- 1Division of Management and Administrative Science, Department of Economics, University of Education, Lahore, Pakistan
- 2Institute of Agricultural and Resource Economics, University of Agriculture, Faisalabad, Pakistan
- 3Department of Economics and Rural Development, Gembloux Agro-Bio Tech, University of Liège, Gembloux, Belgium
- 4Faculty of Environmental Sciences, Czech University of Life Sciences Prague, Prague, Czechia
- 5Faculty of Environmental Science and Engineering, Babeș-Bolyai University, Cluj-Napoca, Romania
- 6Department of Agricultural Economics, Faculty of Agriculture, Ondokuz Mayis University, Samsun, Türkiye
- 7Division of Agribusiness and Agricultural Economics, Department of Agricultural and Consumer Sciences, Tarleton State University, Stephenville, TX, United States
- 8Department of Multidisciplinary Innovation, University of North Texas, Denton, TX, United States
Responsible production is essential for sustainable development and for ensuring global food security. The concept of responsible production has been well studied in other sectors of the economy, but has yet to gain recognition in the agricultural sector. Therefore, this study examined responsible production in the context of agriculture and the factors affecting responsible farm production in the developing country of Pakistan. Face-to-face interviews were conducted to collect data from 196 farmers selected using the multistage random sampling method. An independent sample t-test, chi-square test, and ordered probit model were used to analyze the data. The responsible farm production index was estimated based on the climate change adaptation, resource efficiency, carbon footprints, and economic returns of each farm. The mean value of the responsible farm production index is 0.69. The farmers were divided into low-, moderate-, and highly responsible farm producers using cluster analysis. More than 36% of farms were highly responsible. The results revealed that women’s participation in farming activities, extension services, the use of information and communication technologies, and farmer entrepreneurial orientation dimensions significantly affected responsible farm production. Farm producers using the Internet for agriculture had a 1.4% points higher probability of belonging to the highly responsible farm producer category compared to those who did not use the Internet to obtain agricultural information. Farms with women’s participation in agricultural activities were 33.5% points more likely to belong to the highly responsible farm producer category than farms where only males perform agricultural operations. Therefore, women’s empowerment and farmers’ entrepreneurial skills are absolute necessities of responsible farming. This study piques the interests of stakeholders while also adding to the scant body of knowledge on responsible farm production around the world. Furthermore, this study is critical for developing a roadmap for long-term sustainable agricultural development.
1. Introduction
The Sustainable Development Goals (SDGs) provide a shared blueprint for developed and developing nations to pursue sustainable development (United Nations, 2015). SDG 12 emphasizes the significance of responsible production in the development of all goods and services. The purpose of responsible production is to produce more and better with less. It also emphasizes the essence of decoupling economic growth from environmental degradation, increasing resource efficiency, and promoting sustainable lifestyles (Liu et al., 2021). The concept of “responsible production” has appeared in literature such as Huaccho-Huatuco and Ball (2019), Sleiman and Chahine (2019), and Whitson and French (2021), but it has not yet received widespread recognition, especially in the agriculture sector.
Agriculture production is crucial for ensuring global food security and alleviating poverty (Otsuka, 2013; Haq et al., 2021). Moreover, it provides a livelihood to millions of rural households and is critical for economic development, particularly in developing countries. Moreover, agriculture is the largest consumer of scarce natural resources, and competition among sectors for these scarce natural resources is growing due to increasing population pressure (UNEP, 2016). Therefore, the agriculture sector should utilize natural resources efficiently and sustainably in farm production.
An intriguing characteristic of agricultural production that distinguishes it from other sectors is the interdependence between agriculture and climate. Agriculture is entirely dependent on climate, and thus, the utilization of various farm resources is impacted by climate change (Mulwa et al., 2017; Arora, 2019). Climate change is now a reality and poses a serious threat to agricultural productivity. Specifically, agriculture is a major source of greenhouse gas (GHG) emissions, which are the primary driver of global climate change (Blandford and Hassapoyannes, 2018). Climate change has a negative impact on agriculture by reducing farm production. Therefore, agriculture is both a cause and an effect of climate change (Shahbaz et al., 2022a). Furthermore, agriculture production is distinguished by low farm efficiency and economic returns when compared to other sectors (Toma et al., 2017; Kish and Fairbairn, 2018). The aforementioned concerns imply that farming will not be able to sustain itself in the future, and farm producers will need to be more responsible in utilizing various farm resources for production. The importance of this concept in farming comes from the fact that Skouloudis et al. (2015) describe responsible production as a production-oriented obligation that includes the environment, efficiency, and a sustainable way of life.
This study considered Pakistan as a case study for several reasons. Agriculture contributes nearly one-fifth of the national gross domestic product and employs nearly one-third of the Pakistani population (GoP, 2022). Pakistan also serves as a representative example of developing nations that are very vulnerable to the negative effects of climate change, yet have made little effort to combat those effects. According to Kreft et al. (2016), Pakistan is the 7th most vulnerable country due to climate change in the world. Moreover, Pakistan’s updated national climate change policy in March 2022 aims to make the country more resilient to climate change and lead to a low-carbon society (MOCC, 2022). As a result, supporting responsible consumption and production has become a key priority for decreasing the negative effects of climate change and reducing carbon emissions in the country. One of the crucial policy tools that can assist the Pakistani government in achieving national climate change policy targets for a climate-resilient and low-carbon society is responsible farm production (RFP). Despite the fact that farm production in agriculture is entirely different from the production of goods and services in other sectors, none of the previous studies explicitly focused on responsible production with regard to farming.
Moreover, a plethora of previous studies (Huaccho-Huatuco and Ball, 2019; Sleiman and Chahine, 2019; Whitson and French, 2021) on responsible production have mostly overlooked the agriculture sector in favor of concerns affecting other non-agriculture sectors. Agriculture, as the largest user of natural resources and the driving force of the economy, particularly in developing nations, necessitates greater attention from scholars on the subject of RFP. Therefore, this study bridges the gap by examining the responsible production concept with respect to farm production and addressing three research questions: (1) what is the current status of RFP on farms? (2) What farm and farmer characteristics of farm producers determine RFP status? (3) How does the status of the RFP change in relation to key farm and farmer characteristics?
From a practical standpoint, this study provides a beneficial tool for assessing farm producers’ RFP status. The findings of this study will support policymakers in their on-going attempts to improve farmers’ attitudes toward responsible farm production. In particular, the results point out some of the most important farm and farmer characteristics and key indicators that policymakers all over the world can use to improve RFP.
The rest of study is structured as follows: It begins with the section “Materials and methodology” by defining responsible farm production, introducing the study area and sampling procedure, and discussing the different RFP indicators and techniques for measuring these indicators. In addition, the econometric model utilized to determine the factors influencing RFP has been developed in the same section. The “Results and discussion” section summarizes the study’s findings and discusses the results in light of previous research as well as the county’s ground reality. This study concludes with a summary of results, policy implications, and study limitations in the last section, “Conclusion and policy recommendations.”
2. Materials and methodology
2.1. Responsible farm production
This study uses the literature described in the introduction and the SDG 12.2 target, which outlines sustainable management and the efficient use of natural resources, to develop the definition of RFP: “RFP happens when a farm efficiently integrates its farm resources to optimize economic returns while minimizing negative environmental externalities under changing climate scenarios.”
The RFP definition can be decomposed into four parts: (1) efficient use of farm resources; (2) maximum economic returns; (3) minimum negative ecological degradation; and (4) climate change adaptation (Figure 1). All four parts of the RFP were measured in this study using relevant indicators. The farm’s technical efficiency was used to assess the efficiency of farm resources. Farmers’ economic returns were estimated using their total farm income. The third part is the “minimum negative ecological degradation” of RFP, which was measured in the form of reduced carbon footprints. The fourth part of the RFP description is climate change adaptation, which is estimated by the adoption of climate-smart farming practices (Karimi et al., 2018; Van Meijl et al., 2018; Malhi et al., 2021; Ortiz-Bobea et al., 2021).
2.2. Study area
The study was conducted in the Punjab province of Pakistan, owing to its large share of the country’s rose domestic product (Pasha, 2015). On the agricultural front, the Punjab province alone accounts for more than 60% of the total national agricultural output (Government of the Punjab, 2018). Punjab is also Pakistan’s most populous province, with the majority of its people living in rural areas, and more than one-third of the province’s total population relying on agriculture for a livelihood. Furthermore, Punjab province has an extensive irrigation system, fertile fields, and favorable climate conditions for farming, where crop production covers 10.81 million hectares of its total geographical area (Haq et al., 2021). The agriculture sector of Punjab province plays a crucial role in addressing Pakistan’s food security concerns. In addition, it is worth noting that agriculture serves as a primary means of sustenance for over 45% of the labor force within the province (Ahmad et al., 2019). Between 1980 and 2018, the average minimum and maximum temperatures in Punjab ranged from 16.52 to 21.50°C and 30.09 to 32.75°C, respectively. During the same period, the average annual precipitation in Punjab was recorded to be 532.5 mm, with a significant portion of 50–75% occurring specifically between June and August (Abbas et al., 2019). Punjab, being the largest province of Pakistan, is susceptible to the impacts of climate change owing to its geographical positioning, limited ability to adapt, and significant reliance on the natural environment (Shahbaz et al., 2021). The year 2010 witnessed the most severe flooding in the history of Punjab, resulting in the displacement of a significant number of individuals, extensive damage to agricultural produce, and loss of animal life (Akbar and Aldrich, 2018).
Punjab province was chosen as the target study area for this research due to its substantial rural population, significance to the national economy, contribution to agricultural output, and substantial cropping area (Figure 2).
The Punjab province is divided into different administrative units. Therefore, a multistage random and purposive sampling technique was used to distribute the determined sample size from the largest administrative unit (districts) to the smallest administrative unit (villages). Punjab was chosen as the study area for this research during the first stage of sampling. The selected province is divided into agro-ecological zones (Ahmad et al., 2019). The mixed cropping, maize-wheat mix cropping, and rice agro-ecological zones were chosen in the second stage of sampling. The mixed cropping zone is characterized by an average annual precipitation of 460 mm. The mean minimum and maximum temperatures within this region exhibit variations ranging from 13°C to 40°C. The maize-wheat mixed cropping zone has a yearly average precipitation of 590 mm. The mean minimum and maximum temperatures within this region exhibits yearly variation, spanning from 11°C to 38°C (Ahmad et al., 2019). In the third stage of sampling, one district from each agro-ecological zone was chosen. In the fourth stage of sampling, two towns (local = tehsil) from each district were chosen. In the fifth stage, two union councils were picked from each town. Two villages were chosen from each union council in the sixth step of sampling. In the last round of sampling, farmers or farm producers were chosen. A team of four experienced enumerators collected data using a well-designed questionnaire and a face-to-face survey.
This study’s representative sample size was estimated using the following equation from Cochran (1963):
Where, n is sample size; Z represents the abscissa of the normal curve that cuts off an area α at the tails; e is accuracy level; p is the estimated proportion of an element; and q = 1 – p.
Assuming p = 0.5 (maximum variability), a 95% confidence interval, a ± 7% accuracy level, and a 1.96 Z value, 196 samples were extracted to represent the farmers living in the province.
2.3. Measuring RFP indicators
The technical efficiency (TE) was estimated to check the efficient use of farming resources. TE allows for lower inputs while increasing output or reducing inputs while increasing output (Shahbaz et al., 2022a). Data on all inputs used on farms to produce various crops and their output was obtained from farm producers in order to determine farm technical efficiency. The crops grown on farms vary from farm to farm and region to region due to differences in the climatic conditions of specific agro-ecological zones. Therefore, the input requirements fluctuate, and some crops may require more inputs for production. As a result, in the TE model, all inputs except land and output were expressed in monetary terms.
The farmers’ farm technical efficiency was estimated using data envelopment analysis (DEA). DEA can be either input- or output-oriented (Shahbaz et al., 2022b). In agriculture, an input-oriented approach is generally preferred because of its ability to manage inputs rather than outputs. Input-oriented BCR models aimed at reducing inputs were deemed more appropriate for assessing the TE of farms in this study. Thus, the input-oriented Banker, Charnes, and Cooper method (BCC) was used in this study to measure farm technical efficiency. Solving the following linear programming (LP) problem provides technical efficiency scores for the farmer:
Where, Y represents the vector of outputs; X represents the vector of inputs; and λ is the vector of Nx1.
Total farm income was employed as an indicator to assess the farms’ economic returns. Farm income was calculated by multiplying the quantity of various crops produced on the farm by their sale price. The sale value of all crops grown on the farms was added to obtain the total farm income of the farm producers.
Minimum environmental degradation was measured by estimating carbon footprints from the farms as carbon footprints are the primary source of ecological degradation. Agriculture is a major contributor to carbon footprints, with fertilizer as the primary agricultural input contributing to carbon emissions. Therefore, this study used the following method by Jayasundara et al. (2014) and Jayasundara (2015) to figure out the carbon footprints of different amounts of fertilizer used on farms:
The adoption of climate-smart farming practices was used as an indicator to assess the level of climate change adaptation. The literature on the adoption of climate change practices was thoroughly reviewed before selecting climate change practices on farms. The available literature yielded a total of 11 climate-smart practices appropriate in the study area. Farmers who implemented smart climate change practices to alleviate the impact of climate change on their farms were classified as adopters of that strategy. The level of climate change adoption on a farm is shown by the number of climate change strategies that farm has put into place.
2.3.1. Normalization of indicators
All of the indicators used to assess the RFP had different measurement units. For example, farm resource efficiency was assessed in percent, economic returns in US dollars, carbon footprints in kilograms, and climate change adaptation in numbers. Because estimated indicators are heterogeneous, they must be normalized before being aggregated into a single index. There are several methods for normalizing indicators, but minimum-maximum normalization is a simple and straightforward method for converting variously observed indicators into dimensionless indicators ranging from 0 to 1 or 0 (−1 to 1). The range is determined by the type of data analyzed. Therefore, this study also used the minimum–maximum normalization method to normalize the actual RFP indicators before adding them to a single index. Gunduz et al. (2011) and Ul Haq and Boz (2020) used the same procedure to normalize the various indicators before combining them into a single index.
For those indicators (technical efficiency, farm income, and climate practices) where a higher score is better for a higher RFP, the following minimum–maximum normalization formula was used:
Where, Z is actual value of indicators.
Similarly, the following formula was applied to the RFP indicator (carbon footprints), whose lower score is preferred for a higher RFP:
Where, Z is actual value carbon footprints.
2.3.2. Estimating the responsible farm production index (RFPI)
The next issue, after normalizing RFP indicators, was to assign weights to distinct indicators before combining these indicators into a responsible farm production index (RFPI). One option was to give weights to the RFP indicators subjectively, but doing so has numerous drawbacks. To avoid bias, the following formula was used to figure out the weight of the RFP indicator:
Where, represents the weight of each RFP indicator; is the actual normalized value of indicator i for farm k; and is the sum of the normalized values of four indicators for farm k. The advantage of employing this weight estimation formula is that it distributes weight to each indicator based on its RFP share. For example, if the normalized value of farm technical efficiency is greater than the values of the other three indicators, technical efficiency will be given more weight than the other indicators. As a result of the change in share of each indicator, the weight allocated to all indicators will vary from farm to farm for each indicator. The weighted results showed that technical efficiency received the highest weight, whereas farm income received the least. The following formula was used to determine RFP status for farm producers:
Where, RFPI represents the responsible farm production index; wi is the weight of the ith indicator for the kth farm; and Di represents the normalized value of the ith indicator for the kth farm.
The RFPI has a value between 0 and 1. This value reflects the RFP status of different farms. A score near 1 suggests a higher RFP status for farm producers, whereas a value near 0 indicates a lower RFP status for farm producers.
2.4. Selection of farm and farmer characteristics and hypothesizing their effect on RFP
Literature (Nowak et al., 2015; Haq et al., 2017; Khanal et al., 2018; Trinh et al., 2018; Hamid et al., 2021; Kryszak et al., 2021; Savari and Amghani, 2021; Shahbaz et al., 2022c) related to factors affecting the different indicators (technical efficiency, carbon footprints, economic returns, and climate change adaptation) of RFP was thoroughly examined to select the farm and farmer characteristics for this study as well as their expected contribution to RFP. As a result, 11 socioeconomic variables with a logical relationship to RFP and applicable in the study area were chosen as potential RFP factors. The age of the farm producers was chosen as the first socioeconomic characteristic. The findings on the effect of age on RFP indicators present mixed results. Therefore, this study also assumes both positive and negative effects of age on RFP. The education of farm producers is regarded as one of the most critical RFP determinants. This study assumes a positive relationship between education and RFP because educated farmers are expected to be more responsible in agricultural production.
Total land is an indicator of a farmer’s economic strength, and earlier research has shown that farmers with larger land sizes are more likely to receive institutional support than farmers with smaller land sizes. Furthermore, a greater landholding allows farmers to devote more area to agricultural production, which can lead to increased farm productivity and efficiency. This study anticipates that this variable will have a positive impact on the RFP. Farming is a laborious activity, especially in developing countries where traditional agricultural methods still prevail. The promotion of gender equality and the empowerment of women are widely recognized as crucial factors in the global socio-economic advancement of nations. The concept of women empowerment entails enabling women to gain power and agency in all facets of their lives including agriculture. Women’s empowerment is multidimensional, and it is important to note that empowerment in one dimension does not guarantee empowerment in others (Mahmud et al., 2012). Women empowerment in agriculture can contribute positively to attaining many of these SDGs, as half of women labor is involved in agricultural activities. Family labor and women’s participation in agriculture have a range of implications for land use, crop productivity, family wages, and resource governance. Thus, family labor and women’s participation in farming activities aid in the efficient use of farm resources by providing labor at important times. Furthermore, family labor and female engagement support in climate change adaptation, which can boost economic returns by mitigating the consequences of climate change on farms. This study hypothesizes that these characteristics will have a positive effect on RFP. Due to the contradictory results in the literature about the influence of farming experience on several RFP indicators, both a positive and a negative effect of farming experience on RFP were assumed. Due to differences in farm-related priorities, owner and tenant farmers behave differently. Owners retain the farm permanently, and they are more likely to implement long-term sustainable practices. Tenant farmers, on the other hand, have a share of the crop harvest or have possession of land for a fixed period of time. Their goal may be to maximize profit or crop share during this specific time period, which may lead to these farmers engaging in unsustainable farming techniques. Therefore, this study hypothesizes that ownership has a favorable effect on RFP. Similarly, agricultural extension services, land fragmentation credit utilization are likely to affect RFP positively.
Apart from these socioeconomic characteristics, the study also employed two additional variables as explanatory variables: information and communication technology (ICT) and farmer entrepreneurship. ICT has become a source of information for the farming community, and the usage of ICT for farm-related information is growing by the day. For agricultural information, ICT includes the use of television, radio, and the internet (Das, 2021; Ayim et al., 2022). This study anticipates a positive contribution of ICTs to RFP since contemporary agriculture is heavily reliant on ICTs. Farmer entrepreneurship is crucial in shaping RFP in developing countries. Farmer entrepreneurship, in broad terms, refers to the process of leveraging resources in novel ways to explore opportunities toward the accomplishment of economic and social goals (Fitz-Koch et al., 2018). Farmer entrepreneurship orientation has three dimensions: (1) risk-taking, (2) innovativeness, and (3) pro-activeness. The combination of innovativeness, pro-activeness, and risk-taking behavior opens up new opportunities for farmers. This study hypothesizes that all dimensions of farmer entrepreneurial orientation play a positive role in RFP decisions (Table 1). Different Likert scale statement questions were used to assess three dimensions of farmer entrepreneurship.
2.5. Empirical model
The farm-producers were categorized into three groups by applying the K-mean cluster analysis using their RFPI scores. These were classified as low, moderately, and highly responsible farm producers. Farmers included in the low-responsible farm producer category had a RFPI score less than or equal to 0.69. Similarly, farmers included in the highly responsible farm production category had RFPI scores greater than to 0.79. The farmers were almost equally divided among the low, moderately, and highly responsible farm producer categories. Table 2 shows that there were more farm producers in the moderately responsible farm producer group than in the low and highly responsible farm producer groups.
Following that, these three farmer producer categories were coded as 0 = farmers in the low-responsible farm production category, 1 = farmers in the moderately responsible farm production category, and 2 = farmers in the highly responsible farm production category as the dependent variable of the ordered probit model. The ordered probit model is defined as follows:
Where, y* is the dependent variable as probability of farmer belonging to a responsible farm producers’ category; β′ is vector of coefficients; xi represents vector of explanatory variables.
ε is vector of normally distributed error terms [0, 1]; y is the observed dependent variable as the probability of farmer to be highly responsible farm producer; and μ are the cut off points which indicates the level of inclination of a farmer to be highly responsible farm producer. It explains if there is a natural ordering among the three categories of the dependent variable.
Chen et al. (2002) suggested the following formula for calculating marginal effects:
Where, is a partial derivative of probability with respect to the independent variable . The positive value of marginal effect of explains that the probability of a farmer selecting the specific category increases with and vice versa. The sum of the marginal effects should be zero because the responses are exclusive and thus cancel each other out (Greene, 2002). The marginal effects were used to figure out how much each explanatory variable increased or decreased the chance of a farmer moving to one of the three categories of the dependent variable.
3. Results and discussion
3.1. Farm and farm producers’ characteristics
Farm and farmer characteristics reveal important information about the farm producer’s personal backgrounds and socioeconomic status. Moreover, farm and farmer characteristics influence farm producers’ activities throughout the production process. Table 3 illustrates the various farm and farmer characteristics of farm producers. Farm producers that are highly responsible were found to be younger and better educated than moderately and lowly responsible farm producers. Low and moderately responsible farm producers had less acreage than highly responsible farm producers. Agriculture is the mainstay of life for the majority of rural households, and about two-fifths of the whole country’s population is directly or indirectly involved in farming activities for their livelihood (Government of Pakistan, 2021). In the research area, more than three people were engaged in farming activities from each participating house on average. On highly responsible farms, more people were found to be participating in farming operations than on low and moderately responsible farms. This could be because highly responsible farm producers have larger landholdings, which necessitates having more people to conduct and oversee farm operations due to the dominance of conventional and traditional farming. Women are an integral part of farming activities, and their participation adds labor to farm operations. On low-responsible farms, women participated in farm operations at a lower rate than on moderately and highly responsible farms. More than half of the farmers managed their farms without the participation of women. The explanation for women’s lower engagement in farming activities could be related to societal and cultural constraints that exist in rural regions and hinder women’s ability to work outside the home (Mohiuddin et al., 2020). Experience in farming is also an important component of human capital. Farm producers learn from their previous crop production experiences and conduct their businesses more efficiently in the future because of their previous experience. In this study, farmers were well experienced, as their average farming experience was assessed to be more than half of their average age. However, in comparison to the other two farm producer types, highly responsible farm producers had the least agricultural experience.
Land tenure status is an important farm attribute because farmers with different land tenures behave differently in relation to similar farm operations (Akram et al., 2019). A large majority of highly responsible farm producers were operating on their own land, compared to low and moderately responsible farm producers. Additionally, farm producers in the highly responsible farming category received more extension services than farm producers in the low and moderately responsible farming categories, where agricultural extension services assist with information dissemination. Due to the time lag between agricultural investment and return, the farming community lacks financial resources. Credit usage provides the financial means to acquire farm inputs on time. A lower number of farm producers in the low-responsible farming category utilized credit for farm operations than moderately and highly responsible farm producers. Land fragmentation refers to the presence of many spatially dispersed pieces of farmland controlled by the same farm producer (Alemu et al., 2017). Low-responsible farm producers had a higher number of land fragments than moderately and highly responsible farm producers.
Information and communication technology benefits farm producers by facilitating access to growing contemporary farming technologies, cropping patterns, and real-time market data (Das, 2021; Ayim et al., 2022). The highly responsible farm producers used ICT for agricultural information the most in comparison to the other two farm producer categories. The plausible explanation could be that farmers in the highly responsible farming group had a greater degree of education than farmers in other categories. Farm entrepreneurship is critical for agriculture due to increased competition for natural resources across diverse sectors. Farm producers in the highly RFP group were shown to be more risk-taking, innovative, and proactive in their farming activities than farm producers in the low and moderate farm production categories.
3.2. Graphical presentation of RFP status of all farm producers
A radar presentation of all RFPI scores revealed significant variations in the RFP status of various farm producers. The disparity in their farms and farmers’ characteristics could be the reason for this variation. Although the overall status of farmers was satisfactory, with the vast majority of farm producers having RFPI scores greater than 0.50, no farm producer was fully responsible for farm production. Farmers’ RFPI scores range from 0.38 to 0.92 (Figure 3). This shows that there is a chance for individual farmers to improve their RFP status.
3.3. Relationship between RFP and its indicators
Figure 4 depicts the relationship between RFP and its indicators (CO2 emissions, technical efficiency, farm income, and climate change adaptation). Except for CO2 emissions, all indicators were positively associated with RFP. This indicates that a rise in technical efficiency, farm income, and climate change adaptation will improve farm producers’ overall RFP status. Therefore, farm producers should make better use of their farm resources and boost climate change adaptability to increase RFP. Increased CO2 emissions, on the other hand, will reduce farm producers’ overall RFP status (Figure 4A). For example, a unit increase in CO2 emissions reduces RFP by 0.01. Farm producers should endeavor to reduce CO2 emissions from their fields in order to improve the RFP status. Farmers can reduce CO2 emissions by using less synthetic fertilizer. The trend line between technical efficiency (Figure 4B) is steeper than the trend lines of farm income (Figure 4C) and climate change adaptation (Figure 4D). This result shows that a unit change in technical efficiency has a greater impact on RFP status than a unit change in the other two positively influencing factors. A unit increase in farm technical efficiency, for example, will improve the overall RFP status by 0.318. Similarly, increasing farm climate change adaptation by one unit raises the total RFP status by 0.022. As a result, in order to be more responsible farm producers, farmers need to focus more on using farming resources efficiently.
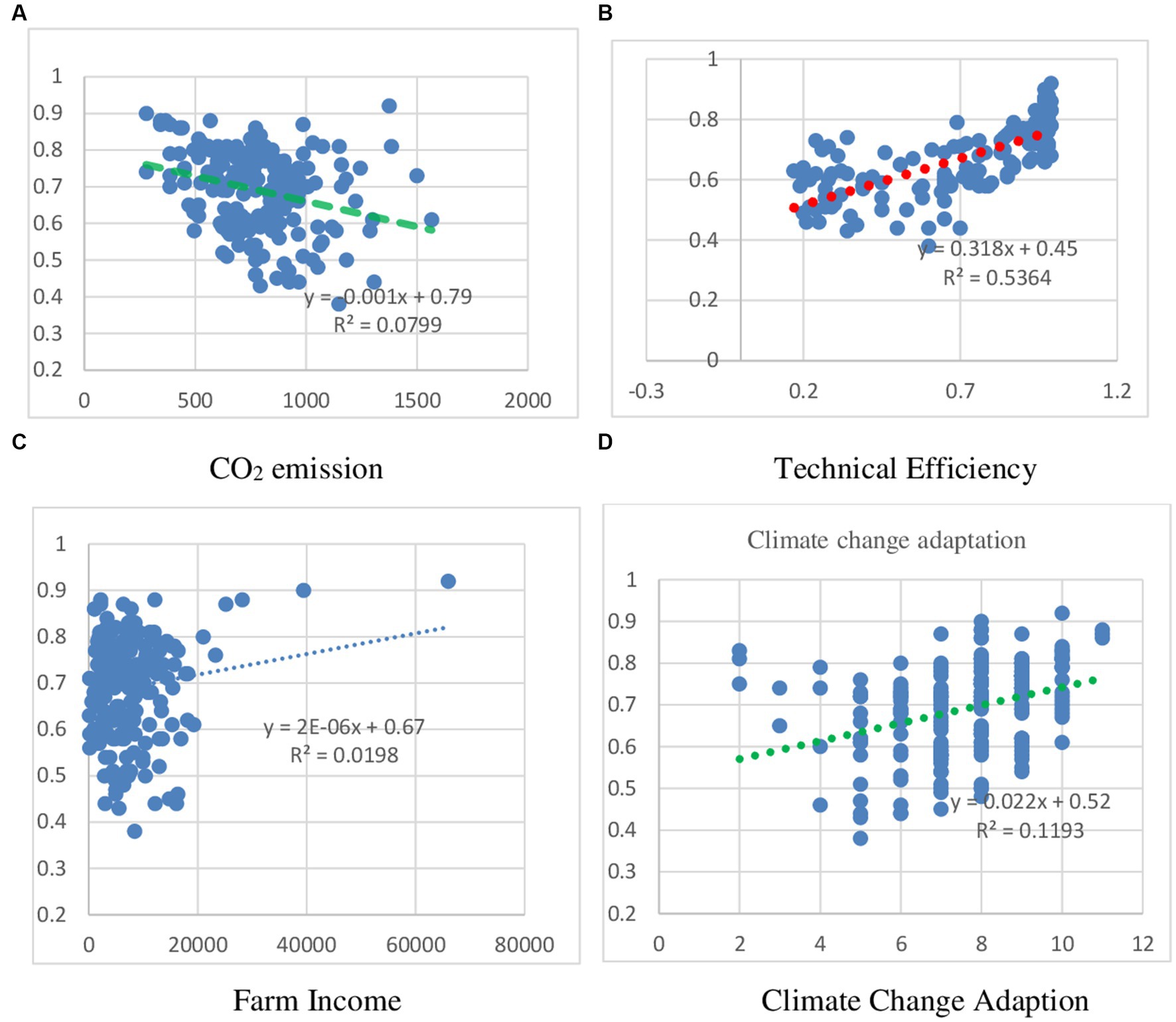
Figure 4. Relationship between RFP and its four indictors (CO2 emissions, technical efficiency, farm income, and climate change adaptation).
3.4. Mean RFPI scores
Figure 5 portrays the RFP status of low, moderately, and highly responsible farm producers based on their mean RFPI scores. The figure also depicts the overall mean RFPI score of all farm producers, which was assessed to be 0.69. Low responsible farm producers had 0.15 and 0.25 lower mean RFPI scores, respectively, than moderately and highly responsible farm producers. The mean RFPI score of highly responsible farm producers was likewise 0.10 higher than that of moderately responsible farm producers. Only low-responsible farm producers had a lower mean RFPI score than all farmers combined. This suggests that poorly responsible farm producers are the primary cause of the farmers’ overall poorer RFP status.
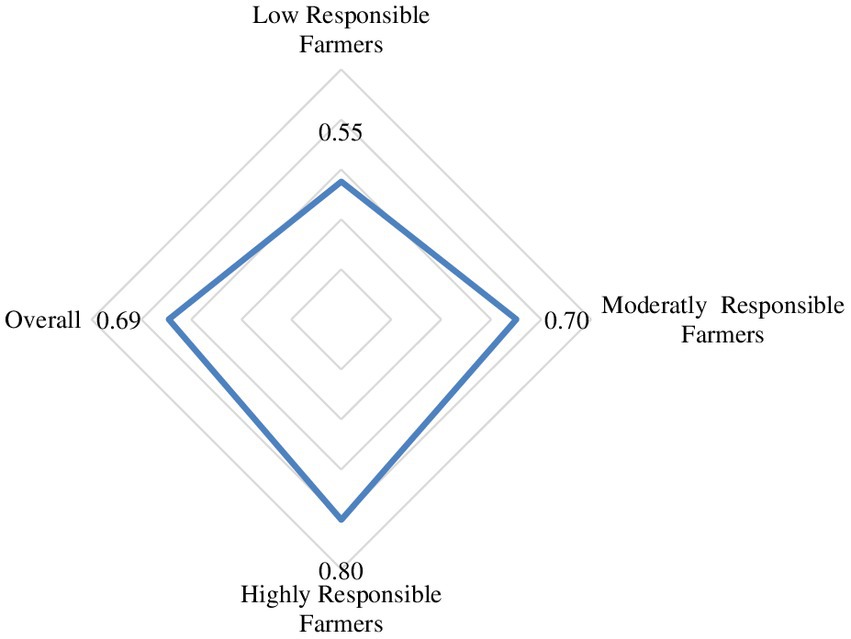
Figure 5. Mean responsible farm production index (RFPI) scores for low, moderately, and highly responsible farm producers.
3.5. Factors affecting the RFP
Farm producers’ actions in the field are important to the RFP. A total of 16 farm and farmer characteristics were considered for analyzing their impact on the RFP. Out of which, nine variables (age, education, women’s participation, extension services, TV and Internet use for agricultural purposes, risk taking, innovativeness, and pro-activeness) were found to significantly affect the RFP. The overall ordered probit model was significant, with a log likelihood ratio of chi square value of-120.666 and a probability of Chi-square of less than 1%.
The age of the farm producers was negatively associated with the RFP (Table 4), which indicates that younger farmers are likely to act more responsibly in farm production as compared to older farmers. This can be explained by the fact that younger farm producers are expected to be better aware of RFP than older farm producers due to their knowledge of modern techniques necessary for efficient use of natural resources, raising income, and implementing climate change adaptation practices. A one-year increase in farm producers’ age increases the likelihood of belonging to the low and moderately responsible farm producer categories by 0.6% points and 1% points, respectively. However, a one-year increase in farm producers’ age reduces the probability of belonging to the highly responsible farming category by 1.6% points. The education of farm producers was also found to significantly affect the RFP. If farm producers’ education improves by a year, their chances of belonging to a highly RFP category increases by 3.5% points. In addition, a one-year decrease in the education of farm producers reduces the probability of being a low- or moderately responsible farm producer by 1.2% points and 2.3% points, respectively. The reason may be that educated farmers can communicate easily with extension workers and credit-providing institutions, helping these farmers utilize their farm resources productively and efficiently, which is necessary for RFP. Moreover, educated farm producers can also use internet facilities to get agriculture-related information, which assists them in different farm operations directly linked to the RFP.
Women’s participation in agricultural activities was also significant in influencing the RFP. This may be because women provide the additional labor force necessary for certain farm operations in traditional agriculture. Traditional agriculture requires more labor for farming activities. Moreover, women tend to be more resource efficient and have healthier environmental behaviors. Thus, the involvement of women in farming activities enhances the probability of a farm producer belonging to a higher RFP category. Farms with women’s participation in agricultural activities were 33.5% points more likely to belong to the highly responsible farm producer category as compared to farms where only males perform agricultural operations. Similarly, women’s participation in agricultural activities decreases the chances of a farm producer belonging to a low or moderately responsible farming category by 11.9% points and 21.6% points, respectively, compared to only male-managed farms.
Agriculture extension services increase farmers’ knowledge, which in turn increases responsible farming by increasing farm efficiency, climate change adaptation, and farm income. Agriculture extension services were found to be positively related to the RFP. Even though the use of other sources for information in agriculture is increasing with the passage of time, extension workers are still the primary source of information on modern farming techniques and the agronomic requirements of different crops for the majority of the farming community in the country. This information is vital for farmers to act more responsibly on their farms. Farm producers who availed of extension services had 7.3% points higher chances of belonging to the highly responsible farming category than those who did not utilize extension services. On the other hand, farm producers who used extension services were less likely to be in the low or moderately responsible farming groups by 2.6% points and 4.7% points than farmers who did not use extension services. This shows that a proactive extension system in the country can contribute to RFP.
Agro-informatics plays a significant role in agriculture. ICT are important sources of information, and their use among the farming community is on the rise worldwide owing to their benefits and the increasing agricultural-related information on these platforms (Nnadi et al., 2012). Moreover, awareness among farmers about the use of ICT is also rising with the passage of time. Farm producers who watch TV for agricultural-related information were 23.9% points more likely to belong in the highly RFP category compared to those who do not watch TV for agricultural information. Contrarily, farm producers who watch TV for agricultural-related information were 8.5% points and 15.4% points less likely to belong in the low or moderately RFP categories compared to those who do not watch TV for agricultural information. Similarly, internet use for obtaining information was also found to be positively associated with RFPs. Farm producers using the internet for agriculture had a 1.4% points higher probability of belonging to the highly responsible farm producer category compared to those who do not use the internet for obtaining agricultural information. This implies that the use of ICT among the farming community can make farm production more responsible. Hamad et al. (2018) and Irungu et al. (2015) found that farmers successfully utilized the internet and social media for sharing production technologies, market information, and money transactions. Similarly, Ma et al. (2020) revealed that the use of ICT, such as smart phones, substantially increases the farm income of farm producers. Thus, the use of ICT assists farmers in improving farm efficiency, income, and climate change adaptation, which ultimately contributes to RFP.
All dimensions of farm entrepreneurial orientation were positively associated with RFP. This means that a farmer acting entrepreneurially in farming activities is likely to be more responsible for farm production than those farmers who do not work entrepreneurially in farm production. The reason may be that farmers working as entrepreneurs have the ability to deviate from traditional farming methods, enabling them to use farm resources more efficiently and cost-effectively. The efficient and cost-effective use of resources is essential part of the RFP. A more risk-taking and innovative farm producer is 3.9% points and 11.5% points more likely to belong to the highly responsible farm producer category, respectively. Similarly, proactive farm producers were, respectively, 3.3% points and 6% points less likely to belong to the low and moderately responsible farm producer categories. The reason may be that pro-activeness entails the capacity to anticipate and respond to future difficulties and opportunities. The literature on the relationship between farmer entrepreneurship and RFP indicators reflects that farmer entrepreneurship positively affects different indicators (farm income, technical efficiency, and climate change adaptation) of RFP (Abbas et al., 2016; Arellano and Reyes, 2019; Kangogo et al., 2021). Thus, farmer entrepreneurship positively affects responsible farming, as found in this study.
3.6. Responsible farm production status based on important farm and farm producer characteristics
Table 5 describes the RFP status in terms of RFPI scores on the basis of important farm and farm producer characteristics. The comparison of the RFPI scores of old and young farm producers revealed that farms managed by young producers were more responsible for farm production than farms operated by old ones. The young farm producer had a 0.06 higher RFPI score than the old farm producer. Similarly, the RFPI scores of high and low-educated farm producers showed that high-educated farm producers performed comparatively better in RFP, as indicated by their 0.08 higher RFPI score than low-educated farm producers. Tenant farm producers were relatively less responsible for farm production than owner farm producers. The reason may be the difference in attitudes towards farm operations. For example, owner farmers take more responsible care of farm resources and use these resources efficiently as compared to tenant farmers. Moreover, owner farmers adopt more sustainable farm practices such as climate-smart practices than tenant farmers. Soule et al. (2000) found that owner-operated farms compared to farms under other land tenure statuses were more likely to adopt practices at their farms with long-run benefits.
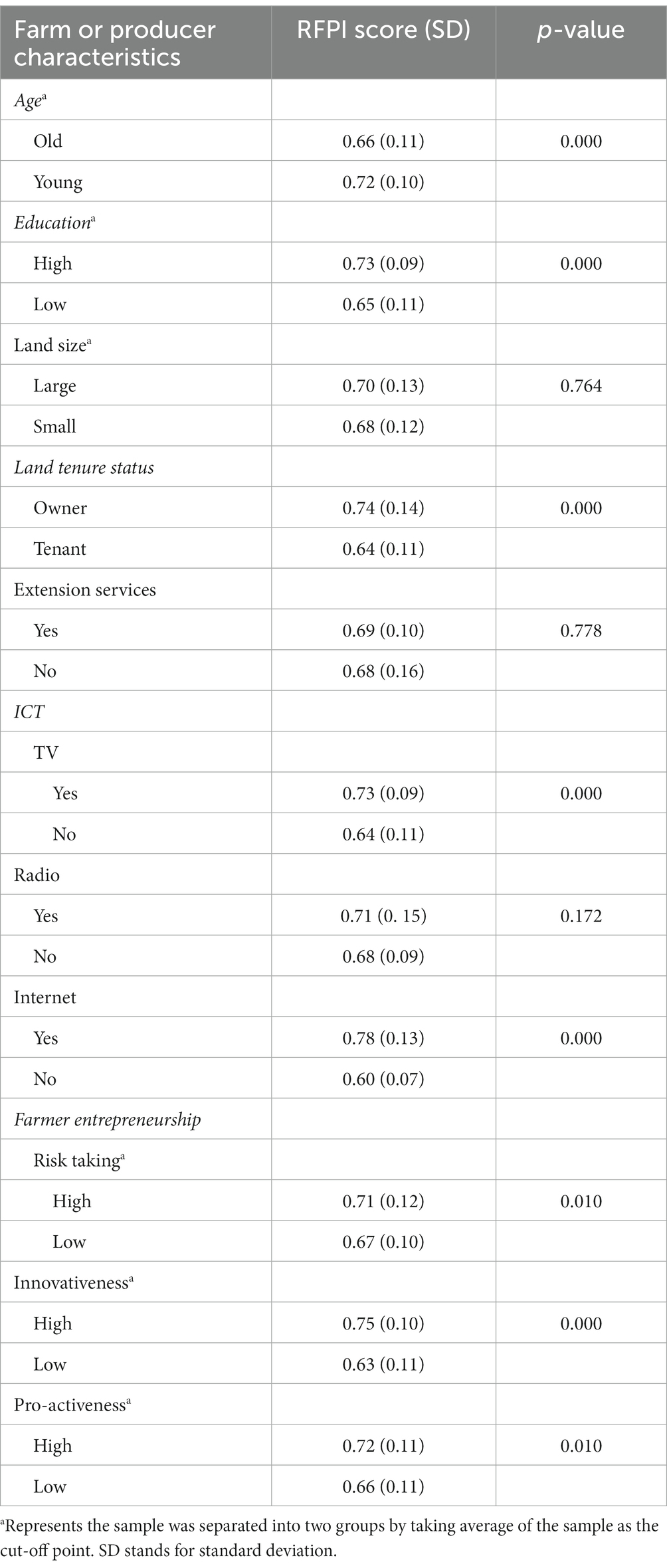
Table 5. Responsible farm production index (RFPI) scores based on important farm and producer characteristics.
Farm producers utilizing ICT such as TV and the Internet for obtaining agricultural information were found to be more responsible farm producers than those who did not use these modern sources of information. The farm producers not utilizing TV and the Internet for farming purposes had 0.09 and 0.18 lower RFPI scores, respectively, than farmers utilizing modern information sources. High-risk-taking farm producers performed better by attaining a higher RFPI score than low-risk-taking farm producers. Similarly, high-innovating farm producers had a 0.0.12 higher RFPI score than low-innovating farm producers. Low-proactive farm producers had a lower RFPI score than highly proactive farm producers. These results show that farm producers managing their farms as an enterprise and working more entrepreneurially can contribute to RFP than other farm producers working traditionally.
4. Conclusion and policy recommendations
In recent academic studies on responsible production, the agriculture sector, which uses the most natural resources, has been ignored. The purpose of this study was to contribute to theory by analyzing responsible production, specifically with regard to farming. The study examined the status of RFPs as well as the factors influencing them. Specifically, our findings addressed three research questions: (1) What is the current status of RFP on farms? (2) What farm and farmer characteristics of farm producers determine RFP status? (3) How does the status of the RFP change in relation to key farm and farmer characteristics? Findings showed that the overall status of the RFP was satisfactory, while the empirical model results showed that farm and producer characteristics significantly affect the RFP. Socio-economic characteristics such as age, education, women’s participation in farming activities, and extension services were found to be positively correlated with the RFP. Similarly, ICT and farmer entrepreneurship also positively affected the RFP.
RFP status was determined by both farm and farmer characteristics, which revealed that owners tend to be more responsible farm producers compared to tenants. Similarly, farmers who managed their farms in a more entrepreneurial manner were more responsible farm producers than farmers managing their farms in a less entrepreneurially manner.
This study has significant policy implications for Pakistan as well as for other developing countries. To begin with, this study proposes a method for measuring farm producers’ RFP status in agriculture. Second, the study emphasizes the significance of ICT in agriculture for enhancing RFP. Therefore, policymakers can adopt policies to increase the use of ICT in agriculture to increase RFP. Furthermore, ICT can also be used to raise farmers’ knowledge and awareness about RFP. Thirdly, the empirical model results show that women’s engagement in agricultural activities is important for enhancing RFP. The government should aim to increase female participation in farming, particularly their role in agricultural decision-making, in order to improve RFP. This can only be accomplished by changing the farming community’s mindset and attitudes towards women’s roles in society. Fourth, the study results demonstrated the importance of managing a farm entrepreneurially for RFP. As a result, developing countries should endeavor to promote agripreneurship culture in agriculture in order to increase RFP involving all agricultural socioeconomic networks.
Moreover, this study urges governments in Pakistan and other developing countries to develop a sustainable agricultural strategy immediately, as formulating policy and its implementation may take some time. For this purpose, developing nations should embrace the sustainable agricultural strategies employed by affluent ones. Explicit rules may also be used to put pressure on farmers to adopt responsible farming methods in developing countries. Agriculture extension organizations must invest in staff training, particularly for agriculture sustainability. This study also proposes setting up a forum where farmers may exchange their best farming practices and models and debate issues pertaining to agricultural sustainability. Governments should take the lead in organizing such meetings to support the RFP in their countries.
As all studies have limitations, this study is no exception. In this study, we relied on farmers’ recall abilities for information regarding several variables, such as farm inputs and pricing, which may lack accuracy. The second limitation of the study was its geographical coverage, as it was undertaken in two agro-ecological zones of Pakistan’s Punjab province. Furthermore, the study’s other drawback was that it used only fertilizers for estimating carbon footprints from the fields. Even with these limitations, this work makes a contribution to theory, and researchers in other developing countries can build on it by gathering more data using different sampling methods and including more indicators for measuring RFP.
Data availability statement
The raw data supporting the conclusions of this article will be made available by the authors, without undue reservation.
Ethics statement
The studies involving humans were approved by the University of Education Lahore Pakistan. The studies were conducted in accordance with the local legislation and institutional requirements. Written informed consent for participation was not required from the participants or the participants’ legal guardians/next of kin because Verbal consent was obtained from the participants.
Author contributions
All authors listed have made a substantial, direct, and intellectual contribution to the work and approved it for publication.
Conflict of interest
The authors declare that the research was conducted in the absence of any commercial or financial relationships that could be construed as a potential conflict of interest.
Publisher’s note
All claims expressed in this article are solely those of the authors and do not necessarily represent those of their affiliated organizations, or those of the publisher, the editors and the reviewers. Any product that may be evaluated in this article, or claim that may be made by its manufacturer, is not guaranteed or endorsed by the publisher.
References
Abbas, A., Amjath-Babu, T. S., Kächele, H., and Müller, K. (2016). Participatory adaptation to climate extremes: an assessment of households’ willingness to contribute labor for flood risk mitigation in Pakistan. J. Water Climate Change 7, 621–636. doi: 10.2166/wcc.2016.002
Abbas, Q., Han, J., Adeel, A., and Ullah, R. (2019). Dairy production under climatic risks: perception, perceived impacts and adaptations in Punjab, Pakistan. Int. J. Environ. Res. Public Health 16:4036. doi: 10.3390/ijerph16204036
Ahmad, A., Khan, M., Shah, S., Kamran, M., Wajid, S., Amin, M., et al. (2019). Agro-ecological zones of Punjab—Pakistan. Food and Agricultural Organization of the United Nations (FAO): Rome, Italy.
Akbar, M. S., and Aldrich, D. P. (2018). Social capital’s role in recovery: evidence from communities affected by the 2010 Pakistan floods. Disasters 42, 475–497. doi: 10.1111/disa.12259
Akram, N., Akram, M. W., Wang, H., and Mehmood, A. (2019). Does land tenure systems affect sustainable agricultural development? Sustainability 11:3925. doi: 10.3390/su11143925
Alemu, G. T., Berhanie Ayele, Z., and Abelieneh Berhanu, A. (2017). Effects of land fragmentation on productivity in northwestern Ethiopia. Adv. Agric. 2017, 1–9. doi: 10.1155/2017/4509605
Arellano, C. A., and Reyes, J. A. D. (2019). Effects of farmer-entrepreneurial competencies on the level of production and technical efficiency of rice farms in Laguna, Philippines. J. Int. Soc. Southeast Asian Agric. Sci. 25, 45–57.
Arora, N. K. (2019). Impact of climate change on agriculture production and its sustainable solutions. Environ. Sustain. 2, 95–96. doi: 10.1007/s42398-019-00078-w
Ayim, C., Kassahun, A., Addison, C., and Tekinerdogan, B. (2022). Adoption of ICT innovations in the agriculture sector in Africa: a review of the literature. Agric. Food Secur. 11, 1–16. doi: 10.1186/s40066-022-00364-7
Blandford, D., and Hassapoyannes, K. (2018). “The role of agriculture in global GHG mitigation” in OECD Food, Agriculture and Fisheries Papers, No. 112 (Paris: OECD Publishing).
Chen, K., Ali, M., Veeman, M., Unterschultz, J., and Le, T. (2002). Relative importance rankings for pork attributes by Asian-origin consumers in California: applying an ordered probit model to a choice-based sample. J. Agric. Appl. Econ. 34, 67–79. doi: 10.1017/S1074070800002157
Das, B. (2021). Dissemination of knowledge in agriculture: Where does old ICT stand in disseminating knowledge among farmers? 3950247. doi: 10.2139/ssrn.3950247
Fitz-Koch, S., Nordqvist, M., Carter, S., and Hunter, E. (2018). Entrepreneurship in the agricultural sector: a literature review and future research opportunities. Entrep. Theory Pract. 42, 129–166. doi: 10.1177/1042258717732958
GoP (2022). Available at: https://www.finance.gov.pk/survey/chapters_21/02-Agriculture.pdf
Government of Pakistan, (2021). Economic survey of Pakistan. Available at: https://www.finance.gov.pk/survey/chapter_20/02_Agriculture.pdf (Accessed on March 20, 2022).
Government of the Punjab, (2018). Punjab development statistics 2018. Available at: https://www.bos.gop.pk/developmentstat (Accessed on March 26, 2022).
Gunduz, O., Ceyhan, V., Erol, E., and Ozkaraman, F. (2011). An evaluation of farm-level sustainability of apricot farms in Malatya province of Turkey. J. Food Agric. Environ. 9, 700–705.
Hamad, M. A., Eltahir, M. E. S., Ali, A. E. M., and Hamdan, A. M. (2018). Efficiency of using smart-mobile phones in accessing agricultural information by smallholder farmers in North Kordofan–Sudan. Available at: https://ssrn.com/abstract=3240758 (Accessed August 29, 2018).
Hamid, F., Yazdanpanah, M., Baradaran, M., Khalilimoghadam, B., and Azadi, H. (2021). Factors affecting farmers’ behavior in using nitrogen fertilizers: society vs. farmers’ valuation in Southwest Iran. J. Environ. Plan. Manag. 64, 1886–1908. doi: 10.1080/09640568.2020.1851175
Haq, S., Boz, I., and Shahbaz, P. (2021). Adoption of climate-smart agriculture practices and differentiated nutritional outcome among rural households: a case of Punjab province, Pakistan. Food Security 13, 913–931. doi: 10.1007/s12571-021-01161-z
Haq, S. U., Shahbaz, P., Boz, I., Yildirim, I. C., and Murtaza, M. R. (2017). Exploring the determinants of technical inefficiency in mango enterprise: a case of Muzafargarh, Pakistan. Custos e@ gronegócio online 13, 2018–2236.
Huaccho-Huatuco, L., and Ball, P. D. (2019). The quest for achieving United Nations sustainability development goals (SDGs) infrastructure and innovation for responsible production and consumption. RAUSP Manag. J. 54, 357–362. doi: 10.1108/RAUSP-04-2019-0068
Irungu, K. R. G., Mbugua, D., and Muia, J. (2015). Information and communication technologies (ICTs) attract youth into profitable agriculture in Kenya. East African Agric. Forestry J. 81, 24–33. doi: 10.1080/00128325.2015.1040645
Jayasundara, S. (2015). How does the fertilizer use affect GHG emission? Available at: https://www.researchgate.net/post/How_does_the_fertilizer_use_affect_GHG_emission (Accessed August 01, 2021).
Jayasundara, S., Wagner-Riddle, C., Dias, G., and Kariyapperuma, K. A. (2014). Energy and greenhouse gas intensity of corn (Zea mays L.) production in Ontario: a regional assessment. Can. J. Soil Sci. 94, 77–95. doi: 10.4141/cjss2013-044
Kangogo, D., Dentoni, D., and Bijman, J. (2021). Adoption of climate-smart agriculture among smallholder farmers: does farmer entrepreneurship matter? Land Use Policy 109:105666. doi: 10.1016/j.landusepol.2021.105666
Karimi, V., Karami, E., and Keshavarz, M. (2018). Climate change and agriculture: impacts and adaptive responses in Iran. J. Integr. Agric. 17, 1–5.
Khanal, U., Wilson, C., Hoang, V. N., and Lee, B. (2018). Farmers' adaptation to climate change, its determinants and impacts on rice yield in Nepal. Ecol. Econ. 144, 139–147. doi: 10.1016/j.ecolecon.2017.08.006
Kish, Z., and Fairbairn, M. (2018). Investing for profit, investing for impact: moral performances in agricultural investment projects. Environ. Plan. A Economy Space 50, 569–588. doi: 10.1177/0308518X17738253
Kreft, S., Eckstein, D., and Melchoir, I. (2016). Global Clımate Rısk Index (2017) Who Suffers Most From Extreme Weather Events? Weather-related Loss Events in 2015 and 1996 to 2015. Berlin: Germanwatch Nord-Süd Initiative eV.
Kryszak, Ł., Guth, M., and Czyżewski, B. (2021). Determinants of farm profitability in the EU regions. Does farm size matter? Agric. Econ. 67, 90–100.
Liu, F., Lai, K. H., and Cai, W. (2021). Responsible production for sustainability: concept analysis and bibliometric review. Sustainability 13:1275. doi: 10.3390/su13031275
Ma, W., Grafton, R. Q., and Renwick, A. (2020). Smartphone use and income growth in rural China: empirical results and policy implications. Electron. Commer. Res. 20, 713–736. doi: 10.1007/s10660-018-9323-x
Mahmud, S., Shah, N. M., and Becke, R. S. (2012). Measurement of women’s empowerment in rural Bangladesh. World Dev. 40, 610–619. doi: 10.1016/j.worlddev.2011.08.003
Malhi, G. S., Kaur, M., and Kaushik, P. (2021). Impact of climate change on agriculture and its mitigation strategies: a review. Sustainability. 13:1318. doi: 10.3390/su13031318
MOCC (2022). Policies. Available at: https://mocc.gov.pk/Policies (Accessed May 10, 2021).
Mohiuddin, I., Kamran, M. A., Jalilov, S. M., Ahmad, M. U. D., Adil, S. A., Ullah, R., et al. (2020). Scale and drivers of female agricultural labor: evidence from Pakistan. Sustainability 12:6633. doi: 10.3390/su12166633
Mulwa, C., Marenya, P., and Kassie, M. (2017). Response to climate risks among smallholder farmers in Malawi: a multivariate probit assessment of the role of information, household demographics, and farm characteristics. Clim. Risk Manag. 16, 208–221. doi: 10.1016/j.crm.2017.01.002
Nnadi, F. N., Chikaire, J., Atoma, C. N., Egwuonwu, H. A., and Echetama, J. A. (2012). ICT for agriculture knowledge management in Nigeria: lessons and strategies for improvement. Sci. J. Civil Agric. Res. Manag.
Nowak, A., Kijek, T., and Domańska, K. (2015). Technical efficiency and its determinants in the European Union. Agric. Econ. 61, 275–283.
Ortiz-Bobea, A., Ault, T. R., Carrillo, C. M., Chambers, R. G., and Lobell, D. B. (2021). Anthropogenic climate change has slowed global agricultural productivity growth. Nat. Clim. Chang. 11, 306–312. doi: 10.1038/s41558-021-01000-1
Otsuka, K. (2013). Food insecurity, income inequality, and the changing comparative advantage in world agriculture. Agric. Econ. 44, 7–18. doi: 10.1111/agec.12046
Pasha, H. A. (2015). Growth of the provincial economies. Institute for Policy Reforms (IPR). Available at: http://ipr.org.pk/wp-content/uploads/2016/04/GROWTH-OF-PROVINCIAL-ECONOMICS-.pdf. Accessed February 10, 2022.
Savari, M., and Amghani, S. M. (2021). Factors influencing farmers’ adaptation strategies in confronting the drought in Iran. Environ. Dev. Sustain. 23, 4949–4972. doi: 10.1007/s10668-020-00798-8
Shahbaz, P., Abbas, A., Aziz, B., Alotaibi, B. A., and Traore, A. (2022a). Nexus between climate-smart livestock production practices and farmers’ nutritional security in Pakistan: exploring level, linkages, and determinants. Int. J. Environ. Res. Public Health 19:5340. doi: 10.3390/ijerph19095340
Shahbaz, P., Boz, I., and Haq, S. U. (2021). Do socio economic characteristics of farming community really matter for the adoption of climate change strategies? A case study of Central Punjab, Pakistan. Fresenius Environ. Bull. 30, 80–92.
Shahbaz, P., Haq, S. U., and Boz, I. (2022c). Linking climate change adaptation practices with farm technical efficiency and fertilizer use: a study of wheat–maize mix cropping zone of Punjab province, Pakistan. Environ. Sci. Pollut. Res. 29, 16925–16938. doi: 10.1007/s11356-021-16844-5
Shahbaz, P., ul Haq, S., Abbas, A., Batool, Z., Alotaibi, B. A., and Nayak, R. K. (2022b). Adoption of Climate Smart Agricultural Practices through Women Involvement in Decision Making Process: Exploring the Role of Empowerment and Innovativeness. Agriculture 12:1161.
Skouloudis, A., Avlonitis, G. J., Malesios, C., and Evangelinos, K. (2015). Priorities and perceptions of corporate social responsibility: Insights from the perspective of Greek business professionals. Manag. Decision 53, 375–401. doi: 10.1108/MD-12-2013-0637
Sleiman, T., and Chahine, T. (2019). Rethinking design: responsible production processes for sustainable consumption in the UAE. Accelerating the Sustainable Development Goals through Digital Transformation:113.
Soule, M. J., Tegene, A., and Wiebe, K. D. (2000). Land tenure and the adoption of conservation practices. Am. J. Agric. Econ. 82, 993–1005. doi: 10.1111/0002-9092.00097
Toma, P., Miglietta, P. P., Zurlini, G., Valente, D., and Petrosillo, I. (2017). A non-parametric bootstrap-data envelopment analysis approach for environmental policy planning and management of agricultural efficiency in EU countries. Ecol. Indic. 83, 132–143. doi: 10.1016/j.ecolind.2017.07.049
Trinh, T. Q., Rañola, R. F. Jr., Camacho, L. D., and Simelton, E. (2018). Determinants of farmers’ adaptation to climate change in agricultural production in the central region of Vietnam. Land Use Policy 70, 224–231. doi: 10.1016/j.landusepol.2017.10.023
Ul Haq, S., and Boz, I. (2020). Measuring environmental, economic, and social sustainability index of tea farms in Rize Province, Turkey. Environ. Dev. Sustain. 22, 2545–2567. doi: 10.1007/s10668-019-00310-x
UNEP, (2016). Food Systems and Natural Resources. A Report of the Working Group on Food Systems of the International Resource Panel. eds. H. Westhoek, J. Ingram, S. Van Berkum, L. Özay, and M. Hajer.
United Nations, (2015). The seventeen goals. Available at: https://sdgs.un.org/goals. Accessed at May 05, 2022.
Van Meijl, H., Havlik, P., Lotze-Campen, H., Stehfest, E., Witzke, P., Domínguez, I. P., et al. (2018). Comparing impacts of climate change and mitigation on global agriculture by 2050. Environ. Res. Lett. 13:064021. doi: 10.1088/1748-9326/aabdc4
Keywords: farmer entrepreneurship, sustainable agriculture, sustainable development goal, sustainable production, natural resource management
Citation: Shahbaz P, Haq S, Abbas A, Azadi H, Boz I, Yu M and Watson S (2023) Role of farmers’ entrepreneurial orientation, women’s participation, and information and communication technology use in responsible farm production: a step towards sustainable food production. Front. Sustain. Food Syst. 7:1248889. doi: 10.3389/fsufs.2023.1248889
Edited by:
Umer Farrukh, Government College Women University Sialkot, PakistanReviewed by:
Niloofar Khalili, Leibniz Center for Agricultural Landscape Research (ZALF), GermanyMohd Anjum, Aligarh Muslim University, India
Copyright © 2023 Shahbaz, Haq, Abbas, Azadi, Boz, Yu and Watson. This is an open-access article distributed under the terms of the Creative Commons Attribution License (CC BY). The use, distribution or reproduction in other forums is permitted, provided the original author(s) and the copyright owner(s) are credited and that the original publication in this journal is cited, in accordance with accepted academic practice. No use, distribution or reproduction is permitted which does not comply with these terms.
*Correspondence: Azhar Abbas, Azhar.Abbas@uaf.edu.pk; Mark Yu, YU@tarleton.edu
†ORCID: Azhar Abbas orcid.org/0000-0003-2045-2971
Hossein Azadi orcid.org/0000-0002-5108-1993