- 1Department of Food Science and Technology, Virginia Tech, Blacksburg, VA, United States
- 2Department of Food Science and Human Nutrition, Citrus Research and Education Center, University of Florida, Lake Alfred, FL, United States
- 3School of Plant and Environmental Sciences, Virginia Tech, Blacksburg, VA, United States
Soil can be a route for contamination of fresh fruits and vegetables. While growers routinely manage soil nutrient levels, little research exists on the synergistic or antagonistic effects of soil nutrients on foodborne pathogens. Data on foodborne pathogen prevalence in unamended soils is also relatively limited in literature. This study evaluated foodborne pathogen prevalence (Salmonella, Listeria monocytogenes) and concentration of indicator bacteria (total coliforms, generic Escherichia coli) in agricultural soils, and characterized associations between soil properties (e.g., macro- and micro-nutrient levels) and each microbial target. Three Virginia produce farms, representing different regions and soil types, were sampled four times over 1 year (October 2021–November 2022). For each individual farm visit, composite soil samples were collected from 20 sample sites (25 m2) per farm per visit for microbial and nutrient analysis (n = 240). Samples (25 g) were processed for Listeria spp. and Salmonella using a modified FDA BAM method; samples (5 g) were enumerated for generic E. coli and total coliforms (TC) using Petrifilm. Presumptive Listeria spp. and Salmonella isolates were confirmed by PCR using the sigB and invA genes, respectively. Soil nutrients from each sample were tested and evaluated for their association with each microbial target by Bayesian Mixed Models. Salmonella prevalence was 4.2% (10/240), with 90% (9/10) recovered on Farm C. Listeria spp. and L. monocytogenes prevalence were 10% (24/240) and 2.5% (6/240), respectively. When samples were positive for generic E. coli (107/240), the average concentration was 1.53 ± 0.77 log10 CFU/g. Soil pH was positively associated with L. monocytogenes [Odds Ratio (OR) = 5.5] and generic E. coli (OR = 4.9) prevalence. There was no association between Salmonella prevalence and any evaluated factor; however, Salmonella was 11.6 times more likely to be detected on Farm C, compared to other farms. Results show pathogen prevalence was relatively low in unamended soils, and that factors influencing prevalence and concentration varied by microbial target and farm.
Introduction
In the last two decades, fresh produce has remained a major vehicle associated with foodborne outbreaks and recalls (Lynch et al., 2009; Callejón et al., 2015; Wadamori et al., 2017; Carstens et al., 2019). For example, a large retail grocery market voluntarily recalled three micro greens, sweet pea leaves, and cat grass products when the supplier found the soil in which these products were grown tested positive for Salmonella (Food and Drug Administration, 2022). While no illnesses were associated with this voluntary recall, due to products being grown in contaminated soil, there was the potential for Salmonella contamination (Food and Drug Administration, 2022).
Managing the risk of produce contamination from soil is complicated due to the ability of foodborne pathogens to survive in soil for extended periods. Studies have demonstrated that Salmonella (Chandler and Craven, 1980; Holley et al., 2006; You et al., 2006; Underthun et al., 2018; Jechalke et al., 2019; Bardsley et al., 2021) and L. monocytogenes (Jiang et al., 2004; McLaughlin et al., 2011) can survive in agricultural soils for up to 1 year. For instance, Salmonella persisted in amended soils for 129 days, with survival differing by soil type (clay-loam > sandy-loam) and irrigation regimen (weekly > daily) (Bardsley et al., 2021). Factors including soil properties (e.g., moisture), meteorological events (e.g., rainfall), and management practices (e.g., tilling) have been shown to influence the likelihood of detection and survival of foodborne pathogens in soils (Danyluk et al., 2008; Ivanek et al., 2009; McLaughlin et al., 2011; Strawn et al., 2013a; Park et al., 2014; Weller et al., 2015; Bardsley et al., 2021; Ramos et al., 2021). For example, a 2013 study in New York (United States) showed rainfall 72 h prior to sampling increased the likelihood of detecting Salmonella in poorly drained soils (Strawn et al., 2013a). A longitudinal study in California (United States) evaluating four foodborne pathogens (Salmonella, L. monocytogenes, Escherichia coli O157:H7, and non-O157 Shiga-toxin producing E. coli) in amended agricultural soils found that prevalence was pathogen-specific and dependent on biological soil amendment, soil type, environmental conditions, and region (Ramos et al., 2021).
Growers routinely test agricultural soil for macro- and micro-nutrients, and other soil properties, to gather data to assist in nutrient management plans and fertility practices (Maguire et al., 2005). A nationwide genomic atlas study observed that the micronutrient molybdenum was associated with increased Listeria spp. prevalence in undisturbed soils from national parks, refuges, or non-agricultural areas (Liao et al., 2021). While previous studies have explored foodborne pathogen prevalence in agricultural soils (Moshtaghi et al., 2003; Rodriguez et al., 2006; Strawn et al., 2013a; Harrand et al., 2020; Ferguson et al., 2023; Murphy et al., 2023), minimal research exists on understanding how soil properties (e.g., macro- and micro-nutrients) impact foodborne pathogen prevalence in unamended agricultural soils. Since the survival and persistence of microorganisms can be impacted by the availability and composition of nutrient sources (Pike et al., 2019); as well as, prior work showing the association between Listeria spp. prevalence and molybdenum, understanding the influence of macro- and micro-nutrients on foodpathogens in soil is of interest.
Indentifying how soil properties interact (synergistically and/or antagonistically) with foodborne pathogens may elucidate information to predict potential risky periods for contamination in agricultural soils (i.e., when soil may test positive for foodborne pathogens). To address research gaps, the present observational study was performed to generate baseline data to inform key soil properties influencing microbial targets in agricultural soils. The aims were to determine the prevalence of Salmonella and L. monocytogenes in unamended agricultural soil, and to investigate if associations exist between soil properties and each microbiological target (Salmonella, L. monocytogenes, generic E. coli, and total coliforms).
Materials and methods
Experimental design
A longitudinal field study was performed on three Virginia farms; each farm represented a different growing region (i.e., Blue Ridge Highlands, Piedmont, Coastal Plain; distance between farms ranged from 338 to 591 km). Within each farm, 20 unique 0.2 ha (5 m x 5 m) sites were selected based on the produce crops grown (e.g., crops that potentially or frequently contact the ground), the feasibility of soil sample collection, and diversity in management practices and topography (Supplementary Table S1). Global Positioning System (GPS) coordinates for each sample site center were recorded and used to ensure the same site was sampled at each visit. Each farm was sampled four times between October 2021 and November 2022 capturing a full growing season. In total, 240 composite soil samples were collected for this study (4 sampling visits, 3 farms, 20 sample sites).
An initial questionnaire, informed by prior field studies (Park et al., 2013; Strawn et al., 2013b) was modified and administered orally to farm management to collect information regarding historical land-use and field-level management practices (e.g., irrigation, worker/equipment presence) for each sample site, prior to the first sampling event on each farm (Supplementary Table S2). Additionally, an observational survey detailing characteristics and management practices was completed for each sampling site during each visit (Supplementary Table S3).
Sample collection and preparation
Sampling was performed following previously described protocols (Strawn et al., 2013a; Weller et al., 2015). Briefly, nitrile gloves were worn for sample collection and changed between each field sample site. Within each sample site, sub-samples from five locations (each of the four corners, and centroid) were collected using sterile scoops (Fisher Scientific, Hampton, NH, United States). Soil was collected from the rhizosphere layer (i.e., the zone surrounding the plant roots), up to approximately 15.2 cm below the surface, and deposited into sterile re-closable 3.79 L storage bags (Fisher Scientific, Hampton, NH). All samples were transported on ice and then stored at 4 ± 2°C for processing within 24 h.
For foodborne pathogen testing, two 25 g composite soil samples were prepared, one for each pathogen, by combining 5 g portions of each of the 5 sub-samples into a sterile filtered Whirl-Pak bag (Nasco, Madison, WI, United States). An additional 1 g from each sub-sample (5 g total) were pooled into a Whirl-Pak bag for enumeration of generic E. coli and total coliforms. Composite samples (150 g) were prepared by combining 30 g portions of each of the five sub-soil samples into a brown paper bag (Fisher Scientific, Hampton, NH, United States). Soil samples were held at 21 ± 2°C until visibly dry (approximately 2 weeks) to determine soil moisture content (Maguire et al., 2005). Air-dried soil samples were shipped and analyzed at Waters Agricultural Laboratories (Camilla, GA, United States) for macro- and micro-nutrients. Samples were analyzed under the Mehlich 3 extraction method (Mehlich, 1984). A list of soil nutrients and properties that were collected (and transformed) can be found in Table 1.
Soil microbial analysis
Listeria detection and isolation was performed using a modified version of the United States Food and Drug Administration’s (FDA) Bacteriological Analytical Manual (BAM) (Hitchins et al., 2022). Briefly, soil samples were diluted 1:10 by adding 225 mL of buffered Listeria enrichment broth (BLEB; Oxoid, Basingstoke, Hampshire) to each sample (25 g) and incubated at 30 ± 2°C for 4 h. After 4 h of incubation, Listeria selective enrichment supplement (Oxoid, Basingstoke, Hampshire) was added to each sample and incubated at 30 ± 2°C. At 24 and 48 h of incubation, 50 μL of each enrichment was streaked for isolation onto Modified Oxford Agar (MOX; Becton Dickinson, Franklin Lakes, NJ, United States) and L. monocytogenes Plating Medium (LMPM; R&F Products, Downers Grove, IL, United States) and incubated at 30 ± 2°C for 48 h and 35 ± 2°C for 48 h, respectively. Up to three presumptive Listeria colonies per time and plating agar combination (e.g., MOX at 24 h and LMPM at 48 h) were sub-streaked onto reversed agar combinations (e.g., MOX plated onto LMPM and LMPM plated onto MOX) and incubated for 48 h at each agar’s respective temperatures. Presumptive positive Listeria colonies were confirmed by a sigB PCR assay and species identified by partial sigB gene sequencing, as previously described (Nightingale et al., 2007).
Salmonella detection and isolation were performed using a modified version of the procedure outlined in the United States FDA BAM (Andrews et al., 2011). Briefly, soil samples were diluted 1:10 by adding 225 mL of Buffered Peptone Water (BPW) to each 25 g composited sample. Samples were incubated at 35 ± 2°C for 24 h. Following incubation, 1.0 and 0.1 mL were transferred to 9.0 and 9.9 mL of Tetrathionate (TT; Oxoid; Basingstoke, Hampshire) and Rappaport Vassiliadis (RV: Oxoid, Basingstoke, Hampshire) broth, respectively. Enrichment broths were incubated at 35 ± 2°C and 42 ± 2°C for 24 and 48 h, respectively. From each broth, 50 μL was placed onto Xylose Lysine Tergitol-4 (XLT-4; Neogen, Lansing, MI, United States) and Hektoen Enteric (HE; Neogen Lansing, MI, United States) agars and incubated at 35 ± 2°C for 24 h. Up to three presumptive Salmonella colonies per enrichment and plating agar combination (e.g., TT-XLT-4, RV-XLT-4) were sub-streaked onto reversed agar combinations (e.g., XLT-4 plated onto HE and HE plated onto XLT-4) and incubated at 35 ± 2°C for 24 h. Presumptive positive Salmonella colonies were confirmed by a PCR assay for the invA gene (Kim et al., 2007). One isolate per Salmonella-positive sample was serotyped by Kauffmann–White classification at the National Veterinary Laboratory Services (Ames, IA, United States).
Generic E. coli and total coliform enumeration were performed as previously described (Berry and Miller, 2005). Briefly, a 5 g composite sample was placed in a filtered Whirl-Pak bag diluted in 45 mL of 2% BPW. Following serial dilution, 1 mL of each dilution was plated onto E. coli/coliform Petrifilm (3 M, Saint Paul, MN, United States) in duplicate. Petrifilm was incubated at 37 ± 2°C for 48 h, and anlyzed according to manufacturers instructions to enumerate E. coli and total coliforms. Counts were log transformed (log10 CFU/g).
Meteorological data collection
Meteorological data including average daily air temperature (°C), total precipitation (mm), and relative humidity (%) for the 5 days prior to sampling were obtained for each farm and sampling visit. Meteorological data were collected from the nearest Virginia Agricultural Research and Extension Center to each farm using the Virginia Tech WeatherSTEM platform.1
Statistical analysis
Analyzes were performed in RStudio version 4.2.2 (R Foundation for Statistical Computing, Vienna, Austria). Descriptive statistics were performed on all variables to characterize trends across farms and sampling visits, and summary statistics were calculated for soil nutrient data. Extreme outliers in soil nutrient data were identified using the Rosner Test (Rosner, 1975, 1983), and biologically implausible outliers were removed. Due to strong skew, some soil properties were log10 transformed (Table 1).
Bayesian mixed models were implemented using the brms package (Bürkner, 2017a,b) to identify factors associated with: (i) the isolation of Listeria spp., L. monocytogenes, and Salmonella; (ii) if log10 generic E. coli levels were above versus below the limit of detection; and (iii) E. coli concentration in samples with enumerable levels. For the logistic models, a Bernoulli distribution with a logit link function was used. For the linear models, an identity link was used. The model outcome was a function of a random effect of site nested in farm, a random effect of month, and a fixed effect of year in addition to a fixed effect for the factor of interest (Supplementary Table S4).
All models were fit using the brms package with uninformative priors, 5,001 iterations per chain, thinning set to 10, and 3 chains (Bürkner, 2017a,b). The Maximum a posteriori estimate (MAP) is the most probable value of the posterior distribution for the effect estimate for the linear models and odds ratio for the logistic models. The presence and strength of an association between the outcome and factor of interest were assessed using the probability of direction (PD), and region of practical equivalence (ROPE) values (Makowski et al., 2019a,b). In addition to assessing associations between soil microbial quality and environmental parameters, models were also implemented to determine if there were substantial differences in soil microbial quality between farms. Since there were no Listeria spp. positive samples from Farm C, and no Salmonella positive samples from Farm A, models were run (i) using data from all farms, and (ii) using only data from farms where there was at least one positive sample for the microbial target. A factor was considered associated with the odds of detecting a microbial target if ROPE was ≤0.025 and the probability of direction (PD) was ≥0.95; for all factors where these thresholds were not met, this was considered as failing to find evidence of an association.
Results
Soil analysis and meteorological data
For each farm and sampling visit, values for calcium (mg/kg), magnesium (mg/kg), phosphorous (mg/kg), potassium (mg/kg), sodium (mg/kg), sulfur (mg/kg), aluminum (mg/kg), copper (mg/kg), iron (mg/kg), manganese (mg/kg), molybdenum (mg/kg), zinc (mg/kg), total nitrogen (%), total carbon (%), organic matter (%), and pH were assessed. Summary statistics for all soil nutrients and properties across farms and sampling visits can be found in Table 1. Furthermore, values for air temperature (°C), dew point (°C), relative humidity (%), and cumulative rainfall (mm) for the 5 days prior to each sampling visit by farm can be found in Supplementary Table S5, or soil type in Supplementary Table S7.
Foodborne pathogen and indicator bacteria prevalence
The overall prevalence of Listeria spp. and L. monocytogenes in soil across the three farms were 10.0% (24/240) and 2.5% (6/240), respectively (Table 2). Farm B had the highest prevalence of both Listeria spp. (18.8%; 15/80) and L. monocytogenes (6.3%; 5/80), while no samples from Farm C were positive for Listeria. On Farm B, the five soil samples positive for L. monocytogenes represented four unique sampling sites, with one site testing positive during two separate sampling visits (visit 1 and 4; both during the fall separated by approximately 1 year). The overall prevalence of Salmonella in soil across the three farms was 4.2% (10/240; Table 2). Ninety percent (9/10) of Salmonella-positive soil samples were recovered on Farm C, with the majority recovered during the first sampling visit (6 of 9). These nine Salmonella-positive soil samples from Farm C represented eight unique sampling sites, with one site testing positive during two separate sampling visits (visit 1 and 2). The site with repeated Salmonella positive soil samples was directly adjacent to a pond used for irrigation. Irrigation on Farm C was weather dependent and applied by drip under plastic mulch. One soil sample was positive for Salmonella from Farm B during sampling visit 4. Farm A yielded no Salmonella- positive samples across all four visits.
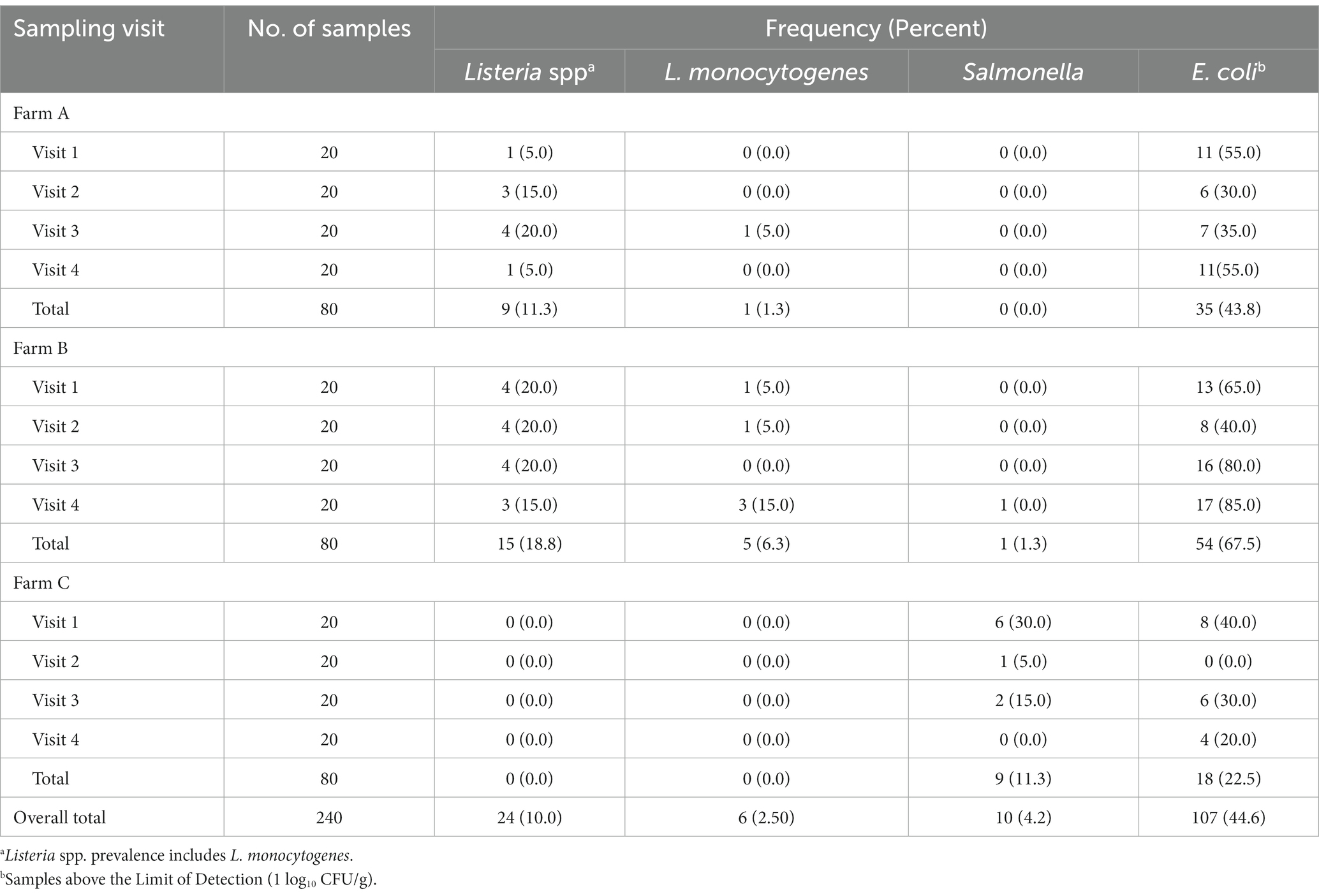
Table 2. Prevalence of Listeria spp., L. monocytogenes, Salmonella, and Escherichia coli by farm and visit.
Generic E. coli levels fell below the lower limit of detection (1 log10 CFU/g) in over half of the soil samples collected in this study (55%; 133/240). The greatest prevalence of generic E. coli was found from soils collected on Farm B (67.5%; 54/80); followed by Farm A (43.8%; 35/80) and Farm C (22.5%; 18/80; Table 2). For the 107 soil samples where generic E. coli were above the limit of detection, the average concentration across all farms and sampling visits was 1.53 ± 0.77 log10 CFU/g (Supplementary Table S6). While not significantly different by farm, Farm B had the highest generic E. coli levels among positive samples at 1.61 ± 0.86 log10 CFU/g, followed by Farm A (1.48 ± 0.70 log10 CFU/g) and Farm C (1.43 ± 0.62 log10 CFU/g), respectively. Total coliform levels were detectable in all 240 samples collected and had an average concentration of 4.21 ± 0.94 log10 CFU/g (Supplementary Table S6). Similar to generic E. coli, Farm B had the highest total coliform levels (4.66 ± 0.63 log10 CFU/g), followed by Farm A (4.55 ± 0.78 log10 CFU/g) and Farm C (3.42 ± 0.85 log10 CFU/g), respectively.
Associations between foodborne pathogens and factors
Since no soil sample from Farm C was positive for Listeria, only data from Farms A and B were used when assessing how odds of isolating Listeria spp. and L. monocytogenes differed across farms. There was no evidence (PD = 0.92; ROPE =0.11) of an association between Listeria spp. and farms when using data from Farms A and B. Only two factors evaluated in the present study were substantially associated with a change in odds of Listeria spp. detection (PD ≥ 0.95 and ROPE ≤0.025); (i) if farm equipment and (ii) if farm workers were present in the field during sampling (Table 3). When farm equipment (e.g., tillage equipment, tractors) and farm workers (employees of the operation) were present during the time of sampling, the odds of detecting Listeria spp. was approximately 9 [MAP = 8.98, 95% Credibility Interval (CrI) =1.63, 38.75] and 8 (MAP = 7.72, CrI = 2.03, 64.17) times more likely, respectively (Table 3). As for L. monocytogenes, soil pH was the only evaluated factor substantially associated with a change in L. monocytogenes detection odds, with each one-unit increase in pH, there was a 5.5 times increase in the odds of L. monocytogenes detection (MAP = 5.48, CrI = 0.75, 345.39; Table 3).
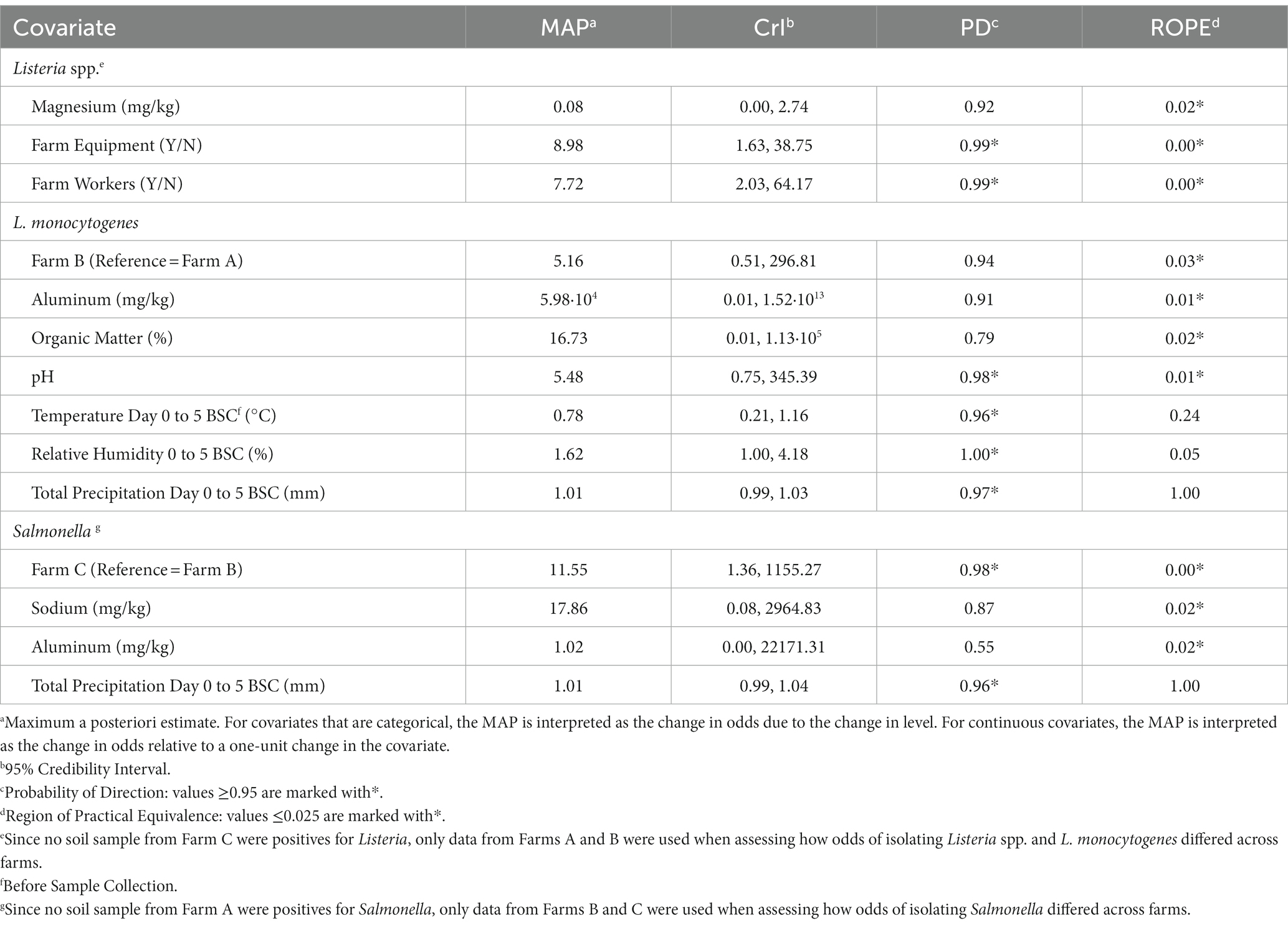
Table 3. Factors associated with the odds of isolating Listeria spp., L. monocytogenes, and Salmonella according to Bayesian mixed models.
Since no Salmonella was detected on Farm A, only data from Farms B and C were used when assessing the odds of detection. Salmonella was 11.6 (CrI = 1.36, 1155.27) times more likely to be recovered on Farm C, compared to Farm B (Table 3). The average concentration of generic E. coli in samples positive and negative for Salmonella were 1.35 ± 0.73 and 1.54 ± 0.78 log10 CFU/g, respectively. There was no evidence of an association between the odds of Salmonella detection and all other factors considered in this study (e.g., weather, soil nutrients, management factors), including both the presence and concentration of generic E. coli or total coliforms.
Odds of indicator bacteria were associated with soil nutrients and properties
Since E. coli levels fell below the lower limit of detection (1 log10 CFU/g) for 55% (133/240) of soil samples, a hurdle model was implemented in order to separately understand factors associated with the presence and concentration of generic E. coli. Odds of generic E. coli detection differed by farm, with the odds of detection being 0.29 and 0.05 times less likely in Farm A and Farm C, compared to Farm B (Table 4). However, when the concentration of E. coli was examined, farm did not substantially impact levels (PD: 0.69–0.79; ROPE: 0.15–0.24). Three soil nutrients and two soil properties examined in this study were substantially associated with increased odds of detecting generic E. coli: calcium (MAP = 4.18, CrI = 0.89, 29.10), iron (MAP = 3.44, CrI = 0.93, 45.29), magnesium (MAP = 7.12, CrI = 1.53, 104.32), organic matter (MAP = 7.81, CrI = 0.75, 97.69), and pH (MAP = 4.87, CrI = 2.31, 12.22; Table 4). Organic matter had the greatest effect on generic E. coli detection with a one-unit change in organic matter (%) resulting in a 7.81 increase in odds of generic E. coli detection. Three soil nutrients were substantially associated with decreased levels of generic E. coli: iron (MAP = 1.71, CrI = −3.22, −0.15), sodium (MAP = −1.31, CrI = −2.21, −0.30), and sulfur (MAP = −0.73, CrI = −1.66, −0.04). A one-unit change in iron (mg/kg) resulted in the largest decrease in generic E. coli levels with a − 1.71 log10 CFU/g change (Table 4).
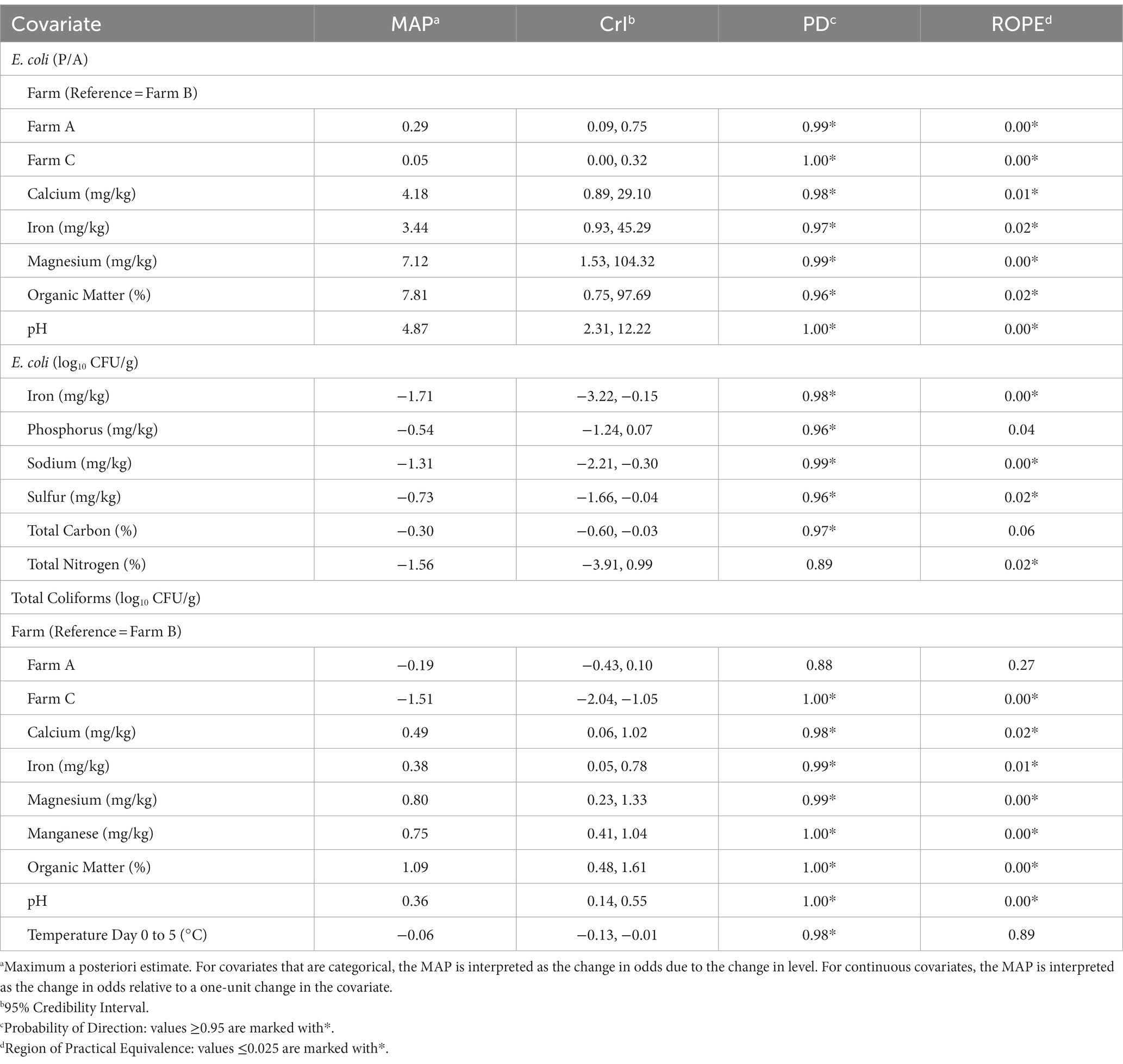
Table 4. Factors associated with odds of generic E. coli detection and levels of generic E. coli and total coliforms according to Bayesian mixed models.
Total coliform concentrations were 1.51 log10 CFU/g lower on Farm C, as compared to Farm B (CrI = −2.04, −1.05; Table 4). No substantial difference in total coliform levels were observed between Farm B and Farm A (PD = 0.88, ROPE = 0.27). Four soil nutrients and two soil properties were substantially positively associated with total coliform levels: calcium (MAP = 0.49, CrI = 0.06, 1.02), iron (MAP = 0.38, CrI = 0.05, 0.78), manganese (MAP = 0.75, CrI = 0.41, 1.04), magnesium (MAP = 0.80, CrI = 0.23, 1.33), organic matter (MAP = 1.09, CrI = 0.48, 1.61), and soil pH (MAP = 0.36, CrI = 0.14, 0.55; Table 4). Similar to E. coli detection, organic matter had the greatest effect with a one-unit change in organic matter (%) resulting in an increase in total coliforms of 1.09 log10 CFU/g.
Salmonella serotypes
From the 10 Salmonella-positive samples, five different Salmonella serovars were identified. Salmonella isolates from Farm B (visit 4) were identified as III 42:z10:e,n,x,z15 (Table 5). The remaining Salmonella isolates, all from Farm C, yielded the following serovars: Enteritidis (1), Florida (2), Javiana (3), and Newport (3). S. enteritidis, S. Florida, and S. Javiana were all isolated from visit 1 on Farm C, while S. Newport was only isolated during visits 2 and 3 (summer timeframe).
Discussion
The current study was performed to investigate foodborne pathogen prevalence (Salmonella, Listeria monocytogenes) and concentration of indicator bacteria (total coliforms, generic Escherichia coli) in agricultural soils; as well as, characterize associations between soil properties (e.g., macro- and micro-nutrient levels) and each microbial target. The goal was to understand how certain soil nutrients and/or properties may synergistically and/or antagonistically affect pathogen prevalence and indicator concentrations in soil. The prevalence of Salmonella, Listeria spp., and L. monocytogenes recovered in unamended soils was low, and also similar to a previous study conducted in Virginia, which also found low Salmonella and L. monocytogenes prevalence in the agricultural soils (0.5–1.8%) (Murphy et al., 2023). Interestingly, the prevalence of foodborne pathogens in unamended soils in the study reported here is lower than pathogen prevalence in amended soils reported in previous work (Gu et al., 2019; Hailu et al., 2021; Ferguson et al., 2023; Pires et al., 2023). For example, a previous study in Ohio (United States) noted that pathogen prevalence (E. coli O157, Salmonella, L. monocytogenes, and Campylobacter) was higher in manure-amended soils (84%), compared to non-amended soil samples (15.9%; p < 0.05) on farms (Hailu et al., 2021). Due to the low prevalence of foodborne pathogens in unamended soils, future studies may opt to investigate pathogen associations with soil nutrients and/or properties may utilize laboratory and/or greenhouse based experiments.
Management practices influenced Listeria prevalence while region influenced Salmonella prevalence
When farm equipment (e.g., tillage equipment, tractors, etc.) and farm workers were present in the field at the time of sampling, the odds of isolating Listeria spp. increased substantially. While we failed to find an association between soil disturbances by animal intrusions and Listeria spp. prevalence, these results suggest that soil disturbances by field management practices may impact Listeria spp. prevalence. This aligns with previous studies which have demonstrated that management practices that disturb the soil and allow the subsurface to become exposed increase the probability of microorganism detection (Vivant et al., 2013; Strawn et al., 2013b). In contrast, an additional study found that within an organic field in Maryland (United States), Listeria spp. populations decreased following tilling events (Reed-Jones et al., 2016). However, since tillage has been shown to affect the microbial structure of soil, leading to the loss of total carbon, total nitrogen, and the breakdown of organic matter (Jackson et al., 2003), more research is needed on the effects of soil disturbances on bacterial detection, specifically Listeria.
The study presented here demonstrated that intrastate region influenced Salmonella prevalence in Virginia soils with Salmonella detection more likely to occur in soils from the Coastal Plain region (Farm C). Additionally, the Salmonella serovar isolated from Farm B was not isolated from Farm C. These results suggest that diversity of Salmonella may differ by region; however, this finding may be an artifact of the small number of positive Salmonella samples in the reported study. This is supported by previous work that has shown Salmonella serovar diversity differs across regions, including interstate regions (Gorski et al., 2011; Strawn et al., 2014; Murphy et al., 2023). These findings are of interest as produce grown and packed in the Coastal Plain region of Virginia have been implicated in Salmonella outbreaks associated with fresh produce (Greene et al., 2008; Bell et al., 2015). Previous research in this region of Virginia has also shown repeated isolation of Salmonella from environmental sources including sediment, water, soil, and wildlife (Greene et al., 2008; Gruszynski et al., 2014; Angelo et al., 2015; Bell et al., 2015; Truitt et al., 2018; Gu et al., 2019; Murphy et al., 2023). It has been hypothesized that Salmonella may be introduced to Coastal Plain soils by wildlife within the production environment (Greene et al., 2008; Gruszynski et al., 2014; Bell et al., 2015; Truitt et al., 2018; Gu et al., 2019). A 2013 study conducted within the Coastal Plain region of Virginia revealed that 8.8% (23/262) of wildlife samples were positive for Salmonella, with the majority originating from avian species (65.2%; 15/23) followed by reptiles (30.4%; 7/23) and mammalian species (4.3%; 1/23) (Gruszynski et al., 2014). Surveys in this study collected from Farm C (costal plain region) listed expected animal intrusion from avian, rodent, and reptile species. While all farms in this study mentioned wildlife control measures were in place, it was noted the difficulty to maintain these barriers and/or controls. Therefore, a continued understanding of how regional factors, such as wildlife in the surrounding area, contribute to Salmonella prevalence could be of value to growers when evaluating the risks associated with their production environments.
Increases in pH resulted in higher prevalence of Listeria monocytogenes and generic Escherichia coli and increased concentrations of total coliforms
The prevalence of both L. monocytogenes and generic E. coli; as well as, the concentration of total coliforms were positively associated with increased pH levels. Farm B soil, which had the highest prevalence of both L. monocytogenes and generic E. coli, and the highest concentration of total coliforms, had the highest pH values at 6.34 ± 0.57 (range 5.30–7.60). Conversely, Farm C had the lowest pH values (5.97 ± 0.44), with no L. monocytogenes-positive samples, the lowest generic E. coli prevalence, and the lowest total coliform concentrations. While L. monocytogenes can grow in both neutral and acidic soil conditions (Locatelli et al., 2013; Linke et al., 2014; Ferguson et al., 2023), a previous study conducted across 2 years from 12 areas in Austria found that from 467 soil samples collected, Listeria isolation was significantly associated with soils that had a neutral pH (7.44; 3.43–9.90; Linke et al., 2014). As for E. coli, greater survival rates have been observed in soils with a moderately neutral to alkaline pH (6.0–8.5), compared to acidic (Reddy et al., 1981; Sjogren, 1994; Wang et al., 2014; Emch et al., 2020). In Virginia, soils tend to be acidic and require lime additions every few years as the pH slowly drops, with lime applications based on soil testing with a target pH of 6.2 for most produce fields (Maguire and Heckendorn, 2022). As this practice may contribute to changes in soil pH, more research is needed to better understand the relationship between soil pH, management practices, and pathogen prevalence.
However, one limitation of the study presented here is data was not collected for when fertilization of sites occurred (e.g., when were additions/applications of nitrogen, phosphorous); as well as, information on exact fertilization sources. This additional data could assist with elucidating potential associations between foodborne pathogens and macro- and micro-nutrients. It is known that crops will uptake soil nutrients for their metabolisms (Näsholm et al., 2009; Bindraban et al., 2015); thus understanding how and at what concentrations foodborne pathogens may require macro-and micro-nutrients could assist in understanding survival and persistence behavior within agricultural soils.
Indicator organisms may not be sufficient to predict pathogen prevalence in soil
Total coliforms and generic E. coli are frequently used as indicators for sanitary conditions and the presence of fecal bacteria; however, since both have known ecological and environmental niches other than fecal sources, their presence is not always indicative of fecal contamination (Cox et al., 1988; Luo et al., 2011). Testing for foodborne pathogens is often more labor, time, and cost-intensive, so testing for E. coli and total coliforms is often still beneficial, under certain circumstances (e.g., flooding). Previous studies have investigated the use of indicator bacteria, including generic E. coli and total coliforms, as indicators of foodborne pathogens in soils, and have found varying results (Natvig et al., 2002; Holvoet et al., 2014; Reed-Jones et al., 2016; Hruby et al., 2018; Emch et al., 2020). Results from this study showed E. coli, both prevalence and concentration, and total coliforms were not associated with Salmonella, Listeria spp., or L. monocytogenes prevalence. Therefore, the use of indicator bacteria for predicting times and locations of increased likelihood of pathogen prevalence in soil may not be appropriate. This result may be due to the limited sample size, complexities in soil properties, and/or environmental conditions, but in the present study indicator bacteria were not associated with Salmonella or L. monocytogenes prevalence.
However, the use of indicator organisms for pathogen prediction under specific soil conditions and situations may be useful, such as after a flooding event (Castro-Ibáñez et al., 2015; Bergholz et al., 2016; Callahan et al., 2017). Flooding events pose a large risk for growers both from a food safety, quality, and operational perspective. A previous study found that, following a flooding event, bacteria could be recovered from soils up to 9 m from the threshold of the flood (Callahan et al., 2017), while an additional study observed irrigation water samples collected 1 week after a flooding event had elevated levels of both coliforms and generic E. coli (Castro-Ibáñez et al., 2015). These findings demonstrate a flood event may lead to increased levels of biological contamination in the environment (e.g., soil, irrigation water). Recent flooding events in other major growing regions of the United States have emphasized this risk, attracting attention from the United States FDA; as well as, trade organizations, such as Western Growers Association and the California Leafy Greens Marketing Agreement (LGMA) (California Leafy Greens Marketing Agreement, 2023; Food and Drug Administration, 2023; Timmins, 2023). Specifically, the California LGMA requires soil testing following a flooding event that suggests growers should wait a minimum of 60 days after a flooding event to begin planting and that fecal coliform levels should be <100 MPN/g in soils (California Leafy Greens Marketing Agreement, 2021; California Leafy Greens Marketing Agreement, 2023). While the research presented here demonstrates that routine testing of soils for indicator organisms may not be able to provide insight into pathogen prevalence, it may be helpful for growers in mitigating risks during unforeseen events or conditions (e.g., flooding), or trending/monitoring when indicator organism levels adjust to baseline levels.
Conclusion
The interface between foodborne pathogens and factors influencing agricultural soils within the production environment is complex. The findings of the study showed the prevalence of Salmonella, L. monocytogenes, and generic E. coli was low in unamended agricultural soils, especially compared to previous pathogen prevalence data in amended soils. The recovery of Salmonella and L. monocytogenes varied substantially by region indicating regional produce safety guidance/best practices may be appropriate. Additionally, the concentration of indicator bacteria and Listeria spp. were also impacted by soil nutrients, soil properties and field management practices. While conducting additional soil testing for generic E. coli and other indicator bacteria may be useful tools during catastrophic flooding events, data from this study and others, demonstrated indicator bacteria presence and levels were not sufficient to predict pathogen prevalence in soil. This study also offered insights into how growers may potentially utilize soil testing (a practice already routinely preformed by growers for soil health and fertility) to identify how foodborne pathogens and/or indicator bacteria may be present in agricultural soils.
Data availability statement
The raw data supporting the conclusions of this article will be made available by the authors, without undue reservation.
Author contributions
CC: Data curation, Formal Analysis, Investigation, Writing – original draft, Writing – review & editing. CD: Writing – review & editing, Conceptualization. DW: Writing – review & editing, Formal Analysis, Visualization. CM: Formal Analysis, Writing – review & editing, Data curation, Writing – original draft. AH: Writing – review & editing, Investigation. MP: Writing – review & editing. RB: Writing – review & editing. SR: Writing – review & editing, Conceptualization, Data curation, Investigation. RM: Data curation, Investigation, Writing – review & editing. MD: Writing – review & editing, Conceptualization, Funding acquisition, Methodology. LS: Conceptualization, Funding acquisition, Methodology, Writing – review & editing, Data curation, Formal Analysis, Investigation, Project administration, Resources, Software, Supervision, Validation, Visualization, Writing – original draft.
Funding
The authors declare financial support was received for the research, authorship, and/or publication of this article. Funding for this work was provided by the Specialty Crop Research Initiative Project 2019-51181-30016 through the United States Department of Agriculture (USDA) National Institute of Food and Agriculture, and Virginia Agricultural Experiment Station. Any opinions, findings, conclusions, or recommendations expressed in this publication are those of the author(s) and do not necessarily reflect the view of the USDA.
Acknowledgments
We are grateful for the laboratory assistance of Kim Waterman, Alyssa Rosenbaum, and Emily Wilcox.
Conflict of interest
The authors declare that the research was conducted in the absence of any commercial or financial relationships that could be construed as a potential conflict of interest.
Publisher’s note
All claims expressed in this article are solely those of the authors and do not necessarily represent those of their affiliated organizations, or those of the publisher, the editors and the reviewers. Any product that may be evaluated in this article, or claim that may be made by its manufacturer, is not guaranteed or endorsed by the publisher.
Supplementary material
The Supplementary material for this article can be found online at: https://www.frontiersin.org/articles/10.3389/fsufs.2023.1269117/full#supplementary-material
Footnotes
References
Andrews, W. H., Acobson, A., and Hammack, T. (2011). Bacteriological analytical manual (BAM). Chapter 5 Salmonella. Available at: https://www.fda.gov/food/laboratory-methods-food/bacteriological-analytical-manual-bam-chapter-5-salmonella (Accessed April 20, 2020).
Angelo, K. M., Chu, A., Anand, M., Nguyen, T.-A., Bottichio, L., Wise, M., et al. (2015). Outbreak of Salmonella Newport infections linked to cucumbers–United States, 2014. Morb. Mortal. Wkly Rep. 64:144.
Bardsley, C. A., Weller, D. L., Ingram, D. T., Chen, Y., Oryang, D., Rideout, S. L., et al. (2021). Strain, soil-type, irrigation regimen, and poultry litter influence Salmonella survival and die-off in agricultural soils. Front. Microbiol. 12:590303. doi: 10.3389/fmicb.2021.590303
Bell, R. L., Zheng, J., Burrows, E., Allard, S., Wang, C. Y., Keys, C. E., et al. (2015). Ecological prevalence, genetic diversity, and epidemiological aspects of Salmonella isolated from tomato agricultural regions of the Virginia eastern shore. Front. Microbiol. 6:415. doi: 10.3389/fmicb.2015.00415
Bergholz, P. W., Strawn, L. K., Ryan, G. T., Warchocki, S., and Wiedmann, M. (2016). Spatiotemporal analysis of microbiological contamination in New York state produce fields following extensive flooding from hurricane Irene, august 2011. J. Food Prot. 79, 384–391. doi: 10.4315/0362-028X.JFP-15-334
Berry, E. D., and Miller, D. N. (2005). Cattle feedlot soil moisture and manure content: II. Impact on Escherichia coli O157. J. Environ. Qual. 34, 656–663. doi: 10.2134/jeq2005.0656
Bindraban, P. S., Dimkpa, C., Nagarajan, L., Roy, A., and Rabbinge, R. (2015). Revisiting fertilisers and fertilisation strategies for improved nutrient uptake by plants. Biol. Fertil. Soils 51, 897–911. doi: 10.1007/s00374-015-1039-7
Bürkner, P.-C. (2017a). Advanced Bayesian multilevel modeling with the R package brms. arXiv 2017:11123. doi: 10.48550/arXiv.1705.11123
Bürkner, P.-C. (2017b). Brms: an R package for Bayesian multilevel models using Stan. J. Stat. Softw. 80, 1–28. doi: 10.18637/jss.v080.i01
California Leafy Greens Marketing Agreement (2021). California LGMA commodity specific food safety guidelines. Available at: https://lgma-assets.sfo2.digitaloceanspaces.com/downloads/August-2021-CA-LGMA-Metrics_FINAL-v20211208_A11Y.pdf (Accessed July 26, 2023).
California Leafy Greens Marketing Agreement (2023). California flooding events soil sampling and testing. Available at: https://lgma-assets.sfo2.digitaloceanspaces.com/downloads/wg_science_34114_23_flood-white-paper_prod.pdf (Accessed July 26, 2023).
Callahan, M. T., Micallef, S. A., and Buchanan, R. L. (2017). Soil type, soil moisture, and field slope influence the horizontal movement of Salmonella enterica and Citrobacter freundii from floodwater through soil. J. Food Prot. 80, 189–197. doi: 10.4315/0362-028X.JFP-16-263
Callejón, R. M., Rodríguez-Naranjo, M. I., Ubeda, C., Hornedo-Ortega, R., Garcia-Parrilla, M. C., and Troncoso, A. M. (2015). Reported foodborne outbreaks due to fresh produce in the United States and European Union: trends and causes. Foodborne Pathog. Dis. 12, 32–38. doi: 10.1089/fpd.2014.1821
Carstens, C., Salazar, J. K., and Darkoh, C. (2019). Multistate outbreaks of foodborne illness in the United States associated with fresh produce from 2010-2017. Front. Microbiol. 10:2667. doi: 10.3389/fmicb.2019.02667
Castro-Ibáñez, I., Gil, M. I., Tudela, J. A., and Allende, A. (2015). Microbial safety considerations of flooding in primary production of leafy greens: a case study. Food Res. Int. 68, 62–69. doi: 10.1016/j.foodres.2014.05.065
Chandler, D., and Craven, J. (1980). Relationship of soil moisture to survival of Escherichia coli and Salmonella Typhimurium in soils. Aust. J. Agric. Res. 31, 547–555. doi: 10.1071/AR9800547
Cox, L. J., Keller, N., and Van Schothorst, M. (1988). The use and misuse of quantitative determinations of Enterobacteriaceae in food microbiology. J. Appl. Microbiol. 65, 237S–249S. doi: 10.1111/j.1365-2672.1988.tb04566.x
Danyluk, M., Nozawa-Inoue, M., Hristova, K., Scow, K., Lampinen, B., and Harris, L. (2008). Survival and growth of Salmonella enteritidis PT 30 in almond orchard soils. J. Appl. Microbiol. 104, 1391–1399. doi: 10.1111/j.1365-2672.2007.03662.x
Emch, A. W., Mohamed, H. M., and Waite-Cusic, J. G. (2020). Survival of generic Escherichia coli and Salmonella in oregon’s agricultural soils. J. Soil Water Conserv. 4, 132–140. doi: 10.36959/624/438
Ferguson, M., Hsu, C.-K., Grim, C., Kauffman, M., Jarvis, K., Pettengill, J. B., et al. (2023). A longitudinal study to examine the influence of farming practices and environmental factors on pathogen prevalence using structural equation modeling. Front. Microbiol. 14:1141043. doi: 10.3389/fmicb.2023.1141043
Food and Drug Administration . (2022). Wegmans food markets, Inc. announces voluntary recall of products containing Micro greens, sweet pea leaves, and cat grass because of possible health risk. Available at: https://www.fda.gov/safety/recalls-market-withdrawals-safety-alerts/wegmans-food-markets-inc-announces-voluntary-recall-products-containing-micro-greens-sweet-pea#:~:text=ROCHESTER%2C%20NY%20%2D%20Wegmans%20Food%20Markets,young%20children%2C%20frail%20or%20elderly (Accessed July 24, 2023).
Food and Drug Administration . (2023). Crops harvested from flooded fields intended for animal food: questions and answers. Available at: https://www.fda.gov/animal-veterinary/resources-you/crops-harvested-flooded-fields-intended-animal-food-questions-and-answers (Accessed July 26, 2023).
Gorski, L., Parker, C. T., Liang, A., Cooley, M. B., Jay-Russell, M. T., Gordus, A. G., et al. (2011). Prevalence, distribution, and diversity of Salmonella enterica in a major produce region of California. Appl. Environ. Microbiol. 77, 2734–2748. doi: 10.1128/AEM.02321-10
Greene, S., Daly, E., Talbot, E., Demma, L., Holzbauer, S., Patel, N., et al. (2008). Recurrent multistate outbreak of Salmonella Newport associated with tomatoes from contaminated fields, 2005. Epidemiol. Infect. 136, 157–165. doi: 10.1017/S095026880700859X
Gruszynski, K., Pao, S., Kim, C., Toney, D., Wright, K., Ross, P., et al. (2014). Evaluating wildlife as a potential source of Salmonella serotype Newport (JJPX 01.0061) contamination for tomatoes on the eastern shore of Virginia. Zoonoses Public Health 61, 202–207. doi: 10.1111/zph.12061
Gu, G., Strawn, L. K., Zheng, J., Reed, E. A., and Rideout, S. L. (2019). Diversity and dynamics of Salmonella enterica in water sources, poultry litters, and field soils amended with poultry litter in a major agricultural area of Virginia. Front. Microbiol. 10:2868. doi: 10.3389/fmicb.2019.02868
Hailu, W., Helmy, Y. A., Carney-Knisely, G., Kauffman, M., Fraga, D., and Rajashekara, G. (2021). Prevalence and antimicrobial resistance profiles of foodborne pathogens isolated from dairy cattle and poultry manure amended farms in northeastern Ohio, the United States. Antibiotics 10:1450. doi: 10.3390/antibiotics10121450
Harrand, A. S., Strawn, L. K., Illas-Ortiz, P. M., Wiedmann, M., and Weller, D. L. (2020). Listeria monocytogenes prevalence varies more within fields than between fields or over time on conventionally farmed New York produce fields. J. Food Prot. 83, 1958–1966. doi: 10.4315/JFP-20-120
Hitchins, A. D., Jinneman, K., and Chen, Y. (2022). BAM chapter 10: detection of Listeria monocytogenes in foods and environmental samples, and enumeration of Listeria monocytogenes in foods. Available at: https://www.fda.gov/food/laboratory-methods-food/bam-chapter-10-detection-listeria-monocytogenes-foods-and-environmental-samples-and-enumeration (Accessed April 29, 2023).
Holley, R. A., Arrus, K. M., Ominski, K. H., Tenuta, M., and Blank, G. (2006). Salmonella survival in manure-treated soils during simulated seasonal temperature exposure. J. Environ. Qual. 35, 1170–1180. doi: 10.2134/jeq2005.0449
Holvoet, K., Sampers, I., Seynnaeve, M., and Uyttendaele, M. (2014). Relationships among hygiene indicators and enteric pathogens in irrigation water, soil and lettuce and the impact of climatic conditions on contamination in the lettuce primary production. Int. J. Food Microbiol. 171, 21–31. doi: 10.1016/j.ijfoodmicro.2013.11.009
Hruby, C. E., Soupir, M. L., Moorman, T. B., Pederson, C., and Kanwar, R. (2018). Salmonella and fecal indicator bacteria survival in soils amended with poultry manure. Water Air Soil Pollut. 229, 1–14. doi: 10.1007/s11270-017-3667-z
Ivanek, R., Gröhn, Y., Wells, M., Lembo, A., Sauders, B., and Wiedmann, M. (2009). Modeling of spatially referenced environmental and meteorological factors influencing the probability of Listeria species isolation from natural environments. Appl. Environ. Microbiol. 75, 5893–5909. doi: 10.1128/AEM.02757-08
Jackson, L., Calderon, F., Steenwerth, K., Scow, K., and Rolston, D. (2003). Responses of soil microbial processes and community structure to tillage events and implications for soil quality. Geoderma 114, 305–317. doi: 10.1016/S0016-7061(03)00046-6
Jechalke, S., Schierstaedt, J., Becker, M., Flemer, B., Grosch, R., Smalla, K., et al. (2019). Salmonella establishment in agricultural soil and colonization of crop plants depend on soil type and plant species. Front. Microbiol. 10:967. doi: 10.3389/fmicb.2019.00967
Jiang, X., Islam, M., Morgan, J., and Doyle, M. P. (2004). Fate of Listeria monocytogenes in bovine manure–amended soil. J. Food Prot. 67, 1676–1681. doi: 10.4315/0362-028X-67.8.1676
Kim, J. S., Lee, G. G., Park, J. S., Jung, Y. H., Kwak, H. S., Kim, S. B., et al. (2007). A novel multiplex PCR assay for rapid and simultaneous detection of five pathogenic bacteria: Escherichia coli O157: H7, Salmonella, Staphylococcus aureus, Listeria monocytogenes, and Vibrio parahaemolyticus. J. Food Prot. 70, 1656–1662. doi: 10.4315/0362-028X-70.7.1656
Liao, J., Guo, X., Weller, D. L., Pollak, S., Buckley, D. H., Wiedmann, M., et al. (2021). Nationwide genomic atlas of soil-dwelling Listeria reveals effects of selection and population ecology on pangenome evolution. Nat. Microbiol. 6, 1021–1030. doi: 10.1038/s41564-021-00935-7
Linke, K., Rückerl, I., Brugger, K., Karpiskova, R., Walland, J., Muri-Klinger, S., et al. (2014). Reservoirs of Listeria species in three environmental ecosystems. Appl. Environ. Microbiol. 80, 5583–5592. doi: 10.1128/AEM.01018-14
Locatelli, A., Spor, A., Jolivet, C., Piveteau, P., and Hartmann, A. (2013). Biotic and abiotic soil properties influence survival of Listeria monocytogenes in soil. PLoS One 8:e75969. doi: 10.1371/journal.pone.0075969
Luo, C., Walk, S. T., Gordon, D. M., Feldgarden, M., Tiedje, J. M., and Konstantinidis, K. T. (2011). Genome sequencing of environmental Escherichia coli expands understanding of the ecology and speciation of the model bacterial species. Proc. Natl. Acad. Sci. 108, 7200–7205. doi: 10.1073/pnas.1015622108
Lynch, M. F., Tauxe, R. V., and Hedberg, C. W. (2009). The growing burden of foodborne outbreaks due to contaminated fresh produce: risks and opportunities. Epidemiol. Infect. 137, 307–315. doi: 10.1017/S0950268808001969
Maguire, R. O., Chardon, W. J., and Simard, R. R. (2005). Assessing potential environmental impacts of soil phosphorus by soil testing. Agric. Ecosyst. Environ. 46, 145–180. doi: 10.2134/agronmonogr46.c6
Maguire, R. O., and Heckendorn, S. E. (2022). Soil test recommendations for Virginia. Available at: https://www.soiltest.vt.edu/content/dam/soiltest_vt_edu/PDF/recommendation-guidebook.pdf (Accessed July 26, 2023).
Makowski, D., Ben-Shachar, M. S., Chen, S., and Lüdecke, D. (2019a). Indices of effect existence and significance in the Bayesian framework. Front. Psychol. 10:2767. doi: 10.3389/fpsyg.2019.02767
Makowski, D., Ben-Shachar, M. S., and Lüdecke, D. (2019b). bayestestR: describing effects and their uncertainty, existence and significance within the Bayesian framework. J. Open Source Softw. 4:1541. doi: 10.21105/joss.01541
Mclaughlin, H. P., Casey, P. G., Cotter, J., Gahan, C. G., and Hill, C. (2011). Factors affecting survival of Listeria monocytogenes and Listeria innocua in soil samples. Arch. Microbiol. 193, 775–785. doi: 10.1007/s00203-011-0716-7
Mehlich, A. (1984). Mehlich 3 soil test extractant: a modification of Mehlich 2 extractant. Commun. Soil Sci. Plant Anal. 15, 1409–1416.
Moshtaghi, H., Garg, S., and Mandokhot, U. V. (2003). Prevalence of Listeria in soil. Indian J. Exp. Biol. 41, 1466–1468.
Murphy, C. M., Weller, D. L., and Strawn, L. K. (2023). Salmonella prevalence is strongly associated with spatial factors while Listeria monocytogenes prevalence is strongly associated with temporal factors on Virginia produce farms. Appl. Environ. Microbiol. 89, e01529–e01522. doi: 10.1128/aem.01529-22
Näsholm, T., Kielland, K., and Ganeteg, U. (2009). Uptake of organic nitrogen by plants. New Phytol. 182, 31–48. doi: 10.1111/j.1469-8137.2008.02751.x
Natvig, E. E., Ingham, S. C., Ingham, B. H., Cooperband, L. R., and Roper, T. R. (2002). Salmonella enterica serovar typhimurium and Escherichia coli contamination of root and leaf vegetables grown in soils with incorporated bovine manure. Appl. Environ. Microbiol. 68, 2737–2744. doi: 10.1128/AEM.68.6.2737-2744.2002
Nightingale, K., Bovell, L., Grajczyk, A., and Wiedmann, M. (2007). Combined sigB allelic typing and multiplex PCR provide improved discriminatory power and reliability for Listeria monocytogenes molecular serotyping. J. Microbiol. Methods 68, 52–59. doi: 10.1016/j.mimet.2006.06.005
Park, S., Navratil, S., Gregory, A., Bauer, A., Srinath, I., Jun, M., et al. (2013). Generic Escherichia coli contamination of spinach at the preharvest stage: effects of farm management and environmental factors. Appl. Environ. Microbiol. 79, 4347–4358. doi: 10.1128/AEM.00474-13
Park, S., Navratil, S., Gregory, A., Bauer, A., Srinath, I., Szonyi, B., et al. (2014). Farm management, environment, and weather factors jointly affect the probability of spinach contamination by generic Escherichia coli at the preharvest stage. Appl. Environ. Microbiol. 80, 2504–2515. doi: 10.1128/AEM.03643-13
Pike, V. L., Lythgoe, K. A., and King, K. C. (2019). On the diverse and opposing effects of nutrition on pathogen virulence. Proc. R. Soc. Biol. Sci. 286:20191220. doi: 10.1098/rspb.2019.1220
Pires, A. F., Ramos, T. D. M., Baron, J. N., Millner, P. D., Pagliari, P. H., Hutchinson, M., et al. (2023). Risk factors associated with the prevalence of Shiga-toxin-producing Escherichia coli in manured soils on certified organic farms in four regions of the USA. Front. Sustain. Food Syst 7:1125996. doi: 10.3389/fsufs.2023.1125996
Ramos, T. D. M., Jay-Russell, M. T., Millner, P. D., Baron, J. N., Stover, J., Pagliari, P., et al. (2021). Survival and persistence of foodborne pathogens in manure-amended soils and prevalence on fresh produce in certified organic farms: a multi-regional baseline analysis. Front. Sustain. Food Syst. 5:355. doi: 10.3389/fsufs.2021.674767
Reddy, K., Khaleel, R., and Overcash, M. (1981). Behavior and transport of microbial pathogens and indicator organisms in soils treated with organic wastes. J. Environ. Qual. 10, 255–266. doi: 10.2134/jeq1981.00472425001000030001x
Reed-Jones, N. L., Marine, S. C., Everts, K. L., and Micallef, S. A. (2016). Effects of cover crop species and season on population dynamics of Escherichia coli and Listeria innocua in soil. Appl. Environ. Microbiol. 82, 1767–1777. doi: 10.1128/AEM.03712-15
Rodriguez, A., Pangloli, P., Richards, H. A., Mount, J. R., and Draughon, F. A. (2006). Prevalence of Salmonella in diverse environmental farm samples. J. Food Prot. 69, 2576–2580. doi: 10.4315/0362-028X-69.11.2576
Rosner, B. (1975). On the detection of many outliers. Technometrics 17, 221–227. doi: 10.2307/1268354
Rosner, B. (1983). Percentage points for a generalized ESD many-outlier procedure. Technometrics 25, 165–172. doi: 10.1080/00401706.1983.10487848
Sjogren, R. E. (1994). Prolonged survival of an environmental Escherchia coli in laboratory soil microcosms. Water Air Soil Pollut. 75, 389–403. doi: 10.1007/BF00482948
Strawn, L. K., Danyluk, M. D., Worobo, R. W., and Wiedmann, M. (2014). Distributions of Salmonella subtypes differ between two US produce-growing regions. Appl. Environ. Microbiol. 80, 3982–3991. doi: 10.1128/AEM.00348-14
Strawn, L. K., Fortes, E. D., Bihn, E. A., Nightingale, K. K., Gröhn, Y. T., Worobo, R. W., et al. (2013a). Landscape and meteorological factors affecting prevalence of three food-borne pathogens in fruit and vegetable farms. Appl. Environ. Microbiol. 79, 588–600. doi: 10.1128/AEM.02491-12
Strawn, L. K., Gröhn, Y. T., Warchocki, S., Worobo, R. W., Bihn, E. A., and Wiedmann, M. (2013b). Risk factors associated with Salmonella and Listeria monocytogenes contamination of produce fields. Appl. Environ. Microbiol. 79, 7618–7627. doi: 10.1128/AEM.02831-13
Timmins, K. (2023). Assistance resources for farmers impacted by recent flooding. Available at: https://www.wga.com/news/assistance-resources-for-farmers-impacted-by-recent-flooding/ (Accessed July 26, 2023).
Truitt, L. N., Vazquez, K. M., Pfuntner, R. C., Rideout, S. L., Havelaar, A. H., and Strawn, L. K. (2018). Microbial quality of agricultural water used in produce preharvest production on the eastern shore of Virginia. J. Food Prot. 81, 1661–1672. doi: 10.4315/0362-028X.JFP-18-185
Underthun, K., De, J., Gutierrez, A., Silverberg, R., and Schneider, K. R. (2018). Survival of Salmonella and Escherichia coli in two different soil types at various moisture levels and temperatures. J. Food Prot. 81, 150–157. doi: 10.4315/0362-028X.JFP-17-226
Vivant, A.-L., Garmyn, D., and Piveteau, P. (2013). Listeria monocytogenes, a down-to-earth pathogen. Front. Cell. Infect. Microbiol. 3:87. doi: 10.3389/fcimb.2013.00087
Wadamori, Y., Gooneratne, R., and Hussain, M. A. (2017). Outbreaks and factors influencing microbiological contamination of fresh produce. J. Sci. Food Agric. 97, 1396–1403. doi: 10.1002/jsfa.8125
Wang, H., Ibekwe, A. M., Ma, J., Wu, L., Lou, J., Wu, Z., et al. (2014). A glimpse of Escherichia coli O157: H7 survival in soils from eastern China. Sci. Total Environ. 476, 49–56. doi: 10.1016/j.scitotenv.2014.01.004
Weller, D., Wiedmann, M., and Strawn, L. K. (2015). Spatial and temporal factors associated with an increased prevalence of Listeria monocytogenes in spinach fields in New York state. Appl. Environ. Microbiol. 81, 6059–6069. doi: 10.1128/AEM.01286-15
Keywords: Salmonella , Listeria , unamended soil, preharvest, produce safety, soil nutrients, prevalence, management practices
Citation: Cook C, Diekman CM, Weller DL, Murphy CM, Hamilton AM, Ponder M, Boyer RR, Rideout SL, Maguire RO, Danyluk MD and Strawn LK (2023) Factors associated with foodborne pathogens and indicator organisms in agricultural soils. Front. Sustain. Food Syst. 7:1269117. doi: 10.3389/fsufs.2023.1269117
Edited by:
Joshua B. Gurtler, Agricultural Research Service (USDA), United StatesReviewed by:
Lisa Gorski, Agricultural Research Service (USDA), United StatesMieke Uyttendaele, Ghent University, Belgium
Achyut Adhikari, Louisiana State University, United States
Copyright © 2023 Cook, Diekman, Weller, Murphy, Hamilton, Ponder, Boyer, Rideout, Maguire, Danyluk and Strawn. This is an open-access article distributed under the terms of the Creative Commons Attribution License (CC BY). The use, distribution or reproduction in other forums is permitted, provided the original author(s) and the copyright owner(s) are credited and that the original publication in this journal is cited, in accordance with accepted academic practice. No use, distribution or reproduction is permitted which does not comply with these terms.
*Correspondence: Laura K. Strawn, THN0cmF3bkB2dC5lZHU=