- 1Department of Animal Science, University of Pretoria, Pretoria, South Africa
- 2Department of Information Science, University of Arkansas at Little Rock, Little Rock, AR, United States
- 3Division of Human Nutrition, Wageningen University, Wageningen, Netherlands
- 4Department of Animal Science, ARUA Centre of Excellence in Sustainable Food Systems, University of Pretoria, Pretoria, South Africa
High-quality food composition data are indispensable for improved decision-making in food security, health policy formulation, food labeling, diet formulation, agricultural policymaking, nutrition research, and many other nutrition-related activities. The optimisation of dietary patterns is a powerful tool to reduce the impact of malnutrition on a population’s health and well-being. Many countries in resource-poor settings lack a framework for developing and managing food composition data appropriate for these purposes. In the article, an overview of available food composition tables in Africa and the origin, use and limitations of theses tables are discussed. It is important that those working on any nutrition-related activity for resource-poor settings understand the limitations of current food composition data. Production of high-quality data requires the harmonization and adoption of international standards and guidelines across Africa. Moreover, continuity in the production, compilation and management of high-quality food composition data is challenged by suboptimal capacity building in terms of organizational, institutional and legal framework development. In this perspective article, the authors deliberate on challenges with a focus on Africa, while discussing new advances in food composition activities. Opportunities (such as the Internet of Things (IoT), wearable devices, natural language processing (NLP) and other machine learning techniques) to improve existing resources must be more actively explored and supported.
1. Introduction
Reliable, high-quality data on the composition of foods intended for human consumption is an important resource for different applications. These data are used in various ways throughout the food system, for example, but not limited to: (1) assessing the dietary intake of individuals or groups and carrying out epidemiological studies and clinical research, (2) formulating diets at the individual and/or population level, (3) educating consumers, (4) formulating agricultural and food policies in relation to public health, and (5) supporting industries for product labeling purposes and improved food processing methods.
There are currently over 150 food composition tables or nutrient databases, printed or electronic, in use worldwide (Schönfeldt and Pretorius, 2019). In general, the quantity, quality and availability of food composition data vary by country and region, but most developing countries still lack sufficient and reliable data (de la Revilla et al., 2023). Many tables are based on data from the United States Department of Agriculture’s (USDA) National Nutrient Database (Schönfeldt and Pretorius, 2019; de la Revilla et al., 2023). In 1968 “Food Composition Table for Use in Africa” (Leung, 1968) was developed and published. In 1988, a collection of data on the regional food composition data of foods most commonly eaten in Eastern Africa evolved from a table compiled for use in a joint research program in Tanzania on vitamin A deficiency (West et al., 1988). Most of this data has been derived from other tables and literature. A regional food composition database, the Composition of Selected Foods from West Africa, was published by the FAO in 2010 and revised in 2012. Most of the collected data were for raw foods, representing average values of the collected compositional data from 9 countries (Benin, Burkina Faso, Gambia, Ghana, Guinea, Mali, Niger, Nigeria and Senegal) (FAO, 2012). This food composition table was superseded by the 2019 Food Composition Tables for Western Africa (FAO, 2020). Over the years, some African countries have also published their own country food composition tables. A comprehensive list of the food composition tables available can be obtained from the Food and Agriculture Organization of the United Nations (FAO) International Network of Food Data Systems (INFOODS) page.1 Additional country-specific tables are also available either as limited addition printed documents or at a cost which restricts access to information.
The data, in especially the older tables but not limited to these tables, are based on a very limited number of samples, a limited number of foods and nutrients (missing values), and analytical methods that are outdated by today’s standards. However, these tables are still being used as there are limited up-to-date tables with country-specific data readily available. Additionally, the reuse of data may propagate nutritional composition values based on outdated analytical methods and equipment, as well as nutritional, food, and recipe values with inadequate descriptions and documentation (de la Revilla et al., 2023). A variety of factors influence food nutrient content over time, including agricultural practices, food policies, and consumer pressure, which are rarely reflected in databases. For example, the nutrient composition of maize and maize meal, a starchy staple in Sub-Saharan Africa can vary geographically due to environmental factors (e.g., soil type and climate), farming and food processing practices (e.g., fertilizer use, milling, storage) and policy (e.g., country-specific fortification) and these variations can be nutritionally relevant leading to an over- or underestimation of nutrient intake.
Despite having a multitude of use cases of undisputable importance, the management of food composition data (FCD) faces several challenges in Africa. This is particularly the case from a data quality management perspective, where ‘missing data’ continues to be a serious challenge for most food composition databases (FCDBs). A lack of adequate documentation and incomplete referencing of these databases may also hide important issues. Challenges in managing FCD can be experienced across its entire data lifecycle, from how the data are created, stored and distributed or shared, through to the consumption or use, and archival or disposal of such data. This article summarizes the authors’ specific viewpoint on current practices, challenges and new advances in the collection and use of food composition data for Africa.
2. Origin, uses and limitations of different types of food composition data
Diet is the most important determinant of disease globally, with large regional differences. Maternal and child malnutrition contributes for more than 25% to the loss of healthy life years in populations with a low socio-demographic index (Institute for Health Metrics and Evaluation (IHME), 2020). That includes many African countries. Relationships between diet and health have not only been shown at the level of the dietary pattern, food groups, and foods but especially also at the level of nutrients (Zinöcker and Lindseth, 2018). Hence, the World Health Organization recommends that the intake of saturated fat and sugar to be less than 10% of total energy intake and the intake of salt not to exceed 5 g per day (equivalent to sodium intake of less than 2 g per day) (WHO/FAO, 2003; FAO, 2010). Therefore, to establish relationships between diet and health, but also for monitoring and surveillance in public health nutrition, reliable information on the composition of foods is indisputable.
Food composition data are generally classified in their different types, based on the method used to collect or acquire the data. There are five recognized types, namely, analytical, copied or borrowed, calculated, imputed and presumed or assumed data. A combination of these methods is often used to create national or regional FCDBs (Kapsokefalou et al., 2019).
Analytical data are renowned for being the most accurate, as data are generated from foods that are commonly consumed in a particular region. Ideally, representative samples collected from selected foods are analyzed using standardized chemical methods. However, generating original analytical food composition data is very expensive in comparison to the other four types. This high cost has contributed to the fact that most FCDBs on the African continent only contain a relatively low amount of analytical data (De Bruyn et al., 2016).
Copied data are acquired from FCDBs, scientific articles, dissertations, laboratory reports and gray literature that were collected in other parts of the world. They are typically collected from foods that are grown and consumed in the country or region of origin, and not necessarily the country that is retrieving the data. It is less likely that copied data will accurately represent foods consumed in the region that is copying the data. This is mainly a result of several factors such as soil composition, climatic conditions, seasons, biodiversity, agricultural practices, industrial processing and food preparation. Thus foods grown and consumed in different geographical locations are unlikely to have the same composition (Ene-Obong et al., 2019). When copying data, guidelines for checking food composition data prior to publication must be established. FAO/INFOODS recommends adjusting proximates and water-soluble components when the moisture content between the foods differs by more than 10 percent, and adjusting fat-soluble components when the fat content differs by more than 10 percent (FAO, 2020).
Imputed data are estimates derived from analytical values obtained from similar food or for another form of the same food (Ispirova et al., 2020). Imputed data can also be a result of calculation from incomplete or partial analysis of food, e.g., chloride calculated from the value for sodium. Consequently, these values carry a lower degree of confidence as it may often be impossible to refer back to the original source and should be included only when original analytical data are not available or are known not to be of sufficient quality (Greenfield and Southgate, 2003).
Calculated data in the context of food composition refers to the values that are estimated or derived through calculations based on various methods rather than directly measured. The energy content of food is typically calculated using the Atwater system. Vitamin equivalents are used to express the total activity of a vitamin in a food or supplement, taking into account different forms of the vitamin that may have varying levels of biological activity. A third example is the procedure for estimating nutrient values for mixed dishes, recipes (including meal preferences and food habits), and processed foods, based on the composition of ingredients and corrected for preparation factors such as retention and yield factors (Greenfield and Southgate, 2003). Recipe calculation methodology is often used by industry for labeling of products or reformulation of existing products. However, there are many challenges associated with recipe analysis, including sourcing of the appropriate nutritional values, convert ingredient amounts from units and household measures to weights, availability of retention and yield factors and assign weight change factors (Church, 2015). Cooking conditions/preparation methods can vary considerably affecting yield and retention, therefore, results obtained by this calculation should almost always be observed as an approximation (Marconi et al., 2018).
The last type of food composition data referred to is presumed or assumed data. It is common for FCDBs to contain values that have been presumed or assumed as being at a certain level or as zero based on current knowledge of the food or current regulations. For example, cholesterol, retinol and vitamin D values are assumed to be zero in unfortified plant-origin foods (Kapsokefalou et al., 2019).
There is growing recognition that food consumption patterns guided through country-specific policies (such as food labeling, food based dietary guidelines (FBDG), food fortification, sodium reduction and taxation on high sugar foods) can reduce disability and premature deaths leading to improved health, well-being, and longevity (Delgado et al., 2021; Pretorius et al., 2021; Dembska et al., 2023). Dietary diversification, through the inclusion of food groups from different food cultures and habits, making it adaptable to different local contexts sustainable diets, can be pursued all over the world in a way that is healthy, accessible, affordable, safe, equitable and culturally acceptable (Dembska et al., 2023). Knowledge of the nutrient content of foods in local diets is necessary at the core of the development of these policies and regulations.
3. Management of food composition data
Relational databases are often used for the storage of FCD, although some countries still manually manage their data in spreadsheets. There are also countries that do not have their FCD in electronic format. Many countries and regional blocks have compiled their own FCDBs. While this localized FCD compilation is of huge importance (Delgado et al., 2021), there is also a need for sharing data between countries or regions, especially with the high cost associated with analytical data generation. To make this data sharing effective, and to unleash the benefits of comparing nutrients between countries or regional blocks, FCD must be standardized or harmonized through the use of standardized thesauri, and harmonized food descriptors and documentation. Food descriptor systems, such as the models created by the International Network of Food Data Systems (INFOODS) (Egan et al., 2007), the European Food Information Resource (EuroFIR) project (Pakkala et al., 2010) and the European Food Safety Authority (EFSA), have made a significant contribution to data harmonization between countries or regions. Even with these efforts, accessing and combining FCD from multiple sources remains a challenging task (Kapsokefalou et al., 2019).
As most FCD are managed in relational databases and/or spreadsheets, the ability to use this data for data analysis, reporting and decision-making, has significantly improved. However, there are still countries in Africa that either do not have the FCD in electronic form or have chosen not to publish them in electronic form. This later case makes access and usage of FCD a huge challenge, as it significantly reduces the ability to use the data for research, data analysis, reporting and decision-making by the ever-growing user community. Having FCD in electronic format also creates an opportunity for the data to be used in modern ways, with the use of mobile, wearable and other digital technologies (Traka et al., 2020). Figure 1 below depicts the wide coverage of modern FCD usage. The bidirectional nature of data flows in the image is an attempt to reflect the responsibility that FCD users have to collaborate and enrich the availability of data in FCDBs.
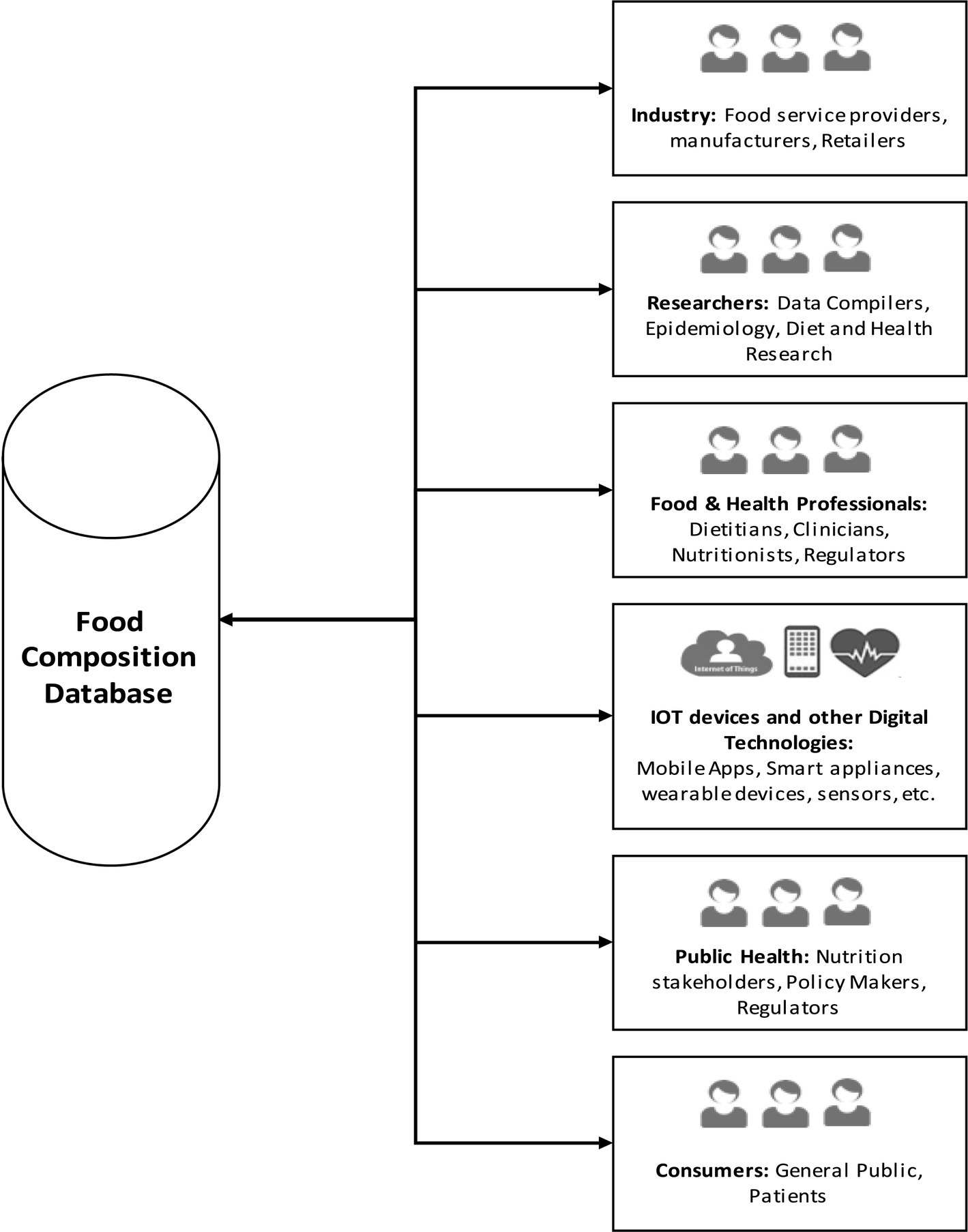
Figure 1. FCD users (adapted from Traka et al., 2020).
In most cases, access to national FCDBs is granted for free. Typically, data are made available online, in spreadsheets or CSV files that can be downloaded at no cost, or through a searchable web tool. However, there are still countries where access to the national FCDBs comes at a fee.
4. Challenges ahead of African food composition databases
Considering the many applications of FCD by different stakeholders, as depicted in Figure 1, high-quality food composition databases deserve a prominent role in all nutrition-related activities in all countries. Although nutrient databases are available in numerous African countries, verifying their accuracy can be challenging and have important limitations that impede relevant applications. These limitations include that only for a restricted number of (mostly raw) foods information is available with a limited coverage of nutrients for each food; the presence of many outdated and unreliable data; lack of nutrient information on underutilized nutrient-dense foods; incomplete food descriptions and poor component and value description; poor distinction between missing values and “zero” values, and poor documentation of data sources and assumptions, thereby complicating the assessment of the quality of the data. Data is sourced from diverse providers who may use different methods, often driven by availability rather than applicability. In addition, certain national laboratories primarily dedicated to food safety testing also perform food composition analysis. This situation can introduce challenges related to equipment reliability and the competence of laboratory personnel.
As laboratory facilities are sparse and nutrient analyzes expensive, most African FCT/FCDBs contain a limited amount of analytical values, and data are often copied from other databases from other parts of the world. For example, in the South African Food Data System, only 44% is from South African-referenced food items. The rest mainly contains copied values from FCDBs collected in other parts of the world, such as the United States and the United Kingdom (SAFOODS, 2017). The open data approach towards the management and use of FCD is an important factor that influences the high usage of the most popular FCDBs, such as the American FCDB (USDA) and British national FCDBs. However, as alluded before, data copied from databases elsewhere, are unlikely to be relevant within the country-specific context for which the database is compiled.
As a consequence of the confinements of food composition databases, errors can accumulate and propagate in the assessment of intake, leading to either an overestimation or underestimation of the proportion of the population that is below the RDA for a specific nutrient. In nutritional epidemiology, errors can lead to weakened associations. In individuals, overestimation or underestimation may lead to wrong advice in clinical practice or misleading consumer information, e.g., incorrect food labeling. Furthermore, data gaps and low-quality data affect areas beyond human nutrition, such as food systems sustainability and the pursuit of Sustainable Development Goals (Ferraz de Arruda et al., 2023). SDGs require food system sustainability, as well as consumers’ dietary shifts influenced by environmental concerns.
5. Conclusions and recommendations
Gathering and curating more and better data; the ability to establish connections between databases; and the ability to search through multiple FCDBs and easily find available data, are essential to the mitigation of further nutritional problems and to solving those already experienced. The use of AI techniques presents opportunities for African FCDBs to evolve and overcome some of these limitations. Artificial Intelligence (AI) techniques such as machine learning can be used as a potentially more accurate and cost effective way to generate imputed food composition data and increase the completeness of FCD in African FCDBs. This imputed data will complement the limited analytical data available, thereby, addressing the huge ‘missing data’ problem and making availability of high-quality food composition data, a more achievable goal for Africa. However, without having reliable historical and current data it will be difficult to train AI models (Ferraz de Arruda et al., 2023). In addition, sources of Big Data such as social media platforms, mobile apps, sensors, Internet of Things (IoT) devices, and wearable devices can all be leveraged to increase the amount, the accuracy, and timeliness of data coverage in African FCDBs. This can be achieved through the use of AI techniques known as natural language processing (NLP), and deep learning (Zhang et al., 2023). These are both subfields of machine learning, which is a form of AI. Intelligent and personalized search capabilities are an added advantage of AI-enabled systems. A specific example is the use of NLP to find specific food items or nutrients, across multiple databases, even if they use different terminologies or synonyms.
To expand the available information on the continent, a combination of AI techniques, specifically natural language processing, and other Information and Communication Technology (ICT) tools, can be used to establish connections between different African databases. Linking these data have the potential to enhance research and decision making for improved nutrition and food security (Zeb et al., 2021). The food system is driven by many actors, from farm to fork. During every phase of the food value chain, significant amounts of data are generated for example: consumer demand and market value, use of fertilizers and pesticides, environmental footprint and nutrient content of raw and final products. Composition and origin data of raw materials are used by manufacturers for product development. Food composition data are also used by the food industry for marketing and promotion or labeling purposes. Examples are “low in salt,” “reduced fat,” “no added sugar,” and “high in fiber.” The nutrition fraternity use this information towards consumer education and to shape consumer choice. Such AI use cases on FCD have already been implemented elsewhere. An example is FoodEx2, a food classification and description system covering different food safety domains maintained by (EFSA) (European Food Safety Authority (EFSA), 2015; Durazzo et al., 2022). Connections between FCDBs can complement information about a certain food or about region-specific indigenous food sources for a certain nutrient (Delgado et al., 2021). However, it must also be highlighted that establishing these connections will be made much easier by making more efforts to standardize and harmonize data (including FCDBs) across countries and regions in Africa.
To ensure the continuous compilation of food composition databases in Africa, it is necessary to focus on capacity building. This involves developing the skills and knowledge required to drive innovation and adhere to international standards and guidelines for managing food composition data. Higher education institutions play a crucial role in this process by providing the necessary training and expertise in areas such as big data, information and communication technology (ICT), and transdisciplinary collaboration, involving among others experts in food composition data, nutrition scientists, and AI specialists. However, capacity building goes beyond human resources development through education and training. It also encompasses organizational development, including the establishment of physical and management infrastructure, processes, and procedures that align with international standards. Additionally, an institutional and legal framework must be developed to gain recognition, authority, and enhance capacities. Advocacy skills, leadership skills, and communication skills are vital in all these domains. Together they define a pre-requirement of ensuring good quality data in food composition databases to provide Africa with safe, sufficient, nutritious and consumer-driven foods.
Data availability statement
The original contributions presented in the study are included in the article/supplementary material, further inquiries can be directed to the corresponding author.
Author contributions
BP and JM wrote the article. PH and HS critically reviewed the article. All authors contributed to the article and approved the submitted version.
Funding
The authors acknowledge(s) funding from the Economic and Social Research Council [grant number ES/T003871/1] under the ARUA-GCRF UKRI Partnership Program as part of the Capacity Building in Food Security (CaBFoodS-Africa) project and the Department of Science and Innovation (DSI)/National Research Foundation (NRF) South African Research Chairs Initiative (SARChl) in the National Development Plan Priority Area of Nutrition and Food Security (Unique number: SARCI170808259212). The grant holders acknowledge that opinions, findings and conclusions or recommendations expressed in this publication are that of the author(s) and that they accept no liability whatsoever in this regard.
Conflict of interest
The authors declare that the research was conducted in the absence of any commercial or financial relationships that could be construed as a potential conflict of interest.
Publisher’s note
All claims expressed in this article are solely those of the authors and do not necessarily represent those of their affiliated organizations, or those of the publisher, the editors and the reviewers. Any product that may be evaluated in this article, or claim that may be made by its manufacturer, is not guaranteed or endorsed by the publisher.
Footnotes
References
Church, S. M. (2015). The importance of food composition data in recipe analysis. Nutr. Bull. 40, 40–44. doi: 10.1111/nbu.12125
De Bruyn, J., Ferguson, E., Allman-Farinelli, M., Darnton-Hill, I., Maulaga, W., Msuya, J., et al. (2016). Food composition tables in resource-poor settings: exploring current limitations and opportunities, with a focus on animal-source foods in sub-Saharan Africa. Br. J. Nutr. 116, 1709–1719. doi: 10.1017/S0007114516003706
de la Revilla, L. S., Ferguson, E. L., Dooley, C., Osman, G., Ander, E. L., and Joy, E. J. M. (2023). The availability and geographic location of open-source food composition data used to estimate micronutrient intakes in sub-Saharan Africa: a scoping review. J. Food Compos. Anal. 120:105322. doi: 10.1016/j.jfca.2023.105322
Delgado, A., Issaoui, M., Vieira, M. C., Saraiva de Carvalho, I., and Fardet, A. (2021). Food composition databases: does it matter to human health? Nutrients 13:2816. doi: 10.3390/nu13082816
Dembska, K., Antonelli, M., Giosuè, A., Calabrese, I., Vaccaro, O., and Riccardi, G. (2023). The double pyramid: bridging nutrition and sustainability recommendations with traditional ways of eating. Eur. J. Clin. Nutr. doi: 10.1038/s41430-023-01278-8
Durazzo, A., Astley, S., Kapsokefalou, M., Costa, H. S., Mantur-Vierendeel, A., Pijls, L., et al. (2022). Food composition data and tools online and their use in research and policy: EuroFIR AISBL contribution in 2022. Nutrients 14, 1–14. doi: 10.3390/nu14224788
Egan, M. B., Fragodt, A., Raats, M. M., Hodgkins, C., and Lumbers, M. (2007). The importance of harmonizing food composition data across Europe. Eur. J. Clin. Nutr. 61, 813–821. doi: 10.1038/sj.ejcn.1602823
Ene-Obong, H., Schönfeldt, H. C., Campaore, E., Kimani, A., Mwaisaka, R., Vincent, A., et al. (2019). Importance and use of reliable food composition data generation by nutrition/dietetic professionals towards solving Africa’s nutrition problem: constraints and the role of FAO/INFOODS/AFROFOODS and other stakeholders in future initiatives. Proc. Nutr. Soc. 78, 496–505. doi: 10.1017/S0029665118002926
European Food Safety Authority (EFSA) (2015). The food classification and description system FoodEx 2 (revision 2). EFSA Support. Pub. 12, 1–90. doi: 10.2903/sp.efsa.2015.en-804
FAO. (2010). “Fats and fatty acids in human nutrition” in Report of an Expert Consultation (Rome: FAO Food and Nutrition Paper)
FAO. (2012). West African food composition table. Food and Agriculture Organization of the United Nations. Available at: https://www.fao.org/3/i2698b/i2698b00.pdf
FAO. (2020). FAO/INFOODS food composition table for Western Africa (2019). Food and Agriculture Organization of the United Nations. Available at: http://www.fao.org/publications/card/ru/c/CA7779B/
Ferraz de Arruda, H., Aleta, A., and Moreno, Y. (2023). Food composition databases in the era of big data: vegetable oils as a case study. Front. Nutr. 9:1052934. doi: 10.3389/fnut.2022.1052934
Greenfield, H., and Southgate, D. A. T. (2003). Food Composition Data: Production, Management and Use. FAO, Rome.
Institute for Health Metrics and Evaluation (IHME). (2020). Child and maternal malnutrition. In Global burden of disease 2019 risk factor summaries. Available at: https://www.healthdata.org/results/gbd_summaries/2019/child-and-maternal-malnutrition-level-2-risk
Ispirova, G., Eftimov, T., and Seljak, B. K. (2020). Evaluating missing value imputation methods for food composition databases. Food Chem. Toxicol. 141:111368. doi: 10.1016/j.fct.2020.111368
Kapsokefalou, M., Roe, M., Turrini, A., Costa, H. S., Martinez-Victoria, E., Marletta, L., et al. (2019). Food composition at present: new challenges. Nutrients 11:1714. doi: 10.3390/nu11081714
Leung, W. T. W. (1968). Food composition table for use in Africa. Available at: https://www.fao.org/3/x6877e/X6877E00.htm#TOC
Marconi, S., Durazzo, A., Camilli, E., Lisciani, S., Gabrielli, P., Aguzzi, A., et al. (2018). Food composition databases: considerations about complex food matrices. Foods 7:2. doi: 10.3390/foods7010002
Pakkala, H., Christensen, T., Martínez de Victoria, I., Presser, K., and Kadvan, A. (2010). Harmonised information exchange between decentralised food composition database systems. Eur. J. Clin. Nutr. 64, S58–S63. doi: 10.1038/ejcn.2010.212
SAFOODS (2017). SAMRC Food Composition Tables for South Africa. eds. A. van Graan, J. Chetty, and M. Jumat. 5th ed. South African Medical Research Council.
Pretorius, B., Ambuko, J., Papargyropoulou, E., and Schönfeldt, H. C. (2021). Guiding nutritious food choices and diets along food systems. Sustainability (Switzerland) 13:9501. doi: 10.3390/su13179501
Schönfeldt, H. C., and Pretorius, B. (2019). “Food composition” in Introduction to Human Nutrition. eds. S. A. Lanham-New, T. R. Hill, A. M. Gallagher, and H. H. Vorster. 3rd ed (Hoboken, NJ: Wiley-Blackwell), 56–73.
Traka, M. H., Plumb, J., Berry, R., Pinchen, H., and Finglas, P. M. (2020). Maintaining and updating food composition datasets for multiple users and novel technologies: current challenges from a UK perspective. Nutr. Bull. 45, 230–240. doi: 10.1111/nbu.12433
West, C. E., Pepping, F., and Temalilwa, C. R. (1988). The composition of foods commonly eaten in East Africa. Wageningen Agricultural University. Available at: https://library.wur.nl/WebQuery/wurpubs/fulltext/466505
WHO/FAO (2003). Diet, nutrition and the prevention of chronic diseases. World Health Organ. Tech. Rep. Ser. 916, 1–149. doi: 10.1093/ajcn/60.4.644a
Zeb, A., Soininen, J.-P., and Sozer, N. (2021). Data harmonisation as a key to enable digitalisation of the food sector: a review. Food Bioprod. Process. 127, 360–370. doi: 10.1016/j.fbp.2021.02.005
Zhang, Y., Deng, L., Zhu, H., Wang, W., Ren, Z., Zhou, Q., et al. (2023). Deep learning in food category recognition. Inf. Fusion 98:101859. doi: 10.1016/j.inffus.2023.101859
Keywords: food composition data, food security, information and communication technology (ICT) tools, machine learning, capacity building
Citation: Pretorius B, Muka JM, Hulshof PJM and Schönfeldt HC (2023) Current practices, challenges and new advances in the collection and use of food composition data for Africa. Front. Sustain. Food Syst. 7:1240734. doi: 10.3389/fsufs.2023.1240734
Edited by:
Uma Tiwari, Technological University Dublin, IrelandReviewed by:
Joycelyn Quansah, University of Ghana, GhanaAmélia Delgado, University of Algarve, Portugal
Maria Traka, Quadram Institute, United Kingdom
Copyright © 2023 Pretorius, Muka, Hulshof and Schönfeldt. This is an open-access article distributed under the terms of the Creative Commons Attribution License (CC BY). The use, distribution or reproduction in other forums is permitted, provided the original author(s) and the copyright owner(s) are credited and that the original publication in this journal is cited, in accordance with accepted academic practice. No use, distribution or reproduction is permitted which does not comply with these terms.
*Correspondence: Beulah Pretorius, beulah.pretorius@up.ac.za
†These authors share first authorship
‡ORCID: Beulah Pretorius, https://orcid.org/0000-0002-4599-8009
Junior M. Muka, https://orcid.org/0000-0001-7820-7595
Paul J. M. Hulshof, https://orcid.org/0000-0003-3260-2658
Hettie C. Schönfeldt, https://orcid.org/0000-0003-2388-4489