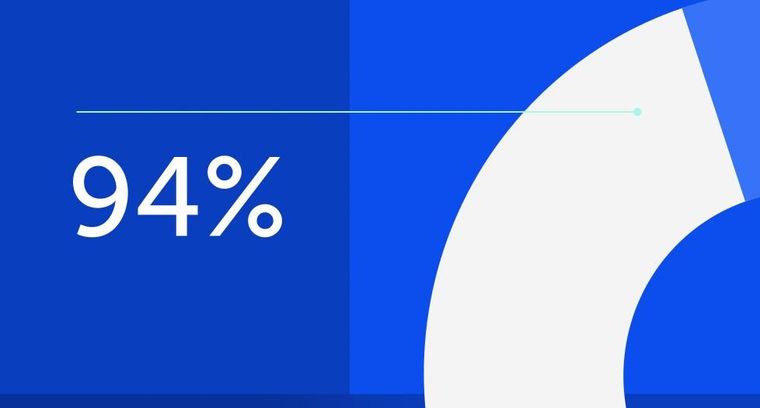
94% of researchers rate our articles as excellent or good
Learn more about the work of our research integrity team to safeguard the quality of each article we publish.
Find out more
ORIGINAL RESEARCH article
Front. Sustain. Food Syst., 14 August 2023
Sec. Land, Livelihoods and Food Security
Volume 7 - 2023 | https://doi.org/10.3389/fsufs.2023.1207913
Banana Xanthomonas wilt (BXW) is one of the most important diseases threatening banana production in Africa south of the Sahara (SSA). In this study, we examine the potential impacts of BXW on banana production, demand, and food security in SSA, if the disease spread across all banana-producing countries in the region. The analysis is based on a multidisciplinary approach that combines a mathematical model of field-level BXW spread over time with a dynamic global partial equilibrium economic model. Since BXW control relies exclusively on management, we analyze three scenarios of BXW spread that are constructed around assumptions about the level of policy response to the disease, and about how this response may affect the adoption of appropriate management practices by farmers to control BXW. Modeling results suggest that if the disease is left uncontrolled, banana production in SSA within 10 years can decrease by as much as 55%, compared to a BXW-free baseline scenario, resulting in economic losses of around 25 billion USD. At the same time, the population at risk of hunger in countries that highly depend on bananas as a staple food is projected to increase by more than 4.6%. Even a limited policy response to BXW can reduce infections and mitigate some of the production, economic, and food security consequences. BXW impacts are almost completely negated when farmers have good knowledge of the disease and fully adopt the appropriate management practices. This result highlights the need for policy frameworks which rely on sustained and coordinated efforts by public and private stakeholders, within and across SSA countries and at different geographical scales. It also aims to raise awareness and promote the adoption of such practices, while also considering local peculiarities and socioeconomic conditions.
Pests and diseases can cause substantial crop yield losses (Oerke, 2006; Savary et al., 2019) and therefore pose a serious threat to farming systems worldwide. The threat is greater for vegetatively propagated crops (Petsakos et al., 2019), for subsistence and food-deficit systems (Savary et al., 2019), for monocultures (Dato et al., 2021), and in regions where farmers have limited access to extension services (Ocimati et al., 2019). Banana and plantain are typical crops that fit the above profile, particularly in Africa south of the Sahara (SSA), where they are grown under various production systems and constitute an important source of food and income for many of the region's poorest households.1 Currently, SSA produces about 50 million tons of banana per year (30% of global production) covering more than seven million hectares (60% of global areas). Some countries in the region, like Uganda, also exhibit the world's highest levels of banana consumption in terms of total daily calorie intake per capita (FAO, 2023).
Despite the importance of banana for the regional agricultural economy, average yields are almost half the global average of 14 t/ha (FAO, 2023). Notwithstanding the importance of abiotic and marketing constraints (Tinzaara et al., 2018), pests and diseases are one of the main reasons for the low banana yields in SSA. Banana Xanthomonas wilt (BXW), caused by the bacterium Xanthomonas vasicola pv. musacearum (Xvm), is the most widespread bacterial disease in Africa (Blomme et al., 2017), and it has been characterized as one of the four most important emerging infectious diseases of crop plants in developing countries (Vurro et al., 2010). First officially discovered in Ethiopia on enset and banana in 1968 and 1974, respectively (Yirgou and Bradbury, 1968, 1974), it is now considered endemic in all East and Central Africa (ECA) countries in the Great Lakes region, comprising Uganda, Rwanda, Tanzania, Kenya, Burundi, and the Democratic Republic (DR) of the Congo. BXW is spreading westwards, putting banana production in the whole SSA region at risk (Ocimati et al., 2019). Although it has no cure, and all banana types are susceptible, a set of cultural practices can be used to contain it in the field (Blomme et al., 2017). When left uncontrolled, or if its management is delayed, BXW can have economically devastating effects, with 100% yield losses and entire banana plantations destroyed (Tushemereirwe et al., 2006; Nkuba et al., 2015; Geberewold, 2019).
Several authors have attempted to assess the expected economic damage if BXW was not controlled, using empirical relationships for spread rates derived from reported infection and yield loss data (Kalyebara et al., 2006; Tushemereirwe et al., 2006). Although such estimates constitute important inputs to our understanding of the economic dimension of a BXW epidemic, the literature has thus far focused on ECA countries—particularly Uganda. However, the possibility of BXW spreading elsewhere in SSA has not yet been considered. The broader impacts of the disease on food systems and food security have also been overlooked, despite field evidence indicating that a decrease in banana yields will likely trigger changes in market prices, crop land allocations and can even prompt farmers to abandon the cultivation of banana altogether in favor of substitute crops (Nkuba et al., 2015; Ocimati et al., 2020; Mbabazi et al., 2021). Consequently BXW-induced yield shocks can lead to changes in the supply and demand of both banana and substitute commodities, thereby affecting producers and consumers alike.
The above discussion suggests that the assessment of BXW impacts on banana output, and on food systems in general, calls for integrated methods and tools representing economic equilibrium across multiple commodities. However, relevant modeling frameworks lack an explicit pest and disease component. As a result, only a handful of studies have ever attempted to model regional or global epidemics (Godfray et al., 2016; Mason-D'Croz et al., 2020). The reason for this gap in the literature is the relatively slow progress made toward pest-crop modeling (Cunniffe et al., 2015; Newbery et al., 2016), contrary to abiotic constraints, like temperature and CO2, that have already found their way into integrated global modeling platforms used for climate change assessments (Nelson et al., 2014).
An equally important modeling challenge is that the spread and impact of crop pests and diseases depend on many interconnected factors which are not only biophysical, but also socioeconomic and therefore difficult to quantify. Evidence from ECA countries reveals that crop yield loss to BXW are contingent on the capacity of farmers to control the disease. In turn, this capacity is highly correlated with the availability and adoption of appropriate technologies, the level of engagement by governments and non-governmental entities to address the problem, and the amount of funding allocated to this goal. In many ECA countries, the mobilization and cooperation of local governments, public research institutions, extension agencies, and other development organizations (hereafter, “policy response”) played a catalytic role in raising awareness, monitoring BXW spread, training farmers on appropriate management practices and fostering the adoption of such practices (Kubiriba and Tushemereirwe, 2014). Despite the constraints imposed by existing national policies, institutions, and strategies (or lack thereof), and the little regional collaboration among ECA countries, stakeholder mobilization has often resulted in controlling the spread of the disease, albeit temporarily, since the required level of policy response was not always maintained (Tinzaara et al., 2013; McCampbell et al., 2018).
Given the need for more comprehensive assessments of BXW risk, this paper presents an initial assessment of the potential production, economic and food security impacts of BXW spread across banana-producing countries in SSA. The analysis is based on a set of scenarios which incorporate assumptions about how the policy response affects farmers' capacity to control BXW in already infected fields, and to prevent it from spreading to new fields. The scenarios draw on the lessons learned about the type, intensity, and effectiveness of policy response to BXW in endemic ECA countries (in the Great Lakes region, where BXW is present and disease control measures have been applied). They represent possible pathways of disease resurgence in ECA, but they also describe trajectories of spread to threatened countries (elsewhere in SSA, where the disease has not been reported yet, and no control measures are in place).
The scenarios are analyzed using a multidisciplinary approach that combines a global multi-year economic partial equilibrium model with a mathematical model of BXW spread which is parameterized according to the different scenarios of policy response. To the extent of our knowledge, this is the first attempt to link an economic partial equilibrium model to a disease spread model. This model linkage allows for multiyear assessments of disease spread that can incorporate the dynamics of market-level responses, but also foresight elements such as population and economic growth. The resulting modeling framework therefore considers various factors that may affect BXW spread across time and space, and at different scales.
The current study is not meant to be a detailed impact assessment of banana BXW in SSA. Instead, it is a “what-if” analysis of the potential spread pathways of BXW across banana growing areas in the region. By proposing a multidisciplinary framework of analysis that accounts for the role of stakeholder interventions, the study also aims at paving the way for more integrated assessments of crop disease risks on food systems. It also aims at initiating a discussion about modeling challenges that arise during such synthesis.
The analysis considers two dimensions of BXW spread over time. For the purposes of this modeling exercise, we define incidence as the proportion of infected plants in a banana field/region, and prevalence as the proportion of infected banana fields in a country. Therefore, we consider spread both within a given banana field (how fast BXW spreads from infected to healthy plants), and across banana fields/regions (how fast BXW reaches new fields in the landscape/country). We use the two concepts for both, disease resurgence in ECA countries, and new outbreaks elsewhere in SSA.
Incidence is estimated for each response scenario with a BXW model developed by Nakakawa et al. (2016). On the other hand, the change in prevalence (the number of additional fields infected every year) is specified through assumptions—based on expert knowledge— that are part of the narrative for each scenario. Field-level incidence over time from the disease model is combined with the prevalence assumptions of the analyzed scenario to calculate annual yield losses at national level. The main assumption during the calculation of annual yield losses with the BXW model is that incidence (the share of infected plants within a field) corresponds to the share of yield loss from the same field. These yield losses are then introduced as productivity shocks into the International Model for Policy Analysis of Agricultural Commodities and Trade (IMPACT) (Robinson et al., 2015), which projects changes in supply, demand, prices for banana in affected countries over a 10-year period. Based on these results, we estimate changes to basic food security indicators and assess the potential economic damage in terms of changes of consumer and producer surpluses. Figure 1 presents a diagrammatic summary of the modeling framework.
Figure 1. Summary depiction of the modeling framework: we first identify N banana fields of 50 km2 size in an affected country. Then, BXW incidence is estimated annually according to management assumptions of the scenario simulated, and for an increasing number of banana fields per year that is determined by the prevalence assumptions of the scenario. Finally, incidence generated by the BXW model, and prevalence assumptions are combined to estimate yield losses which are introduced as productivity shocks in the IMPACT model.
The BXW spread model (Nakakawa et al., 2016) estimates incidence over time, t, in a hypothetical banana field or zone (a banana plantation). The model distinguishes the population of banana stems in the plantation, N(t), into healthy, S(t), and infected, I(t) classes. It assumes a negligible incubation period so that once a stem is visibly infected then it is infectious, and bacteria can be transmitted to other physically attached stems. The population growth of the plantation follows a logistic function r × [1 – N(t)/K], where K is the optimum carrying capacity of the plantation (number of stems per size of field/plantation simulated) and r is the growth/equilibrium rate, which expresses the replenishment of host plants via emergence of new suckers (stems per unit of time). The equilibrium rate is assumed to be influenced by factors that keep the plantation in equilibrium without the disease. These include: the number of stems on a particular mat as the result of sucker emergence, removal of excess suckers and harvesting of fruit-bearing stems.
Healthy stems can get infected either by use of contaminated tools or via the inflorescence by flying vectors. It is further considered that an infected stem may transmit the pathogen to the physically attached suckers (vertical transmission) if not removed in time. In this case, given the incomplete systemicity (Ocimati et al., 2013), we denote the proportion of infected suckers on the infected stems by θ. This implies that (1 – θ) is the proportion of healthy suckers from infected stems. Disease transmission is modeled using standard incidence, whereby the coefficients of effective contact between a healthy and an infected stem are denoted by βt and βv for transmission by tools and vectors respectively (Li and Zhang, 2017). Disease spread in both cases is frequency dependent. This means that transmission is not affected by the total plantation size, or by the number of infected stems. Instead, it depends on how many of these infected stems get in contact with healthy stems either by vectors or tools (Keeling and Rohani, 2011).
Let u be the control term (%) associated with the disinfection of tools used during cultivation, pruning, or harvesting. Then (1–u) indicates failure to disinfect tools. Similarly, if v is the control term (%) associated with de-budding by using a forked stick, (1–v) will represent failure to de-bud. Let δ denote control associated with removal of infected stems, either by rogueing or with the single diseased stem removal (SDSR) technique (Blomme et al., 2014). Then, δ = 1 indicates successful removal of infected stems. Based on these assumptions and the notation introduced, the following system of differential equations describes the dynamics of BXW when control measures are being implemented:
We define the basic reproduction number, R0, as the number of new infections from introducing a single infected plant or tool in a fully healthy plantation. This is a threshold used to determine under what conditions the disease will persist or will be wiped out. To obtain the basic reproduction number we consider the next generation matrix as described in van den Driessche (2017). Thus,
When u = 1 (i.e., successful disinfection of tools), then R0 is based on disease spread by vectors only. Conversely, when v = 1, transmission is by tools only. Transmission from the mother plant to an attached sucker is not captured in the basic reproduction number since it does not imply introduction of new infections within the plantation. The disease attains an endemic equilibrium when R0 > 1, whereas disease infections start to decline when R0 < 1. Therefore, for successful management of BXW, that is, to achieve R0 < 1, the value of the transmission coefficients βt and βv should reduce and the rogueing parameter δ increase. In that case, the disease is eventually eradicated if there are no new infections within the plantation. When R0 > 1, the existing endemic equilibrium point is unique and globally asymptomatically stable. This means that, irrespective of the initial size of infected stems, the plantation may entirely be destroyed if no control measures are implemented to reduce the value of R0 below unity.
IMPACT is a global multi-market partial equilibrium model which is found at the core of an integrated modeling framework that also includes biophysical (water, climate, livestock, and fishery) models (Robinson et al., 2015). It can generate scenario-based projections to 2050 for the global agricultural sector, driven by assumptions about trends in socioeconomic and climate variables. For this reason, IMPACT has been used extensively as a tool for foresight analysis to answer questions related to climate change and food security. Examples include: the analysis of climate change impacts on agriculture and nutrition (Wiebe et al., 2015; Beach et al., 2019; Sulser et al., 2021); the assessment of climate mitigation technologies (Islam et al., 2016); projections of consumption and production of specific commodities (Enahoro et al., 2019; Petsakos et al., 2019; Kruseman et al., 2020); the assessment of impacts of international agricultural research (Fuglie et al., 2022); and crop/animal disease epidemics at various scales (Godfray et al., 2016; Mason-D'Croz et al., 2020).
The model calculates the supply and demand equilibrium for 62 agricultural commodities in 320 Food Production Units (FPUs), which are created by the overlapping of one or more water basins with 158 political units (i.e., individual countries or regions). Supply of crop commodities is calculated at each FPU as the product of crop-specific yield and area functions. Yields and areas are affected by a different set of drivers, including commodity and input prices, climate, and water availability. Demand for food (and non-food) commodities depends on available income, related income demand elasticities, and prices. Different countries are linked by trade, and the model solves global prices that clear commodity markets. IMPACT does not determine diet compositions, but it outputs food security indicators related to caloric intake from an average commodity bundle demanded in each country at the solved market equilibrium (e.g., people at risk of hunger and undernourished children). The model also includes a post solution module which we use in this paper to calculate changes in consumer and producer surplus over time due to BXW infections. Welfare calculations assume a 5% discount rate. Although this module does not capture any welfare impacts outside of agriculture, it can still provide insights about the potential economic consequences of various types of production shocks, including new crop technologies (Petsakos et al., 2018) or, in this case, crop diseases. Furter details about the model structure can be found in the model's documentation (Robinson et al., 2015).
For the purposes of the study, we have assumed that modeling of BXW infections covers the 10-year period 2021–2030. Although IMPACT can provide projections up to 2050, this shorter time frame rationalizes the policy scenarios as a continuation of (or lessons learned from) the response to BXW that has already been observed across endemic ECA countries and as such it constitutes a more policy-relevant horizon. Moreover, 2030 represents a year not far into the future where climate and socioeconomic changes are present but not so pronounced (compared to 2050). There is also less uncertainty around the available technologies and disease development under climate change.
To assess the potential impacts from BXW infections, we first characterize a “baseline” (zero-disease) scenario that serves as a reference situation for the analysis. The baseline scenario is constructed by combining the Shared Socioeconomic Pathway 2 (SSP2) with the Representative Concentration Pathway 8.5 (RCP8.5). For simulating BXW infections for the different types of policy response, we modify the banana yields of this baseline scenario using the outputs of the BXW spread model. A brief discussion about the assumptions underpinning the selected SSP-RCP combination, and the parametrization of IMPACT for this study are given in Supplementary Figure S1.
We specify three policy response scenarios to BXW with which we assess the potential impacts of BXW on SSA countries: (i) Quick and Effective (Q&E); (ii) Slow and Limited (S&L); and (iii) zero response. The scenarios incorporate different assumptions about BXW spread and severity and describe an assumed cycle of events over 10 years, starting from the moment when BXW is first reported, or when it reappears in farmers' fields (in the case of resurgences in ECA countries). A generic narrative for each scenario, is given below:
- Quick and effective policy response
On disease outbreak (or resurgence), the government and other private stakeholders mobilize immediately, and farmers widely apply the appropriate management practices already 1 year after the first BXW cases. As a result, incidence decreases gradually over time. Prevalence increases slightly, as the disease manages to escape to some new banana fields, but because of control efforts, it remains low, at a maximum of 20%. Once the disease is controlled and becomes unobservable, stakeholders reduce their overall engagement and the intensity of the mobilization campaign. Similarly, farmers relax the rigorous application of the management protocols. This eventually leads to new BXW infections, but the government immediately intervenes, and the disease is contained once more without spreading to other areas. The cycle of policy interventions and incidence fluctuations repeats.
- Slow and limited policy response
The government responds to the disease with limited resources and with significant delay, which leads to very low adoption of the recommended control package. Farmers eventually adopt and implement the required management practices, but without adequate or sustained support from the government they quickly abandon them. Subsequent policy interventions are equally limited in intensity and duration. Although interventions temporarily reduce incidence, the disease resurges. The cycle of incidence fluctuations repeats and BXW spreads fast across banana fields throughout the country, reaching 100% by year 6.
- Zero policy response
The government does not respond. The disease quickly reaches high incidence and prevalence levels and farmers do not have enough knowledge on how to contain it. Owing to interventions by private stakeholders (e.g., international, and local NGOs, international research organizations), they learn to apply some management techniques. However, this is not enough to stop the disease from spreading, and the whole country is affected faster than in other scenarios, with prevalence reaching 100% by year 5.
It is important to note that the specification of the scenarios represents the authors' interpretation of possible pathways of BXW spread, which is based on the observed duration and intensity of stakeholder mobilization in ECA during previous BXW outbreaks. We also acknowledge that BXW spread in all scenarios over time will likely be determined by additional factors, beyond just stakeholder mobilization, which are not explicitly mentioned in the scenario narrative (e.g., climate suitability, development of resistant varieties). The “zero” scenario, in particular, describes a worst-case situation in which the disease spreads uncontrollably. Although it is highly unlikely that all threatened SSA countries will exhibit “zero” response to XW simultaneously because of these other factors that are not explicitly captured, we use this scenario as an extreme counterfactual of stakeholder inaction.
Among the countries in the region, Kenya, Rwanda, Tanzania, and Uganda are examples of Q&E response, having already invested in raising awareness about BXW and in training farmers. Burundi initially exhibited a S&L response to BXW since 2010, when the disease was first reported in the country; farmers' awareness about the disease was found to be very low almost 1 year after the first infections (Ndayihanzamaso et al., 2016). Recently, however, farmers, local extension services and other governmental institutions have shown increased interest in the SDSR technique for controlling BXW, which has led to a substantial reduction in infection levels (Kikulwe et al., 2022). Finally, the policy response observed in DR Congo over the past years falls within the S&L and “zero” categories. The limited stakeholder interventions are mainly due to political problems and civil instability in the country (Ndungo et al., 2008). For the above countries, it may be possible to infer a single type of policy response to BXW, which is most likely to occur in the future, given past experience and recent developments. Nevertheless, we decided to model all three scenarios for these countries to consider alternative pathways of BXW resurgence and to simplify the analysis.
From a modeling viewpoint, the type of response in each scenario determines the rates of incidence and prevalence. As regards incidence, the scenarios can be interpreted as sequences of 6-month periods over 10 years, where each period is dominated by one specific state of nature for BXW management, or “management regime,” which represents the overall effectiveness of disease control. The 6-month duration of a regime is the assumed minimum length of a period required for any change to occur in the average level of management, following the mobilization of public and private stakeholders.
We identify three such regimes: (i) No Adoption–NA; (ii) Partial Adoption–PA; and (iii) Full Adoption–FA. The NA regime refers to a situation of high BXW incidence in which farmers receive very limited training and stakeholder support. As a result, they have no or little knowledge about BXW, its symptoms, or about the required management practices. NA. The FA regime describes the opposite situation, and it assumes that farmers have deep knowledge about BXW, they adopt and rigorously apply all the required management techniques. Under FA, farmers receive strong support and proper training, and the regime is characterized by low disease incidence. PA is somewhere between the previous two cases and represents a middle-of-the-road management situation in which farmers receive only some stakeholder support, and partially adopt the recommended control package. PA can also eventually result from adaptation and learning, even without any formal training. Figure 2 presents the schedule of switching management regimes for each of the policy response scenarios.
Figure 2. Specification of policy response scenarios as sequences of management regimes. NA, No adoption of management practices; FA, Full adoption of management practices; PA, Partial adoption of management practices; Q&E, quick and effective response; S&L, slow and limited response.
The BXW model, with which we calculate incidence, reflects the different scenario assumptions by using different values per management regime for a selected set of model parameters. These parameters include the coefficient of tool transmission (βt), and the rates of BXW control by tool disinfection (u), de-budding (ν) and rogueing (δ). A detailed discussion on the selected parameter values for each management regime, and a sensitivity analysis on how each parameter affects the results of the BXW model is given in the Supplementary material.
The specification of the prevalence dimension of the scenarios (i.e., the share of infected fields over time), was based on the calculation of the number of fictitious banana fields in each country, by dividing banana areas, as reported in FAOSTAT for 2020, into hypothetical 50 km2 fields.2 The selected field size of 50 km2 corresponds to the assumed BXW area spread in the East African Highland, or plantain-dominated production systems over a 6-month period (Guy Blomme, personal communication, February 8, 2022). We remind that 6 months is also the time interval used for the specification of incidence and for the switching of management regimes, as described above. This back-of-the-envelope calculation finally resulted in an integer number of banana fields per country (rounded up) that was used in each scenario to determine the additional fields infected over time. More details about how annual prevalence rates were calculated under all policy response scenarios are given in the Supplementary material.
A strong assumption underpinning the above specification of the prevalence dimension is that all banana fields within the same country are considered homogeneous in terms of production conditions, exhibiting similar yields. Although it is reasonable to expect substantial spatial heterogeneity in potential BXW spread, neither the disease model, nor the current version of IMPACT have the capacity to generate spatially explicit outputs. A spatially explicit disease model could provide insights about the potential pathways of BXW spread across SSA and would thus negate the need to specify prevalence assumptions based on expert knowledge. Previous authors have already identified the need for spatially explicit disease models that can be linked to IMPACT in a meaningful way (Godfray et al., 2016). This topic is currently under research.
We use the BXW model to calculate incidence in each 6-month period, and over several fields in a country according to the prevalence assumptions of the scenario. More generally, an increase in prevalence at time t means that the disease has spread to new fields. When simulating BXW incidence for these new fields we assume an initial level of incidence of 0%. For fields that were infected before period t, the simulation of incidence starts from the solution of the BXW model at t−1. Hence, fields assumed to have been infected at the start of year 1 are modeled for all 10 years (or equivalently for 20 six-month periods), whereas fields infected in year 10 are modeled only for a single year (or equivalently for the last two six-month periods).
Since incidence refers to the share of infected plants per field, it can also be interpreted as the share of production lost from each field (we assume that an infected plant does not produce). Because of the homogeneity assumption for fields (the same number of plants–similar yields), the final yield loss at country level at time t was calculated as the total incidence (or number of infected plants) across all infected fields as follows:
Where i = 1, 2,…, t denotes the 6-month period in which BXW infections first appeared (BXW can appear at t = 1, or in any other period up to t = 20). M is the total number of 50 km2 banana fields in the country, and m1 is the number of fields that were infected in period 1, whose incidence in period t is z1,t. The remaining terms in the nominator express the number of fields infected at different moments in time (mi) whose incidence level at t is given by zi,t. Each term thus represents the current stage (at time t) of BXW infection across different banana fields, and the resulting share of production lost from these fields.
We first estimate incidence with the BXW model in a hypothetical 50 km2 field to demonstrate how incidence rates evolve over the 10-year period depending on the selected scenario of policy response (i.e., we assume that BXW first appears or resurges in the hypothetical field in the first 6-month period of year 1). As shown in Figure 3A, the “zero” response scenario leads to a fluctuating but strong upward trend in incidence rates, stemming from the assumption that farmers never fully adopt the required management practices (the FA regime does not appear in the sequence). As a result, incidence exceeds 60% by year 10 of the simulated period. A S&L response also leads to BXW incidence rates that fluctuate substantially, but it exhibits a weaker upward trend compared to the “zero” scenario. Under the S&L scenario, incidence reaches 5% in year 2, but stakeholder intervention brings incidence down to almost 3% in year 3. However, since the interventions are quickly phased out, the disease resurges with incidence exceeding 10% by year 6. Some measures are then reintroduced, and incidence declines once more, albeit briefly. The cycle of fluctuations repeats and maximum incidence during the simulated period reaches 20% at year 9. Contrary to the above, incidence under the Q&E scenario remains below 2% and decreases gradually over time until BXW is practically eliminated from the field.
Figure 3. Results of the BXW spread model. (A) BXW incidence rates (share of infected plants in a 50 km2 field) over time and per scenario of response to BXW infections. (B) Average yield loss to BXW across Sub-Saharan Africa over time and per scenario. Q&E, quick and effective response; S&L, slow and limited response.
Banana yield losses over time (i.e., the share of infected plants at national level) are calculated using equation (1) by combining the above incidence rates with the prevalence assumptions of the scenario examined. Results across countries in each scenario differ slightly, depending on the count of banana fields (M). More specifically, since we assume the disease to be present in at least one field, a low field count (M < 20) increases the starting level of prevalence, which leads to higher initial yield losses compared to countries with a higher field count.3 On average for the entire SSA region, yield losses estimated with the BXW model can reach to 47% without any stakeholder intervention (zero response) and around 12% under a S&L scenario (Figure 3B). Because of the assumed very low prevalence in the Q&E scenario, and the equally low incidence solved by the BXW model, yield losses are slightly above zero. Since prevalence increases linearly across scenarios, at least for countries with a high field count, fluctuations that were previously observed for incidence are now smoothed out.
The country-level yield losses from the BXW model are introduced as productivity shocks in IMPACT, which calculates the new domestic market equilibrium in each country, along with changes in total banana production and cultivated areas in the region. Figure 4A reveals that banana areas are expected to decrease substantially as the result of BXW infections, to a maximum of 15% by year 10 under a “zero” response scenario. Overall, without any stakeholder intervention, banana production in SSA is projected to drop by about 55% due to both, yield losses and area contraction (Figure 4B). The area decrease for the S&L scenario is comparatively much smaller, reaching 3% at year 10. This leads to an aggregate production loss of around 15% during the same year. On the contrary, the Q&E scenario exhibits almost zero decrease in areas and supply (0.02 and 0.23%, respectively by year 10), owing to the assumption of maximum 20% prevalence and the resulting very low yield losses. These results clearly reveal that the impacts of BXW on banana production can be catastrophic for local banana producers when no efforts are made to control the disease (i.e., under the “zero” response scenario); the decrease in supply appears to be almost four times greater compared to even the S&L response scenario which assumes limited and not sustained stakeholder intervention.
Figure 4. Changes in aggregate banana production in Sub-Saharan Africa per scenario of response to BXW infections. (A) Areas. (B) Production. Q&E, quick and effective response; S&L, slow and limited response.
We note that, since crop supply in IMPACT is modeled as the product of areas and yields, which are model variables themselves, the productivity shock from the BXW model leads to endogenous adjustments for both areas and yields in IMPACT. In other words, the original yield losses, which are estimated by combining incidence from the BXW model and the prevalence assumptions of the scenario, are further adjusted when determining the new market equilibrium. This means that the area and production changes in Figure 4 correspond to the final adjusted yields generated by IMPACT and differ from the originally calculated losses with the BXW model (in Figure 3B). The discrepancies between the two values in each country depend on how the productivity shock affects local banana production, consumption, and prices (the “price effect”). They are generally small, but they tend to increase as we move toward scenarios that posit higher yield shocks, thus greater price effects. Supplementary Table S8 presents how the two values differ.
In SSA on average, prices are projected to increase by as much as 16% under a “zero” scenario (Figure 5C) and by 4% under a S&L scenario (Figure 5B). Because of the varying importance of banana as a food crop in SSA, the increase in prices can differ substantially among affected countries. For what follows we distinguish between two country groups according to the share of daily calories from banana, as reported in FAOSTAT for the period 2011–2020; the first group includes countries where banana contributes more than 10% to daily calorie intake per capita (high consumption countries-HiCons), whereas daily calorie intake for the second group is below the 10% threshold (low consumption countries–LoCons).4 Lower supply will have a greater impact on banana prices for the HiCons country group, which are projected to increase by almost 21% under the “zero” scenario (Figure 5C) and by 5.5% under the S&L scenario (Figure 5B). The price increase in LoCons countries is much smaller, reaching 13% and 3.5% for the “zero” and S&L scenarios respectively. Finally, banana prices under a Q&E response to BXW do not increase more than 0.1% on average for the entire SSA region (Figure 5A). In addition, since infections and yield losses in the Q&E scenario tend to stabilize after an initial surge, their marginal impact on prices also decreases over time, although small differences between HiConS&LoCons countries do exist.
Figure 5. Change in domestic consumer banana prices, per country group and scenario of response to BXW infections. (A) Quick and effective–Q&E–scenario. (B) Slow and limited response–S&L–scenario. (C) Zero response scenario. SSA, Sub-Saharan Africa; HiCons, countries where banana contributes more than 10% to daily calorie intake per capita; LoCons, countries where banana contributes <10% to daily calorie intake per capita.
The decrease in household food demand for banana generally mirrors the change in prices presented above but it is numerically smaller in percentage terms. Overall, HiCons countries are expected to reduce food consumption of banana more than LoCons countries under all scenarios. This decrease is more pronounced under the “zero” scenario. Due to space limitations, changes in banana demand are illustrated in Supplementary Figure S2. Given the high production losses presented in Figure 4B, the relatively small impact on demand suggests that an increase in imports from outside SSA will be needed to reach an equilibrium, particularly for the “zero” scenario. We note that trade in IMPACT acts as an equilibrating mechanism for global markets (global net trade is equal to zero at the final solution), but bilateral trade is not modeled explicitly. Since official statistics show that most of banana trade in SSA happens between countries within the region (FAO, 2023), we attempted to introduce import constraints to represent the inability of affected countries to engage in trade with one another. The reasoning is that BXW-induced production shocks would reduce their capacity to export the commodity. However, we were unable to solve the model because of the magnitude of these yield losses which cause major disruptions to domestic markets, as they are represented in IMPACT. For this reason, we did not examine further the impacts of BXW on regional banana trade.
The reduced demand and the change in relative commodity prices also affect the contribution of banana to peoples' diets and the overall intake of calories, which are used herein as food security indicators (Table 1). By the final year of the simulated period, the share of calories from banana for all SSA is reduced by 0.19% under the “zero” scenario and by 0.07% for the S&L scenario. As expected, households in HiCons countries are more affected since this decrease reaches 0.78% and 0.22% for the two scenarios, respectively. A reduction of almost similar magnitude is also projected for the total daily calorie intake per capita across all scenarios. However, the decrease in total calorie intake for HiCons countries is slightly greater than the respective decrease of the banana calorie shares in all scenarios (e.g., 0.81 vs. 0.78% in the “zero” scenario), whereas the opposite is true for LoCons countries. This result suggests that any increase in the consumption of other food commodities by households in HiCons countries is not able to fully compensate for the impact of higher banana prices on their food security status in terms of their calorie intake. It further reveals that, where bananas are staple food, BXW is expected to have much larger impacts on food security, even if the projected decrease in the total intake of calories appears to be very small. Indeed, BXW spread leads to a considerable increase in the number of people at risk of hunger and of malnourished children. Under a “zero” response to BXW, the population at risk of hunger by year 10 is projected to increase by 1.69% in SSA compared to the baseline, whereas the corresponding value for the S&L scenario is only 0.47%. The increase in the number of people at risk of hunger for HiCons countries is even higher, reaching 4.68% under a “zero” scenario. The food security impacts under the Q&E scenario are practically absent for all SSA countries.
Table 1. Change of food security indicators at year 10, per country group and scenario of response to BXW infections (Q&E, quick and effective response; S&L, slow and limited response; HiCons, countries where banana contributes more than 10% to daily calorie intake per capita; LoCons, countries where banana contributes <10% to daily calorie intake per capita).
Overall, BXW infections over the simulated 10-year period are expected to have a negative aggregate economic impact, which we measure herein as the sum of consumer and producer surpluses. Without any response to BXW (“zero” scenario), the total economic damage for this period can surpass 25 billion USD for the entire SSA region (Figure 6C). The economic damage for the S&L scenario is lower, at about 7 billion USD (Figure 6B). Although the yield losses from BXW infections are very small under the Q&E scenario, they are still enough to incur a total economic damage of around 250 million USD over 10 years (Figure 6A).
Figure 6. Change in consumer and producer surplus per country group and scenario of response to BXW infections, in constant 2005 prices. (A) Quick and effective–Q&E–scenario. (B) Slow and limited response–S&L–scenario. (C) Zero response scenario. SSA, Sub-Saharan Africa; HiCons, countries where banana contributes more than 10% to daily calorie intake per capita; LoCons, countries where banana contributes <10% to daily calorie intake per capita.
The distribution of economic losses among producers and consumers varies substantially among scenarios and country groups. As yield losses from BXW increase while moving to scenarios with more limited response to the disease, price growth becomes proportionally smaller than the reduction in banana supply. As a consequence, a larger share of economic losses is borne by producers in the “zero” scenario, and by consumers in the Q&L scenario. This result is more pronounced for HiCons countries, particularly under a Q&E scenario where the increase in prices almost fully compensates producers for any supply losses to the disease. Under all scenarios, producers in LoCons countries appear to suffer heavier economic losses than consumers since banana plays only a small role in peoples' diets.
In this paper we examine the potential impacts of BXW spread across the SSA region using a multidisciplinary approach that combines a field-level BXW spread model with the IMPACT global partial equilibrium model. The analysis covers a 10-year period, and it is based on scenarios specified around the effectiveness of policy response to BXW. The methodological framework presented in this study attempts to address several modeling gaps in the literature. Specifically, it links an equilibrium model with a disease spread model and accounts for the role of management in determining the potential impacts of disease spread. The specification of the scenarios as sequences of management regimes determines the parametrization of the disease model and provides a direct connection between management and model-based spread dynamics. These dynamics are incorporated in IMPACT through a series of steps, starting with the simulation of incidence with the BXW model, whose results are then upscaled at national level according to the assumed prevalence rates of the scenarios.
Results corroborate qualitative conjectures, indicating that if BXW is left uncontrolled (as simulated by the “zero” response scenario), there can be serious impacts on banana production systems in SSA. Within 10 years the disease can cause around 55% reduction in banana supply in the region, leading to an average domestic price increase of more than 16% compared to a baseline (BXW-free) scenario, and causing economic damage of more than 25 billion USD. The analysis further reveals that the economic impacts of an BXW epidemic are not limited to farmers' fields but are expected to affect both, consumers, and producers. Impacts on food security, measured in terms of calories and population at risk of hunger, generally follow the production-side results, but they are contingent on the role of banana in peoples' diets. Countries wherein banana is a staple food are expected to experience a relatively larger deterioration to their food security status compared to the SSA average, particularly under a “zero” policy response. The increase of domestic banana prices also modifies the relative prices for food commodities, and forces consumers to change the composition of their commodity food bundle. Given the available income and food preferences, the latter interpreted as the contribution of banana to the food bundle, these changes lead to an increase in the share of the population at risk of hunger.
Results also show that even a minimum, and non-sustained mobilization of stakeholders (the S&L scenario) can temporarily reduce BXW infections and mitigate some of the negative impacts in the short term. However, this type of response is not sustainable in the long run since infections continue to increase but at a much slower rate compared to “zero” policy response scenario. Ideally, a rapid, effective, concerted, and sustained response to BXW can prevent its spread and concomitant large yield losses. As shown with the Q&E scenario of stakeholder response to BXW, the impacts of the disease in SSA can be almost fully mitigated by proactively raising awareness and knowledge on disease diagnosis, transmission, and promoting the adoption of the appropriate management practices by farmers (Ocimati et al., 2019). For this purpose, communication and education campaigns, trainings, and meetings are needed (Gotor et al., 2020). Avoiding the disease transmission to the new territories must be prevented by coordinated actions at the regional level. Alarmingly, ineffective interaction and collaboration between stakeholders has been already identified as a limiting factor in efforts to control BXW spread in endemic ECA countries (McCampbell et al., 2018). A policy framework for effective BXW control in Africa is needed which integrates crop hygiene, quarantine, and surveillance measures, while promoting user-friendly, low-cost BXW management that includes training in, and use of SDSR. The framework should be designed drawing on the lessons learned from the well documented Uganda experience, and it should accommodate the contextual nuances of each country, in terms of levels of BXW, and agro-ecological and socioeconomic particularities.
Since every model integrates many key assumptions, drawing conclusions on model projections must always consider the related caveats. These caveats can help identify the required modeling or data improvements that will enable researchers to better understand the economic and food-system consequences of BXW spread. Starting from the economic representation of the agricultural sector, this study takes a macro-level view of the potential BXW impacts in SSA, relying on IMPACT to simulate potential changes in banana supply, demand, and prices. The underlying assumption of IMPACT (and every similar model thereof) is the equilibrium state which clears commodity markets at global and national level (supply equals demand). However, rural households in SSA often participate in subsistence agriculture and consume home-grown crops, so they may be less responsive to prices, or engage less in trade than what is assumed by the model.
The role of trade to compensate for production losses has not been examined in this paper because of the large yield losses that did not allow the model to solve with meaningful import constraints. While a small increase in imports for the Q&E and S&L scenarios may not be unreasonable given the role of banana in SSA as a staple food, the very large increase in imports implied for the “zero” scenario seems questionable because of the fragile nature of the crop and the small share of world production that is currently traded. Nevertheless, we remind that the “zero” scenario is an extreme case that posits stakeholder inaction to BXW infections and eventually leads to large yield losses. According to Godfray et al. (2016), it is even possible that such supply shocks will not result in a market equilibrium in the short term, and therefore they cannot be adequately simulated by equilibrium models.
To assess the economic impacts of BXW infections, we have calculated changes in consumer and producer surplus within a partial equilibrium framework as a proxy for economic damage. However, proper welfare analysis requires a general equilibrium approach that examines how shocks in banana markets can be transmitted to other sectors of the economy. IMPACT has been linked in the past to general equilibrium models for the calculation of changes in producer and consumer surpluses, and consequently of aggregate welfare losses—or gains—in affected countries and beyond (Mason-D'Croz et al., 2019). Such synthesis should be able to provide more insights to the economic consequences of a BXW outbreak and could be explored in future work.
Costs related to stakeholder mobilization (e.g., the awareness-raising campaigns) and to the adoption of the disease control practices by farmers are integral part of any welfare analysis but they have not been explicitly considered in this study. On the contrary, we assume that these costs are exogenous to the analysis and that they affect neither how different SSA countries respond to BXW, nor farmers' decision to adopt the recommended BXW control practices. Both choices are predefined by the scenario narrative and are expressed through the specification of the sequence of management regimes. Endogenizing such choices is beyond the scope of the current modeling exercise, as it requires economic models operating at lower geographical scales (micro-level). For example, the decision by farmers to adopt BXW control measures constitutes a self-selection problem that is usually handled by optimization models at the farm/household level, with profit/income maximization often being the decision criterion (Petsakos et al., 2023). Combining IMPACT with more disaggregated models can enhance our understanding about how policy influences farmer decisions and, eventually, disease spread (i.e., the prevalence dimension). It would also address one the shortcomings of the current analysis which is the homogeneity of the 50 km2 production areas that were used for specifying the prevalence dimension of the scenarios. Currently, the reality on the ground (the specificities of individual farms and their production systems in these areas) is not explicitly modeled. Instead, it is assumed that the underlying spatial heterogeneity is captured in the aggregate representation of the agricultural sector in IMPACT. For all the above reasons, combining modeling approaches operating across different scales should be examined as a potential future improvement to the modeling framework. However, such improvement is contingent on data availability at the required level of granularity.
As regards the biophysical aspect of BXW modeling, the projections are based on regional BXW epidemiological knowledge and management expertise, combined with data and experience gained over almost two decades. The results are therefore conditional on the specification of the scenarios as sequences of management regimes and on the parameterization of the BXW model. The scenarios themselves correspond to an attempt to summarize the experience gained from BXW management in ECA for the purpose of examining the potential impacts of the disease resurging and spreading elsewhere in SSA. As such, there is substantial subjectivity in defining some of the scenario dimensions, particularly prevalence, which stems from (i) the lack of a spatially explicit BXW model; (ii) the uncertainty surrounding the type of stakeholder response; and (iii) the overall dynamics of a possible BXW outbreak in SSA. For this reason, the results presented in this study correspond to a “what if” type of analysis and not to an impact assessment per se. However, since we have assumed a uniform implementation of the scenarios across all countries (i.e., all countries will exhibit similar response in every scenario), results could also be seen as an envelope of possible consequences of BXW infections, ranging from a highly optimistic pathway (the Q&E scenario) to an extremely pessimistic one (the “zero” scenario).
Crop-disease interactions are modeled indirectly by invoking the simple assumption that once a banana plant is infected it no longer produces. This assumption circumvents the lack of an appropriate model that can estimate yield losses to BXW. Modeling losses to pests and diseases, particularly under climate change, is in fact a contemporary issue in the literature which has received a lot of attention from crop modelers and pathologists alike (Chakraborty and Newton, 2011; Newbery et al., 2016), but progress toward crop-disease modeling has been very slow (Donatelli et al., 2017). For this reason, although IMPACT is a foresight tool that incorporates assumptions of climate change, the BXW model runs independently without considering the impact of climate on either plantation growth or the spread of the disease. Considering all the above constraints, the BXW spread model used herein provides a sufficient solution to the estimation of field-level incidence and subsequently to the parameterization of yield losses in an economic model functioning at higher geographical scales.
The modeling framework could further improve by distinguishing between cultivars and different uses of banana and plantain across SSA countries. This could reveal additional heterogeneity of the results at different scales and increase the analytical capacity of the approach. The lack of appropriate data in terms of quality and quantity for such improvement is an important limitation for the work presented in this paper, such that the authors relied extensively on expert knowledge to parameterize the BXW model according to the various scenarios examined.
Finally, a word of caution is needed regarding the generalization of this work. The modeling framework proposed in this paper focused on the banana crop and on the BXW disease. The existence of a BXW spread model, accompanied by assumptions on yield loss, played a key role in this research, but the rationale underpinning the approach can also be applied to other pathosystems where similar models are available. Although we acknowledge that appropriate models obviously do not exist for all pathosystems, one of the objectives of this research was to highlight the role of management and to offer a link between policy decisions, farmers' choices and disease spread in the form of response scenarios. We believe that it represents a first step toward a more comprehensive framework of analysis that uses inputs and insights from multiple disciplines and aims to understand the complex dynamics of disease spread and its economic and food security consequences at different scales.
Data supporting the results and conclusions presented in this study are included in the article and Supplementary material, further inquiries can be directed to the corresponding author.
AP and JN performed the model simulations. AP, MK, and GB analyzed the results. AP and MK wrote the first draft of the manuscript. GB, JN, WO, and EG wrote sections of the manuscript. All authors contributed to conception and design of the study, manuscript revision, read, and approved the submitted version.
Funding support for this study was provided by the CGIAR Initiative on Foresight and Metrics to Accelerate Food, Land, and Water Systems Transformation (Foresight & Metrics Initiative) and the CGIAR Initiative on Plant Health and Rapid Response to Protect Food Security and Livelihoods (Plant Health Initiative). We would also like to thank all funders who have supported this research through their contributions to the CGIAR Trust Fund: www.cgiar.org/funders/.
AP, GB, WO, and EG were employed by Bioversity International.
The remaining authors declare that the research was conducted in the absence of any commercial or financial relationships that could be construed as a potential conflict of interest.
The handling editor and the reviewer GM declared a shared research partnership group CGIAR with the authors AP, GB, WO, and EG at the time of review.
All claims expressed in this article are solely those of the authors and do not necessarily represent those of their affiliated organizations, or those of the publisher, the editors and the reviewers. Any product that may be evaluated in this article, or claim that may be made by its manufacturer, is not guaranteed or endorsed by the publisher.
The Supplementary Material for this article can be found online at: https://www.frontiersin.org/articles/10.3389/fsufs.2023.1207913/full#supplementary-material
1. ^Hereafter we will use the term “banana” to refer to both banana and plantain crops, as they are reported by FAO.
2. ^Results from the BXW model are independent of the size of the banana field. Field size is already considered through the parameter expressing the carrying capacity of the plantation (number of stems in the plantation).
3. ^This point is explained in more detail in Supplementary Section 4.
4. ^The countries where the share of daily calories from banana exceeded 10% are Uganda, Rwanda, Ghana, and Gabon (FAO, 2023).
Beach, R. H., Sulser, T. B., Crimmins, A., Cenacchi, N., Cole, J., Fukagawa, N. K., et al. (2019). Combining the effects of increased atmospheric carbon dioxide on protein, iron, and zinc availability and projected climate change on global diets: a modelling study. Lancet Planet. Health 3, e307–e317. doi: 10.1016/S2542-5196(19)30094-4
Blomme, G., Dita, M., Jacobsen, K. S., Vicente, L. P., Molina, A., Ocimati, W., et al. (2017). Bacterial diseases of bananas and enset: current state of knowledge and integrated approaches toward sustainable management. Front. Plant Sci. 8, 1290. doi: 10.3389/fpls.2017.01290
Blomme, G., Jacobsen, K., Ocimati, W., Beed, F., Ntamwira, J., Sivirihauma, C., et al. (2014). Fine-tuning banana Xanthomonas wilt control options over the past decade in East and Central Africa. Eur. J. Plant Pathol. 139, 271–287. doi: 10.1007/s10658-014-0402-0
Chakraborty, S., and Newton, A. C. (2011). Climate change, plant diseases and food security: an overview. Plant Pathol. 60, 2–14. doi: 10.1111/j.1365-3059.2010.02411.x
Cunniffe, N. J., Koskella, B., Metcalf, C. J. E., Parnell, S., Gottwald, T. R., and Gilligan, C. A. (2015). Thirteen challenges in modelling plant diseases. Epidemics 10, 6–10. doi: 10.1016/j.epidem.2014.06.002
Dato, K. M. G., Dégbègni, M. R., Atchad,é, M. N., Zandjanakou Tachin, M., Hounkonnou, M. N., and Aman Omondi, B. (2021). Spatial parameters associated with the risk of banana bunchy top disease in smallholder systems. PLoS ONE 16, e0260976. doi: 10.1371/journal.pone.0260976
Donatelli, M., Magarey, R. D., Bregaglio, S., Willocquet, L., Whish, J. P. M., and Savary, S. (2017). Modelling the impacts of pests and diseases on agricultural systems. Agric. Syst. 155, 213–224. doi: 10.1016/j.agsy.2017.01.019
Enahoro, D., Mason-D'Croz, D., Mul, M., Rich, K. M., Robinson, T. P., Thornton, P., et al. (2019). Supporting sustainable expansion of livestock production in South Asia and Sub-Saharan Africa: scenario analysis of investment options. Glob. Food Sec. 20, 114–121. doi: 10.1016/j.gfs.2019.01.001
FAO (2023). FAOSTAT statistical database. Food and Agriculture Organization of the United Nations (FAO). Available online at: https://www.fao.org/faostat/en/#home (accessed January 12, 2023).
Fuglie, K., Wiebe, K., Sulser, T. B., Cenacchi, N., and Willenbockel, D. (2022). Multidimensional impacts from international agricultural research: Implications for research priorities. Front. Sustain. Food. Syst. 6. doi: 10.3389/fsufs.2022.1031562
Geberewold, A. Z. (2019). Review on impact of banana bacterial wilt (Xhantomonas campestris pv. Musacerum) in East and Central Africa. Cogent. Food Agric. 5, 1586075. doi: 10.1080/23311932.2019.1586075
Godfray, H. C. J., Mason-D'Croz, D., and Robinson, S. (2016). Food system consequences of a fungal disease epidemic in a major crop. Philos. Trans. R Soc. Lond. B. Biol. Sci. 371, 20150467. doi: 10.1098/rstb.2015.0467
Gotor, E., di Cori, V., Pagnani, T., Kikulwe, E., Kozicka, M., and Caracciolo, F. (2020). Public and private investments for banana Xanthomonas Wilt control in Uganda: the economic feasibility for smallholder farmers. Afr. J. Sci. Tech. Innov. Dev. 14, 1–12. doi: 10.1080/20421338.2020.1816616
Islam, S., Cenacchi, N., Sulser, T. B., Gbegbelegbe, S., Hareau, G., Kleinwechter, U., et al. (2016). Structural approaches to modeling the impact of climate change and adaptation technologies on crop yields and food security. Glob. Food Sec. 10, 63–70. doi: 10.1016/j.gfs.2016.08.003
Kalyebara, M. R., Ragama, P. E., Kagezi, G. H., Kubiriba, J., Bagamba, F., Nankinga, K. C., et al. (2006). Economic importance of the banana bacterial wilt in Uganda. Afr. Crop. Sci. J. 14, 93–102. doi: 10.4314/acsj.v14i2.27915
Keeling, M. J., and Rohani, P. (2011). Modeling infectious diseases in humans and animals. Princeton, New Jersey: Princeton University Press. doi: 10.2307/j.ctvcm4gk0
Kikulwe, E., Asindu, M., Ocimati, W., Ajambo, S., Tinzaara, W., Iradukunda, F., et al. (2022). “Scaling banana bacterial wilt management through single diseased stem removal in the great lakes region,” in Root, Tuber and Banana Food System Innovations, eds. G. Thiele, M. Friedmann, H. Campos, V. Polar, and J. W. Bentley (Cham: Springer International Publishing), 289–317. doi: 10.1007/978-3-030-92022-7_10
Kruseman, G., Mottaleb, K. A., Tesfaye, K., Bairagi, S., Robertson, R., Mandiaye, D., et al. (2020). Rural transformation and the future of cereal-based agri-food systems. Glob. Food Sec. 26, 100441. doi: 10.1016/j.gfs.2020.100441
Kubiriba, J., and Tushemereirwe, W. K. (2014). Approaches for the control of banana Xanthomonas wilt in East and Central Africa. Afr. J. Plant Sci. 8, 398–404. doi: 10.5897/AJPS2013.1106
Li, G. H., and Zhang, Y. X. (2017). Dynamic behaviors of a modified SIR model in epidemic diseases using nonlinear incidence and recovery rates. PLoS ONE 12, e0175789. doi: 10.1371/journal.pone.0175789
Mason-D'Croz, D., Bogard, J. R., Herrero, M., Robinson, S., Sulser, T. B., Wiebe, K., et al. (2020). Modelling the global economic consequences of a major African swine fever outbreak in China. Nat. Food 1, 221–228. doi: 10.1038/s43016-020-0057-2
Mason-D'Croz, D., Sulser, T. B., Wiebe, K., Rosegrant, M. W., Lowder, S. K., Nin-Pratt, A., et al. (2019). Agricultural investments and hunger in Africa modeling potential contributions to SDG2 - Zero Hunger. World Dev. 116, 38–53. doi: 10.1016/j.worlddev.2018.12.006
Mbabazi, E. G., Kikulwe, E. M., Kyanjo, J. L., Mulumba, N., Kato, E., and Gotor, E. (2021). Has continued exposure to banana Xanthomonas wilt worsened farmers' welfare over time? Evidence from banana-producing households in Uganda. J. Agric. Sci. 13, 11–22. doi: 10.5539/jas.v13n11p11
McCampbell, M., Schut, M., van den Bergh, I., van Schagen, B., Vanlauwe, B., Blomme, G., et al. (2018). Xanthomonas Wilt of Banana (BBXW) in Central Africa: opportunities, challenges, and pathways for citizen science and ICT-based control and prevention strategies. NJAS-Wageningen J. Life Sci. 86–87, 89–100. doi: 10.1016/j.njas.2018.03.002
Nakakawa, J., Mugisha, J. Y. T., Shaw, M. W., and Karamura, E. (2016). A mathematical model for the dynamics of banana xanthomonas wilt with vertical transmission and inflorescence infection. J. Biol. Syst. 24, 147–165. doi: 10.1142/S021833901650008X
Ndayihanzamaso, P., Niko, N., Niyongere, C., Bizimana, S., Nibasumba, A., Lepoint, P., et al. (2016). Distribution, incidence and farmers knowledge of banana Xanthomonas wilt in Burundi. Afr. J. Agric. Res. 11, 3615–3621. doi: 10.5897/AJAR2016.11210
Ndungo, V., Fiaboe, K. K. M., and Mwangi, M. (2008). Banana Xanthomonas wilt in the DR Congo: impact, spread and management. J. Appl. Biosci. 1, 1–7.
Nelson, G. C., Valin, H., Sands, R. D., Havlík, P., Ahammad, H., Deryng, D., et al. (2014). Climate change effects on agriculture: economic responses to biophysical shocks. Proc. Natl. Acad. Sci. U S A. 111, 3274–3279. doi: 10.1073/pnas.1222465110
Newbery, F., Qi, A., and Fitt, B. D. L. (2016). Modelling impacts of climate change on arable crop diseases: progress, challenges and applications. Curr. Opin. Plant. Biol. 32, 101–109. doi: 10.1016/j.pbi.2016.07.002
Nkuba, J., Tinzaara, W., Night, G., Niko, N., Jogo, W., Ndyetabula, I., et al. (2015). Adverse impact of Banana Xanthomonas Wilt on farmers livelihoods in Eastern and Central Africa. Afr. J. Plant Sci. 9, 279–286. doi: 10.5897/AJPS2015.1292
Ocimati, W., Bouwmeester, H., Groot, J. C. J., Tittonell, P., Brown, D., and Blomme, G. (2019). The risk posed by Xanthomonas wilt disease of banana: Mapping of disease hotspots, fronts and vulnerable landscapes. PLoS ONE 14, e0213691. doi: 10.1371/journal.pone.0213691
Ocimati, W., Groot, J. J. C., Tittonell, P., Taulya, G., Ntamwira, J., Amato, S., et al. (2020). Xanthomonas wilt of banana drives changes in land-use and ecosystem services across infected landscapes. Sustainability 12, 3178. doi: 10.3390/su12083178
Ocimati, W., Ssekiwoko, F., Karamura, E., Tinzaara, W., Eden-Green, S., and Blomme, G. (2013). Systemicity of xanthomonas campestris pv. musacearum and time to disease expression after inflorescence infection in east african highland and pisang awak bananas in uganda. Plant Pathol. 62, 777–785. doi: 10.1111/j.1365-3059.2012.02697.x
Oerke, E.-C. (2006). Crop losses to pests. J. Agric. Sci. 144, 31–43. doi: 10.1017/S0021859605005708
Petsakos, A., Ciaian, P., Espinosa, M., Perni, A., and Kremmydas, D. (2023). Farm-level impacts of the CAP post-2020 reform: a scenario-based analysis. Appl. Econ. Perspect. Policy 45, 1168–1188. doi: 10.1002/aepp.13257
Petsakos, A., Hareau, G., Kleinwechter, U., Wiebe, K., and Sulser, T. B. (2018). Comparing modeling approaches for assessing priorities in international agricultural research. Res. Eval. 27, 145–156. doi: 10.1093/reseval/rvx044
Petsakos, A., Prager, S. D., Gonzalez, C. E., Gama, A. C., Sulser, T. B., Gbegbelegbe, S., et al. (2019). Understanding the consequences of changes in the production frontiers for roots, tubers and bananas. Glob. Food Sec. 20, 180–188. doi: 10.1016/j.gfs.2018.12.005
Robinson, S., Mason-D'Croz, D., Sulser, T., Islam, S., Robertson, R., Zhu, T., et al. (2015). The International Model for Policy Analysis of Agricultural Commodities and Trade (IMPACT): model description for version 3. Washington DC. Available online at: https://ebrary.ifpri.org/utils/getfile/collection/p15738coll2/id/129825/filename/130036.pdf (accessed January 16, 2023).
Savary, S., Willocquet, L., Pethybridge, S. J., Esker, P., McRoberts, N., and Nelson, A. (2019). The global burden of pathogens and pests on major food crops. Nat. Ecol. Evol. 3, 430–439. doi: 10.1038/s41559-018-0793-y
Sulser, T. B., Beach, R. H., Wiebe, K. D., Dunston, S., and Fukagawa, N. K. (2021). Disability-adjusted life years due to chronic and hidden hunger under food system evolution with climate change and adaptation to 2050. Am. J. Clin. Nutr. 114, 550–563. doi: 10.1093/ajcn/nqab101
Tinzaara, W., Karamura, E. B., Blomme, G., Jogo, W., Ocimati, W., Rietveld, A., et al. (2013). Why sustainable management of Xanthomonas Wilt of banana in East and Central Africa has been elusive. Acta Hortic. 986, 157–164. doi: 10.17660/ActaHortic.2013.986.16
Tinzaara, W., Stoian, D., Ocimati, W., Kikulwe, E., Otieno, G., and Blomme, G. (2018). “Challenges and opportunities for smallholders in banana value chains,” in Achieving sustainable cultivation of bananas, eds. G. Kema and A. Drenth (London: Burleigh Dodds Science Publishing), 65–90. doi: 10.19103/AS.2017.0020.10
Tushemereirwe, W. K., Okaasai, O. O., Kubiriba, J., Nankinga, C., Muhangi, J., Odoi, N., et al. (2006). Status of banana bacterial wilt in Uganda. Afr. Crop Sci. J. 14, 73–82. doi: 10.4314/acsj.v14i2.27913
van den Driessche, P. (2017). Reproduction numbers of infectious disease models. Infect. Dis. Model. 2, 288–303. doi: 10.1016/j.idm.2017.06.002
Vurro, M., Bonciani, B., and Vannacci, G. (2010). Emerging infectious diseases of crop plants in developing countries: impact on agriculture and socio-economic consequences. Food Secur. 2, 113–132. doi: 10.1007/s12571-010-0062-7
Wiebe, K., Lotze-Campen, H., Sands, R., Tabeau, A., van der Mensbrugghe, D., Biewald, A., et al. (2015). Climate change impacts on agriculture in 2050 under a range of plausible socioeconomic and emissions scenarios. Environ. Res. Let. 10, 085010. doi: 10.1088/1748-9326/10/8/085010
Yirgou, D., and Bradbury, J. (1968). Bacterial wilt of enset (Ensete ventricosum) incited by Xanthomonas musacearum sp. n. Phytopathology 58, 111–112.
Keywords: Africa, banana, disease model, economic model, food security, policy response, Xanthomonas wilt of banana
Citation: Petsakos A, Kozicka M, Blomme G, Nakakawa JN, Ocimati W and Gotor E (2023) The potential impact of banana Xanthomonas wilt on food systems in Africa: modeling scenarios of policy response and disease control measures. Front. Sustain. Food Syst. 7:1207913. doi: 10.3389/fsufs.2023.1207913
Received: 18 April 2023; Accepted: 24 July 2023;
Published: 14 August 2023.
Edited by:
Jaindra Nath Tripathi, International Institute of Tropical Agriculture (IITA), KenyaReviewed by:
Amadou Sidibé, Agriculture and Agri-Food Canada (AAFC), CanadaCopyright © 2023 Petsakos, Kozicka, Blomme, Nakakawa, Ocimati and Gotor. This is an open-access article distributed under the terms of the Creative Commons Attribution License (CC BY). The use, distribution or reproduction in other forums is permitted, provided the original author(s) and the copyright owner(s) are credited and that the original publication in this journal is cited, in accordance with accepted academic practice. No use, distribution or reproduction is permitted which does not comply with these terms.
*Correspondence: Athanasios Petsakos, YS5wZXRzYWtvc0BjZ2lhci5vcmc=
Disclaimer: All claims expressed in this article are solely those of the authors and do not necessarily represent those of their affiliated organizations, or those of the publisher, the editors and the reviewers. Any product that may be evaluated in this article or claim that may be made by its manufacturer is not guaranteed or endorsed by the publisher.
Research integrity at Frontiers
Learn more about the work of our research integrity team to safeguard the quality of each article we publish.