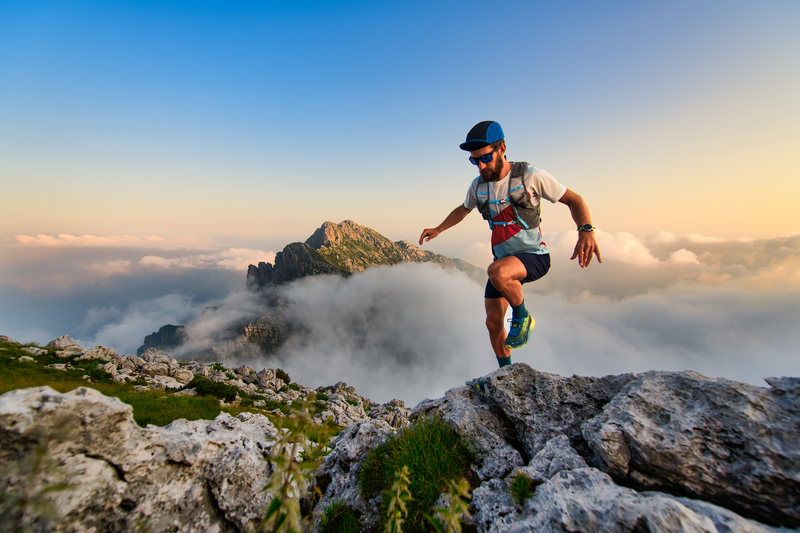
94% of researchers rate our articles as excellent or good
Learn more about the work of our research integrity team to safeguard the quality of each article we publish.
Find out more
ORIGINAL RESEARCH article
Front. Sustain. Food Syst. , 15 August 2023
Sec. Crop Biology and Sustainability
Volume 7 - 2023 | https://doi.org/10.3389/fsufs.2023.1239442
This article is part of the Research Topic Omics Approaches To Improve Crops Yields View all 7 articles
Cultivation of Robusta coffee is likely to gain importance because of its high disease resistance and climate envelope. Robusta coffee genetic resources conserved in field genebanks can play an important role to further improve its cupping quality and other agronomic traits, but such Coffea canephora collections are limited and still poorly characterized. In this study, we characterized the genetic composition of the historically important but until recently neglected INERA Coffee Collection in Yangambi (the Democratic Republic of Congo). We used GBS to discover genome-wide genetic diversity, created and validated a novel multiplex amplicon sequencing (HiPlex) screening assay to genetically screen 730 coffee shrubs of the Yangambi Coffee Collection, grouped clonal material and delineated 263 accessions with unique genetic fingerprints. Comparison to reference material of three genetic origins revealed that the majority of the Yangambi accessions were assigned a ‘Lula’ cultivar origin, four accessions were assigned to Congolese subgroup A and nine accessions were most closely related to local wild accessions. About one-quarter of the accessions was likely derived from hybridization between these groups, which could result from seed-based propagation of the collection, breeding efforts, or natural cross-pollination. Parental analyses discovered eight preferentially used accessions, which may correspond to historically selected founders, or direct descendants thereof, whose seed material was once widely used to establish coffee plantations. Finally, two core collections were proposed using the maximization strategy (CC-I; 100 accessions) and genetic distance method (CC-X; 10 accessions). Our study demonstrates a method for the genetic characterization of Robusta coffee collections in general and contributes to the re-evaluation and exploration of the Robusta coffee genetic resources in the Democratic Republic of the Congo in particular.
Coffee is the world’s most widely consumed hot beverage and the second-most exported product from developing countries (Pendergast, 2009 Lashermes, 2018). Coffee belongs to the Rubiaceae family, and to the genus Coffea that comprises 131 species (Davis and Rakotonasolo, 2021; Stoffelen et al., 2021) of which only Coffea arabica L. (Arabica coffee) and C. canephora Pierre ex A. Froehner (Robusta coffee) are cultivated at a commercial scale. C. canephora has the widest native distribution range among all Coffea species, ranging from West Africa through Cameroon, Central African Republic, Republic of the Congo, the Democratic Republic of the Congo (DRC), Uganda, northern Tanzania down to northern Angola (Cubry et al., 2013). Within its native distribution range, two major origin groups were previously identified, namely the Congolese and Guinean groups (Montagnon et al., 1992; Dussert et al., 1999; Cubry et al., 2013). The Congolese group was further subdivided into seven subgroups: subgroup A in Gabon, the Republic of the Congo and western DRC, subgroup E in the DRC, subgroup C in Cameroon and the western Central Africa region, subgroup B in eastern Central African Republic, subgroup O in Uganda (Gomez et al., 2009), and the two most recently described: subgroup G in Angola and subgroup R in southern DRC (Merot-l'Anthoene et al., 2019). The Guinean group currently corresponds to group D. Of these eight origin groups, materials derived from mainly Congolese subgroup E and subgroup A or the Guinean group D are assumed to be used for C. canephora cultivation (Leroy et al., 1993; Montagnon et al., 1998a; Oliveira et al., 2018).
C. canephora was initially cultivated at a small scale in the late 19th century in Gabon, Angola, Uganda, and the Sankuru region of the DRC (Durand et al., 1898; Chevalier, 1929; Montagnon et al., 1998a; Vanden Abeele et al., 2021). At that time, Arabica coffee cultivation in Asia was threatened by leaf rust disease, and plant hunters were searching for alternative coffee species from Africa. After several unsuccessful attempts to introduce novel species like Coffea liberica, Linden launched in 1900 a robust, pest-resistant, and productive coffee species under the name “Coffea robusta.” These genetic resources were introduced in the trials of the Java Coffee Research Station, which became the first important breeding and distribution center of Robusta (Ferrão et al., 2019). From 1910 on, Robusta was promoted and distributed as an important colonial cash crop, further stimulating Robusta coffee research and breeding activities and leading to the establishment of the Lula Coffee Research Station in the DRC, which was later integrated in the Institut National pour l’Etude Agronomique du Congo Belge (INEAC; after the independence of the DRC, the institute was renamed to INERA). In 1927, the Yangambi Research Station was established less than 100 km from the INERA Coffee Research Station in Lula, starting with a coffee research program focused on Robusta material derived from the Java Coffee Research Station and the INERA Coffee Research Station in Lula. Subsequently, the INERA Coffee Collection in Yangambi was enriched with other wild and cultivated material from the DRC (e.g., from the INERA Coffee Collection in Luki), and abroad, bringing C. canephora genetic resources from different origin groups together. From 1930 until 1960, the Yangambi Research Station (meanwhile also part of the INERA), was taking the lead in Robusta breeding in the DRC, and was distributing ‘Lula’ and ‘INEAC’ elite breeding lines worldwide (Coste et al., 1955; Montagnon et al., 1998b). In 1951–1952, seven mother plants with improved pest resistance, productivity, and quality, were created and their seeds were mixed to form a standard seed material blend that was widely distributed for the creation of plantations (Capot, 1962). With its large number of C. canephora genetic lines (wild accessions and cultivars) and its coffee research program, the Yangambi Research Station became the most important C. canephora selection center by 1950 (Montagnon et al., 1998b).
The once very rich INERA Coffee Collection in Yangambi did not escape the many difficulties the DRC has faced during the last decades and was decimated due to lack of appropriate care and funding (Stoffelen et al., 2019). Since 2016, initiatives have been undertaken to rehabilitate the INERA Coffee Collection in Yangambi and part of those efforts concern (genetic) characterization of the plant material. The collection is, especially since 2020, further enriched with numerous new accessions with a wild and cultivated origin collected from several regions within the DRC. It is currently not known how many and which of the ‘Lula’ and ‘INEAC’ elite breeding lines and other wild and local cultivated material from the original INERA Coffee Collection in Yangambi remain and whether they still correspond to the plant material currently grown in the field genebank. A preliminary survey of the collection management revealed several issues. First, inconsistencies were found between the field maps of the field genebank and the labeled accessions present on the field, and many accessions were missing their original plant label. Second, a broad phenotypic diversity for various morphological and agronomical traits was observed within plots that were assumed to contain clonally propagated (i.e., genetically identical) plant material suggesting incorrect labeling (based on field observations 2020–2021, data not shown). Last, years of multiplication of accessions through sexual propagation (i.e., through seedlings rather than cuttings) and open pollination resulted in the hybridization of the initial accessions rather than maintaining unique lines. Vanden Abeele et al. (2021) provided a first exploratory screening of 45 coffee shrubs from the INERA Coffee Collection in Yangambi using Simple Sequence Repeat (SSR) markers and found that the majority of those accessions, which are referred to as Lula varieties, presumably originated from the Coffee Research Station in Lula. In addition to these Lula varieties, that study identified several rare cultivars originating from the North Kivu, Orientale province, and Equateur province, and four Petit Kwilu cultivars, presumed to originate from western DRC, Republic of the Congo, and Gabon. Vanden Abeele et al. (2021) could also identify two wild genotypes originating from the Ituri and Tshopo provinces (DRC).
Establishing a core collection is key to the future sustainable and effective conservation management and use of the present INERA Coffee Collection in Yangambi, which highly likely contains valuable cultivated and wild genetic resources for coffee production and breeding. A core collection is a subset of the entire collection of germplasm (seeds, plants, or tissues) of a particular species that captures the most diversity with minimal redundancy (Brown, 1989). Currently, there are two complementary, commonly used strategies to construct a core collection: i) the maximization (M) strategy, which focuses on selecting the most diverse loci to maximize the genetic diversity of the core collection and ii) the genetic distance method, which aims to select the most diverse plant material within a collection to maximize the genetic distance between the entries of the core collection (Gu et al., 2023). Leroy et al. (2014) used these two core collection strategies to propose core collections of the genetic resources of C. canephora based on 565 C. canephora accessions collected from the Ivory Coast, Uganda, the DRC, and French Guyana and characterized them with 13 SSR markers. Using three different core sizes (12, 24, and 48 entries), they proposed seven core collections that can be used as a valuable tool for diversity management and preserving the genetic resources of C. canephora, or to serve as a solid basis for breeding programs. By combining the M strategy and genetic distance method, Leroy et al. (2014) created an optimal core collection containing 77 accessions, which can be effectively utilized in research centers and in the context of improving coffee production through breeding efforts.
In this study, we genetically characterized 730 coffee shrubs carefully selected from the pre-2020 INERA Coffee Collection in Yangambi (hereafter referred as “Yangambi coffee collection”) with the aim to: (i) discover genome-wide genetic diversity in an initial screen of a Discovery Panel (n = 218 individuals); (ii) design a multiplex amplicon sequencing (HiPlex) screening assay based on discriminatory loci to identify unique genetic fingerprints; (iii) validate the HiPlex assay by comparison of SNPs, haplotype calls, and genetic fingerprints to genome-wide GBS data (Validation Panel, n = 105); (iv) genotype the Yangambi coffee collection (Screening Panel, n = 730), to classify the 730 individuals in clonal groups (with identical genetic fingerprints) and delineate accessions with unique genetic fingerprints, perform parentage analysis to identify kinship and the underlying network of genetic relationships, and propose representative core collections; (v) explore the genetic structure and origin of the Yangambi coffee collection based on comparison to reference samples (Canephora Panel, n = 514), specifically ‘Lula’ cultivars, cultivars from the INERA Research Station in Luki, Congolese subgroup A, and local wild coffee genotypes from the Yangambi rainforest. These five objectives were aligned to subsequent steps of the data analysis workflow, including subsets of plant materials (Panels), molecular marker sets, software and selection criteria, as outlined in Figure 1.
Figure 1. Overview of the data analysis workflow. Step I, primary screen: a set of diverse genotypes (“Discovery Panel,” n = 218) was compiled based on the field maps and plant labels and genotyped using genome-wide molecular markers (Genotyping-by-Sequencing) to identify unique genetic fingerprints. Step II, HiPlex amplicon design: a high-throughput screening assay based on multiplex amplicon sequencing (HiPlex) was created with sufficient genetic resolution to discriminate the unique genetic fingerprints. Step III, validation of the HiPlex assay: 105 samples with high locus completeness for both GBS and HiPlex data (“Validation Panel”) were compared on the level of high-quality loci, SNPs and haplotypes, Jaccard Inversed Distances (JID) and unique genetic fingerprints. Step IV, genetic composition of the Yangambi coffee collection: the HiPlex assay was then used to comprehensively screen the Yangambi coffee collection (“Screening Panel,” n = 730) and to identify all unique genetic fingerprints. Then, a parentage analysis was used to investigate kinship and to delineate which genotypes were preferentially used for seed-based propagation. In addition, the M strategy (CC-I) and FIGURE 1 (Continued)genetic distance method (CC-X) were tested for the Yangambi coffee collection to propose complementary core collections. Step V, origin detection in the Yangambi coffee collection: in addition to the representatives of the unique genetic fingerprints in the Yangambi coffee collection, reference material for the rainforest in the Yangambi region and Luki coffee collection were genotyped using the HiPlex assay and reference material for Congolese subgroup A was genotyped using WGS (“Canephora Panel,” n = 514). All samples were screened together and fastSTRUCTURE and principal component analysis (PCA) were used to reveal genetic structure in the Yangambi coffee collection, and to estimate the relative abundance of plant material of the different origin groups in the collection.
In this study, we used four different “panels” of plant materials (Discovery, Validation, Screening and Canephora Panel) for two main goals: (i) creation and validation of a HiPlex screening assay and (ii) characterization of the genetic structure and composition of the Yangambi coffee collection (Figures 1, 2A). The “Screening Panel” was a set of 730 samples collected from the INERA Coffee Collection in Yangambi giving the most broad representation of the collection based on field maps and plant labels. This panel was used to identify clonal materials, delineate accessions (unique genetic fingerprints) in the Yangambi coffee collection, to investigate kinship relationships in the collection and to create two complementary core collections. A primary screen of genetic diversity of the Yangambi coffee collection was performed on a subset of 218 samples of the Screening Panel, creating the “Discovery Panel.” Samples in this panel were selected based on field maps and plant labels to have a representation of the assumed genetic diversity of the INERA Coffee Collection in Yangambi. The Discovery Panel was additionally used to selected a minimal set of loci that could discriminate all the unique genetic fingerprints in the Discovery panel to design a HiPlex screening assay. Validation of the HiPlex screening assay was performed on a subset of 105 samples of the Discovery panel, namely the “Validation Panel.” Because validation of genotype calls per sequencing technique requires good quality data of both, the selection of samples for the Validation Panel was based on data completeness for both GBS and HiPlex data. Only samples with GBS data for >80% of the 86 high-quality HiPlex loci were retained in this panel. The “Canephora Panel” (n = 514) contained representative samples of all 263 unique genetic fingerprints of the Yangambi coffee collection and reference material of three potential origin groups, namely Congolese subgroup A (n = 2), ‘Luki’ cultivars (n = 14) and wild genotypes growing in the rainforest in the Yangambi region (n = 235). This panel was used to investigate the genetic composition of the Yangambi coffee collection.
Figure 2. Validation of the HiPlex screening assay. (A) Comparison of SNPs and haplotype calls between HiPlex and GBS data. (B) Pairwise Jaccard Inversed Distances was calculated for all sample pairs within 105 samples based on 10.257 GBS haplotypes within 3,125 polymorphic high-quality GBS loci. (C) Pairwise Jaccard Inversed Distances was calculated for all 105 samples based on 300 HiPlex haplotypes within 86 high-quality HiPlex loci. (D) Correlation between Jaccard Inversed Distances of GBS (X-axis) and HiPlex (Y-axis), with prediction interval shown as red lines and the 95% confidence intervals as green lines.
Leaf material from 730 coffee shrubs (“Screening Panel,” n = 730) was collected from the INERA Coffee Collection in Yangambi (see details in Supplementary Table S1). Genomic DNA was extracted from 20 to 30 mg dried leaf material using an optimized cetyltrimethylammonium bromide (CTAB) protocol adapted from Doyle and Doyle (1987). DNA quantities were measured with the Quantifluor dsDNA system on a Quantus Fluorometer (Promega, Madison, United States). Of these 730 samples, 218 were subjected to GBS (“Discovery Panel,” n = 218) and all 730 samples were subjected to the HiPlex assay (see below). Three genetic resources external to the INERA Coffee Collection in Yangambi were used as reference for potential origin groups. First, genomic DNA extracts of 235 wild coffee shrubs collected from the local rainforest in the Yangambi region were obtained from Depecker et al. (2023). Second, genomic DNA extracts of 14 herbarium coffee samples collected from the INERA Coffee Collection in Luki were supplied by Meise Botanic Garden, Belgium, hereafter referred to as ‘Luki’ cultivars. All samples collected from the local rainforest and from the INERA Coffee Collection in Luki were subjected to the HiPlex assay. Third, whole genome shotgun (WGS) sequencing data of a wild sample from Republic of the Congo (accession 20708) and a cultivated sample from Togo (accession 20723), previously described by Merot-l'Anthoene et al. (2019) were retrieved from NCBI Sequence Read Archive (SRA) (Tournebize et al., 2022; PRJNA803612), and were used as reference for the Congolese subgroup A.
Following Depecker et al. (2023), GBS libraries of the 218 samples of the “Discovery Panel” were prepared (Figure 1, Step I) using a double-enzyme GBS protocol adapted from Elshire et al. (2011) and Poland and Rife (2012). In short, 100 ng of genomic DNA was digested with PstI and MseI restriction enzymes (New England Biolabs (NEB), Ipswich, United States), and barcoded and common adapters were ligated with T4 ligase (NEB) in a final volume of 35 μL. Ligation products were purified with 1.6x MagNA magnetic beads (GE Healthcare Europe, Machelen, BE) and eluted in 30 μL TE. Of the purified DNA eluate, 3 μL was used for amplification with Taq 2x Master Mix (NEB) using an 18 cycles PCR protocol. PCR products were bead-purified with 1.6x MagNA, and their DNA concentrations were quantified using a Quantus Fluorometer. The library quality and fragment size distributions were assessed using a QIAxcel system (Qiagen, Venlo, NL). Finally, equimolar amounts of the GBS libraries were pooled, bead-purified, and 150 bp paired-end sequenced on an Illumina HiSeq-X instrument by Admera Health (South Plainfield, United States).
Reads were processed with a customized script available on Gitlab.1 First, the quality of sequence data was validated with FastQC v0.11 (Andrews, 2010) and reads were demultiplexed using Cutadapt v2.10 (Martin, 2011), allowing zero mismatches in barcodes or barcode-restriction site remnant combination. Next, the 3′ restriction site remnant and the common adapter sequence of forward reads and the 3′ restriction site remnant, the barcode, and the barcode adapter sequence of reverse reads were removed based on sequence-specific pattern recognition and positional trimming using Cutadapt v2.10. After trimming the 5′ restriction site remnant of forward and reverse reads using positional trimming in Cutadapt v2.10, forward and reverse reads with a minimum read length of 60 bp and a minimum overlap of 10 bp were merged using PEAR v0.9.11 (Zhang et al., 2014). Merged reads with a mean base quality below 25 or with more than 5% of the nucleotides uncalled and reads containing internal restriction sites were discarded using GBprocesS. Finally, merged reads were aligned to the C. canephora reference genome sequence (Denoeud et al., 2014) with the BWA-mem algorithm in BWA v0.7.17 (Li, 2013) with default parameters. Alignments were sorted, indexed, and filtered on mapping quality above 20 with SAMtools 1.10 (Li et al., 2009). Next, high-quality GBS loci and Stack Mapping Anchor Points (SMAPs) were identified in the mapped reads using the SMAP delineate module within the SMAP package v4.4.0 (Schaumont et al., 2022)2 with mapping_orientation ignore, min_stack_depth 4, max_stack_depth 400, min_cluster_depth 8, max_cluster_depth 400, completeness 90, and min_mapping_quality 20.
Single nucleotide polymorphisms (SNPs) were called with GATK (Genome Analysis Toolkit) Unified Genotyper v3.7.0 (McKenna et al., 2010). SNP calling was the same for GBS and HiPlex (see below). Only SNPs within high-quality GBS loci as identified with the SMAP delineate module, for GBS read data, or within the 86 high-quality HiPlex loci, for HiPlex read data, were retained. SNPs were filtered using the following parameters: min-meanDP 30, mac 4, and minQ 20, and multi-allelic SNPs were removed with GATK. The remaining SNPs were then subjected to further filtering with the following parameters: minDP 10, minGQ 30, minQ 30, min-alleles 2, max-alleles 2, and maf 0.05 using VCFtools v0.1.16 (Danecek et al., 2011). Only SNPs with a minimum read depth of 10 were retained using a customized Python3 script.
Per GBS or HiPlex locus, haplotypes were called using the SMAP haplotype-sites module within the SMAP package v4.4.0. Read-backed haplotyping was conducted based on the combined variation in SMAPs and SNPs in the GBS read data or based on SNPs in the HiPlex read data using the SMAP haplotype-sites module with mapping_orientation ignore, partial exclude, no_indels, min_read_count 10, min_distinct_haplotypes 2, min_haplotype_frequency 5, discrete_calls dosage, frequency_interval_bounds 10 10 90 90, and dosage_filter 2.
The genetic similarity between samples within the Discovery (n = 218), Validation (n = 105), Screening (n = 730), and Canephora Panel (n = 514) was quantified with the SMAP grm module within the SMAP package v4.4.0, using the Jaccard Inversed Distance (Jaccard, 1912) that was calculated based on the discrete dosage haplotype calls in polymorphic GBS or 86 high-quality HiPlex loci. SMAP grm was run with locus_completeness 0.1, similarity_coefficient Jaccard, distance_method Euclidean, locus_information_content Shared, and partial FALSE creating a pairwise Jaccard Inversed Distance (JID) matrix.
Distribution of JID values across all pairwise comparisons revealed a group of samples with pairwise JID in the range 0.977–1 (GBS data) or 0.965–1 (HiPlex data), which contained the known technical replicates, and a separate group of sample pairs with pairwise Jaccard Inversed Distance ranging between 0.44 and 0.89 (GBS data), respectively 0.32 and 0.88 (HiPlex data) (see Supplementary Figure S1). A JID of 1 means identical haplotype calls at all detected loci (i.e., genetically identical). In practice, a Jaccard Inversed Distance in the range of 0.965 to 1, based on our 86 high quality HiPlex loci, means that one HiPlex locus out of all detected loci in the sample pair may display a different haplotype constitution between two samples (for instance a single instance of a false negative or false positive detection of a haplotype, due to technical errors). Therefore, the minimal JID of 0.977 (for GBS data) or 0.965 (for HiPlex data) was used as a threshold to identify all pairs of genetically identical samples (i.e., clones). All other genotypes (i.e., genetic fingerprints) were considered as different accessions.
To construct a HiPlex assay, a minimal set of loci that could discriminate all 139 unique genetic fingerprints in the Discovery Panel was selected according to the following strategy (Figure 1, Step II). Due to technical restrains for multiplex amplicon sequencing library preparation and sequencing, amplicons must be designed in a narrow length window: max 20 bp difference between the shortest and the longest amplicon. To design primer pairs within GBS loci, while avoiding SNPs at primer binding sites, the GBS loci should have a length of 100–140 bp, and no read mapping polymorphisms (i.e., two SMAPs). To select the suitable loci, SMAP delineate was run on the GBS read data with the following parameters; mapping_orientation ignore, min_stack_depth 4, max_stack_depth 400, min_cluster_depth 8, max_cluster_depth 400, max_smap_number 2, and completeness 90. Our GBS library preparation, which included a size selection step, displayed a strong bias toward short fragments (mostly <100 bp). Out of thousands of GBS loci that were used for genome-wide fingerprinting, only 483 loci had a length of 100–140 bp and two SMAPs and could be used for HiPlex amplicon design (target amplicon size range: 95–115 bp). To check for discriminative power across the 218 samples of the Discovery Panel, haplotypes were called for the loci with two SMAPs and length 100–140 bp and pairwise JID were calculated using the SMAP grm module. Next, HiPlex primer design was performed by running Primer3 v2.4.0 (Untergasser et al., 2012) implemented in the SMAP snp-seq utility tool on the 483 selected loci with parameter settings -d 300 -t 50 -u 20 -min 95 -max 115 -max_mis 12 -ex 10, taking all known GBS SNP positions into account. Only 139 suitable loci met the criteria for good primer design, had no SNPs at primer binding sites, and one or more SNPs between the primers. Genotype calls within the designed amplicons were simulated using the GBS read data and the amplified loci nested within the GBS loci. To check if each GBS-based genetic fingerprint (based on GBS markers) could still be differentiated using the simulated HiPlex genotype calls, haplotypes were called using SMAP haplotype-sites and pairwise JID was calculated using SMAP grm based on the simulated HiPlex genotype calls. Third, 96 loci with two to four haplotypes per locus were selected, without losing the loci that discriminate highly similar genetic fingerprints.
To validate the HiPlex assay, samples of the Discovery Panel (n = 218) were subjected to HiPlex sequencing (Figure 1, Step III). HiPlex amplification reactions and library preparations were done by Floodlight Genomics LLC (Knoxville, United States). The libraries were sequenced with 150 PE on a HiSeq3000 instrument (Admera Health, South Plainfield, United States). Forward and reverse reads were merged with PEAR v0.9.11 (Zhang et al., 2014), and the merged reads were aligned to the C. canephora reference genome sequence (Denoeud et al., 2014) with the BWA-mem algorithm in BWA v0.7.17 (Li, 2013) with default parameters. Alignments were sorted, indexed, and filtered on mapping quality above 20 with SAMtools v1.10 (Li et al., 2009).
Distribution of reads depth across the 96 HiPlex loci was analyzed to select high-quality HiPlex loci. Ten loci showed completeness lower than 10% and were excluded from further analysis.
We selected 105 samples with GBS data in at least 80% of the 86 high-quality HiPlex loci, in both GBS read data and HiPlex read data, further referred to as the “Validation Panel” (n = 105), and ran GATK on all these bam files together. To compare the polymorphisms within the GBS and HiPlex SNP data, SNPs within the 86 high-quality HiPlex loci were called with GATK with the same parameters as above (SNP calling) on all GBS and HiPlex bam files, resulting in a SNP call file for the two genotyping techniques together. GBS SNPs were separated from HiPlex using the keep function in VCFtools and only polymorphic SNPs were retained by using a minor allele count of 2 for GBS and HiPlex data separately. On a per-sample basis, all SNP genotype calls derived from GBS data were compared to those of HiPlex data across the 86 high-quality HiPlex loci. Read-backed haplotyping was conducted with the SMAP haplotype-sites module based on the SNPs within the 86 high-quality HiPlex loci for both GBS and HiPlex read data. On a per-sample basis, all haplotype calls derived from GBS data were compared to those of HiPlex data across the 86 high-quality HiPlex loci (Figure 3A).
Figure 3. Overview of the Discovery, Validation, Screening, and Canephora Panel set. (A) Number of samples used within and shared between the four different panel sets. The black line indicates the unique genetic fingerprints identified within the Yangambi coffee collection. (B) Number of unique and common HiPlex SNPs found within and between the Validation, Screening, and Canephora Panel set. The number of GBS SNPs within the Discovery Panel was placed separately to indicate the difference in magnitude between GBS and HiPlex. (C) Number of unique and common HiPlex haplotypes found within and shared between the Validation, Screening, and Canephora Panel set. The number of GBS haplotypes within the Discovery panel was placed separately to indicate the difference in magnitude between GBS and HiPlex.
Since the selection of HiPlex loci can introduce bias in the calculation of genetic distances between all pairs of samples and the population structure is calculated on these genetic distances, we calibrated whether pairwise JID calculated on GBS data (Figure 3B) was correlated to that based on HiPlex data (Figure 3C). Pairwise JID was calculated with the same parameters as above (see Genetic similarity), once based on the GBS data within all polymorphic high-quality GBS loci and once based on the HiPlex data within the 86 high-quality HiPlex loci. A correlation between the pairwise JID in the GBS data and HiPlex data was calculated using the Kendall rank correlation test in the “ggpubr” R package (Kassambara and Kassambara, 2020). Prediction values and confidence intervals were analyzed with the predict function in R.
To reveal parent-progeny pairs in the Yangambi coffee collection, a parentage analysis was performed on the 263 representatives of the unique genetic fingerprints of the collection (Figure 1, Step IV). We first ran an allele frequency analysis on the 86 high-quality HiPlex loci using the program CERVUS v3.0.7 (Kalinowski et al., 2007). Next, a parentage analysis simulation, which uses a pairwise likelihood comparison-based approach to assign parent pairs with unknown sexes, was run for 10,000 progenies produced by 263 candidate parents, with 30% parent samples, 50% proportion of loci sampled, 1% proportion of loci mistyped, and confidence levels assessed by LOD distribution (relaxed >80%, strict >95%). Based on the simulation, all 263 unique genetic fingerprints were tested as both progeny and parent, and only parent-progeny pairs with strict (>95%) confidence levels were retained. The network between the retained parent-progeny pairs was manually created in Adobe Illustrator.
Core Hunter 3 v3.2.0 R package (De Beukelaer et al., 2018) was used to test two core collection strategies for the 263 unique genetic fingerprints within the Yangambi coffee collection (Figure 1, Step IV): the M strategy (hereafter referred to as CC-I), which focuses on selecting the most diverse loci to maximize the genetic diversity and; the genetic distance method (hereafter referred to as CC-X), which aims to select the most diverse plant material within a collection to maximize the genetic distance between the entries of the core collection. For both core types allele coverage (CV), diversity within and between alleles [He and Shannon’s index (SH)], and average genetic Modified Roger’s (MR) distance between entry-accession (AN) and entry-to-nearest-entry (EN) were calculated for nine different core sizes (3, 5, 10, 25, 50, 100, 150, 200, and 263 accessions). Core collection size 263 is a representation of all unique genetic fingerprints currently identified in the Yangambi coffee collection. For CC-I, an optimal core size was determined based on maximized genetic diversity (He and SH) and minimized AN distance. For CC-X, an optimal core size was determined based on maximized genetic diversity (He and SH) and maximized EN distance. The function set.seed 100 was used to eliminate randomness in assigning accessions to the core subsets. Accessions were assigned to the core subset using the function sampleCore within the Core Hunter 3 R package with objective AN (CC-I) or EN (CC-X) and MR, steps 500, and size equals the optimal core size.
To investigate genetic structure, composition and origin of the Yangambi coffee collection (Figure 1, Step V), HiPlex read data of representatives of the 263 unique genetic fingerprints, 14 ‘Luki’ cultivars, 235 wild coffee shrubs, and WGS read data of two samples of the Congolese subgroup A was mapped on the reference genome sequence as described above and used for joined SNP calling on 86 high-quality HiPlex loci. Based on documentation present in the INERA Coffee Collection in Yangambi, seven samples (G0094FOG_2065, G0119FOG_2624, G0105FOG_2676, G0198FOG_2800, G0121FOG_2824, G0138FOG_3231, and G0197FOG_3232) were ‘Lula’ cultivars (Supplementary Table S1). A principal component analysis (PCA) was performed using the R package ADEGENET (Jombart, 2008). Additionally, a Bayesian clustering implemented in fastSTRUCTURE v1.0 (Raj et al., 2014) was run given the most optimal number of genetic clusters (K). Hundred iterations were run for each expected cluster setting K, ranging from 2 to 9. The StructureSelector software (Li and Liu, 2018) was used to determine the most optimal number of K, by first plotting the mean log probability of each successive K and then using the Delta K method following Evanno et al. (2005).
Based on available documentation (field maps and plant labels), a set of 218 samples (Discovery Panel) was selected from the INERA Coffee Collection in Yangambi and subjected to GBS (Figure 1, Step I), yielding 7,627 high-quality GBS loci with read depth > 8 in more than 90% of the samples. These GBS loci had two types of polymorphic sites, namely 18,225 read mapping polymorphisms (SMAPs) identified by the SMAP delineate module and 11,488 SNPs called with GATK (referred to as GBS SNPs; Figure 2). The polymorphic sites (SNPs and SMAPs) were converted into 10,257 haplotypes using the SMAP haplotype-sites module (referred to as GBS haplotypes), yielding a genome-wide marker set of 3,177 polymorphic high-quality GBS loci.
The Jaccard Inversed Distance (JID) matrix, constructed with the SMAP grm module, was arranged to reveal blocks of sample pairs with high similarity (Supplementary Figure S1). In addition, the distribution of all pairwise JID values showed a group of sample pairs with similarities greater than 0.977, which, as expected, contained known replicates (Supplementary Figure S1). Therefore, we used the minimal JID of the group of sample pairs containing the known replicates (JID > 0.977) as a threshold to identify all pairs of genetically identical samples (i.e., clones). In turn, sample pairs with JID values below this threshold were considered different accessions, and a simple iterative sorting of the JID matrix was used to group all samples into a minimal set of unique genetic fingerprints, yielding 139 groups with unique genetic fingerprints (here labeled like G0001) (Supplementary Table S1). Pairwise JID between the 139 unique genetic fingerprints ranged between 0.44 and 0.89. This grouping confirmed that a priori known replicates were genetically identical. However, this analysis also revealed that within unique genetic groups, some individuals had distinct “documented identities” according to field maps and plant labels, indicating incorrect labeling. Conversely, some individuals with the same documented identity were divided over distinct genetic groups, e.g., samples with documented identity “YB001” comprised a total of four different unique genetic fingerprints with a JID range of 0.67–0.81 (Supplementary Table S1).
Next, a multiplex amplicon sequencing assay was designed (Figure 1, Step II). First, to comply with primer design criteria (see Material and Method), 483 GBS loci without read mapping polymorphisms (i.e., two SMAPs) and locus length between 100 and 140 bp were selected within the 3,177 polymorphic high-quality GBS loci set of the Discovery Panel. Within those 483 loci, and taking all known GBS SNP positions into account, Primer3 implemented in SMAP snp-seq could successfully design 205 amplicons. Of these 205 loci, 136 loci were selected based on haplotype complexity (range of two to four haplotypes per locus), and of those a set of 96 amplicons were selected. After each HiPlex selection step it was checked if there was still enough discriminative power to robustly distinguish all the 139 unique genetic fingerprints as defined by the genome-wide GBS markers described above. This HiPlex assay was then performed on all 730 samples collected from the INERA Coffee Collection in Yangambi, on 235 samples collected from the local rainforest in the Yangambi region (Depecker et al., 2023), and also on 14 ‘Luki’ cultivars supplied by Meise Botanic Garden.
Next, the HiPlex assay was empirically validated by comparison to GBS data (Figure 1, Step III). Eighty-six high-quality HiPlex loci gave sufficient read depth across samples with minimum 250,000 reads per library and were retained for further analysis. Hundred-and-five samples with sufficiently deep sequencing per locus in both GBS and HiPlex data were used as Validation Panel. This panel (Figure 2) yielded 235 SNPs in the entire set, of which 213 polymorphic SNPs were found for GBS, 231 polymorphic SNPs for HiPlex, and 210 SNPs were found in both. The 235 SNPs were converted into 300 haplotypes within 86 loci.
On a per-sample basis, all genotype calls of GBS data were compared to those of HiPlex data across the 86 loci, yielding a per-sample genotype call reproducibility across the two genotyping techniques of, on average, 94.8% ± 3.18 (SD) across all samples (Figure 3A). On a per-sample basis, all haplotype calls of GBS data were compared to those of HiPlex data across the 86 loci, yielding a per-sample haplotype call reproducibility across the two genotyping techniques of 96.8% ± 3.52 (SD) across all samples (Figure 3A).
Pairwise JID was calculated on GBS data (Figure 3B), of which 18,225 SMAPs and 11,488 SNPs were converted into 10,257 haplotypes within 3,125 loci, and on HiPlex data (Figure 3C), of which 231 SNPs were converted into 300 haplotypes within 86 loci. The pairwise JID of the GBS data showed a positive correlation with the pairwise JID of the HiPlex data in corresponding sample pairs (R2 Adj. = 0.78, p < 0.05) showing that the genetic similarities estimated by the genome wide markers (GBS) are accurately reflected by the much smaller set of 86 HiPlex markers (Figure 3D). In addition, our set of 86 validated HiPlex loci could distinguish all unique genetic fingerprints (pairwise JID range between 0.32 and 0.88) by at least 20 discriminative loci, and would group clonal material by pairing with haplotype mismatches at JID >0.965 corresponding to at most one discriminative locus out of all detected HiPlex loci in the sample pair (allowing for the least possible technical error). Exactly the same (clonal) grouping was obtained using 86 HiPlex loci as with 3,125 GBS loci.
Next, HiPlex data was analyzed across 730 samples of the Screening Panel (Figure 1, Step IV), yielding 235 SNPs (screening SNPs) and 309 haplotypes (screening haplotypes) within 86 loci (Figures 2B, C). Pairwise JID was calculated on the 309 haplotypes and the distribution of all pairwise similarity values showed a group of replicates with JID values greater than 0.965. Using the minimal JID value of 0.965 as a threshold to collapse individuals into groups of individuals with shared unique genetic fingerprints (i.e., clones), the 139 unique genetic fingerprints, initially identified within the Discovery Panel, were reconstituted and complemented by 124 novel genetic fingerprints, resulting in a total of 263 unique genetic fingerprints (further referred to as G0001-G0263) (Supplementary Table S1).
Next, the genetic structure and composition of the Yangambi coffee collection was analyzed (Figure 1, Step V). HiPlex data of 14 ‘Luki’ cultivars, 235 wild coffee shrubs, and 263 unique genetic fingerprints within the Yangambi coffee collection and WGS data of two representatives of Congolese subgroup A (“Canephora Panel”; n = 514) was analyzed yielding a total of 318 SNPs and 396 haplotypes within 86 high-quality HiPlex loci (Figures 2B,C). Pairwise JID was calculated on the 396 haplotypes and the distribution of all pairwise similarity values showed a group of replicates with pairwise JID values greater than 0.977. Using the minimal JID values of 0.977 as a threshold, one clonal pair was found for the ‘Luki’ cultivars and three clonal pairs were found for the wild samples resulting in 510 unique genetic fingerprints identified. All 514 samples of the Canephora Panel were then used to further explore the genetic structure in the Yangambi coffee collection and determine the presence of these genetic resources.
The PCA performed on 318 SNPs of the Canephora Panel showed that all 235 samples collected from the local rainforest of the Yangambi region (Depecker et al., 2023) clustered together on the positive PC1-axis (Figure 4A). ‘Luki’ cultivars clustered together with the two Congolese subgroup A samples on the positive PC2-axis, and ‘Lula’ cultivars on the negative PC1 and PC2-axes. Unique genetic fingerprints, identified within the Yangambi coffee collection, were scattered between these three clusters. Second, Bayesian clustering, implemented in fastSTRUCTURE, was performed on 318 SNPs of the Canephora Panel, revealing three genetic clusters (Figure 4B). Similar to the PCA, the cluster analysis separated wild coffee shrubs collected from the local rainforest in the Yangambi region, hereafter referred as wild samples, from Congolese subgroup A and from ‘Lula’ cultivars. All three genetic clusters were present in the collection, including 181 samples with ‘Lula’ ancestry (Q > 80%), nine with wild ancestry, and four with Congolese subgroup A ancestry. Twenty-nine samples from the collection that were located between the ‘Lula’ cultivars and wild samples in the PCA showed an admixed ancestry with partial ‘Lula’ and wild ancestry. Thirty-two Yangambi coffee collection samples positioned close to the Congolese subgroup A in the PCA showed an admixed ancestry proportion of ‘Lula’ and Congolese subgroup A ancestry. All 14 samples collected from the INERA Coffee Collection in Luki were assigned to the Congolese subgroup A.
Figure 4. Population genetic structure and parent-progeny pairs within the Yangambi coffee collection. (A) Principal component analysis of the Canephora Panel using 318 SNPs indicating ‘Lula’, ‘Luki’, Congolese subgroup A, and wild genetic resources. G-codes indicate the unique genetic fingerprints which were more than five times identified as a parent. (B) fastSTRUCTURE bar plot representing three clusters (K = 3). Colors define subpopulations: green (wild), blue (Congolese subgroup A), and red (‘Lula’ cultivars). (C) Parentage analysis was performed on the 263 unique genetic fingerprints showing parent-progeny pairs with confidence levels higher than 95%. The arrow indicates which sample is the parent of which progeny.
A parentage analysis performed on the 263 unique genetic fingerprints (Figure 1, Step IV) with 227 SNPs and 299 haplotypes within the 86 high-quality HiPlex loci assigned 126 progenies and 108 parents in total, of which 39 samples were identified only as progenies, 21 only as parent, and 87 as both progenies and parent revealing a complex network of hybridization (Figure 4C). Only eight unique genetic fingerprints were often identified as parent (Figures 4A,C). Based on the fastSTRUCTURE results and parentage analysis, 75 progenies were assigned to ‘Lula’, four to Congolese subgroup A, nine to wild, 24 to ‘Lula’- subgroup A hybrid, and 14 to ‘Lula’-wild hybrid. For the parents, 58 unique genetic fingerprints were assigned to ‘Lula’, four to Congolese subgroup A, nine to wild, 21 to ‘Lula’- subgroup A hybrid, and 16 to ‘Lula’-wild hybrid.
Comparison of HiPlex SNPs and haplotypes of the Canephora Panel to the HiPlex SNPs and haplotypes of the Discovery, Validation, and Screening Panel, revealed 86 SNPs and 258 haplotypes that were unique to the Canephora Panel (Figures 2B,C), showing that the HiPlex assay is able to detect novel SNPs and haplotypes.
Two core collection strategies, CC-I and CC-X, were tested for the 263 unique genetic fingerprints within the Yangambi coffee collection (Figure 1, Step IV). For CC-I, the optimal core size (see Materials and Methods) comprised 100 unique genetic fingerprints as the genetic diversity was higher than other core sizes (He of 0.20 and SH of 5.75), all alleles were accounted for (CV of 1) and entry-to-accession distance was low (AN of 0.13) (Figure 5A). The accessions assigned to the CC-I core collection were evenly distributed across the ordination space of the Yangambi coffee collection (Figure 5D). For CC-X, the optimal core size comprised 10 unique genetic fingerprints as the genetic diversity (He of 0.23 and SH of 5.79) was higher than other core sizes tested for CC-X, almost all alleles were accounted for (CV of 0.93) and entry-to-nearest-entry distance was high (EN = 0.35) (Figure 5B). The accessions assigned to the CC-X core collection consisted of one Congolese subgroup A genotype, one wild genotype, five ‘Lula’ cultivars, one ‘Lula’- subgroup A hybrid, and two ‘Lula’-wild hybrids (Figure 5E).
Figure 5. Simulation of different core sizes for core collection strategies CC-I and CC-X. (A) Genetic diversity (Allele coverage and He) and distances measures (AN and EN) for core collection strategies CC-I. (B) Genetic diversity (Allele coverage and He) and distances measures (AN and EN) for core collection strategies CC-X. (C) Shannon’s index was calculated for CC-I and CC-X. Core collection size 263 is a representation of all unique genetic fingerprints currently identified in the Yangambi coffee collection. A principal component analysis was performed on the 263 unique genetic fingerprints within the Yangambi coffee collection indicating the assigned accessions of core collection CC-I (D) and CC-X (E).
To enable sustainable conservation and future use of the historically important C. canephora collection in Yangambi (DRC), the characterization of its genetic composition is critical. Here, we complemented genome-wide GBS marker sets with a novel versatile HiPlex assay, allowing genetic screening of the INERA Coffee Collection in Yangambi and creating opportunities for future genetic screening in a cost-effective manner. By using the HiPlex screening assay on 730 individuals, we were able to reveal the genetic structure, identify the origin of the material, and estimate the relative contribution of clonal propagation and crossing products in the collection. In addition, we proposed two core collections which could facilitate the ex-situ conservation of C. canephora genetic resources of the INERA Coffee Collection in Yangambi in the future.
A previous investigation of the INERA Coffee Collection management revealed that the field maps and plant labels of the collection did not correspond with the accessions present in the field (based on field observations 2020–2021, data not shown). Additionally, many plant labels were lost for a substantial portion of the collection. Most clonally propagated accessions do not differ much in terms of morphology from seed-propagated accessions, whereby it is hard to distinguish them by sight in the field. Therefore, genetic screening of the collection would help to distinguish with certainty the clonally propagated accessions from the seed-propagated accessions. In the present study, a total of 730 samples comprising 117 different plant labels (Supplementary Table S1) were genotyped at 86 high-quality HiPlex loci, and a total of 263 unique genetic fingerprints were identified. Our study revealed that the unique genetic fingerprints did not correspond with the plant labels at individual shrubs. For instance, the number of unique genetic fingerprints is more than two times the number of current labels present in the collection, which means that shrubs carrying the same plant label can be divided into distinct genotypes. Conversely, within groups of genetically identical plants (clones), some individuals carried different plant labels.
Mislabeling accessions is a common problem in ex-situ collections or genetic stocks in general (Bergelson et al., 2016). For example, Akpertey et al. (2021) showed that 18.6% of 400 C. canephora accessions from a coffee improvement program at the Cocoa Research Institute of Ghana (CRIG) were mislabeled. They suggested that the majority of the mislabeling originated from the nursery, where trees with the same genotype profile got a different label, or from the wrong replacement of dead plants in the field. In our case, we suspect that the labeling on field became inconsistent with the genetic identities as many accessions were poorly documented and missing their original plant label. Apart from accidental mislabeling, the discrepancy between plant labels and the genetic identity may be the result of labeling practice where siblings are labeled according to parental lines while genetic fingerprints discriminate genetically unique siblings resulting from seed propagation of the collection. To re-establish correct plant labeling, all 730 samples currently collected from the INERA Coffee Collection in Yangambi, were assigned a new label (G0001–G0263) corresponding to their unique genetic fingerprint obtained with the HiPlex assay. The cost-efficient HiPlex assay and JID reference matrix can now be routinely used to screen the rest of the collection and further install correct labeling.
Different types of molecular markers have previously been used to genotype C. canephora cultivars (sequencing based markers and their use in the coffee collections were reviewed by Vi et al., 2023), including DArTseq markers for cultivated C. canephora in Vietnam and Mexico (Garavito et al., 2016), sequence-related amplified polymorphism (SRAP) and start codon targeted (SCoT) markers for a C. canephora germplasm collection in India (Huded et al., 2020), SSR and AFLP markers for a C. canephora gene pool in India (Prakash et al., 2005), SSR and RFLP for the CNRA collection in Côte-d’Ivoire (Gomez et al., 2009) or only SSR markers for a C. canephora germplasm collection in Brazil (Souza et al., 2013) and for the INERA Coffee Collection in Yangambi that was the subject of this study (Vanden Abeele et al., 2021). Here, we opted to use highly multiplex (HiPlex) amplicon sequencing as it is a simple, accurate, and cost-effective amplicon-based targeted DNA sequencing technique (Kumar et al., 2010). We created a validated HiPlex assay that discriminates the unique genetic fingerprints within the Yangambi coffee collection. We choose for the HiPlex method because it can routinely identify genetic polymorphisms at a set of 86 predefined loci, spread across the genome. Using read-backed haplotyping with the SMAP haplotype-sites module, we can transform bi-allelic SNP data to multi-allelic haplotypes to increase genetic resolution per locus. In turn, the combination of all haplotypes defines a unique genetic fingerprint. In addition, since HiPlex is based on resequencing, it allows for discovering novel genetic polymorphisms at all three hierarchical levels (SNPs, haplotypes, and unique genetic fingerprints). For example, 235 SNPs (respectively, 309 haplotypes, and 263 unique genetic fingerprints) were identified in 730 samples representing the Yangambi coffee collection, while 86 novel SNPs (respectively, 128 novel haplotypes, and 247 novel unique genetic fingerprints) were identified in the Canephora Panel, which includes 251 novel samples external to the Yangambi coffee collection (Figure 2A). Identification of new SNPs made it possible to distinguish cultivated from wild samples, and representatives of the Congolese subgroup A from ‘Lula’ cultivars (Figure 4). Since the HiPlex assay can detect new SNPs within the targeted loci, the tool can also be used to genotype yet other C. canephora collections to obtain a global overview of the genetic diversity of C. canephora collections and compare their genotypes with the unique genetic fingerprints of the Yangambi coffee collection.
Furthermore, genetic fingerprints can have the same SNPs and haplotypes but still differ from each other based on their linear combination of SNPs and haplotypes. Therefore, we can still identify novel unique genetic fingerprints based on pairwise Jaccard Inversed Distance even if the number of SNPs and haplotypes barely differ between sample sets. For example, 96 unique genetic fingerprints were identified in 105 samples of the Validation Panel (respectively 231 SNPs and 300 haplotypes), while 167 novel unique genetic fingerprints were identified in the Screening Panel (respectively three novel SNPs and 11 novel haplotypes), that includes 625 novel samples external to the Validation Panel (Figure 2A). Therefore, if the remaining coffee shrubs from the INERA Coffee Collection in Yangambi are genotyped, we suspect that the number of SNPs and haplotypes will not increase substantially but the number of unique genetic fingerprints will increase.
Comparing GBS data to HiPlex data illustrates the trade-off between the cost and efficiency of genotyping (lower cost, higher efficiency with HiPlex) versus accuracy in the quantitative estimation of genetic similarities and population structure (better accuracy with GBS). An attempt to maintain a minimum number of loci that captures global genetic diversity while enforcing the discriminative power between closely related genotypes slightly inflated the genetic distances between highly similar unique genetic fingerprints. This means that the HiPlex assay is ideal for high-throughput screening of diverse genetic materials, to identify clonal replicates and discriminate unique genetic fingerprints, but at the cost of a slight bias in the quantitative estimation of genetic relationships. In addition, while the HiPlex assay is designed to maximize the diversity in the Yangambi coffee collection and is capable to discover new polymorphisms, it still can be optimized to capture diversity external to the pre-2020 INERA Coffee Collection in Yangambi. Using materials described by Gomez et al. (2009) and Merot-l'Anthoene et al. (2019) at the species native level, Kiwuka et al. (2021) for Uganda, and Vanden Abeele et al. (2021) for the DRC and our protocol to select discriminatory loci, novel primer sets can be developed to complement the current HiPlex assay with a more balanced distribution of genetic diversity in C. canephora material worldwide. This novel HiPlex primer set can then be used to screen and compare C. canephora field genebanks around the world to further catalog the genetic diversity captured in C. canephora field genebanks.
HiPlex and GBS markers both have their own benefits for collection maintenance and breeding. HiPlex may be used cost-efficiently to establish correct labeling, and to discriminate clonally propagated and seed-propagated material in the breeding germplasm. First, confirming clonal identities in field trials is important for replicated phenotyping. Second, tracing parent-progeny relationships is important to construct an accurate pedigree scheme of a breeding program, especially if the crop mating system is based on open-pollination. Third, a classical breeding scheme may select parental lines, make crossings, phenotype the progeny, and genotype the progeny for parental assignment or confirmation. Then, the breeding value is estimated per parent, and the best parents are combined in iterative rounds of crossing, phenotypic and genotypic evaluation and selection. Parallel maternal and paternal selection maximizes the genetic gain per generation, and parentage assignment can be routinely performed with the cost-effective HiPlex assay as demonstrated here. Furthermore, in a backcrossing scheme, the HiPlex assay, optionally extended with additional markers for improved genome coverage, may be used for marker-assisted background selection. For marker-assisted foreground selection, one first needs to identify molecular markers for beneficial alleles associated with important agronomic traits, such as yield, disease resistance or cupping quality. QTL or association mapping studies require the careful construction of appropriate germplasm panels. Initially, HiPlex assays can be used to construct such panels, either confirming full-sib identity in bi-parental crosses for QTL populations, or by maximizing genetic diversity for association mapping panels (similar to the core collections, Figure 1 Step IV, while identifying clonal replicates for replicated phenotyping). Then, GBS can be performed for a panel of several hundreds of selected individuals (Figure 1, Step I), to generate genome-wide molecular markers for quantitative genetics. Upon detection of significant marker-trait association, selected GBS markers may be transformed into HiPlex amplicons (Figure 1, Step II) and added to the general HiPlex screening assay for combined marker-assisted foreground and background selection in materials known to carry those alleles.
From 1930 to 1960, the INERA Coffee Collection in Yangambi consisted of Robusta derived material from the Java Research Station, INERA Coffee Research Station in Lula and other wild and cultivated material from the DRC (e.g., from the INERA Coffee Collection in Luki), and abroad. Due to the many difficulties the DRC has faced during the last decades, many of these Robusta cultivars and other wild and cultivated material have been lost. To restore the INERA Coffee Collection in Yangambi, since 2016 the collection is being continuously enriched with numerous new accessions including wild and cultivated material collected mainly by local botanists in several regions within the DRC. To investigate the genetic diversity of the INERA Coffee Collection in Yangambi, the unique genetic fingerprints within the Yangambi coffee collection were compared to ‘Lula’ cultivars, ‘Luki’ cultivars from the INERA Coffee Collection in Luki, reference sample for the Congolese subgroup A (Merot-l'Anthoene et al., 2019), and wild samples collected from the local rainforest of the Yangambi region (Depecker et al., 2023). The PCA and structure analyses showed that materials derived from three different genetic resources are present in the INERA Coffee Collection in Yangambi, which is in line with the observations of Vanden Abeele et al. (2021). Most of the unique genetic fingerprints are highly similar to the ‘Lula’ cultivars and some are highly similar to Congolese subgroup A or the local wild genotypes (Figure 4A). Vanden Abeele et al. (2021) could identify very few local wild genotypes within the INERA Coffee Collection in Yangambi and therefore proposed to enrich the collection with local wild genotypes to increase the genetic resources available for future breeding and conservation purposes. Our study surveyed a much broader sample set of the INERA Coffee Collection in Yangambi and consequently identified more local wild genotypes (nine in total) than Vanden Abeele et al. (2021). This is consistent with the expansion of the collection with local wild materials as part of the recent rehabilitation initiatives.
In the study of Merot-l'Anthoene et al. (2019), wild material that was collected from the same rainforest in the Yangambi region, before the study of Depecker et al. (2023), was assigned to the Congolese subgroup BE (a hybrid between subgroup B and E). Therefore, we can assume that the wild coffee shrubs collected by Depecker et al. (2023) also belong to the Congolese subgroup BE. This ensures that there are currently two different origin groups, namely Congolese subgroup A and BE, present in the collection, but our data shows that the ‘Lula’ cultivars are not assigned to either group. Based on information within the archives of INERA, ‘Lula’ cultivars are assumed to be derived from crossings between “Coffea robusta L.” and “Coffea sankuriensis” but originally collected in the Sankuru region (DRC). In order to reveal the origin of the ‘Lula’ cultivars the material have to be compared to each of the eight genetic groups as defined by Merot-l'Anthoene et al. (2019). In contrast to the ‘Lula’ cultivars, we were able to assign the ‘Luki’ cultivars to the Congolese subgroup A because they were genetically similar to the corresponding reference material (Merot-l'Anthoene et al., 2019).
The Structure analysis showed that around one-quarter of the unique genetic fingerprints had a hybrid identity (29 ‘Lula’-wild hybrid and 32 ‘Lula’-subgroup A hybrid) (Figure 4B). These hybrid identities could be a result of dedicated crosses or open pollination. In addition, 29 ‘Lula’-wild hybrid genotypes were found indicating that local wild genotypes are already being used for crossing activities. To investigate the contribution of breeding to the observed genetic structure in the Yangambi coffee collection, a parentage analysis was performed on the 263 genetic fingerprints, which revealed a complex network of hybridization (Figure 4C). Most of the parent-progeny pairs found were crosses between ‘Lula’ cultivars and some parent-progeny pairs found were crosses between only wild genotypes within the coffee collection. No specific parent-progeny pairs between ‘Lula’ and Congolese subgroup A, ‘Lula’ and wild or Congolese subgroup A and wild were discovered, but we uncovered multiple parent-progeny pairs between ‘Lula’ and ‘Lula’- subgroup A hybrids. Moreover, eight unique genetic fingerprints were frequently identified as a parent, of which seven were identified as ‘Lula’ cultivars and one as Congolese subgroup A. As Capot (1962) noted that in 1951 seven mother plants were selected for seed distribution, these unique genetic fingerprints may correspond to the ancient selected mother plants or direct descendants thereof.
There are multiple strategies to construct a core collection, but in recent years, the maximization strategy, which aims to maximize the genetic diversity, and the genetic distance method, which aims to maximize the genetic distance, are the two most commonly used strategies (Gu et al., 2023). In this study, we applied these two core collection strategies to the Yangambi coffee collection, representing the screened genetic diversity within the 263 unique genetic fingerprints (Figure 5). We used Core Hunter as this software optimize the genetic distance and allelic diversity simultaneously by weighting the Modified Roger’s distance and Shannon diversity index differently based on entry-accession (AN) and entry-to-nearest-entry (EN) distance (De Beukelaer et al., 2018). The main objective of the maximization strategy (CC-I) was to select the most diverse loci to maximize the genetic diversity and by this maintain a uniform representation of the original genetic diversity. We found that the optimal core size would be 100 entries, capturing all alleles in the Yangambi coffee collection. The main objective of the genetic distance method (CC-X) was to select the most diverse genotypes to maximize the genetic distance between the entries of the core collection and is rather orientated more toward breeding activities. Here, the optimal core collection size of 10 entries captures 93% of all alleles in the Yangambi coffee collection with a maximal entry-to-nearest-entry distance. For genetic resource conservation purposes, the CC-I strategy is the most suited but with an optimal core size of 100 entries, it would be a less cost-effective approach than the CC-X strategy with a core size of 10 individuals. Notably, these core collections were proposed based on genetic diversity without considering the phenotypic or agronomic traits present in the INERA Coffee Collection in Yangambi. If a core collection oriented toward breeding activities would be established for the INERA Coffee Collection, it would be necessary to expand the CC-X core collection with phenotypical or agronomical interesting accessions.
Following the best practices for validating the identity of genetic stocks (Bergelson et al., 2016), we created a HiPlex assay to routinely check plant labeling within the INERA Coffee Collection in Yangambi. The INERA Coffee Collection had not previously been described on such a large sampling scale and with high resolution genetic markers. Using the HiPlex screening assay, we investigated the genetic structure and composition, and discovered the presence of materials from two known origin groups, Congolese subgroup A and BE, while the most abundant material was most closely related to ‘Lula’ cultivars, yet are currently of unknown origin compared to the natural distribution range. In addition, we were able to identify parent-progeny relationships and found eight accessions that were preferentially used in crossings, which could possibly be the historically selected mother plants of the collection, or direct descendants thereof. Now that the genetic structure and identities are described, it is important to maintain the genetic diversity of the coffee collection and therefore we proposed two core collections that can contribute to the breeding activities and sustainable and effective management of the INERA Coffee Collection. In this study, we implemented a strategy to create a HiPlex screening assay that could be applied to other coffee collections (e.g., in Ivory Coast and Uganda) in order to expand our HiPlex marker set so that different C. canephora collections around the world could be compared and to build a comprehensive catalog of the genetic diversity of C. canephora in field genebanks such as the INERA Coffee Collection held at Yangambi. In addition, the strategy could also be applied to other crops or could be useful for breeders to record an accurate pedigree scheme, to select the best parental lines by paternity testing, or to implement marker-assisted foreground and background selection in backcrossing schemes.
The data that support the findings of this study are available on request (Y3VyYXRvckBwbGFudGVudHVpbm1laXNlLmJl). In accordance with the Democratic Republic of the Congo and international regulations, restrictions apply on the availability of these data, which were used under license for this study.
TR, OH, PS, RB, and LV designed the study. RB, J-LK, TE, and BK participated in fieldwork. RB and PS supplied the genomic DNA extracts of the 14 herbarium samples collected from the INERA Coffee Collection in Luki. JD collected the leaf material of 235 wild coffee shrubs from the Yangambi region. VP supplied the WGS data of the two Congolese subgroup A samples. LV executed the lab work. LV, RB, and TR analyzed the data. LV, RB, PS, OH, and TR wrote the manuscript. All authors contributed to finalizing the manuscript.
This study was funded by Research Foundation-Flanders, a research project granted to OH (FWO; G090719N) and the Belgian Science Policy Office (BELSPO) under the contract no. B2/191/P1/COFFEEBRIDGE (CoffeeBridge Project) of the Belgian Research Action through Interdisciplinary Networks (BRAIN-be 2.0). Since 2016 Meise Botanic Garden was helped INERA to rehabilitate and characterize their coffee collection with support of the European Union’s Development Fund (FED/2016/381–145), through the “Formation, Recherche et Environnement dans la Tshopo” (FORETS) project implemented by the Center for International Forestry Research (CIFOR), the Belgian Science Policy (CoffeeBridge Project), and the Flemish Climate Fund (Climcoff Project).
We would like to thank the Institut National pour l'Étude et la Recherche Agronomiques (INERA) for giving access to their collection; Rachel Ndezu and CIFOR (through the FORETS project, funded by the EU 11th Development Fund) for the logistic and administrative support during fieldwork in the Democratic Republic of the Congo. We would also like to express our sincere gratitude to the Ministère de L'Environnement et Développement Durable (MEDD) for their help with obtaining permits (N°008/ANCCB-RDC/SG-EDD/BTB/11/2020, N°004/ANCCB-RDC/SG-EDD/BTB/2021, N°014/ANCCB-RDC/SG-EDD/BTB/11/2021, and N°025/ANCCB-RDC/SG-EDD/BTB/11/2022).
The authors declare that the research was conducted in the absence of any commercial or financial relationships that could be construed as a potential conflict of interest.
All claims expressed in this article are solely those of the authors and do not necessarily represent those of their affiliated organizations, or those of the publisher, the editors and the reviewers. Any product that may be evaluated in this article, or claim that may be made by its manufacturer, is not guaranteed or endorsed by the publisher.
The Supplementary material for this article can be found online at: https://www.frontiersin.org/articles/10.3389/fsufs.2023.1239442/full#supplementary-material
Akpertey, A., Padi, F. K., Meinhardt, L., and Zhang, D. (2021). Effectiveness of single nucleotide polymorphism markers in genotyping germplasm collections of Coffea canephora using KASP assay. Front. Plant Sci. 11:612593. doi: 10.3389/fpls.2020.612593
Andrews, S. (2010). FastQC: a quality control tool for high throughput sequence data. Available at: http://www.bioinformatics.babraham.ac.uk/projects/fastqc.
Bergelson, J., Buckler, E. S., Ecker, J. R., Nordborg, M., and Weigel, D. (2016). A proposal regarding best practices for validating the identity of genetic stocks and the effects of genetic variants. Plant Cell 28, 606–609. doi: 10.1105/tpc.15.00502
Brown, A. D. H. (1989). “The case for core collection” in The use of plant genetic resources. eds. A. D. H. Brown, O. H. Frankel, D. R. Marshall, and J. T. Williams (Chichester: John Wiley & Sons, Baffins Lane), 136–156.
Capot, J. (1962). Le caféier Robusta dans la cuvette centrale Congolaise. Bull. d'Inf. INEAC 11, 33–40.
Chevalier, A. (1929). Les caféiers du globe, fasc. 1: Généralités sur les caféiers. Encycl. Biol. 5, 1–196.
Coste, R., Vayssière, P., and Barat, H. (1955). Caféiers et les cafés dans le monde. Tome 1er. Larose.
Cubry, P., De Bellis, F., Pot, D., Musoli, P., and Leroy, T. (2013). Global analysis of Coffea canephora Pierre ex Froehner (Rubiaceae) from the Guineo-Congolese region reveals impacts from climatic refuges and migration effects. Genet. Resour. Crop. Evol. 60, 483–501. doi: 10.1007/s10722-012-9851-5
Danecek, P., Auton, A., Abecasis, G., Albers, C. A., Banks, E., DePristo, M. A., et al. (2011). The variant call format and VCFtools. Bioinformatics 27, 2156–2158. doi: 10.1093/bioinformatics/btr330
Davis, A. P., and Rakotonasolo, F. (2021). Six new species of coffee (Coffea) from northern Madagascar. Kew Bull. 76, 497–511. doi: 10.1007/s12225-021-09952-5
De Beukelaer, H., Davenport, G. F., and Fack, V. (2018). Core hunter 3: flexible core subset selection. BMC Bioinformatics 19, 203–212. doi: 10.1186/s12859-018-2209-z
Denoeud, F., Carretero-Paulet, L., Dereeper, A., Droc, G., Guyot, R., Pietrella, M., et al. (2014). The coffee genome provides insight into the convergent evolution of caffeine biosynthesis. Science 345, 1181–1184. doi: 10.1126/science.1255274
Depecker, J., Verleysen, L., Asimonyio, J. A., Hatangi, Y., Kambale, J. L., Mwanga Mwanga, I., et al. (2023). Genetic diversity and structure in wild Robusta coffee (Coffea canephora a. Froehner) populations in Yangambi (DR Congo) and their relation to forest disturbance. Heredity 130, 145–153. doi: 10.1038/s41437-022-00588-0
Doyle, J., and Doyle, J. (1987). A rapid DNA isolation procedure for small quantities of fresh leaf tissue. Phytochem. Bull 19, 11–15.
Durand, T., De Wildeman, É., Micheli, M., Briquet, J., Hallier, H., and Pax, F. (1898). Materiaux pour la Flore du Congo. Bull. Soc. R. Bot. Belg. 37, 44–128.
Dussert, S., Lashermes, P., Anthony, F., Montagnon, C., Trouslot, P., Combes, M. C., et al. (1999). Le caféier, Coffea canephora. Divers. Génét. Plantes Trop. Cultiv. 9:194. doi: 10.1186/1471-2148-9-167
Elshire, R. J., Glaubitz, J. C., Sun, Q., Poland, J. A., Kawamoto, K., Buckler, E. S., et al. (2011). A robust, simple genotyping-by-sequencing (GBS) approach for high diversity species. PLoS One 6:e19379. doi: 10.1371/journal.pone.0019379
Evanno, G., Regnaut, S., and Goudet, J. (2005). Detecting the number of clusters of individuals using the software STRUCTURE: a simulation study. Mol. Ecol. 14, 2611–2620. doi: 10.1111/j.1365-294X.2005.02553.x
Ferrão, R., Volpi, P., Ferrão, M., da Fonseca, A., and Verdin Filho, A. C. (2019). Origin, geographical dispersion, taxonomy and genetic diversity of Coffea canephora. In Conilon coffee. 3rd ed., ed. L. H. MunerDe, Vitória: Incaper, 85–109.
Garavito, A., Montagnon, C., Guyot, R., and Bertrand, B. (2016). Identification by the DArTseq method of the genetic origin of the Coffea canephora cultivated in Vietnam and Mexico. BMC Plant Biol. 16, 242–212. doi: 10.1186/s12870-016-0933-y
Gomez, C., Dussert, S., Hamon, P., Hamon, S., de Kochko, A., and Poncet, V. (2009). Current genetic differentiation of Coffea canephora Pierre ex a. Froehn in the Guineo-Congolian African zone: cumulative impact of ancient climatic changes and recent human activities. BMC Evol. Biol. 9, 1–19. doi: 10.1186/1471-2148-9-167
Gu, R., Fan, S., Wei, S., Li, J., Zheng, S., and Liu, G. (2023). Developments on Core collections of plant genetic resources: do we know enough? Forests 14:926. doi: 10.3390/f14050926
Huded, A. K. C., Jingade, P., Bychappa, M., and Mishra, M. K. (2020). Genetic diversity and population structure analysis of coffee (Coffea canephora) germplasm collections in Indian Gene Bank employing SRAP and SCoT markers. Int. J. Fruit Sci. 20, S757–S784. doi: 10.1080/15538362.2020.1768618
Jaccard, P. (1912). The distribution of the flora in the alpine zone. 1. New Phytol. 11, 37–50. doi: 10.1111/j.1469-8137.1912.tb05611.x
Jombart, T. (2008). Adegenet: a R package for the multivariate analysis of genetic markers. Bioinformatics 24, 1403–1405. doi: 10.1093/bioinformatics/btn129
Kalinowski, S. T., Taper, M. L., and Marshall, T. C. (2007). Revising how the computer program CERVUS accommodates genotyping error increases success in paternity assignment. Mol. Ecol. 16, 1099–1106. doi: 10.1111/j.1365-294X.2007.03089.x
Kassambara, A., and Kassambara, M. A. (2020). Package ‘ggpubr’. R package version 0.1.6. Availabe at: https://CRAN.R-project.org/package=ggpubr
Kiwuka, C., Goudsmit, E., Tournebize, R., De Aquino, S. O., Douma, J. C., Bellanger, N. S., et al. (2021). Genetic diversity of native and cultivated Ugandan Robusta coffee (Coffea canephora Pierre ex A. Froehner): Climate influences, breeding potential and diversity conservation. PLoS One 16: e0245965. doi: 10.1371/journal.pone.0245965
Kumar, G. R., Sakthivel, K., Sundaram, R. M., Neeraja, C. N., Balachandran, S. M., Rani, N. S., et al. (2010). Allele mining in crops: prospects and potentials. Biotechnol. Adv. 28, 451–461. doi: 10.1016/j.biotechadv.2010.02.007
Lashermes, P. (2018). Achieving sustainable cultivation of coffee: breeding and quality traits. Cambridge: Burleigh Dodds Science Publishing Limited.
Leroy, T., De Bellis, F., Legnate, H., Musoli, P., Kalonji, A., Loor Solorzano, R. G., et al. (2014). Developing core collections to optimize the management and the exploitation of diversity of the coffee Coffea canephora. Genetica 142, 185–199. doi: 10.1007/s10709-014-9766-5
Leroy, T., Montagnon, C., Charrier, A., and Eskes, A. B. (1993). Reciprocal recurrent selection applied to Coffea canephora Pierre: II. Estimation of genetic parameters. Euphytica 74, 121–128. doi: 10.1007/BF00033776
Li, H. (2013). Aligning sequence reads, clone sequences and assembly contigs with BWA-MEM. arXiv Available at: https://arxiv.org/pdf/1303.3997.pdf (Accessed January 9, 2023).
Li, H., Handsaker, B., Wysoker, A., Fennell, T., Ruan, J., Homer, N., et al. (2009). The sequence alignment/map format and SAMtools. Bioinformatics 25, 2078–2079. doi: 10.1093/bioinformatics/btp352
Li, Y. L., and Liu, J. X. (2018). StructureSelector: a web-based software to select and visualize the optimal number of clusters using multiple methods. Mol. Ecol. Resour. 18, 176–177. doi: 10.1111/1755-0998.12719
Martin, M. (2011). Cutadapt removes adapter sequences from high-throughput sequencing reads. EMBnet. J. 17, 10–12. doi: 10.14806/ej.17.1.200
McKenna, A., Hanna, M., Banks, E., Sivachenko, A., Cibulskis, K., Kernytsky, A., et al. (2010). The genome analysis toolkit: a MapReduce framework for analyzing next-generation DNA sequencing data. Genome Res. 20, 1297–1303. doi: 10.1101/gr.107524.110
Merot-l'Anthoene, V., Tournebize, R., Darracq, O., Rattina, V., Lepelley, M., Bellanger, L., et al. (2019). Development and evaluation of a genome-wide coffee 8.5 K SNP array and its application for high-density genetic mapping and for investigating the origin of Coffea arabica L. Plant Biotechnol. J. 17, 1418–1430. doi: 10.1111/pbi.13066
Montagnon, C., Leroy, T., and Eskes, A. (1998a). Amélioration variétale de Coffea canephora. 1: critères et méthodes de sélection. Plant. Rech. Dév. 5, 18–33.
Montagnon, C., Leroy, T., and Eskes, A. (1998b). Amélioration variétale de Coffea canephora. 2: les programmes de sélection et leurs résultats. Plant. Rech. Dév. 5, 89–98.
Montagnon, C., Leroy, T., and Yapo, A. (1992). Diversité génotypique et phénotypique de quelques groupes de caféiers (Coffea canephora Pierre) en collection. Conséquences sur leur utilisation en sélection. Café Cacao Thé 36, 187–198.
Oliveira, L. N. L. D., Rocha, R. B., Ferreira, F. M., Spinelli, V. M., Ramalho, A. R., and Teixeira, A. L. (2018). Selection of Coffea canephora parents from the botanical varieties Conilon and Robusta for the production of intervarietal hybrids. Ciência Rural 48:4. doi: 10.1590/0103-8478cr20170444
Pendergast, M. (2009). Coffee second only to oil? Is coffee really the second largest commodity? Mark Pendergrast investigates and finds some startling results. Tea & Coffee Trade Journal 4, 38–41.
Poland, J. A., and Rife, T. W. (2012). Genotyping-by-sequencing for plant breeding and genetics. Plant Genom. 5, 92–102. doi: 10.3835/plantgenome2012.05.0005
Prakash, N. S., Combes, M. C., Dussert, S., Naveen, S., and Lashermes, P. (2005). Analysis of genetic diversity in Indian robusta coffee genepool (Coffea canephora) in comparison with a representative core collection using SSRs and AFLPs. Genet. Resour. Crop. Evol. 52, 333–343. doi: 10.1007/s10722-003-2125-5
Raj, A., Stephens, M., and Pritchard, J. K. (2014). fastSTRUCTURE: variational inference of population structure in large SNP data sets. Genetics 197, 573–589. doi: 10.1534/genetics.114.164350
Schaumont, D., Veeckman, E., Van der Jeugt, F., Haegeman, A., van Glabeke, S., Bawin, Y., et al. (2022). Stack mapping anchor points (SMAP): a versatile suite of tools for read-backed haplotyping. bioRxiv. doi: 10.1101/2022.03.10.483555
Souza, F. D. F., Caixeta, E. T., Ferrão, L. F. V., Pena, G. F., Sakiyama, N. S., Zambolim, E. M., et al. (2013). Molecular diversity in Coffea canephora germplasm conserved and cultivated in Brazil. Crop Breed. Appl. Biotechnol. 13, 221–227. doi: 10.1590/S1984-70332013000400001
Stoffelen, P., Anthony, F., Janssens, S., and Noirot, M. (2021). A new coffee species from south-West Cameroon, the principal hotspot of diversity for Coffea L.(Coffeeae, Ixoroideae, Rubiaceae) in Africa. Adansonia 43, 277–285. doi: 10.5252/adansonia2021v43a26
Stoffelen, P., Ithe, M. M., Bienfait, K., Salvator, N., Chantal, S., Céphas, M., et al. (2019). An answer to the coffee challenge: from herbarium to coffee genetic resource collections in the Democratic Republic of Congo. BGjournal 16, 20–24.
Tournebize, R., Borner, L., Manel, S., Meynard, C. N., Vigouroux, Y., Crouzillat, D., et al. (2022). Ecological and genomic vulnerability to climate change across native populations of Robusta coffee (Coffea canephora). Glob. Chang. Biol. 28, 4124–4142. doi: 10.1111/gcb.16191
Untergasser, A., Cutcutache, I., Koressaar, T., Ye, J., Faircloth, B. C., Remm, M., et al. (2012). Primer3—new capabilities and interfaces. Nucleic Acids Res. 40:e115. doi: 10.1093/nar/gks596
Vanden Abeele, S., Janssens, S. B., Asimonyio Anio, J., Bawin, Y., Depecker, J., Kambale, B., et al. (2021). Genetic diversity of wild and cultivated Coffea canephora in northeastern DR Congo and the implications for conservation. Am. J. Bot. 108, 2425–2434. doi: 10.1002/ajb2.1769
Vi, T., Vigouroux, Y., Cubry, P., Marraccini, P., Phan, H. V., Khong, G. N., et al. (2023). Genome-wide admixture mapping identifies wild ancestry-of-origin segments in cultivated Robusta coffee. Genome Biology and Evolution 15:evad065. doi: 10.1093/gbe/evad065
Keywords: Coffea canephora, HiPlex amplicon sequencing, genetic structure, core collection, ex-situ conservation, crop wild relatives, Democratic Republic of Congo
Citation: Verleysen L, Bollen R, Kambale J-L, Ebele T, Katshela BN, Depecker J, Poncet V, Assumani D-M, Vandelook F, Stoffelen P, Honnay O and Ruttink T (2023) Characterization of the genetic composition and establishment of a core collection for the INERA Robusta coffee (Coffea canephora) field genebank from the Democratic Republic of Congo. Front. Sustain. Food Syst. 7:1239442. doi: 10.3389/fsufs.2023.1239442
Received: 13 June 2023; Accepted: 02 August 2023;
Published: 15 August 2023.
Edited by:
Christophe Montagnon, Centre de Coopération Internationale en Recherche Agronomique pour le Développement, FranceReviewed by:
Bing Cheng, Beijing Academy of Agricultural and Forestry Sciences, ChinaCopyright © 2023 Verleysen, Bollen, Kambale, Ebele, Katshela, Depecker, Poncet, Assumani, Vandelook, Stoffelen, Honnay and Ruttink. This is an open-access article distributed under the terms of the Creative Commons Attribution License (CC BY). The use, distribution or reproduction in other forums is permitted, provided the original author(s) and the copyright owner(s) are credited and that the original publication in this journal is cited, in accordance with accepted academic practice. No use, distribution or reproduction is permitted which does not comply with these terms.
*Correspondence: Tom Ruttink, dG9tLnJ1dHRpbmtAaWx2by52bGFhbmRlcmVuLmJl
Disclaimer: All claims expressed in this article are solely those of the authors and do not necessarily represent those of their affiliated organizations, or those of the publisher, the editors and the reviewers. Any product that may be evaluated in this article or claim that may be made by its manufacturer is not guaranteed or endorsed by the publisher.
Research integrity at Frontiers
Learn more about the work of our research integrity team to safeguard the quality of each article we publish.