- 1The International Center for Tropical Agriculture (CIAT), Cali, Colombia
- 2University of Vermont (UVM), Burlington, VT, United States
- 3The Gund Institute for Environment, University of Vermont, Burlington, VT, United States
- 4California Polytechnic State University—San Luis Obispo, San Luis Obispo, CA, United States
Tracking agriculture and land-use greenhouse gas (GHG) emissions is necessary to inform global climate policy, yet UNFCCC country-reported data and three independent global databases show inconsistent estimates of countries’ emissions. Data for developing countries are particularly inconsistent, yet also collectively the largest source of emissions. Here, we provide transparency about available country-level emissions data for agriculture and related land use and characterize their data quality and consistency to enable better understanding of available data and tracking of climate change mitigation. We call for increased consistency in official national agricultural GHG inventory data and transparency about the differences among scientific data sources to enable decision makers to track progress, set priorities and manage emissions.
1. Introduction
Reducing greenhouse gas (GHG) emissions from agriculture and the land sector are essential to meeting climate policy targets (IPCC, 2019; Lynch et al., 2021), yet tracking these emissions to inform global decisions remains challenging. While the United Nations Framework Convention on Climate Change (UNFCCC) provides a framework and guidance for countries to report emissions (e.g., IPCC, 1996a,b, 2006), it is well-known that official country reporting is often not up to date or of high quality, especially for low-and-middle-income countries (LMICs) where capacity and resources are limited (Rosenstock and Wilkes, 2021). UNFCCC Annex I countries (high-income) currently report emissions annually following the same IPCC guidelines (IPCC, 2006) and a Common Reporting Format (CRF). In contrast, non-Annex I parties (mostly LMICs) are not required to provide reports in as much detail or as regularly as Annex I countries and use different IPCC guidelines (e.g., Revised IPCC 1996 Guidelines for National Greenhouse Gas Inventories, IPCC 2006 Guidelines for National Greenhouse Gas Inventories, or a combination of the two) in National Communications (NCs) or Biennial Update Reports (BURs) submitted to the UNFCCC. Hence, differences are to be expected in the methods and quality of national GHG inventories, henceforth referred to as inventories, being reported to the UNFCCC (Perugini et al., 2021; see Supplementary material).
Meanwhile, scientific emissions data sources are available from several sources, including the United Nations Food and Agriculture Organization (FAO) (FAOSTAT, 2021), World Resources Institute Climate Analysis Indicators Tool (WRI-CAIT) (CAIT Climate Data Explorer, 2017) and the Emissions Database for Global Atmospheric Research (EDGAR) (Crippa et al., 2020 2021). However, the data are not consistent with each other or with the UNFCCC data despite IPCC guidelines and encouragement of countries to use independently compiled inventory databases, such as EDGAR, to verify emissions and removals (Deng et al., 2022). Decision-makers concerned with global comparisons are therefore faced with competing information sources to formulate mitigation strategies, manage emissions and identify global hotspots. Above all, inaccurate country-reported inventories paired with inconsistencies among alternative data sources impedes effective decision making for investment in and management of the agricultural sector.
Here, our goal is to provide transparency about available country-level emissions data for agriculture and related land use and characterize their data quality and consistency to enable better use of these data and tracking of climate change mitigation. To this end we evaluated the official UNFCCC country-reported GHG data through 2019 via NCs or BURs for non-Annex I countries and CRF tables for Annex I countries as well as the inventory methodology used. We then compared UNFCCC data with FAOSTAT, WRI-CAIT, and EDGAR data. Our results highlight a gap in LMIC reporting in the UNFCCC and a need for more uniform reporting methodologies, especially to manage food security in conjunction with climate change adaptation and mitigation. The analysis provides an in-depth catalog of national reporting issues that affect GHG inventories depending on the methodology used and provides recommendations on how inventories can be enhanced to achieve climate mitigation goals based on a review of each country’s GHG inventory submitted to the UNFCCC. It also raises the question of whether alternative, non-UNFCCC emissions data resources should be the go-to for emissions-related decisions. We conclude with how decision makers can make informed choices about national emissions data and call for support of better reporting of official UNFCCC data and more transparency in the differences among current data sources.
2. Methods
2.1. Inventory compilation and comparison
Using UNFCCC data, we compiled national carbon dioxide (CO2), methane (CH4), and nitrous oxide (N2O) emissions for (i) emissions and removals across all sectors, (ii) agricultural non-CO2 emissions, (iii) agriculture, forestry and other land use (AFOLU) or land use, land-use change, and forestry (LULUCF) emissions and removals, and (iv) cropland and grassland emissions and removals from NCs or BURs for non-Annex I countries and CRF tables for Annex I countries through 2019. The compiled agricultural GHG inventory was compared to similar GHG inventories from FAOSTAT (2021), WRI-CAIT (CAIT Climate Data Explorer, 2017) and EDGAR (Crippa et al., 2021) by evaluating the absolute difference in country-level agricultural non-CO2 emissions by inventory source. Data from all GHG inventories were accessed via their respective websites as of December 2019. The absolute difference in inventory GHGs were calculated as:
where V1 is agricultural non-CO2 emissions for a countries’ emissions reported to the UNFCCC and V2 is agricultural non-CO2 emissions for a countries’ reported emissions by FAOSTAT, WRI-CAIT or EDGAR. The absolute difference between UNFCCC reported data and independent inventories, shown in Figure 1, was generated using the ggplot2 package (Wickham, 2016) in R Studio (R Foundation for Statistical Computing, Vienna, Austria; RStudio Team, 2015).
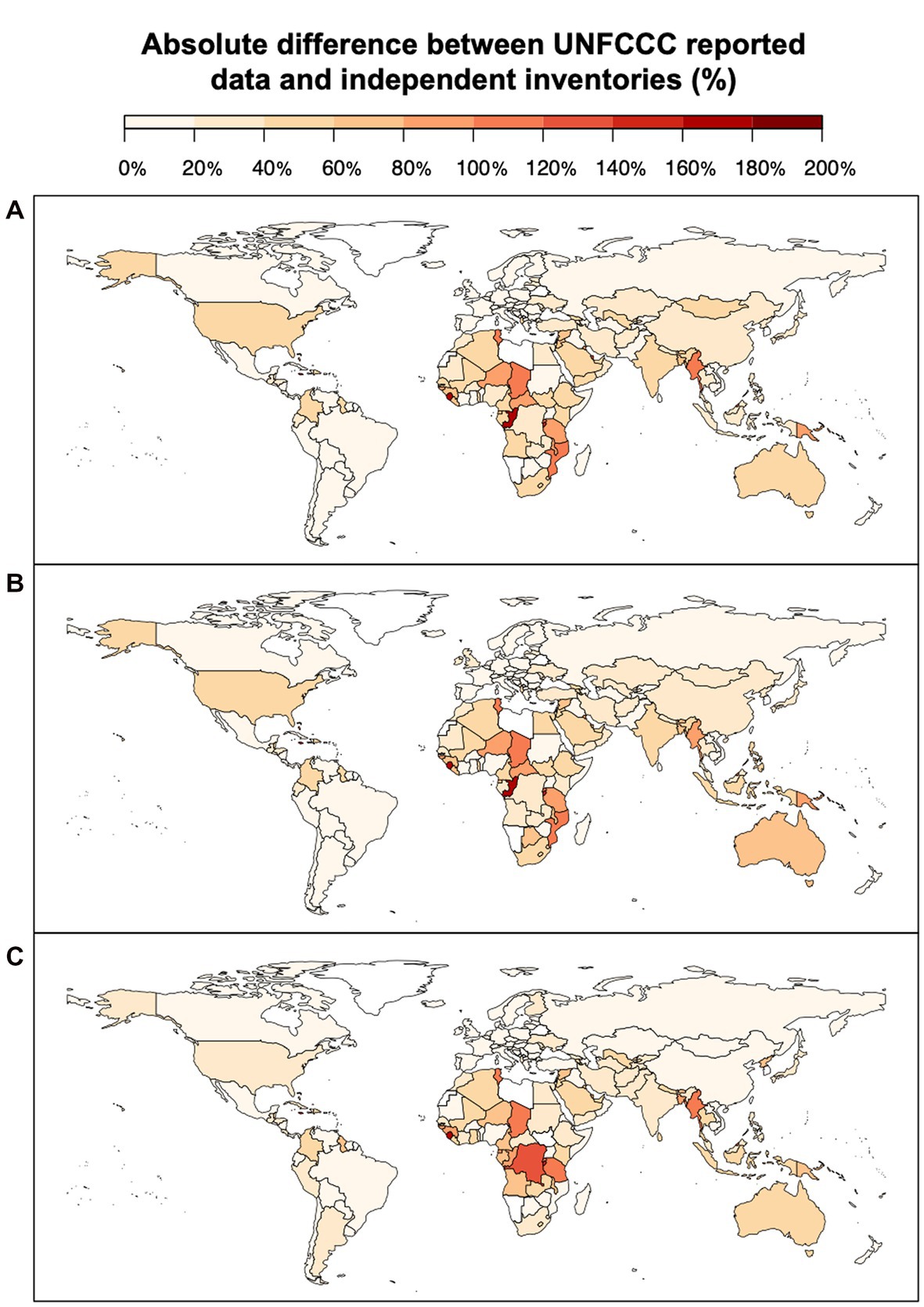
Figure 1. Absolute difference, represented as a percentage, between UNFCCC inventories and (A) FAOSTAT (B) WRI-CAIT and (C) EDGAR inventories for agricultural emissions. Absolute differences of 200% occur when one of the inventories reported zero emissions. White countries = no NC/BUR or data.
2.2. UNFCCC inventory analysis
Differences in global warming potential (GWP) standards and reporting formats and quality were also noted for the UNFCCC data. Emissions and removals were adjusted to be comparable using GWPs from the IPCC Fifth Assessment Report (IPCC, 2013) (CH4 = 28, N2O = 265). For countries that reported agricultural gases individually, we calculated CO2 equivalent (CO2e) using the AR5 GWPs. For countries that only reported emissions in CO2e without identifying the GWP used or did not disaggregate GHGs, we used the reported CO2e value.
We compared agricultural emissions and removals and emissions per capita using AR5, IPCC Second Assessment Report (AR2) and IPCC Fourth Assessment Report (AR4) GWPs with t-tests (R Foundation for Statistical Computing, Vienna, Austria; RStudio Team, 2015) to determine if emissions significantly differed across countries based on GWP selection. Each country’s agricultural emissions (CH4 and N2O only) were calculated as (i) national total, (ii) percentage of total emissions, and as (iii) emissions per capita (see Dittmer et al., 2021 for the dataset). Country population data for 2018 was acquired from FAOSTAT (2021).
3. Overview of GHG inventories
The four inventories examined here differ in terms of their developer and designated audience, which have shaped the nature of their databases. The UNFCCC inventory was designed to enable countries to report their emissions to the UNFCCC (country reporting). EDGAR’s data were compiled primarily for a scientific audience (scientific audience). FAOSTAT’s database was designed to provide a readily available public source of data based on a consistent methodology and using intergovernmental data (public intergovernmental). WRI-CAIT was also created to provide a public source of data in user-friendly and interactive format, drawing on existing data [public-research nongovernmental organization (NGO)]. As the country reporting is decentralized, UNFCCC is most variable. The other three inventories are managed centrally and therefore more internally consistent.
In terms of country coverage, for the UNFCCC data, 194 of 197 countries, plus the European Union (analyzed here as individual countries), reported GHG emissions in their NC, BUR, or CRF table. The FAOSTAT, WRI-CAIT, and EDGAR databases reported GHG emissions for 211, 195, and 218 countries or territories, respectively.
In terms of timing of posting and coverage of years, FAOSTAT posts countries’ inventory data, making them readily comparable to FAOSTAT’s emissions estimates. More than 75% of the NCs through 2019 were submitted in 2006 or after and 36% submitted as of 2016. All Annex I countries updated their inventory through 2019. In contrast, non-Annex I inventories date as far back as 1994, with the mean inventory year being 2010 (Supplementary Figure S1), thus making comparisons among countries difficult. We analyzed comparable data from EDGAR and WRI-CAIT inventories for 2018 and FAOSTAT for 2019.
Despite their centralized management, the independent inventories from FAOSTAT, WRI-CAIT, and EDGAR are challenging to compare as they differ in terms of the number of countries/territories reported, GWPs, data sources, emission factor tier level and usability (see Supplementary information). Interdependencies also exist among the databases, making it difficult to use the different sources for triangulation. For example, FAOSTAT computed national emissions using activity data (Tier 1) mainly reported to FAO by its member countries party to the UNFCCC when available (Tubiello et al., 2021) with GWPs from AR5 (IPCC, 2013). WRI-CAIT used FAOSTAT emissions data, but used the GWPs from AR4 (IPCC, 2007). EDGAR posted raw data, i.e., GWPs were not used to report CH4 and N2O emissions in CO2e, and their metadata did not specify the tier level nor the agricultural data sources used for calculating emissions. As national governments are responsible for compiling GHG inventories to the UNFCCC, nations used a mixed tier approach depending on the national circumstances.
Reporting of CO2 from cropland and grassland, which includes carbon sequestration in the soil and biomass, was uneven. Only 58 of 154 non-Annex I countries reported CO2 data, whereas all Annex I countries except one did. Our results are consistent with previous observations that non-Annex I countries often lack the expertise or resources to estimate emissions and removals, including on a regular basis, potentially leading to large data gaps and significant data quality issues [Food and Agriculture Organization of the United Nations (FAO) and Global Research Alliance on Agricultural Greenhouse Gases (GRA), 2020]. While all independent inventories focused on non-CO2 emissions for the agricultural sector, only the most recent FAOSTAT emissions database (Food and Agriculture Organization of the United Nations (FAO), 2021) considered CO2 emissions and removals from cropland and grassland, though only did so for peatland drainage.
We found FAOSTAT and WRI-CAIT to be the most user-friendly and interactive databases, whereas EDGAR has the option to export the database as an Excel document to view agricultural emissions by country. FAOSTAT is the only database that allows users to visualize national agricultural emissions, making global emissions comparisons easily feasible. These data sources are widely known by the scientific community but not necessarily by decision makers.
Below we provide detail for the UNFCCC inventory, where the highest inconsistencies were found.
4. UNFCCC inventory quality and methodologies
While other analysis of inventories have indicated a general lack of quality in UNFCCC reporting (Rosenstock and Wilkes, 2021), we identified specific areas for improved consistency. Lack of reporting of numbers, inconsistent GWPs, mistakes and legibility all affected the quality of countries’ reports. Some countries provided agricultural emissions only in graphs, making it difficult to identify precise numbers. Aggregated non-CO2 emissions using AR2 and AR4 GWPs could not be converted to current AR5 GWPs without making assumptions about emission sources of individual GHGs. Mistakes were not uncommon, such as tables labeled with 1 year but reporting data from another, reporting of inconsistent values for the sector in separate sections of the report. Some reports, although disseminated through the UNFCCC website, were illegible.
While more than half of all countries used the most recent IPCC (2006) guidelines (Table 1) (72 non-Annex I countries, 41 Annex I countries), non-Annex I countries still relied on the older revised 1996 IPCC guidelines (55 countries) or combined this with the 2006 guidance (22 countries). Three non-Annex I and two Annex I countries did not specify the guidelines used.
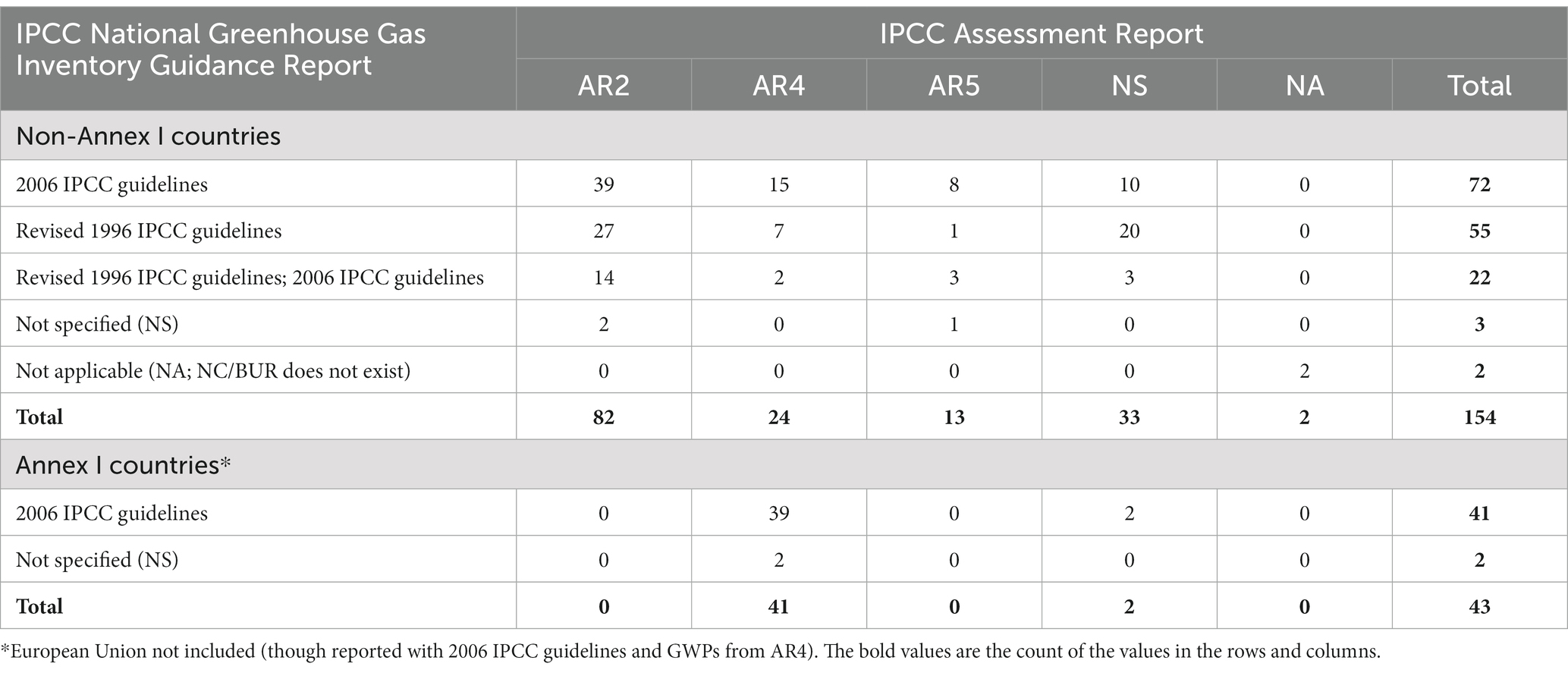
Table 1. Count of non-Annex I and Annex I countries’ use of IPCC National GHG Guidance Report and Assessment Reports used to compile their respective GHG inventories.
Most non-Annex I countries used the GWP recommended in AR2 (82 of 154). All Annex I countries used AR4 GWPs, though two countries did not specify the GWPs used. Thirty-three non-Annex I countries did not state their GWPs (Table 1). Global efforts to reduce GHG emissions may be undermined if countries continue to report aggregated non-CO2 emissions using mixed GWPs. To achieve the required emission reductions set forth by the Paris Agreement, each ton of any GHG needs to be accounted for in the same way so that actions related to the equitable and fair share of emissions and emission reductions are accounted for at the national level (Rogelj and Schleussner, 2019).
With respect to land-use GHG reporting, 57 non-Annex I countries reported emissions using AFOLU formatting and 115 countries separated Agriculture and LULUCF. Although 2006 IPCC guidelines suggest the use of AFOLU reporting, all Annex I countries reported using LULUCF, consistent with Decision 24/CP.19. Reporting using LULUCF format tends to be more complicated as emissions, removals and carbon storage are possible in LULUCF, whereas only emissions are reported in agriculture for AFOLU.
Inconsistency in reporting makes compiling of the UNFCCC data and its comparison problematic. The selection of IPCC guidance, format of emission data, use of LULUCF v. AFOLU reporting, detail on agricultural GHGs and carbon sequestration and GWP values varied among countries. Inventories that did not include summary tables (IPCC, 2006) with agricultural emissions broken down by source (e.g., enteric fermentation, paddy rice, etc.), or where inventories were only reported within figures or text as aggregated CO2e were the most problematic for extracting data as GWPs had to be assumed and numbers estimated. Approximately 21 non-Annex I countries reported data of this nature, which we flagged as poor quality for the purpose of compilation and comparison. This was only an issue for non-Annex I countries as all Annex I countries reported emissions in CRF tables, which have been built on the basis of the reporting table provided in the 2006 IPCC guidelines (IPCC, 2006). Indeed, the establishment of rigorous guidelines through the UNFCCC has led to the improvement of inventories for Annex I countries, however the low quality of national inventories for non-Annex I countries reflects the lack of technical, financial and institutional capacity.
5. Comparison of inventories: agricultural emissions and agricultural emissions per capita results
China, U.S.A. and Brazil reported the greatest total agricultural non-CO2 emissions among all countries, respectively, accounting for 33% of total global agricultural emissions. The top ten countries accounted for 53% of global emissions (Supplementary Table S1; Supplementary Figure S2A). Of the top ten agricultural emitters, two were Annex I countries (U.S.A., Russia) and the remaining eight were non-Annex I countries. Seven countries, six of which were non-Annex I, did not report any agricultural emissions.
On average, agriculture contributed 19% of countries’ gross national GHG emissions. Agriculture’s contribution among countries ranged from 0 to 87% of each country’s gross GHGs (Supplementary Table S1). Non-Annex I countries had a greater fraction of gross national GHG emissions from agriculture (mean 21%), compared to Annex I countries (mean 12%). Seventeen countries, all non-Annex I, reported more than 50% of their national emissions from agriculture.
The top 10 countries for agricultural emissions per capita consisted of three Annex I countries (New Zealand, Ireland, and Australia) using GWPs from AR5 (Supplementary Table S1) and AR4. Two Annex I countries were in the top 10 agricultural emissions per capita using AR2 GWPs. The choice of GWP mattered for national emissions of countries with CH4 as the primary agricultural GHG and the ranking of countries’ emissions per capita, although was not statistically significant otherwise (p = 0.39 for AR5 v. AR2 GWPs; p = 0.85 for AR5 v. AR4 GWPs). The GWP value used influenced national emissions per capita by as much as 33% (AR2) and 15% (AR5) relative to AR5. These results demonstrate that decisions as simple as GWP selection may have large impacts on how inventories are interpreted.
Comparing the UNFCCC compiled data to FAOSTAT, WRI-CAIT and EDGAR inventories after conversion to CO2e with GWPs from AR5 (CH4 = 28; N2O = 265), FAOSTAT and WRI-CAIT were most consistent with UNFCCC, which differed globally by 2.3 and 2.6%, respectively. The EDGAR inventory differed from the UNFCCC inventory by 5.7% globally. China, USA, and India consistently had the greatest absolute difference for all three independent inventories relative to the UNFCCC inventory. This is likely a result of (i) the magnitude of each of these countries’ GHG contributions (Supplementary Figure S2), (ii) inconsistent reporting of territories for these countries among inventories, (iii) the discrepancies in the latest inventory reporting years, (iv) Tier 1 estimates, which do not yet produce sufficiently accurate emissions estimates from agriculture (National Research Council, 2010), and (v) national reporting issues for UNFCCC data, predominately from LMICs.
However, countries in sub-Saharan Africa and other least developed nations, or countries with low shares of agricultural emissions, generally show the largest difference between independent inventory sources (Figure 1). Data gaps for robust activity data and emission factors are notoriously scarce in these regions, leading to high levels of uncertainty for national emissions reporting. For example, there were fewer than 100 site-years of agricultural emission data for sub-Saharan Africa in 2016, with activity data often estimated based on expert opinion, outdated surveys or extracted from international compendiums with unknown uncertainties (Rosenstock and Wilkes, 2021). Increasing the quantity and quality of data is a necessity for improving national statistical reporting systems for accurate GHG accounting in these regions. This is particularly important when considering that populations in non-Annex I countries, which already struggle with food security, are rapidly increasing and intensifying agriculture, leading to higher emissions over time (Metz et al., 2007; Smith et al., 2007) yet emissions may not be accurately quantified.
6. Discussion
High quality data are paramount to tracking countries’ Paris Agreement commitments. Our work emphasizes that inventories currently have limitations for accurate and transparent emissions reporting. These include poor reporting by LMICs, uncertainties in reported data (predominately CH4 and N2O as well as CO2 in land use), and lack of robust activity data and country/region specific emission factors to calculate emissions and removals. For perspective, only 4 of 46 sub-Saharan Africa countries quantified the uncertainty for livestock enteric methane, one of the largest agricultural GHG sources in the region, in inventories submitted to UNFCCC as of 2016 (Wilkes et al., 2017). Our work further demonstrates that using independent inventories to compensate or compliment UNFCCC data may create further problems based on the interdependencies that exist among inventories yet differences in the applied methodologies used to estimate emissions. Although absolute differences in independent inventories and UNFCCC data only differ by 2.3–5.7% globally, individual countries differed by as much as 180% (Figure 1). As the greatest differences generally occurred in non-Annex I countries and countries with small agricultural emissions, it is clear that data availability and accessibility remain a constraint in LMICs but also highlights that the level of granularity needed for small agricultural emitters may not be enough. Thus, these nations are hindered in their ability to make accurate and meaningful emission estimates and subsequent reductions.
As most non-Annex I countries rely on Tier 1 approaches and lack good quality activity data (Rosenstock and Wilkes, 2021), making use of multiple sources of emissions estimates may help fill information gaps. Use of remote sensing, modeling, and machine learning with data products such as NASA satellite imagery and Global Environmental Outlook datasets can help address these gaps (Yona et al., 2020), as can lower cost for use of expert judgment [Food and Agriculture Organization of the United Nations (FAO) and Global Research Alliance on Agricultural Greenhouse Gases (GRA), 2020]. Better use of technological and scientific advances along with big data could aid the adoption of a systematic process that combines knowledge and GHG emissions and sinks with land cover data to improve inventories.
Second, inventory guidelines should require a short summary table, developed by the IPCC, to better meet UNFCCC principles of transparency, accuracy, completeness, comparability and consistency. Furthermore, countries only reporting in CO2e units should state the GWP used for conversion for each non-CO2 gas. An interactive platform for submitting data would aid formatting for consistency and legibility for non-Annex I countries. The UNFCCC GHG inventory software, for example, facilitates the compilation and reporting of GHG emissions in a stepwise approach which helps to prevent possible errors and increases the transparency of the GHG inventory process. Though the IPCC’s guidelines for inventories are paramount to shaping climate policy, improving the transparency and accuracy, as well as reliability of inventories still requires urgent attention. Facilitating engagement among stakeholders, including inventory compilers and users, will be important to clarify feasible inventory compilation methods and plans for improvement over time.
More importantly, decision makers must have access to reliable data, which can be challenging to interpret differences with multiple data sources. UNFCCC reported data should be the most official, though it is often the most incomplete. Despite this, it is apparent that independent inventory databases are evolving to become more transparent: FAOSTAT’s metadata stands as an example as to how transparency in inventory compilation can be achieved (e.g., explicit mention of tier calculation, GWPs, IPCC guidelines, inventory scope, main sources of error); WRI-CAIT moved their database to Climate Watch (Climate Watch Historical GHG Emissions, 2021), which allows for the visualization of national and sectoral emissions. Until UNFCCC data is satisfactory, for example by implementing and supporting a more rigorous common review system or interactive platform to compile inventories for non-Annex I countries, decision-makers should use at least one of these independent sources for triangulation.
Fortunately, the 2019 Refinement to the 2006 IPCC guidelines for National GHG Inventories (IPCC, 2019) provides supplementary methodologies and improved emission factors for GHG sources and sinks where there were previously gaps or where new technologies and production processes have emerged, potentially providing a more robust opportunity to track and report GHG emissions and removals to achieve climate mitigation goals. Still, the capacity of developing countries to update inventories on a regular and transparent basis remains inadequate. This raises the question as to whether the principle of common but differentiated responsibilities, where developed countries are to provide financial resources to assist developing countries in implementing the objectives of the UNFCCC, has created a tradeoff between policy in reporting which gives greater flexibility to LMICs yet leads to failure in consistent and accurate reporting of inventories. Thus, as GHG inventories are the foundation for accounting and tracking progress towards mitigation goals, primary focus must be on developed nations intensifying sustained support and finance to develop and maintain institutional capacity and tools and training for preparing inventories in LMICs. Developed nations cannot continue to fall short on their climate-finance pledges (Timperley, 2021).
Data availability statement
Publicly available datasets were analyzed in this study. This data can be found at: UNFCCC Agricultural Greenhouse Gas Database 2021: https://hdl.handle.net/10568/116279. All independent inventory data are available on the respective organizational website: FAOSTAT Emissions database: http://www.fao.org/faostat/en/#data/GT. WRI-CAIT: https://www.climatewatchdata.org/data-explorer/historical-emissions?historicalemissions-data-sources=cait&historical-emissions-gases=all-ghg&historical-emissionsregions=&historical-emissions-ectors=agriculture&page=1. EDGARv6.0: https://edgar.jrc.ec.europa.eu/dataset_ghg60.
Author contributions
EW and KD conceived the study and drafted the manuscript. KD, MC, and CE developed and conducted the analytical methodology and data compilation. All authors reviewed and commented on the final version of the manuscript.
Funding
This work was funded by US Agency for International Development (USAID). This work was implemented as part of the CGIAR Research Program on Climate Change, Agriculture and Food Security (CCAFS), which is carried out with support from the CGIAR Trust Fund and through bilateral funding agreements. The CCAFS Program closed at the end of 2021. More information can be found on the CGIAR (https://www.cgiar.org). The views expressed in this document cannot be taken to reflect the official opinions of these organizations.
Acknowledgments
We would like to thank Francesco Tubiello and Ciniro Costa Junior for their thoughtful feedback on the analysis and initial manuscript.
Conflict of interest
The authors declare that the research was conducted in the absence of any commercial or financial relationships that could be construed as a potential conflict of interest.
Publisher’s note
All claims expressed in this article are solely those of the authors and do not necessarily represent those of their affiliated organizations, or those of the publisher, the editors and the reviewers. Any product that may be evaluated in this article, or claim that may be made by its manufacturer, is not guaranteed or endorsed by the publisher.
Author disclaimer
The views expressed in this document cannot be taken to reflect the official opinions of these organizations.
Supplementary material
The Supplementary material for this article can be found online at: https://www.frontiersin.org/articles/10.3389/fsufs.2023.1156822/full#supplementary-material
References
CAIT Climate Data Explorer. (2017). Washington, DC: World Resources Institute. Available at: http://cait.wri.org
Climate Watch Historical GHG Emissions. (2021). World Resources Institute, Washington, DC. Available online at: https://www.climatewatchdata.org/ghg-emissions
Crippa, M., Guizzardi, D., Muntean, M., Schaaf, E., Lo Vullo, E., Solazzo, E., et al. (2021). EDGAR v6.0 greenhouse gas emissions. European Commission, Joint Research Centre (JRC) [Dataset]. Available at: http://data.europa.eu/89h/97a67d67-c62e-4826-b873-9d972c4f670b
Crippa, M., Guizzardi, D., Muntean, M., Schaaf, E., Solazzo, E., Monforti-Ferrario, F., et al. (2020). Fossil CO2 and GHG emissions of all world countries: 2020 report. Publications Office of the European Union, Luxembourg.
Deng, Z., Ciais, P., Tzompa-Sosa, Z. A., Saunois, M., Qiu, C., Tan, C., et al. (2022). Comparing national greenhouse gas budgets reported in UNFCCC inventories against atmospheric inversions. Earth Syst. Sci. Data 14, 1639–1675. doi: 10.5194/essd-14-1639-2022
Dittmer, K. M., Wollenberg, E., Cohen, M., and Egler, C.. (2021). UNFCCC agricultural greenhouse gas database 2021. CCAFS database. Wageningen: CGIAR Research Program on Climate Change, Agriculture and Food Security (CCAFS). Available at: https://hdl.handle.net/10568/116279
Food and Agriculture Organization of the United Nations (FAO) (2020). FAOSTAT emissions database, agriculture, agriculture total. New Zealand. Available at: http://www.fao.org/faostat/en/#data/GT
Food and Agriculture Organization of the United Nations (FAO) (2021). FAOSTAT statistical database. Rome: FAO.
Food and Agriculture Organization of the United Nations (FAO) and Global Research Alliance on Agricultural Greenhouse Gases (GRA). (2020). Livestock activity data guidance (L-ADG): Methods and guidance on compilation of activity data for tier 2 livestock GHG inventories.
IPCC (1996a) in Climate change 1995: the science of climate change, contribution of working group I to the second assessment report of the intergovernmental panel on climate change. eds. J. T. Houghton, L. G. Meira Filho, B. A. Callander, N. Harris, A. Kattenberg, and K. Maskell (Cambridge: Cambridge University Press)
IPCC (1996b) in Revised 1996 IPCC guidelines for National Greenhouse gas Inventories. eds. J. T. Houghton, L. G. M. Filho, B. Lim, K. Treanton, I. Mamaty, and Y. Bonduki, et al. (Bracknell: Intergovernmental Panel on Climate Change, Meteorological Office)
IPCC (2006) in 2006 IPCC guidelines for national greenhouse gas inventories, prepared by the National Greenhouse Gas Inventories Programme. eds. H. S. Eggleston, L. Buendia, K. Miwa, T. Ngara, and K. Tanabe (Rome: IGES)
IPCC (2007) in Climate change 2007: The physical science basis. Contribution of working group I to the fourth assessment report of the intergovernmental panel on climate change. eds. S. Solomon, D. Qin, M. Manning, Z. Chen, M. Marquis, and K. B. Averyt, et al. (Cambridge: Cambridge University Press)
IPCC (2013) in Climate change 2013: The physical science basis. Contribution of working group I to the fifth assessment report of the intergovernmental panel on climate change. eds. T. F. Stocker, D. Qin, G.-K. Plattner, M. Tignor, S. K. Allen, and J. Boschung, et al. (Cambridge, United Kingdom and New York: Cambridge University Press) 1535
IPCC (2019) in 2019 refinement to the 2006 IPCC guidelines for national greenhouse gas inventories. eds. E. Calvo Buendia, K. Tanabe, A. Kranjc, J. Baasansuren, M. Fukuda, and S. Ngarize, et al. (Rome: IPCC)
Lynch, J., Cain, M., Frame, D., and Pierrehumbert, R. (2021). Agriculture’s contribution to climate change and role in mitigation is distinct from predominantly fossil CO2-emitting sectors. Front. Sustain. Food Syst. 4:518039. doi: 10.3389/fsufs.2020.518039
Metz, B., Davidson, O., Bosch, P., Dave, R., and Meyer, L.. (2007). Climate change 2007: Mitigation, contribution of working group III to the fourth assessment report of the intergovernmental panel on climate change. Cambridge: Intergovernmental Panel on Climate Change.
National Research Council (Ed.). (2010). Verifying greenhouse gas emissions: methods to support international climate agreements. National Academies Press, Washington, DC.
Perugini, L., Pellis, G., Grassi, G., Ciais, P., Dolman, H., House, J. I., et al. (2021). Emerging reporting and verification needs under the Paris Agreement: How can the research community effectively contribute? Environ. Sci. Policy. 122, 116–126. doi: 10.1016/j.envsci.2021.04.012
Rogelj, J., and Schleussner, C. F. (2019). Unintentional unfairness when applying new greenhouse gas emissions metrics at country level. Environ. Res. Lett. 14:114039. doi: 10.1088/1748-9326/ab4928
Rosenstock, T. S., and Wilkes, A. (2021). Reorienting emissions research to catalyse African agricultural development. Nat. Clim. Chang. 11, 463–465. doi: 10.1038/s41558-021-01055-0
RStudio Team (2015). RStudio: Integrated development for R. Retrieved from: http://www.rstudio.com/
Smith, P., Martino, D., Cai, Z., Gwary, D., Janzen, H., Kumar, P., et al. (2007). Policy and technological constraints to implementation of greenhouse gas mitigation options in agriculture. Agric. Ecosyst. Environ. 118, 6–28. doi: 10.1016/j.agee.2006.06.006
Timperley, J. (2021). The broken $100-billion promise of climate finance—and how to fix it. Nature 598, 400–402. doi: 10.1038/d41586-021-02846-3
Tubiello, F. N., Rosenzweig, C., Conchedda, G., Karl, K., Gütschow, J., Xueyao, P., et al. (2021). Greenhouse gas emissions from food systems: building the evidence base. Environ. Res. Lett. 16:065007. doi: 10.1088/1748-9326/ac018e
Wickham, H. (2016). ggplot2: elegant graphics for data analysis. Springer-Verlag New York, NY. Available at: https://ggplot2.tidyverse.org.
Wilkes, A., Reisinger, A., Wollenberg, E., and van Dijk, S.. (2017). Measurement, reporting and verification of livestock GHG emissions by developing countries in the UNFCCC: current practices and opportunities for improvement. CCAFS report no. 17. Wageningen: CGIAR Research Program on Climate Change, Agriculture and Food Security (CCAFS) and Global Research Alliance for Agricultural Greenhouse Gases (GRA)
Keywords: national greenhouse gas emission inventory, UNFCCC (United Nations Framework Convention on Climate Change), climate change, agricultural emissions, greenhouse gasses (GHG)
Citation: Dittmer KM, Wollenberg E, Cohen M and Egler C (2023) How good is the data for tracking countries’ agricultural greenhouse gas emissions? Making use of multiple national greenhouse gas inventories. Front. Sustain. Food Syst. 7:1156822. doi: 10.3389/fsufs.2023.1156822
Edited by:
Ngonidzashe Chirinda, Mohammed VI Polytechnic University, MoroccoReviewed by:
Elena Lioubimtseva, Grand Valley State University, United StatesCopyright © 2023 Dittmer, Wollenberg, Cohen and Egler. This is an open-access article distributed under the terms of the Creative Commons Attribution License (CC BY). The use, distribution or reproduction in other forums is permitted, provided the original author(s) and the copyright owner(s) are credited and that the original publication in this journal is cited, in accordance with accepted academic practice. No use, distribution or reproduction is permitted which does not comply with these terms.
*Correspondence: Kyle M. Dittmer, k.dittmer@cgiar.org