- 1Institute of Agricultural Economics and Development, Chinese Academy of Agricultural Sciences, Beijing, China
- 2Agricultural Information Institute, Chinese Academy of Agricultural Sciences, Beijing, China
- 3Institute of Geographic Sciences and Natural Resources Research, Chinese Academy of Sciences, Beijing, China
Recently, COVID-19 pandemic, locust plague, drought and conflict have seriously affected the development of agriculture in Africa, which make Africa countries difficultly to achieve the Sustainable Development Goals (SDGs) 1 and 2. As the cornerstone of agricultural development, technological progress has made brilliant contributions to achieving food security and nutrition improvement in African countries. And as the largest economic and trade partner of Africa, analyzing the agricultural technology gap between China and African countries and exploring optimal paths also has great significance for achieving SDGs 8 and 9. Therefore, the paper used the Meta-frontier SBM model to measure the agricultural technology gap between China and African countries from 2003 to 2019, and explores sources of the gap. On this basis, 24 African countries were taken as samples to identify multiple paths for narrowing the technology gap between China and Africa with the help of the configuration analysis method of the fuzzy-set qualitative comparative analysis (fsQCA). The results showed that the overall agricultural technology gap between China and Africa was narrowing, which was mainly caused by the reduction of pure technical inefficiency. However, sources of technology gap in African countries with different economic development levels were different. Configuration analysis found that agricultural technology innovation and institutional environment were the key conditional variables to narrow the agricultural technology gap between China and Africa. Five paths had been formed around two key conditional variables, and further summarized into three driving modes: “technology-environment” driving mode, “technology-organization” driving mode and “organization-environment” driving mode. Furthermore, this paper explored the multiple concurrent causality of narrowing the technology gap, which overcomes the deficiency of using regression methods. The paper highlights the importance of enhancing the integration of technical, organizational, and environmental conditions in African countries to collectively advance agricultural scientific and technological progress
1. Introduction
In recent years, the COVID-19 pandemic, the Russian Ukrainian war, locust plague, drought, flood and conflict have increased the vulnerability of global food security (FAO, IFAD, UNICEF, WFP, and WHO, 2022; Li and Lin, 2023), especially having a significant impact on the continuous improvement of food security and nutrition in Africa (ECOSOC, 2022). Africa is the main region where food crisis or sudden food insecurity occurs. In 2019, 228 million people in African countries were suffering from hunger, the number of people affected by hunger in Africa increased by 35 million in 2020 and 15 million in 2021, a total increase of 50 million in 2 years (FAO, IFAD, UNICEF, WFP, and WHO, 2022). The achievement of SDGs 1 and 2 in African countries will be even more difficult. The current agricultural production mode in developing countries is characterized by high resource input and high energy consumption, which is unsustainable (Li and Lin, 2022; Li and Lin, 2023). The fundamental way to achieve food security in the future is only technological progress and the adoption of new technologies (Garnett et al., 2013; Gouvea et al., 2022; Tyczewska et al., 2023). At present, investment in agricultural science and technology in Africa is relatively low. There are only more than 400 institutions engaged in agricultural research and development in Africa. Farmers are seriously lack of practical agricultural technology. Therefore, African countries rely more on technology transfer from other countries to achieve agricultural technology progress (Olasehinde et al., 2023). Current studies generally confirm the important role of different types of technology transfer modes on agricultural development and food security in Africa (Kijima et al., 2012; Walker and Hofstetter, 2016; Kirui and Kozicka, 2018). As the largest investor in Africa among developing countries, China has been Africa’s largest trading partner since 2009 (UN COMTRADE 2018). Currently, China has continuously increased technology transfer to Africa, built a number of new joint laboratories, and trained the next generation of scientists for Africa in combination with the major scientific and technological development needs, basic conditions for scientific research and willingness to cooperate of countries along the “belt and road” (Cyranoski, 2018). However, there is evidence that although African countries have been implementing the order of innovation in the north and imitation in the south for nearly two decades, up to now, African countries are still far away from the technological frontier (Gebrerufael, 2021). The large technology gap will also hinder the late development advantage of African countries, which is not conducive to the realization of technological progress and food security goals. With the increasing transfer of agricultural technology from China to Africa, it is crucial to clarify the agricultural technology gap between Africa and China, identify its underlying causes, and explore the paths for African countries to narrow this gap. This will ultimately contribute to achieving food security and improving nutrition in African countries.
Technology is the third factor of production apart from labor and capital. Considering the different development levels of different countries, there will be a gap due to the asymmetry of products or process technology, which may determine their comparative advantages (Jordaan, 2017). Technological gap theory holds that a large part of trade between countries is based on the existence of technology gap. The current academic research on technology gap mainly focuses on its measurement and influencing factors. In terms of technology gap measurement, current studies mainly use the single indicator method, total factor productivity (TFP) method and the metafrontier analysis (MFA) method to measure technology gap. The single indicator method uses capital stock, patents and other indicators as the representation of a subject’s technical level, and the technology gap among subjects is expressed by the difference or ratio of technical level. For example, Guo et al. (2012) used patent differences to measure the technology gap among transnational corporations. Bednarek (2016) used capital differences to measure the technology gap and argued that the high technology gap would increase output in the long term. The total factor productivity method takes the ratio of total factor productivity as the representation of the technology gap among subjects. For example, Ha et al. (2009) used TFP differences as a token of the technology gap between South Korea and Chinese Taipei. Xie and Zhang (2020) used the TFP ratio of China and the United States to measure the technology gap between these two countries. The single indicator method and the total factor productivity method do not take into account the differences in the production frontier of different entities, while the MFA method proposed by Battese et al. (2004) can be used to measure and compare the efficiency of different entities with different technical levels, so it is widely used to measure the technology gap among entities. For example, Kontolaimou et al. (2016) used the MFA method to measure the technology gap among European countries, finding that the technology gap between developing countries and developed countries was large. Chaffai (2020) used the MFA method to measure the technology gap between Islamic banks and traditional banks in the MENA region. In terms of influencing factors of technology gap, according to Verspagen (1991), the technology gap between southern and northern countries could not be automatically narrowed, so how to narrow the gap has always been the focus of academic attention. Current studies have confirmed the positive role of technology innovation, foreign direct investment (FDI), capacity building, organizational learning, human capital and other factors in narrowing the technology gap (Eltis, 1978; Ahmed and Krishnasamy, 2013; Landini and Malerba, 2017; Amankwah-Amoah et al., 2019). In addition, some studies believe that cultural and religious factors can improve technical efficiency and thus narrow the technology gap (Tanko and Ismaila, 2021).
In summary, the existing research has conducted useful explorations in the measurement and the influencing factors of technology gap, which also provides a relevant basis for this study. However, there is still room for improvement in the existing research, which is also the contribution of this paper: (1) The existing empirical research on the technology gap between northern and southern countries is almost old, and out of the empirical literature, there is no single exclusive article produced on the dynamics of the technology gap in China and Africa. As Africa’s largest trading partner, it is particularly important for China to clarify the technology gap with Africa. (2) The present measurement methods of technology gap mainly include single indicator method, total factor productivity method, the MFA method, etc. Since the MFA method can realize the measurement and comparison of the efficiency of different clusters with different technical levels, it is widely used to measure the technology gap among subjects, such as the research conducted by Kontolaimou et al. (2016) and Chaffai (2020). However, these studies only measured the technology gap, while ignoring the subdivision of technology gap sources. Therefore, this paper adopts the meta-frontier theory and the data envelopment analysis (DEA) method to build a meta-frontier slack based measure (SBM) model to measure the agricultural technology gap between China and Africa, and clarify the causes of the gap. (3) Most of the existing studies on the influencing factors of technology gap are unidirectional, that is, the regression method is used to explore the impact of single factors on technology gap. However, the agricultural technology gap between China and Africa is a systematic problem caused by multiple concurrent causality, rather than the impact of any single factor. So this paper selects the fuzzy-set qualitative comparative analysis method (fsQCA), takes “agricultural technology gap - 1” as the outcome variable, aims to analyze the path to narrow the agricultural technology gap between China and Africa.
2. Theoretical basis and analysis framework
The theoretical analysis framework of this paper is constructed on the basis of the TOE theory (technology organization environment) proposed by Tornatzky et al. (1990), which was initially used to emphasize the technical, organizational and environmental conditions that affect an organization’s technology innovation. Later, it was widely used by scholars to discuss the adoption and application of organizational technology (Chatterjee et al., 2021; Dadhich and Hiran, 2022). The theory divides the scenarios of technology adoption and application into technical conditions, organizational conditions and environmental conditions. Technical conditions refer to the existing technical resources and technical capabilities of an organization, as well as the available technical resources outside the organization. Technical conditions emphasize the characteristics of the technology itself and the maximum output that the technology can achieve (Chau and Tam, 1997). Organizational conditions usually refer to the characteristics of an organization in terms of resource utilization and adoption, covering the size of the organization, basic conditions of the organization, human resources status, and relevant resources available to the organization, etc., emphasizing the initiative of the organization (Walker, 2014). Environmental conditions refer to the macro environment in which an organization conducts business or activities, emphasizing the impact of the institutional environment (Oliveir and Martins, 2011). According to its definition, the TOE theory is appropriate to be applied in the research on the optimization path of agricultural technology gap between China and Africa. In addition, in order to solve the shortcomings of the TOE theory in dealing with the combination of multiple conditions and explaining the underlying mechanism, this paper introduces configuration analysis method for correction, and finally forms a comprehensive analysis framework of three primary conditions, namely technical conditions, organizational conditions and environmental conditions, and totally six secondary conditions (Figure 1).
First, technical conditions. Technical conditions include a secondary condition namely agricultural technology innovation. The innovation theory proposed by Schumpeter emphasizes that technology innovation is the source of core technology, and triggers the process of technology transformation and new product generation, which can bring economic development and promote social progress (Zheng et al., 2021; Li et al., 2022). Firstly, technology innovation can realize the iterative upgrading of technology and production mode, which leads to the continuous optimization of technology level and production level, so as to realize technological progress (Wang and Zhu, 2020; Liang et al., 2022). Secondly, the way of technological progress is Poisson flow (Lin and Mao, 2023). Agricultural technology innovation strengthens the Poisson flow density of African countries, which helps to achieve technological progress and efficiency improvement. Third, through agricultural technology innovation, African countries can gradually improve their R&D endowment structure, strengthen their absorptive capacity, and participate in domestic and international value chains to capture more innovative “learning effects,” thus contributing to technological progress and efficiency improvement (He et al., 2019).
Second, organizational conditions. Organizational conditions include three secondary conditions: agricultural producer level, agricultural infrastructure and FDI. In the process of agricultural production, agricultural practitioners improve production efficiency by exerting their subjective initiative, and scholars have generally affirmed the positive correlation between producer level and TFP (Ahsan and Haque, 2017; Atesagaoglu et al., 2017; Okunade et al., 2022). In the agricultural production practice in Africa, it is crucial to introduce, digest and absorb foreign technologies. A high-level agricultural practitioner is bound to have a leading edge in the process of technology learning to realize the optimal allocation of resources and rapid learning of technology (Nonaka, 1994). As a public service product in rural areas, agricultural infrastructure has a strong positive external effect, which directly affects agricultural production practice, helps to drive the transformation and upgrading of rural economy, promote the integration of the three industries, and thus achieve agricultural technological progress. For example, productive infrastructure can improve agricultural production conditions and reduce agricultural production costs; welfare infrastructure can enhance rural education level, improve the absorptive capacity of agricultural practitioners, and optimize resource allocation (Aggarwal, 2018; Asturias et al., 2018; Alsan and Goldin, 2019; Hjort and Poulsen, 2019). FDI has a demonstration effect and competition effect. When FDI flows into the host country, the host country can introduce advanced technology and management experience of transnational enterprises to improve its technical level and production efficiency, and generate technology spillovers on the host country through competition effect and demonstration effect (Havranek and Irsova, 2011; Chen et al., 2022), thus promoting the improvement of agricultural technology level and market structure of the host country, and ultimately affecting the stability and efficiency of growth.
Third, environmental conditions. Environmental conditions include two secondary conditions: government governance level and institutional environment. Institutions and government governance levels are considered crucial in explaining differences in technology innovation capabilities of different countries (Rodríguez-Pose, 2013; Peng et al., 2017; Rodríguez-Pose and Zhang, 2020). Scholars generally believe that the level of government governance determines the safety of the regulatory framework, the effectiveness of laws and regulations, and the level of corruption. Efficient government governance can not only create an environment for investment and innovation activities (Tebaldi and Elmslie, 2013; Fuentelsaz et al., 2018), but also effectively attract foreign investment (Cheung and Qian, 2009) to improve technology innovation capabilities. On the other hand, a good institutional environment is conducive to the integration and aggregation of production factors such as talents, capital, investment, science and technology, so as to provide continuous factor support for the improvement of technology (Haschka et al., 2022; Zhao et al., 2022).
3. Materials and methods
3.1. Research methods
3.1.1. Meta-frontier SBM model
Reference to relevant research (Chen et al., 2023), this paper adopts the meta-frontier theory and the DEA method to build a meta-frontier SBM model to measure the agricultural technology gap between China and Africa, and clarify the causes of The gap. The meta-frontier theory was proposed by Battese et al. (2004). The meta-frontier theory can measure and compare The efficiency of clusters with different technical levels. For a certain cluster l, Its decision-making unit planning dual model can determine an envelope curve that can encompass All decision-making units of cluster l, which is the frontier edge of cluster l. The meta-frontier envelopes The frontier of clusters with different technical levels, which is the envelope curve of the frontier edge of different clusters (as shown in Figure 2). Suppose that under the input of X0, the outputs of two clusters with different technical levels on their frontier are Y1 and Y2 respectively, and the outputs on the meta-frontier curve are Y3. As it can be seen, under the given input level of X0, Y1, and Y2 of clusters with different technical levels are smaller than Y3 on the meta-frontier curve; the ratio between the optimal output of different clusters and the optimal output on the meta frontier is called the technology gap ratio (TGR). The larger The TGR value is, the smaller The gap between the cluster frontier and the meta frontier will be.
Based on the above theory, a nonparametric meta-frontier and distance function are constructed using the SBM-DEA model, and the SBM model is written in linear programming. The efficiency values of clusters and meta-frontier are the optimal values of the following linear programming problems (1) and (2):
is the efficiency value under the two frontiers that need to be measured. and are slack variables for and , respectively; m and are the number of indicators for the input and output of the decision-making unit respectively, k is variables, is the weight coefficient. Then, , y)/ . TGR is used to measure the gap between the cluster and the optimal technology. The larger the TGR value is, the closer the technical level of the cluster is to the optimal technical level. According to different sources of efficiency loss, the technology gap level (TEI) can be measured and decomposed (Chiu et al., 2012) as follows:
Among them, TGRI represents pure technical inefficiency, which is the efficiency loss caused by the technology gap among clusters, and belongs to exogenous resistance. MI means management inefficiency, which is caused by management errors and decision-making errors among clusters, and is an endogenous obstacle.
3.1.2. Configuration analysis method (fsQCA)
This paper adopts the fsQCA method to analyze the improving path of agricultural technology gap between China and Africa. The fsQCA method uses the membership degree fuzzy set to form a truth table to find out which subset of cause feature combinations the results characteristics belong to. Finally, the Boolean algebra algorithm is used to simplify these cause feature combinations. It mainly uses the set relation and the rules of logic operation to explore the influence of the predetermined cause conditions on the results in multiple cases (Kraus et al., 2018; Ding, 2022). Main reasons for choosing the fsQCA method: (1) The improving path of agricultural technology gap between China and Africa is a systematic problem caused by multiple concurrent causality, rather than the impact of any single factor. So the traditional method based on linear assumption and focusing on the “net effect” of a single condition is ineffective in providing the impact of different combinations of factors on the agricultural technology gap between China and Africa, and cannot be used to explore the mechanism between variables and results. (2) The fsQCA method can effectively mine multiple equivalent paths. Since too many African countries are taken as the studied objects in this paper, there are bound to be different equivalent paths to improve the technology gap. (3) The fsQCA method follows the asymmetric hypothesis of causality. Its application in this paper can not only mine the path of high degree condition combination, but also identify the path of non-high degree condition combination. In addition, since the data in this paper are all continuous, the fsQCA is selected for configuration analysis to mine potential paths that affect the agricultural technology gap between China and Africa, and identify multiple equivalent paths to narrow the gap.
3.2. Variable description and data source
3.2.1. Outcome variables
The result variable of this article is “agricultural technology gap - 1.” The input and output variables for calculating the agricultural technology gap are as follows: The output variable is the total output index of agriculture, forestry, animal husbandry and fishery, and the input variable is the land input index, labor input index, capital input index and raw material input index (Table 1). The above data comes from the USDA’s international agricultural productivity database. The USDA’s international agricultural productivity database is carried out by the Economic Research Service (ERS) since November 2013. The data can be found on the ERS website,1 including 180 countries or regions, from 1961. The data are updated and published every year since 2013.
3.2.2. Conditional variable
Agricultural technology innovation: This paper selects the agricultural R&D investment in African countries as the characterization variable of agricultural technology innovation. Data are obtained from the ASTI database of the International Food Policy Research Institute (IFPRI).
Agricultural producer level: Agricultural producer level is represented by the gross secondary school enrollment, due to the data lack in African countries that can be used to characterize the human capital level of agricultural producers. We drew inspiration from the research approaches of Narteh-Yoe et al. (2022), Agbloyor (2019) and Djokoto et al. (2022), and used the gross secondary school enrollment to represent agricultural producer level for the further analysis. The data of gross secondary school enrollment are from the WDI database.
FDI: FDI is expressed by the proportion of foreign capital inflows to GDP of African countries. Data come from the WDI database.
Agricultural infrastructure: referring to the method proposed by Straub and Hagiwara (2011), indicators such as transportation, energy and communication are selected to characterize the level of infrastructure, and rural infrastructure indicators are also added to the index construction. Transportation infrastructure indicators include the total railway kilometers per 10,000 people and the air traffic volume per 10,000 people. Energy infrastructure indicators include the oil equivalent of energy consumption per capita and the kilowatt hour of electricity consumption per capita. Communication infrastructure indicators include the Internet users per 100 people and the mobile wireless telephone rental per 100 people. Rural infrastructure indicators include the proportion of rural people who have access to improved water sources and the proportion of rural people who have access to improved health facilities. The above data are from the World Bank database. When quantifying, weight method proposed by Mitra et al. (2016) is applied to construct the infrastructure level index with the above data.
The equation is as follows:
In which, is the infrastructure level index, is the score of an infrastructure indicator j, is the weight of this infrastructure indicator, i represents the country, and t represents the year. By means of extreme difference to value the formula of jit value is as follows:
is the value of a certain infrastructure index j of country i in year t, is the minimum value of index j, and is the maximum value.
In terms of weight setting, referring to the method of Mitra et al. (2016), the four infrastructures are set to the same weight, i.e., 0.25, and the weight of each subdivision index is set to 0.125. According to the calculation, the infrastructure level indicator is between 0 and 100. The higher the value is, the higher the infrastructure level will be, otherwise the lower, the lower. See Table 2 for various infrastructure indicators and weights.
Government governance level: it is represented by the government governance effectiveness index. Data are from World Governance Indicators (WGI). The index interval is [−2.5, 2.5]. The higher the value is, the more effective the governance will be.
Institutional environment: It is represented by the average value of corruption index, regulatory level, laws and regulations level, and policy stability. Data come from WGI database. According to Kaufmann et al. (2011), the index interval is [−2.5, 2.5]. The larger the value is, the better the institutional environment will be.
All the variables are annual. The period of analysis is 2003–2019. The scope of the study is the technology gap between China and Africa. China has published its official direct investment data from 2003, so that this study uses data from 2003. Also, the data availability is considered, so the period of 2003–2019 is selected. The sample includes the following countries: South Africa, Nigeria, Angola, Kenya, Ethiopia, Cameroon, Cote d’Ivoire, Tanzania, Zambia, Uganda, Gabon, Botswana, the Democratic Republic of the Congo, Congo, Senegal, Mozambique, Namibia, Mauritius, Mali, Madagascar, Zimbabwe, Chad, Benin, Rwanda, Niger, Malawi, Mauritania, Togo, Lesotho, Burundi, Central Africa, Cape Verde. The descriptive results of the above variables are shown in Table 3.
4. Results and analysis
4.1. Analysis of agricultural technology gap between China and Africa
First of all, according to “3.1.1 meta-frontier SBM model,” the technology gap ratio (TGR), the agricultural technology gap (TEI) between China and Africa are calculated, and the agricultural technology gap is decomposed into pure technical inefficiency (TGRI) and management inefficiency (MI) to analyze the root causes of the agricultural technology gap in African countries. From the results, the average of TGR in China from 2003 to 2019 was 1, indicating that China’s agricultural technology has always been on the meta-frontier, without TGRI and MI. The average of TGR in African countries from 2003 to 2019 was 0.932, indicating that there is still a certain gap between the agricultural technology frontier and the meta-frontier in African countries, with TGRI and MI. In addition, according to the proportion of pure technical inefficiency (TGRI) and management inefficiency (MI) in the agricultural technology gap (TEI), the focus of improving agricultural technology efficiency in African countries is determined. If the proportion of TGRI or MI is less than 50%, the importance degree is recorded as “▲.” If the proportion of TGRI or MI is between 50 and 85%, the importance degree is recorded as “▲▲.” If the proportion of TGRI or MI exceeds 85%, the importance degree is recorded as “▲▲▲.” Figure 3 shows the evolution trend of the agricultural technology gap value and its decomposition items between African countries and China. Table 4 lists the agricultural technology gap value and its decomposition results between African countries and China.
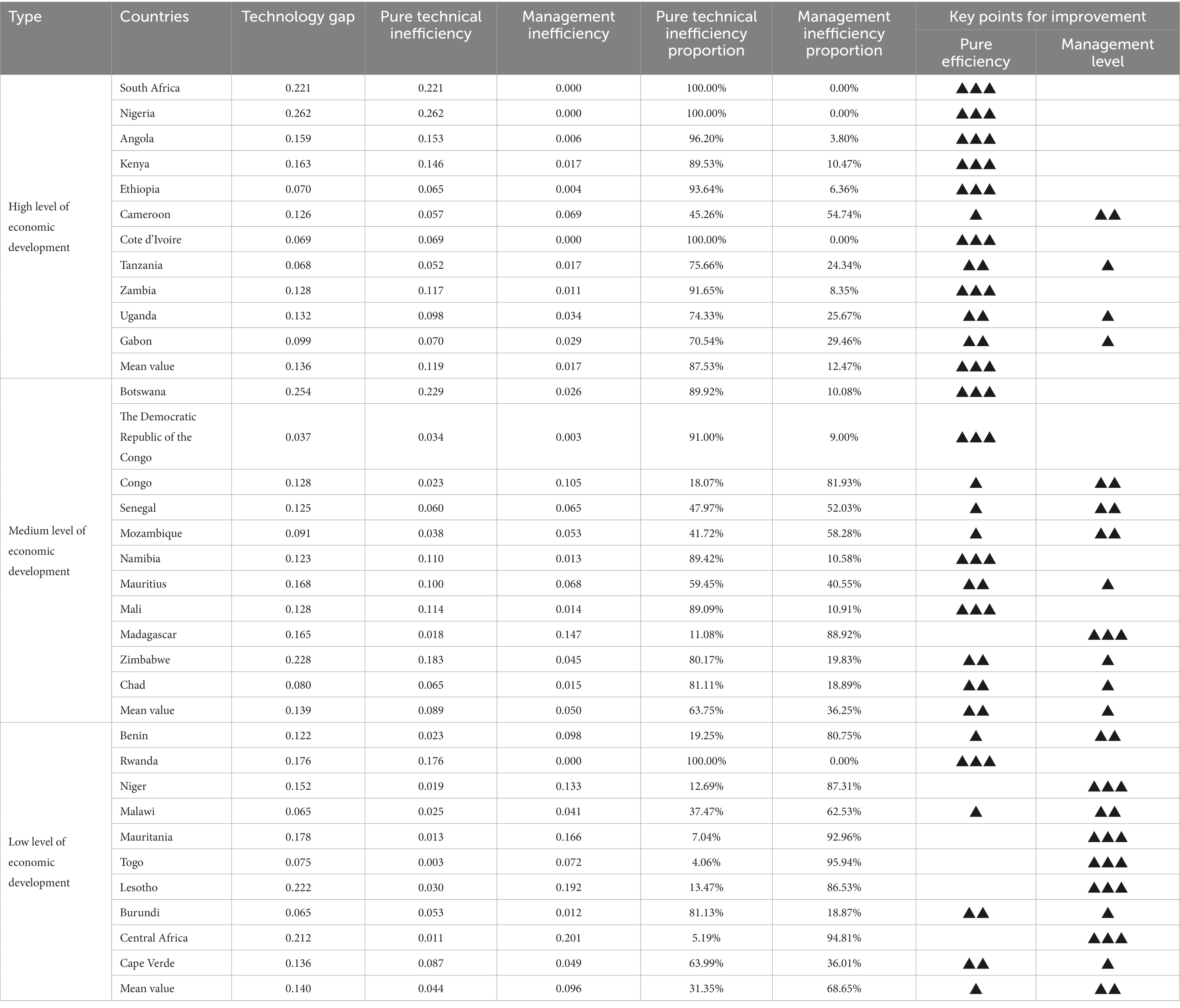
Table 4. Technology gap among African countries with different levels of economic development and its decomposition.
As can be seen from Figure 3, the overall agricultural technology gap between China and Africa shows a narrowing trend from 0.233 in 2003 to 0.127 in 2019. In terms of the decomposition of the agricultural technology gap between China and Africa, the pure technical inefficiency rate decreased from 0.161 in 2003 to 0.082 in 2019, and the management inefficiency rate decreased from 0.072 in 2003 to 0.045 in 2019. In general, the main reason for the narrowing of the agricultural technology gap between China and Africa from 2003 to 2019 is the reduction of pure technical inefficiency, while the reduction of management inefficiency is not obvious. In addition, it can be seen that although the overall agricultural technology gap between China and Africa shows a narrowing trend from 2003 to 2019, it began to widen again in 2015, which verifies the necessity of focusing on the agricultural technology gap between China and Africa in this paper.
Table 4 shows that the average value of agricultural technology gap between China and Africa from 2003 and 2019 is 0.138, of which the pure technical inefficiency rate is 0.085, accounting for about 61.59%, while the management inefficiency rate is 0.053, accounting for about 38.41%. The pure technical inefficiency rate of African countries with high-level economic development is 0.119, accounting for about 87.53%, and the management inefficiency rate is 0.017, accounting for about 12.47%; the pure technical inefficiency rate of African countries with medium-level economic development is 0.089, accounting for 63.75%, and the management inefficiency rate is 0.050, accounting for 36.25%; the pure technology inefficiency rate of African countries with low-level economic development is 0.044, accounting for 31.35%, and the management inefficiency rate is 0.096, accounting for 68.65%. The results demonstrate that, on the whole, the agricultural technology gap between China and Africa in 2003–2019 is mainly caused by the pure technical inefficiency rate, but there are some differences for African countries with different economic development levels. For African countries with high economic development level, the agricultural technology gap with China is mainly caused by the pure technical inefficiency rate; for African countries with medium economic development level, both pure technical inefficiency rate and management inefficiency rate cause the gap with China; for those countries with low economic development level, the gap with China is mainly caused by management inefficiency.
From the perspective of African countries: (1) The agricultural technology gap between African countries such as South Africa, Nigeria, Angola, Kenya, Ethiopia, Cote d’Ivoire, Zambia, Botswana, the Democratic Republic of the Congo, Namibia, Mali, Rwanda, etc. and China is mainly caused by pure technical inefficiency, while the efficiency loss caused by ineffective management is relatively low. These countries have an excellent performance in agricultural production management, but there is strong external resistance, and the pure technical level needs to be improved urgently. At the same time, it can be noted that these countries all have a medium or high level of economic development. Therefore, these countries should rely on their own good economic conditions, tilt to the agricultural field, strengthen their agricultural science and technology innovation and agricultural technology introduction, so as to improve the level of agricultural pure technology and narrow the agricultural technology gap with China. (2) The gap between African countries such as Madagascar, Niger, Mauritania, Togo, Lesotho and Central Africa, etc. and China in agricultural technology is mainly caused by management inefficiency, while the loss of pure technical inefficiency rate is relatively low, indicating that these countries have many deficiencies in agricultural production operation and decision-making, and are faced with strong endogenous dynamic constraints. Moreover, it can be noted that these countries mainly have a low level of economic development. Therefore, they should improve their agricultural production decision-making level by means of strengthening agricultural technology training and agricultural technology promotion, so as to narrow the agricultural technology gap with China. (3) The agricultural technology gap between countries such as Cameroon, Tanzania, Uganda, Gabon, Congo, Senegal, Mozambique, Mauritius, Zimbabwe, Chad, Benin, Malawi, Burundi, Cape Verde, etc. and China stems from TGRI and MI. These countries mainly have a medium or low level of economic development. Technology gaps caused by the inefficiency of pure technology and management in these countries account for a certain proportion. In the future, it is necessary to start from the national agricultural pure technical level and its own production management decision-making to eliminate the internal and external resistance that affect the agricultural technology gap. While improving the internal management and operation system construction, they should create a good environment for agricultural technology development and improve their agricultural technology level.
4.2. Optimization path of agricultural technology gap between China and Africa
On the basis of measuring the agricultural technology gap between African countries and China, this paper takes African countries in 2019 as samples, takes (China Africa Agricultural Technology Gap-1) as the outcome variable, selects six conditional variables of agricultural technology innovation level, agricultural labor level, agricultural infrastructure, FDI, government governance level and institutional environment, and uses the fsQCA method to explore how far the agricultural technology gap between China and Africa can be narrowed. Due to the severe missing values in the data of the gross secondary school enrollment in African countries, we processed the data as follows: First, interpolation method was performed on the gross secondary school enrollment data of countries with less severe data missing (Nigeria, Ethiopia, Uganda, the Democratic Republic of the Congo, Mali, Benin, Niger, Togo). Second, sample deletion operations were carried out on countries with severe data loss and inability to perform interpolation processing (Angola, Kenya, Zambia, Botswana, Congo, Namibia, Zimbabwe, Central Africa). Then, we obtained the gross secondary school enrollment data of 24 African countries, including Benin, Burundi, Cabo Verde, Chad, Côted’ Ivore, Gabon, etc.
4.2.1. Data calibration
The fsQCA method is based on the set theory to convert variables into set intervals between [0 ~ 1], and uses the direct calibration method to calibrate the data (Fiss, 2011). According to the sample data, outcome variables and six conditional variables are calibrated based on the four value set, namely, “completely non-subordinate” (25%), “midpoint” (50%), and “completely subordinate” (75%). The calibration of non-high configuration is realized by the non-set of high configuration. The data calibration results are shown in Table 5 (since this paper aims to study how to narrow the agricultural technology gap between China and Africa, therefore, the outcome variable is China Africa Agricultural Technology Gap-1):
4.2.2. Analysis of necessary conditions
After calibrating the data, the consistency and coverage of the six conditional variables that affect the agricultural technology gap between China and Africa are measured with the fsQCA3.0 software, so as to conduct the necessary condition analysis. That is, if the consistency of a conditional variable is greater than 0.9, the conditional variable is a necessary condition for the outcome variable. According to the results in Table 6, the consistency level of the six conditional variables is lower than 0.9, which indicates that in this study, all conditional variables are not necessary for the outcome variables, and it is difficult for any of these conditional variables to narrow the agricultural technology gap between China and Africa alone. This suggests that the agricultural technology gap between China and Africa is a complex process, and its optimization path is affected by multiple factors, rather than a single factor. And it also verifies the necessity of configuration analysis under the framework of the TOE theory.
4.2.3. Conditional configuration analysis
Through set model operation, the configuration of 6 conditional variables is well completed, and 4 configurations were obtained to explain the narrowing of agricultural technology gap between China and Africa. As shown in Table 7, the overall consistency of these 4 configurations is 0.906887, higher than the acceptable reference value of 0.8, and the overall coverage is 0.430131, indicating that the 4 configurations can cover and interpret more than 40% of the cases. Therefore, these 4 configurations can be considered as the sufficient condition combination of the outcome variables in this paper. By observing the distribution of conditional configurations and combining the comparison results between the intermediate solution and the simple solution, it can be found that none of the 6 elements such as agricultural technology innovation can constitute the necessary conditions to narrow the agricultural technology gap between China and Africa separately, but agricultural technology innovation and institutional environment play a major role in the 4 configurations. Therefore, centering on these two key conditional variables, this paper summarizes the 4 paths narrowing the agricultural technology gap between China and Africa into 3 models.
(1) “Technology-environment” driving mode. This mode corresponds to Configuration 1 in Table 7. Configuration 1 is expressed by the formula: Agricultural technology innovation * ~ Agricultural infrastructure * ~ FDI * Government governance level * Institutional environment, in which agricultural technology innovation, government governance level appears as the core conditions, institutional environment appear as auxiliary condition. This configuration demonstrates that in the case of good agricultural technology innovation, government governance and institutional environment in African countries, even if agricultural infrastructure and FDI are poor, agricultural science and technology progress can be achieved, thus narrowing the agricultural technology gap with China. This configuration can explain 19.33% of the cases of narrowing the agricultural technology gap between China and Africa, of which about 6.83% can only be explained by this configuration. The typical African countries corresponding to this configuration such as Togo attach great importance to agricultural development, agricultural inputs and technology introduction, enhancing the ability of agricultural technology innovation, and narrowing the agricultural technology gap with China. For example, Togo holds a national farmers’ forum every year, increase investment in the agricultural field, aiming to improve agricultural production efficiency (Ministry of Commerce of the People's Republic of China (MCPRC), 2021). From the core conditions, Configuration 1 can be referred to the “technology environment” driving mode.
(2) “Technology-organization” driving mode. This mode corresponds to configuration 3 in Table 7, where two key conditions of agricultural technology innovation and agricultural infrastructure appear, and other conditions are absent. This configuration can explain 9.17% of the cases of narrowing the agricultural technology gap between China and Africa, of which about 6.75% can only be explained by this configuration. Configuration 3 is expressed by the formula: Agricultural technology innovation * ~ Agricultural producer level * Agricultural infrastructure * ~ FDI * ~ Government governance level * ~ Institutional environment. This configuration shows that even if African countries have poor agricultural producers, FDI, government governance and institutional environment, they can achieve agricultural scientific and technological progress by relying on agricultural technology innovation and agricultural infrastructure, thus narrowing the agricultural technology gap with China. Typical African countries corresponding to this configuration such as Gabon, Mauritania, etc. These countries will vigorously develop agricultural industry and increase infrastructure construction. For example, Gabon has formulated the development direction of “green Gabon” and increased infrastructure investment [Ministry of Foreign Affairs of the People's Republic of China (MFAPRC), 2022]. From the perspective of core conditions, Configuration 3 can be referred to the “technology organization” driving mode.
(3) “Organization-environment” driving mode. This mode corresponds to Configuration 4 in Table 7. Configuration 4 is expressed by the formula as follows: ~Agricultural technology innovation * Agricultural producer level * Agricultural infrastructure * FDI * Government governance level * Institutional environment, in which agricultural producer level, Agricultural infrastructure and institutional environment appear as the core conditions, FDI and Government governance level appear as auxiliary conditions. This configuration shows that even if African countries have poor agricultural technology, they can also achieve agricultural scientific and technological progress by improving the level of agricultural producers, improving agricultural infrastructure and optimizing the institutional environment, thus narrowing the technology gap with China. This configuration can explain 12.09% of the cases of narrowing the agricultural technology gap between China and Africa, of which about 8.17% can only be explained by this configuration. Typical African countries corresponding to this configuration such as Senegal, are committed to improving infrastructure construction, possessing high-level education, and a stable institutional environment. Since the launch of the “Revitalization Plan for Senegal” in 2014, infrastructure construction has become an important task for Senegal. At the same time, Senegal attaches great importance to education, with an enrollment rate of up to 90% in primary and secondary schools, and has one of the oldest higher education institutions in Africa, Université Cheikh Anta Diop de Dakar. In addition, Senegal has a good institutional environment, its democratization process has been continuously accelerating since 1990. From the perspective of core conditions, Configuration 4 can be referred to the “organization environment” driving mode.
(4) Except Configuration 1, Configuration 3, and Configuration 4, Configuration 2 can expressed by the formula: Agricultural technology innovation * Agricultural producer level * ~ FDI * Government governance level * Institutional environment, in which agricultural technology innovation, agricultural producer level, government governance level, institutional environment are all appear as the auxiliary conditions. This configuration can explain 20.84% of the cases of narrowing the agricultural technology gap between China and Africa, of which about 6.92% can only be explained by this configuration. Configuration 2 indicates that although the coupling of agricultural technology innovation, agricultural producer level, government management level, and institutional environment can affect the outcome variable, however, these four conditional variables only appear as auxiliary conditions. Therefore, the causality between this configuration and the outcome variable is weak, so this configuration cannot be referred to the “technology organization environment” driving model.
5. Discussion
Firstly, this paper found that from 2003 to 2019, the agricultural technology gap between Africa and China showed a narrowing trend. This conclusion verifies the narrowing trend in the technology gap between Africa and OECD and other countries (You et al., 2020; Gebrerufael, 2021). However, this study not only focuses on the agricultural field in Africa, but also explores the specific causes of the agricultural technology gap between African countries and China (whether the source of the technology gap is management inefficiency or technical inefficiency). In addition, we further analyzed the causes of the agricultural technology gap between African countries (classified by economic development levels) and China, which will help African countries to narrow the agricultural technology gap with China more accurately. Meanwhile, the paper also confirmed that the transfer of agricultural technology in Africa in recent years had positive impacts on agricultural production practice, which verifies the conclusions of Garnett et al. (2013), Gouvea et al. (2022), and Tyczewska et al. (2023).
Secondly, this paper also found that agricultural technology innovation and institutional environment optimization were the key conditional variables to narrow the agricultural technology gap between China and Africa. Around these two key conditional variables, there were five paths to narrow the agricultural technology gap between African countries and China. This conclusion confirms that technological innovation are crucial to narrow the technological gap (Verspagen, 1991). However, the study of how to narrow the agricultural technology gap between African countries and China is a complex and systematic issue, which cannot be achieved by single factors. Therefore, we use the configuration analysis method (fsQCA) to explore the multiple concurrent causality of narrowing the agricultural technology gap between China and Africa, it overcomes the shortage of using regression method to discuss the influence of a single factor on the technology gap (Ahmed and Krishnasamy, 2013; Landini and Malerba, 2017), and puts forward five paths to narrow the technology gap between China and Africa.
This paper has the following research contributions: First of all, this paper adopts the meta-frontier theory and the DEA method to build a meta-frontier SBM model to study the agricultural technology gap between China and Africa, which not only realizes the efficiency measurement and comparison of different clusters with different technical levels, but also expands the existing research on measuring technology gap using meta-frontier SBM model and subdivides the sources of technology gap. Secondly, based on the configuration thinking, this paper introduces qualitative analysis method into the study on narrowing the agricultural technology gap between China and Africa, and uses fsQCA to explore the multiple concurrent causality of narrowing the gap, which overcomes the deficiency of using regression methods to discuss the impact of single factor on technology gap, and proposes potential combinations and multiple paths of elements to narrow technology gap between China and Africa. It explains the complex causal mechanism of narrowing the agricultural technology gap between China and Africa, and improves and enriches relevant theories and practices.
6. Conclusion
In order to clarify the agricultural technology gap between Africa and China, and explore the conditions for narrowing the gap, this paper first takes China and 32 African countries from 2003 to 2019 as samples, and uses meta-frontier SBM to measure the agricultural technology gap between those African countries and China. On this basis, samples of 24 African countries are taken and six conditional variables including agricultural technology innovation, agricultural producer level, agricultural infrastructure, FDI, government governance level and institutional environment are selected based on the TOE theory, and the fsQCA method is adopted to explore the multiple paths of narrowing the agricultural technology gap between China and Africa. The main conclusions are as follows:
1. (1) From the perspective of agricultural technology gap between China and Africa: ① From 2003 to 2019, the overall agricultural technology gap between China and Africa shows a narrowing trend, which mainly due to the reduction of pure technical inefficiency while the reduction of management inefficiency is not obvious. In addition, although the overall agricultural technology gap has been narrowing from 2003 to 2019, it began to widen again in 2015, which verifies the necessity of focusing on the agricultural technology gap between China and Africa in this paper. ② Sources of agricultural technology gap in African countries with different economic development levels are different. Technical gaps in countries with high-level economic development are mainly caused by pure technical inefficiency, gaps in countries with middle-level economic development are caused by pure technical inefficiency and management inefficiency, while gaps in countries with low-level economic development are mainly caused by management inefficiency.
2. (2) Through configuration analysis, it is found that none of the 6 conditional variables, such as agricultural technology innovation, can independently constitute the necessary conditions for narrowing the agricultural technology gap between China and Africa. Therefore, it is necessary to explore the path to narrow the agricultural technology gap between China and Africa from the perspective of configuration. However, agricultural technology innovation and institutional environment play a key role in the four configurations.
3. (3) Focusing on two key conditional variables of agricultural technology innovation level and institutional environment, there are four paths to narrow the agricultural technology gap between China and Africa, which can be summarized into three driving modes: “technology environment” driving mode, “technology organization” driving mode and “organization environment” driving mode. At present, there is no balanced driving mode of “technology organization environment” in African countries.
Owing to the different economic development levels, geographical locations, agricultural resource endowment conditions, agricultural foundations, development opportunities, etc., African countries have different effects and paths to achieve agricultural technological progress and narrow the agricultural technology gap with China. Therefore, African countries should first identify the obstacles and difficulties hindering the advance of science and technology according to their current development situation of “technical conditions-organizational conditions-environmental conditions,” then select and adjust the TOE development model in the light of their own specific scenarios to give policy preferences and support from the two aspects of agricultural technology innovation level and institutional environment, so as to stimulate the endogenous power of agricultural scientific and technological progress in African countries. In addition, African countries should further strengthen the integration of technical conditions, organizational conditions and environmental conditions to produce a superposition effect with the balance of structure and function, and jointly promote agricultural scientific and technological progress in African countries.
This article also has some limitations: Firstly, based on the TOE theory and the research experience of current literature, this paper selects six representative conditional variables, but the selection of these conditional variables are inconsistencies in the current literature, and the interpretability of these conditional variables remains to be verified; secondly, due to the very imperfect disclosure of agricultural data in African countries, we can only use the gross secondary school enrollment to approximately replace the conditional variables of agricultural producer level.
Data availability statement
The original contributions presented in the study are included in the article/supplementary material, further inquiries can be directed to the corresponding authors.
Author contributions
QL and JW: conceptualization, methodology, software, validation, investigation, resources, data analysis, writing—original draft preparation, writing—review and editing, visualization, and funding acquisition. SM and JL: conceptualization, software, writing—review and editing, supervision, validation, and project administration. All authors contributed to the article and approved the submitted version.
Funding
“Research on the Mechanisms and Impacts of Sino-African Agricultural Technology Transfer” (7171101119).
Conflict of interest
The authors declare that the research was conducted in the absence of any commercial or financial relationships that could be construed as a potential conflict of interest.
Publisher’s note
All claims expressed in this article are solely those of the authors and do not necessarily represent those of their affiliated organizations, or those of the publisher, the editors and the reviewers. Any product that may be evaluated in this article, or claim that may be made by its manufacturer, is not guaranteed or endorsed by the publisher.
Footnotes
References
Agbloyor, E. (2019). Foreign direct investment, political business cycles and welfare in Africa. J. Int. Dev. 31, 345–373. doi: 10.1002/jid.3408
Aggarwal, S. (2018). Do rural roads create pathways out of poverty? Evidence from India. J. Dev. Econ. 133, 375–395. doi: 10.1016/j.jdeveco.2018.01.004
Ahmed, E., and Krishnasamy, G. (2013). Are Asian technology gaps due to human capital quality differences? Econ. Model. 35, 51–58. doi: 10.1016/j.econmod.2013.06.032
Ahsan, H., and Haque, M. (2017). Threshold effects of human capital: schooling and economic growth. Econ. Lett. 156, 48–52. doi: 10.1016/j.econlet.2017.04.014
Alsan, M., and Goldin, C. (2019). Watersheds in child mortality: the role of effective water and sewerage infrastructure, 1880–1920. J. Polit. Econ. 127, 586–638. doi: 10.1086/700766
Amankwah-Amoah, J., Chen, X., Wang, X., and Khan, Z. (2019). Overcoming institutional voids as a pathway to becoming ambidextrous: the case of China’s Sichuan telecom. Long Range Plan. 52:101871. doi: 10.1016/j.lrp.2019.02.004
Asturias, J., García-Santana, M., and Ramos, R. (2018). Competition and the welfare gains from transportation infrastructure: evidence from the Golden quadrilateral of India. J. Eur. Econ. Assoc. 17, 1881–1940. doi: 10.1093/jeea/jvy039
Atesagaoglu, O., Elgin, C., and Oztunali, O. (2017). TFP growth in Turkey revisited: the effect of informal sector. Central Bank Rev. 17, 11–17. doi: 10.1016/j.cbrev.2017.02.002
Battese, G., Rao, D., and O'Donnell, C. (2004). A Metafrontier production function for estimation of technical efficiencies and technology gaps for firms operating under different technologies. J. Prod. Anal. 21, 91–103. doi: 10.1023/B:PROD.0000012454.06094.29
Bednarek, Z. (2016). Pure technology gaps and production predictability. Q. Rev. Econ. Finance 59, 39–50. doi: 10.1016/j.qref.2015.02.009
Chaffai, M. (2020). Hyperbolic distance function, technical efficiency and stability to shocks: a comparison between Islamic banks and conventional banks in MENA region. Glob. Financ. J. 46:100485. doi: 10.1016/j.gfj.2019.100485
Chatterjee, S., Rana, N., Dwivedi, Y., and Baabdullah, A. (2021). Understanding ai adoption in manufacturing and production firms using an integrated tam-toe model. Technol. Forecast. Soc. Chang. 170:120880. doi: 10.1016/j.techfore.2021.120880
Chau, K., and Tam, Y. (1997). Factors affecting the adoption of open systems: an exploratory study. MIS Q. 21, 1–24. doi: 10.2307/249740
Chen, Z., Chen, Z., and Ma, J. (2022). Analysis on FDI productivity spillovers in the industrial sectors - an empirical study based on time series input-output tables in China. Shanghai. J. Econ. 8, 46–59. doi: 10.19626/j.cnki.cn31-1163/f.2022.08.003
Chen, Y., Zhang, R., and Miao, J. (2023). Unearthing marine ecological efficiency and technology gap of China’s coastal regions: a global meta-frontier super SBM approach. Ecol. Indic. 147:109994. doi: 10.1016/j.ecolind.2023.109994
Cheung, Y., and Qian, X. (2009). Empirics of China's outward direct investment. Pacific Econ. Rev. 14, 312–341. doi: 10.1111/j.1468-0106.2009.00451.x
Chiu, C., Liou, J., Wu, P., and Fang, C. (2012). Decomposition of the environmental inefficiency of the Meta-frontier with undesirable output. Energy Econ. 34, 1392–1399. doi: 10.1016/j.eneco.2012.06.003
Cyranoski, D. (2018). China to train African scientists as part of $60-billion development plan. Nature 562, 15–16. doi: 10.1038/d41586-018-06722-5
Dadhich, M., and Hiran, K. (2022). Empirical investigation of extended TOE model on corporate environment sustainability and dimensions of operating performance of SMEs: a high order PLS-ANN approach. J. Clean. Prod. 363:132309. doi: 10.1016/j.jclepro.2022.132309
Ding, H. (2022). What kinds of countries have better innovation performance?–a country-level fsQCA and NCA study. J. Innov. Knowl. 7:100215. doi: 10.1016/j.jik.2022.100215
Djokoto, J., Gidiglo, F., Srofenyo, F., and Agyeiwaa-Afrane, A. (2022). Human development effects of food manufacturing foreign direct investment. Int. J. Food Agric. Econ. 10, 23–39. doi: 10.22004/ag.econ.319341
ECOSOC. (2022). Progress report on the 10 year framework of programmes on sustainable consumption and production patterns. In: United Nations conference on sustainable development, Rio, Brazil. available at: https://sdgs.un.org/sites/default/files/publications/1444HLPF_10YFP2.pdf (accessed July 11, 2022).
Eltis, R. (1978). Modern capitalism: its growth and transformation. Econ. J. 88:585. doi: 10.2307/2232063
FAO, IFAD, UNICEF, WFP, and WHO. (2022). “The state of food security and nutrition in the world 2022,” in Repurposing food and agricultural policies to make healthy diets more affordable Rome: FAO.
Fiss, P. (2011). Building better causal theories: a fuzzy set approach to typologies in organization research. Acad. Manag. J. 54, 393–420. doi: 10.5465/AMJ.2011.60263120
Fuentelsaz, L., Maicas, J., and Montero, J. (2018). Entrepreneurs and innovation: the contingent role of institutional factors. Int. Small Bus. J. 36, 686–711. doi: 10.1177/0266242618766235
Garnett, T., Appleby, M., Balmford, A., Bateman, I., Benton, T., Bloomer, P., et al. (2013). Sustainable intensification of agriculture: premises and policies. Science 341, 33–34. doi: 10.1126/science.1234485
Gebrerufael, S. (2021). Dynamics of technology gap between OECD and African countries: a structural estimation. Sci. Afr. 11:e00674. doi: 10.1016/j.sciaf.2020.e00674
Gouvea, R., Kapelianis, D., Li, S., and Terra, B. (2022). Innovation, ICT & food security. Glob. Food Sec. 35:100653. doi: 10.1016/j.gfs.2022.100653
Guo, R., Wu, X., Xiong, L., and Chen, X. (2012). Technology gap, technology competition and MNCs' technology licensing strategies: a transaction cost theory perspective. Int. Symp. Manag. Technol. 2012, 595–598. doi: 10.1109/ISMOT.2012.6679542
Ha, J., Kim, Y., and Lee, J. (2009). The optimal structure of technology adoption and creation: basic research vs. development in the presence of distance to frontier. Asian Dev. Bank 163:1611476. doi: 10.2139/SSRN.1611476
Haschka, R., Herwartz, H., Struthmann, P., Tran, V., and Walle, Y. (2022). The joint effects of financial development and the business environment on firm growth: evidence from Vietnam. J. Comp. Econ. 50, 486–506. doi: 10.1016/j.jce.2021.11.002
Havranek, T., and Irsova, Z. (2011). Estimating vertical spillovers from FDI: why results vary and what the true effect is. J. Int. Econ. 85, 234–244. doi: 10.1016/j.jinteco.2011.07.004
He, Y., Han, Q., and Zeng, Y. (2019). A research on the impact of indigenous innovation on international competitiveness of China’s manufacturing industry. Science research. Management 40, 33–46. (In Chinese). doi: 10.19571/j.cnki.1000-2995.2019.07.004
Hjort, J., and Poulsen, J. (2019). The arrival of fast internet and employment in Africa. Am. Econ. Rev. 109, 1032–1079. doi: 10.1257/aer.20161385
Jordaan, J. (2017). Producer firms, technology diffusion and spillovers to local suppliers: examining the effects of foreign direct investment and the technology gap. Environ. Plann. 49, 2718–2738. doi: 10.1177/0308518X17731942
Kaufmann, D., Kraay, A., and Mastruzzi, M. (2011). The worldwide governance indicators: methodology and analytical issues. Soc. Sci. Elect. Publish 3, 220–246. doi: 10.1017/S1876404511200046
Kijima, Y., Ito, Y., and Otsuka, K. (2012). Assessing the impact of training on lowland rice productivity in an african setting: evidence from Uganda. World Dev. 40, 1610–1618. doi: 10.1016/j.worlddev.2012.04.008
Kirui, O., and Kozicka, M. (2018). Vocational education and training for farmers and other actors in the Agri-food value chain in Africa. Working paper 164.
Kontolaimou, A., Giotopoulos, I., and Tsakanikas, A. (2016). A typology of European countries based on innovation efficiency and technology gaps: the role of early-stage entrepreneurship. Econ. Model. 52, 477–484. doi: 10.1016/j.econmod.2015.09.028
Kraus, S., Ribeiro-Soriano, D., and Schüssler, M. (2018). Fuzzy-set qualitative comparative analysis (fsQCA) in entrepreneurship and innovation research – the rise of a method. Int. Entrep. Manag. J. 14, 15–33. doi: 10.1007/s11365-017-0461-8
Landini, F., and Malerba, F. (2017). Public policy and catching up by developing countries in global industries: a simulation model. Camb. J. Econ. 41, 927–960. doi: 10.1093/cje/bex017
Li, J., Dong, K., and Dong, X. (2022). Green energy as a new determinant of green growth in China: the role of green technological innovation. Energy Econ. 114:106260. doi: 10.1016/j.eneco.2022.106260
Li, J., and Lin, Q. (2022). Can the adjustment of china’s grain purchase and storage policy improve its green productivity? Int. J. Environ. Res. Public Health 19:6310. doi: 10.3390/ijerph19106310
Li, J., and Lin, Q. (2023). Threshold effects of green technology application on sustainable grain production: evidence from China. Front. Plant Sci. 14:1107970. doi: 10.3389/fpls.2023.1107970
Liang, T., Zhang, Y., and Qiang, W. (2022). Does technological innovation benefit energy firms’ environmental performance? The moderating effect of government subsidies and media coverage. Technol. Forecast. Soc. Chang. 180:121728. doi: 10.1016/j.techfore.2022.121728
Lin, Q., and Mao, S. (2023). Independent innovation and transformation of scientific and technological achievements of enterprises: subsidies or policies. Studies in science of. Science 41, 70–79. doi: 10.16192/j.cnki.1003-2053.20220222.004
Ministry of Commerce of the People's Republic of China (MCPRC) (2021). Togo guidelines of the Ministry of Commerce of the People's Republic of China 2021. Available at: http://tg.mofcom.gov.cn/index.shtml (accessed January 1, 2021).
Ministry of Foreign Affairs of the People's Republic of China (MFAPRC). (2022). Overview of Gabon. Available at: https://www.mfa.gov.cn/web/gjhdq_676201/gj_676203/fz_677316/1206_677800/1206x0_677802/ (accessed January 1, 2022).
Mitra, A., Sharma, C., and Véganzonès-Varoudakisc, M. (2016). Infrastructure, information & communication technology and firms' productive performance of the Indian manufacturing. J. Policy Model 38, 353–371. doi: 10.1016/j.jpolmod.2016.02.013
Narteh-Yoe, S., Djokoto, J., and Pomeyie, P. (2022). Aid, domestic and foreign direct investment in small states. Econ. Res. Ekonomska Istraživanja 18, 1–18. doi: 10.1080/1331677X.2022.2160998
Nonaka, I. (1994). A dynamic theory of organizational knowledge creation. Organ. Sci. 5, 14–37. doi: 10.1287/orsc.5.1.14
Okunade, O., Alimi, A., and Olayiwola, A. (2022). Do human capital development and globalization matter for productivity growth? New evidence from Africa. Soc. Sci. Human. Open. 6:100291. doi: 10.1016/j.ssaho.2022.100291
Olasehinde, T., Jin, Y., Qiao, F., and Mao, S. (2023). Marginal returns on Chinese agricultural technology transfer in Nigeria: who benefits more? China Econ. Rev. 78:101935. doi: 10.1016/j.chieco.2023.101935
Oliveir, T., and Martins, M. (2011). Literature review of information technology adoption models at firm level. Electr. J. Inform. Syst. Eval. 14, 110–121.
Peng, M., Ahlstrom, D., Carraher, S., and Shi, W. (2017). History and the debate over intellectual property. Manag. Organ. Rev. 13, 15–38. doi: 10.1017/mor.2016.53
Rodríguez-Pose, A. (2013). Do institutions matter for regional development? Reg. Stud. 47, 1034–1047. doi: 10.1080/00343404.2012.748978
Rodríguez-Pose, A., and Zhang, M. (2020). The cost of weak institutions for innovation in China. Technol. Forecast. Soc. Change 153:119937. doi: 10.1016/j.techfore.2020.119937
Straub, S., and Hagiwara, A. (2011). Infrastructure and growth in developing Asia. Asian Dev. Rev. 28, 119–156. doi: 10.2139/ssrn.1783168
Tanko, M., and Ismaila, S. (2021). How culture and religion influence the agriculture technology gap in northern Ghana. World Dev. Perspect. 22:100301. doi: 10.1016/j.wdp.2021.100301
Tebaldi, E., and Elmslie, B. (2013). Does institutional quality impact innovation? Evidence from cross-country patent grant data. Appl. Econ. 45, 887–900. doi: 10.1080/00036846.2011.613777
Tornatzky, L., Fleischer, M., and Chakrabarti, A. (1990). Processes of technological innovation[M]. Lexington: Lexington books. 1–20.
Tyczewska, A., Twardowski, T., and Woźniak-Gientka, E. (2023). Agricultural biotechnology for sustainable food security. Trends Biotechnol. 41, 331–341. doi: 10.1016/j.tibtech.2022.12.013
UN COMTRADE (2018). UN Comtrade Database. Available at: https://comtrade.un.org/ (Accessed July 10, 2019).
Verspagen, B. (1991). A new empirical approach to catching up or falling behind. Struct. Chang. Econ. Dyn. 2, 359–380. doi: 10.1016/S0954-349X(05)80008-6
Walker, R. (2014). Internal and external antecedents of process innovation: a review and extension. Public Manag. Rev. 16, 21–44. doi: 10.1080/14719037.2013.771698
Walker, K., and Hofstetter, S. (2016). Study on agricultural technical and vocational education and training (ATVET) in developing countries. In the Swiss Agency for Development and Cooperation (SDC). Available at: https://www.shareweb.ch/site/Agriculture-and-Food-Security/focusareas/Documents/ras_capex_ATVET_Study_2016.pdf.
Wang, Z., and Zhu, Y. (2020). Do energy technology innovations contribute to CO2 emissions abatement? A spatial perspective. Sci. Total Environ. 726:138574. doi: 10.1016/j.scitotenv.2020.138574
Xie, J., and Zhang, N. (2020). Technology gap, technology spillover and technological progress: an analysis based on Sino-US trade data. World Econ. Stud. 1, 12-24–12-135. doi: 10.13516/j.cnki.wes.2020.01.002
You, K., Bianco, S., and Amankwah-Amoah, J. (2020). Closing technological gaps to alleviate poverty: evidence from 17 sub-Saharan African countries. Technol. Forecast. Soc. Chang. 157:120055. doi: 10.1016/j.techfore.2020.120055
Zhao, X., Yi, C., Zhan, Y., and Guo, M. (2022). Business environment distance and innovation performance of EMNEs: the mediating effect of R&D internationalization. J. Innov. Knowl. 7:100241. doi: 10.1016/j.jik.2022.100241
Keywords: China, Africa, technology gap, configuration analysis, fsQCA, MFA
Citation: Lin Q, Wang J, Mao S and Li J (2023) Research on agricultural technology gap between China and Africa and its optimization path: based on meta-frontier SBM and fsQCA. Front. Sustain. Food Syst. 7:1174989. doi: 10.3389/fsufs.2023.1174989
Edited by:
Gheorghe Adrian Martau, University of Agricultural Sciences and Veterinary Medicine of Cluj-Napoca, RomaniaReviewed by:
Guangqin Li, Anhui University of Finance and Economics, ChinaLuisa Marti, Universitat Politècnica de València, Spain
Justice Gameli Djokoto, Central University (Ghana), Ghana
Copyright © 2023 Lin, Wang, Mao and Li. This is an open-access article distributed under the terms of the Creative Commons Attribution License (CC BY). The use, distribution or reproduction in other forums is permitted, provided the original author(s) and the copyright owner(s) are credited and that the original publication in this journal is cited, in accordance with accepted academic practice. No use, distribution or reproduction is permitted which does not comply with these terms.
*Correspondence: Shiping Mao, MTkwNTk2MDA2QHFxLmNvbQ==; Jingdong Li, bGlqaW5nZG9uZ0BpZ3NucnIuYWMuY24=
†These authors have contributed equally to this work