- 1College of Animal Science and Technology, Yangzhou University, Yangzhou, Jiangsu, China
- 2Jiangsu Key Laboratory of Crop Genetics and Physiology, Agricultural College of Yangzhou University, Yangzhou, Jiangsu, China
- 3College of Landscape Architecture, Fujian Agriculture and Forestry University, Fuzhou, Fujian, China
- 4College of Horticulture and Landscape Architecture, Yangzhou University, Yangzhou, Jiangsu, China
Introduction: The evaluation on the trade-off/synergy relationship of urban cultivated land-use function conflicts (CLUFCs) for improving the sustainability of cultivated land ecosystem is one of the solutions to coordinate the contradiction between land health and economic development and alleviate food crises, and achieve “zero hunger” in the United Nations Sustainable Development Goals (SDGs).
Methods: In this study, Yancheng City was taken as the research object, and the cultivated land ecological function (CLEF) was evaluated by the morphological spatial pattern analysis-minimum cumulative resistance (MSPA-MCR) model from the perspective of multi-objective coordinated development of cultivated land, and cultivated land productive function (CLPF) was evaluated by net primary productivity (NPP). In addition, combined with local indications of spatial association (LISA) analysis, the spatial trade-off/synergy relationship between CLPF and CLEF was quantified and analyzed. The spatial principal co-coordinates-redundancy analysis (SPCoA-RDA) was used to explore the influencing factors and mechanisms of CLUFCs.
Results: The results show that there are obvious spatial heterogeneity and aggregation distribution characteristics of CLUFCs in Yancheng City. Through SPCoA-RDA, it is found that the spatial differentiation of CLUFCs is the result of the combination of internal (population density, road network, and water network) and external (spatial spillover effect) driving factors.
Discussion: Based on these results, the study area was divided into eight types of cultivated land suitability zones under four aggregation relationships of CLUFCs. Comprehensively considering production, CLUFCs were evaluated by socio-economic and geo-spatial statistical dates, and strategies for guiding the coordinated development and sustainable management of urban cultivated land had been put forward. This study can provide a theoretical framework and certain feasible suggestions for the sustainable development and the transformation of cultivated land system in agriculture cities.
1 Introduction
Food system is the basis of human survival, and is closely related to life, production and ecology. Achieving “zero hunger “is currently one of the top priorities of the United Nations (UN) Sustainable Development Goals (SDGs). The Committee on World Food Security introduced sustainability into food systems in 2014, and the sustainable transformation of food systems has become an important foundation for achieving the UN 2030 SDGs (Chaudhary et al., 2018). In 2022, the 27th United Nations Climate Change Conference launched the “Food and Agriculture for Sustainable Transformation Initiative” to promote the sustainable transformation of agri-food systems. China is also one of the countries in the world facing the challenge of food system problems. It is an important issue to coordinate the human-land coupling relationship of the cultivated land use system and divide the cultivated land use regulation zone according to the suitability, which is in line with China’s national conditions and promotes the sustainable land use (Dengerink et al., 2021).
Cultivated land, a typical semi-artificial and semi-natural composite ecosystem, is affected by human activities and natural ecology for a long time, which has dual functions of production and ecology (Bai et al., 2019). However, the excessive pursuit of high grain yield in traditional intensive production cannot meet the requirements of green agricultural development in the new era. Currently, one third of soil in the world has poor conditions, and single production functions and ecological pressures, such as soil degradation, reduced fertility, production overload, soil erosion, and unsustainable management methods (Ibrahim et al., 2020). Therefore, it is necessary to shift the focus and use model of land function from soil fertility and yield improvement, so as to maintain soil health of cultivated land and agricultural sustainability.
There have been quite a number of reports focusing on the functional use of urban land and its relevance to sustainable development (Jia et al., 2022; Zhao et al., 2022). Focusing on the potential land-use function conflicts is a new direction to coordinate the contradiction between land health and social and economic development (Cao J. H. et al., 2022; Zhao S. et al., 2023). However, previous studies on the conflict of land use function mainly focus on urban land and economically developed areas, and the research on cultivated land is still incomplete (Yang X. D. et al., 2022). The study of cultivated land-use function conflicts (CLUFCs), which aims to address the current situation of single production function utilization of cultivated land, and achieve its sustainable management and multi-objective synergistic development, has attracted the attention of researchers and decision-makers (Zhang Y. et al., 2019).
In the context of ensuring global food security and achieving sustainable ecological development goals, researchers from various countries have made tremendous efforts to classify, quantify, and enhance CLUFCs systems, and have sought different solutions (Jin et al., 2019; Longato et al., 2019). However, how to achieve the coordination between multiple functions of cultivated land and ecological sustainability remains challenging.
At present, studies on CLUFCs are mostly concentrated in two aspects. One is the theoretical framework, such as the construction of index system, multi-functional evaluation system and pattern recognition of cultivated land (Li X. et al., 2022); the other is the practical aspect, such as the dynamic change of cultivated land in time and space, driving mechanism, multifunctional evaluation, measurement and zoning planning (He et al., 2019; Yu et al., 2019; Wang et al., 2022). In the visualization of CLUFCs, it can be divided into administrative regions [such as city (Cai et al., 2022), district (Hu J. et al., 2018) and county (Peng et al., 2015)], and functional scales [like river basin (Zhang et al., 2022), region (Wang et al., 2023) and grid]. Despite some study results, researches on the construction of CLUFCs evaluation index system are not perfect nowadays. Combined with the related research on monitoring the spatial differentiation of CLUFCs from the macroscopic perspective of city scale by 3S technology, it is still essential to develop a comprehensive evaluation method that can coordinate the CLUFCs. This may be related to the complexity of CLUFCs, the lack of functional division and evaluation system, and the difficulty in quantifying and coupling functions (Li Y. et al., 2023). Nowadays, there is no consensus on the division of functional system of cultivated land. Generally, the common practice is to divide it into three sub-functions of production, society and ecology (Peng et al., 2015; Chen et al., 2020; Zou et al., 2021; Li C. et al., 2022). In the quantification and coupling methods of CLUFCs, researchers have also explored the evaluation methods, such as the entire-array-polygon indicator method (Su et al., 2019), the coupling coordination model (Tang et al., 2023) and spatial auto-correlation (Meng et al., 2022). Among them, based on the spatial dependence theory (Merk and Otto, 2022), a bivariate spatial auto-correlation analysis which can reflect the conflict and coordination between multiple functions of cultivated land is developed (Cai et al., 2022; Wang et al., 2023). On this basis, we can coordinate grain production, spatial services and ecological balance, and tap the potential of cultivated land with the development direction of regional leading functions. Although the above-mentioned advantages, it limits the single analysis to only two functions. Therefore, there is an urgent need for an evaluation method that can incorporate multiple influencing factors and couple multiple cultivated land functions.
Ecological security assessment provides a new research perspective. It can reflect the ability of ecosystems to maintain their own stability and support sustainable social development, and focuses on social development and human well-being (Jogo and Hassan, 2010; An et al., 2020). Based on the ArcGIS platform, multiple analysis methods are adopted, such as morphological spatial pattern analysis (MSPA), the spatial principal components analysis (SPCA) and redundancy analysis (RDA; De Montis et al., 2019; Li S. et al., 2023). Moreover, various models were selected, such as the InVEST model, the minimum cumulative resistance (MCR) model, and circuit theory (Jongman et al., 2004). It is indicated that ecological security assessment is essentially a visual analysis of spatial structure of habitat patches (Zhang et al., 2021). Therefore, natural and social indicators can be feasible to freely choose based on research purposes (Zhang et al., 2022), a multi-function evaluation system of cultivated land can be established, and potential conflicts between functions can be analyzed (Tang et al., 2023).
With the development of semi-urbanization in China, the ecological function and stability of urban ecosystem, especially the cultivated land ecosystem, are also weakening (Han et al., 2022). This study takes Yancheng City as the study area and focuses on solving the following problems: (1) to quantify CLUFCs at the grid scale, and analyze its spatial differentiation characteristics; (2) to analyze the spatial trade-off/synergy aggregation relationship of CLUFCs, and reveal the influencing factors and mechanisms of different aggregation relationships; (3) to propose a multifunctional spatial integration development model for cultivated land based on dominant functions, and establish a comprehensive utilization development plan for CLUFCs. The aim of this study is to guide the quality protection and multi-functional collaborative development of urban cultivated land based on the spatial differentiation of the functional aggregation relationship of cultivated land through the combination of interdisciplinary models and analytical methods. This study has important theoretical and practical significance on stabilize the cultivated land ecosystem, expand and enhance the value of cultivated land, and establish a comprehensive utilization guarantee mechanism.
2 Study area and data sources
2.1 Study area
The research area with a total area of 6,340 km2 is located in Yancheng City, China (Figure 1). Yancheng City is a typical coastal city, and the ocean plays a very obvious role in regulating the urban climate. The annual average temperature, average precipitation and total sunshine hours were 14.1°C, 1,309 mm and 2,280 h, respectively. The entire area of Yancheng City is characterized by plain landforms, and the altitude of its most areas is no more than 5 m.
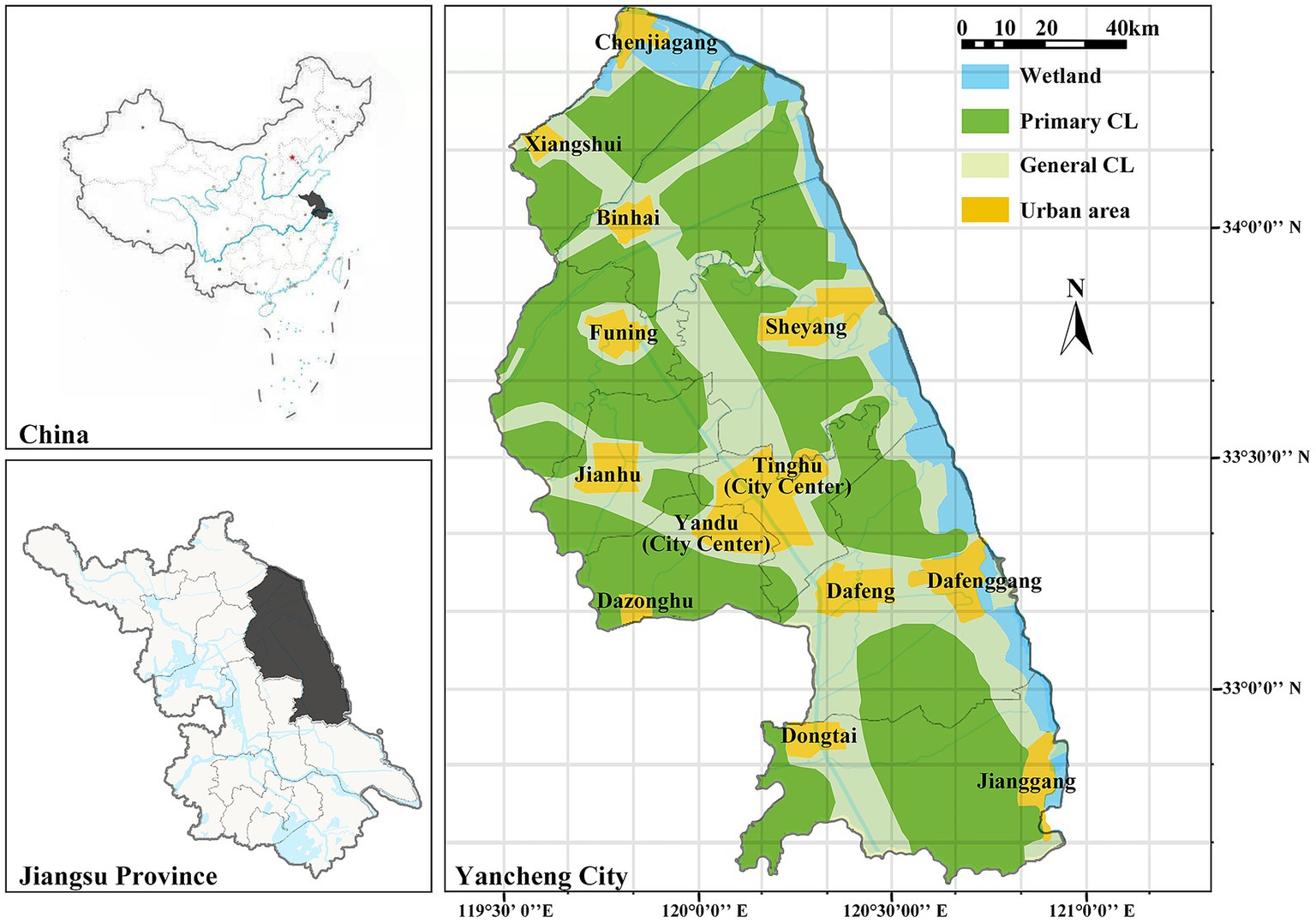
Figure 1. Location and urban protection zoning of the study area. The subgraph at the upper-left shows the map of China ‘s administrative divisions and the geographical location of Jiangsu Province. The lower-left corner shows the administrative map of Jiangsu Province and the geographical location of Yancheng City. And the right figure shows the urban main functional zoning. CL, cultivated land.
With the rapid development of agricultural and industrial activities, Yancheng City becomes a strategic overlapping area of China, including the integration of Yangtze River Delta, the ecological economic belt of Huaihe River, and high-quality coastal development. Its cultivated land area accounts for 76% of the city’s total area, and the main crops are wheat, rice, and corn. With flat terrain and dense water network, Yancheng City accounts for 0.66% of China’s cultivated land and 1.1% of its grain production, and nearly 1/5 of Jiangsu Province’s. Known as the “Jiangsu grain warehouse,” it is an important grain-producing area in China (Yancheng City, Bureau of Statistics, 2021). According to the planning zoning of cultivated land in the Overall Urban Planning of Yancheng City from 2013 to 2030 (Yancheng People’s Government, 2014), cultivated land was divided into primary cultivated land (CL) and general CL (Figure 1). Among them, the primary CL area is a large continuous cultivated land far away from the urban area, mainly some large farms and high-quality cultivated lands; general CL are is scattered farmland belonging to individual farmers interspersed between the urban area and the primary CL area.
2.2 Data sources and preprocessing
In Supplementary Table S1, multiple datasets for the study area in 2020 (accessed on 29 October 2022) were listed, including socio-economic statistical data and geo-spatial information used for analysis. The spatial resolution of land use and land cover (LULC) data was 10 × 10 m. According to its ecological foundation and research objectives, land-use types of the study area were then divided into five types (vegetation, wetland, cultivated land, water area, and artificial surface). All data were than reclassed to the same resolution (10 m) as LULC.
3 Methods
As shown in Figure 2, CLUFCs were evaluated by using multi-source remote sensing data to achieve the goal of multi-objective synergistic development of cultivated land in Yancheng City. In this study, the following three steps are included:
1. To build a CLUFCs evaluation system for Yancheng City. CLPF was represented by net primary productivity (NPP); morphological spatial pattern analysis-minimum cumulative resistance (MSPA-MCR) model was introduced to construct a cultivated land ecological security evaluation system, and geospatial and socio-economic indicators related to cultivated land were integrated into a CLEF. Furthermore, gradation evaluation of CLUFCs of Yancheng City’s cultivated land in 2020 was conduct.
2. To analyze the CLUFCs spatial distribution. The natural breakpoint method was used to grade CLUFCs, and Getis-Ord Gi* analysis was adopted to analyze hot and cold spots of function aggregation and evaluate its spatial heterogeneity. The local indications of spatial association (LISA) ware utilized to visualize the trade-off/synergy function aggregation area of cultivated land.
3. To analyze the influencing factors and mechanisms of CLUFCs. Spatial principal co-ordinates-redundancy analysis (SPCoA-RDA) was used to analyze influencing factors and mechanisms of four functional aggregation areas qualitatively and quantitatively. A multi-functional spatial integration development model of cultivated land based on dominant functional differences was than proposed, and a comprehensive utilization development plan was finally established.
3.1 Evaluation of CLEF
3.1.1 Description of MSPA-MCR model
The morphological spatial pattern analysis-minimum cumulative resistance (MSPA-MCR) model is a classic ecological security evaluation model (Knaapen et al., 1992; Fu et al., 2020). According to various research purposes, it can freely combine multiple indicators, such as geospatial, economic, cultural, and tourism indicators. At present, the mainstream research paradigm of “eco-source identification - minimum accumulative resistance surface construction” has been formed (Closset-Kopp et al., 2016). With comprehensive analysis indicators, wide application, high industry recognition and high universality, it is a widely applied model in ecological security assessment, species protection and landscape pattern research (Xu et al., 2021).
3.1.2 Identification of eco-sources
On the Guidos Toolbox 2.8 platform, through spatial morphological analyses (such as corrosion, expansion, and opening/closing operations), binary raster images were reinterpreted into seven mutually exclusive landscape types, including core, bridge, loop, branch, islet, edge, and perforation (Zhang P. et al., 2019). The definitions and ecological implications of the seven landscape types in MSPA were described in detail in Supplementary Table S2. Areas with less human interference, diverse ecological functions, and abundant species (vegetation, wetlands, and water) were regarded as the “foreground”; areas with high human interference and single ecological function (cultivated land and artificial surface) were considered as “background” to reclass landscape pattern and spatial characteristics of LULC data (Soille and Vogt, 2009). Cultivated land belongs to a typical semi-artificial and semi-natural ecosystem, which is highly affected by human activities, and its basic function is to provide food production. Compared with those of “foreground,” ecological functions of cultivated land, such as climate regulation, maintaining biodiversity, and carbon sequestration, are weaker (Closset-Kopp et al., 2016). Therefore, cultivated land was not considered as an eco-source in this study.
The realization of many landscape ecology processes and functions depends on the connectivity of landscape structure (Wickham et al., 2010). Probability of connectivity (PC) can characterize the overall landscape connectivity at the landscape level, and quantify the possibility of direct diffusion of ecological flow between two patches. When a patch is removed, the overall landscape structure will also undergo corresponding changes. Delta of PC (dPC) is used to simulate the importance of the removed patch (Pascual-Hortal and Saura, 2006). The higher the PC value, the better the connectivity between patches; the higher the dPC value, the more important the patch is. The calculation formulas are as following (Saura and Pascual-Hortal, 2007):
Where, n is the total number of patches; ai and aj are the area of patches i and j respectively; is the maximum probability value of species diffusion between patches i and j; AL is the total area of the patches; and PCremove is the calculation result of connectivity after removing a particular element.
In this study, PC and dPC indicators were used as important references for quantifying the importance of patches and selecting eco-sources in Conefor2.6 software (accessed on 15 December 2022).1 Based on relevant researches and the actual situation of study area, distance threshold, connection probability, area threshold and dPC threshold were set to be 1,000, 0.5, 3 km2 and 0.26, respectively, which were used to screen the cores classified by the MSPA. According to ecological functions and dominant patches, eco-sources selected were divided into three categories (vegetation, wetland, and water).
3.1.3 Construction and correction of ecological security level
Based on the MCR model, an ecological security evaluation system was built for Yancheng City. According to previous literature researches (Yang C. et al., 2022) and the actual situation of study area, ecological security evaluation factors were selected. Geo-spatial factors [digital elevation model (DEM), Slope, LULC, normalized difference vegetation index (NDVI), and MSPA data] were chosen to represent the natural ecological foundation of this region, and socio-economic factors (population density, distance from water network, and distance from road network data), were selected to represent the degree of human interferences. The weight of each factor was determined by the analytic hierarchy process (AHP) method (Supplementary Table S3; Peng et al., 2018), and an evaluation scale (Supplementary Table S4) was thereby constructed. The calculation formula is as following:
Where, Dij is the distance from patch i to j; Ri is the resistance of material flow between habitat patches; and f is the positive correlation between MCR and ecological process.
3.2 Judgement of CLUFCs area
Spatial auto-correlation analysis (Bivariant Moran’s I analysis) can represent the degree of correlation and spatial aggregation between the attribute values of a spatial patch and adjacent patches (Anselin, 2010), and explore the spatial relationships between multiple functions. In this study, CLEF and CLPF were divided into fishing nets by 500 m (Li X. et al., 2022), and bivariant global and local Moran’s I analysis was adopted to analyze the internal spatial trade-off/synergy relationship between the two by GeoDa (1.20.0.36) software (Wang et al., 2023). The global Moran’s I represents a global positive or negative correlation between CLEF and CLPF, and the local Moran’s I indicates the aggregation of synergy or trade-off relationship between the two bariables: high CLPF – high CLEF (H–H) or low CLPF – low CLEF (L–L) aggregation, that is, two variables are both high or low values in one fishing net; high CLPF– low CLEF (H–L) or low CLPF – high CLEF (L–H) aggregation, that means, two variables have a high and a low value in one fishing net. On this basis, four aggregation patterns are thus formed, and the aggregation and differentiation characteristics of spatial variables are visualized by the LISA distribution map (Longato et al., 2019).
3.3 Identification of influencing factors of CLUFCs
3.3.1 SPCoA
The spatial principal co-coordinates analysis (SPCoA), developed from the principal co-ordinates analysis (PCoA) and the spatial principal components analysis (SPCA), is a combination of statistical principles and GIS, which reflects the differences between multiple influencing factors on a two-dimensional coordinate map and visually displays the difference distribution between sample points (Ibrahim et al., 2020). It is an effective method to judge the significance of differences among grouped samples (Cao J. S. et al., 2022). Both PCoA and SPCA are developed from Principal Component Analysis (PCA). PCA is a simplified analysis technique for dimensionality reduction of complex data, based on Euclidean distance calculation. PCoA is a visualization method on the basis of PCA to study the similarity or difference of data developed. Based on the Bray-Curtis distance algorithm, the potential principal components in the influencing factors of samples are obtained by unconstrained data dimensionality reduction (Stegen et al., 2012).
3.3.2 RDA
Redundancy analysis (RDA) can visually display the positive or negative correlations and significance degrees among the sample groups, multiple independent and dependent variables (Chen et al., 2018). RDA is a sorting method based on the correspondence analysis, which combines correspondence analysis with multiple regression analysis. Each step of the calculation is regressed with the dependent variable. Different from the ordinary regression methods (OLS, GWR, etc.) which can only analyze the relationship between one dependent and several independent variables (Guo et al., 2021), RDA can show the influence of multiple independent and dependent variables at the same time (Goethem et al., 2018). Both SPCoA and RDA use R statistical software package (version 0.99.446, RStudio, Inc., 2015) to perform operations and draw images.
4 Results
4.1 Evaluation and analysis of CLEF
4.1.1 Evaluation of CLEF
The MSPA method was used to process the LULC data of Yancheng City, and the distribution map of landscape types was obtained. In the binary grid diagram of foreground and background, the foreground area is 1614.63 km2, accounting for only 10.41% of the total area. Foreground elements were divided into seven landscape types (Supplementary Figure S1A), and the proportion of each type of foreground elements in the total area was shown in Supplementary Table S5. Moreover, dPC was used as an important index to screen eco-sources, and 16 eco-sources were obtained finally. Eco-sources were numbered in descending order, and were divided into three categories according to the dominate land-use types with the highest proportion (Supplementary Figure S1B). These 16 eco-sources, including large-scale forests, protected areas, large-scale coastal wetlands, mainstream rivers and eco-tourism areas in the study area, are green eco-areas with important eco-functions in Yancheng City. According to the grading diagram of each evaluation factor (Supplementary Figure S2) and its weight, the image of ecological security grade (Figure 3A) was then obtained.
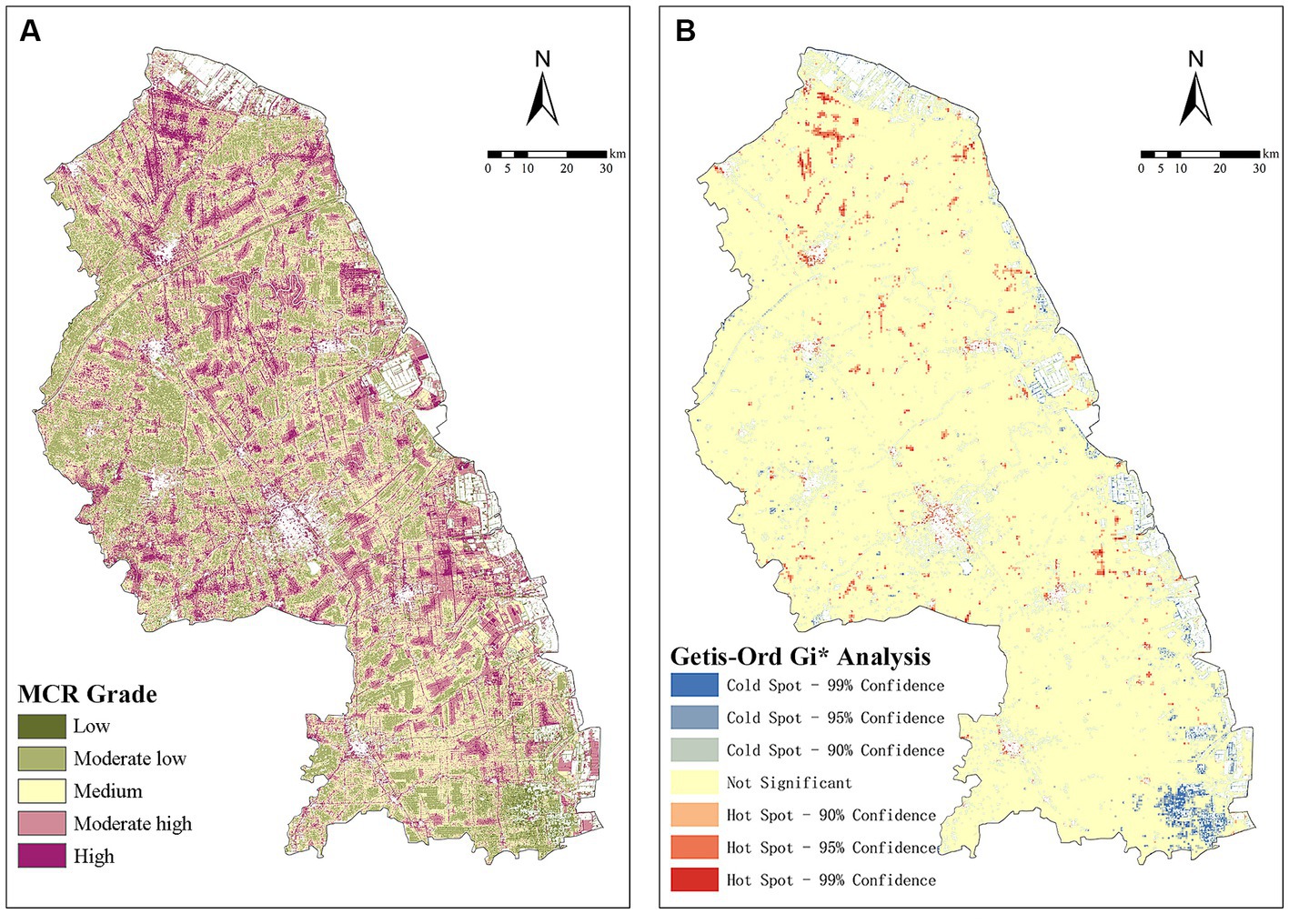
Figure 3. (A) Spatial variations and (B) hot−/cold-spots of cultivated land ecological function (CLEF). MCR, minimum cumulative resistance.
As a typical coastal city, the distribution of eco-sources fully showed the characteristics of ecological spatial pattern of coastal cities. Eco-sources were mainly wetlands and waters, which were unique landscape elements of coastal cities. In addition, they were concentrated in the coastal wetlands in the eastern city and water areas, but sporadically distributed in the western and central regions. Generally speaking, spatial distribution of ecological resources showed obvious spatial imbalance, and Yancheng City had ecological and development needs for ecological security assessment.
4.1.2 Spatial distribution of CLEF
The ecological security level of cultivated land in Yancheng City was evaluated and quantified by the MSPA-MCR model, and displayed according to the natural breakpoint method. The higher the MCR value, the lower the CLEF in one grid, and the more susceptible it is to damage caused by external factors.
The results showed that CLEF in Yancheng City had obvious spatial heterogeneity, of which high CLEF were only accounts for 5.36% of total area, while middle-low and low CLEF areas accounted for 37.87%; and the cultivated land generally had poor risk resistance ability to cope with ecological shocks (Figure 3A). However, the proportion of moderate CLEF area was relatively high (37.61%), making it a key optimization area with certain ecological buffering functions. Meanwhile, the spatial distribution changes of CLEF were closely related to social factors, which were manifested in the city center of Tinghu district, Yandu district, Dafenggang town (a large coastal port in the eastern part of Jiangsu), Chenjiagang town (a chemical concentration area), and other built-up areas. CLEF was relatively lower near built-up areas, but higher in the primary CL area far from the urban area.
In ArcGIS, the Getis-Ord Gi* analysis tool was adopted to analyze the spatial aggregation of hot−/cold-spots, which statistically visualized the spatial aggregation of low/high CLEF values (Wang et al., 2022). Cold-spots in the figure indicated high CLEF value, which were mainly concentrated around eco-sources (wetlands, waters), economically underdeveloped areas with low population density (Sheyang county, Funing county), and areas with high green coverage (Jianggang town). The aggregation of low CLEF value represented by hot-spots showed that the ecological security level of the region was relatively lower, while the ecological quality of cultivated land was poorer (Figure 3B).
4.2 Spatial distribution of CLPF
CLPF with NPP as the evaluation index represented strong spatial heterogeneity. NPP showed a significant geographical gradient pattern of decreasing from coastal to inland areas (Figure 4A). CLEF is affected by natural and social factors, such as DEM, slope, distance from water system, main road system, and population density. However, CLPF is more affected by sunshine, rain, agricultural foundation, soil fertility and other factors. In this study, hot-spots with high CLPF value were distributed in the northern part of Sheyang county, the middle area between Sheyang county and Dafeng county, and the primary CL near Jianggang town (Figure 4B). The main reason may be that Sheyang county and Dafeng county are located in the coastal agricultural zone of Jiangsu Province, which are traditional agricultural counties and commodity grain production bases with multiple large farms concentrated and a good agricultural foundation. Cold-spots with low CLPF value were concentrated in the main urban area, the northern part of Jianhu town, and near Dazonghu town. Compared with CLEF, CLPF showed a more obvious trend of urban–rural transition. Furthermore, the effects of different locations and development planned on CLPF vary. These results are of great significance for the development and ecological utilization of urban cultivated land.
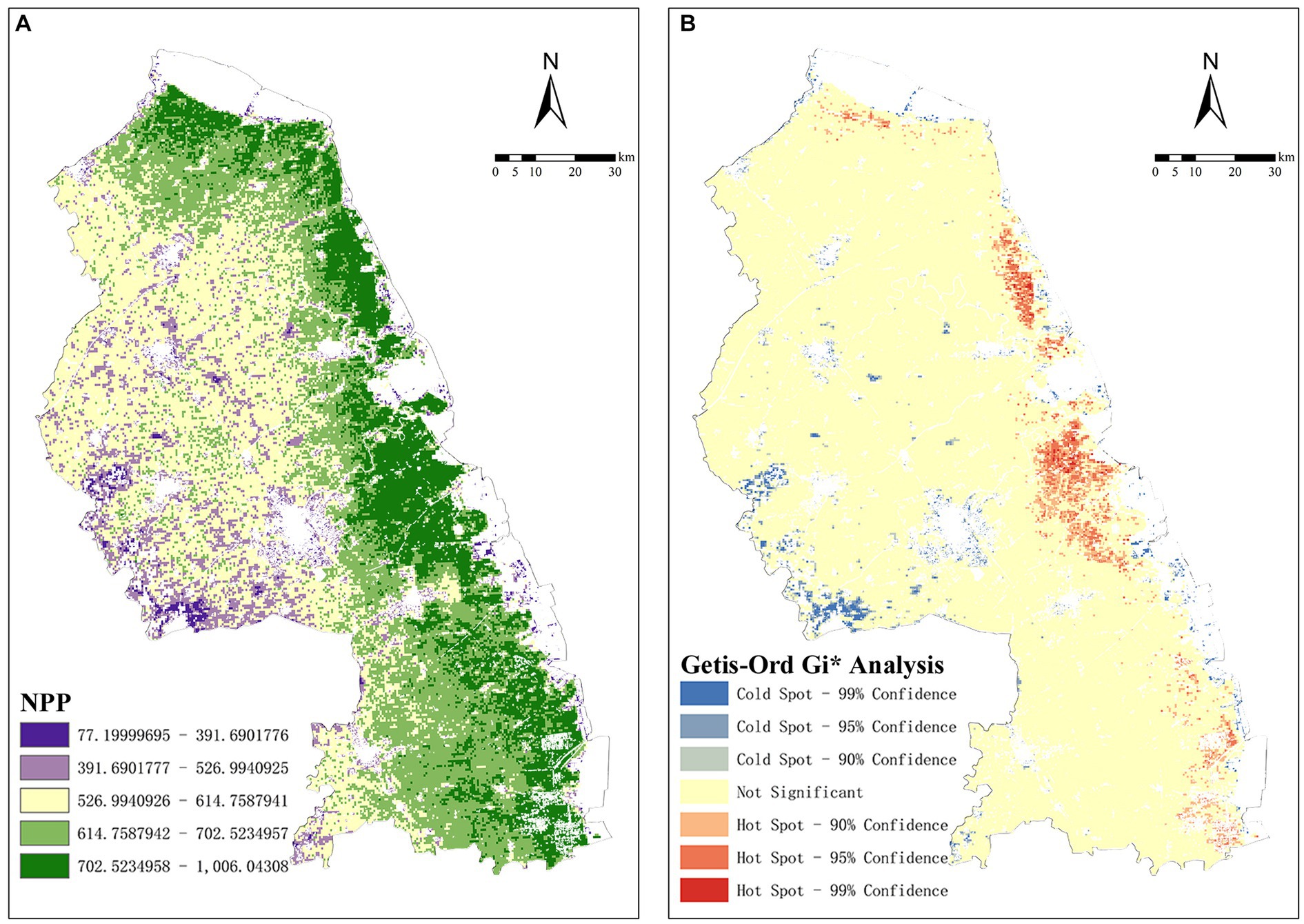
Figure 4. (A) Spatial variations and (B) hot−/cold-spots of cultivated land productive function (CLPF). NPP, net primary productivity.
4.3 Spatial relationship of CLUFCs
In this study, the interaction and dependency relationship of CLPF and CLEF was determined and visualized by LISA analysis (Figure 5A). The results showed that for 32.78% of cultivated land, there was a correlation in the spatial relationship of CLUFCs (p < 0.05; Figures 5B,C). In terms of the overall spatial relationship of CLUFCs, there were differences in the spatial distribution of CLEF and CLPF, with a large degree of dispersion and a negative spatial correlation (Moran’s I = −0.106; Figure 5D).
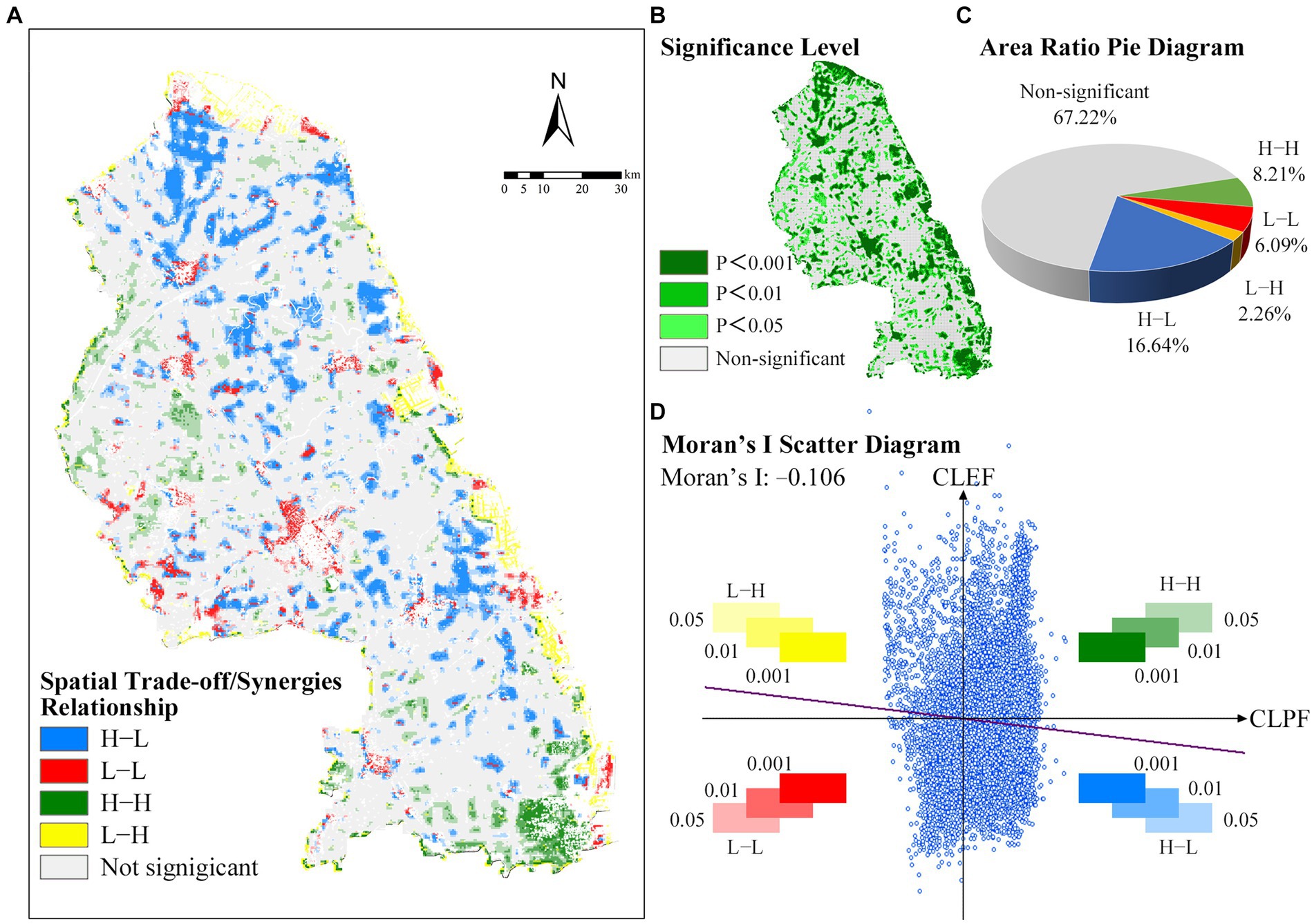
Figure 5. (A) Local indications of spatial association (LISA) analysis. (B) Significance level of LISA analysis. (C) Area ratio pie diagram of four kinds of spatial aggregation relations. (D) Moran’s I scatter diagram of four kinds of spatial aggregation relations. H–H, high CLPF – high CLEF; L–L, low CLPF – low CLEF; H–L, high CLPF – low CLEF; L–H, low CLPF – high CLEF.
On the local spatial relationship of CLUFCs, there were four types of spatial aggregation relationships based on spatial aggregation characteristics (Figure 5A). The total area of trade-off relationship (H–L, L–H) accounted for 18.90% of the study area, which was 1.32 times the total area of synergy relationship (H–H, L–L). The H–H synergy area was mainly distributed in the concentrated cultivated land and farms around Funing county, Jianhu county, Dongtai county and Jianggang town far away from the urban built-up areas. There were two types of L–L synergy zones. One was mainly concentrated around the built-up area, which was highly related to urban expansion; the other was concentrated around coastal protection wetlands, belonging to primitive undeveloped areas. The distribution of trade-off degree showed that H–L trade-off areas were distributed in Binhai county, Sheyang county, Dafeng county and other important farms; L–H trade-off zones were concentrated near rivers and lakes, such as the protected wetlands and the Dazhong Lake ecological zone.
4.4 Influencing mechanism of CLUFCs
4.4.1 Analysis of internal driver factors
The trade-off/synergy relationship between natural and social factors affecting CLUFCs were qualitatively identified by SPCoA and Spearman’s correlation analysis (Figure 6A). The results showed that two functions had trade-off responses to most of the influencing factors related to CLUFCs. Only NDVI shown a positive correlation to CLPF and CLEF; population density, and CO2 concentration had negative correlations to two functions. It is necessary to further quantitatively analyze the influencing degree and mechanism of each factor.
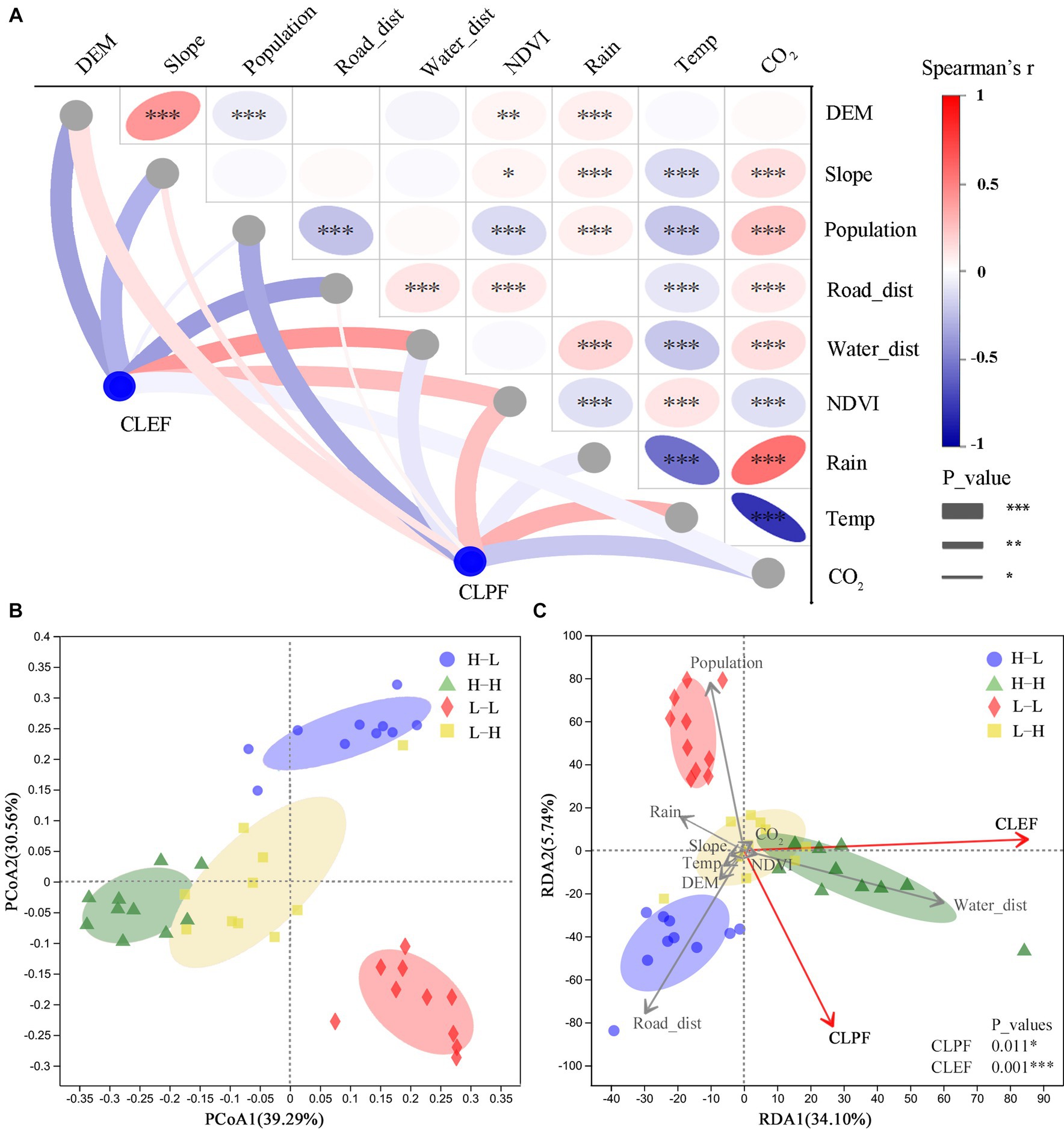
Figure 6. (A) Pairwise comparisons of factors shown with a color gradient representing Spearman’s correlation coefficients. Spearman’s correlation analyses depict the association of CLEF and CLPF with socio-economic and geo-spatial factors. The color gradient represents positive or negative correlation, and the width of each edge matches Spearman’s p values. (B) PCoA of four kinds of spatial aggregation relations based on Bray-Curtis distance. (C) RDA of four kinds of spatial aggregation relations. Socio-economic and geo-spatial indicators were fitted to the ordination plots using a 999-permutation test (p values). The asterisks denote statistical significance: *p < 0.05, **0.001 < p < 0.01, ***p < 0.001. DEM, digital elevation model; NDVI, normalized difference vegetation index.
PCoA analysis was carried out on 10 random sampling points in each of the four spatial aggregation relationship areas obtained by LISA analysis to test the differences of these areas (Ibrahim et al., 2020). As shown in Figure 6B, PCoA_1 and PCoA_2 axes had a total variance interpretation rate of 69.85%, and sample groups had obvious intra-group aggregation relations and inter-group differences (Li S. et al., 2023).
Further, decision curve analysis (DCA) was conducted on four spatial aggregation relation areas. The value of axis-length of DCA_1 axis is 1.871, which indicates that the data distribution conforms to the linear model and the conditions for RDA analysis (Chen et al., 2018). Finally, RDA analysis was carried out to intuitively reflect the relationship between these areas and the influencing factors on the two-dimensional ranking diagram, and to screen the key indicators (Hu W. et al., 2018). The results showed that the influencing factors on CLEF and CLPF were significant (p = 0.011*) and extremely significant (p = 0.001***) respectively. Distance from water network, distance from main road network and population density were the top three key factors that had great influences on CLUFCs (Figure 6C). Among them, H–H aggregation was mainly distributed in the area close to the water network; L–L aggregation was related to high population density; and H–L aggregation was correlated to the main road network nearby.
4.4.2 Spatial spillover effect of multiple land-use patterns
The spatial pattern of CLUFCs is complex, which is affected by various mechanisms and processes. In addition to social factors (population density, distances from major water and road networks), different land-use patterns around cultivated land, namely spatial spillover effects, are also exogenous driving factors (Liu et al., 2021). The spatial spillover effect initially applied in the field of economics refers to the interaction and influence between regions with different levels of economic development due to diffusion and regurgitation (Liang and Li, 2020). At analysis on geographical level, CLUFCs is affected by the spatial externality of adjacent regions, and shows typical spatial dependence and heterogeneity (Tong et al., 2013).
In this study, the coordinated development pattern of two functions of cultivated land showed responses to spatial spillover effect of surrounding land-use types. The process of semi-urbanization had caused significant production and ecological pressures on the cultivated land in urban suburbs (Figure 7A), leading to a large area of L–L aggregation; the drive of construction and policies of coastal wetlands (Figure 7B) was one of the influencing factors for the L–H aggregation of coastal cultivated land.
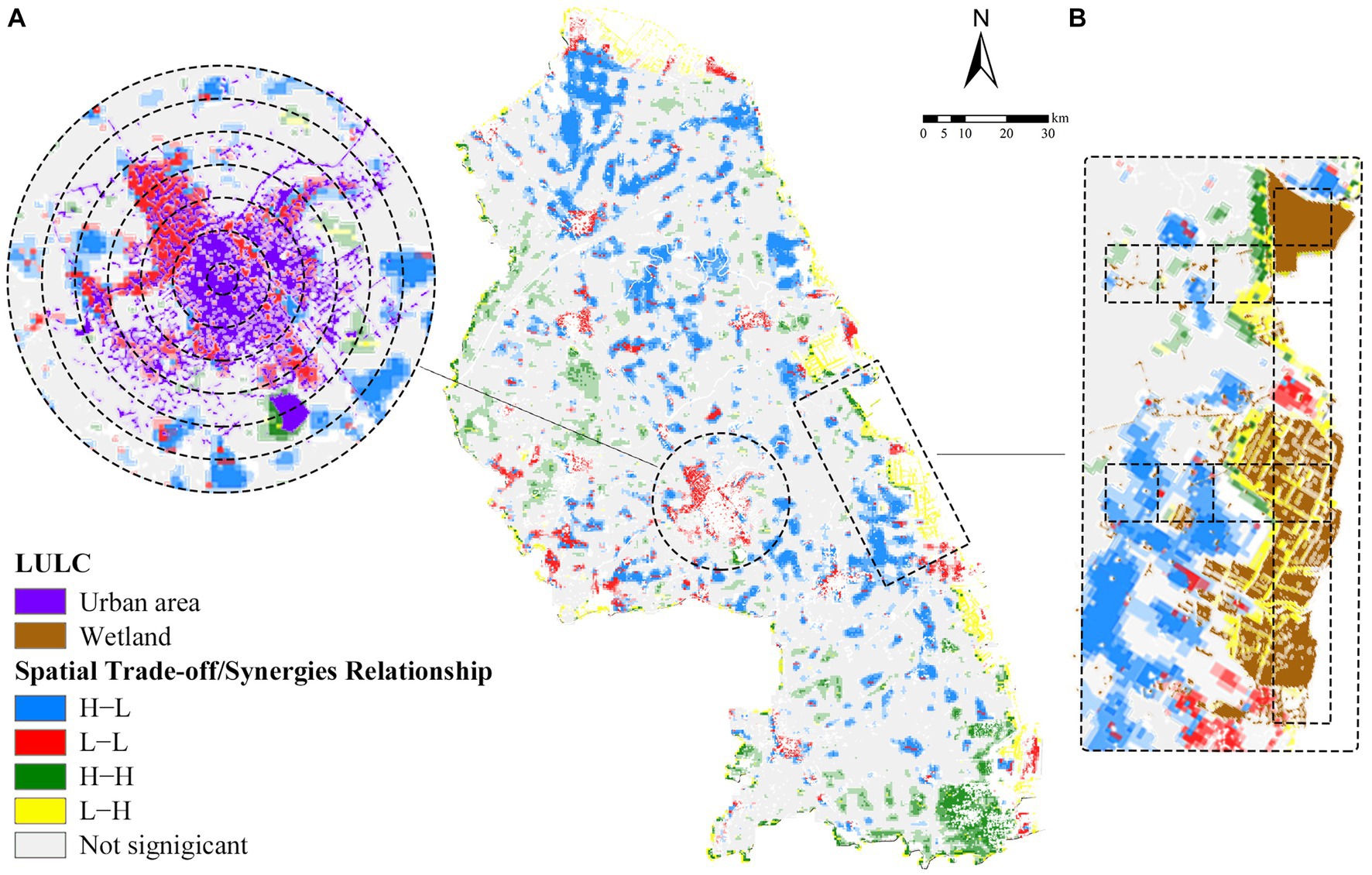
Figure 7. (A) Echelon ring diagram of urban areas. (B) Strip diagram of wetlands. LULC, land use and land cover.
5 Discussions
5.1 Spatial distribution of CLUFCs
The spatial distribution of CLUFCs had a certain spatial heterogeneity and a trend of urban–rural transition. Cold-spots of CLEF were concentrated around eco-sources, while hot-spots were aggregated near built-up areas (Figure 3B). It was indicated that increasing the number of eco-sources or optimizing the quality of habitats around cultivated land can improve CLEF. The cultivated land near built-up and protected areas had been identified as cold-spots of CLPF, while the primary CL far from the built-up areas was the hot-spot concentration areas of CLPF (Figure 4B). It was shown that location and development positioning of the city have a significant influence on the spatial differentiation of CLPF. This was consistent with previous researches on the urban–rural distribution trends of CLEF and CLPF (Qiu et al., 2022; Wang et al., 2023). However, not all cultivated land near cities (Sheyang County, Jianggang Town) was under pressure from semi-urbanization construction, indicating that centralized development and reasonable ecological utilization of cultivated land could play a role in alleviating ecological pressures. It was also worth noting that CLPF exhibits a significant geographical gradient pattern of decreasing from coastal to inland areas (Figure 4A). This was different from the traditional conclusions that increasing salinity in coastal cultivated land can lead to a decrease in crop yield (Xie et al., 2021). The main reason may be that the central-eastern part of Yancheng City is a traditional agricultural cultivation area, where several large farms are gathered. Intensive production and long-term soil improvement measures, such as straw returning, are beneficial for improving the quality of cultivated land in coastal saline alkali lands (Zuo et al., 2022).
In terms of the overall spatial relationship of CLUFCs, there were differences with a large degree dispersion and a negative spatial correlation in the spatial distribution of CLEF and CLPF (Figure 5D). It was proven that there was a conflict between the pursuit of improving cultivated land productivity and ecological stability. CLUFCs exhibited a high degree of geographic spatial dependence in its local spatial relationships, which had been confirmed in other case studies (Zhang Y. et al., 2019). H–H synergy zones were concentrated away from the urban built-up areas; L–L synergy zones gathered in the undeveloped areas around the suburbs of built-up areas and coastal wetlands; H–L trade-off zones were aggregated in agricultural high-yield areas; and L–H trade-off zones were distributed near the wetland protection areas along the eastern coast and the Dazhong Lake ecological area in Dazhong Lake town (Figure 5A).
5.2 Influencing mechanism of CLUFCs
Multiple case studies have shown that the spatial differentiation of CLUFCs is affected by internal and external driving factors (Wang et al., 2023). Huang et al. (2022) researched on CLUFCs in coastal areas of Jiangsu, and proposed that human intervention, one of the factors, affected CLUFCs. Fan et al. (2021) held that the distance from roads, an important influencing factor, affected the balance between urban, rural living and ecological functions. In this study, RDA identified the key internal driving factors and mechanisms that affected CLUFCs. Distances from main water and road networks, and population density are the dominant factors that form H–H, H–L, and L–L aggregation patterns, respectively (Figure 6C). The spatial spillover effect of land-use types also has an influence on the development pattern of cultivated land (Zhao T. et al., 2023). Moreover, the expansion of semi-urbanization construction and the driving of coastal wetland protection policies are important external factors that affect the distribution results of CLUFCs.
5.3 Development implications for CLUFCs
In the context of limited resources and environmental constraints, macro agricultural intervention measure is an effective means to enhance the sustainability of cultivated land ecosystems (Xie et al., 2023). Compared with traditional monoculture agriculture, it utilizes the synergistic effect of CLUFCs to improve the efficiency of land-use, alleviate functional conflicts, and enhance the service functions of agricultural ecosystem (Ayambire et al., 2019). However, the current cultivated land policy does not take into account the definition and maintenance of CLUFCs, and there is a lack of corresponding development policies and optimization strategies (Lyu et al., 2022).
Previous researches on influencing factors and mechanisms of CLUFCs aggregation relationships propose an optimization plan for 8 types of cultivated land development suitability zones under the corresponding 4 aggregation relationships. Based on four trade-off/synergy aggregation relationship zones obtained from LISA analysis, it is combined with the surrounding land-use types and development layout (Figure 8) to achieve the balance of cultivated land production function improvement, urban development expansion demand and ecological sustainability (Fan et al., 2021).
1. H–H synergy zones are planned and developed to be “multifunctional demonstration zones.” In these zones, the primary function of cultivated land is to maintain food production and ensure food security. Due to the coordinated development of production and ecology, H–H trade-off areas are positioned as the core production areas and built into demonstration areas of cultivated land in Yancheng City (Zhong et al., 2010). In production, it needs to reduce environmental pollution, and achieve green and ecological coordinated development with high yield and efficiency. It should be noted that the H–H aggregation areas are relatively close to the water network, and the use of pesticides and fertilizers should be reduced in production to avoid water pollution (Zhang et al., 2021).
2. L–L synergy zones are planned and constructed to be “urban feedback to suburban zones” and “ecological barrier zones.” It is affected by strong spatial spillover effect, so that L–L synergy zones near the built-up area is positioned as “urban feedback to suburban areas.” Relying on the distance advantage near the built-up area, the cultivated land in the suburban areas is back-fed, the industrial structure is optimized, and new and smart agriculture is developed. The L–L synergy zones near the protected areas are positioned as “ecological barrier areas,” serving as buffer zones between coastal wetland protection areas and productive cultivated land (Yin et al., 2017).
3. H–L trade-off zones as key areas for optimization are planned to be “new agricultural demonstration zones,” “ecological improvement zones” and “ecological stepping stones,” so as to give play to the advantages of CLPF in small areas, and coordinate the synchronous improvement of CLEF. The “new agricultural demonstration zones” which distributed in large farms and primary CL in agricultural counties, need to focus on ensuring the quality of cultivated land and promoting sustainable development of CLEF. The “ecological improvement zones” are distributed in the general CL, which belongs to individual farmers. These areas need to strictly control the red line of cultivated land protection and prohibit non-productive occupation (Zhu et al., 2021). Ye et al. (2020) emphasized that the construction of roads blocked the connectivity of animal corridors, leading to “ecological break-points.” Therefore, it is necessary to add “ecological stepping stones” at H–L trade-off zones near the road network system to restore CLEF as animal corridors.
4. L–H trade-off zones are planned and developed to be “agricultural ecological landscape zones” and “saline-alkali land productivity improvement zones.” “Agricultural ecological landscape zones” distributed in areas with weak CLPF near water eco-areas, are encouraged to combine surrounding tourism resources, promote the development of rural tourism industries, and coordinate the functional balance and development of production, ecology, and life, and improve the comprehensive quality of cultivated land (Yuan et al., 2021). In addition to being affected by the spatial spillover effect of coastal wetlands, the coastal saline alkali soil formed by long-term seawater immersion as another important factor also affects L–H trade-off zones (Zuo et al., 2022). Therefore, these areas are positioned as “saline-alkali land productivity improvement zones.” It focuses on improving soil physical and chemical properties through the scientific application of comprehensive measures, such as physics, chemistry, and biology, so as to achieve rapid, economic, and effective saline-alkali land improvement.
Wang et al. (2023) proposed a similar optimization strategy in his study on the CLUFCs of cultivated land in the suburbs of Changchun City. Based on hot−/cold-spots of the regional dominant function of cultivated land, the research area is divided into 9 multi-functional zones for enhancing the strategy. However, the remission and development of CLUFCs is a long-term task and systematic engineering that requires the establishment of long-term mechanisms, and comprehensive coordination of cultivated land functions and more scientific policy guidance. Therefore, it is necessary to correctly guide business entities to reach a development consensus, and drive high-quality agricultural development.
5.4 Contribution, limitations, and future research directions
Under the background of world food crisis, sustainable development of cultivated land and 17 UN SDGs, all levels of cities around the world, especially agricultural cities, need a research system that can coordinate and optimize the versatility of urban cultivated land ecosystem, improve the capacity and quality of cultivated land production, and optimize the urban spatial layout, so as to form a healthy and stable cultivated land ecosystem structure on the macro-scale of urban planning and ensure the quality and sustainability of cultivated land.
In this paper, LISA was used to analyze the spatial aggregation relationship coupled with CLEF and CLPF, and the planning method of future development suitability zoning of agricultural cities was emphatically studied. Compared with the conventional research methods of CLUFCs, the research method in this study has the following innovations: (1) NPP is selected as the index of CLPF. Compared with net biome productivity (NBP), it has the advantages of simple data processing, and small error when the yield of a single cultivated land type is only studied and analyzed (Qiu et al., 2023). This is one of the commonly used data sources for measuring production in agricultural informatics. (2) SPCoA-RDA analysis is carried out is to reveal the influencing factors and mechanisms of CLUFCs are revealed (Li S. et al., 2023). Compared with widely used SPCA for dimensionality reduction analysis based on Euclidean distance, SPCoA has the advantage of choosing other distance algorithms based on Euclidean distance (Bray-Curtis, Jaccard, Canberra distance, etc.). SPCoA-RDA analysis has advantages in exploring the spatial dependence of CLUFCs, which expands the understanding of the interaction process and mechanism among multiple functions of cultivated land. (3) From the perspective of spatial coupling of CLEF and CLPF, the universal and suitable development planning method of urban cultivated land under different ecological basic conditions is explored. Supplement of the spatial superposition analysis of socio-economic statistical data further improves the scientificity and reliability of CLUFCs evaluation (Li X. et al., 2022).
However, CLUFCs requires the construction of a complex and flexible evaluation system. The evaluation index, model construction and data acquisition difficulty are quite significant for CLUFCs quantification, and the existing macro remote sensing data cannot explain all the related factors of cultivated land productivity (Boori et al., 2022). In the further research, we can consider the comprehensive analysis of more cultivated land functions, such as air pollution, social security, etc., and make a more targeted exploration of urban CLUFCs and its driving factors. Additionally, our proposed arable land suitability zoning will bring long-term ecological benefits. In future studies, gross ecosystem product (GEP) can be combined to characterize the ability of agro-ecosystems to provide ecological products and assess ecological benefits (Zheng et al., 2023).
6 Conclusion
From the above results and discussions, the overall spatial relationship of CLUFCs in Yancheng City shows a negative spatial correlation, while its local spatial relationship represents a high degree of geographic spatial dependence. Both functions have significant spatial heterogeneity and different aggregation characteristics. The spatial differentiation of CLUFCs is the result of the combination of internal and external driving factors. On this basis, eight optimization schemes of cultivated land development zoning under four aggregation relationships were proposed. This study can provide a reference for the multi-function spatial integration model and the future development of suitability land-use planning of cultivated land in Yancheng City.
The report emphasizes the coordination of the human-land coupling relationship of cultivated land use, divides the suitability zones of food system transformation based on the potential CLUFCs, and explores the regulation policies of sustainable cultivated land-use under different functional subjects. This also has a certain practical significance for agriculture cities at all levels in the world. In the context of the food crisis and the realization of “zero hunger” in SDGs, the synergistic development strategy of cultivated land ecosystem in Yancheng City can provide theoretical reference for other agricultural cities that urgently need to balance the ecological quality of cultivated land.
Data availability statement
The original contributions presented in the study are included in the article/Supplementary material, further inquiries can be directed to the corresponding author.
Author contributions
NZ: Conceptualization, Methodology, Software, Writing – original draft, Writing – review & editing. QM: Formal analysis, Investigation, Writing – original draft. JA: Data curation, Supervision, Writing – original draft. ZZ: Methodology, Software, Writing – original draft. CZ: Methodology, Supervision, Writing – original draft.
Funding
The author(s) declare financial support was received for the research, authorship, and/or publication of this article. This work was supported by the Special Financial Grant of Grand Canal Cultural Belt Construction Institute (DYH22ZL08).
Acknowledgments
We thank Tao Liu and Zhengwang Li from Yangzhou University for the helpful criticism and linguistic assistance during the preparation of this manuscript. And we also thank the dear editors and reviewers.
Conflict of interest
The authors declare that the research was conducted in the absence of any commercial or financial relationships that could be construed as a potential conflict of interest.
Publisher’s note
All claims expressed in this article are solely those of the authors and do not necessarily represent those of their affiliated organizations, or those of the publisher, the editors and the reviewers. Any product that may be evaluated in this article, or claim that may be made by its manufacturer, is not guaranteed or endorsed by the publisher.
Supplementary material
The Supplementary material for this article can be found online at: https://www.frontiersin.org/articles/10.3389/fsufs.2023.1274980/full#supplementary-material
Footnotes
References
An, Y., Liu, S., Sun, Y., Shi, F., and Beazley, R. (2020). Construction and optimization of an ecological network based on morphological spatial pattern analysis and circuit theory. Landsc. Ecol. 36, 2059–2076. doi: 10.1007/s10980-020-01027-3
Anselin, L. (2010). Local indicators of spatial association–LISA. Geogr. Anal. 27, 93–115. doi: 10.1111/j.1538-4632.1995.tb00338.x
Ayambire, R. A., Amponsah, O., Peprah, C., and Takyi, S. A. (2019). A review of practices for sustaining urban and peri-urban agriculture: implications for land use planning in rapidly urbanising Ghanaian cities. Land Use Policy 84, 260–277. doi: 10.1016/j.landusepol.2019.03.004
Bai, X., Huang, Y., Ren, W., Coyne, M., Jacinthe, P., Tao, B., et al. (2019). Responses of soil carbon sequestration to climate-smart agriculture practices: a meta-analysis. Glob. Chang. Biol. 25, 2591–2606. doi: 10.1111/gcb.14658
Boori, M. S., Choudhary, K., Paringer, R., and Kupriyanov, A. (2022). Using RS/GIS for spatiotemporal ecological vulnerability analysis based on DPSIR framework in the republic of Tatarstan. Russia. Ecol. Inform. 67:101490. doi: 10.1016/j.ecoinf.2021.101490
Bureau of Statistics (2021). Available at: http://tjj.yancheng.gov.cn/ (Accessed October 30, 2022).
Cai, T., Zhang, X., Xia, F., and Lu, D. (2022). Function evolution of oasis cultivated land and its trade-off and synergy relationship in Xinjiang, China. Landscape 11:1399. doi: 10.3390/land11091399
Cao, J. H., Law, S. H., Wu, D. S., and Yang, X. D. (2022). Impact of local government competition and land finance on haze pollution: empirical evidence from China. Emerg. Mark. Financ. Tr. 59, 3877–3899. doi: 10.1080/1540496X.2022.2138326
Cao, J. S., Yang, Y. Q., Deng, Z. Y., and Hu, Y. D. (2022). Spatial and temporal evolution of ecological vulnerability based on vulnerability scoring diagram model in Shennongjia. Sci. Rep-UK 12:5168. doi: 10.1038/s41598-022-09205-w
Chaudhary, A., Gustafson, D., and Mathys, A. (2018). Multi-indicator sustainability assessment of global food systems. Nat. Commun. 9:848. doi: 10.1038/s41467-018-03308-7
Chen, Y., Jiang, Y., Huang, H., Mou, L., Ru, J., Zhao, J., et al. (2018). Long-term and high-concentration heavy-metal contamination strongly influences the microbiome and functional genes in Yellow River sediments. Sci. Total Environ. 637, 1400–1412. doi: 10.1016/j.scitotenv.2018.05.109
Chen, T., Peng, L., and Wang, Q. (2020). From multifunctionality to sustainable cultivated land development? A three-dimensional trade-off model tested in Panxi region of southwestern China. Nat. Resour. Model. 33:e12278. doi: 10.1111/nrm.12278
Closset-Kopp, D., Wasof, S., and Decocq, G. (2016). Using process-based indicator species to evaluate ecological corridors in fragmented landscapes. Biol. Conserv. 201, 152–159. doi: 10.1016/j.biocon.2016.06.030
De Montis, A., Ganciu, A., Cabras, M., Bardi, A., Peddio, V., Caschili, S., et al. (2019). Resilient ecological networks: a comparative approach. Land Use Policy 89:104207. doi: 10.1016/j.landusepol.2019.104207
Dengerink, J., Dirks, F., Likoko, E., and Guijt, J. (2021). One size doesn’t fit all: regional differences in priorities for food system transformation. Food Secur. 13, 1455–1466. doi: 10.1007/s12571-021-01222-3
Fan, Y., Gan, L., Hong, C., Jessup, L. H., Jin, X., Pijanowski, B. C., et al. (2021). Spatial identification and determinants of trade-offs among multiple land use functions in Jiangsu Province. China. Sci. Total Environ. 772:145022. doi: 10.1016/j.scitotenv.2021.145022
Fu, Y. J., Shi, X. Y., He, J., Yuan, Y., and Qu, L. L. (2020). Identification and optimization strategy of county ecological security pattern: a case study in the loess plateau, China. Ecol. Indic. 112:106030. doi: 10.1016/j.ecolind.2019.106030
Goethem, M. W. V., Pierneef, R., Bezuidt, O. K. I., Peer, Y. V. D., Cowan, D. A., and Makhalanyane, T. P. (2018). A reservoir of 'historical' antibiotic resistance genes in remote pristine antarctic soils. Microbiome 6:40. doi: 10.1186/s40168-018-0424-5
Guo, B., Wang, Y., Pei, L., Yu, Y., Liu, F., Zhang, D., et al. (2021). Determining the effects of socioeconomic and environmental determinants on chronic obstructive pulmonary disease (COPD) mortality using geographically and temporally weighted regression model across Xi'an during 2014–2016. Sci. Total Environ. 756:143869. doi: 10.1016/j.scitotenv.2020.143869
Han, S., Zhang, H. B., Liu, Y. Q., Xu, Y., Wang, J., and Jiang, C. (2022). Spatiotemporal change of landscape elasticity in Yancheng coastal wetland of China. Appl. Ecol. Environ. Res. 20, 4935–4949. doi: 10.15666/aeer/2006_49354949
He, S., Su, Y., Shahtahmassebi, A. R., Huang, L., Zhou, M., Gan, M., et al. (2019). Assessing and mapping cultural ecosystem services supply, demand and flow of farmlands in the Hangzhou metropolitan area. China. Sci. Total Environ. 692, 756–768. doi: 10.1016/j.scitotenv.2019.07.160
Hu, W., Zhang, S., Song, Y., Liu, T., Lin, Y., and Zhang, A. (2018). Effects of multifunctional rural land use on residents’ wellbeing: evidence from the Xinzhou district of Wuhan City. Sustainability 10:3787. doi: 10.3390/su10103787
Hu, J., Zhao, F., Zhang, X. X., Li, K., Li, C., Ye, L., et al. (2018). Metagenomic profiling of ARGs in airborne particulate matters during a severe smog event. Sci. Total Environ. 615, 1332–1340. doi: 10.1016/j.scitotenv.2017.09.222
Huang, S., Wang, Y., Liu, R., Jiang, Y., Qie, L., Pu, L., et al. (2022). Identification of land use function bundles and their spatiotemporal trade-offs/synergies: a case study in Jiangsu coast. China. Land 11, 286. doi: 10.3390/land11020286
Ibrahim, M. M., Tong, C., Hu, K., Zhou, B., Xing, S., and Mao, Y. (2020). Biochar-fertilizer interaction modifies N-sorption, enzyme activities and microbial functional abundance regulating nitrogen retention in rhizosphere soil. Sci. Total Environ. 739:140065. doi: 10.1016/j.scitotenv.2020.140065
Jia, S. S., Yang, C. Y., Wang, M. X., and Failler, P. (2022). Heterogeneous impact of land-use on climate change: study from a spatial perspective. Front. Environ. Sci. 10, 3877–3899. doi: 10.3389/fenvs.2022.840603
Jin, X. T., Li, Y., Sun, D. Q., Zhang, J. Z., and Zheng, J. (2019). Factors controlling urban and rural indirect carbon dioxide emissions in household consumption: a case study in Beijing. Sustain. For. 11:6563. doi: 10.3390/su11236563
Jogo, W., and Hassan, R. (2010). Balancing the use of wetlands for economic well-being and ecological security: the case of the Limpopo wetland in southern Africa. Ecol. Econ. 69, 1569–1579. doi: 10.1016/j.ecolecon.2010.02.021
Jongman, R., Külvik, M., and Kristiansen, I. (2004). European ecological networks and greenways. Landsc. Urban Plan. 68, 305–319. doi: 10.1016/S0169-2046(03)00163-4
Knaapen, J. P., Scheffer, M., and Harms, B. (1992). Estimating habitat isolation in landscape planning. Landsc. Urban Plan. 23, 1–16. doi: 10.1016/0169-2046(92)90060-D
Li, S., He, W., Wang, L., Zhang, Z., Chen, X., Lei, T., et al. (2023). Optimization of landscape pattern in China Luojiang Xiaoxi basin based on landscape ecological risk assessment. Ecol. Indic. 146:109887. doi: 10.1016/j.ecolind.2023.109887
Li, Y., Sang, S., Mote, S., Rivas, J., and Kalnay, E. (2023). Challenges and opportunities for modeling coupled human and natural systems. Natl. Sci. Rev. 10:nwod054. doi: 10.1093/nsr/nwad054
Li, C., Wang, X., Ji, Z., Li, L., and Guan, X. (2022). Optimizing the use of cultivated land in China’s main grain-producing areas from the dual perspective of ecological security and leading-function zoning. Int. J. Environ. Res. Public Health 19:13630. doi: 10.3390/ijerph192013630
Li, X., Xiao, P., Zhou, Y., Xu, J., and Wu, Q. (2022). The spatiotemporal evolution characteristics of cultivated land multifunction and its trade-off/synergy relationship in the two Lake plains. Int. J. Environ. Res. Public Health 19:15040. doi: 10.3390/ijerph192215040
Liang, X., and Li, P. (2020). Empirical study of the spatial spillover effect of transportation infrastructure on green total factor productivity. Sustain. For. 13:326. doi: 10.3390/su13010326
Liu, C., Xu, Y., Lu, X., and Han, J. (2021). Trade-offs and driving forces of land use functions in ecologically fragile areas of northern Hebei Province: spatiotemporal analysis. Land Use Policy 104:105387. doi: 10.1016/j.landusepol.2021.105387
Longato, D., Gaglio, M., Boschetti, M., and Gissi, E. (2019). Bioenergy and ecosystem services trade-offs and synergies in marginal agricultural lands: a remote-sensing-based assessment method. J. Clean. Prod. 237:117672. doi: 10.1016/j.jclepro.2019.117672
Lyu, Y., Wang, M., Zou, Y., and Wu, C. (2022). Mapping trade-offs among urban fringe land use functions to accurately support spatial planning. Sci. Total Environ. 802:149915. doi: 10.1016/j.scitotenv.2021.149915
Meng, J., Cheng, H., Li, F., Han, Z., Wei, C., Wu, Y., et al. (2022). Spatial-temporal trade-offs of land multi-functionality and function zoning at finer township scale in the middle reaches of the Heihe River. Land Use Policy 115:106019. doi: 10.1016/j.landusepol.2022.106019
Merk, M. S., and Otto, P. (2022). Estimation of the spatial weighting matrix for regular lattice data—An adaptive lasso approach with cross-sectional resampling. Environmetrics 33:e2705. doi: 10.1002/env.2705
Pascual-Hortal, L., and Saura, S. (2006). Comparison and development of new graph-based landscape connectivity indices: towards the priorization of habitat patches and corridors for conservation. Landsc. Ecol. 21, 959–967. doi: 10.1007/s10980-006-0013-z
Peng, J., Liu, Z., Liu, Y., Hu, X., and Wang, A. (2015). Multifunctionality assessment of urban agriculture in Beijing City, China. Sci. Total Environ. 537, 343–351. doi: 10.1016/j.scitotenv.2015.07.136
Peng, J., Pan, Y. J., Liu, Y. X., Zhao, H. J., and Wang, Y. L. (2018). Linking ecological degradation risk to identify ecological security patterns in a rapidly urbanizing landscape. Habitat Int. 71, 110–124. doi: 10.1016/j.habitatint.2017.11.010
Qiu, S., Fang, M., Yu, Q., Niu, T., Liu, H., Wang, F., et al. (2023). Study of spatialtemporal changes in Chinese forest eco-space and optimization strategies for enhancing carbon sequestration capacity through ecological spatial network theory. Sci. Total Environ. 859:160035. doi: 10.1016/j.scitotenv.2022.160035
Qiu, S., Yu, Q., Niu, T., Fang, M., Guo, H., Liu, H., et al. (2022). Restoration and renewal of ecological spatial network in mining cities for the purpose of enhancing carbon sinks: the case of Xuzhou, China. Ecol. Indic. 143:109313. doi: 10.1016/j.ecolind.2022.109313
Saura, S., and Pascual-Hortal, L. (2007). A new habitat availability index to integrate connectivity in landscape conservation planning: comparison with existing indices and application to a case study. Landsc. Urban Plan. 83, 91–103. doi: 10.1016/j.landurbplan.2007.03.005
Soille, P., and Vogt, P. (2009). Morphological segmentation of binary patterns. Pattern Recogn. Lett. 30, 456–459. doi: 10.1016/j.patrec.2008.10.015
Stegen, J. C., Lin, X. J., Konopka, A. E., and Fredrickson, J. K. (2012). Stochastic and deterministic assembly processes in subsurface microbial communities. ISME J. 6, 1653–1664. doi: 10.1038/ismej.2012.22
Su, Y., Xue, H., and Liang, H. (2019). An evaluation model for Urban Comprehensive carrying capacity: An empirical Case from Harbin City. Int. J. Environ. Res. Public Health 16:367. doi: 10.3390/ijerph16030367
Tang, F., Li, Y., Liu, X., Huang, J., Zhang, Y., and Xu, Q. (2023). Understanding the relationships between landscape eco-security and multifunctionality in cropland: implications for supporting cropland management decisions. Int. J. Environ. Res. Public Health 20:1938. doi: 10.3390/ijerph20031938
Tong, T., Yu, T. H. E., Cho, S. H., Jensen, K., and De La Torre Ugarte, D. (2013). Evaluating the spatial spillover effects of transportation infrastructure on agricultural output across the United States. J. Transp. Geogr. 30, 47–55. doi: 10.1016/j.jtrangeo.2013.03.001
Wang, G., Peng, W., Xiang, J., Ning, L., and Yu, Y. (2022). Modelling spatiotemporal carbon dioxide emission at the urban scale based on DMSP-OLS and NPP-VIIRS data: a case study in China. Urban Clim. 46:101326. doi: 10.1016/j.uclim.2022.101326
Wang, X., Wang, D., Wu, S., Yan, Z., and Han, J. (2023). Cultivated land multifunctionality in undeveloped peri-urban agriculture areas in China: implications for sustainable land management. J. Environ. Manag. 325:116500. doi: 10.1016/j.jenvman.2022.116500
Wickham, J. D., Riitters, K. H., Wade, T. G., and Vogt, P. (2010). A national assessment of green infrastructure and change for the conterminous United States using morphological image processing. Landsc. Urban Plan. 94, 186–195. doi: 10.1016/j.landurbplan.2009.10.003
Xie, H., Li, J., Zhang, Y., Xu, X., Wang, L., and Ouyang, Z. (2021). Evaluation of coastal farming under salinization and optimized fertilization strategies in China. Sci. Total Environ. 797:149038. doi: 10.1016/j.scitotenv.2021.149038
Xie, W., Zhu, A., Ali, T., Zhang, Z., Chen, X., Wu, F., et al. (2023). Crop switching can enhance environmental sustainability and farmer incomes in China. Nature 616, 300–305. doi: 10.1038/s41586-023-05799-x
Xu, W., Wang, J., Zhang, M., and Li, S. (2021). Construction of landscape ecological network based on landscape ecological risk assessment in a large-scale opencast coal mine area. J. Clean. Prod. 286:125523. doi: 10.1016/j.jclepro.2020.125523
Yancheng People’s Government (2014). Available at: http://www.yancheng.gov.cn/ (Accessed October 30, 2022).
Yang, C., Guo, H., Huang, X., Wang, Y., Li, X., and Cui, X. (2022). Ecological network construction of a national park based on MSPA and MCR models: An example of the proposed national parks of “Ailaoshan-Wuliangshan” in China. Landscape 11:1913. doi: 10.3390/land11111913
Yang, X. D., Wang, W. L., Su, X. F., Ren, S. Y., Ren, Q. Y., Wang, J. L., et al. (2022). Analysis of the influence of land finance on haze pollution: An empirical study based on 269 prefecture-level cities in China. Growth Chang. 54, 101–134. doi: 10.1111/grow.12638
Ye, H., Yang, Z., and Xu, X. (2020). Ecological corridors analysis based on MSPA and MCR model—a case study of the Tomur world natural heritage region. Sustain. For. 12:959. doi: 10.3390/su12030959
Yin, G. Y., Liu, L. M., and Jiang, X. L. (2017). The sustainable arable land use pattern under the tradeoff of agricultural production, economic development, and ecological protection-an analysis of Dongting Lake basin, China. Environ. Sci. Pollut. Res. 24, 25329–25345. doi: 10.1007/s11356-017-0132-x
Yu, M., Yang, Y., Chen, F., Zhu, F., Qu, J., and Zhang, S. (2019). Response of agricultural multifunctionality to farmland loss under rapidly urbanizing processes in Yangtze River Delta. China. Sci. Total Environ. 666, 1–11. doi: 10.1016/j.scitotenv.2019.02.226
Yuan, B., Fu, L., Zou, Y., Zhang, S., Chen, X., Li, F., et al. (2021). Spatiotemporal change detection of ecological quality and the associated affecting factors in Dongting Lake Basin, based on RSEI. J. Clean. Prod. 302:126995. doi: 10.1016/j.jclepro.2021.126995
Zhang, W., Liu, G., Yang, Q., and Yang, Z. (2021). Urban ecological risk transmission model based on Bayesian network. J. Clean. Prod. 296:126559. doi: 10.1016/j.jclepro.2021.126559
Zhang, Y., Guan, D., Wu, L., Su, X., Zhou, L., Peng, G., et al. (2022). How can an ecological compensation threshold be determined? A discriminant model integrating the minimum data approach and the most appropriate land use scenarios. Sci. Total Environ 852:158377. doi: 10.1016/j.scitotenv.2022.158377
Zhang, Y., Long, H., Tu, S., Ge, D., Ma, L., and Wang, L. (2019). Spatial identification of land use functions and their tradeoffs/synergies in China: implications for sustainable land management. Ecol. Indic. 107:105550. doi: 10.1016/j.ecolind.2019.105550
Zhang, P., Shi, X., Sun, Y., Cui, J., and Shao, S. (2019). Have China’s provinces achieved their targets of energy intensity reduction? Reassessment based on nighttime lighting data. Energy Policy 128, 276–283. doi: 10.1016/j.enpol.2019.01.014
Zhao, S., Cao, Y., Feng, C., Guo, K., and Zhang, J. (2022). How do heterogeneous R&D investments affect China's green productivity: revisiting the porter hypothesis. Sci. Total Environ. 825:154090. doi: 10.1016/j.scitotenv.2022.154090
Zhao, S., Cao, Y., Hunjra, A. I., and Tan, Y. (2023). How does environmentally induced R&D affect carbon productivity? A government support perspective. Int. Rev. Econ. Financ. 88, 942–961. doi: 10.1016/j.iref.2023.07.022
Zhao, T., Pan, J., and Bi, F. (2023). Can human activities enhance the trade-off intensity of ecosystem services in arid inland river basins? Taking the Taolai River asin as an example. Sci. Total Environ. 861:160662. doi: 10.1016/j.scitotenv.2022.160662
Zheng, H., Wu, T., Ouyang, Z. Y., Polasky, S., Ruckelshaus, M., Wang, L. J., et al. (2023). Gross ecosystem product (GEP): quantifying nature for environmental and economic policy innovation. Ambio 52, 1952–1967. doi: 10.1007/s13280-023-01948-8
Zhong, L., Liu, L., and Liu, Y. (2010). Natural disaster risk assessment of grain production in Dongting Lake area, China. Agric. Agric. Sci. Procedia 1, 24–32. doi: 10.1016/j.aaspro.2010.09.004
Zhu, Y., Zhou, X., Gan, Y., Chen, J., and Yu, R. (2021). Spatio-temporal differentiation and driving mechanism of the resource curse of the cultivated land in main agricultural production regions: a case study of Jianghan plain, Central China. Int. J. Environ. Res. Public Health 18:858. doi: 10.3390/IJERPH18030858
Zou, L. L., Liu, Y. S., Wang, J. Y., and Yang, Y. Y. (2021). An analysis of land use conflict potentials based on ecological-production-living function in the southeast coastal area of China. Ecol. Indic. 122:107297. doi: 10.1016/j.ecolind.2020.107297
Keywords: cultivated land-use function conflicts, ecological function, productive function, MSPA-MCR model, LISA analysis, SPCoA-RDA
Citation: Zhu N, Ma Q, Ai J, Zeng Z and Zhou C (2023) Potential land-use function conflicts of cultivated land for urban sustainable development: a case study in Yancheng City, China. Front. Sustain. Food Syst. 7:1274980. doi: 10.3389/fsufs.2023.1274980
Edited by:
Albie F. Miles, University of Hawaii–West Oahu, United StatesCopyright © 2023 Zhu, Ma, Ai, Zeng and Zhou. This is an open-access article distributed under the terms of the Creative Commons Attribution License (CC BY). The use, distribution or reproduction in other forums is permitted, provided the original author(s) and the copyright owner(s) are credited and that the original publication in this journal is cited, in accordance with accepted academic practice. No use, distribution or reproduction is permitted which does not comply with these terms.
*Correspondence: Chunhua Zhou, Y2h6aG91QHl6dS5lZHUuY24=