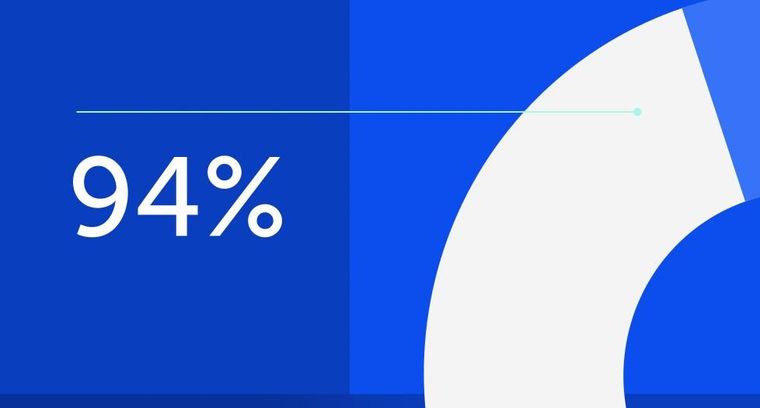
94% of researchers rate our articles as excellent or good
Learn more about the work of our research integrity team to safeguard the quality of each article we publish.
Find out more
ORIGINAL RESEARCH article
Front. Sustain. Food Syst., 19 July 2023
Sec. Agroecology and Ecosystem Services
Volume 7 - 2023 | https://doi.org/10.3389/fsufs.2023.1207972
Mediterranean olive growing characterizes, identifies, and can sustain the socioeconomic viability of rural areas, not only through the production of primary products but also through the management of renewable natural resources, and the conservation of landscape and biodiversity. However, high levels of mechanization, monocultures, intensive farming techniques, and the use of synthetic pesticides and fertilizers are threatening the environment and affecting rural communities in turn. In the last years, the agroecological discourse emerged as a transdisciplinary science merging agronomy, ecology, and socioeconomic sciences with the purpose of responding to socioeconomic and environmental concerns, responding to the globalized industrial food processing and intensive agriculture. In this context, the research project Sustainolive, guided by an international consortium of stakeholders from academia and the productive sector, has the aim of promoting sustainability in the olive oil sector through the implementation and promotion of innovative and technological solutions based on agroecological concepts. Through an innovative, transdisciplinary, and multi-actor approach, Sustainolive combines different types of knowledge (e.g., scientific, empirical, and traditional), disciplines (ranging from engineering to the humanities), and methodological approaches (e.g., Life Cycle Sustainability Assessment, Social Agrarian Metabolism and multicriterial analysis tools) to provide practical solutions that address the complexity of the olive sector. The results from the application of this methodology are expected to highlight which agroecological practices are more environmentally, economically, and socially sustainable and uncover how Mediterranean societies use agrarian biophysical resources.
Agriculture is among those anthropic activities that have direct relations with natural resources, i.e., extracting and consuming inputs from the environment to provide food, feed, and fiber. Innovation and technological advances in farming systems have allowed to improve land use and increase agricultural production, but with consequences. The intensive use of external energetic and chemical inputs for agriculture raised high concerns for the environment and also rural societies, leading to the rise of the agroecological debate.
According to Gliessman (2018), the word “agroecology” appeared in the scientific literature at the beginning of the 20s. It has rapidly spread as a scientific discipline merging agronomy and ecology; then, it assumed the purpose of responding to socioeconomic concerns besides environmental ones, as a response to the globalized industrial food processing and intensive agriculture (Porter and Francis, 2016).
Today, agroecology is related to a set of practices and management operations based on ecological principles and on nature-based processes which involve the whole agri-food systems and food supply chains, including socioeconomic, cultural, and political strategies. Some of these principles and strategies include, among others:
- taking advantage of the positive interactions, complementarity, and synergies among elements (plants, animals, soil, air, water, and humans) of the agroecosystems;
- reducing the dependency on external inputs (pesticides and synthetic products) and non-renewable sources;
- recycling and closing cycles of nutrients and biomass;
- maintaining and promoting biodiversity;
- supporting adaptation and resilience to climate changes;
- promoting fair remuneration for agricultural production and agroecosystem services;
- fostering stewardship and participation in agrifood supply chain decision-making (Deguine et al., 2023).
According to the FAOSTAT database (2021), the most important agricultural production in the Mediterranean basin, in terms of area and yields, is represented by olive growing. This crop accounts for 38%, 33%, 21%, and 40% of the utilized area of Greece, Portugal, Spain, and Tunisia, respectively. In absolute terms, Spain, Tunisia, Italy, and Morocco are the four countries in the world with a higher area devoted. Furthermore, in 2021, Spain, with 8.256.550 tons, and Italy, with 2.270.630 tons, were the main olive fruit producers worldwide (FAOSTAT, 2021).
In these countries, the cultivation of olive has historical roots connected with ancient traditions and involves cultural and identity aspects of the local communities: it guided the socioeconomic and cultural life of many villages and is the dominant landscape of many areas of the Mediterranean basin.
Therefore, the application of the agroecological perspective to olive growing is of utmost importance to understand how to promote the transition of agriculture toward sustainability.
Mediterranean olive groves provide approximately 80% of the world's olive oil production, of which 70% is produced in Europe and 10% in Tunisia and Morocco, with a total surface of 7.7 million hectares and a production of more than 3.3 million tons of olive oil (2020–2021 olive campaign; International Olive Council - IOC, 2021) (Figure 1). In Mediterranean regions, olive growing is the principal driver for the maintenance of rural economies: the olive grove sector generates employment (more than one million annual workers), safeguards marginal territories from hydrogeological instability, shapes agricultural landscapes, fosters the rural identity, keeps cultural traditions, and brakes rural depopulation, among others (Bernardi et al., 2021).
Figure 1. World production and consumption of olive oil (2020–2021 campaign). Source: Our elaboration on FAOSTAT (2021).
Nevertheless, the top-of-the-range olive oil (the extra virgin oil) is worldwide recognized for its nutritional properties and appreciated as a healthy food, being a principal component of the Mediterranean diet model, today an intangible cultural heritage of the UNESCO.
Olive-growing farming systems are very variegated across the globe and are rapidly evolving according to the challenging needs to safeguard the environment while ensuring productivity and profitability (Iofrida et al., 2018a). According to Russo et al. (2016), in the European Union, 48% of olive orchards are farmed with traditional or extensive systems, 47% are farmed with semi-intensive systems, and 5% with super-intensive systems (6% in Spain, the leading country in this sense). In Northern African countries, the traditional system is the most diffused, and it is characterized by low tree densities, low mechanization levels, and reduced use of pesticides.
Olive growing has direct positive and/or negative consequences on natural resources, depending on the pedoclimatic conditions, the farming system, the cultivar, and the presence of endemic pathologies, among others.
As agricultural systems can provide a balance of agroecosystem services while ensuring economic viability and social liveability, systemic evaluations of the diverse services and trade-offs provided by different combinations of management practices are required (Wittwer et al., 2021). Indeed, there is a research gap concerning quantitative and comprehensive methodological approaches capable to link social, economic, and environmental domains to assess how agricultural systems are effective in meeting sustainability goals (Haberl et al., 2019).
Therefore, specific, effective, and reliable tools are needed to evaluate the performance of farming systems to reduce environmental and socioeconomic impacts.
Balancing profitability while reducing environmental impacts is of utmost importance, and it requires suitable tools for farmers to organize and manage their businesses to reach these purposes (De Luca et al., 2018; Iofrida et al., 2020). Preserving the environment, and therefore the agroecosystems, meeting consumers' needs, and paying attention to workers' wellbeing and other social issues, by adapting or modifying managerial and organizational features is the challenge for current agri-food productions.
Despite a wide assortment of definitions and indicators, guidelines, scientific literature, and technical reports (Mottet et al., 2020), there is a lack of approaches to combine socioeconomic and environmental sustainability (D'Annolfo et al., 2017; Landert et al., 2020) and few studies analyzed the environmental loads of agroecological systems from a life cycle and energetic perspective (Stillitano et al., 2021, 2022).
In this context, the international project called “Sustainolive” (novel approaches to promote the SUSTAINability of OLIVE cultivation in the Mediterranean), which consists of a consortium of 22 partners from Spain, Portugal, Italy, Greece, Tunisia, and Morocco, and funded by PRIMA (Partnership for Research and Innovation in the Mediterranean Area) program, and co-funded by Horizon 2020, has tried to integrate life cycle with biophysical and energy perspective of different models of olive grove cultivation.
The main objective of the project is to provide scientific knowledge about how to foster the sustainability of the olive oil sector, selecting and analyzing a set of innovative sustainable management solutions, based on agroecological concepts and the exchange of knowledge and participation of multiple stakeholders and final users. The project is organized in work packages (WP) and tasks; the current study presents the research activities planned within the fifth WP, which is devoted to the socioeconomic and environmental evaluation of the potential impacts in terms of environmental burdens, economic benefits, and social repercussions and, finally, the overall sustainability of specific “olive farms case studies” in the partners' areas. In particular, this study presents a new methodological proposal for sustainability assessment based on the Social Agrarian Metabolism (SAM) and Life Cycle approaches to evaluate the environmental and socioeconomic performances of different olive farms (in Spain, Italy, Greece, Portugal, Tunisia, and Morocco)—by comparing “agroecological” (i.e., Sustainable Technological Solutions—STSs) and “ordinary” (i.e., non-STSs) management principles. The rationale behind the joint application of the two methodological approaches mentioned above is based on the systemic interdisciplinary research frameworks that can help to integrate scientific knowledge from different disciplines, overcoming the great divides among life sciences, social sciences, and humanities (Haberl et al., 2019).
Sustainolive project aims to find solutions and strategies to reconcile profitable and sustainable practices in olive groves that protect the environment and avoid the overexploitation of natural resources integrating ecological, territorial, and socioeconomic knowledge, designing and evaluating sustainable strategies and methods in the cultivation of the olive grove based on agroecological concepts. To achieve these objectives, the overall vision of Sustainolive (https://sustainolive.eu) is grounded on developing innovation strategies linked to management practices based on agroecological concepts and methods to devise more efficient and site-specific solutions for Mediterranean olive contexts with the active involvement of different actors to implement the set of sustainable technological solutions (STSs) and measures.
The identification and implementation of a site-specific set of Sustainable Technical solutions (STSs) is the cornerstone of the different tasks of the project. Based on the scientific knowledge about agroecology, the STSs to be tested on experimental olive farms have been co-defined with the farmers and are based on the use of natural resources and on closing biological cycles at the farm or local level, entailing the reduction of external inputs and the improvement in the quality and efficiency of use of internal inputs. These latter are produced and reproduced on the farm or obtained through socially regulated exchange from farmers and other actors in the food chain to foster farmers' autonomy which translates into a pillar of economic and ecological resilience (van der Ploeg et al., 2019). Increasingly, research indicates (e.g., Jeswani et al., 2018) that the level of internal regulation of ecological functions in agroecosystems is largely dependent on their level of biodiversity, as the element that delivers a variety of agroecosystem services that span beyond the production of food, and that include the recycling and retention of nutrients, regulation of micro-climates and local hydrological processes, and control or mitigation of pest and diseases. This leads to improving benefits such as crop protection and soil fertility even when external inputs (water, nutrients…) are limited, as is frequently the case in the Mediterranean context. Sustainolive is aimed at testing if and how this improvement may be achieved through a combination of management and strategic actions in olive farming systems aimed to increase their biodiversity.
Social Metabolism (SM) refers to the set of theories and methodological tools that allow analyzing a society's biophysical behavior (Matthews, 2000; Haberl, 2001; Weisz et al., 2006). It provides valuable information to assess a society's environmental sustainability and has even turned into a new perspective on human beings' relationships with their physical environment through flows of energy, materials, and information (González de Molina and Toledo, 2014). In this sense, human societies carry out two basic material tasks: on the one hand, they produce goods and services and distribute them among society's individuals; and on the other, they reproduce the conditions that make production possible, thus gaining stability over time. A substantial share of social relations is therefore oriented toward the organization and maintenance of exchanges of energy, materials, and information; this is metabolic activity. It can therefore be applied to agriculture: Social Agrarian Metabolism (SAM) is the exchange of energy, materials, and information that agroecosystems perform with their socio-ecological environment according to González de Molina et al. (2020), Guzmán et al. (2022) (Figure 2). The purpose of metabolic activity is appropriating biomass to satisfy the human species' endosomatic consumption directly or indirectly through livestock while providing basic agroecosystem services. SAM refers to the appropriation of biomass by members of society by managing the agroecosystems (Guzmán and González de Molina, 2017), configuring specific landscapes and specific agroecosystem arrangements. The landscape is the visible mark left on the territory, although hidden marks may materialize in a different, sometimes distant territory, from which natural resources (land embodied and virtual land) are imported (Infante-Amate et al., 2018).
The metabolic exchange is composed of inflows and outflows of agroecosystems, as described in the Economy-wide Material Flow Accounting (EW-MEFA) methodology (European Commission Eurostat, 2018). However, SAM not only quantifies these flows but also carefully measures whether these flows maintain the dissipative structures or fund elements they are endowed with (i.e., capitals and stocks). The distinction between flows and funds was borrowed from Georgescu-Roegen (1971) and Giampietro et al. (2014) who affirmed that the economy's ultimate goal is not the production and consumption of goods and services, but the reproduction and improvement of the processes necessary for their production and consumption. This implies that, from a biophysical point of view, we need to shift our attention from the production and consumption of goods and services to sustainability, and whether both production and consumption can be maintained indefinitely.
Accordingly, it is essential to distinguish between flows and funds. Flows include energy and materials that are consumed or dissipated during the metabolic process, such as raw materials or fossil fuels. The rhythm of these flows is controlled by external factors—relating to the accessibility of the environment's resources in which the metabolic activity unfolds—and by internal factors—related to the processing capacity of energy and materials, relying in turn on the technology used and the knowledge to manage it. For their part, fund elements are dissipative structures that use inputs to transform them into goods, services, and waste, i.e., into outputs, within a given time scale; they remain constant during the dissipative process (Scheidel and Sorman, 2012).
Agroecosystems are dissipative structures that can be decomposed, in turn, into other structures, be social or ecological. For Sustainolive, four fund elements were taken into account: land, livestock, agrarian population, and technical means of production (technical capital). The four funds are closely connected and represent the fullest manifestation of the socio-ecological relationships at the heart of each agroecosystem and the center of the metabolic exchange. The articulation between the four fund elements is fundamental to explaining metabolic dynamics.
Each fund element has a different nature, either biophysical or social, and therefore each fund element works with different quality flows and different metrics. As pointed out by Giampietro et al. (2014), the flows' characteristics are closely related to the fund they come from. For example, a territory is colonized or land is appropriated by society to generate useful biomass flows; it is usually measured in hectares and subdivided into different uses that produce vegetal biomass, expressed in tons of vegetal biomass per hectare (t ha−1)—or its equivalent in energy, MJ ha−1–or net primary productivity (NPP). The agrarian population is the fund element that generates the human workflows. They are usually measured in hours or days of work (hours or days year−1). Finally, the “means of production” fund brings together the supply of production tools that generate mechanical workflows or vegetal health services and other services. It is usually measured, for example, in terms of installed capacity, expressed in kW of power or cv, and its flows in kW hour−1 or MJ ha−1, etc.
In SAM not only biophysical flows are exchanged but also flows of information. These flows can order and organize components of physical, biological, and social systems. For this project, information flows are defined as follows: flows originating in the agrarian population fund element, in the form of work and incorporated management decisions; and monetary flows stemming from the agroecosystem's social environment and ending up in this population fund, in the form of money obtained in exchange for production. The prices received by farmers, the prices paid for inputs, and agricultural income will constitute the main information flows in this research.
The four fund elements that operate in SAM mutually affect each other. This interrelation is expressed in each metabolic arrangement in a particular way, giving rise to a sort of unstable equilibrium that makes the agrarian metabolism (AM) function as a whole in a specific way. The ability to process energy and materials that each fund element has determines not only the magnitude of the flows it generates but also generates restrictions for others. Land availability and its capacity to produce biomass have a direct impact on the magnitude of the flows originating in human work, livestock, or means of production. Low availability of human work can, for example, limit the capacity of the land to produce useful biomass, favoring, for example, livestock use of the land and vice versa; very strong traction power can lead to more intensive agricultural use of the land that would not correspond to the size of the population and its capacity to work; while an excessive volume of the technical means of production or livestock may require importing energy from the outside, in the form of biomass or fossil fuels. Nevertheless, these relations between fund elements are more evident at a larger scale.
The fund elements' mutual dependence not only explains the structure, functioning, and dynamics of AM but also constitutes the key to its degree of sustainability, i.e., whether each fund element is capable of providing the services required by SAM itself to function (Giampietro et al., 2014; Giampietro, 2019) and to do so in a balanced manner over time. The imbalance between each fund's capacity and the generated flows can make it necessary to seek a new equilibrium, thus causing changes that compromise the medium- and long-term viability of the established metabolic arrangement. This consistency between flows and funds and among funds themselves has been made operational through the so-called “sudoku effect” (Giampietro et al., 2014; Giampietro and Bukkens, 2015). A variable part of the energy and material flows must necessarily be invested in the maintenance and reproduction of the funds and these in turn must preserve the necessary relation of congruity in such a way that the flows originated in one fund can help the others to function and vice versa. The imbalances or lack of correspondence between the funds and their flows explain the metabolic dynamics and, therefore, tendencies toward unsustainability and metabolic change.
Within the theoretical framework of Life Cycle Thinking (LCT) (Heiskanen, 2002), many methodologies have been developed to assess the sustainability impacts of the production and consumption of goods and services, throughout their life cycle, i.e., from the “cradle” (e.g., the planning phase) to the “grave” (e.g., the disposal phase). Life Cycle Costing (LCC) was the first methodology to be developed and applied since the 50s for industrial investment appraisals. Life Cycle Assessment (LCA) is the one that most attracted the attention of researchers and practitioners, and is now a renowned and standardized methodology (ISO, 2021); it assesses the environmental impacts of products or processes referring to specific midpoint or endpoint impact categories, concerning human health, ecosystem quality, and resource use (Horne et al., 2009). Social Life Cycle Assessment (SLCA) is the most recent methodology and is aimed at assessing the social impacts deriving from the life cycle of products and services. The process of standardization is long and difficult due to the multiparadigmatic characteristics of social sciences (Iofrida et al., 2018b). Almost recently, Social Organizational Life Cycle Assessment (SOLCA) has been proposed to assess the behavior—in terms of social performance—of companies.
The ISO (2021) standards describe four different and iterative steps to conduct an LCA study, which are a reference also for the other life cycle methodologies, as an umbrella procedure to homogenize the evaluations:
1. Goal and Scope, which consist of: the definition of the purpose of the evaluation, the extent of the system boundaries, the identification of foreground and background systems, the choice of selection and allocation criteria, and the selection of the functional unit (FU), which is the unit of measurement to which all impacts relate and is necessary to compare life cycle phases or different scenarios.
2. Life Cycle Inventory, i.e., the collection of quantitative and qualitative data (primary and/or secondary data), inbound and outbound flows of energy, materials, and emissions. Data quality check is of utmost importance to ensure the reliability of results. Secondary environmental data are available for many productive sectors: the most used for agricultural systems are Ecoinvent, Agri-footprint, and World Food LCA Database.
3. Impact assessment of product or service life cycle, using recognized environmental characterization models that allow estimating midpoint and endpoint impact such as ReCiPe2016 (Goedkoop et al., 2013; Huijbregts et al., 2016), LC-Impact (Verones et al., 2016), and Impact World+.
4. Interpretation of results. This is a critical step aimed at identifying, checking, and comparing information from the results obtained to retrieve new knowledge; inventory and impacts assessment results are summarized, and insights are inferred, to provide conclusions and recommendations for readers exhaustively and comprehensively (Hernandez et al., 2019). Therefore, interpreting life cycle results means identifying significant issues, and comparing life cycle phases and/or productive scenarios to conclude while identifying limitations and further research needs.
LCC's first purpose is to account for all financial costs across the life cycle phases; the main difference with LCA is that there are no specific standardized norms for all sectors, neither databases nor characterization models (Spada et al., 2022). Three possible approaches exist, namely financial or conventional LCC (C-LCC), environmental LCC (E-LCC), and societal LCC (S-LCC). C-LCC is a quasi-dynamic model that evaluates internal costs like the total cost of ownership (TCO); E-LCC allows estimating the external costs in terms of the monetary value of indirect damages that are not marketable; S-LCC includes the monetary value of externalities corresponding to environmental and social impacts and can provide interesting insights for public authorities and policy decision-makers. Societal LCC also aims to assess the socioeconomic efficiency of given scenarios by estimating welfare loss or gain (Stillitano et al., 2021).
Social LCA (SLCA) is aimed at evaluating social impacts deriving from products or services' life cycles, affecting different typologies of stakeholders, such as workers, local communities, value chain actors, consumers, societies, and children (UNEP, 2020). SLCA is still not consensually defined, and the most diverse methodologies have been proposed in the literature. A specific standard, the ISO norm 14075 “Principles and framework for social life cycle assessment”, is under development (in the preparatory phase). After approximatively 10 years, UNEP (2020) updated the Guidelines for SLCA and the Methodological Sheets for subcategories in SLCA (UNEP, 2021), providing some guidance for social evaluations of life cycles, such as a list of possible impact categories, subcategories, and indicators per each stakeholders' groups. According to them, there are two main families of approaches, corresponding to different impact assessment procedures, and each of them responds to different practical research aims: the Reference Scale Approach (Type I) and the Impact Pathway Approach (Type II). SLCA Type I assesses the social performance of companies of organizations involved in the product system, by comparing their behavior to a reference scenario (for example, specific legal regulations), with a characterization process mainly based on interpretation. SLCA Type II evaluates social impacts through causal or correlation/regression-based relationships between the product or service life cycle. The characterization process is based on an analytical and quantifiable identification of the consequences of the life cycle. According to UNEP (2020), the SLCA Type II is epistemologically and methodologically more in line with environmental LCA, where inventory inputs are quantitatively linked with environmental impacts. This duality of the procedure is due to the multiparadigmatic characteristics of social sciences: actually, in SLCA, both interpretivist (like Type I) and post-positivist epistemological positions (like Type II) have been applied in the scientific literature (Iofrida et al., 2018b).
Finally, Life Cycle Sustainability Assessment emerged in the academic panorama to meet the requirement of an overall assessment methodology, able to cover all aspects of sustainability. Many procedures have been proposed for a comprehensive assessment methodology that can cover all dimensions of sustainability, called Life Cycle Sustainability Assessment (LCSA); among them, the most implemented procedure is represented by the equation LCSA= LCA+LCC+SLCA. The integration or combination of sustainability results can be made in many ways. According to De Luca et al. (2017), a Multicriteria Decision Analysis (MCDA) can help LC practitioners to overcome trade-offs among the different dimensions of sustainability, but also to deal with subjective assumptions in an objective way, and take into consideration stakeholders' values and desiderata.
Farms were selected as study cases of the project according to their suitability to respond to the Sustainolive experimental design, and therefore the selection included olive farms as business as usual, and others that were similar (comparable in terms of surfaces, plants age and density, yields, and pedoclimatic conditions) but using agroecological practices (STSs). Therefore, within the Sustainolive project, pairs of STS and non-STS olive farms from Portugal, Spain, Italy, Greece, Morocco, and Tunisia were selected. One member of each pair consisted of a typical conventional olive grove (business as usual), whereas the other member consisted of a comparable olive farm but with a given combination of agroecological management practices implemented during at least 8 years.
By applying SAM and LCSA methodologies, the sustainability performance of contrasted models of olive cultivation, representative of business as usual (nSTS) and agroecological models (STS) can be delineated. This experimental design follows the main criteria for sustainability evaluations:
i. The choice of an epistemological perspective. In the case of this systemic interdisciplinary analysis framework, the epistemological stance is framed within the paradigms of post-positivism, i.e., in the search for causal relationships between olive growing systems and possible impacts on society, the economy, and the environment through quantitative methodologies, i.e., SAM and LCSA. The epistemological alignment among methodologies is by no means a given in this field; it is of utmost importance because it ensures reliable results in the evaluation of all sustainability dimensions.
ii. The delimitation and sizing of the survey, context, and spatio-temporal scope. Data gathered were referred to the last 5 years of production (from 2017 to 2021 included) to retrieve average data and overcome eventual fluctuations due to external constraints (weather, productive cycles, and market fluctuations).
iii. The selection of the units of analysis, the identification of the population, and the choice of the sample to be analyzed.
iv. The definition of the analytical method to be applied, i.e., the choice of the data to be collected and the most suitable tools for collecting, processing, and summarizing the information. The survey instrument chosen is the semi-structured questionnaire, to collect quantitative and qualitative data, organized in thematic sections according to a logical sequence, to guide the respondents in their compilation. The farms were interviewed de visu, by appropriately trained interviewers via web meetings.
v. Testing of the questionnaire with a set of volunteers and prefiguring the type of results obtainable.
Therefore, the questionnaire has been organized into four sections: general data on the farm and olive grove (Section A), agroeconomic data (Section B), agroecological data (Section C), and livestock associated with olive growing (Section D). More details about the structure of the questionnaire and the information investigated are provided in Table 1.
For the survey on olive farms, 59 key informers from olive farms (including those involved in field trials) involved in the Sustainolive project were selected, i.e., six Portuguese farms, 16 Spanish farms, seven Moroccan farms, 11 Tunisian farms, nine Italian farms, and 10 Greek farms; direct interviews were carried out during 2022, by interviewers appropriately instructed and trained to collect data in a univocal manner in all the countries involved.
The STSs currently under study in the experimental farms that have been selected according to the scientific literature and the involvement of stakeholders are described in Table 2. They have been selected in a previous Work Package of the project, i.e., WP2 “Synopsis of olive grove farming, including conceptual approaches, methods and STSs identification.” Therefore, a specific group of experts and scientists provided a synopsis and characterization of the diversity of conditions under which olive groves are cultivated, in terms of diversity of agronomic management practices, the pedo-climatic conditions, ecological landscapes, and reviewed—from scientific literature—key agroecological and sustainable concepts and methods to identify relevant STSs to be applied.
To know which are the best agroecological practices to improve the sustainability of Mediterranean olive groves, the STSs have been implemented in the study cases at least during the eight previous years, and the following methodological proposal has been set up to assess their performances. SAM and LCSA methodologies have been chosen to be combined (Table 3) because of their ability to grasp the bridge between natural and social sciences providing appropriate knowledge about the sustainability of agroecosystems and information for monitoring and policymaking.
The methodology and conceptual framework of Social Agrarian Metabolism (SAM) have been applied to the 59 Mediterranean olive farms according to the guidelines proposed in Energy in Agroecosystems (Guzmán and González de Molina, 2017). SAM is suitable for any scale—from farm scale to global scale. However, the converters and reference values gathered in the aforementioned work need to be updated according to new research and adapted to each case study. Indeed, field data and historical data must always be the preferred source of information, recurring to secondary sources only when it is unavoidable.
During 2020 and 2021, samples of biomass, organic amendments, and soil were taken in the network of 59 Sustainolive olive groves and analyzed in the laboratory, in addition to data collected from face-to-face interviews (Figure 3 and Table 2). Samples were analyzed in terms of density, weight, organic matter content, and other physical-chemical characteristics.
A long-term experiment was conducted in four Spanish olive groves plots to determine the amount of litter naturally falling throughout the year and during harvest. In each plot, three healthy trees were selected and open boxes were set under their canopies to collect the litter. The litter was sampled every 3 months during the year. In addition, biomass residues falling during the harvest were also measured and sampled. The litter and residue production per hectare was calculated for all Sustainolive olive groves by multiplying the average production per square meter of the canopy by the total canopy area. In the olive groves where cover crops were left to grow, five samples of aerial biomass were taken in five random squares of 0.25 m2. The net productivity calculated was assigned to the area covered by the cover crop to know the aerial biomass production of cover crops per hectare. Other material flows as root biomass were modeled or estimated from converters proposed by Guzmán and González de Molina (2017). Material flows involving livestock—feed consumption and excreta—were determined according to the animal dietary balance.
Data from the interview were used to adjust the measured and modeled flows and to entirely estimate some of the material flows such as the olive yield, the organic amendments, the agrochemicals, and the machinery use. Energy flows were determined based on the material flows and the energy converters proposed by Guzmán and González de Molina (2017).
To perform the calculations in a systematic and, to some extent, automatic manner for a potential decision support systems design, the Microsoft Excel® program was chosen. The model created is divided into four excel sheets: two spreadsheets have been dedicated to the calculations of material and energy flows, while the other two, linked to the previous ones, contained the converters and factors needed for the calculations.
The Material Flow spreadsheet (Table 4) obtained was divided into sections following the classification of Agrarian Metabolism. It contains inputs and outputs, as well as internal cycles of material and energy; it also reports different sections for specific material flows (i.e., different phases of the same flow, which means different physical expressions of the same energy). All data are expressed in kilograms of dry matter per hectare and year.
Actual NPP = SVB + RuB + UhB + AB. Recycled biomass (RcB) is the sum of RuB and UhB, and represents all the biomass that recirculates within the system no matter if it is intentionally or not intentionally incorporated. External input (EI) is the sum of organic and inorganic inputs; human labor, and water are not considered material flows. Total Inputs Consumed (TIC) is the sum of EI and RcB.
The main metabolic indicator from the EW-MEFA methodology has been calculated. Material converters spreadsheet contains factors to obtain or estimate all flows on dry matter. Some of these converters come from Guzmán and González de Molina (2017), and the rest from our experimental data or reviewed field studies.
Dry matter converters were applied to transform fresh matter into dry matter. Biomass partitioning factors included the residue index (fresh weight of the residues produced from the fresh weight of the corresponding products) and the root-to-shoot ratio (relation between the aerial part, excluding fruits, and the belowground portion) for olive trees, weeds, and cereals. In the case of olive trees, an equation and some reference values are provided to estimate the production of wood and light pruning from the olive production. Similarly, conversion factors to estimate the straw production from grain production in cereals are shown.
In addition to biomass partitioning factors, material converters also include the different percentages of nutrient content (N, P2O5, K2O, SO3) of most common fertilizers (ammonia, ammonium nitrate, ammonium sulfate, calcium-ammonium nitrate, calcium nitrate, urea, potassium nitrate, etc.).
Finally, manure nutrient contents are provided along with calculations to assess the livestock balance (daily energy ingesta/kg of body weight, daily kg dry matter excreta/kg body weight).
Once we have calculated the materials flows, and by applying the energy converters (see following section), the energy flows of the olive groves are calculated. All the data are given in joules (or a multiple) per hectare and year. This spreadsheet covers the same sections as the Material flow spreadsheet.
The NPP components, the Socialized Animal Biomass, and the biomass recirculation through livestock excreta express the gross energy of their equivalent material flows. Furthermore, the energy flow of external organic inputs reflects the gross energy of their equivalent material flow plus the energy required in processing and transporting them (embodied energy). It is important to clarify that the energy flow of external organic inputs (seed, feed, organic residues, etc.) does not account for the energy required in their production process since that energy is imputed to the agroecosystem in which they were produced. On the other hand, the embodied energy of industrial inputs accounted for the extraction of raw materials (including the energy carriers consumed), the manufacturing process, the distribution, and, in the case of fossil fuels and electricity, the gross energy of the element. Finally, the energy flow of human labor reflects the dietary energy consumption per hour.
After calculating the magnitude and direction of energy flows, the energy efficiency as a proxy of agroecosystem sustainability was assessed by using the EROIs proposed by Tello et al. (2016) and Guzmán and González de Molina (2017).
The first group of indicators is referred to as Economic EROIs. These EROIs have traditionally been calculated on the sole basis of supply services, given that these have a direct impact on society or the farmer, ignoring the fact that regulation and maintenance services are essential for supply services to survive over time (Tello et al., 2016). For this reason, they relate the internal and/or external inputs to the final output crossing the agroecosystem boundaries.
Final EROI indicates the return on the energy investment made by society.
External final EROI assesses to what extent the agroecosystem analyzed becomes a net provider or rather a net consumer of energy.
Internal final EROI assesses the efficiency with which the biomass that is intentionally returned to the agroecosystem is transformed into a product that is useful to society.
On the other hand, Agroecological EROIs have been developed to inform about the sustainability of the agroecosystem (Guzmán and González de Molina, 2015).
NPPact EROI estimates the real productive capacity of the agroecosystem, whatever the origin of the energy it receives, i.e., solar for biomass or fossil for an important portion of the external inputs. This is an indicator that provides integrative information on the state of the agroecosystem, beyond the particular situation of each fund element. A decreasing trend in NPPact EROI values of an agroecosystem over time indicates degradation of the productive capacity (Guzmán et al., 2018).
Agroecological final EROI gives a better idea of the energy investment required to obtain the production transferred to society.
Biodiversity EROI provides useful information on the extent to which energy invested in the agroecosystem contributes to sustaining the food chains of heterotrophic species (Guzmán et al., 2018).
Woodening EROI estimates whether the energy added to the system is contributing to the storing of energy in the system as AB. A decreasing trend in this EROI indicates a deterioration of the woodland fund element (Guzmán et al., 2018).
Further information about the calculation in energy terms of NPPact and its components, and of the external inputs, can be found in detail in Guzmán and González de Molina (2017) and Guzmán et al. (2017).
Additionally, some other indicators were calculated:
Renewable energy efficiency assesses the energy produced in the agroecosystem for each energy unit of renewable energy inverted, whereas non-renewable energy efficiency determines to what extent the production of the plot is dependent on non-renewable sources.
To estimate all the energy flows, several energy converters were used. The energy converters spreadsheet allowed to:
a) convert biomass (dry matter) into gross energy.
b) calculate embodied energy of the external inputs, either organic or industrial.
c) calculate the proportion of renewable and non-renewable energy in the embodied energy of each item.
The first group of converters has been taken from the work of Guzmán and González de Molina (2017). Gross energy values of biomass fractions were obtained from the analysis in a calorimetric bomb of field biomass samples. When this analysis was not possible, it was obtained from its composition and standardized energy values of fats, proteins, and carbohydrates. Since biomass produced in olive groves (olives, pruning, weeds, etc.) is highly stable in terms of composition, this is a fair approach to calculating the gross energy.
To detail the origin and suitability of the energy converters of the industrial inputs, they have been classified as follows:
- Agrochemicals. Guzmán and González de Molina (2017) provide a set of energy converters to calculate the embodied energy of different fertilizers and pesticides. In the case of fertilizers, embodied energy can be calculated from the content of the different nutrients (N, P2O5, and K2O) according to their chemical formulation. In the case of pesticides, it can be calculated from the content of active matter. When data about specific fertilizers or active matters of pesticides were not available in this study, the embodied energy of these products was obtained from LCA studies or some of the SimaPro databases.
- Fossil fuels. The energy converters for fossil fuels were entirely obtained from the study of Guzmán and González de Molina (2017).
- Machinery and implements. Leaving aside the fuel consumed during field operations, the embodied energy of machinery and implements can be broken down into (i) energy attributable to the extraction and processing of raw materials (including fossil fuels), (ii) energy attributable to equipment manufacture, (iii) energy attributable to the transport of this equipment from the manufacture location to its destination, and (iv) energy attributable to the maintenance. The study of Guzmán and González de Molina (2017) provides historical data on the energy requirements of each process for three categories: tractors, tillage implements, and other implements. For all of them, the energy converter we used was the total embodied energy in MJ per kg of machinery. Based on the approach described in Material Flow Spreadsheet, we applied the embodied energy per kg of machinery to the kg of machinery attributable to one hectare.
- Irrigation. Energy requirements for irrigation can be very variable according to the energy requirements of water extraction and the embodied energy of irrigation infrastructure. Average values of energy requirements of irrigation infrastructure from Guzmán and González de Molina (2017) were used in all the plots, and, when direct energy consumption of water pumping was not available, the energy requirement for water extraction was calculated from the well depth and the energy converter from Guzmán and González de Molina (2017).
- Organic external inputs. The embodied energy of organic external inputs was calculated as the sum of their gross energy and the energy required in processing (composting) and transporting them. Guzmán and González de Molina (2017) provide reference values for both the gross energy and the processing and transporting energy for different products.
The life cycle approach proposed in Sustainolive consists of the following framework:
which entails the integration of LCA, economic and environmental LCC, and SLCA using a multicriteria decision analysis tool, in this case, the Analytic Hierarchy Process (AHP). Data have been organized in a single inventory to serve, then, the impact assessment methodologies for each sustainability dimension: then, impacts can be quantified using cause–effect relationships, to epistemologically align the evaluations and allow the integration of results through AHP. Reference flow refers to the measurement of inputs required by a process within a system to fulfill the function of a defined system; for this study, the reference flow is 1 hectare of olive groves. The functional unit refers to the “quantified performance of a product for use as a reference unit” (ISO, 2021), it is necessary to compare farming scenarios, and in this study, impacts will be referred to 1 kg of olives. The allocation procedures consist of partitioning the input and/or output flows of a process to the product system under study; for LCA and LCC, it is the economic one, which is advised as a baseline method for most allocation situations, while for SLCA, the allocation of impacts is hourly applied.
As the goal of the project is to assess the sustainability of different agroecological methods applied in the experimental plots and/or farms, the system boundary chosen is cradle to gate; therefore, all life cycle phases from planting to the harvesting of olives are included. The time boundary is dependent on the average life of the plants. Primary data, used mainly to acquire information for foreground activities, have been collected using the questionnaire described in paragraph 3.1. The inventory consisted of collecting, per each farming operation, the typology of operation, the energy consumption, the mass consumed, the machinery used, the costs afforded, the working hours, the ergonomic postures (standing, sedentary, and loading), and the working exposure (to pesticides, temperature, lights, dust, noise, and vibrations). Secondary data, for background activities in the upstream and core process, have been retrieved from ad hoc available databases such as Eco-invent V. 3.5, Agri-footprint, and World Food LCA databases, IPCC (2019) for the estimate of N2O, and Brentrup et al. (2000) for the estimate of nitrate leaching. Impact categories (Figure 4, Table 5) and assessment model are retrieved from ReCiPe 2016 and Environmental Prices method.
Figure 4. Midpoint impact categories from ReCiPe 2016 (from Goedkoop et al., 2013; Caballero et al., 2020).
The Environmental LCC methodology (ELCC), in alignment with LCA, evaluates the internal and external costs of Sustainolive scenarios. The common database was based on the same functional unit and system boundaries, and followed the same methodological steps. Internal costs (Table 6) included all those costs incurred throughout the life cycle of the farm analyzed (STS vs. non-STS). The agricultural life cycle analyzed was different according to the country or the cultivar analyzed (up to 80 years). Anyway, each scenario was divided into six main stages, with different durations (in years): planting phase, unproductive phase, increasing production phase, constant production phase, decreasing production phase, and end-of-life phase (disposal or recycling).
External costs were accounted for through the monetization of externalities, i.e., by assigning an economic value to the environmental impacts of the life cycle: therefore, LCA results were economically assessed through the Environmental Prices approach (De Bruyn et al., 2018), which expresses the willingness to pay (WTP) for reducing that environmental pollution, calculated in € kg-1 of pollutant. The same software used for LCA, SimaPro, is used to account for these external costs: it assigns a monetary weight to each environmental indicator using an external cost factor from the Environmental Prices Handbook that provides average values for emissions in the EU28, divided into categories (De Bruyn et al., 2018).
Total revenues were calculated for the whole life cycles of STS and non-STS farms, taking into account current market prices, and including EU CAP subsidies when applicable; they allowed analyzing the efficiency of investments through the following indicators and their formulas calculated for the Functional Unit of 1 kg of olives (Falcone et al., 2022):
The discount rate considered represented an opportunity cost, i.e., the alternative investments with similar risks and at the same times were used; furthermore, during the life cycle, constant prices were taken into account while excluding adjustments for inflation (De Luca et al., 2018; Falcone et al., 2022).
Concerning the evaluation of social impacts deriving from the product life cycle, the Psychosocial Risk Factors impact pathway PRF-IP (Iofrida et al., 2019), a post-positivism-oriented SLCA methodology (type II according to UNEP, 2020), is applied, because it allows making an objective assessment of potential impacts directly linked to inventory inputs, in alignment with ISO norms 14040-44 (2021), and therefore, with the other life cycle evaluations (Iofrida et al., 2020). Functional units, system boundaries, and cutoff criteria are the same as the environmental and economic evaluations; therefore, all social impacts will be scaled to 1 kg of olives.
This methodology allows quantifying the risk of psychosocial impacts on olive growing workers, in terms of duration of exposure (hours) to certain conditions that can lead to health issues (Table 7).
PRFs are defined as the aspects and characteristics of work planning and management that can potentially lead to physical or psychological damage (Cox et al., 2000). The psychosocial risks will be measured using odds ratios (ORs), a statistical measure of the intensity of association between two variables, e.g., the ratio between the odds of exposure for people with a disease and the odds of exposure for healthy people (Szumilas, 2010).
where:
a = number of cases with exposure; d = number of controls without exposure;
b = number of controls with exposure; c = number of cases without exposure.
ORs are widely used in epidemiological studies; it is a retrospective study of a phenomenon but can give information about an outcome to occur; it is expressed with a non-dimensional value, assuming values between 0 and + ∞. A value of 1 indicates that there is no association between disease and exposure, while values > 1 indicate a positive association (the risk factor can provoke the disease/disorder); higher values show a stronger association between exposure and disease (Bottarelli and Ostanello, 2011). For example, an odds ratio of 2 means that exposure to a certain condition increases the risk of disease/disorder by 100%.
According to Bottarelli and Ostanello (2011), the intensity of association is weak when 1 < OR < 1.3; moderate when 1.3 < OR < 1.7, strong when 1.7 < OR < 8; and very strong when OR > 8.
Specific odds ratios have been retrieved from published scientific studies that examined the relationships between specific living and working conditions and diseases (or disorders): indeed, there is a wide literature available about the health consequences of farming occupation, especially concerning pesticide exposure. When studies for specific agricultural tasks were not found, similar situations from other work typologies were taken into account; it is the example of the exposure to firewood, which can occur during the practice of burning pruning residues, and can be considered similar to the domestic exposure for cooking, or the physical workload, which is common to many other working conditions.
A PRF matrix was constructed putting in relation to every condition of exposure that occurred in the scenarios to physical or psychosocial disease. The assessment of social impacts is conducted through the quantification of hours when stakeholders are exposed to particular working conditions and therefore to one or more psychosocial risks. When a task exposes to more than one risk (for example, body vibrations and insecticides exposure), the duration of the task is accounted for more times, i.e., one time per each possible risk. Some examples of olive-growing tasks are given in Figure 5.
The Analytic Hierarchy Process by Saaty (1980) has been chosen as an MCDA tool to combine the environmental, economic, and social results into an overall sustainability assessment and, therefore, allows comparing the possible agroecological scenarios characterized by specific STSs. This multicriterial tool has been already successfully used in a previous study with similar purposes (De Luca et al., 2018). The reasons behind this methodological choice lay in the following characteristics of AHP, as described by Shao et al. (2020):
- it is easily understandable and suitable for complex issues;
- it decomposes a problem into smaller sub-levels hierarchically organized;
- it allows the comparison of the criteria and their importance;
- it is applicable for quantitative and qualitative evaluations;
- the consistency of the decision is checked, reducing the bias.
The propaedeutic phase of the AHP consists of the creation of a hierarchical structure through the decomposition of the decisional problem into levels and sublevels (Figure 6), which means structuring a hierarchy among objectives of the assessment, criteria, and alternative scenarios. Then, AHP consists of two main steps: pairwise comparison and weighted linear combination.
The second phase consists of pairwise comparison at each level: stakeholders are interviewed to compare each criterion of impact evaluation. Comparative judgments consist of pairwise comparing the n criteria and thus a matrix of order n is implemented based on these comparisons. All entries of the matrix are positive and:
Saaty (1980) proposed a specific scale for paired comparison, enabling to comparison of the set of alternatives and a subset of rational numbers that retrieve the relative importance of each alternative over another, i.e., the importance of the ith alternative over the jth alternative. A scale (from 1 to 9) is used to help interviewees express a comparison between alternatives.
Each element of the AHP priority matrix is normalized and a consistency ratio (CR) of the pairwise comparison matrix (Saaty, 1990) is calculated to discard answers with CR higher than 10%. Normalized data will be multiplied for the weights (environment, economy, and society), allowing us to obtain a ranking among scenarios in terms of overall sustainability performance.
The AHP is easily understandable and suitable for complex issues: a complex decision process is decomposed into smaller sub-problems at hierarchical levels (Shao et al., 2020). The importance of criteria is obtained through paired comparison, allowing an objective and reliable representation of the decision problem; it allows transforming qualitative judgments into quantitative measures; and bias in the decision-making progression are limited by checking the consistency (Shao et al., 2020; Kumar and Pant, 2023).
A recent literature review by Kumar and Pant (2023) highlighted the importance and utility of multicriterial techniques like AHP in sustainability assessments in agriculture, almost imperative for attaining sustainable development goals (SDGs).
This study presented a methodological proposal for sustainability assessment within the international ongoing project Sustainolive, which is based on the joint implementation of Social Agrarian Metabolism (SAM) and Life Cycle approaches to evaluate environmental and socioeconomic performances of different Mediterranean olive farms by comparing “agroecological” and “ordinary” management principles. The assessment of environmental, economic, and social sustainability is as complex and intricate as the ecosystem processes that are involved. This is particularly true in olive-growing agroecosystems, which are strongly linked to economic viability and quality of life in Mediterranean rural areas.
The Social Agrarian Metabolism (SAM) and the Life Cycle approach (LCSA, in particular) were successfully demonstrated that can be combined to provide a holistic and multiperspective assessment of the sustainability of different models of olive farming, contributing to improving the effectiveness of farm processes, also by the co-construction of novel knowledge that can be useful to key actors in the olive-oil sector.
The first approach (SAM) recalls the concept of “biophysical accounting” to measure the mutual dependences incurred during the interaction between nature and society by measuring biophysical fund-flow integrated and reproductive (González de Molina et al., 2020) to identify the optimal configuration of land uses, livestock densities, and other elements characterizing agrarian systems. The implementation of SAM provides information on biophysical functioning and produces synthetic indicators of sustainability that can be easily monitored. By assessing the flows (e.g., production of goods and services) and funds (e.g., reproduction and improvement of the series of processes required for the production and consumption of goods and services), it can be evaluated whether STSs olive groves are more (or less) sustainable when comparing with that of comparable non-STSs olive groves. SAM also will analyze the role played by energy flows within olive groves, considering not only the harvested olives but also the unharvested, which is essential to fuel the heterotrophic components providing ecosystem services to olive farmers.
The first step in this methodological proposal is to gather primary, on-farm, data using a questionnaire proposed to local actors, which should be combined with secondary data—from literature—not only for background activities in upstream and core processes but also to fill some gaps. The second and third steps are to process the information and integrate it through a multi-criteria technique to identify the main hot spots within an overall sustainability framework and a comprehensive overview of STSs potential implications.
The two methodologies, SAM and LCSA, have in common the ability to uncover society–nature interactions at different spatio-temporal scales (Haberl et al., 2019). SAM covers biophysical flows exchanged between a society or community and its natural environment as well as the flows of mass and energy within and between social systems; LCSA is an integrated assessment model that allows measuring the performance of a system in environmental, economic, and social terms.
The main objectives of these methodological proposals are to identify and quantify the potential impacts in terms of environmental burdens, economic benefits, and social repercussions, and, therefore, the overall sustainability of specific “olive farms case studies” in the countries' partners (Italy, Greece, Portugal, Spain, Morocco, and Tunisia), with the involvement and participation of key actors. Climate changes are strongly and growingly threatening agricultural production and farming processes. Farmers should take advantage of the opportunities provided by agroecological practices to make their productions more resilient.
Interdisciplinary research is increasingly required in sustainability sciences, but it is a challenging task at the same time. Often, the tendency is to accommodate multiple—rather than singular—scientific paradigms, but this methodological proposal succeeded to frame the evaluations in a quantitative perspective, analyzing cause–effect relationships. The integration of the social agrarian metabolism approach with the life cycle approach is expected to strengthen the ability of the former to comply with thermodynamic principles and systematically take into account feedback between different resources, as highlighted in the review by Haberl et al. (2019).
The results from the application of this methodology are expected to highlight which agroecological practices are more environmentally, economically, and socially sustainable and uncover how Mediterranean societies use agrarian biophysical resources.
Therefore, academics and researchers are required to contribute to improving and supporting the reengineering of management practices toward sustainability, providing information and knowledge that can be useful to key actors in the sector, including stakeholders, shareholders, consumers, and a vast array of local actors.
The raw data supporting the conclusions of this article will be made available by the authors, without undue reservation.
AIDL, MG, NI, and RG: conceptualization. NI, ES, PD, and GF: data curation and formal analysis. AIDL, MG, and RG: funding acquisition, project administration, and supervision. GF, NI, ES, and PD: investigation. AIDL, NI, MG, PD, GF, and RG: methodology and writing the original draft. AIDL, MG, GG, and RG: writing review and editing. All authors contributed to the article and approved the submitted version.
This research is part of the Sustainolive research project (https://sustainolive.eu), grant agreement no. 1811, funded by the PRIMA (Partnership for Research and Innovation in the Mediterranean Area) program, supported by the European Union, and co-funded by Horizon 2020.
The authors declare that the research was conducted in the absence of any commercial or financial relationships that could be construed as a potential conflict of interest.
All claims expressed in this article are solely those of the authors and do not necessarily represent those of their affiliated organizations, or those of the publisher, the editors and the reviewers. Any product that may be evaluated in this article, or claim that may be made by its manufacturer, is not guaranteed or endorsed by the publisher.
Avgerinou, C., Giannezi, I., Theodoropoulou, S., Lazaris, V., Kolliopoulou, G., Zikos, P., et al. (2017). Occupational, dietary, and other risk factors for myelodysplastic syndromes in Western Greece. Hematology. 22, 419–429. doi: 10.1080/10245332.2016.1277006
Barrón Cuenca, J., Tirado, N., Barral, J., Ali, I., Levi, M., Stenius, U., et al. (2019). Increased levels of genotoxic damage in a Bolivian agricultural population exposed to mixtures of pesticides. Sci. Total Environ. 695, 133942.
Bernardi, B., Falcone, G., Stillitano, T., Benalia, S., Bacenetti, J., and De Luca, A. I. (2021). Harvesting system sustainability in Mediterranean olive cultivation: other principal cultivar. Sci. Total Environ. 766, 142508. doi: 10.1016/j.scitotenv.2020.142508
Bottarelli, E., and Ostanello, F. (2011). Epidemiologia. Teoria ed esempi di medicina veterinaria. Milano: Edagricole. p. 228.
Bovenzi, M., and Betta, A. (1994). Low-back disorders in agricultural tractor drivers exposed to whole-body vibration and postural stress. Appl. Ergon. 25, 231–241. doi: 10.1016/0003-6870(94)90004-3
Brentrup, F., Küsters, J., Lammel, J., and Kuhlmann, H. (2000). Methods to estimate on-field nitrogen emissions from crop production as an input to LCA studies in the agricultural sector. Int. J. LCA 5, 349–357.
Caballero, J. A., Labarta, J. A., Quirante, N., Carrero-Parreño, A., and Grossmann, I. E. (2020). Environmental and economic water management in shale gas extraction. Sustainability. 12, 168. doi: 10.3390/su12041686
Chen, Y., Chang, E. T., Liu, Q., Cai, Y., Zhang, Z., Chen, G., et al. (2021). Occupational exposures and risk of nasopharyngeal carcinoma in a high-risk area: A population-based case-control study. Cancer 127, 2724–2735. doi: 10.1002/cncr.33536
Cox, T., Griffiths, A., and Rial-Gonzalez, E. (2000). Research on Work-related Stress. Office for Official Publications of the European Communities. Available online at: http://europa.eu.int
D'Annolfo, R., Gemmill-Herren, B., Graeub, B., and Garibaldi, L. A. (2017). A review of social and economic performance of agroecology. Int. J. Agri. Sustain. 15, 632–644. doi: 10.1080/14735903.2017.1398123
De Bruyn, S., Bijleveld, M., de Graaff, L., Schep, E., Schroten, A., Vergeer, R., et al. (2018). Environmental Prices Handbook EU28 Version. CE Delft. p. 175.
De Luca, A. I., Falcone, G., Stillitano, T., Iofrida, N., Strano, A., and Gulisano, G. (2018). Evaluation of sustainable innovations in olive growing systems: a life cycle sustainability assessment case study in southern Italy. J. Cleaner Prod. 171, 1187–1202. doi: 10.1016/j.jclepro.2017.10.119
De Luca, A. I., Iofrida, N., Leskinen, P., Stillitano, T., Falcone, G., Strano, A., et al. (2017). Life cycle tools combined with multi-criteria and participatory methods for agricultural sustainability: insights from a systematic and critical review. Sci. Total Environm. 595, 352–370. doi: 10.1016/j.scitotenv.2017.03.284
Deguine, J. P., Aubertot, J.N., Bellon, S., Cote, F., Lauri, P. -E., Lescourret, F., et al. (2023). Agroecological crop protection for sustainable agriculture. Adv. Agronom. 178, 1–59. doi: 10.1016/bs.agron.2022.11.002
Domenighetti, G., D'Avanzo, B., and Bisig, B. (1999). Health effects of job insecurity among employees in the Swiss general population. Int. J. Health Serv. 30, 477–490.
Ekberg, K., Karlsson, M., Axelson, O., Björkqvist, B., Bjerre-Kiely, B., and Malm, P. (1995). Cross-sectional study of risk factors for symptoms in the neck and shoulder area. Ergonomics 38, 971–980. doi: 10.1080/00140139508925163
Elbaz, A., Clavel, J., Rathouz, P. J., Moisan, F., Galanaud, J. P., Delemotte, B., et al. (2009). Professional exposure to pesticides and Parkinson disease. Ann. Neurol. 66, 494–504.
European Commission Eurostat (2018). Economy-Wide Material Flow Accounts Handbook: 2018 edition. Available online at: https://data.europa.eu/, doi/10.2785/158567 (accessed January 10, 2023).
Falcone, G., and Stillitano T Iofrida N Spada E Bernardi B Gulisano G. (2022). Life cycle and circularity metrics to measure the sustainability of closed-loop agri-food pathways. Front. Sustainable Food Syst. 6, 1014228 doi: 10.3389/fsufs.2022.1014228
FAOSTAT (2021). Crops and Livestock Products, Online Data Warehouse. Available online at: https://www.fao.org/faostat/en/#data/QCL
Fritschi, L., Benke, G., Hughes, A. M., Kricker, A., Turner, J., Vajdic, C. M., et al. (2005). Occupational exposure to pesticides and risk of non-Hodgkin's lymphoma. Am. J. Epidemiol. 162, 849–857. doi: 10.1093/aje/kwi292
Georgescu-Roegen, N. (1971). The Entropy law and the Economic Process. Cambridge, MA: Harvard University Press.
Giampietro, M. (2019). On the circular bioeconomy and decoupling: implications for sustainable growth. Ecol. Econ. 162, 143–156. doi: 10.1016/j.ecolecon.2019.05.001
Giampietro, M., Aspinallis, R. J., Ramos-Martin, J., and Bukken, S. G. F. (2014). Resource Accounting for Sustainability Assessment: The Nexus Between Energy, Food, Water and Land Use. London: Routledge.London.
Giampietro, M., and Bukkens, S. G. F. (2015). Quality assurance of knowledge claims in governance for sustainability: transcending the duality of passion vs. reason. Int. J. Sustain. Develop. 18, 282–309.
Gliessman, S. (2018). Defining agroecology. Agroecol. Sustain. Food Syst. 42, 599–600. doi: 10.1080/21683565.2018.1432329
Goedkoop, M., and Heijungs R Huijbregts M De Schryver A Struijs J van Zelmet R. (2013). ReCiPe 2008 A life cycle impact assessment method which comprises harmonised category indicators at the midpoint and the endpoint level First edition (version 1.08) Report I: Characterisation. Hague: Ministerie van VROM Rijnstraat 8, 2515 XP Den Haag (NL).
González de Molina, M., Soto Fernández, D., Guzmán Casado, G., Infante-Amate, J., Aguilera Fernández, E., Vila Traver, J., et al. (2020). “Agrarian Metabolism: The Metabolic Approach Applied to Agriculture,” in The Social Metabolism of Spanish Agriculture, 1900–2008. Environmental History, vol 10. Cham: Springer. doi: 10.1007/978-3-030-20900-1_1
González de Molina, M., and Toledo, V. M. (2014). The Social Metabolism: A Socio-Ecological Theory of Historical Change. Cham: Springer.
Gupta, N., Christiansen, C.S., Hallman, D.M., Korshøj, M., Carneiro, I.G., and Holtermann, A. (2015). Is objectively measured sitting time associated with low back pain? A cross-sectional investigation in the NOMAD study. PLoS One. 10, 1–18. doi: 10.1371/journal.pone.0121159
Guzmán, G. I., Aguilera, E., García-Ruiz, R., Torremocha, E., Soto, D., Infante-Amate, J., et al. (2018). The agrarian metabolism as a tool for assessing agrarian sustainability, and its application to Spanish Agriculture (1960-2008). Ecol. Soc. 23, 1–20.
Guzmán, G. I., Fernández, D. S., Aguilera, E., Infante-Amate, J., and González de Molina, M. (2022). The close relationship between biophysical degradation, ecosystem services and family farms decline in Spanish agriculture (1992–2017). Ecosyst. Serv. 56, 101456. doi: 10.1016/j.ecoser.2022.101456
Guzmán, G. I., and González de Molina, M. (2015). Energy efficiency in agrarian systems from an agroecological perspective. Agroecol. Sustain. Food Syst. 39, 924–952. doi: 10.1080/21683565.2015.1053587
Guzmán, G. I., and González de Molina, M. (2017). Energy in Agroecosystems: A Tool for Assessing Sustainability. Boca Raton, FL: CRC Press.
Guzmán, G. I., González de Molina, M., Soto, D., Infante-Amate, J., and Aguilera, E. (2017). Spanish agriculture from 1900 to 2008: a long-term perspective on agroecosystem energy from an agroecological approach. Reg. Environ. Chang. 149, 335–348.
Haberl, H. (2001). The energetic metabolism of societies. I: accounting concepts. J. Ind. Ecol. 5, 11–33.
Haberl, H., Wiedenhofer, D., Pauliuk, S., Krausmann, F., Müller, D. B., and Fischer-Kowalski, M. (2019). Contributions of sociometabolic research to sustainability science. Nat. Sustainab. 2, 173–184. doi: 10.1038/s41893-019-0225-2
Hernandez, P., Oregi, X., Longo, S., and Cellura, M. (2019). “Life cycle assessment of buildings,” in Handbook of Energy Efficiency in Buildings, eds U. Desideri and F. Asdrubali (Butterworth-Heinemann), 207–261.
Hildebrandt, V.H., Bongers, P.M., Van Dijk, F. J. H., Kemper, H. C. G., and Dul, J. (2001). Dutch musculoskeletal questionnaire: Description and basic qualities. Ergonomics 44, 1038–1055. doi: 10.1080/00140130110087437
Hoppin, J. A., Umbach, D. M., London, S. J., Alavanja, M. C., and Sandler, D. P. (2004). Diesel exhaust, solvents, and other occupational exposures as risk factors for wheeze among farmers. Am. J. Respir. Crit. Care Med. 169, 1308–1313. doi: 10.1164/rccm.200309-1228OC
Horne, R., Grant, T., and Verghese, K. (2009). Life Cycle Assessment. Principles, Practice and Prospects. CSIRO Publishing.
Huijbregts, M.a.J., Steinmann, Z.J., Elshout, P.M.F., Stam, G., Verones, F. Vieira, M.D.M., Zijp, M., et al. (2016). ReCiPe 2016: a harmonized life cycle impact assessment method at midpoint and enpoint level - report 1: characterization. Natl. Inst. Publ. Health Environ. 194, 1246 doi: 10.1007/s11367-016-1246-y
Infante-Amate, J., Aguilera, E., Palmeri, F., Guzmán, G., Soto, D., García-Ruiz, R., et al. (2018). Land embodied in Spain's biomass trade and consumption (1900-2008): historical changes, drivers and impacts. Land Use Policy 78, 493–502.
International Olive Council - IOC. (2021). EU Olive Oil Figures. Available online at: https://www.internationaloliveoil.org/wp-content/uploads/2021/12/HOCE901-17-12-2021-P.pdf (accessed January 10, 2023).
Iofrida, N., De Luca, A. I., Gulisano, G., and Strano, A. (2018a). An application of Q-methodology to Mediterranean olive production – stakeholders' understanding of sustainability issues. Agricult. Syst.s 162, 46–55. doi: 10.1016/j.agsy.2018.01.020
Iofrida, N., De Luca, A. I., Silveri, F., Falcone, G., Stillitano, T., Gulisano, G., et al. (2019). Psychosocial risk factors' impact pathway for social life cycle assessment: an application to citrus life cycles in South Italy. Int. J. Life Cycle Assess. 24, 767–780. doi: 10.1007/s11367-018-1482-4
Iofrida, N., De Luca, A. I., Strano, A., and Gulisano, G. (2018b). Can social research paradigms justify the diversity of approaches to social life cycle assessment? Int. J. Life Cycle Assess. 23, 464–480. doi: 10.1007/s11367-016-1206-6
Iofrida, N., Stillitano, T., Falcone, G., Gulisano, G., Nicolò, B.F., and De Luca, A. I. (2020). The socio-economic impacts of organic and conventional olive growing in Italy. New Medit. 19, 117–131. doi: 10.30682/nm2001h
IPCC. (2019). N2O Emissions From Managed Soils And CO2 Emissions From Lime And Urea Application. IPCC.
ISO (2021). Environmental Management - Life Cycle Assessment – Principles and framework; Requirements and Guidelines. Geneva: International Organization for Standardization.
Jeswani, H. K., Hellweg, S., and Azapagic, A. (2018). Accounting for land use, biodiversity and ecosystem services in life cycle assessment: impacts of breakfast cereals. Sci. Total Environm. 645, 51–59. doi: 10.1016/j.scitotenv.2018.07.088
Kachuri, L., Demers, P. A., Blair, A., Spinelli, J. J., Pahwa, M., McLaughlin, J. R., et al. (2013). Multiple pesticide exposures and the risk of multiple myeloma in Canadian men. Int. J. Cancer. 133, 1846–1858. doi: 10.1002/ijc.28191
Keawduangdee, P., Puntumetakul, R., Chatchawan, U., Kaber, D., and Siritaratiwat, W. (2012). Prevalence and associated risk factors of low-back pain in textile fishing net manufacturing. Human Fact. Ergonom. Manufactur. 22, 562–570. doi: 10.1002/hfm.20375
Kumar, A., and Pant, S. (2023). Analytical hierarchy process for sustainable agriculture: an overview. MethodX. 10, 101954. doi: 10.1016/j.mex.2022.101954
Landert, J., Pfeifer, C., Carolus, J., Schwarz, G., Albanito, F., Muller, A., et al. (2020). Assessing agro-ecological practices using a combination of three sustainability assessment tools. Landbauforschung. 70, 129–144. doi: 10.3220/LBF1612794225000
Matthews, E. (2000). The Weight of Nations: Material Outflows From Industrial Economies. Washington, DC: World Resources Institute.
Min, Y. I., Anugu, P., Butler, K. R., Hartley, T. A., Mwasongwe, S., Norwood, A. F., et al. (2017). Cardiovascular disease burden and socioeconomic correlates: findings from the Jackson heart study. J. Am. Heart Assoc. 6, e004416. doi: 10.1161/JAHA.116.004416
Mottet, A., Bicksler, A., Lucantoni, D., De Rosa, F., Scherf, B., Scopel, E., et al. (2020). Assessing transitions to sustainable agricultural and food systems: A tool for agroecology performance evaluation (TAPE). Front. Sustain. Food Syst. 4, 1–21. doi: 10.3389/fsufs.2020.579154
Porter, P., and Francis, C. A. (2016). “Agroecology: Farming Systems with Nature as Guide, Second Edition. Ed,” in Encyclopedia of Applied Plant Sciences. Amsterdam: Elsevier. doi: 10.1016/B978-0-12-394807-6.00239-2
Rabbani, U., and Fatmi, Z. (2018). Incidence, patterns and associated factors for occupational injuries among agricultural workers in a developing country. Med. J. Islam Repub. Iran. 32, 88. doi: 10.14196/mjiri.32.88
Raeisi, S., Namvar, M., Golabadi, M., and Attarchi, M. (2014). Combined effects of physical demands and shift working on low back disorders among nursing personnel. Int. J. Occup. Saf. Ergon. 20, 159–166. doi: 10.1080/10803548.2014.11077034
Russo, C., Cappelletti, G.M., Nicoletti, G.M., Di Noia, A.E., and Michalopoulos, G. (2016). Comparison of European olive production systems. Sustainability. 8, 825. doi: 10.3390/su8080825
Saaty, T. L. (1990). How to make a decision: The analytic hierarchy process. Euro. J. Operat. Res. 48, 9–26. doi: 10.1016/0377-2217(90)90057-I
Scheidel, A., and Sorman, A. H. (2012). Energy transitions and the global land rush: ultimate drivers and persistent consequences. Glob. Environ. Change 22, 559–794.
Shao, M., Han, Z., Sun, J., Xiao, C., Zhang, S., and Zhao, Y. (2020). A review of multi-criteria decision making applications for renewable energy site selection. Renew. Energy 157, 377–403. doi: 10.1016/j.renene.2020.04.137
Spada, E., Stillitano, T., Falcone, G., Iofrida, N., Gulisano, G., and De Luca, A. I. (2022). Economic sustainability assessment of Mediterranean crops: A comparative Life Cycle Costing (LCC) analysis. Front. Sustainable Food Syst. 6, 1004065. doi: 10.3389/fsufs.2022.1004065
Stillitano, T., Falcone, G., Iofrida, N., Spada, E., Gulisano, G., and De Luca, A. I. (2022). A customized multi-cycle model for measuring the sustainability of circular pathways in agri-food supply chains. Sci. Total Environm. 844, 157229. doi: 10.1016/j.scitotenv.2022.157229
Stillitano, T., Spada, E., Iofrida, N., Falcone, G., and De Luca, A. I. (2021). Sustainable agri-food processes and circular economy pathways in a life cycle perspective: State of the art of applicative research. Sustainability (Switzerland). 13, 1–29. doi: 10.3390/su13052472
Stock, S., Vézina, N., Seifert, A. M., Tissot, F., and Messing, K. (2006). Les troubles musculo-squelettiques, la détresse psychologique et les conditions de travail au Québec: relations complexes dans un monde du travail en mutation. Santé.Société Solidar. 2, 45–58.
Szumilas, M. (2010). Explaining odds ratios. J. Canad. Acad. Child Adolesc. Psychiatry 19, 227–229. doi: 10.1136/bmj.c4414
Tello, E., Galán, E., Sacristán, V., Cunfer, G., Guzmán, G. I., González de Molina, M., et al. (2016). Opening the black box of energy throughputs in farm systems: a decomposition analysis between the energy returns to external inputs, internal biomass reuses and total inputs consumed, (the Vallès County, Catalonia, c.1860 and 1999). Ecol. Econ. 121, 160–174.
UNEP (2020). Guidelines for Social Life Cycle Assessment of Products and Organizations 2020. Benoît Norris, C., Traverso, M., Neugebauer, S., Ekener, E., Schaubroeck, T., Russo Garrido, S., et al. (eds.). United Nations Environment Programme (UNEP).
UNEP (2021). “Methodological sheets for subcategories in social life cycle assessment (S-LCA) 2021,” in United Nations Environment Programme (UNEP), Traverso, M., Valdivia, S., Luthin, A., Roche, L., Arcese, G., Neugebauer, S., et al. (eds.).
van der Ploeg, J. D., Barjolle, D., Bruil, J., Brunori, G., Costa Madureira, L. M., Dessein, J., et al. (2019). The economic potential of agroecology: empirical evidence from Europe. J. Rural Stud. 71, 46–61. doi: 10.1016/j.jrurstud.2019.09.003
Verones, F., Hellweg, S., Azevedo, L. B., Chaudhary, A., Cosme, N., Fantke, P., et al (2016). LC-IMPACT Version 0.5- A Spatially Differentiated Life Cycle Impact Assessment Approach. Available online at: http://www.lc-impact.eu/ (accessed April 28, 2017).
Weisz, H., Krausmann, F., Amann, C., Eisenmenger, N., Erb, K. H., Hubacek, K., et al. (2006). The physical economy of the European Union: Crosscountry comparison and determinants of material consumption. Ecol. Econ. 58, 676–698.
Keywords: agroecology, sustainability, ecosystem services, circular economy, olive growing, Social Agrarian Metabolism, Life Cycle Sustainability Assessment
Citation: De Luca AI, Iofrida N, González de Molina M, Spada E, Domouso P, Falcone G, Gulisano G and García Ruiz R (2023) A methodological proposal of the Sustainolive international research project to drive Mediterranean olive ecosystems toward sustainability. Front. Sustain. Food Syst. 7:1207972. doi: 10.3389/fsufs.2023.1207972
Received: 18 April 2023; Accepted: 26 June 2023;
Published: 19 July 2023.
Edited by:
Luuk Fleskens, Wageningen University and Research, NetherlandsReviewed by:
Jacopo Bacenetti, University of Milan, ItalyCopyright © 2023 De Luca, Iofrida, González de Molina, Spada, Domouso, Falcone, Gulisano and García Ruiz. This is an open-access article distributed under the terms of the Creative Commons Attribution License (CC BY). The use, distribution or reproduction in other forums is permitted, provided the original author(s) and the copyright owner(s) are credited and that the original publication in this journal is cited, in accordance with accepted academic practice. No use, distribution or reproduction is permitted which does not comply with these terms.
*Correspondence: Nathalie Iofrida, bmF0aGFsaWUuaW9mcmlkYUB1bmlyYy5pdA==
Disclaimer: All claims expressed in this article are solely those of the authors and do not necessarily represent those of their affiliated organizations, or those of the publisher, the editors and the reviewers. Any product that may be evaluated in this article or claim that may be made by its manufacturer is not guaranteed or endorsed by the publisher.
Research integrity at Frontiers
Learn more about the work of our research integrity team to safeguard the quality of each article we publish.