- 1Equipe Système de Production et Gestion des Ressources Naturelles de Sikasso, Institut d’Economie Rurale (IER), Bamako, Mali
- 2Plant Production Systems, Wageningen University and Research, Wageningen, Netherlands
Smallholder farming systems are vulnerable to disruptions. The COVID-19 pandemic weakened the financial ability of the Malian government in the 2020–21 growing season to subsidize cotton farmers’ access to mineral fertilizers and the cotton company (CMDT) could not offer a good cotton price. Consequently, farmers refused to grow cotton, leading to a cotton crisis with implications on crop production and farmers’ livelihoods. We used data collected over three consecutive growing seasons in the old cotton basin of Koutiala and analysed them using two-way mixed ANOVA over selected indicators related to farm and household components. The analysis was done for farms of different resource endowment, through comparing the cotton crisis season to the two previous normal seasons. Besides the abandonment of cotton, the total cropped area and area devoted to maize reduced in 2020–21, while the area allocated to millet, sorghum and cowpea increased, especially for cotton farmers with medium and high resource endowments. In addition, the nitrogen use intensity dropped at the farm level and particularly for the cereal crops, but without negative effect on yields of maize, millet and sorghum. Food self-sufficiency and income per capita significantly increased for the medium resource farms, while income dropped for the high resource farms with large herds. The farming system was able to absorb the shock of limited access to fertilizer for one season, due to the elimination of otherwise strong labour competition between cotton and cereal crops, favourable weather conditions and farmers’ responsive coping with the cotton crisis. This study revealed the importance of disaggregated livelihood evaluations, because resource endowments have implications not only for the actual effect on livelihoods, but also for farmers’ adaptive capacity.
1 Introduction
Smallholder farmers and their farming systems are vulnerable to different types of local to global disruptions. As a recent example of the latter, the outbreak of the Corona virus disease (COVID-19) provoked a global recession (Ozili and Arun, 2020), which resulted not only in reduced international demand for exported products such as cotton, but also in price collapses in the international market (Edmonds et al., 2020). This situation has caused severe disruptions of food systems (Aday and Aday, 2020; Ivanov, 2020) and impacted livelihoods of smallholder farmers in low-and medium income countries that strongly depend on exported commodities (Tröster and Küblböck, 2020), such as cotton in Mali. The outbreak of COVID-19 reached Mali in March 2020 (CRISIS24, 2020), prompting the Malian government to take emergency measures (INSTAT, 2020) to contain the spread of the virus and to mitigate its social and economic impact, which has led to increased public expenditures (International Monetary Fund, 2020). These measures, along with the global recession, created fiscal deficits and financing needs (International Monetary Fund, 2020) and reduced the budget for economic sectors such as agriculture (Theriault et al., 2021). Besides, since 2012, Mali has faced a challenging social and security situation, caused by terrorism, internal armed conflicts and climate change (Dal Santo and van der Heide, 2018), with significant negative effect on the state’s income and expenditures.
In Mali, the cotton produced in 2019 was processed and sold at the international market in 2020. The disruptions in the international market of cotton caused by the COVID-19 pandemic negatively affected not only the export of cotton fibre by “Compagnie Malienne pour le Développement des Textiles” (CMDT), but also the cotton production during the 2020 growing season (May–November). Cotton cultivation supports the livelihoods of more than four million people (CMDT, 2018), in terms of their access and use of key inputs (e.g., fertilizer) for improved crop yields to meet food and income needs. In addition, cotton plays a crucial role in soil fertility management, because the residual nutrients from fertilizer applied to cotton fields are available to the next crop in the rotation (Ripoche et al., 2015; Falconnier et al., 2016). Therefore, cotton cultivation contributes to the livelihood of producers (Tefft, 2010) and to sustainable food production (Ripoche et al., 2015). Further, cotton cultivation drives the whole farming system because CMDT facilitates the provision of extension services and other support to the village-based farming communities (Soumaré, 2008; Camara, 2015). Despite that, farmers are facing many challenges within the cotton-based system, including poverty, dependence on the international cotton market (Theriault et al., 2013), climate change (Traoré et al., 2013) and degradation of natural resources (Benjaminsen et al., 2010), including declining soil fertility.
The Malian government launched a subsidy program directly after the food crisis of 2007–08 to boost agricultural productivity especially for cotton and the main food crops for which mineral fertilizers (i.e., NPK and urea) were subsidized at about 45% of the market price (Koné et al., 2019). In cotton growing areas, the procurement and distribution of agricultural inputs are facilitated by the CMDT that pre-finances the subsidy every year against reimbursement by the government. However, since 2016 the government did not compensate the subsidy funds to the CMDT, which exacerbated its already precarious financial situation (Berthé, 2020). It was further aggravated with the immediate effects of COVID-19 in 2020 related to the low demand for cotton fibre and reduced prices in the international market (Edmonds et al., 2020).
The financial situation for both the government and the CMDT seriously affected the terms of exchange for cotton cultivation in the 2020–21 growing season. Consequently, a reduction of about 9% (from 275 to 250 FCFA/kg) compared to the previous season in the cotton price paid to farmers was negotiated and agreed with the national union of cotton producers in April, at the beginning of the 2020 growing season(IPC, 2020). Concurrently, changes were announced by the cotton value chain stakeholders concerning fertilizer subsidies (IPC, 2020). These changes implied a shift from subsidizing fertilizer for cotton and cereals to a subsidy of the cotton price loss. These terms of exchange in the 2020–21 season were not acceptable for many cotton growers (Berthé, 2020), after relatively high purchase prices of cotton and subsidized access to inorganic fertilizer in the previous 5 years (2015–2019). In response, farmers massively protested through boycotting cotton production in the 2020–21 season, and the national cotton area fell to 165,000 ha from around 700,000 ha during the previous three seasons (CMDT, 2022). This institutional shock—related to both declining cotton price paid to farmers, and changes in the fertilizer subsidy policy—led to what we call the “cotton crisis”, with farmers losing cotton income in abandoning cotton cultivation, and therefore having no access to (un)subsidized inputs for cereals on credit supported by the cotton contract schemes. Whereas effects on crop acreages, input use, agricultural productivity, food self-sufficiency and income seem inevitable, no quantitative information is available about the implications of the cotton crisis on the farming system and livelihoods of smallholder farmers in Mali.
Farms exhibit diverse resource endowments within farming systems (Giller et al., 2011), which can be captured using a farm typology (Falconnier et al., 2015). The cotton crisis may differently affect agricultural production and livelihoods of smallholders of different resource endowments. While access to fertilizer and agricultural productivity differs between farm types with the poorest farms often left behind (Falconnier et al., 2015), it is likely that abandoning cotton would have a larger effect on the high resource farms that cultivate more cotton and strongly depend on cotton for their access to inputs and income generation. A better understanding of equity dynamics in smallholder systems—through assessing differentiated impacts (Thuijsman et al., 2022) —can help in supporting the adaptive capacities of farmers of different resources. In the present study, we aim to investigate the implications of the cotton crisis induced by the COVID-19 pandemic on agricultural productivity and livelihoods of smallholders with different resource endowments in the cotton-based farming system of Koutiala, in southern Mali.
The following questions were addressed:
i. What was the effect of the cotton crisis on land allocation to crops, fertilizer use and crop yields for different farm types within the farming system in southern Mali?
ii. What were changes in food self-sufficiency and income generation in the 2020–21 growing season compared to the previous years for different farm types?
We hypothesized that higher resource endowed farms were less resilient to the cotton crisis, because of their stronger dependence on cotton. This research particularly seeks to fill the dearth of quantitative studies on the effects of institutional shocks induced by the COVID-19 pandemic on smallholder farming systems, in contrast to studies addressing the direct and immediate effects of containment measures (e.g., Workie et al., 2020; Goswami et al., 2021; Nchanji et al., 2021). These studies reported large negative effects, for instances, on specific groups, such as horticultural farmers (Rapid Country Assessment: Mali, 2020; Middendorf et al., 2021) due to disruptions in transport and marketing in March–April 2020. However, at the strongest moment of the measures, farmers in this study area were using food and crop income from 2019 growing season (May–October). In contrast, the institutional shocks affected crop production in 2020 growing season and household food and income needs afterwards (October–September 2020–21). The paper uses disaggregated livelihood evaluations for farms of different resource endowments to improve our understanding on differentiated adaptive capacities and associated contributing factors of farmers’ resilience to induced institutional shocks in the supply chain of a smallholder-dependent exported commodity. Our study focusses on the scale levels of the farm and the farming system, leaving out the perspectives of the other major stakeholders, especially those in the cotton value chain, such as the CMDT and the state.
2 Materials and methods
2.1 Study area
This study was conducted in the old cotton basin of Koutiala, a rainfed area located in southern Mali with more than one million people (RGPH, 2009) where nearly 90% of the population is engaged in cotton production. The growing season lasts about 6 months, starting in May and ending in October, with annual cumulative rainfall ranging from 600 to 1,200 mm (Traoré et al., 2013). The farming system is based on the cultivation of annual crops, including cotton, cereals and legumes on poor soils (Benjaminsen et al., 2010; Laris et al., 2015). In this area, the CMDT and the National Union of Cotton Producers coordinate the procurement and distribution of agricultural inputs including (un)subsidized fertilizer to cotton producers (Koné et al., 2019). In parallel, farmers have access to unsubsidized fertilizer in the market (Theriault et al., 2018). Both organic and inorganic fertilizers are applied solely on cotton and maize (Kanté, 2001; Blanchard, 2010). Mineral fertilizers for cotton and maize are provided on credit as part of the cotton growing agreement (Dissa et al., 2021). Cotton and groundnut are produced for cash while cereals are mainly produced for household consumption. Livestock keeping, including cattle, sheep, goats and donkeys, is also an important component of the farming system, as main sources of income, draught power and manure. Crop-livestock interactions form the backbone of the farming system to support the livelihoods and the resilience of the farming communities, enabling good crop performance, food self-sufficiency and income generation. Manure application has positive effects on crop yields (Kanté, 2001), while draught power supports the timely execution of major crop operations. Farms in this area are heterogenous in terms of resource endowment with implications on their access to productive resources and can be classified in four farm types, using key farm characteristics, including annual cropped area, number of workers, number of draught tools (e.g., ploughs, weeder, and sowing machines) and livestock ownership (Falconnier et al., 2015). The four types are High Resource Endowed with Large Herds (HRE-LH), High Resource Endowed (HRE), Medium Resource Endowed (MRE) and Low Resource Endowed (LRE). Following Falconnier et al. (2015), farms owning more than 21.4 Tropical Livestock Units (TLU) of 250 kg were classified into HRE-LH. Of the remaining farms, those having more than 9.5 workers were categorized as HRE. After that, farms with more than 5.8 ha, 2.2 TLU and/or 2 draught tools were included in the group of MRE, and lastly farmers that did not meet the aforementioned criteria were considered as LRE (see Supplementary Figure S1). The resource endowment is positively correlated with better access to productive resources. For instance, the large ownership of livestock by HRE-LH farms favours their manure access (Powell et al., 2004; Blanchard, 2010).
2.2 Data collection
The data collection relied on annual household surveys conducted over three consecutive seasons (2018–19 to 2020–21) from the same farms using structured questionnaires in six villages. Three of the six villages (M’Peresso, Nampossela, Nitabougouro) were situated 15–20 km south of the main city (Koutiala) and were difficult to reach due to poor accessibility, whereas the other three (Deresso, N’Tiesso, and Signe) were located in the north near the main tarred road, at a comparable distance. The villages were selected as representative of existing villages within the old cotton basin farming system of Koutiala. The farms were selected proportionally per village and per farm type to account for heterogeneity in terms of resource endowments. Individual farmers were selected, from the list of farmers for each village, according to their availability and willingness to participate in our surveys. Farmers were surveyed by enumerators based on self-reporting information at the end of each season (from August to September), because at that time all the information was available. The enumerators were trained prior to the survey to minimize bias, which was especially relevant for questions involving multiple sources and household members, such as off-income. Also, a particular attention was paid to seasonality related to the timing of production (May–October) and household consumption (October–September). At the end of each survey, the reliability of the respondent’s answer was scored—with regards to the information accuracy and willingness to respond to questions—by the enumerator using a 5-likert scale (ranging from highly dubious to highly reliable). Only survey data with reliable information were analysed. The information collected included farm and household data including the total cropped land, acreage per crop, use of mineral fertilizer and crop production, as well as income from other on-farm activities (e.g., livestock keeping) and off-farm sources (e.g., remittance).
A total number of 125 farmers (17 to 24 farmers per village for all farm types) were repeatedly interviewed in the 2018–19, 2019–20 and 2020–21 growing seasons. However, only 83 farmers (6 to 20 per village for all farm types) had complete information to calculate total farm income for two seasons (2018–19 and 2020–21), because of missing data, for instance, on livestock or off-farm income. There were 25 HRE-LH, 53 HRE, 37 MRE and 10 LRE farms among the 125 farms surveyed (Table 1), while the 83 farms comprised 18 HRE-LH, 28 HRE, 27 MRE, and 10 LRE farms. This distribution among the farm types was similar to other studies in the Koutiala district (Falconnier et al., 2015; Huet et al., 2020). The relatively small sample size for the income calculation can possibly undermine the accuracy of the estimate, particularly for farm types with fewer farms, such as the LRE. Table 1 presents the averages and standard deviations of the key farm characteristics used to classify the 125 sampled farms. As expected, the size of the resources increases from the LRE to HRE(-LH) farmers, which is not the case for all the ratios. For instance, the MRE farms had the lowest people-to-land ratio.
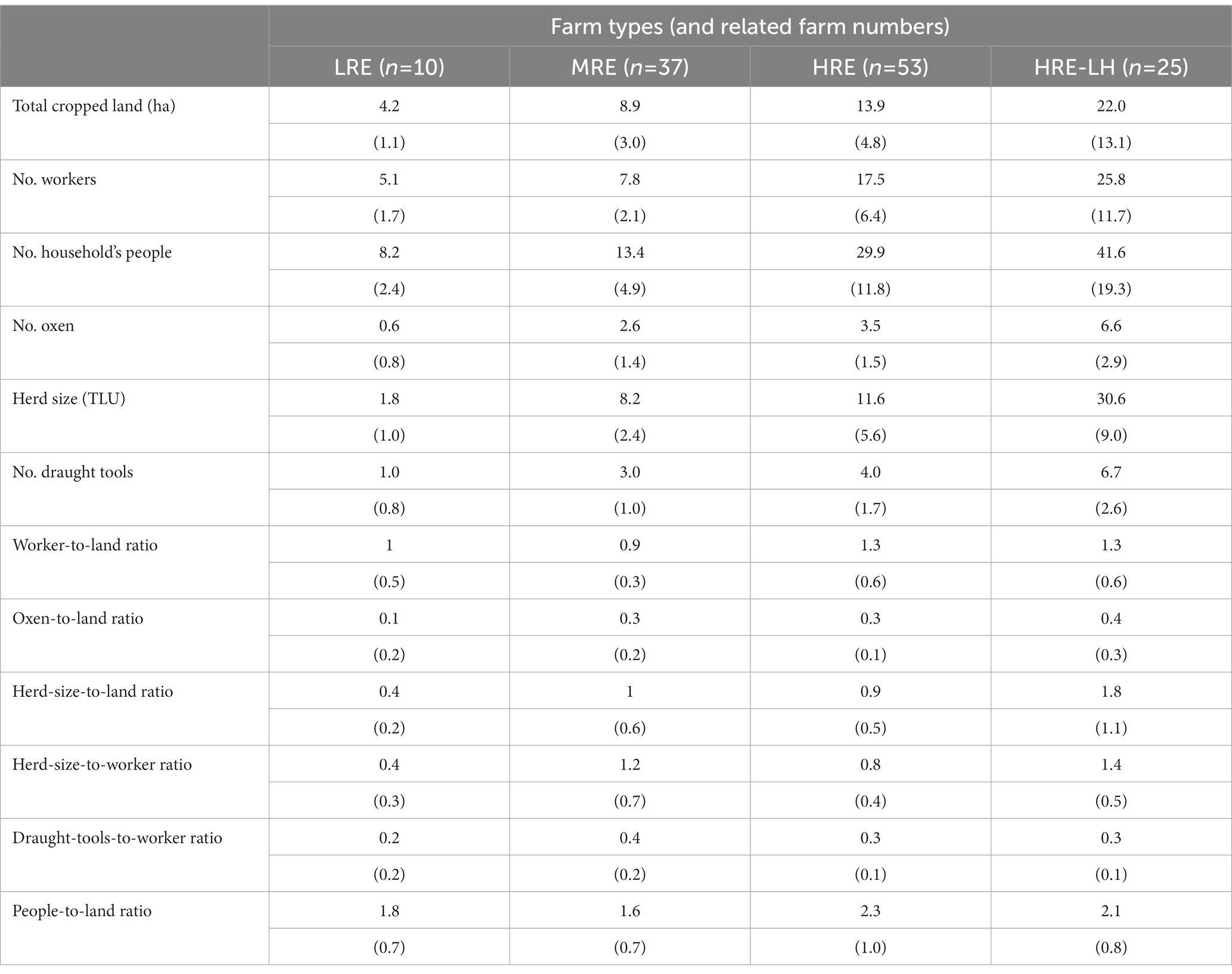
Table 1. Averages (and standard deviations in the brackets) of the key farm characteristics used in classifying the sampled farms into the four farm types.
2.3 Description and calculation of indicators
Crop-and household-level indicators were used to analyse changes in farmers’ livelihood in terms of outputs, outcomes and potential impacts (DfID, 2007). The changes, due to the cotton crisis, mainly included farmers’ access to and use of assets (e.g., land) and inputs (e.g., fertilizer) to implement activities (e.g., crop production) for high productivity (e.g., yield) to meet their livelihood needs in terms of food and income. To capture the changes in livelihoods, we selected a set of indicators, including total cropped area, acreage of the major crops and yields of the cereal crops, nitrogen use intensity (NUI) for the cereal crop and for the entire farm, food self-sufficiency and income per capita. In so doing, we acknowledge a partial livelihood analysis due to the focus on the productivity, economic and social dimensions of the cotton crisis. However, the indicators were assessed across four farm types to allow differentiated livelihood evaluation (Dorward et al., 2005) in each season from 2018–19 to 2020–21. The calculated indicators were particularly used to examine differences between the cotton crisis season (2020–21) and the preceding two seasons. In what follows details are given for the calculation of the indicators (see also Supplementary Table S1).
2.3.1 Total cropped area and acreage of the major crops
The total cropped area (ha) and the acreages of each major crop (cotton and cereals) were calculated per farm and per season. The areas allocated to the legume crops, including groundnut, cowpea as a sole crop and soyabean, were presented individually and then summed for the statistical analysis. Where cowpea was grown in intercropping with cereal crops, the intercropped cowpea area was excluded due to lack of reliable data.
2.3.2 Nitrogen use intensity
Farmers in southern Mali use NPK (15,15:15) and urea (46% N) fertilizer for the production of cotton and cereals, in addition to animal manure. Also, the cultivated legume crops bring atmospheric nitrogen into the systems, but they rarely receive mineral fertilizer. Here we focus on NUI related to the use of external inputs of mineral N. Information about the individual amounts of NPK and urea for each crop was collected for the growing season of 2018–19, while the combined amount of fertilizer (both NPK and urea) per crop was asked for the seasons of 2019–20 and 2020–21. For these two seasons, the amounts of NPK and urea per crop were estimated based on the proportions of NPK and urea derived from the 2018–19 data, cross-checked with key informants. The proportions were approximately 75% NPK and 25% urea of the combined amount applied to cotton and 50% NPK and 50% urea for cereals, irrespective of farm type. The combined amount of fertilizer (both NPK and urea) used per crop was converted to total N, which was divided by the crop area, to obtain the NUI (kg N/ha) per crop. Afterwards, the NUI at farm level was calculated by taking into account the area of each crop, excluding legumes.
2.3.3 Land productivity
The yields were estimated only for cereals. Cotton was excluded because it was not planted in the 2020–21 growing season. The legume crops were not considered due to lack of reliable data. Yields were obtained dividing the total harvested amount by the area allocated to each crop within the farm.
2.3.4 Food self-sufficiency
The food self-sufficiency (FSS) was calculated in terms of the fulfilment of household annual energy need in each growing season, by dividing on-farm produced energy by the household energy requirement. First, on-farm produced energy was estimated through converting the on-farm production of the main cereals (i.e., maize, millet, and sorghum) to energy content (kcal/year) based on crop-specific energy content, and then adding these up per farm. An average energy content of 3,580, 3,630, and 3,290 kcal/kg for maize, millet, and sorghum, respectively, was considered in this calculation.1 Purchased food was not included and all on-farm production of cereals was assumed to be self-consumed. We focus here on self-sufficiency, as farmers’ main objective for the most consumed cereals (Balié et al., 2013), and also most farms in this area are food secure (Giller et al., 2021) in normal situations. Also, livestock products (meat and milk) were ignored, because of the low frequency of their consumption in this research area (Generoso, 2015). Second, the household energy requirement was estimated and expressed in Adult Male Equivalents (AME) based on the gender (i.e., male and female) and the generation (i.e., children less than 18 years of age and adults) of household members, following Britten et al. (2006) and assuming that the lifestyle of people in rural communities is active (see Supplementary Table S2 for more information). The number of people within households belonging to different gender and generation groups was collected only once during the study period and assumed to remain constant during the three years of the study.
2.3.5 Income per capita and poverty line
The income per capita for each season was obtained dividing the inflation-adjusted total household income by the household size expressed in Adult Equivalents (AE). The conversion into AE allows to take into account household composition in a gender-neutral way (Atkinson, 1995) with regard to income generation, in contrast to the Adult Male Equivalents (AME) that gives different weights to men and women because of different energy requirements. We use the “Oxford scale” (Atkinson, 1995) that is mostly used for developing countries. This conversion scale assigns a value of 1 to the household head (man or woman), of 0.7 to each additional adult and of 0.5 to each child.
Total household income is a summation of net crop income (i.e., revenue minus costs of inputs such as fertilizer and seeds), gross income from livestock and milk sales and off-farm income. Livestock costs were ignored because purchased feed was typically for oxen (Amole et al., 2022), which are durable productive assets not considered in the annual income flow. For both the net crop income and livestock revenues, the costs of (family and hired) labour were excluded from the computation, as disaggregated labour data per operation (e.g., weeding) and per crop (e.g., cotton) were not collected due to time and financial resource limitations. Also, labour costs were not accounted for because the share of hired labour in the total labour is marginal (Coulibaly, 2011; Soumaré et al., 2018), while deducting the cost of family labour in this context of semi-subsistence farming would result in underestimated income for people that relied on their own labour for their income needs. Also, our calculation did not include the depreciation cost of machines. Mid-season prices (in May) for products with volatile prices such as cereals and prices paid by farmers to obtain (un)subsidized fertilizer were used to calculate crop income. The off-farm income for each season (October–September) comprised various income sources for all household members such as remittances, small businesses, crafts, aid and donation, non-agricultural activities related to the production of charcoal and (wild) tree products.
The total household income was adjusted to inflation for comparison across seasons using inflation rates2 in 2019 and 2021. The income per capita was converted in US dollars purchasing power parity to allow comparison with the international poverty line (Jolliffe and Prydz, 2016) of 1.9 $PPP/day/AE for the world poorest countries such as the Mali, according to the list of low income countries by the world bank.3 The most recent PPP conversion factor4 (in 2020) from FCFA to $PPP was used, which was 211.41.
2.4 Data analysis
The longitudinal data (2018–19 to 2020–21) were analysed using the open-source software R (version 4.0.0, R Core Team, 2020). The analysis firstly relied on descriptive tools, based on visualization, and then on inferential analysis using mixed two-way ANOVA, with the indicators as dependent variables and “farm type” and “season” as factors. The two-way mixed ANOVA design was motivated by the fact that it considers repeated measures over time of the four different farm types to estimate their effects on the calculated indicators. In this respect the season is the “within-subject” factor while the farm type is the “between-subject” factor, making the two-way ANOVA design more powerful in detecting effects than a standard two-way ANOVA (Verma, 2015). The validity of the two-way mixed ANOVA estimates depends on whether key assumptions were met (see Supplementary Table S3). The assumption of normality of residuals was checked with Shapiro–Wilk test and Q-Q plots, whereas homoscedasticity was tested using the Levene’s test. Where these assumptions were violated, the output variable was square root-transformed, and then the checks were done again. The p-values of the factors were interpreted only when all the ANOVA assumptions were met; otherwise, plots were used to illustrate the effects of factor variables on the indicators. Further, relevant post hoc analysis was done for different indicators depending on significant two-way interaction at α = 0.05. When the interaction was significant, one-way ANOVA of the within-subject factor season at each level of the between-subject factor farm type was performed to isolate the season effect, particularly for the cotton crisis season. Then, paired t-test comparisons between seasons for each farm type were done, based on Bonferroni p-value adjustment for repeated measures with a significance level of p ≤ 0.05. Inversely, one-way ANOVA of the between-subject factor farm type at each level of the within-subject factor season was performed to isolate the farm type effect, using independent t-test comparison also based on Bonferroni p-value adjustment. When the interaction was not significant, main effects of factors on the indicators were analysed based on multiple comparison using the paired sample t-test for the within-subject factor and using independent t-test for the between-subject factor. The season of the cotton crisis (2020–21) was compared to each of the two previous seasons, reducing the risk of comparing it to a season that may be exceptional for other reasons, such as weather or other factors that can influence livelihood outcomes. Further, the statistical analysis was backed up with farmers’ perception of changes on crop production and access to inputs due to the cotton crisis.
3 Results
3.1 Total cultivated area
Farm type and season significantly affected the total cultivated land area but the interaction between the two factors was not significant (Supplementary Table S4:Section a). Farmers did not grow cotton in the 2020–21 growing season. As a result, the total cropped area strongly decreased (p < 0.001), especially for the HRE-LH and HRE farms in comparison to the two previous seasons (Figure 1). Indeed, the average cultivated areas were approximately 19 ha and 13 ha, respectively, for the HRE-LH and HRE farms in the previous seasons and they dropped to 15 ha and 11 ha in the cotton crisis season. A decrease was observed in the total cropped area for the MRE farms in 2020–21, which was significant compared with 2018–19, but not compared with 2019–20. No significant change was observed in the area cropped over the three seasons for LRE farms.
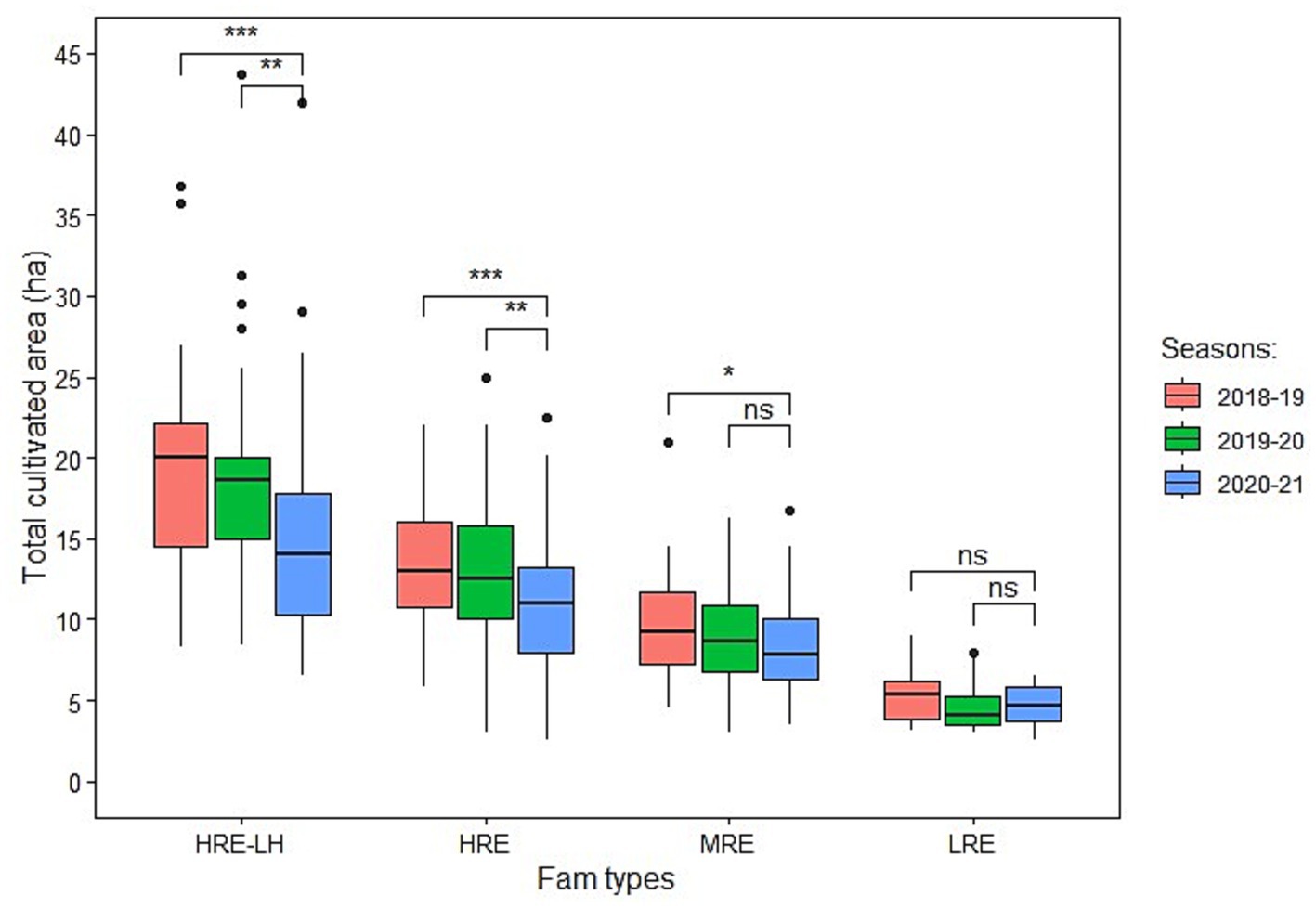
Figure 1. Total cultivated area per farm type over three growing seasons. The horizontal black line in the boxplot indicates the median. The height of the box represents the interquartile range. The whiskers extend to the most extreme data point that is no more than 1.5 times the interquartile range from the edge of the box. Statistical significance of the p-values (p) with ns (not significant): p > 0.05; *, p ≤ 0.05; **, p ≤ 0.01; ***, p ≤ 0.001.
3.2 Area allocation to crops
The estimates of area allocation to different crops were heavily skewed and violated the normality and homoscedasticity assumptions of the ANOVA, therefore the results could not be interpreted with confidence. Nevertheless, cotton was hardly cultivated in the 2020–21 growing season (Figure 2). More than 80% of the interviewed farmers motivated this choice by the low cotton price and removal of subsidized access to mineral fertilizer for cotton and cereals (results not shown). Without being involved in cotton production, farmers had to purchase (un)subsidized fertilizer for cereals in a harsh period of cash scarcity. Consequently, the area allocated to the nutrient-demanding maize crop was also smaller in 2020–21 compared to the previous two seasons for all farm types (Figure 2A). Additionally, the proportion of farmers who grew maize decreased by about 8% in 2020–21 compared with the previous seasons in which it was grown by almost all farmers. Overall, the median cultivated maize area was around 1 ha in 2020–21 against 1.5 ha in the previous seasons (Supplementary Figure S2). In contrast, the acreages of millet and sorghum were larger in 2020–21 relative to the previous seasons, especially for HRE-LH, HRE and MRE who were the main cotton growers in the normal seasons (Figure 2A). The median cultivated millet area was 4 ha in 2020–21 against 3 ha in the previous seasons. For sorghum, the median was 2 ha in 2020–21 with farmers above it allocating a larger area compared to the previous seasons (Supplementary Figure S2). Our results related to area of cereal crops were confirmed by the perceptions of farmers (not shown). The area allocated to legumes (i.e., groundnut, cowpea and soyabean) as sole crops taken together remained more or less the same in 2020–21 compared with 2018–19 but increased compared with 2019–20 (Figures 2A,B). Approximately 85, 85 and 20% of farmers grew groundnut, cowpea (i.e., sole and/or intercropping) and soyabean, respectively, over the three seasons (not shown). Intercropping of cowpea with maize and sorghum is a common practice in southern Mali. No data was available to accurately estimate the intercropped area of cowpea across the three seasons. That said, farmers perceived an increase in area allocation to cowpea in 2020–21.
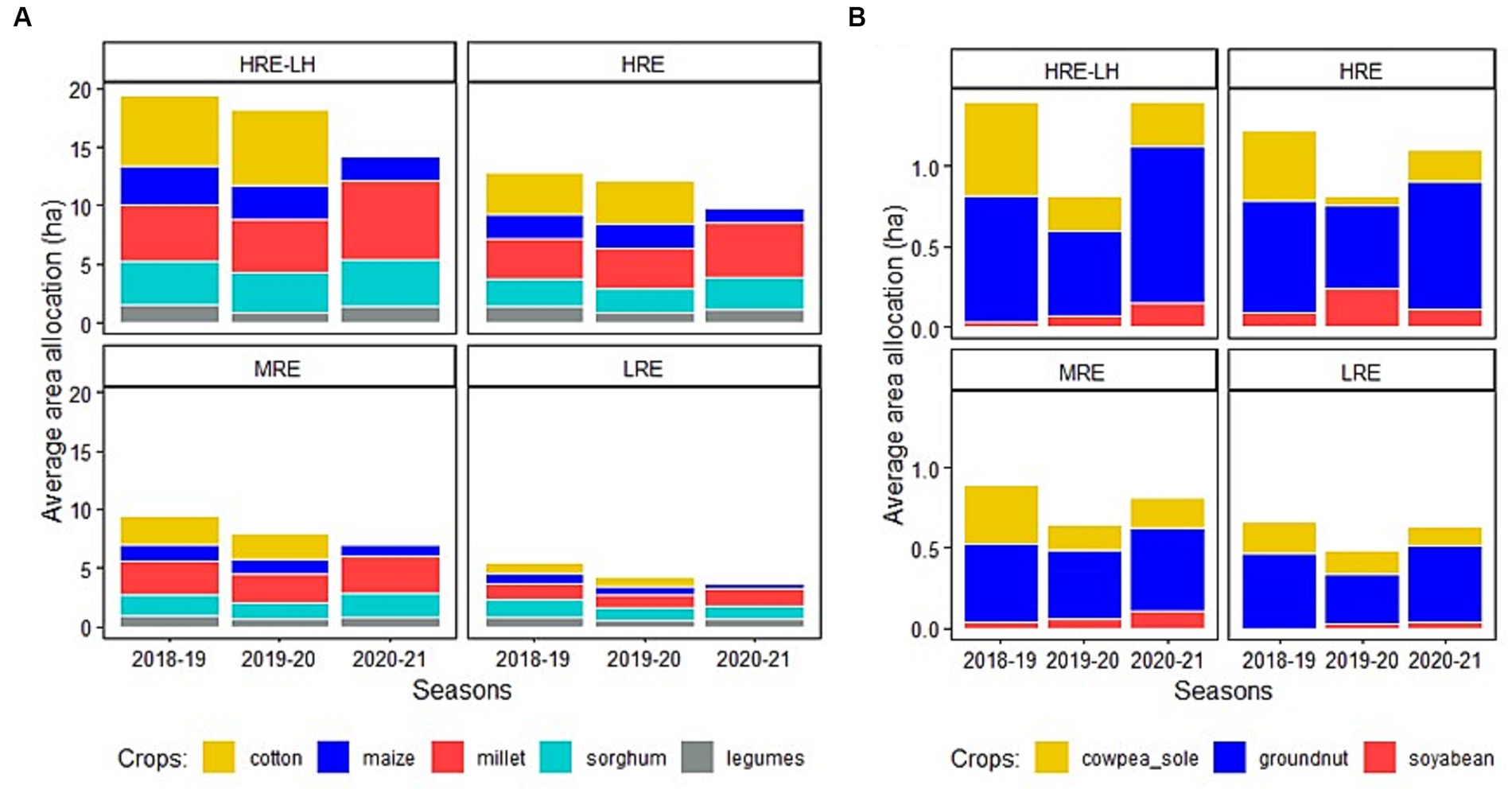
Figure 2. Crop area (ha) per farm type over the three seasons. (A) Area of cotton, maize, millet, sorghum, and legumes. (B) Area of cowpea, groundnut, and soyabean. The area of cowpea grown in intercropping with maize and sorghum is excluded due lack of reliable data.
3.3 Farmers’ access to mineral fertilizer and N use intensity
In previous seasons, cotton farmers used to obtain mineral fertilizer on credit from their village-based cotton cooperatives, which cost was repaid with the cotton revenue after the harvest. However, farmers’ access to mineral fertilizer was negatively affected in 2020–21 because of abandoning cotton and having access only to unsubsidized fertilizer as described above. While the subsidized price was approximately 20 US dollars per bag of 50 kg (for NPK or urea), the market price fluctuated between 34 and 38 US dollars for NPK per bag, and between 27 and 30 US dollars for urea per bag in 2020–21. With the abandonment of cotton, the proportion of farmers accessing mineral fertilizer from cotton cooperatives dropped from 95 to 40% between 2019–20 and in 2020–21 growing seasons, while the proportion of farmers purchasing from the market raised from 8 to 50% (not shown). As a result, the share of fertilizer from cotton cultivation in the total amount also dropped from 98 to 52% between 2019–20 and in 2020–21. Therefore, some farmers (42%) could benefit fertilizer inputs on credit through cotton cooperatives without cotton cultivation in 2020–21. This access was possible because some cooperatives received (unsubsidized) fertilizer prior to the growing season and the stock was independently managed by the cooperatives. At the farm level, all farmers used mineral fertilizer (i.e., NPK and/or urea) in 2019–20, while only 85% of them used fertilizer in the cotton crisis season. Among these farmers, about 26, 26, 16 and 14% of them relied on sales of cereals, livestock, other income source and remittances to purchase mineral fertilizer, respectively.
3.3.1 Nitrogen use intensity at the farm level
The effects of both season and farm type on the NUI (kg N/ha) were significant (see Supplementary Table S4:Section b), but the interaction between season and farm type was not (p = 0.234). The difference in NUI between 2020–21 and the previous two seasons was highly significant (p < 0.001), irrespective of the farm type, while it was not significant between 2018–19 and 2019–20 growing seasons (Figure 3). Overall, the NUI at farm level was approximately 30 N kg/ha (for HRE-(LH) and MRE farms) in the two normal seasons and then decreased to 10 N kg/ha in 2020–21 due to the cotton crisis. LRE farms used less fertilizer compared with the other farm types in each of the three seasons (Figure 3). While the difference in the NUI for the LRE farms was significantly lower compared with the other farm types in the two previous growing seasons, it was not significant in 2020–21, indicating that the cotton cultivation strongly contributes to the farm-level NUI for the other farm types who generally cultivate large cotton area (Figure 2A).
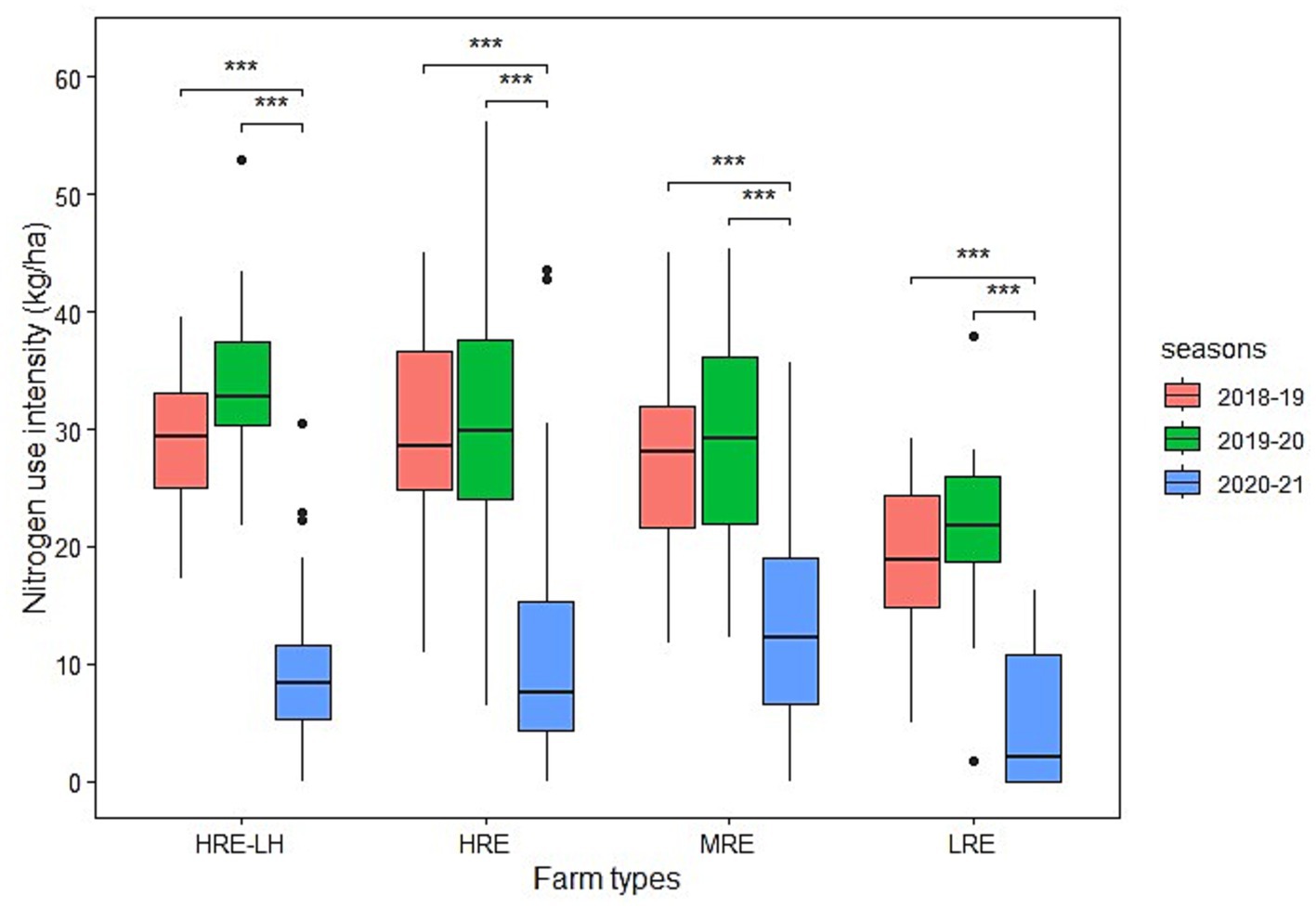
Figure 3. Nitrogen use intensity per farm type over three cropping seasons. The horizontal black line in the boxplot indicates the median. The height of the box represents the interquartile range. The whiskers extend to the most extreme data point that is no more than 1.5 times the interquartile range from the edge of the box. Statistical significance of the p-values (p) with ns (not significant): p > 0.05; *, p ≤ 0.05; **, p ≤ 0.01; ***, p ≤ 0.001.
3.3.2 Nitrogen use intensity of maize, millet, and sorghum
Farm type and season had significant effects on the NUI of maize and millet, while only the season effect was significant for sorghum. The interaction between farm type and season was not significant for any of the cereals (see also Supplementary Table S4:Section c, d, e). Overall, the average NUI in the two normal seasons was approximately 55, 12 and 8 kg N/ha for maize, millet, and sorghum, respectively and then fell significantly (p < 0.001) in the 2020–21 growing season to 45, 6 and 3 kg N/ha. The shares of farms within each farm type that did not use any mineral fertilizer for a cereal crop (i.e., crop NUI = 0) increased in 2020–21 compared with the previous seasons, involving 14 to 20%, 43 to 70% and 75 to 88% of farms for maize, millet, and sorghum, respectively (Supplementary Table S5). In addition, the shares of farms for each farm type that did not grow a cereal crop also increased in 2020–21, in particular the LRE farms for maize, indicating that most poor farmers could not afford applying mineral fertilizer on their maize fields. No significant differences were observed between the farm types for maize in the normal seasons and for millet in the cotton crisis season (Figure 4). Specifically, maize NUI was strongly reduced for the LRE farms compared with the other farm types in the cotton crisis season. The LRE farms also used the least fertilizer on millet and sorghum in the normal seasons.
3.4 Cereal crop yield
Maize yield seemed not to be affected by the cotton crisis as season had no significant effect on maize yield. However, season had a very strong and significant effect (p < 0.001) on the yields of millet and sorghum, which were significantly lower in the 2018–19 season than in the following two seasons (Figure 5). However, no significant difference was observed in millet and sorghum yields between 2019–20 and 2020–21 seasons. Yields of maize and millet (p < 0.01) varied significantly among farm types but there were no differences in sorghum yield (see also Supplementary Table S4:Section f, g, h). Specifically, the maize and millet yields of LRE farms were smaller than of the other farm types (Figure 5), while no difference was found between the yields of MRE, HRE and HRE-LH farms in any season. No significant difference was observed for the sorghum yield between seasons. Maize yield was related to the resource endowment with HRE-LH having the best yield in each of the three seasons. The interaction between season and farm type had no significant effect on the yields of any of the cereal crops.
3.5 Food self-sufficiency for three agricultural seasons
Across the three seasons, more than 75% of farmers were food self-sufficient in terms of basic energy requirement (Figure 6). The degree of food self-sufficiency (FSS) differed among the farm types and from one season to another. Indeed, the effects of both farm type and season on household FSS were strongly significant (p < 0.001, see also Supplementary Table S4:Section i). Overall, FSS was significantly less in 2018–19 (p < 0.0001) compared to 2019–20 and 2020–21. Specifically, no significant difference existed between the cotton crisis season (2020–21) and 2019–20, even though an increase in FSS was noticeable from 2019–20 to 2020–21, except for LRE farms which showed a decreasing trend. FSS of MRE farms was much better than of farms belonging to HRE (p = 0.001) and to LRE (p = 0.03), whereas no difference was observed between MRE and HRE-LH farms in the 2020–21 season. About 50% of farms belonging to HRE-LH and MRE met twice or more of their food needs in the 2020–21 growing season, which was more compared to the normal seasons and to the other farm types. When surpassing the FSS requirement farmers usually sell their cereal surpluses to generate income, indicating that the HRE-LH and MRE farms were in a better position to generate a large income from the cereal surpluses compared to HRE and LRE farms. The interaction between season and farm type had no significant effect on FSS.
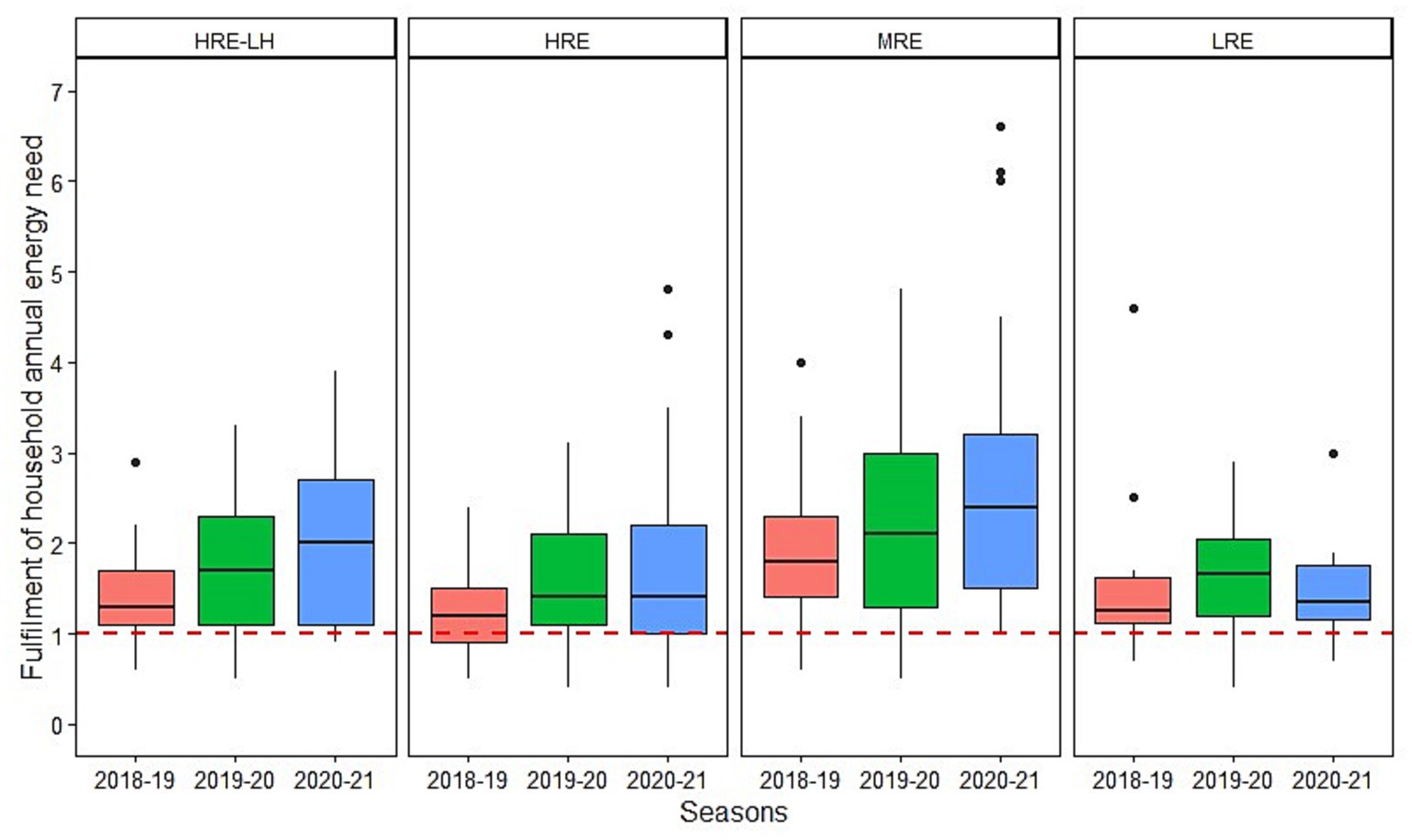
Figure 6. Fulfilment of household annual energy needs from 2018–19 to 2020–21 agricultural seasons for different farm types. The horizontal red dashed line indicates the fulfilment of food self-sufficiency. The horizontal black line in the boxplot indicates the median. The height of the box represents the interquartile range. The whiskers extend to the most extreme data point that is no more than 1.5 times the interquartile range from the edge of the box. Statistical significance of the p-values (p) with ns (not significant): p > 0.05; *, p ≤ 0.05; **, p ≤ 0.01; ***, p ≤ 0.001.
3.6 Income per capita for two agricultural seasons (2018–19 and 2020–21)
The household income in this area was mainly earned with crop production, followed by sales of livestock and livestock products (including milk) and off-farm income (Figure 7). In the absence of cotton income, many farmers saw their income from other crops increase in 2020–21. Similarly, some farmers increased their livestock income in 2020–21, through sales of (small)ruminants (Supplementary Figure S5). In contrast, the off-farm income strongly decreased in 2020–21 for the few farmers with large off-farm income in 2018–19. The income per capita of about 60% of farms fell below the international poverty line (1.9$ PPP/day/AE), for each of the two seasons considered. The income per capita was not stable over time for many farms, irrespective of farm type, with some farmers below the poverty line in 2018–19 having stepped up above it in 2020–21 and vice-versa (Figure 7). The proportion of farms with less income per capita in 2020–21 compared to 2018–19 was 72, 54, 33 and 50% of HRE-LH, HRE, MRE, and LRE, respectively (not shown). Indeed, half of the MRE farms were below the poverty line in 2018–19 and above it in 2020–21, whereas the HRE-LH farms exhibited the contrary dynamic. The ANOVA analysis (Supplementary Table S4: Section j) confirmed that the effect of farm type on income per capita was significant (p = 0.008), whereas the effect of season was not (p = 0.947). The interaction between the season and farm type had a significant effect on income (p = 0.032). The post hoc analysis confirmed that the income per capita was strongly influenced by the farm type in 2020–21 (p = 0.02) in comparison to 2018–19 (p = 0.07). The comparison also showed that the income per capita was particularly higher for MRE farms in 2020–21 compared to HRE farms (p = 0.028) and to LRE farms (p = 0.054). Overall, although the income per capita was not affected by the cotton crisis, MRE farms were better-off in 2020–21, while HRE-LH farmers were negatively affected (Supplementary Figure S4).
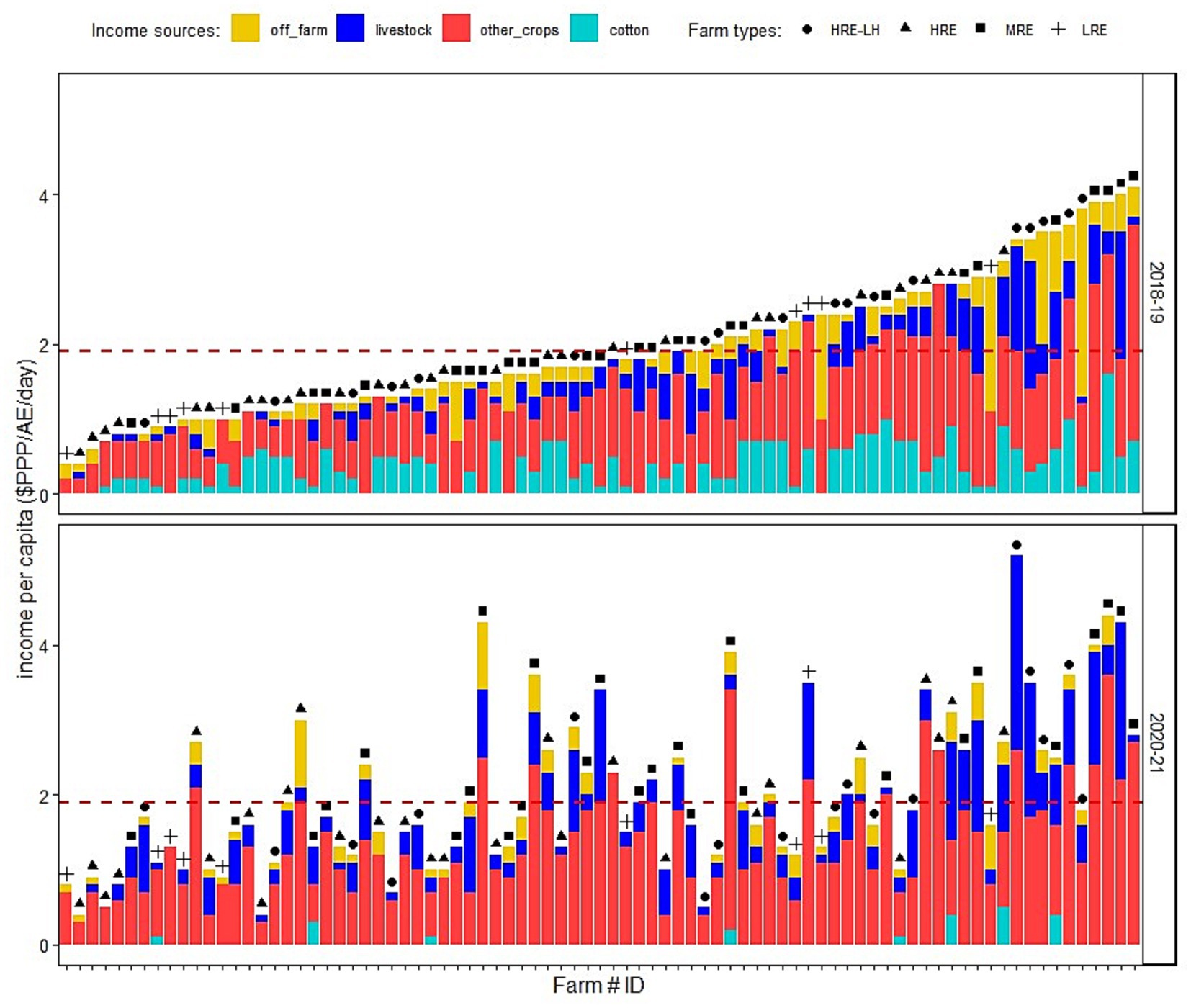
Figure 7. Income per capita over two agricultural seasons (2018–19 and 2020–21) for different farm types. Income per capita was calculated for 83 farmers for which we have full information on crop yields, livestock (and milk) sales and off-farm income. The horizontal dashed line indicates the World Bank international poverty line (1.9 $ a day). For both seasons, farms are ranked according to total income in 2018–19.
4 Discussion
This study assessed the effects of the cotton crisis, induced by the COVID-19 pandemic during the 2020–21 growing season, on the agricultural production and farm households within the cotton basin of Koutiala, in southern Mali. Due to the cessation of cotton production in 2020–21, the total cropped area and area devoted to maize reduced, whereas the land allocated to millet, sorghum and cowpea increased, especially for HRE-(LH) and MRE farms. In addition, even though the NUI at the farm level and for the cereal crops dropped in 2020–21, the yields of maize, millet and sorghum were not negatively affected. Food self-sufficiency and income per capita significantly increased for the MRE farms, while the income dropped for the HRE-LH farms.
4.1 Changes in crop production indicators due to the cotton crisis
4.1.1 Land allocation
The total cropped area decreased strongly due to the cotton crisis in the 2020–21 season compared with the two normal seasons for MRE, HRE, and HRE-LH farmers, while no change took place for LRE farms. This can be explained by the fact that these three farm types typically allocate a larger share of the total land to cotton during normal seasons compared with the LRE farms (Falconnier et al., 2015). The abandonment of cotton production was accompanied by a reduction in maize area, while the acreages of millet and sorghum increased (Figure 2A), which results could not be formally tested because the skewed nature of the data meant that statistical tests were not conclusive. The shift to millet and sorghum could be motivated by the limited demand of these cereal crops for mineral fertilizer compared to maize. Our results confirm earlier findings (Coulibaly, 2011; Laris et al., 2015) that maize cultivation is driven by the mineral fertilizer accessed through cotton cultivation. Additionally, maize is mostly cultivated for household consumption, because of land scarcity and population increase in this area (Soumaré, 2008; Falconnier et al., 2018), as it has higher yield per ha compared with other cereals (Figure 5). The decline in maize area was tactically compensated with an increase in area of substitute food crops to secure household food self-sufficiency.
We observed no change in area allocated to groundnut and soyabean, which can be explained by the limited scope for these crops to replace cotton for income generation. Indeed, groundnut was grown and sold within a very specific but limited period (August–October), to take advantage of seasonal cash opportunities (Ollenburger et al., 2018). Soyabean could be an alternative income opportunity as there is growing interest for it to prepare the local condiment “sumbala” (Falconnier et al., 2017). However, the market of soyabean is under-developed and not able to absorb a large supply. Therefore, producing soyabean for the market is not yet an option for most farmers. These findings support a conclusion by Rietveld (2009) that farmers face many limitations in choosing other crops than cotton for income generation, suggesting the need for market development of groundnut and soyabean to expand the adaptive capacity of farmers to cope with shocks. In contrast to groundnut and soyabean, farmers perceived that the cowpea area increased in the cotton crisis season compared with the previous seasons. Whereas farmers grow cowpea for grains and fodder, the poor and variable grain yield of cowpea (Falconnier et al., 2016) often discourages farmers to cultivate this crop. Farmers explained that they increased the cowpea area to produce fodder to mitigate the lack of concentrate feed, such as cottonseed cake, which they would normally purchase with cotton income.
4.1.2 Effects of limited access to mineral fertilizer on land productivity
We found that the NUI significantly decreased for all the cereal crops in the 2020–21 growing seasons compared with the previous ones (Figure 4). Surprisingly, the decrease in NUI was not associated with a decrease in cereal yields (Figure 5). This is in contrast to a study conducted in South Africa (Mthembu et al., 2022) which reported a decrease in the yields of staples, such as maize, compared with pre-COVID-19 season, due to limited access to inputs. The maintained yields of cereal crops can be explained by the following factors. First, critical yield-enhancing crop operations, such as weeding and hoeing, were timely implemented for the cereal crops as labour was not a limiting factor in the absence of cotton cultivation. Indeed, in normal seasons cotton was prioritized in allocating labour, and also the operation frequency was twice as high for cotton and maize compared to millet and sorghum (Dissa et al., 2023, under review5). Second, weather conditions were favourable for the growth of cereals in the growing season of 2020–21 with regular rains during crop growth and maturity (Supplementary Figure S6). Third, in the absence of cotton, manure was applied in most maize fields in 2020–21, while millet and sorghum were grown on fertile soils usually dedicated to the cultivation of cotton and maize (Supplementary Figure S3). These soils mostly contained residual nutrients from the previous season when fertilizer was applied, which positively affected yields, as also concluded by Ripoche et al. (2015). In contrast, in previous seasons, millet and sorghum were typically cultivated on poor soils, with negative effects on yields. Although yields of the cereal crops were not immediately penalized in the cotton crisis season, many farmers feared to face lower yields in the subsequent season as a result of declining soil fertility due to lower application of mineral fertilizer in 2020–21 and depletion of leftover nutrients from the previous seasons. This underscores the need to support farmers for integrated soil fertility management (Vanlauwe et al., 2015) in order to sustain land productivity.
Similar to area allocation, we found that the NUI at farm level was strongly related to the involvement of different farm types in cotton cultivation and their access to subsidized fertilizer inputs (Figure 3). Indeed, the NUI at farm level strongly decreased in 2020–21 relative to the normal seasons for all farm types. The results are in line with Falconnier et al. (2015) who reported a decrease in the NUI at farm level during a multi-year cotton crisis between 2004–05 and 2008–09 for the MRE, HRE and HRE-LH farms. Whereas there was no difference in NUI between the LRE farms and the other types in 2020–21, the LRE had a lower NUI in the crisis between 2004–05 and 2008–09 (Falconnier et al., 2015). This difference in NUI between the 2004–05–2008–09 and 2020–21 crises could be due to the fact that, although mineral fertilizer was not subsidized, its price did not change much throughout the previous crisis from 2004–05 to 2008–09 (Theriault et al., 2013). In addition, additional N supply through organic manure from livestock was not included in our calculation while it was included in the study by Falconnier et al. (2015). This could have led to reduced N supply estimates in our study but did not affect differences between seasons. Access to manure is correlated with livestock ownership (Blanchard, 2010), as farmers exclusively rely on their own produced manure. However, producing additional manure in the short term, as to mitigate limited access to mineral fertilizer, was not possible between the announcement of changes in the terms of exchange in mid-April and the start of the growing season in mid-May.
We found that the LRE farms had the strongest decrease in maize NUI in the 2020–21 season, while no difference was observed for millet and sorghum between the farm types in this season (Figure 4). Also, they had the lowest NUI for millet and sorghum in the normal seasons. This result suggests that access to mineral fertilizer by the LRE farms was particularly penalized by the increased prices during the 2020–21 season. We also found that the LRE farms had the lowest yields of maize and millet relative to the other farm types across the three seasons (Figure 5), as also reported by Falconnier et al. (2015). In addition, the FSS of LRE farms deteriorated in 2020–21, while it did not for the other farm types. The poor performance of this farm type can be explained by certain limitations of this type, including (1) low access to inorganic fertilizers as a result of a small share of cotton in total cropped area, (2) low production and application of manure due to limited ownership of livestock but also the lack of transport equipment, and (3) lack of oxen to implement critical crop operations on time. Overall, the findings related to LRE farms show that they were equally affected by the cotton crisis relative to the other farm types with regard to area allocation between maize, millet, sorghum and cowpea, while they were less exposed with regard to the total cropped area and farm-level NUI and highly exposed with regard to the NUI for maize. Also, the results indicate that the LRE were more sensitive and therefore vulnerable to institutional shocks, calling for special attention to enhance their adaptive capacity, for instance by facilitating their access to equipment (e.g., cart and oxen).
4.2 Changes in household indicators due to the cotton crisis
We found that FSS did not significantly increase in 2020–21 compared with 2019–20 although an increasing trend was noticeable, especially for HRE-(LH) and MRE farms. The stability in FSS suggests that the larger area allocation to millet and sorghum and the realized harvests could compensate the reduced maize cultivation, which was observed as well for the cotton crisis from 2004–05 to 2008–09 (Falconnier et al., 2015). This finding supports the conclusions of other studies that food self-sufficiency is a primary production objective within the farming system (Bosma et al., 1999; Ollenburger et al., 2018). The fulfilment of self-sufficiency was better for the MRE farms compared to the other farm types (but not significantly different with the HRE-LH) because MRE farms have the lowest people-to-land ratio relative to the other types, favouring the fulfilment of food needs from on-farm production. The LRE farms had the lowest fulfilment of FSS among all the farm types, especially in 2020–21, because of low cereal productivity. Furthermore, we found that the on-farm production of staple crops was enough to cover the annual food needs for at least 75% of farmers, irrespective of farm types and seasons.
We found that the total income per capita mainly came from cropping activities, which corroborates previous findings (Abdulai and CroleRees, 2001). The income per capita did not change at the farming system level, which can be explained by the rising income from cereals for many farmers, coupled with a 12% higher maize price in 2020–21 compared to last 5 years average (OMA and WFP, 2021). In addition, some farmers in our sample, especially HRE-LH and MRE farms, largely complemented crop income with income from livestock products including milk in 2020–21 season (Figure 7). Milk income could be an alternative to cotton, however, milk is not a viable option yet due to the high labour demand to produce cowpea fodder (De Ridder et al., 2015) and the lack of competitiveness of local milk in a dairy sector dominated by imported low-priced milk products (Corniaux et al., 2012). At the farm-level, the income per capita was not stable for most farmers from 2018–19 to 2020–21 and the change was related to farm resource endowment (Supplementary Figure S4). The income per capita particularly increased for most MRE farms in 2020–21, while it decreased for the HRE-LH farms that are heavily involved in cotton production. Therefore, the cotton crisis negatively affected the income of better resource endowed farms, despite their increased cultivation and income of cereals.
4.3 Resilience of farmers to the cotton crisis
Overall, the farming system coped well with the cotton crisis induced by the COVID-19 pandemic, because crop yields, income per capita and food self-sufficiency did not decrease in 2020–21 compared with the previous seasons. The overall income dynamics within the farming system mask income changes within farming households over the two periods (Figure 7), with income increase for some and income decrease for other households. As such, the cotton crisis of 2020–21 revealed the sensitivity (Urruty et al., 2016) of different farm types to changes in the institutional context. While this cotton crisis was a short, one-season shock, a longer period of impaired input access would likely result in a decline in the overall farm productivity and associated livelihoods. This concern was voiced by many farmers and other stakeholders. The apparent robustness of the farming system in 2020–21 can be explained by the elimination of otherwise strong labour competition between cotton and cereal crops (i.e., millet and sorghum), favourable weather conditions and farmers’ responsive coping with the cotton crisis. In the earlier multi-year cotton crisis, from 2004–05 to 2008–09, farmers mitigated the reduced access to cotton income through sales of livestock and off-farm activities including sales of forest products (timber and charcoal), small business and remittances from migrants (Droy et al., 2012; Soumaré et al., 2018). Soumaré et al. (2018) reported that many farms sold productive assets, such as oxen, to mitigate the consequence of the multi-year cotton crisis. The ability to sell assets contributes to system robustness, but undermines the system’s adaptability and capacity to maintain resilience in the long-term (Darnhofer, 2014; Urruty et al., 2016). The income reduction during the multi-year cotton crisis weakened the cohesion of some large farm households due to disagreement among the members about the income management (Soumaré et al., 2018). This situation led to certain high resource endowed farms, such as HRE-(LH), to split into two or more new farms with limited productive assets (e.g., LRE farms). In contrast, the one-season cotton crisis induced by the COVID-19 caused limited hardship in terms of prolonged cotton income reduction and did not seem to have strongly affected the cohesion within farms.
The cotton crisis induced by the COVID-19 was limited to one season because lessons learned from previous crises motivated quick policy responses, including the restoration of a favourable cotton price and the continuation of the fertilizer subsidy program. However, it should be recognized that the measures were motivated by the urge to support the cotton sector and the national economy, rather than to support the livelihoods of smallholder farmers, due to the multiplier effects of cotton supply on the whole economy (Camara, 2015). The quick response of the government sheds light on two facts. First, it underscores that smallholder farmers are key actors in the national economy through their production of cotton. Second, it emphasizes that farm management flexibility is a key prerequisite (Tendall et al., 2015) to increase the resilience and sustainability of farming systems, but also the national economy. However it also reveals a trade-off between policy choices, because the large subsidy program puts a heavy burden on public expenditures and limits spending in other sectors (Marenya et al., 2012; Koné et al., 2019).
4.4 Reflection on the effects of the cotton crisis
The maintained yields of cereal crops in the 2020–21 growing season indicate that the weather pattern within the growing season is a major determinant of yields. The three seasons considered in our study all had a late and erratic start of the growing seasons (from mid-May to late June, Supplementary Figure S6), which is unfavourable for cotton yield and favourable for cereals (Traoré et al., 2014). Hence, part of the reason for farmers not to grow cotton could be to lower the risk of cotton failure. However, more than 80% of the surveyed farmers formally indicated the lower cotton price and unsponsored access to fertilizers as their motives to not grow cotton in 2020–21. Household food self-sufficiency and income per capita were also maintained in the previous seasons with late and erratic start of the seasons, due to crop diversification (Makate et al., 2016) through tactical adjustment in land allocation (Dissa et al., 2023, under review, see footnote 5).
Across sub-Saharan Africa, Ayanlade and Radeny (2020) show that farming communities producing food crops for their own consumption, such as cereals, were less directly affected by the containment measures in 2020. This is also true for farmers in southern Mali who primarily produce for their own consumption, making them less dependent on the market for food access. Food consumed by these farmers during the moments of stringent measures in 2020 was already produced in the last growing season from May to October 2019. Also, farmers could sell agricultural products during the measures to meet their income needs, because the transport sector was mainly affected between March–April 2020 only (Rapid Country Assessment: Mali, 2020). Further, in March 2020, most farmers already received their cotton income for the last season.
As an exported cash crop the cotton supply chain is highly connected to the world market, in contrast to cereals that are traded in local food supply chains. Bui et al. (2021) show that local food supply chains were effective to support the market participation of smallholders in Vietnam during the COVID-19, as farmers could flexibly sell their products at the local market. Similar findings were also reported by Nchanji and Lutomia (2021) showing that local supply chains of common beans, vegetables, fish and fruit were effective to sustain rural and urban livelihood against the shock of COVID-19 in Eastern and Southern Africa. Therefore, farmers that were linked to the supply chains of exported cash crops such as cotton were most affected. This corroborates our finding that HRE-LH who usually allocate a large area to cotton production were more negatively affected compared with the other farm types.
We acknowledge that our study is somehow partial because data on fertilizer use and yields for the legumes were not available. However, legumes represented a very small share (less than 10%) in the total cropped area (Section 3.2) and normally did not receive fertilizer so that their yields as well as their contribution to the whole farming system were not expected to be affected. The farming system is heavily cereal-and cotton-based, both depending on fertilizer, and these crops showed indeed responses to the cotton crisis, in terms of their relative areas (Section 3.2), input use (Section 3.3.2), and yields (Section 3.4), leading to a relatively large contribution that matters to the entire system.
5 Conclusion
This study aimed to investigate the effects of institutional shocks induced by the COVID-19 pandemic on the smallholder farming systems, using a case within the old cotton basin of Koutiala in southern Mali. Based on a comprehensive picture of the changes in farming and household indicators, we generated strong evidence about the implications of the cotton crisis on farms of different resource endowments. The longitudinal approach allowed us to capture changes over time and the inclusion of two previous seasons reduced the risk of comparing the cotton crisis season to an exceptional season.
Our results reveal that the global COVID pandemic, the resulting public budget deficit, the collapse in cotton prices and the policy responses of the government and CMDT had repercussions for the production of cotton and food crops. We found that farmers hardly grew cotton in the 2020–21 growing season because of the low purchase price of cotton and the removal of subsidies on inorganic fertilizer. As a result, cotton growers shifted from fertilizer-demanding crops, such as maize, to millet and sorghum which are rarely fertilized. Although nitrogen use intensity decreased irrespective of the farm types, cereal yields were unaffected. The increased production of millet and sorghum contributed to maintaining food self-sufficiency, and an increased share of on-farm income in absence of cotton for medium to high resource-endowed farms. Overall, although the income per capita did not decrease at the farming system level, it was not stable at the farm-level for farms with different resources.
Our findings suggest that farms could absorb the shock of limited access to fertilizer inputs for at least one season. Stronger effects were prevented because the government reinstated favourable cotton prices and subsidized fertilizer the next season. However, should the shock persist over time with the system not receiving inputs, we would expect a decline in the overall productivity and associated contribution to people’s livelihoods. The exhibited farming system’s resilience for a single season was primarily due to the absence of intense labour competition between cotton and cereal crops, good weather conditions for cereal crops, and adaptive farm management by farmers in handling the challenges posed by the cotton crisis.
Our study suggests that resource endowments have implications, not only on farmers’ adaptive capacity, but also on how their livelihoods may be affected by major shocks in the institutional context, such as the cotton crisis in 2020–21. The changes in farmers’ livelihoods were investigated based on aggregated indicators (food self-sufficiency and income per capita) at the level of the farming households. It would be interesting in future studies to look at intra-household changes related to consumption and income generation, but also to analyse food security and poverty from their multidimensional implications on the overall livelihoods of people.
Data availability statement
The raw data supporting the conclusions of this article will be made available by the authors upon reasonable request.
Ethics statement
Ethical approval was not required for the studies involving humans because Ethical approval for this study was not required according to the checklist of the Social Sciences Ethics Committee of Wageningen University and Research. The studies were conducted in accordance with the local legislation and institutional requirements. The participants provided their written informed consent to participate in this study.
Author contributions
AD: Conceptualization, Data curation, Formal analysis, Investigation, Software, Visualization, Writing – original draft. MS: Supervision, Validation, Writing – review & editing. KG: Supervision, Writing – review & editing. KD: Conceptualization, Funding acquisition, Methodology, Supervision, Writing – review & editing.
Funding
The author(s) declare financial support was received for the research, authorship, and/or publication of this article. This work was supported by the McKnight Foundation, through the project “Pathways to agroecological intensification in the crop-livestock farming systems in southernMali” (Grant No. 19–310). The study also benefited from additional financial support from the Africa Research in Sustainable Intensification for the Next Generation (Africa RISING) programme.
Acknowledgments
We thank farmers from the villages of Nampossela, M’Peresso, Nitabougouro, N’Tiesso, Deresso and Signe in Koutiala district for their willingness to participate in the data collection over the three consecutive seasons. We extend our gratitude to the enumerators (Elie Togo, Seydou Maïga and Séry Coulibaly) who helped in the data collection. A word of thanks to Tenzin Wangchuck for the first data analysis while doing his internship. We also thank the reviewers for their valuable comments that helped to improve the manuscript.
Conflict of interest
The authors declare that the research was conducted in the absence of any commercial or financial relationships that could be construed as a potential conflict of interest.
Publisher’s note
All claims expressed in this article are solely those of the authors and do not necessarily represent those of their affiliated organizations, or those of the publisher, the editors and the reviewers. Any product that may be evaluated in this article, or claim that may be made by its manufacturer, is not guaranteed or endorsed by the publisher.
Supplementary material
The Supplementary material for this article can be found online at: https://www.frontiersin.org/articles/10.3389/fsufs.2023.1269355/full#supplementary-material
Footnotes
1. ^FAO: http://www.fao.org/3/t0818e/T0818E0b.htm, accessed 23/02/22.
2. ^From the world bank database, https://donnees.banquemondiale.org/indicator/FP.CPI.TOTL.ZG?end=2021&locations=ML&start=2018&view=chart, accessed on 15/09/2023.
3. ^https://data.worldbank.org/country/XM, accessed on 20/10/2021.
4. ^From the world bank database, https://data.worldbank.org/indicator/PA.NUS.PPP?locations=ML, accessed on 22/11/2021.
5. ^Dissa, A., Slingerland, M. A., Giller, K. E., and Descheemaeker, K. (2023). Navigating seasonality in cotton-based farming systems in southern. Mali. Clim. Risk Manag.
References
Abdulai, A., and CroleRees, A. (2001). Determinants of income diversification amongst rural households in southern Mali. Food Policy 26, 437–452. doi: 10.1016/S0306-9192(01)00013-6
Aday, S., and Aday, M. S. (2020). Impact of COVID-19 on the food supply chain. Food Qual. Saf. 4, 167–180. doi: 10.1093/fqsafe/fyaa024
Amole, T., Augustine, A., Balehegn, M., and Adesogoan, A. T. (2022). Livestock feed resources in the west African Sahel. Agron. J. 114, 26–45. doi: 10.1002/agj2.20955
Atkinson, A. B. (1995). Income distribution in OECD countries. Evidence from Luxemburg income study.
Ayanlade, A., and Radeny, M. (2020). COVID-19 and food security in sub-Saharan Africa: implications of lockdown during agricultural planting seasons. NPJ Sci. Food 4, 13–16. doi: 10.1038/s41538-020-00073-0
Balié, J., Diallo, F., and Mas Aparisi, A. (2013). Analyse des incitations et penalisations pour le mil et le sorgho au Mali.
Benjaminsen, T. A., Aune, J. B., and Sidibé, D. (2010). A critical political ecology of cotton and soil fertility in Mali. Geoforum 41, 647–656. doi: 10.1016/j.geoforum.2010.03.003
Berthé, B. (2020). "Pr Baba Berthé, PDG de la CMDT: «C’est une année vraiment catastrophique»", (ed.) Essor. (Bamako: Malijet).
Blanchard, M. (2010). Gestion de la fertilité des sols et rôle du troupeau dans les systèmes coton-céréales-élevage au Mali-Sud, savoirs techniques locaux et pratiques d'intégration agriculture élevage. Thèse de doctorat: Science de l'univers et environnement Thesis, Université Paris-Est Créteil Val-de-Marne.
Bosma, R., Bos, M., Kante, S., Kebe, D., and Quak, W. (1999). The promising impact of ley introduction and herd expansion on soil organic matter content in southern Mali. Agric. Syst. 62, 1–15. doi: 10.1016/S0308-521X(99)00038-4
Britten, P., Marcoe, K., Yamini, S., and Davis, C. (2006). Development of food intake patterns for the MyPyramid food guidance system. J. Nutr. Educ. Behav. 38, S78–S92. doi: 10.1016/j.jneb.2006.08.007
Bui, T. N., Nguyen, A. H., Le, T. T. H., Nguyen, V. P., Le, T. T. H., Tran, T. T. H., et al. (2021). Can a short food supply chain create sustainable benefits for small farmers in developing countries? An exploratory study of Vietnam. Sustainability 13:2443. doi: 10.3390/su13052443
Camara, M. (2015). Atouts et limites de la filière coton au Mali. Thèse de doctorat en Sciences Économiques Thesis, Université de Toulon.
CMDT (2018). Compagnie Malienne pour le Développement des Textiles: Nos zones d’intervention. Compagnie Malienne pour le Développement des Textiles (CMDT). Available at: https://www.cmdt-mali.net/index.php/nos-zones-d-interventions.html (Accessed July 14, 2022).
CMDT (2022). Compagnie Malienne pour le Développement des Textiles: Production agricoles [Online]. Bamako: Compagnie Malienne pour le Développement des Textiles (CMDT). Available at: https://www.cmdt-mali.net/index.php/activites/activites-agronomiques/production-agricole.html (Accessed July 14, 2022).
Corniaux, C., Alary, V., Gautier, D., and Duteurtre, G. (2012). Producteur laitier en Afrique de l'ouest: Une modernité rêvée par les techniciens à l'épreuve du terrain. Autrepart N° 62, 17–36. doi: 10.3917/autr.062.0017
Coulibaly, J. Y. (2011). Diversification or cotton recovery in the Malian cotton zone: Effects on households and women. Doctor of Philosophy PhD thesis, Purdue University.
CRISIS24 (2020). Mali_ Authorities confirm first COVID-19 cases March 25 /update 2. Available at: https://crisis24.garda.com/alerts/2020/03/mali-authorities-confirm-first-covid-19-cases-march-25-update-2 (Accessed Feb 07, 2022).
Dal Santo, E., and van der Heide, E. J. (2018). Escalating complexity in regional conflicts: connecting geopolitics to individual pathways to terrorism in Mali. Afr. Secur. 11, 274–291. doi: 10.1080/19392206.2018.1505232
Darnhofer, I. (2014). Resilience and why it matters for farm management. Eur. Rev. Agric. Econ. 41, 461–484. doi: 10.1093/erae/jbu012
De Ridder, N., Sanogo, O. M., Rufino, M. C., van Keulen, H., and Giller, K. E. (2015). Milk: the new white gold? Milk production options for smallholder farmers in southern Mali. Animal 9, 1221–1229. doi: 10.1017/S1751731115000178
DfID, U. (2007). Sustainable livelihoods guidance sheets. UK DFID Department for International Development London.
Dissa, A., Bijman, J., Slingerland, M., Sanogo, O. M., Giller, K. E., and Descheemaeker, K. (2021). Growing cotton to produce food: unravelling interactions between value chains in southern Mali. Dev. Policy Rev. 40:e12605. doi: 10.1111/dpr.12605
Dorward, A., Anderson, S., Nava Bernal, Y., Pattison, J., Paz, R., Rushton, J., et al. (2005). A guide to indicators and methods for assessing the contribution of livestock keeping to livelihoods of the poor.
Droy, I., Bélières, J.-F., and Bidou, J. E. (2012). Entre Crise et Rebond: Questions Autour de la Durabilité des Systèmes de Production Cotonniers au Mali. Eur. J. Dev. Res. 24, 491–508. doi: 10.1057/ejdr.2012.12
Edmonds, B., Bachelier, B., and Lançon, J. (2020). “Potential impacts of COVID-19 on African cotton sectors” in The ICAC RECORDER (Washington DC: International Cotton Advisory Committee).
Falconnier, G. N., Descheemaeker, K., Mourik, T. A. V., and Giller, K. E. (2016). Unravelling the causes of variability in crop yields and treatment responses for better tailoring of options for sustainable intensification in southern Mali. Field Crop Res. 187, 113–126. doi: 10.1016/j.fcr.2015.12.015
Falconnier, G. N., Descheemaeker, K., Traore, B., Bayoko, A., and Giller, K. E. (2018). Agricultural intensification and policy interventions: exploring plausible futures for smallholder farmers in southern Mali. Land Use Policy 70, 623–634. doi: 10.1016/j.landusepol.2017.10.044
Falconnier, G. N., Descheemaeker, K., Van Mourik, T. A., Adam, M., Sogoba, B., and Giller, K. E. (2017). Co-learning cycles to support the design of innovative farm systems in southern Mali. Eur. J. Agron. 89, 61–74. doi: 10.1016/j.eja.2017.06.008
Falconnier, G. N., Descheemaeker, K., Van Mourik, T. A., Sanogo, O. M., and Giller, K. E. (2015). Understanding farm trajectories and development pathways: two decades of change in southern Mali. Agric. Syst. 139, 210–222. doi: 10.1016/j.agsy.2015.07.005
Generoso, R. (2015). How do rainfall variability, food security and remittances interact? The case of rural Mali. Ecol. Econ. 114, 188–198. doi: 10.1016/j.ecolecon.2015.03.009
Giller, K. E., Delaune, T., Silva, J. V., van Wijk, M., Hammond, J., Descheemaeker, K., et al. (2021). Small farms and development in sub-Saharan Africa: farming for food, for income or for lack of better options? Food Secur. 13, 1431–1454. doi: 10.1007/s12571-021-01209-0
Giller, K. E., Tittonell, P., Rufino, M. C., van Wijk, M. T., Zingore, S., Mapfumo, P., et al. (2011). Communicating complexity: integrated assessment of trade-offs concerning soil fertility management within African farming systems to support innovation and development. Agric. Syst. 104, 191–203. doi: 10.1016/j.agsy.2010.07.002
Goswami, R., Roy, K., Dutta, S., Ray, K., Sarkar, S., Brahmachari, K., et al. (2021). Multi-faceted impact and outcome of COVID-19 on smallholder agricultural systems: integrating qualitative research and fuzzy cognitive mapping to explore resilient strategies. Agric. Syst. 189:103051. doi: 10.1016/j.agsy.2021.103051
Huet, E., Adam, M., Giller, K., and Descheemaeker, K. (2020). Diversity in perception and management of farming risks in southern Mali. Agric. Syst. 184:102905. doi: 10.1016/j.agsy.2020.102905
INSTAT (2020). Note d’information sur le produit intérieur brut (PIB) trimestriel. résultats rrovisoires du deuxième trimestre 2020 [Online]. Institut National de la Statistique. Available at: https://www.instat-mali.org/laravel-filemanager/files/shares/pub/pibmali2t20_pub.pdf.
International Monetary Fund (2020). "Mali_ Requests for Disbursement Under the Rapid Credit Facility and Rephasing of Access Under the Extended Credit Facility Arrangement-Press Release; Staff Report; and Statement by the Executive Director for Mali", in: IMF Staff Country Reports. (ed.) A. Dept.
IPC (2020). "COMMUNIQUE DE PRESSE: prix d'achat du coton et prix des engrais pour la campagne 2020–2021 ". (Bamako: Interprofession du Coton du Mali, 07 juin 2020).
Ivanov, D. (2020). Predicting the impacts of epidemic outbreaks on global supply chains: a simulation-based analysis on the coronavirus outbreak (COVID-19/SARS-CoV-2) case. Transp. Res. E Logist. Transp. Rev. 136:101922. doi: 10.1016/j.tre.2020.101922
Jolliffe, D., and Prydz, E. B. (2016). Estimating international poverty lines from comparable national thresholds. J. Econ. Inequal. 14, 185–198. doi: 10.1007/s10888-016-9327-5
Kanté, S. (2001). Gestion de la fertility des sols par classe d'exploitation au Mali. PhD thesis, Wageningen University & Research.
Koné, Y., Thériault, V., Kergna, A. O., and Smale, M. (2019). La Subvention Des Engrais Au Mali_ Origines, Contexte Et Evolution. East Lansing, Michigan: Michigan State University.
Laris, P., Foltz, J. D., and Voorhees, B. (2015). Taking from cotton to grow maize: the shifting practices of small-holder farmers in the cotton belt of Mali. Agric. Syst. 133, 1–13. doi: 10.1016/j.agsy.2014.10.010
Makate, C., Wang, R., Makate, M., and Mango, N. (2016). Crop diversification and livelihoods of smallholder farmers in Zimbabwe: adaptive management for environmental change. Springerplus 5, 1–18. doi: 10.1186/s40064-016-2802-4
Marenya, P., Nkonya, E., Xiong, W., Deustua, J., and Kato, E. (2012). Which policy would work better for improved soil fertility management in sub-Saharan Africa, fertilizer subsidies or carbon credits? Agric. Syst. 110, 162–172. doi: 10.1016/j.agsy.2012.04.004
Middendorf, B. J., Faye, A., Middendorf, G., Stewart, Z. P., Jha, P. K., and Prasad, P. V. (2021). Smallholder farmer perceptions about the impact of COVID-19 on agriculture and livelihoods in Senegal. Agric. Syst. 190:103108. doi: 10.1016/j.agsy.2021.103108
Mthembu, B. E., Mkhize, X., and Arthur, G. D. (2022). Effects of COVID-19 pandemic on agricultural food production among smallholder farmers in northern Drakensberg areas of Bergville, South Africa. Agronomy 12:531. doi: 10.3390/agronomy12020531
Nchanji, E. B., and Lutomia, C. K. (2021). COVID-19 challenges to sustainable food production and consumption: future lessons for food systems in eastern and southern Africa from a gender lens. Sustain. Prod. Consum. 27, 2208–2220. doi: 10.1016/j.spc.2021.05.016
Nchanji, E. B., Lutomia, C. K., Chirwa, R., Templer, N., Rubyogo, J. C., and Onyango, P. (2021). Immediate impacts of COVID-19 pandemic on bean value chain in selected countries in sub-Saharan Africa. Agric. Syst. 188:103034. doi: 10.1016/j.agsy.2020.103034
Ollenburger, M., Crane, T., Descheemaeker, K., and Giller, K. E. (2018). Are farmers searching for an African green revolution? Exploring the solution space for agricultural intensification in southern Mali. Exp. Agric. 55, 288–310. doi: 10.1017/s0014479718000169
OMA and WFP (2021). Bulletin de conjoncture: bulletin d’analyse prospective du marché agricole juin 2021. Bamako: Observatoire du marché agricole et Programme alimentaire mondiale. Available at: https://fscluster.org/mali/document/oma-bulletin-danalyse-prospective-du.
Ozili, P. K., and Arun, T. (2020). Spillover of COVID-19: impact on the global economy. Available at: SSRN 3562570.
Powell, J. M., Pearson, R. A., and Hiernaux, P. H. (2004). Crop–livestock interactions in the west African drylands. Agron. J. 96, 469–483. doi: 10.2134/agronj2004.4690
Rapid Country Assessment: Mali (2020). The impact of COVID-19 on the food system. Wageningen: Wageningen University & Research and Royal Tropical Institute.
Rietveld, A. (2009). Livelihood strategies in a globalizing world. MSc Thesis, Rural Sociology and Plant Production Systems, Wageningen University & Research.
Ripoche, A., Crétenet, M., Corbeels, M., Affholder, F., Naudin, K., Sissoko, F., et al. (2015). Cotton as an entry point for soil fertility maintenance and food crop productivity in savannah agroecosystems–evidence from a long-term experiment in southern Mali. Field Crop Res. 177, 37–48. doi: 10.1016/j.fcr.2015.02.013
Soumaré, M. (2008). Dynamique et durabilite des systèmes agraires à base de coton au Mali. Thèse de Doctorat en Géographie Humaine, Economique et Régionale Thesis, Université de Paris X Nanterre.
Soumaré, M., Bélières, J.-F., Passouant, M., and Sidibé, M. (2018). “Integration into international markets of cotton family farms in Mali” in Diversity of family farming around the world. quae ed (Springer), 43–59.
Tefft, J. (2010). “Mali’s white revolution: Smallholder cotton, 1960–2006,” in Successes in African agriculture: Lessons for the future, eds. S. Haggblade and P.B.R. Hazell. (Baltimore: The Johns Hopkins University Press for the International Food Policy Research Institute), 113–162.
Tendall, D. M., Joerin, J., Kopainsky, B., Edwards, P., Shreck, A., Le, Q. B., et al. (2015). Food system resilience: defining the concept. Glob. Food Sec. 6, 17–23. doi: 10.1016/j.gfs.2015.08.001
Theriault, V., Serra, R., and Sterns, J. A. (2013). Prices, institutions, and determinants of supply in the Malian cotton sector. Agric. Econ. 44, 161–174. doi: 10.1111/agec.12001
Theriault, V., Smale, M., and Assima, A. (2018). The Malian fertiliser value chain post-subsidy: an analysis of its structure and performance. Dev. Pract. 28, 242–256. doi: 10.1080/09614524.2018.1421145
Theriault, V., Tschirley, D., and Maredia, M. (2021). The effects of COVID-19 on food security in urban and rural Mali. Michigan State University: Policy Research Note.
Thuijsman, E. S., den Braber, H. J., Andersson, J. A., Descheemaeker, K., Baudron, F., López-Ridaura, S., et al. (2022). Indifferent to difference? Understanding the unequal impacts of farming technologies among smallholders. A review. Agron. Sustain. Dev. 42, 1–16. doi: 10.1007/s13593-022-00768-6
Traoré, B., Corbeels, M., van Wijk, M. T., Rufino, M. C., and Giller, K. E. (2013). Effects of climate variability and climate change on crop production in southern Mali. Eur. J. Agron. 49, 115–125. doi: 10.1016/j.eja.2013.04.004
Traoré, B., van Wijk, M. T., Descheemaeker, K., Corbeels, M., Rufino, M. C., and Giller, K. E. (2014). Evaluation of climate adaptation options for Sudano-Sahelian cropping systems. Field Crop Res. 156, 63–75. doi: 10.1016/j.fcr.2013.10.014
Tröster, B., and Küblböck, K. (2020). Unprecedented but not unpredictable: effects of the COVID-19 crisis on commodity-dependent countries. Eur. J. Dev. Res. 32, 1430–1449. doi: 10.1057/s41287-020-00313-9
Urruty, N., Tailliez-Lefebvre, D., and Huyghe, C. (2016). Stability, robustness, vulnerability and resilience of agricultural systems. A review. Agron. Sustain. Dev. 36, 1–15. doi: 10.1007/s13593-015-0347-5
Vanlauwe, B., Descheemaeker, K., Giller, K. E., Huising, J., Merckx, R., Nziguheba, G., et al. (2015). Integrated soil fertility management in sub-Saharan Africa: unravelling local adaptation. Soil 1, 491–508. doi: 10.5194/soil-1-491-2015
Verma, J. (2015). Repeated measures design for empirical researchers. New Jersey and Canada simultaneously: John Wiley & Sons.
Keywords: food self-sufficiency, income, farm diversity, institutional shock, fertilizer, cereals
Citation: Dissa A, Slingerland M, Giller KE and Descheemaeker K (2024) Effects of the COVID-19 induced cotton crisis on agricultural production and livelihoods of smallholders in southern Mali. Front. Sustain. Food Syst. 7:1269355. doi: 10.3389/fsufs.2023.1269355
Edited by:
Gideon Kruseman, Alliance Bioversity International and CIAT, FranceReviewed by:
Jeffrey Alwang, Virginia Tech, United StatesPatricia Urteaga-Crovetto, Pontifical Catholic University of Peru, Peru
Copyright © 2024 Dissa, Slingerland, Giller and Descheemaeker. This is an open-access article distributed under the terms of the Creative Commons Attribution License (CC BY). The use, distribution or reproduction in other forums is permitted, provided the original author(s) and the copyright owner(s) are credited and that the original publication in this journal is cited, in accordance with accepted academic practice. No use, distribution or reproduction is permitted which does not comply with these terms.
*Correspondence: Arouna Dissa, ZGlzc2Fyb3VuYUB5YWhvby5mcg==