- 1International Institute of Tropical Agriculture (IITA), Lilongwe, Malawi
- 2International Institute of Tropical Agriculture (IITA), Nairobi, Kenya
- 3Tanzania Agricultural Research Institute (TARI), Dodoma, Tanzania
- 4International Institute of Tropical Agriculture (IITA), Ibadan, Nigeria
- 5International Institute of Tropical Agriculture (IITA), Kampala, Uganda
Soil and water conservation technologies are critical in reducing drought and soil erosion risks and increasing crop yields and incomes. Yet, there is limited empirical evidence on the extent and impacts of adopting soil and water conservation technologies in Tanzania. The study’s objective is to evaluate the adoption (as well as the duration of adoption) and the impacts of soil and water conservation technologies on income and food security in Tanzania. The study employs a control function approach and the instrumental variable quantile treatment effects model to survey data from 575 households to estimate the average and distributional impacts of adoption. The results show that the adoption and duration of adopting soil and water conservation technologies had significant and positive effects on the total value of crop production and household income. Moreover, we find that the adoption and its duration had a significant and positive impact on the food security indicator—household dietary diversity. The results from the instrumental variable quantile treatment effects model also show that the impacts of adopting soil and water conservation technologies on the outcome variables are positive and significant, although they vary significantly across the income and food security distributions. The results indicate that even though adoption benefits households in both the lower and upper quantiles of the income and food security distributions, the marginal impacts of adoption are generally more significant for the households in the upper quantiles. The paper concludes by discussing the policy options for increasing and sustaining the adoption and impacts of soil and water conservation technologies in Tanzania.
1. Introduction
Soil nutrient deficiency and moisture stress are major factors limiting crop productivity in many parts of sub Saharan Africa (SSA) (Mueller et al., 2012). These problems are often exacerbated when agricultural production predominantly depends on seasonal rainfall and is characterized by limited use of fertilizer and soil and water conservation technologies (SWCT) that would reduce soil fertility loss through erosion. The dependence on rain-fed agriculture exposes farmers to climatic risks such as droughts, which can dramatically reduce crop yields and livestock production (Schmidhuber and Tubiello, 2007), especially in semi-arid regions.
In the last four decades, Tanzania has experienced a series of severe droughts and floods, which have increased the uncertainty in seasonal rainfall prediction (FAO, 2014). This has negatively affected crop productivity, especially in the semi-arid region of central Tanzania. In addition, soil erosion resulting from inappropriate crop management practices, tillage, and livestock grazing systems have reduced crop productivity due to the loss of soil organic matter and nutrients. In response to these problems, research and development organizations have been testing and promoting sustainable intensification (SI) practices to increase crop productivity, incomes, and food security among smallholder farmers while mitigating the adverse effects on the environment. The adoption of SWCT offers a potential solution to arrest declining soil fertility. However, there is limited empirical evidence on the extent and impacts of adopting SWCT in Tanzania.
While there is a large body of literature on the productivity and farm income effects of the adoption of SWCT (e.g., Kassie et al., 2008; Di Falco et al., 2011; Kassie et al., 2011; Di Falco and Veronesi, 2013; Abdulai and Huffman, 2014), there are relatively few studies that have examined the relationship between SWCT and food/nutrition security (Habtemariam et al., 2019; Issahaku and Abdulai, 2019). Previous research has demonstrated a linkage between adopting improved crop varieties and dietary diversity/specific nutrient consumption (Smale et al., 2015; Mumin and Abdulai, 2021). However, empirical evidence on the impact of SWCT on food security outcomes among rural households is significantly lacking in the literature. Moreover, most of the previous studies have assumed that the effects of the adoption of SWCT are homogenous, ignoring the fact that the returns to the adoption of most agricultural innovations in sub-Saharan Africa are heterogeneous (e.g., Suri, 2011; Zeng et al., 2015; Wossen et al., 2018; Manda et al., 2019). This study aims to fill this gap in the literature by examining the average and distributional impacts of the adoption and duration of adoption of SWCT on the total value of production (TVP), household income, and the indicators of food security, i.e., household dietary diversity (HDD), and household food insecurity access scale (HFIAS).
We contribute to the growing literature on SWCT in the following ways. First, unlike previous studies (e.g., Kassie et al., 2008, 2011; Abdulai and Huffman, 2014), we estimate the impact of adoption on HDD and HFIAS. These indicators measure the quantity and quality of food access at the household level and have an element of household nutrition. For example, HDD is associated with diet quality since it helps ensure adequate intake of essential nutrients and promotes good health (Leroy et al., 2015). The HFIAS is based on subjective responses to questions that capture universal aspects of the experience of food insecurity, information on food shortage, food quantity, and quality of diet to determine the status of a given household’s access to food (Carletto et al., 2013). In estimating adoption impacts, we control for observed and unobserved characteristics that would otherwise result in biased estimates.
Furthermore, since the benefits of adopting ISFM technologies may take time to be realized (Kassie et al., 2013; Jayne et al., 2019), we also consider the impact of the duration of the adoption of SWCT on the outcomes mentioned above. To the best of our knowledge, the study by Maggio et al. (2021) is one of the few studies that have considered the impact of sustained adoption of ISFM practices on smallholder welfare outcomes. Second, we examine the distributional effects of the adoption of SWCT using the instrumental variable unconditional quantile treatment effects (IVQTEs) model. Most of the previous studies have either used conditional quantile treatment effects (QTEs) (e.g., Issahaku and Abdulai, 2019; Ogutu et al., 2019) or unconditional treatment effects without controlling for unobservable characteristics (e.g., Mishra et al., 2015; Ainembabazi et al., 2018; Khanal et al., 2018). According to Frölich and Melly (2010, 2013), unlike the conditional QTEs, which change with the set of conditioning covariates, the unconditional QTEs do not depend on other covariates to be consistently estimated. The second advantage of unconditional effects is that they can be estimated consistently without any parametric restrictions, which is impossible for conditional effects. Finally, to attach a causal interpretation to our QTEs, we use instrumental variable (IV) techniques to correct the adoption decision’s endogeneity. We also complement the IVQTEs results with the smoothing-differencing (SD) method of estimating the distributional effects of adoption to examine how treatment effects vary with the propensity to adopt SWCT.
The rest of the article is organized as follows: The next section describes the sampling strategy, data, conceptual, and empirical approaches. Section 3 presents the results and discussion, and the last section draws conclusions and policy recommendations.
2. Methods
2.1. Sampling strategy and data
The data used in this paper come from a survey of 575 sample households conducted between September and October 2020 in the semi-arid districts of Kiteto and Kongwa in central Tanzania. A survey questionnaire was prepared and designed in Surveybe, a computer-assisted personal interviewing (CAPI) software and administered by trained enumerators who collected data from households through personal interviews.
A multistage random sampling procedure was used to select sample households. In the first stage, five wards (Oluboloti, Njoro, Mlali, Nghumbi, and Sagara) were purposively selected from the two districts where there has been actively testing and promotion of SWCT. In the second stage, five villages from the five wards were selected using probability proportional to size sampling (PPS). With the help of the ward extension agents, and the Tanzania Agricultural Research Institute (TARI), a sampling frame was developed by listing all households (including adopters and non-adopters of SWCT). In the final stage, 120 households were randomly selected from each village resulting in 240 households from Kiteto district and 360 households from Kongwa district. However, 340 households were interviewed in Kongwa district because the remaining 25 could not be traced.
Detailed information was collected on demographic and socio-economic characteristics (e.g., household head’s age, sex, and education, livestock ownership, farm size, and crop production).
2.2. Conceptual and empirical frameworks
Droughts and floods affect crop production, lives, health, livelihoods, assets, and infrastructure, contributing to food insecurity and poverty among smallholder farmers in SSA (Shiferaw et al., 2014). The adoption of SWCT offers a potential solution to these problems by reducing water runoff and soil erosion and increasing soil fertility, thereby enhancing crop productivity and farm incomes of smallholder farmers. The productivity gains through the adoption of SWCT are expected to increase household income and ultimately result in increased expenditure on diverse, high-calorie, protein, and micronutrient-rich foods, finally improving household food security. As mentioned earlier, the impact of SWCT on crop productivity is well established in the literature, both from on-farm trials and plot-level surveys (e.g., Tsubo et al., 2005; Kassie et al., 2008; Kato et al., 2011). In this study, we envisage that the adoption and duration of SWCT will mainly increase TVP, household incomes, and food security through the crop productivity impact pathway.
Estimating the causal effect of the adoption (including its duration) of SWCT on income and food security is not trivial, especially with non-experimental data. This is so because farmers actively self-select into the adoption category based on their potential gains, which may lead to endogeneity problems (Alene and Manyong, 2007; Abadie and Cattaneo, 2018). This implies that regressing the adoption variables on the income and food security indicators with added control variables may lead to biased estimates. To properly account for self-selection and endogeneity, we use the control function (CF) approach and IV techniques (Terza et al., 2008; Navarro, 2010; Wooldridge, 2014; Wooldridge, 2015). In the CF approach, the adoption decision is modeled using a probit model, while two separate linear outcome equations for adopters and non-adopters are specified. In the duration of the adoption model, we specify a linear IV regression model estimated using the two-stage least squares (2SLS) method.
2.3. Control function approach and IV regression model
2.3.1. Control function approach
To set the stage for our estimation strategy, let D = 1 denote adopters and let D = 0 denote non-adopters of SWCT. Given whether farmers choose to adopt SWCT or not, we can only observe any of these two states at a time, such that the observed outcome (i.e., income and food security) for a given farmer is if they are adopters and by if non-adopters. That is, the observed outcome Y can be written as:
A farmer will adopt SWCT if the outcome from adoption is higher than the case of non-adoption. More formally this can be written as:
where 1[.] is an indicator function that takes the value of 1 if the statement inside the brackets is true and 0 if it is false. We can define appropriately as:
where is a set of household and farm characteristics determining adoption, and the error term . The potential outcome for adopters and non-adopters can also be defined as:
Where X are vectors of weakly exogenous covariates, while and are parameters to be estimated for the adopter and non-adopter regimes, respectively, and; ei is an unobserved random component. The unobserved components in the potential outcome equations should be independent of Vi such that the correlation between Di and the unobserved components ( and ei) is equal to zero. Endogeneity arises if the error term in the adoption equation (equation 3) contains the same random variables as those in the outcome equations (equations 4a, 4b), such that the correlation between the error terms in the adoption and outcome equations is not equal to zero.
Endogeneity arises due to selection bias, simultaneity between adoption and the outcome variables and omitted variable bias (Terza et al., 2008; Maggio et al., 2021). We use the CF with an IV to break the correlation between the potential endogenous adoption variable and unobservable factors affecting the outcome variables. To achieve this, the V variables in the adoption model need to contain an IV that significantly affects the adoption of SWCT conditional on covariates (relevance condition) and affects income and food security only through D, but not directly (exclusion restriction). We use group membership (Abdulai and Huffman, 2014) as an identifying instrument. We checked whether the IV was correlated with the adoption status (relevance condition), and the reported results in section 3.2 show that the IV is relevant. Several previous studies have used this instrument (e.g., Kabunga et al., 2012; Abdulai and Huffman, 2014). Nonetheless, one might argue that the exclusion restriction can be violated if farmers learn about income and food security-enhancing practices beyond SWCT, such as livestock assets and information regarding climate adaptation strategies. We have tried to control for several social-economic characteristics in our specification, including livestock ownership and information variables.
To estimate the impact of adopting SWCT on the outcome variables, i.e., the average treatment effect on the treated (ATT), we first estimate the adoption equation together with the instruments using a probit model and obtain generalized residuals . In the second stage, we estimate equations 4a, 4b with as an additional regressor. In this case, the generalized residuals save as a CF, and by adding appropriate CFs, the adoption variable becomes aptly exogenous in estimating the outcome equations (Wooldridge, 2015). The ATT can be defined simply as:
To recover the ATTs and to obtain correct standard errors, equation 5 is estimated using generalized methods of moments (GMM).
To estimate the impact of the duration of adoption on the outcome variables, we specify the following linear regression model:
where Yi, and X are defined as above; and is the error term. is the duration of the adoption of SWCT.
2.3.2. The instrumental variable unconditional quantile treatment effects model
The violin plots presented above suggest that the effects of adoption are likely to be conditional on adopters observed and unobserved characteristics. To estimate the distributional or heterogeneous effects of adopting SWCT on the income and food security indicators, we use the IVQTE following Frölich and Melly (2010, 2013). Estimating QTEs is essential to evaluate the effect of a variable on different points of the outcome distribution and therefore allows for the identification of effects even in situations where the mean of the outcome variable remains unchanged. Let Y1i (D = 1) and Y0i (D = 0) be the potential outcomes of household i (as defined above). Following Frölich and Melly (2010, 2013), the unconditional QTE (for quantile τ) can generally be given by:
where is the quantile for Y1i and is the th quantile of Y0i.
As mentioned above, the decision to adopt is endogenous; hence identification can only be achieved through an IV, Z. The treatment effects can be arbitrarily heterogeneous, such that the effects can only be identified for the population that responds to a change in the value of the instrument, i.e., compliers (Frölich and Melly, 2013). Therefore, we focus on the QTEs for the compliers given as:
The unconditional IVQTE for compliers proposed by Frölich and Melly (2013) can be defined as a bivariate quantile regression estimator with weights:
where denote the weights proposed by Frölich and Melly (2010, 2013). , where is the asymmetric absolute loss function or check function (Wooldridge, 2010). The weights are defined as:
where is a binary instrumental variable and are the propensity scores.
2.4. Smoothing-differencing heterogeneous treatment effects
As a key robustness check for the distributional impacts of adoption, we also estimate treatment heterogeneity by conditioning on a full set of covariates but without controlling for unobserved heterogeneity. Following Brand and Xie (2010) and Xie et al. (2012), we use the SD method to analyze how treatment effects vary with the propensity to adopt SWCT. The method follows three steps: First, we estimate equation 3 using a logit model to obtain the conditional probability of adopting SWCT (propensity score). Second, we fit separate, non-parametric regressions (local polynomial regression) of the income and food security variables on the propensity score for the adopters and non-adopters. Third, we estimate the difference in the non-parametric regression line between the adopters and non-adopters at different levels of the propensity score. This enables one to obtain a pattern of treatment effect heterogeneity as a function of the propensity score.
3. Results and discussion
3.1. Descriptive statistics
Table 1 shows the definition and summary statistics of the variables used in this study. The results show that, on average, 22% adopted SWCT in the 2019/2020 growing season. In this paper, we define SWCT as either tied ridging or fanya-juu terraces.2 Similarly, we define the duration of adoption as the average number of seasons (including the 2019/2020 season) that a farmer has used either tied ridging or fanya-juu terraces. Tied ridging is an in-situ rainwater harvesting technique that collects rainwater in the field to facilitate water infiltration, subsequently increasing crop productivity (Habtemariam et al., 2019). It involves blocking ridge furrows with earth ties spaced at a fixed distance apart to form a series of micro-catchment basins in the field (Wiyo et al., 2000). The use of fanya-juu terracing system also can reduce water runoff, soil erosion, and siltation of rivers, lakes, and dams and thus improve soil infiltration, fertility, and crop yields (Kassie et al., 2011; Saiz et al., 2016). Fanya-juu terracing system consists of constructing embankments along a slope by digging out trenches following contour lines and depositing the soil uphill of the trench to form a mound.
We use four outcome variables: TVP, household income (hereafter, income), HDD, and HFIAS (hereafter, food security). TVP is the total value of all the crops grown by the household and is a proxy for crop productivity and income. Household income is an indicator of household welfare among smallholder farmers. It includes all income from crops, livestock products, salaries, remittances, farm labor wage income, pension, and businesses.
The HDD is a proxy for the quantity and quality of food access at the household level (Leroy et al., 2015). Apart from being a measure of household access to various foods, it is also a proxy for diet quality. In this study, we used HDD scores (HDDS) as an indicator of HDD (Kennedy et al., 2010). During the survey, households were asked to mention the food items they consumed in the last 24 h. These included Cereals, white roots and tubers, vitamin A-rich vegetables and tubers, dark green rich vegetables, other vegetables, vitamin A-rich fruits, other fruits, organ meat, flesh meats, eggs, fish and seafood, legumes, nuts and seeds, milk and milk products, oil and fats, sweets, and spices. Following Kennedy et al. (2010), we combined the vegetables, meats, and fruits with 12 food groups, each with a score of 1 if they consumed a food item. The HDDS were then constructed by summing these food groups such that the scores ranged from 0 to 12. The HFIAS uses a set of questions that represents universal domains and subdomains of experiencing household food insecurity and lack of access to food (Leroy et al., 2015). The scale was developed through the Food and Nutrition Technical Assistance Project (FANTA), and details on how it is constructed are outlined in Coates et al. (2007). The HFIAS ranges from 0 to 27, so the higher the score, the more food insecurity the household experiences, and the lower the score, the less food insecurity a household experiences.
Table 1 also shows several demographic and socio-economic variables that are hypothesized to affect the adoption of SWCT. Household characteristics such as age, sex, and education of the household head; and socio-economic characteristics such as access to credit, livestock ownership, and access to off-farm employment are important determinants of agricultural innovations (Feder et al., 1985; Feder and Umali, 1993; Kassie et al., 2013). Farmers who have access to information related to adaptation to climate change are expected to adopt SWCT because these technologies are vital in addressing problems related to adverse climatic conditions such as droughts and floods. The number of years a household head has lived in the village, kinship, and membership in farmer organizations are meant to capture social capital and networking (Kassie et al., 2013; Abdulai and Huffman, 2014). The variables related to the distance to the district capital, extension agent’s office, and district market are proxies for transaction costs associated with the adoption of SWCT. On average, it takes a farmer only 25 min to walk to the extension agent’s office and over 4 h to the district market.
Figure 1 shows the distribution of the outcome variables by the adoption status. Violin plots combine the basic summary statistics of a box plot with the visual information provided by a local density estimator to reveal the distributional structure in a variable. Like a box plot, the violin plot displays the median as a short horizontal line with a dot, the interquartile range (first-to-third) as a narrow-shaded box, and the lower-to-upper adjacent value range as a vertical line.
Figure 1 shows significant heterogeneity with groups of households clustering in the upper and lower tails of the distributions, suggesting that the adoption of SWCT might unduly affect less productive and underprivileged households. This justifies the use of IVQTE to explore further the distributional impacts of the adoption of SWCT in section 4.
3.2. Determinants and impacts of the adoption of SWCT
The CF estimates of the determinants of the adoption of SWCT are shown in Table 2. These are the results emanating from estimating equation 3 using a probit model. The outcome equations (equations 4a, 4b) for all the outcome variables are presented in Appendix Table 1 for the adopter and non-adopter categories.3
The first stage results from the four equations are interpreted together because the coefficient estimates differ slightly.4 Consistent with Issahaku and Abdulai (2019), results show that female-headed households are more likely to adopt SWCT than male-headed households. The results also indicate that educated farmers are more likely to adopt SWCT as they might acquire new knowledge and process information more quickly (Adegbola and Gardebroek, 2007; Abdulai and Huffman, 2014). We find that household size increases the probability of the adoption of SWCT by smallholder farmers in Tanzania. The common finding in studies investigating the adoption of labor-intensive technologies is that household size is associated with an increase in the adoption rate of such technologies (Kassie et al., 2008; Di Falco and Veronesi, 2013; Ojo and Baiyegunhi, 2020). One explanation advanced for this finding is that household size is a proxy for household labor endowments, especially in developing countries.
Results further suggest that the adoption of SWCT increases with the number of traders trusted by the farmers. A recent study in Kenya by Mulwa et al. (2021) shows that large grain traders are essential in increasing the adoption of SI technologies due to their considerable financial and operational capacities, which enable them to provide reliable output markets, credit, inputs, and agricultural extension to smallholders farmers. The number of years the household has lived in the village capture the social capital and networks at the village level. Contrary to our a priori expectations, the probability of adoption of SWCT reduces with the number of years the household head has lived in the village. We also find that farm households with information on adaptation to climate change have a higher propensity to adopt SWCT, and this is partly due to the reasons explained above. Most SWCT activities are concentrated in Kongwa district, implying that farmers receive relatively more support services such as extension, group membership and other farmers who have adopted these technologies. The significant coefficient evidences this on the Kongwa dummy, showing that farmers in Kongwa are more likely to adopt SWCT than those in Kiteto district. Membership in a farmer’s organization increases the probability of adopting SWCT, and this also shows that it is a relevant instrument in identifying IV-based models specified above. Membership in farmer groups reflects the intensity of contact with other farmers (Adegbola and Gardebroek, 2007) and may also indicate exposure to information on the SWCT.
3.3. Impacts on household welfare
The estimates for the average treatment effects on the treated (ATT) from the CF model, which show the effects of SWCT adoption on income and food security, are presented in Table 3. The results show that adopters’ TVP and household income that can be attributed solely to the adoption of SWCT was 11% and 7% higher than non-adopters. The results also show that adopting SWCT significantly increased HDDS by 43%. The impact estimates are broadly consistent with studies on climate-smart agricultural practices and SWCT (Kassie et al., 2008; Di Falco et al., 2011; Abdulai and Huffman, 2014; Issahaku and Abdulai, 2019).
Table 4 shows the second stage 2SLS results from the estimation of equation 6, while the first stage results are presented in Appendix Table 2. The coefficient on the variable “duration of adoption” indicates the impact of the duration on the outcome variables, i.e., average treatment effect (ATE). The results show that an additional year or season of sustained adoption of SWCT leads to a 36% and a 54% increase in TVP and household income, respectively5. Similarly, holding other variables constant, HDDS, on average, increase by 82% for every additional year of adoption. The results in Table 4 point to the importance of considering both the adoption and duration when assessing the effect of improved agricultural technologies.
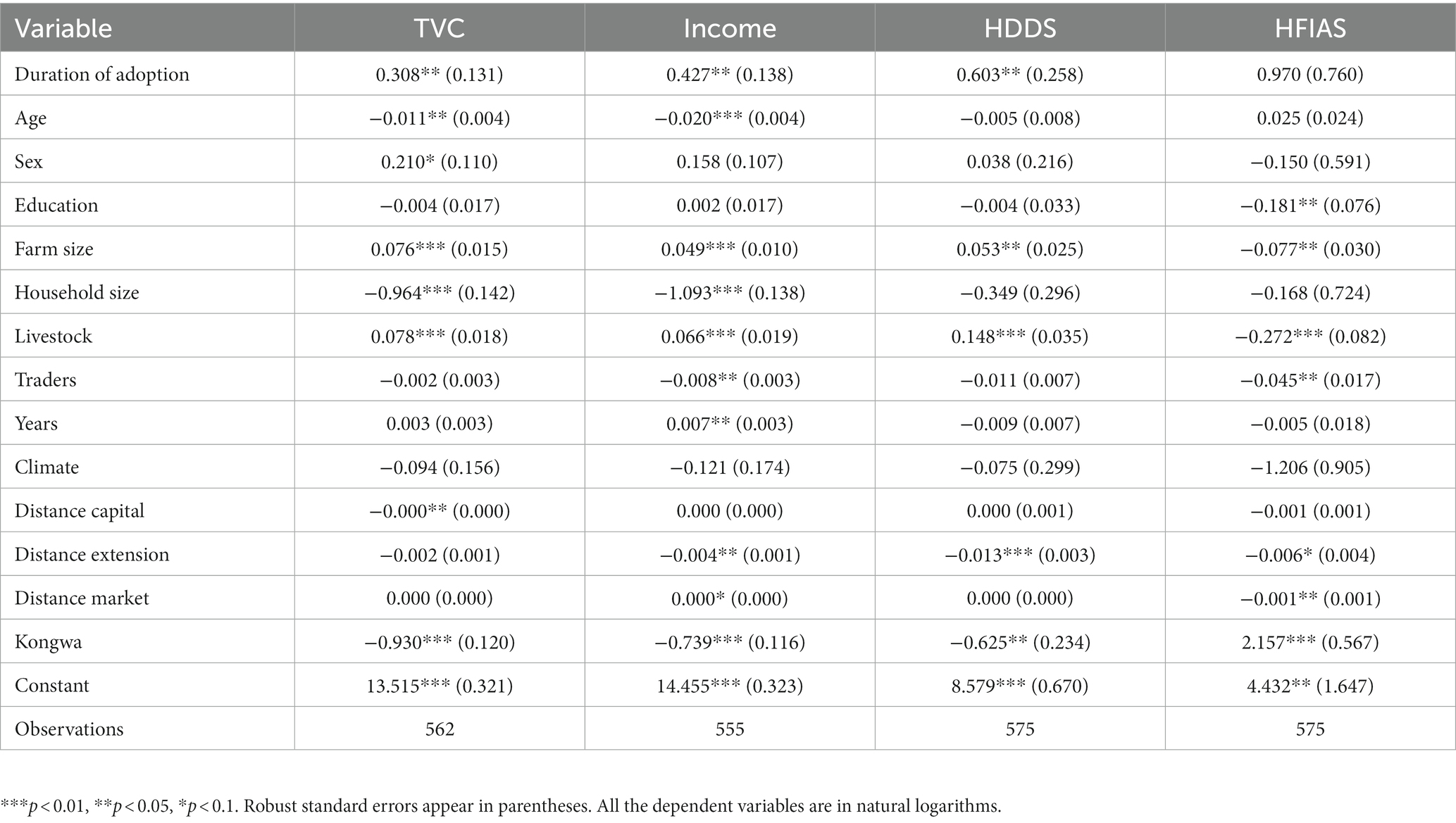
Table 4. Impact of the duration of adoption of SWCT on income and food security (IV regression estimates).
3.4. Distributional impacts of the adoption of SWCT
Table 5 reports the estimated IVQTEs of the adoption of SWCT on the outcome variables for the 0.1–0.9 quantiles. The 0.1 quantile includes the households with the lowest income and food security, while the 0.9 quantile includes the sample households with the highest income and food security. Unlike previous studies which report conditional or unconditional quantile treatment effects based on observed characteristics, results in Table 5 also account for unobserved characteristics to ascribe a causal interpretation to the results. The results show that the impacts of adopting SWCT on all the outcome variables are significant in some of the quantiles. However, they are not homogenous as they vary significantly across income and food security distributions. Contrary to the results found by Issahaku and Abdulai (2019), our results generally show that even though adoption benefits households in the lower and upper quantiles alike, the marginal impacts of adoption are more prominent for the households in the upper quantiles and smaller for those in the lower quantiles. However, the results agree with those of Manda et al. (2017) and Wossen et al. (2018) for improved crop varieties in Zambia and Nigeria.
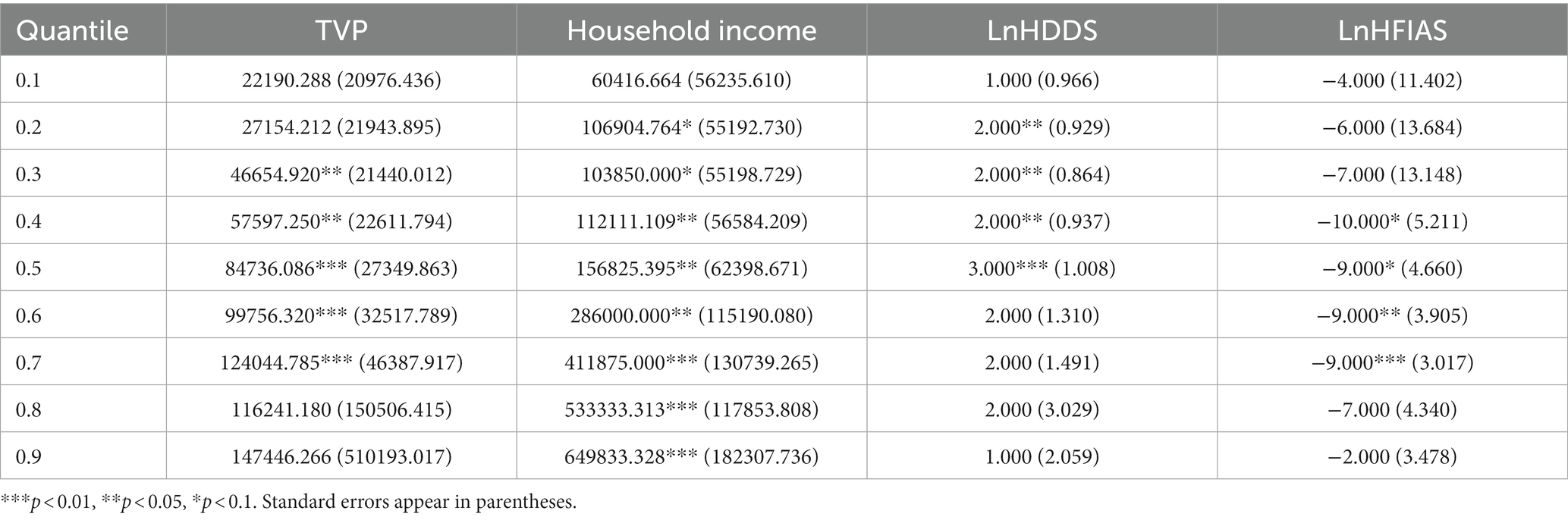
Table 5. Unconditional quantile treatment effects of the adoption of SWCT on income and food security.
Considering income (TVP and household income), the adoption effects are positive and significantly different from zero across most of the distribution. We can infer from these results that the increase in income associated with adopting SWCT tends to grow as income increases. The distributional impacts of SWCT on HDDS are slightly different as the effects are only significant in the lower half quantiles of the HDDS distribution, even though the impact also increases as we move from lower to upper quantiles. This attests that even households with comparatively lower HDDS can benefit from adopting SWCT. The results also reveal that the food insecurity-reducing effects of the adoption of SWCT are only significant in the upper half of the HFIAS distribution. Taken together, the results suggest that the adoption of SWCT mainly benefits the households in the upper quantiles of the income and food security distributions.
As mentioned earlier, we also estimated the distributional effects of SWCT using the SD method based on the household’s propensity to adopt the SWCT. This is important because IVQTEs results may be sensitive to the assumptions that come with the identification of the model. Following the approach described in section 2.4, the difference in the non-parametric regression line between the adopters and non-adopters at different levels of the propensity score is depicted in Figure 2. The x-axes indicate the estimated propensity for adopting SWCT, and the y-axes show the matched differences between adopters and non-adopters. The results generally show a steady and increasing TVP, household income, and HDDS response to different levels of the estimated propensity scores. This suggests that farmers with a higher propensity to adopt benefit the most from adopting SWCT. We observe a similar trend with the HFIAS curve, which show a negative slope, indicating a reduction in food insecurity as the propensity to adopt increases. Results in Figure 2 suggest that farmers self-select into adoption based on their comparative advantage (positive selection), consistent with Suri (2011). The smoothing-differencing results are consistent and lend credence to the IVQTEs results presented above.
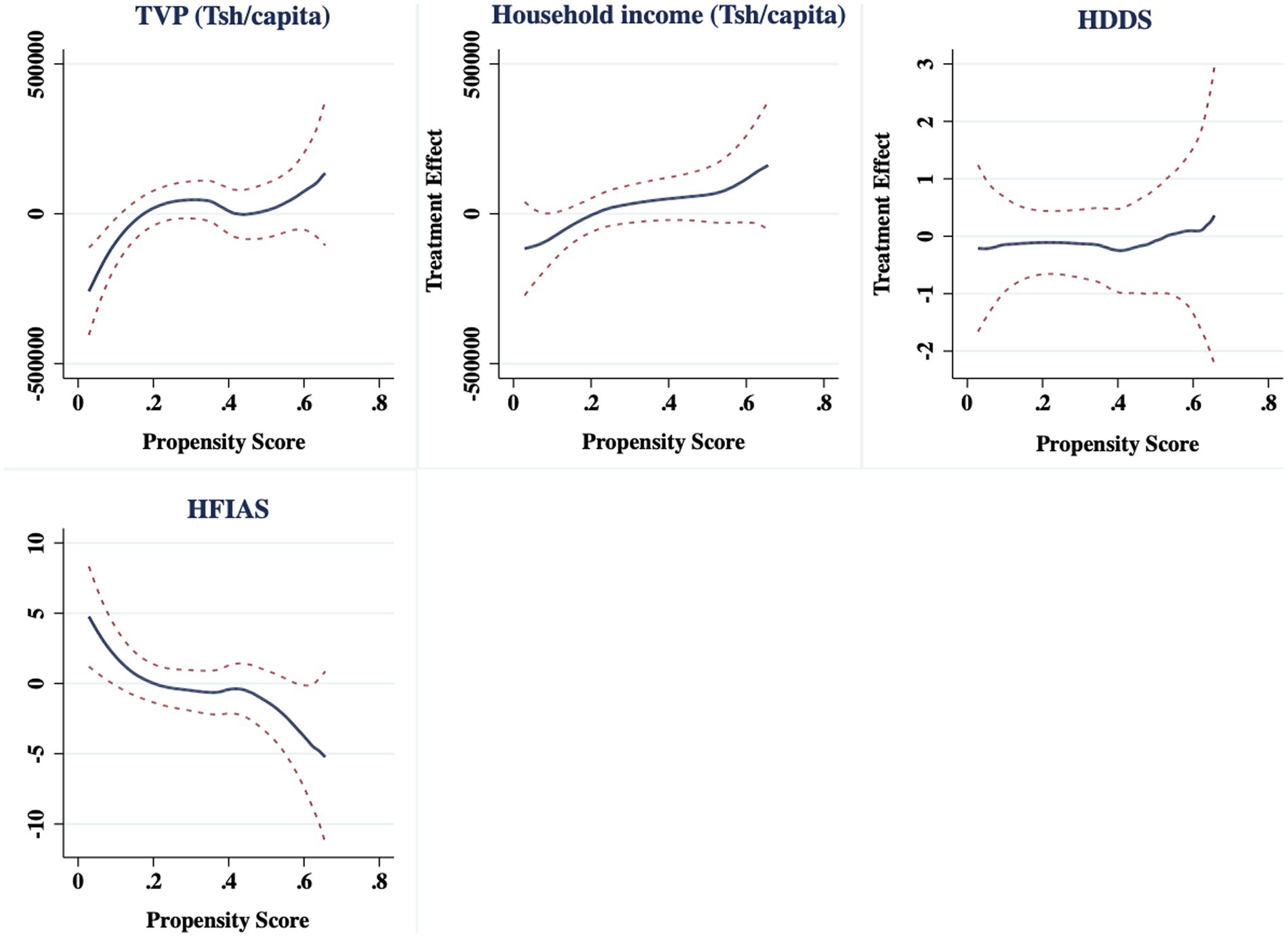
Figure 2. Smoothing-differencing heterogenous SWCT adoption effects on TVP, household income, HDDS, and HFIAS.
4. Summary and conclusions
The central region of Tanzania is predisposed to frequent droughts and significant topsoil erosion, which has negatively affected the productivity of many crops in the country. Previous studies show that adopting SWCT is a potential solution to some of these problems by reducing drought and soil erosion risks and increasing crop yields and incomes. Nevertheless, in most of these studies, much attention has been given to assessing the impact of the adoption of SWCT on crop yields and net farm returns, with a few analyzing the effect on household income and food security. Moreover, empirical evidence on the distributional impacts of adopting SWC technologies is still thin. This paper contributes to the empirical literature in this area by examining the average and distributional effects of the adoption and the duration of adoption of SWCT on incomes and food security in central Tanzania. We use several robust econometric approaches that account for observed and unobserved factors, coupled with recent household survey data from a sample of over 500 households, to achieve our objective.
Consistent with previous adoption studies, our results indicate that the main factors influencing the adoption of SWCT are the sex and education of the household head, household size, information on the adaptation strategies to climate change, and farmers’ group membership,. The results further show that the adoption and sustained adoption of SWCT significantly increases household income and food security. This underscores the importance of adopting SWCT in mitigating the adverse effects of climate change, such as frequent droughts and soil erosion, which are common in the semi-arid regions of central Tanzania.
The IVQTEs, complimented with the SD heterogeneous results, offer a more nuanced description of the relative effects of adopting SWCT over the entire income and food security distributions. Unlike the average treatment effects from the CF and 2SLS models, the quantile treatment effects mainly show that the marginal impacts of adoption are more significant for the households in the upper quantiles and smaller for the households in the lower quantiles of the income and food security distributions, indicating that the effects are not uniform but heterogeneous.
Overall, two main policy recommendations can be drawn from this study. First, the significance of information on adaptation to climate change and social networks (membership in a farmers’ organization) implies that exposure to SWCT is essential to increase the adoption of these technologies. Therefore, the results suggest the need for policies and strategies that promote farmer organizations and effective extension services for greater adoption of soil enhancing and water harvesting technologies. Similarly, policies that center on farmer-to-farmer extension can go a long way in increasing the adoption and diffusion of SWCT. Activities that focus on promoting the exchange of information among farmers, such as farmer field days, can increase the efficiency of social networks in promoting the adoption of agricultural technologies (Mumin and Abdulai, 2021).
Second, the IVQTEs provide a basis for effective targeting of SWCT aimed at improving the well-being of smallholder farmers. As mentioned earlier, SWCT are labor intensive, and farm households require substantial investments to adopt the technologies. This implies that farmers who are likely to be adopters and benefit from SWCT are also expected to incur high fixed and variable costs. Easing these barriers would therefore increase the benefits of adoption. Policies targeting the poor non-adopters, such as improved access to credit facilities and enhanced information on SWCT, are vital for poor farmers to adopt and benefit from these technologies.
Data availability statement
The raw data supporting the conclusions of this article will be made available by the authors, without undue reservation.
Ethics statement
The studies involving human participants were reviewed and approved by IITA Internal Review Board. Written informed consent for participation was not required for this study in accordance with the national legislation and the institutional requirements.
Author contributions
JM conceptualized and designed the study, conducted the analysis of the data, and wrote the first draft of the manuscript. All authors contributed to the article and approved the submitted version.
Funding
This work was supported by the United States Agency for International Development (USAID) through the Africa Research in Sustainable Intensification for the Next Generation (Africa RISING) program.
Acknowledgments
The content of this manuscript has been presented [IN PART] at the [International Conference of Agricultural Economists (ICAE), 17th–31st August 2021; Manda et al., 2021].
Conflict of interest
The authors declare that the research was conducted in the absence of any commercial or financial relationships that could be construed as a potential conflict of interest.
Publisher’s note
All claims expressed in this article are solely those of the authors and do not necessarily represent those of their affiliated organizations, or those of the publisher, the editors and the reviewers. Any product that may be evaluated in this article, or claim that may be made by its manufacturer, is not guaranteed or endorsed by the publisher.
Supplementary material
The Supplementary material for this article can be found online at: https://www.frontiersin.org/articles/10.3389/fsufs.2023.1146678/full#supplementary-material
Footnotes
2. ^A farmer is considered to have adopted if they used SWCT in the 2019/2020 growing season.
3. ^As our primary interest is examining the determinants and impacts of adopting SWCT, we are not going to interpret these results.
4. ^The results are not the same owing to somewhat different specification and number of observations across the models.
5. ^This was transformed to percent using the following formula: [e(Impact estimate) – 1]*100.
References
Abadie, A., and Cattaneo, M. D. (2018). Econometric methods for program evaluation. Annu. Rev. Econ. 10, 465–503. doi: 10.1146/annurev-economics-080217-053402
Abdulai, A., and Huffman, W. (2014). The adoption and impact of soil and water conservation technology: an endogenous switching regression application. Land Econ. 90, 26–43. doi: 10.3368/le.90.1.26
Adegbola, P., and Gardebroek, C. (2007). The effect of information sources on technology adoption and modification decisions. Agric. Econ. 37, 55–65. doi: 10.1111/j.1574-0862.2007.00222.x
Ainembabazi, J. H., Abdoulaye, T., Feleke, S., Alene, A., Dontsop-Nguezet, P. M., Ndayisaba, P. C., et al. (2018). Who benefits from which agricultural research-for-development technologies? Evidence from farm household poverty analysis in Central Africa. World Dev. 108, 28–46. doi: 10.1016/j.worlddev.2018.03.013
Alene, A., and Manyong, V. M. (2007). The effects of education on agricultural productivity under traditional and improved technology in northern Nigeria: an endogenous switching regression analysis. Empir. Econ. 32, 141–159. doi: 10.1007/s00181-006-0076-3
Brand, J. E., and Xie, Y. (2010). Who benefits most from college? Evidence for negative selection in heterogeneous economic returns to higher education. Am. Sociol. Rev. 75, 273–302. doi: 10.1177/0003122410363567
Carletto, C., Savastano, S., and Zezza, A. (2013). Fact or artefact: the impact of measurement errors on the farm size-productivity relationship. J Dev. Econ. 103, 254–261. doi: 10.1016/j.jdeveco.2013.03.004
Coates, J., Swindale, A., and Bilinsky, P. (2007). Household Food Insecurity Access Scale (HFIAS) for Measurement of Household Food Access: Indicator Guide (v. 3). Washington, D.C.: Food and Nutrition Technical Assistance Project, Academy for Educational Development.
Di Falco, S., and Veronesi, M. (2013). How can African agriculture adapt to climate change? A counterfactual analysis from Ethiopia. Land Econ. 89, 743–766. doi: 10.3368/le.89.4.743
Di Falco, S., Veronesi, M., and Yesuf, M. (2011). Does adaptation to climate change provide food security? A micro-perspective from Ethiopia. Am. J. Agric. Econ. 93, 829–846. doi: 10.1093/ajae/aar006
FAO. (2014). Adapting to climate change through land and water management in eastern Africa. Results of pilot projects in Ethiopia, Kenya, and Tanzania. Food and agriculture Organization of the United Nations. Rome
Feder, G. R., Just, R. E., and Zilberman, D. (1985). Adoption of agricultural innovations in developing countries: a survey. Econ. Dev. Cult. Chang. 33, 255–298. doi: 10.1086/451461
Feder, G., and Umali, D. L. (1993). The adoption of agricultural innovations: a review. Technol. Forecast. Soc. Change 43, 215–239. doi: 10.1016/0040-1625(93)90053-A
Frölich, M., and Melly, B. (2010). Estimation of quantile treatment effects with Stata. Stata J. 10, 423–457. doi: 10.1177/1536867x1001000309
Frölich, M., and Melly, B. (2013). Unconditional quantile treatment effects under endogeneity. J. Bus. Econ. Stat. 31, 346–357. doi: 10.1080/07350015.2013.803869
Habtemariam, L. T., Mgeni, C. P., Mutabazi, K. D., and Sieber, S. (2019). The farm income and food security implications of adopting fertilizer micro-dosing and tied-ridge technologies under semi-arid environments in Central Tanzania. J. Arid Environ. 166, 60–67. doi: 10.1016/j.jaridenv.2019.02.011
Issahaku, G., and Abdulai, A. (2019). Can farm households improve food and nutrition security through the adoption of climate-smart practices? Empirical evidence from northern Ghana. Appl. Econ. Perspect. Policy 42, 559–579. doi: 10.1093/aepp/ppz002
Jayne, T. S., Snapp, S., Place, F., and Sitko, N. (2019). Sustainable agricultural intensification in an era of rural transformation in Africa. Glob. Food Sec. 20, 105–113. doi: 10.1016/j.gfs.2019.01.008
Kabunga, N. S., Dubois, T., and Qaim, M. (2012). Yield effects of tissue culture bananas in Kenya: accounting for selection bias and the role of complementary inputs. J. Agric. Econ. 63, 444–464. doi: 10.1111/j.1477-9552.2012.00337.x
Kassie, M., Jaleta, M., Shiferaw, B., Mmbando, F., and Mekuria, M. (2013). Adoption of interrelated sustainable agricultural practices in smallholder systems: evidence from rural Tanzania. Technol. Forecast. Soc. Change 80, 525–540. doi: 10.1016/j.techfore.2012.08.007
Kassie, M., Köhlin, G., Bluffstone, R., and Holden, S. (2011). Are soil conservation technologies “win-win?” a case study of Anjeni in the north-western Ethiopian highlands. Nat. Resour. Forum 35, 89–99. doi: 10.1111/j.1477-8947.2011.01379.x
Kassie, M., Pender, J., Yesuf, M., Kohlin, G., Bluffstone, R., and Elias, M. (2008). Estimating returns to soil conservation adoption in the northern Ethiopian highlands. Agric. Econ. 38, 213–232. doi: 10.1111/j.1574-0862.2008.00295.x
Kato, E., Ringler, C., Yesuf, M., and Bryan, E. (2011). Soil and water conservation technologies: a buffer against production risk in the face of climate change? Insights from the Nile basin in Ethiopia. Agric. Econ. 42, 593–604. doi: 10.1111/j.1574-0862.2011.00539.x
Kennedy, G., Ballard, T., and Dop, M. C. (2010). Guidelines for measuring household and individual dietary diversity. Food and Agriculture Organization of the United Nations.
Khanal, A. R., Mishra, S. K., and Honey, U. (2018). Certified organic food production, financial performance, and farm size: an unconditional quantile regression approach. Land Use Policy 78, 367–376. doi: 10.1016/j.landusepol.2018.07.012
Leroy, J. L., Ruel, M., Frongillo, E. A., Harris, J., and Ballard, T. J. (2015). Measuring the food access dimension of food security: a critical review and mapping of indicators. Food Nutr. Bull. 36, 167–195. doi: 10.1177/0379572115587274
Maggio, G., Mastrorillo, M., and Sitko, N. J. (2021). Adapting to high temperatures: effect of farm practices and their adoption duration on Total value of crop production in Uganda. Am. J. Agric. Econ. 104, 385–403. doi: 10.1111/ajae.12229
Manda, J., Alene, A. D., Tufa, A. H., Abdoulaye, T., Kamara, A. Y., Olufajo, O., et al. (2019). Adoption and ex-post impacts of improved cowpea varieties on productivity and net returns in Nigeria. J. Agric. Econ. 71, 165–183. doi: 10.1111/1477-9552.12331
Manda, J., Azzarri, C., Feleke, S., Kotu, B., Claessens, L., and Bekunda, M. (2021). Welfare impacts of smallholder farmers’ participation in multiple output markets: Empirical evidence from Tanzania. PLoS One. 16, 1–20. doi: 10.1371/journal.pone.0250848
Manda, J., Khonje, M. G., Alene, A. D., and Gondwe, T. (2017). Welfare impacts of improved groundnut varieties in eastern Zambia: a heterogeneous treatment effects approach. Agrekon 56, 313–329. doi: 10.1080/03031853.2017.1400447
Mishra, A. K., Mottaleb, K. A., and Mohanty, S. (2015). Impact of off-farm income on food expenditures in rural Bangladesh: an unconditional quantile regression approach. Agric. Econ. 46, 139–148. doi: 10.1111/agec.12146
Mueller, N. D., Gerber, J. S., Johnston, M., Ray, D. K., Ramankutty, N., and Foley, J. A. (2012). Closing yield gaps through nutrient and water management. Nature 490, 254–257. doi: 10.1038/nature11420
Mulwa, C. K., Muyanga, M., and Visser, M. (2021). The role of large traders in driving sustainable agricultural intensification in smallholder farms: Evidence from Kenya. Agric. Econ. 52, 329–341. doi: 10.1111/agec.12621
Mumin, Y. A., and Abdulai, A. (2021). Social networks, adoption of improved variety and household welfare: evidence from Ghana. Eur. Rev. Agric. Econ. 49, 1–32. doi: 10.1093/erae/jbab007
Navarro, S. (2010). “Control functions” in Microeconometrics. (London: Palgrave Macmillan UK), 20–28.
Ogutu, S. O., Gödecke, T., and Qaim, M. (2019). Agricultural commercialisation and nutrition in smallholder farm households. J. Agric. Econ. 71, 534–555. doi: 10.1111/1477-9552.12359
Ojo, T. O., and Baiyegunhi, L. J. S. (2020). Determinants of climate change adaptation strategies and its impact on the net farm income of rice farmers in south West Nigeria. Land Use Policy 95:103946. doi: 10.1016/j.landusepol.2019.04.007
Saiz, G., Wandera, F. M., Pelster, D. E., Ngetich, W., Okalebo, J. R., Rufino, M. C., et al. (2016). Long-term assessment of soil and water conservation measures (Fanya-juu terraces) on soil organic matter in south eastern Kenya. Geoderma 274, 1–9. doi: 10.1016/j.geoderma.2016.03.022
Schmidhuber, J., and Tubiello, F. N. (2007). Global food security under climate change. Proc. Natl. Acad. Sci. 104, 19703–19708. doi: 10.1073/pnas.0701976104
Shiferaw, B., Kassie, M., Jaleta, M., and Yirga, C. (2014). Adoption of improved wheat varieties and impacts on household food security in Ethiopia. Food Policy 44, 272–284. doi: 10.1016/j.foodpol.2013.09.012
Smale, M., Moursi, M., and Birol, E. (2015). How does adopting hybrid maize affect dietary diversity on family farms? Micro-evidence from Zambia. Food Policy 52, 44–53. doi: 10.1016/j.foodpol.2015.03.001
Suri, T. (2011). Selection and comparative advantage in technology adoption. Econometrica 79, 159–209. doi: 10.3982/ecta7749
Terza, J. V., Basu, A., and Rathouz, P. J. (2008). Two-stage residual inclusion estimation: addressing endogeneity in health econometric modeling. J. Health Econ. 27, 531–543. doi: 10.1016/j.jhealeco.2007.09.009
Tsubo, M., Walker, S., and Hensley, M. (2005). Quantifying risk for water harvesting under semi-arid conditions. Agric Water Manag 76, 77–93. doi: 10.1016/j.agwat.2005.01.008
Wiyo, K. A., Kasomekera, Z. M., and Feyen, J. (2000). Effect of tied-ridging on soil water status of a maize crop under Malawi conditions. Agric Water Manag 45, 101–125. doi: 10.1016/S0378-3774(99)00103-1
Wooldridge, J. M. (2010). Econometric analysis of cross-section and panel data. 2nd Edn. Cambridge, Massachusetts: MIT press.
Wooldridge, J. M. (2014). Quasi-maximum likelihood estimation and testing for nonlinear models with endogenous explanatory variables. J. Econ. 182, 226–234. doi: 10.1016/j.jeconom.2014.04.020
Wooldridge, J. M. (2015). Control function methods in applied econometrics. J. Hum. Resour. 50, 420–445. doi: 10.1093/ajae/aay017
Wossen, T., Alene, A., Abdoulaye, T., Feleke, S., Rabbi, I. Y., and Manyong, V. (2018). Poverty reduction effects of agricultural technology adoption: the case of improved cassava varieties in Nigeria. J. Agric. Econ. 70, 392–407. doi: 10.1111/1477-9552.12296
Xie, Y., Brand, J. E., and Jann, B. (2012). Estimating heterogeneous treatment effects with observational data. Sociol. Methodol. 42, 314–347. doi: 10.1177/0081175012452652
Keywords: soil and water conservation technologies, distributional impacts, income, food security, Tanzania
Citation: Manda J, Tufa AH, Alene A, Swai E, Muthoni F, Hoeschle-Zeledon I and Bekunda M (2023) The income and food security impacts of soil and water conservation technologies in Tanzania. Front. Sustain. Food Syst. 7:1146678. doi: 10.3389/fsufs.2023.1146678
Edited by:
Ole Mertz, University of Copenhagen, DenmarkReviewed by:
John N. Ng'ombe, North Carolina Agricultural and Technical State University, United StatesTemitope Oluwaseun Ojo, Obafemi Awolowo University, Nigeria
Copyright © 2023 Manda, Tufa, Alene, Swai, Muthoni, Hoeschle-Zeledon and Bekunda. This is an open-access article distributed under the terms of the Creative Commons Attribution License (CC BY). The use, distribution or reproduction in other forums is permitted, provided the original author(s) and the copyright owner(s) are credited and that the original publication in this journal is cited, in accordance with accepted academic practice. No use, distribution or reproduction is permitted which does not comply with these terms.
*Correspondence: Julius Manda, ai5tYW5kYUBjZ2lhci5vcmc=