the existing situation and practical recommendations (Warner et al., 2016; Wulansari et al., 2018). This analytical framework has gained acceptance in diverse fields and contexts, including the catering and hotel industry (Back, 2012), education (O’Neill and Palmer, 2004), healthcare (Abalo et al., 2007), banking (Joseph et al., 2005), public management and administration (Van Ryzin and Immerwahr, 2007), employee service management improvement (Chang, 2013), and information and telecommunication technologies (Levenburg and Magal, 2005). Consequently, the IPA matrix can also be effectively employed to assess customer satisfaction with agricultural services. IPA uses a Likert-type scale to measure importance and satisfaction. The quadrants of the IPA matrix are defined by the means of the importance and satisfaction scores. The attributes will be placed in the four quadrants based on their scores. Each quadrant of IPA is interpreted as having implications for the prioritization and management of attributes (Figure 2 and Table 1).
Farmer’s satisfaction with the adoption of AAS was analyzed in relation to respondents’ perceptions of the credibility, salience, and legitimacy of climate information and services (Cash et al., 2003; Tang and Dessai, 2012; Daly et al., 2016). Credibility refers to scientific adequacy, trustworthiness, and reliability of knowledge. Salience refers to the relevance of knowledge to practical decision-making. Legitimacy refers to the openness and fairness of knowledge, meaning that it incorporates diverse perspectives and is equally beneficial to all users (Daly et al., 2016; van Oudenhoven et al., 2018). Hence, each indicator, namely credibility, salience, and legitimacy, was measured on three items each. Five-point Likert-type scales have been used to measure the importance and performance levels. Scales range from 1, least important, to 5, most important, in the importance part, and from 1, highly dissatisfied, to 5, highly satisfied, in the satisfaction part. The questionnaire was prepared in two languages: Telugu and English. The Cronbach alpha for the attribute statements was 0.84, indicating high internal consistency (Tables 2–4). Analysis of the Customer Satisfaction Index (CSI) is aimed to determine the level of satisfaction of farmers as consumers in applying AAS in the field. The calculation of this index is done through four stages.
Table 5 depicts the demographic characteristics of the respondents in both the Kurnool and Anantapur districts. The results revealed that the majority of respondents in both districts were middle-aged. However, a significant proportion of young respondents, 16 and 19% in Kurnool and Anantapur, respectively, were also present. In Anantapur, 46% of farmers had attained a high school level of education, while the corresponding figure for Kurnool district was 33%. Agriculture (farming) was the primary occupation for most of the respondents in both districts. Semi-medium farmers accounted for 52% in Kurnool and 33% in Anantapur, while small farmers constituted 39 and 47% in Kurnool and Anantapur, respectively. Rainfall was the main source of irrigation for crops in both districts.
The personality and communication characteristics of the adopter farmers have been depicted in Table 6. The Mann–Whitney U-test was conducted to examine the difference between the farmers of the two districts on personality and communication characteristics. As shown in Table 6, a significant difference was found between the farmers in their ability to cope with risk and uncertainties, their interconnectedness with the social system at a 1 % level of significance, and contact with change agents at a 5 % level of significance. For traits such as empathy, fatalism, and dogmatism, the majority of farmers from both districts fell into the average category. However, for traits such as the ability to cope with uncertainty and risk, ability to deal with abstraction, attitude toward change, attitude toward science, cosmopoliteness, contact with change agents, degree of opinion leadership, exposure to mass media, social participation, interconnectedness with the social system, seeking information about the innovation, and knowledge about the innovation, the majority of farmers were classified as being in a low category.
The innovation-decision process constitutes a systematic approach employed by prospective adopters for the purpose of gathering information and rendering decisions concerning novel technologies or practices. It typically commences with the awareness of the innovation in question and the acquisition of knowledge from diverse sources. Figure 3 delineates the various information sources through which farmers initially garnered knowledge about Agromet Advisory Services (AAS). The findings elucidate that personal interactions with officials emerged as the most prevalent source of initial awareness about AAS among farmers in Anantapur, comprising 52% of the cases. In contrast, merely 34% of Kurnool farmers derived their primary information from officials. Intriguingly, in Kurnool, fellow farmers, panchayat leaders, and progressive farmers played a more substantial role in disseminating initial knowledge about AAS, collectively constituting the primary sources for the majority of cases. Conversely, a mere 22% of Anantapur farmers received their initial awareness from these same sources. In both districts, a small percentage of farmers (17% in Anantapur and 13% in Kurnool) acquired their initial knowledge about AAS through short messaging services (SMS; Figure 3).
Figure 3. First-time information sources received by the farmers in these districts (Based on the author’s field survey).
The Agricultural Knowledge and Information Systems (AKIS), developed in the late 1980s, emphasized the observation that farmers acquire knowledge from a multitude of sources, often extending beyond formal channels (Sutherland and Marchand, 2021). Peer-based learning among farmers has played a pivotal role in promoting the widespread adoption of advanced agricultural technologies on a global scale. In the current study, we focused on examining the communication patterns among farmers regarding the adoption of Agromet Advisory Services (AAS) within three villages situated in the Kurnool District of Andhra Pradesh, India (Figures 4–6 and Table 7). The graph density of Yagantipalle (Figure 5; density = 0.019) was the lowest, followed by Mirapur (density = 0.025) and Chirolokuttur (density = 0.079). Generally, density measures the level of connected edges within a network relative to the total possible value and ranges from zero to 1. According to Wasserman and Faust (1994), centralization “can be viewed as a measure of how unequal individual actors are. It is a measure of variability, dispersion, or spread.” In the case of the Chirolokuttur network, the degree of centralization (Degree centralization = 0.294) was found to be the highest among the three villages, followed by Yagantipalle and Mirapur. These findings suggest that in this particular village, central actors are closely clustered within a more centralized structure centered around a focal actor. The network graphs of Chirolokuttur (Figure 6) depict a scenario where the village has a limited number of opinion leaders operating within a centralized structure (average degree = 5.222) characterized by a high degree of interconnectedness (connectedness = 0.963) compared to the other two networks.
The study assessed the perceived importance and satisfaction levels associated with nine attributes of Agromet advisories by farmers. The Friedman test was employed to identify statistical disparities in the importance and satisfaction levels between farmers from the two districts receiving Agromet Advisory Services (AAS), as presented in Table 8. Among farmers in the Kurnool district, the highest mean importance ratings were attributed to “Scientific adequacy” in the credibility category, “Relevance of the knowledge to present decision making” in the salience category, and “Usefulness/expected benefits of the information” in the legitimacy category. In contrast, farmers in Anantapur district accorded the highest mean importance ratings to “Trustworthiness of the source” in the credibility category, “Timely availability of the forecasts” in the salience category, and “Usefulness/expected benefits of the information” in the legitimacy category. Significant statistical differences in the importance levels were observed between the two districts for the attributes of “Trustworthiness of the source,” “Timely availability of the forecasts,” and “Frequency of the forecast.” Overall, the farmers of Anantapur placed more importance on these features than the farmers from Kurnool District. With reference to the perceived level of satisfaction, scientific adequacy forecasts and usefulness/expected benefits were given the highest mean for both the districts, while timely availability of the information and frequency of the forecast had the highest mean for Kurnool and Anantapur in the category of salience, respectively. Significant statistical difference in the satisfaction level was observed for the following features: scientific adequacy, reliability of the information given in the forecast, frequency of the forecast, open and fairness of knowledge, and applicability to a large number of farmers between the two districts. The results obtained in this study, using the Friedman test, can significantly help to understand the difference in the perception of the farmers in adjoining districts on attributes of AAS.
For the IPA, the means of the importance levels (y-axis) of the nine attributes examined were plotted along with the means of their levels of satisfaction (x-axis). The overall median of both attributes was used as the crossing point in the IPA matrix, a standard procedure, resulting in four quadrants. A complete picture of the position of indicators in the Cartesian quadrant can be shown in Figures 7, 8. The reliability of the information given in the forecast (Credibility 3) was found in quadrant I for both districts. The position of “Reliability of the information given in the forecast” is notable because it was perceived as the most important attribute of AAS, but the farmers had low satisfaction with this attribute. Quadrant I, or main priority, is an attribute that is considered to affect farmers’ satisfaction and is considered important for the continuous adoption of AAS. “Scientific adequacy,” “Trustworthiness of the source,” “Timely availability of the forecasts,” and “Usefulness/expected benefits of the information” were the attribute present in the quadrant II for both Kurnool and Anantapur districts. In the third quadrant, “Frequency of the forecast,” “Open and fairness of knowledge,” and “Applicable to large number of farmers” was present for Kurnool district, while for Anantapur, “Relevance of the knowledge to present decision making” was also observed. In Quadrant IV, the frequency of the forecast was observed for the Anantapur district. The result of quadrant IV indicates that a factor affecting customers is less important, but excessive implementation has been done.
The Customer Satisfaction Index considers the significance of each attribute in influencing the level of satisfaction with that attribute. This, in turn, has an impact on the overall satisfaction level of farmers when they adopt Agromet Advisory Services (AAS) in their fields. Top-of-form customer satisfaction was analyzed using the customer satisfaction index (Table 9). It is evident from Table 9 that the customer satisfaction index for the farmers receiving AAS was about 84.02, which falls in the highly satisfied category. Based on the above results, if the CSI analysis is associated with the results of IPA analysis, it can be concluded that the CSI value of 84.02% comes from the variables that are in quadrant II as the variables in quadrant II have a high level of performance and importance and quadrant IV has a low level of performance level and importance level. The variables in quadrant IV have been able to influence the level of satisfaction of AAS farmers. The quadrant III variable is a quadrant that shows that the attributes in this quadrant are less important for the consumer, and the implementation by the extensionists is also considered normal.
The constraints faced by the farmers in adopting Agromet Adviosres are depicted in Table 10. Friedman test was carried out to study the difference in the constraints. A statistically significant difference (f = 258.08, p = 0.000) was observed among the constraints experienced by the farmers. The highest mean rank (Mean rank = 4.88) was accorded to inputs not being available on time while implementing the messages in the field. Farmers faced the problem of scarcity of inputs when they were advised to sow early or apply fertilizers, pesticides, etc. The second highest constraint (mean rank = 3.57) that the respondents faced was difficulty in understanding the message while implementing. The third most prevalent constraint arises from messages conflicting with their traditional practices or established routines, leading to hesitation or incorrect adoption of the advisories. This discord between the messages and existing practices can result in both non-adoption and misinterpretation of the guidance provided. The fourth constraint was no service to clarify the doubts (mean rank = 3.37), followed by information not applicable to what we are growing (mean rank = 2.95) and advice not received on time (mean rank = 2.84). Farmers require help during implementing the message in the field. As the mobile agro advisory service is a one-way communication, if any doubt arises, it was very difficult for the farmers to get clarification, but in the Kurnool and Anantapur districts, the presence of a village-level extension worker has helped the farmers in clarifying their doubts regarding AAS. This has led to continuous adoption and even the spread of AAS in nearby villages.
The study systematically explored different dimensions with respect to the adoption of AAS by farmers. It investigated the socio-demographic profiles of the farmers adopting AAS in the two districts of Andhra Pradesh, India. The study of demographic factors is important in understanding the social situation of the landscape. The results revealed the predominance of middle-aged farmers following the trend of farming situations all over the world. The issue of an aging farming population is prevalent in most rural areas of the developing world, as younger individuals and men seek alternative income-generating opportunities outside of the rural domain. Several researches have shown that age determines vulnerability to climate change and also plays a major role in adopting climatic change adaptation measures (Villamayor-Tomas, 2018; Mwadzingeni et al., 2022). It is argued that due to their experience, older farmers adopt adaptive interventions but are slow to adopt innovative technological interventions (Aldosari et al., 2019). It is evident in our study that though the AAS was introduced in 2011 and 2015, it took more time to be adopted in the social system. The findings clearly indicated that most farmers were male, had a primary level of education, and fell under the small and semi-medium category in land ownership. The findings are consistent with the study by Oladele et al. (2019).
The investigation also delved into the personality and communication patterns of the farmers. These characteristics play an important role in influencing the farmer’s decision to adopt an innovative technology/practice (Rogers, 2003). Personality traits are individual differences in patterns of thinking, feeling, and behaving. Past studies have pointed out that economic or social factors alone do not convincingly explain the reasons for technology adoption or rejection by farmers. Moreover, researchers have been calling to theorize resilient, intangible, and non-material dimensions of agriculture (e.g., subjective, emotive, and relational forms) that emerge from specific local social-cultural-ecological contexts (Brown, 2014; Shah et al., 2017; Bukchin and Kerret, 2020). The results are consistent with many studies reporting farmers’ low information-seeking behavior and their attitude toward change and science (Rahman et al., 2020; Lahnamäki-Kivelä, 2022). The results on personality and communication characteristics further showed that farmers were in the low category on parameters such as their ability to cope with uncertainties and risk. This trait is particularly important as these areas are highly vulnerable to climate change. Similar studies in the past highlighted that farmers are known to be often risk-averse and, as such, reluctant to adopt new technologies (Mukasa, 2018). A significant difference was found between the Kurnool and Anantapur districts in their contact with change agents, which may arise due to the fact that Krishi Vigyan Kendra Yagantipalle was located near the selected villages in Kurnool. The present investigation recorded the initial information acquisition channel of farmers in the selected districts. Prior studies (Adio et al., 2016; Ndimbwa et al., 2020) concluded that agricultural information and knowledge are vital in empowering smallholder farmers to improve their productivity. The findings also underscore the significance of personal interactions with officials as a primary information source for farmers, especially in Anantapur. It may be attributed to the fact that many extension agencies, such as public and private NGOs and even ICRISAT, are working with farmers in Anantapur. Additionally, the selected villages were quite far from each other, making them more dependent on external agencies for information sources. However, the results also suggested that in Kurnool, peer-to-peer communication and the influence of progressive farmers played a prominent role. Villages in Kurnool were near to each other and were highly interconnected. Similar findings were reported by Patel et al. (2020). The findings are particularly vital for developmental agencies to plan their intervention accordingly. The study emphasizes the imperative for the development of targeted and efficacious communication strategies to facilitate the adoption of innovative agricultural technologies. Furthermore, the informal communication structure was studied by asking farmers in the Kurnool district to name individuals, along with their respective relationships, from whom they acquire information regarding Agromet Advisory Services (AAS). The lowest graph density of Yagatinpalle was due to its proximity to Krishi Vigyan Kendra, Yagatinpalle, the central entity responsible for disseminating Agromet Advisories. Furthermore, Yagatinpalle enjoys robust connectivity with neighboring districts. Consequently, these circumstances have led to frequent visits by numerous agencies, including government officials, scientists, and other stakeholders, resulting in the formation of less densely connected and somewhat fragmented subgroups within the village. The low graph density in Yagantipalle village suggests that communication regarding Agromet Advisory Services (AAS) among local farmers occurs through sparse networks, while Chirolokuttur village, being remote and less accessible on the hill, results in a higher exchange of AAS information from relatively homogenous sources (Scales et al., 2013; Rohit et al., 2021). Centralization was found to be highest in Chirolokuttur due to the presence of a few progressive/opinion leaders who were contacted by most of the farmers in the village. Yi et al. (2021) noted that villages in China also had similar social network structures. Adopters of climate-resilient agricultural practices identify their peers as their primary information sources, and recent evidence suggests that peer influence has a positive impact on farmers’ adoption of climate change adaptation measures (Di Falco et al., 2020; Johnson et al., 2023). Modern farmers are at the core of the social network, and they are better able to control the information flow within the community (Albizua et al., 2021).
The importance-performance matrix was conducted to study the satisfaction of farmers with regard to AAS. Generally, IPA was used profoundly in the tourism sector, and its application was limited in the agricultural field. However, recently, many agricultural researchers have been using the IPA methodology to study satisfaction and performance (Zhang et al., 2021; Dewi et al., 2022). The IPA method demonstrated that the reliability of the forecast fell in the first quadrant for both districts, indicating that it should be given priority and more attention to enhance farmers’ satisfaction. Although great strides have been made in enhancing the reliability of the forecast, farmers should be made aware of the uncertainties involved in weather predictions. Steps should be taken to enhance farmers’ satisfaction in this area. The result of the study is in line with Ramachandrappa et al. (2018), while it is in contrast with work done by Daly et al. (2016). They reported that farmers indicated that climate information does not need to be 100% accurate to be credible and that they understand that the forecasts are probabilistic. The four attributes, namely scientific adequacy, trustworthiness of the source, timely availability of the forecasts, and usefulness/expected benefits of the information, were in the maintenance quadrant, and their advantage should be maintained. Open and fairness of knowledge and applicability to a large number of farmers was observed in the third quadrant for both the districts, indicating that low priority should be given as it has low importance and satisfaction. The frequency of forecast was found to be in the third quadrant in Kurnool while in the fourth quadrant in Anantapur. The results from the third quadrant shows that the concerned authorities/extension agencies are giving undue attention to these attributes. Farmers were quite satisfied with the frequency of forecasts, which was twice a week in Kurnool. During the discussion with the farmers in the Kurnool district, it was found that they did not wish to get a forecast more than twice a week. In Anantapur, though, the frequency of forecast attributes is considered important by farmers, but the performance of these attributes is excessive or exceeds the level of the farmer’s interest. Therefore, the energy and attention of the extension functionaries should be channeled to other attributes of AAS, which can further increase the adoption of even complex recommendations. The study by Nesheim et al. (2017) was on a similar line. These findings present a clear picture regarding the satisfaction of farmers with various attributes of AAS. Results can be of great use for extension and developmental agencies for devising actionable interventions for enhancing and sustaining the adoption of AAS. The Customer Satisfaction Index showed that the farmers were highly satisfied with AAS. The findings are also in line with Dupdal et al. (2020), who reported 85 and 90% satisfaction levels of farmers toward AAS. High satisfaction with Agromet advisories can also be attributed to the fact that the farmers valued Agromet information for the ability to undertake precautionary actions; this also involved low-risk actions in the case of imprecise advice (Nesheim et al., 2017). Constraints in adopting AAS were also studied during the investigation to formulate an overall recommendation package for sustained adoption. Unavailability of input on time and difficulty in understanding the message while implementing were the major constraints faced by the farmers. During the interaction with farmers, they expressed difficulties in comprehending advisory messages when implementing them in real field conditions. Several contributing factors underpin these challenges. First, farmers often lack practical exposure to the recommended technologies, rendering effective application challenging. Furthermore, some of the disseminated information may not consistently align with the specific circumstances encountered by farmers. The conciseness of the messages, dictated by character limitations, further compounds the challenge of comprehension. For example, a significant issue faced by farmers pertains to the application of insecticides, presenting a formidable obstacle. Muema et al. (2018) also opined that climate information should be such that it can be easily understood by smallholder farmers.
The findings of the study are applicable to farmers in similar socio-ecological systems. This research, like any study, has certain limitations. The study relied on a survey method, which has its own informant biases. Due to time and budget constraints, this research was conducted on a smaller scale. To provide policymakers and practitioners with a more comprehensive understanding of the continued adoption of AAS, future research should be conducted in the larger domain.
Based on the findings of the study, the following recommendations are furnished toward increasing the uptake rate and persistence over time of AAS by farmers following Roger’s innovation-decision process.
1. In both districts, farmers’ initial exposure to AAS was primarily through fellow farmers or agricultural officials. This limited dissemination scope stems from the infrequent presence of agriculture extension officers in farmers’ communities. To maximize access to climate information at the local level, it is likely that multiple delivery channels will need to be developed and utilized. The use of mobile phones and other ICT-based communication media can be a faster and more effective mode of innovation dissemination (Knowledge stage).
2. Peer-to-peer communication has proven to be a crucial strategy for disseminating AAS among farmers. Additionally, encouraging smallholder farmers to actively participate in local groups is essential, as these groups serve as primary channels for disseminating relevant information. Extension functionaries can play a pivotal role in enhancing farmers’ literacy and knowledge levels through non-formal and informal educational programs, as well as extension education using the farmer-field schools’ method (Persuasion stage).
3. The analysis of farmer satisfaction with different climate information attributes can inform the development of tailored AAS packages. A notable disconnect was observed between farmers’ perceived importance and satisfaction with various climate information attributes. While forecast frequency was considered important, it was not deemed as useful for decision-making at local scales. To enhance the delivery of climate information, it is recommended to adopt targeted approaches and investment strategies aligned with expressed user needs and preferences, empowering farmers to make informed decisions regarding AAS adoption (Decision stage).
4. The results pertaining to the constraints faced by farmers indicated that they encountered difficulties in comprehending the advisory messages when implementing them under field conditions. Hence, efforts should be directed toward building the capacities of the local extension functionaries to cater to the timely needs of the farmers (Implementation stage).
5. The AAS messages should be developed in a participatory and multi-stakeholder mode so that they meet the expectations and increase the trust of the farmers. Opportunities should be provided to the stakeholders to engage in open dialogs and discuss the intricacies of AAS adoption and implementation in the field condition. This will enhance the credibility, salience, and legitimacy of AAS, thereby increasing its uptake and continuation (confirmation stage).
Weather-based agro advisories have been recognized as a climate-resilient practice having a unique ability to help farmers prepare for uncertain weather conditions. Sustained adoption of AAS has shown economic and social benefits to farmers. Keeping this in mind, the present study aimed to investigate the communication patterns and satisfaction of farmers adopting Agromet Advisory Services (AAS). In addition, communication patterns and satisfaction, as well as various dimensions, including demographic variables, personality, and communication characteristics, were studied to get an overall idea of a farmer’s profile. Findings suggested that the majority of farmers were middle-aged, had small to semi-medium landholdings, and were rainfed. With respect to their personality and communication characteristics, the majority of respondents were in the low category for their ability to cope with uncertainties and risk, interconnectedness with the social system, seeking information about the innovation, and knowledge about the innovation. Communication patterns pointed out extension agencies as their first source of information in Anantapur, while it was fellow farmers in Kurnool. The study further investigated the communication structure in Kurnool, revealing the presence of a sparse network in the village of Yagantipalle and a dense one in Mirapuram and Chirolokuttur. Additionally, the satisfaction assessment showed that, on the whole, farmers expressed satisfaction with AAS. However, attributes such as the reliability of the forecast need further attention, while the frequency of the forecast should not be overemphasized. Organizations should keep working on scientific adequacy, trustworthiness, timeliness, and usefulness, as these attributes will help to maintain the farmer’s satisfaction. Support during the implementation of AAS in field conditions can greatly enhance the continuous adoption of AAS.
Empirical evidence underscores the need for governments, non-governmental organizations, and other stakeholders to collaborate in promoting the continued adoption of agricultural advisory services (AAS). Additionally, there is a pressing need to strengthen the capabilities of extension organizations to effectively address the challenges that impede the widespread adoption of AAS. Furthermore, the mere receipt of AAS messages does not necessarily guarantee their effective implementation at the field level. Therefore, concerted efforts should be directed toward enhancing user satisfaction with AAS attributes while also addressing the underlying issues that hinder its adoption.
The raw data supporting the conclusions of this article will be made available by the authors, without undue reservation.
The studies involving humans were approved by ICAR-CIRDA Institute Review Council. The studies were conducted in accordance with the local legislation and institutional requirements. The ethics committee/institutional review board waived the requirement of written informed consent for participation from the participants or the participants’ legal guardians/next of kin because the farmers were informed orally.
JR: Conceptualization, Data curation, Investigation, Methodology, Writing – original draft, Writing – review & editing. SB: Conceptualization, Writing – review & editing. AB: Investigation, Writing – review & editing. KN: Methodology, Writing – review & editing. KR: Methodology, Supervision, Writing – review & editing. GN: Writing – review & editing, Methodology. Pushpanjali_ Writing – review & editing. JS: Data curation, Writing – review & editing, Conceptualization, Methodology. VG: Data curation, Writing – review & editing. RN: Methodology, Supervision, Writing – review & editing. PKP: Writing – review & editing, Conceptualization, Supervision. MP: Conceptualization, Writing – review & editing. VS: Conceptualization, Supervision, Writing – review & editing.
The author(s) declare that no financial support was received for the research, authorship, and/or publication of this article.
The authors acknowledge the support given by ICAR-CRIDA, Hyderabad, for the completion of the project. The authors also acknowledge the support given by NICRA, AICRPDA, AICRPPAM, KVK, Yagantipalle, and AICRPDA center Anantapuram for providing necessary support during the project.
The authors declare that the research was conducted in the absence of any commercial or financial relationships that could be construed as a potential conflict of interest.
All claims expressed in this article are solely those of the authors and do not necessarily represent those of their affiliated organizations, or those of the publisher, the editors and the reviewers. Any product that may be evaluated in this article, or claim that may be made by its manufacturer, is not guaranteed or endorsed by the publisher.
Abalo, J., Varela, J., and Manzano, V. (2007). Importance values for importance–performance analysis: a formula for spreading out values derived from preference rankings. J. Bus. Res. 60, 115–121. doi: 10.1016/j.jbusres.2006.10.009
Adio, E. O., Abu, Y., Yusuf, S. K., and Nansoh, S. (2016). Use of agricultural information sources and services by farmers for improve productivity in Kwara State. Libr. Philos. Pract. 1456, 1–10. Available at: http://digitalcommons.unl.edu/libphilprac/1456
Albizua, A., Bennett, E. M., Larocque, G., Krause, R. W., and Pascual, U. (2021). Social networks influence farming practices and agrarian sustainability. PLoS One 16:e0244619. doi: 10.1371/journal.pone.0244619
Aldosari, F., Al Shunaifi, M. S., Ullah, M. A., Muddassir, M., and Noor, M. A. (2019). Farmers’ perceptions regarding the use of information and communication technology (ICT) in Khyber Pakhtunkhwa, northern. J. Saudi Soc. Agric. 18, 211–217. doi: 10.1016/j.jssas.2017.05.004
Arpitha, S. N., Naresh, N. T., Munawery, A., Shambhavi, S., and Rajath, H. P. (2022). Utility and impact of Agromet advisory services among farmers in Mandya District. Karnataka. Int. J. Environ. Clim. 12, 168–172. doi: 10.9734/ijecc/2022/v12i930751
Ashraf, S., Khan, G. A., Ali, S., Ahmed, S., and Iftikhar, M. (2015, 2015). Perceived effectiveness of information sources regarding improved practices among citrus growers in Punjab, Pakistan. Pak. J. Agric. Sci. 522, 861–866.
Babu, S. C., Glendenning, C. J., Okyere, K. A., and Govindarajan, S. K. (2012). Farmers’ information needs and search behaviors: case study in Tamil Nadu, India (IFPRI Discussion Paper 01165) International Food Policy Research Institute: Washington, DC, USA, (no. 1007-2016-79468).
Back, K. J. (2012). Impact-range performance analysis and asymmetry analysis for improving quality of Korean food attributes. Int. J. Hosp. Manag. 31, 535–543. doi: 10.1016/j.ijhm.2011.07.013
Borgatti, S. P., Everett, M. G., and Freeman, L. C. (2002). Ucinet for Windows: Software for social network analysis. Harvard, MA: analytic technologies. 6, 12–15.
Brown, K. (2014). Global environmental change I: a social turn for resilience? Prog. Hum. Geogr. 38, 107–117. doi: 10.1177/030913251349883
Buadi, D. K., Anaman, K. A., and Kwarteng, J. A. (2013). Farmers’ perceptions of the quality of extension services provided by non-governmental organisations in two municipalities in the central region of Ghana. Agric. Syst. 120, 20–26. doi: 10.1016/j.agsy.2013.05.002
Bukchin, S., and Kerret, D. (2020). Character strengths and sustainable technology adoption by smallholder farmers. Heliyon. 6. doi: 10.1016/j.heliyon.2020
Carr, E. R., Goble, R., Rosko, H. M., Vaughan, C., and Hansen, J. (2020). Identifying climate information services users and their needs in sub-Saharan Africa: a review and learning agenda. Clim. Dev. 12, 23–41. doi: 10.1080/17565529.2019.1596061
Cash, D. W., Clark, W. C., Alcock, F., Dickson, N. M., Eckley, N., Guston, D. H., et al. (2003). Knowledge systems for sustainable development. Proc Natl Acad Sci U S A PNAS 100, 8086–8091. doi: 10.1073/pnas.1231332100
Chang, C. C. (2013). Improving employment services management using IPA technique. Expert Syst. Appl. 40, 6948–6954. doi: 10.1016/j.eswa.2013.06.027
Chattopadhyay, N., and Chandras, S. (2018). Agrometeorological advisory services for sustainable development in Indian agriculture. Biodivers. Int. J. 2, 13–18. doi: 10.15406/bij.2018.02.00036
Chaubey, D., Prakash, V., Patel, A. B., and Yadav, T. C. (2018). Role of Agro-meteorological advisory services on risk mitigation in agriculture. Int J Pure App Biosci. 6, 27–32.
Craufurd, P. Q., and Balaji, V. (2014). “Using information and communication technologies to disseminate and exchange agriculture-related climate information in the Indo-Gangetic Plains” in CCAFS working paper. CCAFS working paper no. 78 (Copenhagen, Denmark: Agriculture and Food Security (CCAFS))
Daly, M. E., West, J. J., and Yanda, P. Z. (2016). Establishing a baseline for monitoring and evaluating user satisfaction with climate services in Tanzania CICERO, Report 201 Norway: Oslo 201.
de Oca, M., Munguia, O., Pannell, D. J., and Llewellyn, R. (2021). Understanding the adoption of innovations in agriculture: a review of selected conceptual models. Agronomy 11:139. doi: 10.3390/agronomy11010139
Dewi, Y. A., Yulianti, A., Hanifah, V. W., Jamal, E., Sarwani, M., Mardiharini, M., et al. (2022). Farmers’ knowledge and practice regarding good agricultural practices (GAP) on safe pesticide usage in Indonesia. Heliyon. 8;e08708. doi: 10.1016/j.heliyon.2021.e08708
Di Falco, S., Doku, A., and Mahajan, A. (2020). Peer effects and the choice of adaptation strategies. Agric. Econ. 51, 17–30. doi: 10.1111/agec.12538
Dupdal, R., Dhakar, R., Rao, C. A. R., Samuel, J., Raju, B. M. K., Kumar, P. V., et al. (2020). Farmers’ perception and economic impact assessment of agromet advisory services in rainfed regions of Karnataka and Andhra Pradesh. J. Agrometeorol. 22, 258–265. doi: 10.54386/jam.v22i3.187
Elias, A., Nohmi, M., Yasunobu, K., and Ishida, A. (2016). Farmers’ satisfaction with agricultural extension service and its influencing factors: a case study in north West Ethiopia. J. Agric. Sci. Technol. 18, 39–53.
Ferrer, A. J. G., Thanh, L. H., Chuong, P. H., Kiet, N. T., Trang, V. T., Duc, T. C., et al. (2023). Farming household adoption of climate-smart agricultural technologies: evidence from north-Central Vietnam. Asia-Pac. j. reg. sci. 7, 641–663. doi: 10.1007/s41685-023-00296-5
Franks, J. R., and Emery, S. B. (2013). Incentivising collaborative conservation: lessons from existing environmental stewardship scheme options. Land Use Policy 30, 847–862. doi: 10.1016/j.landusepol.2012.06.005
Fry, A., Ryley, T., and Thring, R. (2018). The influence of knowledge and persuasion on the decision to adopt or reject alternative fuel vehicles. Sustain. For. 10:2997. doi: 10.3390/su10092997
Goddard, L., Aitchellouche, Y., Baethgen, W., Dettinger, M., Graham, R., Hayman, P., et al. (2010). Providing seasonal-to-interannual climate information for risk management and decision-making. Procedia Environ. Sci. 1, 81–101. doi: 10.1016/j.proenv.2010.09.007
Haigh, T., Morton, L. W., Lemos, M. C., Knutson, C., Prokopy, L. S., Lo, Y. J., et al. (2015). Agricultural advisors as climate information intermediaries: exploring differences in capacity to communicate climate. Weather Clim Soc. 7, 83–93. doi: 10.1175/WCAS-D-14-00015
Han, M., Wu, J., Wang, Y., and Hong, M. (2018). A model and empirical study on the user’s continuance intention in online China brand communities based on customer-perceived benefits. J. Open Innov.: Technol. Mark. Complex. 4:46. doi: 10.3390/joitmc4040046
Hansen, J. W., Vaughan, C., Kagabo, D. M., Dinku, T., Carr, E. R., Körner, J., et al. (2019). Climate services can support African farmers’ context-specific adaptation needs at scale. Front. Sustain. Food Syst. 3:21. doi: 10.3389/fsufs.2019.00021
Johnson, D., Almaraz, M., Rudnick, J., Parker, L. E., Ostoja, S. M., and Khalsa, S. D. S. (2023). Farmer adoption of climate-smart practices is driven by farm characteristics, information sources, and practice benefits and challenges. Sustain. For. 15:8083. doi: 10.3390/su15108083
Joseph, M., Allbright, D., Stone, G., Sekhon, Y., and Tinson, J. (2005). Importance–performance analysis of UK and US bank customer perceptions of service delivery technologies. Int. J. Bank Mark. 23, 397–413. doi: 10.1108/02652320510612474
Kassem, H. S., Alotaibi, B. A., Muddassir, M., and Herab, A. (2021). Factors influencing farmers’ satisfaction with the quality of agricultural extension services. Eval. Program Plan. 85:101912. doi: 10.1016/j.evalprogplan.2021.101912
Lahnamäki-Kivelä, S. (2022). Coping with uncertainty: exploring the foresight actions’ role in supporting growth-orientation among Finnish dairy farmers. Futures 135:102870. doi: 10.1016/j.futures.2021.102870
Lemos, M. C., and Rood, R. B. (2010). Climate projections and their impact on policy and practice. Wiley Interdiscip. Rev. Clim. Chang. 1, 670–682. doi: 10.1002/wcc.71
Levenburg, N. M., and Magal, S. R. (2005). Applying importance–performance analysis to evaluate e-business strategies among small firms. E-Service Journal. 3, 29–48. doi: 10.2979/esj.2004.3.3.29
Li, P., Chen, Y., Hu, W., Li, X., Yu, Z., and Liu, Y. (2019). Possibilities and requirements for introducing Agri-environment measures in land consolidation projects in China, evidence from ecosystem services and farmers’ attitudes. Sci. Total Environ. 650, 3145–3155. doi: 10.1016/j.scitotenv.2018.10.051
Luo, L., Qin, L., Wang, Y., and Wang, Q. (2016). Environmentally-friendly agricultural practices and their acceptance by smallholder farmers in China—a case study in Xinxiang County. Henan Province. Sci. Total Environ. 571, 737–743. doi: 10.1016/j.scitotenv.2016.07.045
Maini, P., and Rathore, L. S. (2011). Economic impact assessment of the agrometeorological advisory Service of India. Curr. Sci., 101, 1296–1310.
Marchiori, D., and Franco, M. (2020). Knowledge transfer in the context of inter-organizational networks: foundations and intellectual structures. J. Innov. Knowl. 5, 130–139. doi: 10.1016/j.jik.2019.02.001
Martilla, J. A., and James, J. C. (1977). Importance-performance analysis. J. Mark. 41, 77–79. doi: 10.1177/002224297704100112
Massaro, M., Moro, A., Aschauer, E., and Fink, M. (2019). Trust, control and knowledge transfer in small business networks. Rev. Manag. Sci. 13, 267–301. doi: 10.1007/s11846-017-0247-y
Mitra, A. K., Momin, I. M., Rajagopal, E. N., Basu, S., Rajeevan, M. N., and Krishnamurti, T. N. (2013). Gridded daily Indian monsoon rainfall for 14 seasons: merged TRMM and IMD gauge analyzed values. J. Earth Syst. Sci. 122, 1173–1182. doi: 10.1007/s12040-013-0338-3
Mittal, S., and Hariharan, V. K. (2018). Mobile-based climate services impact on farmers risk management ability in India. Clim. Risk Manag. 22, 42–51. doi: 10.1016/j.crm.2018.08.003
Moschitz, H., Roep, D., Brunori, G., and Tisenkopfs, T. (2015). Learning and innovation networks for sustainable agriculture: processes of co-evolution, joint reflection and facilitation. The J. Agric. Educ. Ext. 21, 1–11. doi: 10.1080/1389224X.2014.991111
Muema, E., Mburu, J., Coulibaly, J., and Mutune, J. (2018). Determinants of access and utilisation of seasonal climate information services among smallholder farmers in Makueni County, Kenya. Heliyon 4:e00889. doi: 10.1016/j.heliyon.2018.e00889
Mukasa, A. N. (2018). Technology adoption and risk exposure among smallholder farmers: panel data evidence from Tanzania and Uganda. World Dev. 105, 299–309. doi: 10.1016/j.worlddev.2017.12.006
Mwadzingeni, L., Mugandani, R., and Mafongoya, P. L. (2022). Socio-demographic, institutional and governance factors influencing adaptive capacity of smallholder irrigators in Zimbabwe. PLoS One 17:e0273648. doi: 10.1371/journal.pone.0273648
Ndimbwa, T., Mwantimwa, K., and Ndumbaro, F. (2020). Channels used to deliver agricultural information and knowledge to smallholder farmers. IFLA J. 47, 153–167. doi: 10.1177/0340035220951828
Nesheim, I., Barkved, L., and Bharti, N. (2017). What is the role of agro-met information services in farmer decision-making? Uptake and decision-making context among farmers within three case study villages in Maharashtra. India. Agriculture 7:70. doi: 10.3390/agriculture7080070
O’Neill, M. A., and Palmer, A. (2004). Importance–performance analysis: a useful tool for directing continuous quality improvement in higher education. Qual. Assur. Educ. 12, 39–52. doi: 10.1108/09684880410517423
Ofoegbu, C., and New, M. (2022). Evaluating the effectiveness and efficiency of climate information communication in the African agricultural sector: a systematic analysis of climate services. Agriculture 12:160. doi: 10.3390/agriculture12020160
Oladele, O. I., Gitika, M. P., Ngari, F., Shimeles, A., Mamo, G., Aregawi, F., et al. (2019). Adoption of agro-weather information sources for climate smart agriculture among farmers in Embu and Ada’a districts of Kenya and Ethiopia. Inf. Dev. 35, 639–654. doi: 10.1177/0266666918779639
Page, G., and Bellotti, B. (2015). Farmers value on-farm ecosystem services as important, but what are the impediments to participation in PES schemes? Sci. Total Environ. 515, 12–19. doi: 10.1016/j.scitotenv.2015.02.029
Pagliacci, F., Defrancesco, E., Mozzato, D., Bortolini, L., Pezzuolo, A., Pirotti, F., et al. (2020). Drivers of farmers’ adoption and continuation of climate-smart agricultural practices. A study from northeastern Italy. Sci. Total Environ. 710:136345. doi: 10.1016/j.scitotenv.2019.136345
Patel, N., Dixit, A. K., and Singh, S. R. K. (2020). Effectiveness of WhatsApp messages regarding improved agricultural production technology. Ind J. Ext. Educ. 56, 54–58.
Price, J. C., and Leviston, Z. (2014). Predicting pro-environmental agricultural practices: the social, psychological and contextual influences on land management. J. Rural. Stud. 34, 65–78. doi: 10.1016/j.jrurstud.2013.10.001
Pulwarty, R. S., and Sivakumar, M. V. (2014). Information systems in a changing climate: early warnings and drought risk management. Weather Clim. Extrem. 3, 14–21. doi: 10.1016/j.wace.2014.03.005
Ragasa, C., and Mazunda, J. (2018). The impact of agricultural extension services in the context of a heavily subsidized input system: The case of Malawi. World Dev. 105, 25–47. doi: 10.1016/j.worlddev.2017.12.004
Rahman, T., Ara, S., and Khan, N. A. (2020). Agro-information service and information-seeking behaviour of small-scale farmers in rural Bangladesh. Asia Pac J Rur Dev. 30, 175–194. doi: 10.1177/1018529120977259
Ramachandrappa, B. K., Thimmegowda, M. N., Krishnamurthy, R., Srikanth Babu, P. N., Savitha, M. S., Srinivasarao, C., et al. (2018). Usefulness and impact of Agromet advisory services in eastern dry zone of Karnataka. Indian J. Dryland Agric. Res. Dev. 33, 32–36. doi: 10.5958/2231-6701.2018.00005.2
Ramaraj, A. P., Rao, K. P. C., Kumar, G. K., Ugalechumi, K., Sujatha, P., Rao, S. A., et al. (2023). Delivering context specific, climate informed agro-advisories at scale: a case study of iSAT, an ICT linked platform piloted with rainfed groundnut farmers in a semi-arid environment. Clim. Serv. 31:100403. doi: 10.1016/j.cliser.2023.100403
Rama Rao, C. A., Raju, B. M. K., Islam, A., Subba Rao, A. V. M., Rao, K. V., Ravindra Chary, G., et al. (2019). Risk and Vulnerability Assessment of Indian Agriculture to Climate Change, ICAR-Central Research Institute for Dryland Agriculture, Hyderabad, 124.
Rampersad, G., Troshani, I., and Plewa, C. (2012). IOS adoption in innovation networks: a case study. Ind. Manag. Data Syst. 112, 1366–1382. doi: 10.1108/02635571211278974
Rohit, J., Nirmala, G., Beevi, A., Shankar, K. R., Nagasree, K., Pankaj, P. K., et al. (2021). Structural analysis of social network among farmers for information acquisition in rainfed areas: a study on farmers’ information acquisition dynamics. Ind J. Ext. Educ. 57, 1–6. doi: 10.5958/2454-552X.2021.00010.4
Scales, T. L., Streeter, C. L., and Cooper, H. S. (Eds.). (2013). Rural social work: Building and sustaining community capacity. US: John Wiley and Sons.
Shah, S. H., Angeles, L. C., and Harris, L. M. (2017). Worlding the intangibility of resilience: the case of rice farmers and water-related risk in the Philippines. World Dev. 98, 400–412. doi: 10.1016/j.worlddev.2017.05.004
Sutherland, L. A., and Marchand, F. (2021). On-farm demonstration: enabling peer-to-peer learning. J. Agric. Educ. Ext. 27, 573–590. doi: 10.1080/1389224X.2021.1959716
Tall, A., Hansen, J., Jay, A., Campbell, B., Kinyangi, J., Aggarwal, P. K., et al. (2014). “Scaling up climate services for farmers: Mission possible. Learning from good practice in Africa and South Asia; CCAFS report no. 13” in CGIAR research program on climate change (Copenhagen, Denmark: Agriculture and Food Security (CCAFS)), 44.
Tang, S., and Dessai, S. (2012). Usable science? The U.K. climate projections 2009 and decision support for adaptation planning. Weather Clim. Soc. 4, 300–313. doi: 10.1175/WCAS-D-12-00028.1
Tran, N. L. D., Rañola, R. F. Jr., Ole Sander, B., Reiner, W., Nguyen, D. T., and Nong, N. K. N. (2020). Determinants of adoption of climate-smart agriculture technologies in rice production in Vietnam. Int. J. Clim. Chang. Strateg. Manag. 12, 238–256. doi: 10.1108/IJCCSM-01-2019-0003
Valujeva, K., Freed, E. K., Nipers, A., Jauhiainen, J., and Schulte, R. P. (2023). Pathways for governance opportunities: social network analysis to create targeted and effective policies for agricultural and environmental development. J. Environ. Manag. 325:116563. doi: 10.1016/j.jenvman.2022.116563
van Oudenhoven, A. P., Schröter, M., Drakou, E. G., Geijzendorffer, I. R., Jacobs, S., van Bodegom, P. M., et al. (2018). Key criteria for developing ecosystem service indicators to inform decision making. Ecol. Indic. 95, 417–426. doi: 10.1016/j.ecolind.2018.06.020
Van Ryzin, G. G., and Immerwahr, S. (2007). Research note: importance performance analysis of citizen satisfaction surveys. Public Adm. 85, 215–226. doi: 10.1111/j.1467-9299.2007.00641.x
Villamayor-Tomas, S. (2018). Disturbance features, coordination and cooperation: an institutional economics analysis of adaptations in the Spanish irrigation sector. J. Inst. Theor. Econ. 14, 501–526. doi: 10.1017/S1744137417000285
Wale, E., and Mkuna, E. (2023). Smallholder satisfaction with the quality of agricultural information, and their preferences among the sources: empirical evidence from KwaZulu-Natal. South Africa. J. Agric. Food Res. 14:100715. doi: 10.1016/j.jafr.2023.100715
Warner, L. A., Chaudhary, A. K., and Lamm, A. J. (2016). Using importance-performance analysis to guide extension needs assessment. J. Ext. 54:21. doi: 10.34068/joe.54.06.21
Wasserman, S., and Faust, K. (1994). Social network analysis: Methods and applications. Cambridge: Cambridge University Press.
Webster, P. J., Jian, J., Hopson, T. M., Hoyos, C. D., Agudelo, P. A., Chang, H. R., et al. (2010). Extended-range probabilistic forecasts of Ganges and Brahmaputra floods in Bangladesh. Bull. Am. Meteorol. Soc. 91, 1493–1514. doi: 10.1175/2010BAMS2911.1
Wulansari, D. R., Sutopo, W., and Hisjam, M. (2018). “The use of importance and performance analysis (IPA) to evaluate effectiveness of the forward auction market agro commodities: a case study” in AIP conference proceedings, vol. 1931 (Indonesia: AIP Publishing LLC), 030019.
Yaqub, R. M. S., and Halim, F. (2018). Revisiting customer loyalty in telecom sector: insights from Oliver four-stages loyalty model and principles of reciprocity. J Mark Manage Cons Behav. 2, 16–30.
Yi, J., Ryan, C., and Wang, D. (2021). China’s village tourism committees: a social network analysis. J. Travel Res. 60, 117–132. doi: 10.1177/0047287519892324
Zhang, P., Ye, Q., and Yu, Y. (2021). Research on farmers’ satisfaction with ecological restoration performance in coal mining areas based on fuzzy comprehensive evaluation. Glob. Ecol. Conserv. 32:e01934. doi: 10.1016/j.gecco.2021.e01934
Keywords: adoption, Agromet advisories services, communication network, credibility, importance performance analysis, satisfaction
Citation: Rohit J, Bal SK, Anshida Beevi CN, Nagasree K, Ravi Shankar K, Nirmala G, Pushpanjali, Samuel J, Veni VG, Nagarjuna Kumar R, Pankaj PK, Prabhakar M and Singh VK (2024) Exploring farmers’ communication pattern and satisfaction regarding the adoption of Agromet advisory services in semi-arid regions of southern India. Front. Sustain. Food Syst. 7:1284880. doi: 10.3389/fsufs.2023.1284880
Received: 13 September 2023; Accepted: 20 November 2023;
Published: 04 January 2024.
Edited by:
Shahrina Md Nordin, Universiti Teknologi Petronas, MalaysiaReviewed by:
Ding Hooi Ting, University of Technology Petronas, MalaysiaCopyright © 2024 Rohit, Bal, Anshida Beevi, Nagasree, Ravi Shankar, Nirmala, Pushpanjali, Samuel, Veni, Nagarjuna Kumar, Pankaj, Prabhakar and Singh. This is an open-access article distributed under the terms of the Creative Commons Attribution License (CC BY). The use, distribution or reproduction in other forums is permitted, provided the original author(s) and the copyright owner(s) are credited and that the original publication in this journal is cited, in accordance with accepted academic practice. No use, distribution or reproduction is permitted which does not comply with these terms.
*Correspondence: Jagriti Rohit, amFncy5yb2hpdEBnbWFpbC5jb20=
Disclaimer: All claims expressed in this article are solely those of the authors and do not necessarily represent those of their affiliated organizations, or those of the publisher, the editors and the reviewers. Any product that may be evaluated in this article or claim that may be made by its manufacturer is not guaranteed or endorsed by the publisher.
Research integrity at Frontiers
Learn more about the work of our research integrity team to safeguard the quality of each article we publish.