- 1Department of Atmospheric and Climate Science, University of Energy and Natural Resources, Sunyani, Ghana
- 2African Institute for Mathematical Sciences, Cape Coast, Ghana
- 3Ghana Meteorological Agency, Accra, Ghana
- 4Climate System Analysis Group, University of Cape Town, Cape Town, South Africa
- 5Institute for Climate and Atmospheric Science, University of Leeds, Leeds, United Kingdom
Rainfall onset date (ROD) influences farmer planting decisions, yet there is a dearth of information on the extent to which ROD influences crop yield. This study assesses the effect of ROD on the yield of four crops (Maize, millet, rice, and sorghum) in Ghana. It uses crop yields from the Ministry of Food and Agriculture (MoFA) and the Food and Agriculture Organization (FAO), and employs the Decision Support System for Agro-technology Transfer (DSSAT) crop model to simulate maize yields from 1985 to 2004. The crop model simulations were forced with weather data from the gridded Global Meteorological Forcing Dataset (GMFD). The relationship between crop yields and RODs from three datasets (observed, satellite, and GMFD) are studied. The results of the study show a good correlation between MoFA and FAO crop yield data (with correlation coefficient (r) of 0.97, 0.92, 0.77, and 0.99 for maize, millet, rice, and sorghum, respectively). RODs from satellite observation feature a high correlation with RODs from station observation (r = 0.72), but RODs from GMFD feature weak correlations (r < 0.3) with both observation datasets. The study finds a negative correlation between observed RODs and crop yields (i.e. an early onset corresponds to high yields) but a positive correlation between GMFD RODs and crop yields (i.e. an early onset correspondence to low yields). The DSSAT model reproduces the observed yield pattern, but with substantial biases. The findings of this study can be used to advise small-holder farmers on planting dates and crop variety selection.
1. Introduction
Rainfed agriculture is the main practice by many smallholder farmers across the West African sub-region. According to Limantol et al. (2016), rainfed agriculture is a widely practiced form of agriculture in Ghana, particularly among peasant farmers. It is documented that Eighty-two percent of the cropland worldwide is cultivated under rainfed conditions. The importance of rainfed agriculture varies regionally, but it is of utmost significance for sub-Saharan Africa (Laux et al., 2010). An approximation of 95% of the total cropland is managed under rainfed conditions (Wani et al., 2009). Hence, the spatial and temporal variations of crop yield may have a profound impact on the national economies of sub-Saharan countries, which are primarily dependent on the agricultural sector.
Even though soil type and agricultural management practices are important in the agricultural industry, the sector’s dependency on rainfall especially in Sub-Sahara Africa makes it more vulnerable to climate, weather and their associated indices that emerge as a result of dynamics in the climate system (Chisanga et al., 2017). The variation in climate affects various stages of development in crops ranging from sowing to maturity, and yield. Extreme weather events such floods and droughts resulting from precipitation anomalies and temperatures changes have significant impact on crop yield Blanc (2011), making the industry resort to the use of drought-tolerant and early maturing crops, together with the applications of recommended agricultural crop management practices for optimum yield in various parts of West Africa (Danso et al., 2018).
The importance of rainfall in the planning of agricultural activities from planting to harvesting cannot then be underestimated. Plant water availability strongly depends on the onset, cessation, and length of the rainy season (Laux et al., 2010). Rainfall onset is the start or beginning of the rainy season, and this event occurs once in a year. Knowledge of the onset date is important for planning farming activities across West Africa. It guides farmers in preparation of their farmlands, selection of crops or seeds, and for determining the optimal planting time (to avoid the risk of planting too early or too late). The wrong timing of the onset of the rainy season or insufficient rainfall during a season pose a major risk to crop yields and can lead to food scarcity and famine (Nicholson et al., 2000; Omotosho et al., 2000; Abiodun et al., 2008). Thus, the onset of the rainy season is the most important variable for agricultural management (e.g., Ingram et al., 2002; Ziervogel and Calder, 2003).
Understanding crop responses to possible rainfall onset dates (RODs) is useful for many reasons. For instance, in the designing of crop models for assessment of climate change impacts, it is important to test the simulation response to different onset dates to fine-tune the model for optimal yield. Previous studies commonly use only changes in seasonal or annual aggregated total rainfall (e.g., Sultan et al., 2013). Again, if certain aspects of rainfall (e.g., RODs) are particularly important to crops, this knowledge could help prioritize climate research needs toward reducing uncertainties in simulating those aspects that are most relevant (Guan et al., 2015). According to Dobor et al. (2016), planting date matters and should not be fixed in crop simulation model for various years. The insufficient knowledge of RODs, which could lead to wrong planting dates, may be considered as one of the most important factors affecting agricultural productivity in Ghana and the entire West African region. Before planting or sowing, it is vital for farmers to know whether the rains will be continuous to ensure sufficient soil moisture during planting and whether this level will be maintained or increased during the growing period to avoid total crop failure (Walter, 1967), since planting too early might lead to crop failure in case no significant rains fall within days thereafter. Conversely, planting too late may reduce valuable growing time and hinder the crop from reaching maturity (Dieng et al., 2018). However, there is still no consensus in literature about the question of how much rain over which period defines the onset of the rainy season for agro-climatological impact studies (Laux et al., 2010). The definition of Stern et al. (1981), employed in this study, is possibly the most widespread rainfall-based definition used to estimate local rainfall onset dates. Stern’s definition strongly depends on local weather conditions, soil types, and the evaporative demands of crops, cropping practices, and other factors. Laux et al. (2008) extended the Stern definition to regional usability in a case study for the Volta basin (West Africa) using a fuzzy logic approach. In addition, the authors derived trends of the rainfall onset dates and developed methodologies for predicting the onset dates on the regional scale. Again, Laux et al. (2009) made recommendations for agricultural decision support, including maps of optimal planting dates and rainfall probabilities for the Volta basin using Stern’s definition.
For proper agricultural management and planning, the planting date, which usually coincides with the rainfall onset date, is of utmost importance for crop productivity under rainfed agriculture. Consequently, the success of planting depends on the knowledge of the agricultural onset of the rainy season, especially in semiarid regions where the rainy season is limited to a few months per year. Many studies have dealt with the impact of the planting date exclusively (e.g., Baldwin and Cossar, 2009; Blanche and Linscombe, 2009; Egli and Cornelius, 2009; Garcia et al., 2009) or in combination with other management factors (e.g., Pedersen and Lauer, 2004; Soltani and Hoogenboom, 2007; Kamara et al., 2009).
The significance of the various synergies for improving the agricultural industry has warrant the various scientific disciplines to combine efforts in addressing this situation through research into climate and crop yield, best crop management practices and model development that allows the research community to consider complex problems and take informed decisions (Ahmed et al., 2013; Jones et al., 2017). Different crop models have been developed combining the climate and agricultural practices to mimic and simulate crop yield. The Decision Support System for Agrotechnology Transfer (DSSAT), a dynamic crop simulation model offers the opportunity to simulate crop growth, development and yield taking into consideration climatic factors, soil mechanisms and prescribed or simulated crop management practices (Jones et al., 2003) and has been widely used by researchers across the African continent to simulate crop performance and yield due to its functional component that allows for calibration of external drivers such as weather parameters, planting options, soil properties and other management information to appreciate how these changes affect the output (Ruiz Nogueira et al., 2001; Masika, 2016; Mourice et al., 2014; Zinyengere et al., 2015).
This study evaluates the effects of inter-annual variation of rainfall onset dates on crop yield over Ghana, and how the Decision Support System for Agro-technology Transfer version 4.7.5.0 (DSSATv4.7.5.0) model is able to replicate the impact of RODs on maize yield over some selected stations in Ghana. It is hypothesized that crops will have good yield when yearly RODs are considered before planting. The paper also evaluate how satellite and model climatic data could replicate the patterns of the observed data in crop simulation. This is very necessary for simulation of crop yield in the areas where observed data is unavailable.
2. Data and method
2.1. Data
The observed daily rainfall dataset is from the Ghana Meteorological Agency, collected over the 22 synoptic stations across the country (Figure 1). The satellite dataset is the African Rainfall Climatology version 2 (ARC2; Novella and Thiaw, 2013). ARC2 consists of daily precipitation estimation data over Africa at 0.1° × 0.1° horizontal grid resolution.
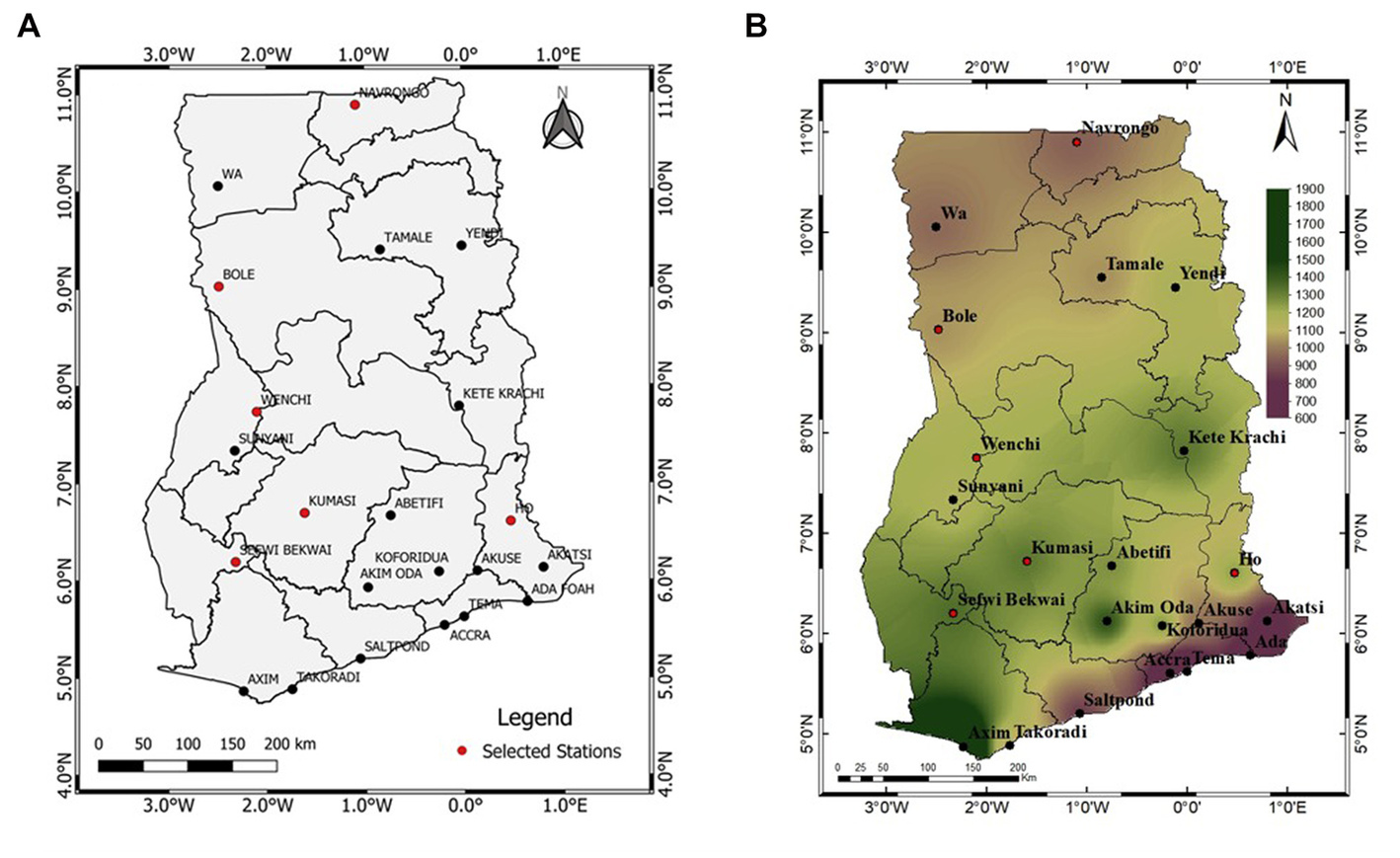
Figure 1. The map of Ghana showing (A) the 22 synoptic stations and those selected (in red dots) for the DSSAT Model Simulation, and (B) annual climatological rainfall distribution from 1985 to 2004.
Observed yearly crop yield data were obtained from the Ministry of Food and Agriculture (MoFA) in Ghana, and also downloaded from the website of the Food and Agriculture Organization (FAO). The crops that were considered for this study are maize, millet, rice and sorghum.
The weather data for this study was downloaded from the gridded Global Meteorological Forcing Dataset (GMFD). The dataset is constructed by combining a suite of global observation-based datasets with the NCEP/NCAR (National Centers for Environmental Prediction/National Center for Atmospheric Research) reanalysis. The GMFD data has a horizontal grid resolution of 0.44° x 0.44°. The parameters that were put in the WeatherMan component of the DSSAT model are maximum and minimum temperatures, rainfall, solar radiation, wind speed, and relative humidity.
2.2. The DSSAT model
The Decision Support System for Agro-technology Transfer (DSSAT) cropping system model (CSM) was used in this study. The DSSAT is a simulation model for crops that describes daily phenological development and growth in response to environmental factors (soil, weather, and management). The model was developed to simulate growth, development, and yield of a crop growing on a uniform area of land under prescribed or simulated management as well as the changes in soil water, carbon, and nitrogen that take place under the cropping system over time and comprises of a weather module, a soil module, a crop template module for the simulation of different crops (Jones et al., 2003). The simulation of soil water in the DSSAT crop model is based on the Ritchie et al. (1998) cascading water balance approach, which describes movement of water between soil layers.
The model requires daily weather values, among other factors to function. The model also uses details of soil characteristics such as drainage, runoff, evaporation and soil water-holding capacity, and rooting preference coefficients for multiple soil layers and initial soil water content (Jones et al., 2003). Crop characteristics are determined through crop- and cultivar specific genetic coefficients. The DSSAT family of models has separate plant modules that simulate growth and yield for individual species, e.g., CERES for maize, sorghum, wheat, and rice (Zinyengere et al., 2015), as well as CROPGRO for soybean. Among a limited number of biophysical crop models, DSSAT was selected for this study because of its global use and proven performances in impact studies (White et al., 2011). Regarding the study area, DSSAT has been applied in the West African Sudan Savanna in a number of studies (Naab et al., 2015; Akinseye et al., 2017; Amouzou et al., 2018).
2.3. Input data for the DSSAT model
An assessment of a crop model’s performance involves comparison of model outputs with real data and a determination of suitability for an intended purpose (Zinyengere et al., 2015).
Maize was selected for this study because it is commonly cultivated in Ghana (Figure 1), and also one of the major staple food crops in West Africa (Garrity et al., 2010). Again, Maize is documented to be one of the most important food crops in the world, and the model for the crop (CERES-Maize) has a long history of development and improvement (Ritchie et al., 1998; Soltani and Hoogenboom, 2007). The crop has also been evaluated for a wide range of experimental practices and environmental conditions (Jones et al., 2003).
The crop has different varieties (cultivars), growth characteristics and yields depending on the weather pattern, soil properties and other management or treatment practices such as fertilizer application, irrigation, and pest and disease control among others. The obatampa (maize) cultivar was selected because of its early maturity (between 100 to 110 days), growth characteristics in the areas selected, high yield and preference by consumers. In addition, this variety (maize) was selected because it has been calibrated and used in previous studies (e.g., MacCarthy et al., 2017). Six daily weather parameters (described in Section 2.2) were used for this study.
2.4. Crop varieties and management in the DSSAT model
The CERES-maize model in the simulation option component of the DSSAT by Jones et al. (2003) was used for the simulation of maize growth and yield, respectively, in this study. Crop management information such as crop varieties (cultivar), planting information, treatment options, and simulation options required by DSSAT was collected from MoFA through an interactive interview and from literature. The planting date integrated in the model was generated based on the rainfall onset dates calculated over Ghana from the definition by Stern et al. (1981). Seeds were planted at two plants per hole at a distance of 70 cm by 40 cm in rows at a depth of 5 cm. No fertilizer and irrigation were applied in this study because the result (outcome) is strictly to advise peasant or smallholder farmers, who usually do not apply any fertilizer or irrigation in their farming activities. All other relevant information required for the simulation was entered into the crop template module of DSSAT-CSM.
2.5. Method
In this study, the RODs were calculated using the definition by Stern et al. (1981) as shown in Table 1. The simulations were conducted using the crop management (XBuild v 4.7.5) option in DSSAT (Hoogenboom et al., 2019). Weather data is used to force DSSAT to simulate crop yield, thus, the weather data for each selected station was first uploaded differently into the DSSAT crop system model. The experiments for the simulation were further set up for each location. It was assumed in the experiment that pepper was initially planted on the soil. There are four key areas in the experiment that must be completed before the simulation can run successfully. And these are the simulation date option, planting date option, soil type option, and the weather data option.
In each experiment, the simulation date for each station was set at least 1 month before the planting date, and the planting date was set at 2 weeks after the start of rains. The number of seeds planted at random was 5,000, with 3 seeds planted per hole and later narrowed to 2 stands at emergence. The plant inter-row spacing and planting depth were set at 20 cm and 5 cm, respectively. The soil data for each location were uploaded into DSSAT and the corresponding soil type for each station was selected for each experiment. The weather data was also chosen based on the location where the simulation is being run. The cultivar of maize considered was obatampa. Subsequently, the experiments were saved and run, and the grain weight results of each simulation were analyzed.
3. Results and discussion
3.1. Uncertainties in the crop yield data
Figure 2 shows the standardized anomaly plot of observed (MoFA) and FAO yields of selected crops in Ghana. There is a very high correlation coefficient (i.e., 0.97, 0.92, 0.77, and 0.99 for maize, millet, rice, and sorghum, respectively) between the observed and FAO yields, which signifies a good agreement between them. Although it is not readily known whether the two datasets are from the same source or database, both are in phase (either below or above average yield) for most of the years within the study period and for all the crops. For instance, both datasets shows that there were below average yields for all the crops for the years 1985-1987, 1989-1990, 1992, 2001 (except for rice, Figure 2C), while there were above average yields for the years 1993-2000, 2002-2004 (except for millet, Figure 2B), although rice had a slight difference (1993-1996 and 1999-2004; Figure 2C). This decline in maize yield (Figure 2A), especially for the year 2001, agrees with Owusu-Sekyere et al. (2011), who carried out similar research in the central region of Ghana. Conversely, the discrepancy between the observed and FAO yields occurred in the year 1988 for all the crops except sorghum Figure 2D). This decline in maize yield, especially for the year 2001, agrees with Owusu-Sekyere et al. (2011), who carried out similar research in the central region of Ghana. Conversely, the discrepancy between the observed and FAO yields occurred in the year 1988 for all the crops except sorghum. Again, there was disagreement between the datasets for the year 1991 (sorghum), 1998 (rice) and 2004 (for millet). The inconsistency between the datasets for these years may be as a result of data collection method, area coverage, timelines and completeness. For example, the FAO uploads data for dissemination once a year irrespective of when they arrived 1 while MoFA may not be limited to the number of times data should be entered for archiving and subject to quality control. However, the result of this study has shown that there is a very high correlation between the MoFA and FAO dataset for the selected crops, so in the event where a researcher is unable to secure the MoFA data, the FAO data can be used for model evaluation.
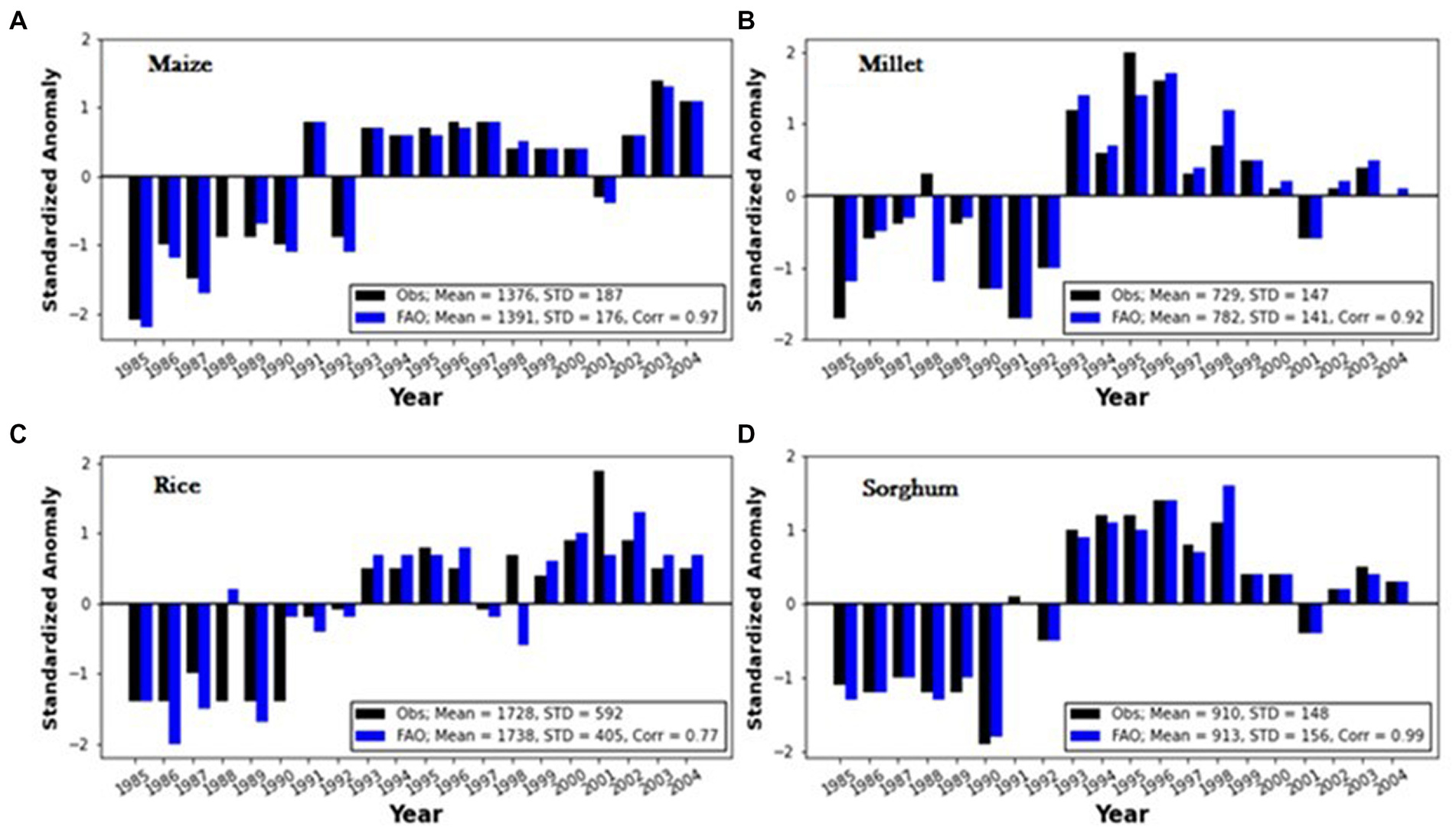
Figure 2. The standardized anomaly of selected crops yield in Ghana for (A) Maize, (B) Millet, (C) Rice, and (D) Sorghum in the period 1985–2004.
3.2. Uncertainties in rainfall onset dates
Before assessing the relationship between RODs and crop yields, it is important to measure the uncertainties in RODs over the study area. In general, the observed, satellite and GMFD data show a good agreement in the interannual variability of RODs over Ghana (Figure 3), and this is seen in the correlation coefficient between them (corr = 0.72 between observed and satellite; corr = 0.31 between observed and GMFD). For example, they show early RODs (below normal) in the years 1986, 1988, 1991, 1996, 2001, and 2003, while they show late RODs (above normal) in 1987, 1989–1990, 1994, and 1998.
However, there are some notable differences between RODs produced by these datasets. Such differences in RODs produced by different datasets over the same study area have been reported by some previous studies (e.g., Mounkaila et al., 2014; Abiodun et al., 2017; Kumi et al., 2020). For instance, in the year 1985, the observation and GMFD dataset produced an early onset (below normal) while the satellite data says there was a late onset (above normal) and the opposite occurred in the year 1997 for the datasets. Also, the observed and satellite data produced a late onset in 1992 and 2004 while the GMFD data suggests an early start of the rains. The reverse of this assertion occurred in 1995, 1999, and 2002. The early start of the rains in 2002, as suggested by the observed data, has been confirmed by Owusu-Sekyere et al. (2011), where according to them, the rainfall season began in February in the central region. Then in 1993 and 2000, while the observed data produced a late onset, the satellite produced a normal onset and the GMFD gave an early start of the rains over the study area. The disagreement between these datasets may be as a result of the fact that the GMet data covers only the 22 synoptic stations over Ghana (Figure 1) while the satellite data covers a horizontal grid resolution of 0.1° × 0.1° across the country. Moreover, the satellite (and GMFD with a resolution of 0.44° x 0.44°) data may start recording its 24-h daily values from 12 am (midnight) while the GMet records its daily values from 9 am. This means that any rainfall data before 9 am is regarded as a previous day’s data by GMet, which may alter the daily rainfall recordings from the sources used in this study. For this reason, the calculation of the RODs might be affected (see Figure 3).
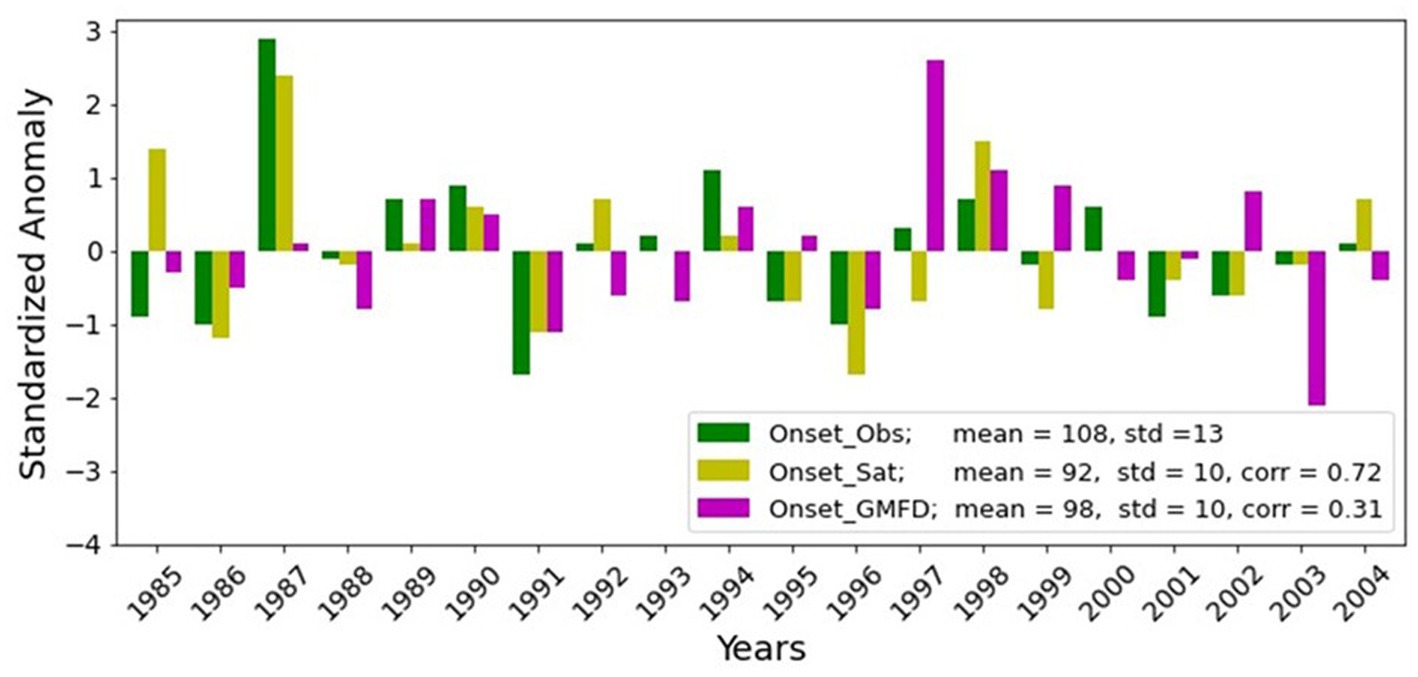
Figure 3. The standardized anomaly of observed, Satellite and GMFD rainfall onset dates in Ghana (1985 to 2004; 20 years).
3.3. Relationship between rainfall onset dates and crop yield
The results in Figure 4 establish the linear relationship between RODs and crop yield. This is essential to reveal the trend followed by crop yield in regards to RODs. From the observation data (based on the regression line), it is clear that early onset corresponds to high yield for all the selected crops, while late onset brings about a decrease in crop yield except millet (which virtually shows otherwise). The satellite data also replicate a similar pattern for all the crops, but the GMFD indicates an early onset correspondence with low yields for millet and sorghum. The regression line shows that there is a positive linear relationship between the crop yield and the onset dates. This means that RODs play a role in the determination of crop yield. This is consistent with the findings of Wakjira et al. (2021), who found that rainfall onset negatively affects cereal crop production in particular, implying a reduction in cereal crop production due to late onset and vice versa. The authors also assert that on average, a late onset of the rainy season leads to about 1.5% of rainfed cereal crop production loss per pentad. Mugalavai et al. (2008) also added that crop yields are likely to suffer significantly due to late onset.
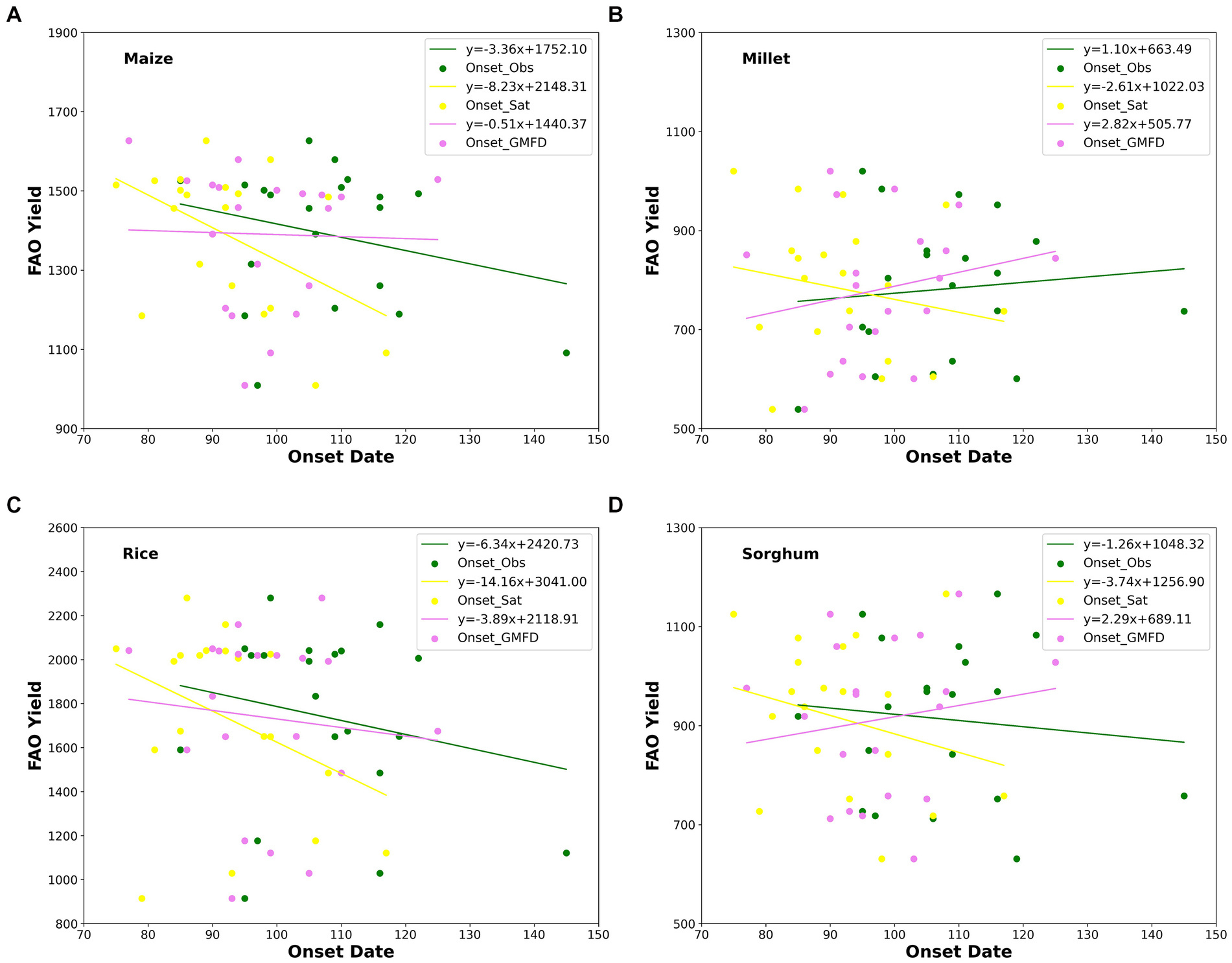
Figure 4. A scatter plot of crop yield and rainfall onset dates (1985 to 2004; 20 years) for (A) Maize, (B) Millet, (C) Rice and (D) Sorghum.
Figure 5 shows the standardized anomaly plot of observed (MoFA), FAO, and simulated maize yield for yearly onset (Sim_Yr_Onset Yield) and climatological onset (Sim_Cl_Onset Yield) RODs in Ghana. There is a very high correlation coefficient (corr = 0.97) between the observed and FAO yield. At the same time, there is good agreement between the observed and simulated yield from climatological onset date (corr = 0.52), while the correlation coefficient value for the observed and simulated yield from yearly onset is quite low (0.23), notwithstanding, they are all in phase with the observation data. The difference in the correlation values indicates that, although the model is able to replicate the observed yield pattern, there are considerable biases in the magnitude and interannual variations (especially from the Sim_Yr_Onset Yield). For instance, in 1985 and 2001, the observed, FAO, and simulated data agreed that there were below average yields. This decline in maize yield, especially for the year 2001, agrees with Owusu-Sekyere et al. (2011), who carried out similar research in the central region of Ghana. Again, the observed, FAO and simulated yield from climatological onset (Sim_Cl_Onset Yield) agreed to a below normal yield in 1989 and 1990, while in 1986 and 1992 the simulated yield from yearly onset (Sim_Yr_Onset Yield), FAO and observation also agreed on a below normal yield. Moreover, the observed (MoFA and FAO) and simulated yields showed good agreement in 1997, 1999, 2002, and 2003 where they all exhibited above average yields, though the model overestimated the yield in 1999 and 2002 (Sim_Cl_Onset Yield). Additionally, the FAO, observed and Sim_Cl_Onset Yield agreed to an above normal yield in 1996, while the observed (MoFA and FAO) and Sim_Yr_Onset Yield also showed above normal yield in 1991, 1994 and 1998.
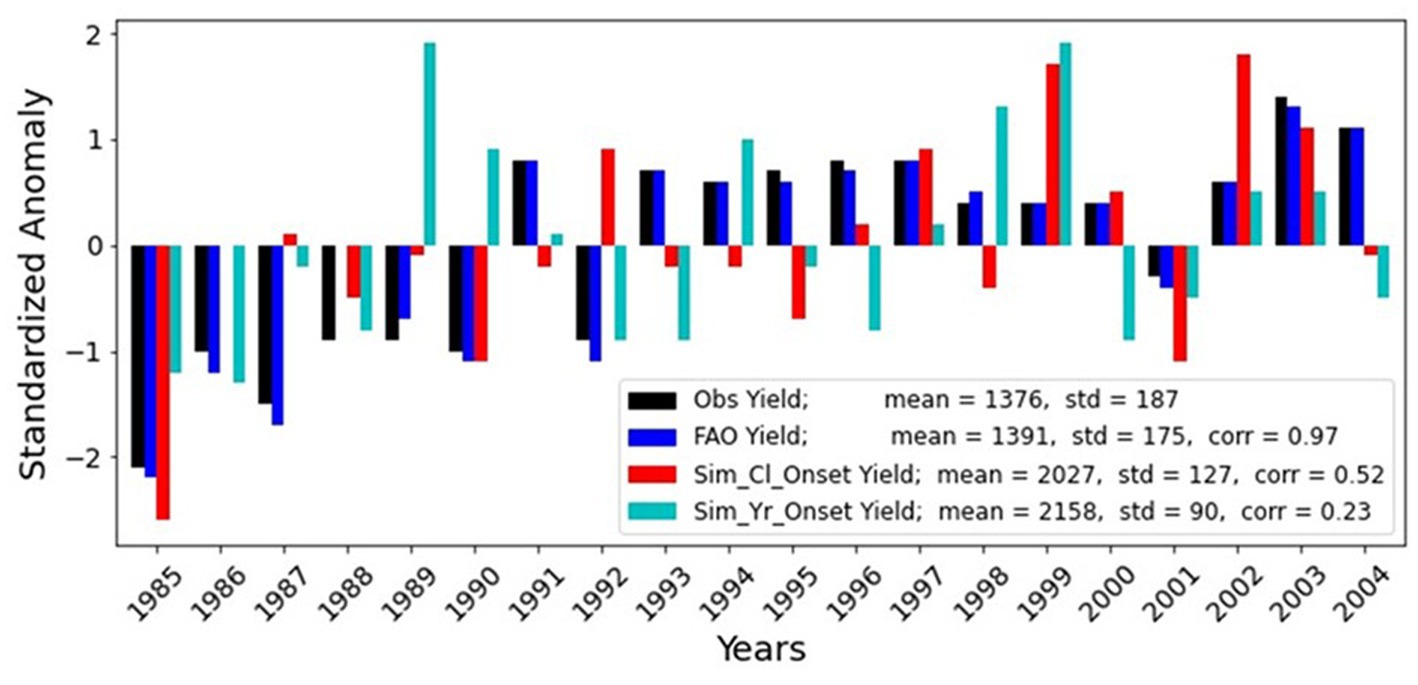
Figure 5. Standardized anomaly of observed, FAO and simulated maize yield (for yearly onset; Sim_Yr_Onset Yield and climatological onset; Sim_Cl_Onset Yield) in Ghana (1985 to 2004; 20 years).
Conversely, the discrepancy between the datasets occurred in 1986–1996, 1998, 2000 and 2004. In 1993, 1995 and 2004, the observation data showed above average yield while the model showed opposite. According to the observed and simulated yield from yearly onset, 1986 and 1987 experienced below average but the simulated yield from climatological onset suggested a relatively no change in yield (especially in 1986). Similar incidence occurred in 1992, where the simulated yield from climatological onset depicted an above normal yield but the other data proved otherwise. Again, the observed and yield from climatological onset showed below average in 1989 and 1990, while the simulated yield from yearly onset rather depicted an above normal trend. Consequently, the reverse of this occurred in 1996 and 2000. Furthermore, in 1991, 1994, and 1998, the simulated yield from yearly onset and that of observation depicted an above normal yield and the climatological onset data proved otherwise. Finally, the model agrees with the observed (MoFA) data that there was below average yield in the year 1988 but the FAO data suggests no change in yield over the study area.
Generally, the correlation between the observed (GMet) and GMFD rainfall data is low for all the selected stations (Figure 6). The highest correlation coefficient value is 0.18 over Navrongo (Figure 6B), and the lowest (0.03) is in Ho (Figure 6E), with the remaining selected stations (Figures 6C,D,F), except Bole (Figure 6A), having the same correlation coefficient of 0.06. These correlation values across the selected stations indicate a poor agreement between the observed and GMFD data (red line on Figure 6). The large discrepancy between the datasets is quite evident as the GMFD produces low rainfall amounts compared to the observation. As a result, the low rainfall values seem to be causing a problem in the calculation of RODs as well as the simulated maize yield produced by this data. This outcome is consistent with the findings of Guirguis and Avissar (2008), who stated that the GMFD data has a high level of phase error when compared to other datasets used. And that in relation to other datasets, the GMFD shows the wettest conditions of long term mean precipitation except during winter, spring, and fall in the Pacific northwest.
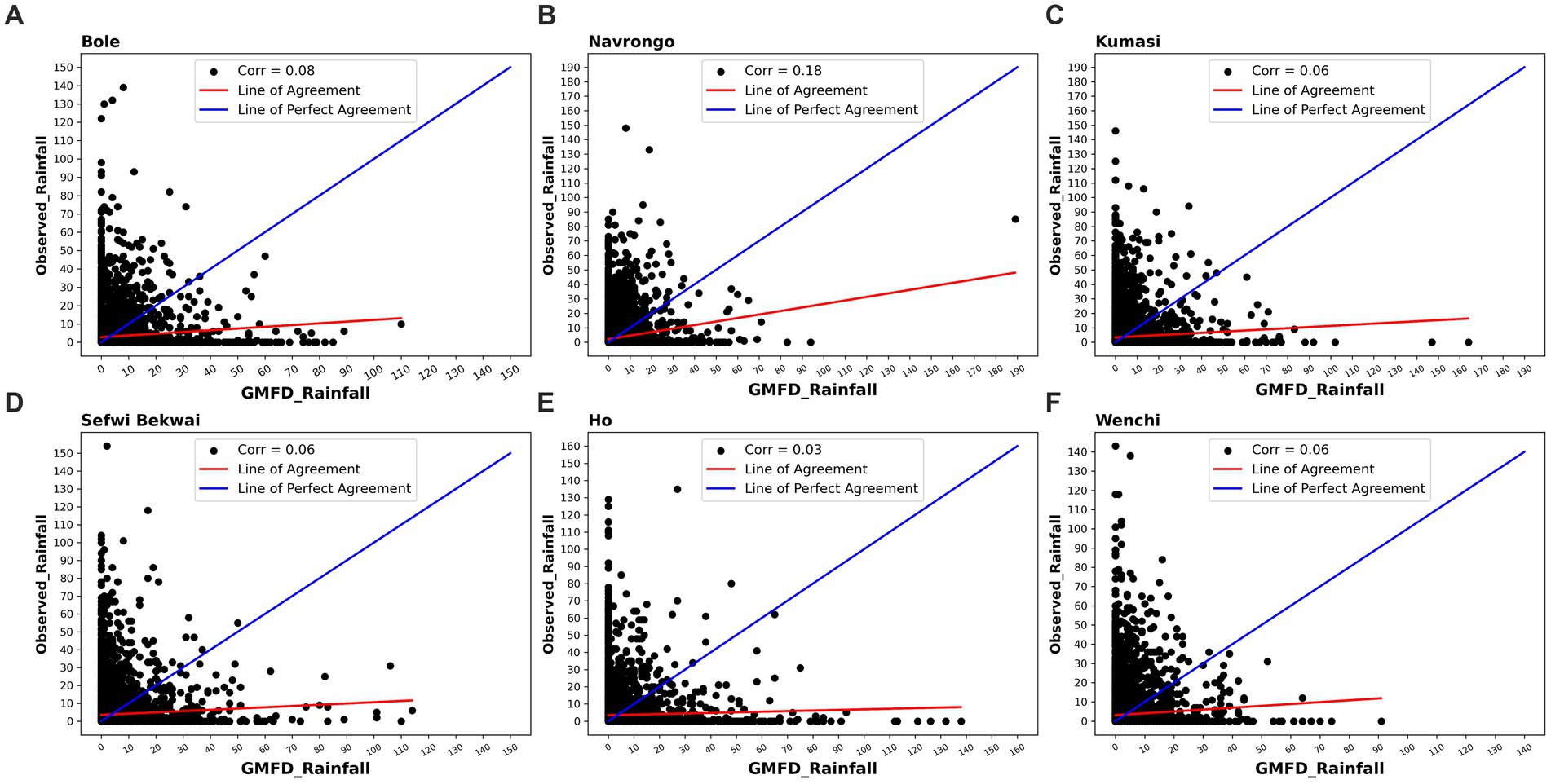
Figure 6. A scatter plot showing the line of agreement between the observed and GMFD rainfall amount for six selected stations in Ghana (1985 to 2004; 20 years); (A) Bole, (B) Navrongo, (C) Kumasi, (D) Sefwi Bekwai, (E) Ho and (F) Wenchi.
To propose a strong basis for agricultural planning and decision-making purposes, this study can be improved in several ways. For instance, there were limitations to the number of crops to be simulated because most of the selected crops have not yet been calibrated in the DSSAT model over the study area. Moreover, the disparity among the recording of the rainfall datasets used in this study may be the cause for some of the biases. To address these limitations, developers of the DSSAT crop model can increase the number of calibrated crop cultivars, which may help in the evaluation and wide use of the model in the study area by researchers. Again, GMet can amend the recording time of their daily rainfall values to reconcile with that of satellite recordings. Moreover, the GMFD datasets need reassessment over the study area because its disparity with observation (in situ) data is quite significant.
4. Conclusion
This study has investigated the extent to which rainfall onset date impacts crop yield. Four crops were considered in this study - Maize, millet, rice, and sorghum, but only maize was simulated using the DSSAT crop model version 4.7.5, because it has been calibrated in this crop model over the study area. Weather data from the GMFD was used to force the crop system model. RODs from three datasets (observed, satellite, and GMFD) were compared and their relation with crop yield were also considered. The main findings of the study can be summarized as follows:
• There is a very high correlation between the MoFA and FAO dataset for the selected crops, and so in the circumstance where a researcher is unable to secure the MoFA data, the FAO data can be used for model evaluation.
• Onset date has influence on crop yield and this has been proven in both observation and simulation, where early onset corresponds to high yield for most of the selected crops.
• The satellite and GMFD data are able to reproduce the observed interannual variability of RODs over Ghana although with some biases. However, there seem to be a problem with the GMFD data and that has manifested in the calculation of RODs, as well as the rainfall amount.
• The DSSAT model is able to significantly replicate the observed yield pattern although there are considerable biases in the magnitude and interannual variation, which is as a result of the GMFD data.
• There is a significant difference between the observed and GMFD rainfall datasets; the GMFD data produces low rainfall amounts which has affected the RODs and simulated maize yield produced by this data.
The current study has shown that the DSSAT model is able to simulate crop yield over Ghana if the right cultivars are selected for both observed and model data. The study has also shown that the FAO yield data can be used in situations where a researcher is unable to retrieve actual yield information from the country. The results from this study may be beneficial to researchers, small-holder farmers, policy makers and the general public in planning agricultural activities in a highly variable climate. The purpose of this study was to evaluate the ability of the crop model to simulate the yields of the selected crops in past climate. The authors are working on the potential impacts of climate change on the selected crop (maize) yield in Ghana at specific global warming levels.
Data availability statement
Publicly available datasets were analyzed in this study, and can be found as follows: The GMFD data was downloaded at https://rda.ucar.edu/datasets/ds314.0/, while the FAO data was downloaded at https://www.fao.org/faostat/en/#data/QCL. Also, the satellite rainfall data (ARC2) was downloaded at https://www.icpac.net/data-center/arc2/. The authors went to the Ghana Meteorological Agency (GMet) to collect the data from their client service unit. The link to the website of GMet is https://www.meteo.gov.gh/gmet/. Moreover, the authors went to the Ministry of Food and Agriculture (MoFA) head office at Accra to collect the crop yield data. The link to the website of MoFA is https://mofa.gov.gh/site/.
Author contributions
NK and BA: conceptualization. TA, VA, and BA: data acquisition. NK, TA, and VA: methodology. NK: writing - original draft preparation and funding acquisition. BL and other authors: writing – review and editing. BA: supervision. All authors contributed to the article and approved the submitted version.
Funding
This work was funded by a grant from the African Institute for Mathematical Sciences, www.nexteinstein.org, with financial support from the Government of Canada, provided through Global Affairs Canada, www.international.gc.ca, and the International Development Research Centre, www.idrc.ca.
Conflict of interest
The authors declare that the research was conducted in the absence of any commercial or financial relationships that could be construed as a potential conflict of interest.
Publisher’s note
All claims expressed in this article are solely those of the authors and do not necessarily represent those of their affiliated organizations, or those of the publisher, the editors and the reviewers. Any product that may be evaluated in this article, or claim that may be made by its manufacturer, is not guaranteed or endorsed by the publisher.
Supplementary material
The Supplementary material for this article can be found online at: https://www.frontiersin.org/articles/10.3389/fsufs.2023.1176385/full#supplementary-material
Footnotes
References
Abiodun, B. J., Adegoke, J., Abatan, A. A., Ibe, C. A., Egbebiyi, T. S., Engelbrecht, F., et al. (2017). Potential impacts of climate change on extreme precipitation over four African coastal cities. Clim. Chang. 143, 399–413. doi: 10.1007/s10584-017-2001-5
Abiodun, B. J., Pal, J. S., Afiesimama, E. A., Gutowski, W. J., and Adedoyin, A. (2008). Simulation of West African monsoon using RegCM3 part II: impacts of deforestation and desertification. Theor. Appl. Climatol. 93, 245–261. doi: 10.1007/s00704-007-0333-1
Ahmed, M., Asif, M., Sajad, M., Khattak, J. Z. K., Ijaz, W., Wasaya, A., et al. (2013). Could agricultural system be adapted to climate change?: a review. Aust. J. Crop. Sci. 7, 1642–1653.
Akinseye, F. M., Adam, M., Agele, S. O., Hoffmann, M. P., Traore, P. C. S., and Whitbread, A. M. (2017). Assessing crop model improvements through comparison of sorghum (sorghum bicolor L. moench) simulation models: a case study of West African varieties. Field Crop Res. 201, 19–31. doi: 10.1016/j.fcr.2016.10.015
Amouzou, K. A., Naab, J. B., Lamers, J. P., and Becker, M. (2018). CERES-maize and CERES-Sorghum for modeling growth, nitrogen and phosphorus uptake, and soil moisture dynamics in the dry savanna of West Africa. Field Crop Res. 217, 134–149. doi: 10.1016/j.fcr.2017.12.017
Baldwin, B. S., and Cossar, R. D. (2009). Castor yield in response to planting date at four locations in the south-Central United States. Ind. Crop. Prod. 29, 316–319. doi: 10.1016/j.indcrop.2008.06.004
Blanc, É. (2011). The Impact of Climate Change on Crop Production in Sub-Saharan Africa. Doctoral dissertation (University of Otago).
Blanche, S. B., and Linscombe, S. D. (2009). Stability of rice grain and whole kernel milling yield is affected by cultivar and date of planting. Agron. J. 101, 522–528. doi: 10.2134/agronj2008.0160x
Chisanga, C. B., Phiri, E., and Chinene, V. R. (2017). Climate change impact on maize (Zea mays L.) yield using crop simulation and statistical downscaling models: a review. Sci. Res. Essays 12, 167–187. doi: 10.5897/SRE2017.6521
Danso, I., Webber, H., Bourgault, M., Ewert, F., Naab, J. B., and Gaiser, T. (2018). Crop management adaptations to improve and stabilize crop yields under low-yielding conditions in the Sudan savanna of West Africa. Eur. J. Agron. 101, 1–9. doi: 10.1016/j.eja.2018.08.001
Dieng, D., Laux, P., Smiatek, G., Heinzeller, D., Bliefernicht, J., Sarr, A., et al. (2018). Performance analysis and projected changes of Agroclimatological indices across West Africa based on high-resolution regional climate model simulations. J. Geophys. Res. Atmos. 123, 7950–7973. doi: 10.1029/2018JD028536
Dobor, L., Barcza, Z., Hlásny, T., Árendás, T., Spitkó, T., and Fodor, N. (2016). Crop planting date matters: estimation methods and effect on future yields. Agric. For. Meteorol. 223, 103–115. doi: 10.1016/j.agrformet.2016.03.023
Egli, D. B., and Cornelius, P. L. (2009). A regional analysis of the response of soybean yield to planting date. Agron. J. 101, 330–335. doi: 10.2134/agronj2008.0148
Garcia, A. G., Guerra, L. C., and Hoogenboom, G. (2009). Impact of planting date and hybrid on early growth of sweet corn. Agron. J. 101, 193–200. doi: 10.2134/agronj2007.0393
Garrity, D. P., Akinnifesi, F. K., Ajayi, O. C., Weldesemayat, S. G., Mowo, J. G., Kalinganire, A., et al. (2010). Evergreen agriculture: a robust approach to sustainable food security in Africa. Food Sec. 2, 197–214. doi: 10.1007/s12571-010-0070-7
Guan, K., Sultan, B., Biasutti, M., Baron, C., and Lobell, D. B. (2015). What aspects of future rainfall changes matter for crop yields in West Africa? Geophys. Res. Lett. 42, 8001–8010. doi: 10.1002/2015GL063877
Guirguis, K. J., and Avissar, R. (2008). An analysis of precipitation variability, persistence, and observational data uncertainty in the western United States. J. Hydrometeorol. 9, 843–865. doi: 10.1175/2008JHM972.1
Hoogenboom, G., Porter, C. H., Boote, K. J., Shelia, V., Wilkens, P. W., Singh, U., et al. (2019). “The DSSAT crop modeling ecosystem” in Advances in Crop Modelling for a Sustainable Agriculture (Burleigh Dodds Science Publishing), 173–216. Available at: https://www.fao.org/faostat/en/#data/QCL/metadata
Ingram, K. T., Roncoli, M. C., and Kirshen, P. H. (2002). Opportunities and constraints for farmers of West Africa to use seasonal precipitation forecasts with Burkina Faso as a case study. Agric. Syst. 74, 331–349. doi: 10.1016/S0308-521X(02)00044-6
Jones, J. W., Antle, J. M., Basso, B., Boote, K. J., Conant, R. T., Foster, I., et al. (2017). Brief history of agricultural systems modeling. Agric. Syst. 155, 240–254. doi: 10.1016/j.agsy.2016.05.014
Jones, J. W., Hoogenboom, G., Porter, C. H., Boote, K. J., Batchelor, W. D., Hunt, L. A., et al. (2003). The DSSAT cropping system model. Eur. J. Agron. 18, 235–265. doi: 10.1016/S1161-0301(02)00107-7
Kamara, A. Y., Ekeleme, F., Chikoye, D., and Omoigui, L. O. (2009). Planting date and cultivar effects on grain yield in dryland corn production. Agron. J. 101, 91–98. doi: 10.2134/agronj2008.0090
Kumi, N., Abiodun, B. J., and Adefisan, E. A. (2020). Performance evaluation of a subseasonal to seasonal model in predicting rainfall onset over West Africa. Earth and space. Science 7:e2019EA000928-T. doi: 10.1029/2019EA000928
Laux, P., Jäckel, G., Tingem, R. M., and Kunstmann, H. (2010). Impact of climate change on agricultural productivity under rainfed conditions in Cameroon—a method to improve attainable crop yields by planting date adaptations. Agric. For. Meteorol. 150, 1258–1271. doi: 10.1016/j.agrformet.2010.05.008
Laux, P., Kunstmann, H., and Bárdossy, A. (2008). Predicting the regional onset of the rainy season in West Africa. Int. J. Climatol. 28, 329–342. doi: 10.1002/joc.1542
Laux, P., Wagner, S., Wagner, A., Jacobeit, J., Bárdossy, A., and Kunstmann, H. (2009). Modelling daily precipitation features in the Volta Basin of West Africa. Int. J. Climatol. 29, 937–954. doi: 10.1002/joc.1852
Limantol, A. M., Keith, B. E., Azabre, B. A., and Lennartz, B. (2016). Farmers’ perception and adaptation practice to climate variability and change: a case study of the Vea catchment in Ghana. Springerplus 5, 830–838. doi: 10.1186/s40064-016-2433-9
MacCarthy, D. S., Adiku, S. G., Freduah, B. S., Gbefo, F., and Kamara, A. Y. (2017). Using CERES-maize and ENSO as decision support tools to evaluate climate-sensitive farm management practices for maize production in the northern regions of Ghana. Front. Plant Sci. 8:31. doi: 10.3389/fpls.2017.00031
Masika, D. (2016). Using Dssat-Ceres Maize Model to Estimate District-Level Yields in Northern Ghana. Ghana: Department of Soil Science. University Of Ghana.
Mounkaila, M. S., Abiodun, B. J., and Omotosho, J. B. (2014). Assessing the capability of CORDEX models in simulating onset of rainfall in West Africa. Theor. Appl. Climatol. 119, 255–272. doi: 10.1007/s00704-014-1104-4
Mourice, S. K., Rweyemamu, C. L., Tumbo, S. D., and Amuri, N. (2014). Maize cultivar specific parameters for decision support system for agrotechnology transfer (dssat) application in tanzania. Am. J. Plant Sci. 05, 821–833. doi: 10.4236/ajps.2014.56096
Mugalavai, E. M., Kipkorir, E. C., Raes, D., and Rao, M. S. (2008). Analysis of rainfall onset, cessation and length of growing season for western Kenya. Agric. For. Meteorol. 148, 1123–1135. doi: 10.1016/j.agrformet.2008.02.013
Naab, J. B., Boote, K. J., Jones, J. W., and Porter, C. H. (2015). Adapting and evaluating the CROPGRO-peanut model for response to phosphorus on a sandy-loam soil under semi-arid tropical conditions. Field Crop Res. 176, 71–86. doi: 10.1016/j.fcr.2015.02.016
Nicholson, S. E., Some, B., and Kone, B. (2000). An analysis of recent rainfall conditions in West Africa, including the rainy seasons of the 1997 El Niño and the 1998 La Niña years. J. Clim. 13, 2628–2640. doi: 10.1175/1520-0442(2000)013%3C2628:AAORRC%3E2.0.CO;2
Novella, N. S., and Thiaw, W. M. (2013). African rainfall climatology version 2 for famine early warning systems. J. Appl. Meteorol. Climatol. 52, 588–606. doi: 10.1175/JAMC-D-11-0238.1
Omotosho, J. B., Balogun, A. A., and Ogunjobi, K. (2000). Predicting monthly and seasonal rainfall, onset and cessation of the rainy season in West Africa using only surface data. Int. J. Climatol. 20, 865–880. doi: 10.1002/1097-0088(20000630)20:8%3C865::AID-JOC505%3E3.0.CO;2-R
Owusu-Sekyere, J. D., Alhassan, M., and Nyarko, B. K. (2011). Assessment of climate shift and crop yields in the Cape Coast area in the Central Region of Ghana. Asian J. Agric. Biol. 6, 49–54.
Pedersen, P., and Lauer, J. G. (2004). Response of soybean yield components to management system and planting date. Agron. J. 96, 1372–1381. doi: 10.2134/agronj2004.1372
Ritchie, J. T., Singh, U., Godwin, D. C., and Bowen, W. T. (1998). “Cereal growth, development and yield” in Understanding options for agricultural production (Dordrecht: Springer), 79–98.
Ruiz Nogueira, B., Boote, K. J., and Sau, F. (2001). Calibration and use of CROPGRO-soybean model for improving soybean management under rainfed conditions. Agric. Syst. 68, 151–173. doi: 10.1016/S0308-521X(01)00008-7
Soltani, A., and Hoogenboom, G. (2007). Assessing crop management options with crop simulation models based on generated weather data. Field Crop Res. 103, 198–207. doi: 10.1016/j.fcr.2007.06.003
Stern, R. D., Dennett, M. D., and Garbutt, D. J. (1981). The start of the rains in West Africa. Int. J. Climatol. 1, 59–68. doi: 10.1002/joc.3370010107
Sultan, B., Roudier, P., Quirion, P., Alhassane, A., Muller, B., Dingkuhn, M., et al. (2013). Assessing climate change impacts on sorghum and millet yields in the Sudanian and Sahelian savannas of West Africa. Environ. Res. Lett. 8:014040. doi: 10.1088/1748-9326/8/1/014040
Wakjira, M. T., Peleg, N., Anghileri, D., Molnar, D., Alamirew, T., Six, J., et al. (2021). Rainfall seasonality and timing: implications for cereal crop production in Ethiopia. Agric. For. Meteorol. 310:108633. doi: 10.1016/j.agrformet.2021.108633
Wani, S. P., Sreedevi, T. K., Rockström, J., and Ramakrishna, Y. S. (2009). “Rainfed agriculture–past trends and future prospects” in Rainfed agriculture: Unlocking the potential, vol. 7 (Wallingford UK: CABI), 1–33.
White, J. W., Hoogenboom, G., Kimball, B. A., and Wall, G. W. (2011). Methodologies for simulating impacts of climate change on crop production. Field Crop Res. 124, 357–368. doi: 10.1016/j.fcr.2011.07.001
Ziervogel, G., and Calder, R. (2003). Climate variability and rural livelihoods: assessing the impact of seasonal climate forecasts in Lesotho. Area 35, 403–417. doi: 10.1111/j.0004-0894.2003.00190.x
Keywords: planting activities, observed yield, crop model, simulate, replicate
Citation: Kumi N, Adeliyi TE, Asante VA, Abiodun BJ and Lamptey BL (2023) Impact of rainfall onset date on crops yield in Ghana. Front. Sustain. Food Syst. 7:1176385. doi: 10.3389/fsufs.2023.1176385
Edited by:
Siyabusa Mkuhlani, International Institute of Tropical Agriculture (IITA), KenyaReviewed by:
Lovemore Chipindu, International Maize and Wheat Improvement Centre (CIMMYT), ZimbabweAhmed Kheir, International Center for Agriculture Research in the Dry Areas (ICARDA), Egypt
Copyright © 2023 Kumi, Adeliyi, Asante, Abiodun and Lamptey. This is an open-access article distributed under the terms of the Creative Commons Attribution License (CC BY). The use, distribution or reproduction in other forums is permitted, provided the original author(s) and the copyright owner(s) are credited and that the original publication in this journal is cited, in accordance with accepted academic practice. No use, distribution or reproduction is permitted which does not comply with these terms.
*Correspondence: Naomi Kumi, naomi.kumi@uenr.edu.gh
†ORCID: Naomi Kumi, https://orcid.org/0000-0003-0072-9857
Tolulope E. Adeliyi, https://orcid.org/0000-0002-7001-4403
Vincent A. Asante, https://orcid.org/0000-0001-6182-6410
Babatunde J. Abiodun, https://orcid.org/0000-0002-3878-0116
Benjamin L. Lamptey, https://orcid.org/0000-0003-0491-9965