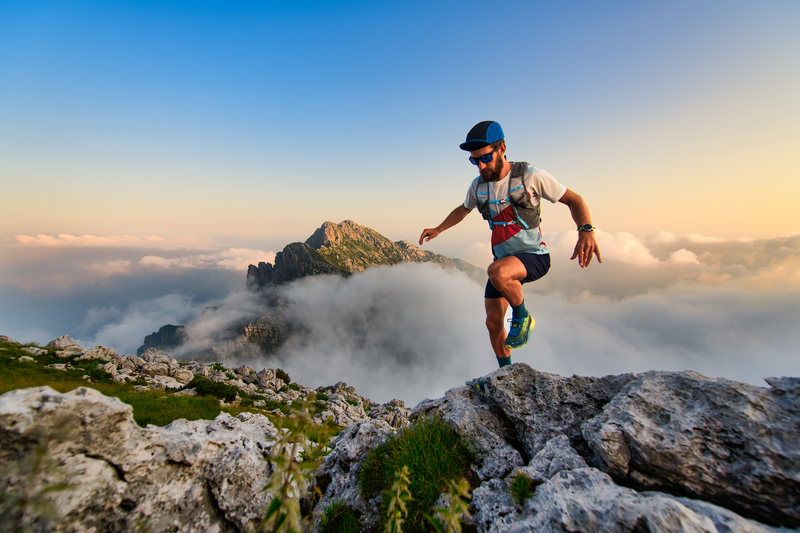
95% of researchers rate our articles as excellent or good
Learn more about the work of our research integrity team to safeguard the quality of each article we publish.
Find out more
ORIGINAL RESEARCH article
Front. Sustain. Food Syst. , 29 August 2023
Sec. Land, Livelihoods and Food Security
Volume 7 - 2023 | https://doi.org/10.3389/fsufs.2023.1132732
Introduction: Uncertainty in the yield of maize due to variability in weather is a major challenge to smallholder farmers in Sub Sahara Africa. This study explores the potential of combining locally available organic resources and inorganic fertilizer to increase grain yield and reduce variability in yields associated with variations in rainfall distribution.
Methods: To assess the effectiveness of this practice, the Agricultural Production Systems sIMulator (APSIM) crop model was calibrated and evaluated using maize experiments on nutrient management options. The evaluated model was used to simulate maize growth and yield using multiple-year data (1984–2018) under different planting windows for two growing seasons. The treatments were (i) control, (ii) inorganic fertilizer alone (INOFRecom), combining organic resources [empty fruit bunch of palm (EFB) and compost with inorganic fertilizer (INOF) to make up equivalent nutrients in (ii)]; (iii) EFB + INOF and (iv) Comp + INOF.
Results: Though all the soil amendments boosted grain output, the EFB + INOF treatment outperformed the other treatments in the major season with gains of between 161 and 211% and the most stable (least inter-annual variability of 27%) yield. Across the planting windows, the INOFRecom and EFB + INOF treatments achieved comparable yield increments in the minor season. Though grain yield variability was high during the minor rainy season, combining organic and inorganic fertilizers reduced inter-annual yield variability, thus, lowering uncertainty in yield due to variable inter-annual rainfall. Combining local organic resources with a reduced amount of inorganic fertilizer produced higher yields and better yield stability compared to using only inorganic fertilizer.
Discussions: Thus, such soil fertility management solutions might sustain resource use and boost maize grain yield in the study area, where strategies for sustainable crop nutrition remain a critical necessity. The improved nitrogen management regimes may result in fewer environmental hazards for vulnerable rainfed agricultural systems.
Maize is a major staple crop in most countries in Sub-Sahara Africa. It is an essential source of food, feed and an industrial crop in the sub-region. Hence, it is essential for food and nutrition security as well as contributes to livelihoods of many of its population (Badu-Apraku and Fakorede, 2017). Approximately 20% of the calorie intake for about 50% of the population comes from maize. Yet, its productivity between 2015 and 2018 averaged about 2 Mg ha−1 which is only approximately 20% of the average yield produced from North America and Europe (Leitner et al., 2020). This yield gap is due to several constraints, such as inherently poor and declining soil fertility and sub-optimal agronomic practices. Stewart et al. (2020) indeed attributed poor soil fertility in Sub-Sahara Africa (SSA) to decades of continuous cropping with little or no external inputs resulting in poor productivity over the years. Another major yield-limiting factor is the heavy reliance on rainfall which is increasingly becoming more erratic (MacCarthy et al., 2017; Van Loon et al., 2019).
Despite the issue of low and declining soil fertility, the use of inorganic fertilizers remains limited due to the inability of farmers to afford them (Shiferaw et al., 2014; MacCarthy et al., 2018a) as well as the low efficiency of inorganic fertilizers due to, among other factors, low soil organic carbon content. The continuous depletion of soil organic carbon and the rising cost of inorganic fertilizers (Lamers et al., 2015) make recycling of locally available crop residues an important strategy for soil fertility management in the predominantly low-inputs maize-based cropping systems in Ghana. The recycling of residues has important benefits such as the build-up of soil organic matter and nutrient reserves which subsequently affect nutrient supply and uptake, soil water-conservation and use efficiency of crops (Adetunji, 1997; Naab et al., 2015; Bationo et al., 2018) as well as soil health. Integrating organic resources with inorganic fertilizers has been reported to control soil acidity (Hue, 1992; Noble et al., 1996) and enhance the nutrient use efficiency of inorganic fertilizers (MacCarthy et al., 2020). Hence, the growing interest in the use of crop residues to sustain the efficiency of fertilizer use (Khalid et al., 2000; Ayeni et al., 2008a,b; Adekayode and Olojugba, 2010) in the Integrated Soil Fertility Management (ISFM) packages (Bationo et al., 2007). However, the use of organic resources in crop production is constrained due to its low nutrient content which require large amount for application, and competing use as feed for livestock, and/or as domestic fuel (Lal, 2006).
The major organic residues used for the study were empty fruit bunches (EFB) (Rhebergen et al., 2020). These are residues generated from the processing of palm fruits into oil. About 2,000,000 metric tons of fresh fruit bunches (FFB) are produced annually with 60% coming from small-scale processors (Opoku and Asante, 2008; Osei-Amponsah et al., 2012). Approximately 22% of the FFB processed into oil end up as EFB (Ng et al., 2012) which is usually burnt as a means of disposal in small-scale mills, resulting in heaps of ash dotted in major oil palm producing areas in the country (Adjei-Nsiah, 2012). Given the large quantities of EFB generated annually, it can be composted or used as mulch in combination with inorganic fertilizer for soil fertility management and crop production. Abu Bakar et al. (2011) in their ten-year study reported significant increase in the yield of fresh palm fruits when EFB was applied to palm. The yield increase was attributed to the positive effects of the EFB on soil physicochemical properties. EFB contains both macro- and micro- nutrients (Kavitha et al., 2013) and can improve soil structure (Shindo et al., 2006) and water holding capacity, and reduce soil temperature (Rosenani and Hoe, 1996). It is estimated that EFB contains 30–40% K2O and could thus serve as a source of potassium (Lim and Zaharah, 2000).
An important attribute of soil fertility technology that encourages its adoption by smallholders is its ability to reduce inter-annual yield variation (Begho et al., 2022) due mainly to variability in rainfall distribution. Although several studies have reported on the benefits of EFB, there is still limited information on its performance under variable weather conditions as reported studies usually span a period of up to 3 years. Promoting the use of EFB as an organic resource in integrated nutrient management requires its evaluation under varied weather conditions and planting dates. To achieve this requires gathering experimental data for several years that will be time-consuming and costly; hence, cropping systems models that can simulate crop yields under varied crop management practices, variable soil, and weather (MacCarthy et al., 2015) are explored (calibrated and evaluated with 2 years of experimental data) to answer the above research question.
Given the increasing cost of inorganic fertilizers, can we reduce the amounts needed by supplementing with locally available organic resources to obtain comparable yield (when only recommended inorganic fertilizer is used) while minimizing inter-annual variability in yield? This study assessed the short-term benefits of integrating locally available organic resources (such as EFB mulch and EFB-based compost) with inorganic fertilizer on maize productivity and yield stability, as well as water use efficiency using a crop simulation model.
The experiments were conducted at the Soil and Irrigation Research Centre (SIREC), Kpong, and the Forest and Horticultural Crops Research Centre (FOHCREC), Kade (Figure 1) for the calibration and evaluation (respectively) of Agricultural Production Systems sIMulator (APSIM). The SIREC site is situated, in the coastal Savannah agro ecological zone. It is located at latitude 6° 09′ N and longitude 00°04′ E. The research station has a bi-modal rainfall pattern (major from May to July and minor season from September to November) and a brief dry spell in August and a dry season from December to April) with an annual rainfall of between 800–1,326 mm (MacCarthy et al., 2018b). The dominant soils are classified as, Calcic Vertisols. Similarly, the FOHCREC is located in the semi-deciduous forest agro-ecological zone of Ghana. It lies between latitude 6°09’and 6°06’N and longitude 0°55’and 0°49’W at 135.9 m above sea level. It has annual rainfall ranging from 1,300–1800 mm (Adjei-Nsiah, 2012). The soils are predominantly forest ochrosol derived from precambium phyllitic rock (Ahn, 1961). They are deep and well-drained soils that are generally classified as Acrisols according to the FAO-UNESCO Revised Legend.
The Agricultural Production Systems sIMulator (APSIM v 7.4) model allows users to estimate crop growth, development, and yield using management strategies and environmental factors as input parameters. The model is capable of simulating soil water, C, N and P dynamics and their interactions within crop/management systems using daily climatic data (solar radiation, maximum and minimum temperatures, rainfall). Using stage-related radiation-use efficiency (RUE) which is regulated by climate and available leaf area, daily potential production for a range of crop species is calculated and further limited to actual above-ground biomass production on a daily basis by soil water, nitrogen and (for some crop modules) phosphorus availability (Keating et al., 2003). APSIM consists of a range of component modules (crop, soil and utility modules) that users can use to configure specific modes. The modules used for this study are the Maize, SURFACEOM, soilP, soilN, and soilWAT modules. SoilP module describes the availability of P from labile P pool and fluxes into and out of this pool. Dynamics and transformation of both carbon (C) and nitrogen (N) on a layer basis in the soil are handled by SoilN. These include organic matter decomposition, N immobilization, urea hydrolysis, ammonification, nitrification and denitrification. The SURFACEOM module simulates the fate of the above-ground crop residue that can be removed from the system, incorporated into the soil or left to decompose on the soil surface. It differentiates soil organic C into two pools; “biom” the more labile and “hum” the less labile form. The soil’s fresh organic matter (FOM) pool, which can decompose to form the BIOM (microbial biomass), HUM (humus), and mineral N (NO3 and NH4) pools, is made up of the crop residue that has been tilled into the ground along with the roots of previous crops. The more labile soil microbial biomass and microbial products are conceptually represented by the BIOM pool, whilst the remaining soil organic matter (SOM) is conceptually represented by the more stable HUM pool (Probert et al., 1998). Flows between pools are calculated in terms of C, while the corresponding N is determined by the CN ratio of the receiving pool. The water balance and solute movements within APSIM model are handled by the soilWAT (a cascading layer model) (Probert et al., 1998; Keating et al., 2003). Additional information on the APSIM model can be found in Holzworth et al., (Holzworth et al., 2014) and Keating et al., (Keating et al., 2003).
Four experimental trials were carried out at SIREC. Data used for the calibration were obtained from two sets of data obtained from (i) a planting date trial with three (3) sowings done on 13th July, 6th September, and 26th September 2014 and (ii) a second data set with sowing done on 31st May 2016 in the major season using Abontem maize variety. Seeding was done at four (4) seeds per hill at 0.8 m x 0.4 m spacing and later thinned to two (2) plants per hill 10 days after emergence. Supplemental irrigation was applied on July 7th, August 12th, September 5th and 9th in2014 to avoid water stress on plants. A total of 90 kg of N/ha, and 45 kg/ha of P and K in the form of Triple Super Phosphate (TSP) and Potassium Chloride (KCl) respectively were applied to the plants. The basal application involved half the amount of N and all the P and K rates applied at 14 days after emergence. The remaining half of the N rate was applied as top dressing 45 days after planting. Weed control was done manually by the use of a hoe. Soil profile data were sampled and analysed for model calibration. The samples (disturbed and undisturbed) were analysed for particle size distribution using Bouyoucos hydrometer method as modified by Day, (1965). Soil organic carbon was determined following Walkley and Black, (1934) method. Soil pH was determined in a soil-to-water ratio of 1:1. Bulk density was determined using the core sampler approach (Blake and Hartge, 1986). A pedo-transfer function as described in MacCarthy et al. (2015) was then used to derive field capacity, wilting point and saturation water content of the soils.
The APSIM-maize model was calibrated using soil, weather, and management datasets collected from the trials conducted in Kpong. The soil data in Appendix 1 summarizes the parameters used in model calibration at SIREC. Weather data (daily rainfall, minimum and maximum temperature, and solar radiation) were obtained from the agro-meteorological station at the SIREC meteorological station. Data on crop phenology together with the daily weather data were used to estimate thermal times and genetic co-efficient (Table 1) for the maize variety. Calibration of crop genetic coefficients associated with phenology was first done, followed by those associated with growth parameters and yield parameters. The Thermal time (Tt) is calculated as a function of the mean temperature above a base temperature (Tb).
where Tb is the base temperature taken as 8°C for maize. The genetic coefficients obtained after calibrating the Abontem maize cultivar are presented in Table 1.
Four seasons experiment was conducted at Kade from 2017 to 2018. The experiment consisted of four treatments (Table 2) and was laid out in a randomized complete block design with three replicates. The quantity of inorganic fertilizer added to the organic fertilizer (EFB and EFB-based compost) for the EFB + INOF and COMP +INOF treatments ensured that they have similar nutrient (N, P, and K) content as the treatment with the recommended inorganic fertilizer (INOFRecom).
Sowings of maize were done on 16th May and 3rd October in 2017 and, 19th April and 3rd October in 2018 with a plant density of 3.5 plants m-2 of the Abontem maize (an early maturing cultivar). The EFB was applied as mulch while the EFB-based compost was incorporated into the soil two weeks prior to sowing and the amounts used are presented on Table 2. Inorganic N fertilizers were applied at 14 and 45 days after planting while the P and K fertilizers were applied at 14 days after planting. Soil samples were taken at six different depths (Table 3). The samples (disturbed and undisturbed) were analysed for particle size distribution, organic carbon, and pH. As was done for the SIREC site, a pedo-transfer function was then used to derive the field capacity, wilting point, and saturation water content of the soils. The relevant soil data (Table 3) together with weather data collected from a nearby weather station as well as management data from the experiment served as input that was used to evaluate the performance of the APSIM model in reproducing crop phenology, total biomass, and grain yield. Simulated phenology (duration to anthesis and maturity), total biomass, and grain yield were compared to those obtained from the experiment using statistical indices elaborated in the next section to assess model performance.
The ability of the model to reproduce observed data on crop phenology, grain, and biomass yield was assessed using root mean square error (RMSE), relative root mean square error (RRMSE), and Willmott d-index.
The root means square error (RMSE) indicates the error associated with the predicted outcomes and a value of zero signifies a perfect prediction. It is defined as:
Where Pi is model predicted value, Oi is observed value, i is the index of observation and n is the total number of observations. A low RMSE is desirable, as this would signify a better agreement between the predicted and observed data. Relative root mean square is defined as;
where is the average of observed values.
Willmott’s d index is defined as:
The Willmott d-value ranges from 0 to 1. A value of 1 signifies a perfect prediction of observed data.
The impact of the various treatments under variable weather conditions was assessed with 35-year (1984–2018) weather data downloaded from NASA power (NASA Langley Research Center, 2019). The data set from 2017 and 2021 was compared to observed monthly station data for the same years to evaluate its suitability to capturing monthly rainfall (monthly accumulated), minimum and maximum temperature (monthly average). The NASA power data compared favouarably with station data with RMSE of 4.87 mm, 0.07°C, and 0.18°C for rainfall, Tmax and Tmin, respectively. Comparison using Norman Pearson correlation gave coefficients of 0.86, 0.87 and 0.61, respectively, for monthly rainfall, Tmax and Tmin distributions. The long-term NASA power data was deemed to capture the weather of the study area and hence, used in the scenario analysis. Ten (10) sowing windows (6 in the major season and 4 in the minor season) with each window lasting two weeks were created. The entire sowing windows in the major season started on March 15 and ended on June 6 whilst the minor season sowing windows started on September 1 and ended on October 26. The model was set up to sow when a 25 mm cumulative rainfall amount was acquired from 3 rainfall events within each sowing window to mimic farmer practices of sowing their crops after rainfall events. Automatic sowing was applied each year when the sowing criteria were met for each window. Simulations for each year and season were independent of each other as soil parameters were reinitialised 30 days prior to planting in each season and year. Hence, simulated yields for the 35 years were independent of each other.
Inter-annual variability in grain yield under the various treatments was determined as in equation 5:
Where SGYt is the standard deviation of grain yield across the years per treatment while MGYt is the average grain yield across the years per treatment.
To determine the effects of the treatments on grain yield, yearly grain yields obtained from the seasonal simulations were subjected to repeated measures ANOVA to determine if a significant difference exists between the seasons. Each planting window was further subjected to analysis of variance (ANOVA) to determine if there were statistically significant differences among the treatments as influenced by changing climate. The 35-year average of each treatment was computed together with the variance. The GENSTAT 12th Edition was used to perform an analysis of variance (ANOVA) in order to compare yield and total biomass data from the different treatments. The relationship between grain yield and rainfall amount was analysed through correlation with the null hypothesis that there is no statistically significant correlation between grain yield and cumulative rainfall from sowing to harvest for each sowing.
The weather as experienced during the field experiment at FOHCREC, Kade, is shown in Figure 2. Mean temperature recorded over the period was 32.6°C and 22.1°C for maximum (Tmax) and minimum (Tmin) temperatures, respectively. The average solar radiation throughout the experiment was 17.5 MJ m−2 day−1. Annual rainfall was 1,310 mm and 1,591 mm for 2017 and 2018, respectively.
Figure 2. Daily temperature and precipitation during the (A) Major season 2017, (B) Minor season 2017, (C) Major season 2018 and (D) Minor season 2018.
Analysis carried out on the long-term weather data for the location gave an average annual rainfall amount of 1,281 mm (Figure 3). Mean annual minimum and maximum temperatures were 22 and 31°C, respectively. The average onset of the rains for the major season was May 4 (124 day of year (DOY)) with an average end of season on August 6 (218 DOY). Using an onset criterion of 20 mm accumulated over 2 days with no dry spell for the next 21 days, the onset of the major season rain ranged from April 2 (92 DOY) to June 21 (172 DOY) whilst the end of season ranged from July 2 (183 DOY) to November 22 (326 DOY). For the minor season, onset of the rain ranged from August 2 (214 DOY) to October 29 (302 DOY) with an average on September 4 (247 DOY). End of the minor reason ranged from November 2 (306 DOY) to December 5 (339 DOY) with a mean on November 12 (316 DOY) according to the long-term data analysis. The analysis of temporal distribution of the temperature over the period showed a general bimodal distribution with the least temperature occurring in the months of July and August. One peak occurs between February and March, while the other between October to December.
Figure 3. Average monthly minimum and maximum temperature and precipitation at FOHCREC, Kade from 1983–2018. Solid and broken lines represent maximum and minimum temperatures, respectively.
Following the calibration of the genetic coefficients, the model adequately captured the phenology of maize with flowering and maturity at 45 days (RMSE and RRMSE of 2.5 days and 5.2%, respectively) after sowing (DAS) and 86 DAS (RMSE and RRMSE of 3.0 days and 3.4%, respectively), respectively. The model realistically predicted the observed biomass yield with d-value of 0.70 and RRMSE of 15%. Observed grain yield (3,815 kg ha−1) was also accurately simulated with d-value of 0.95 and RRMSE of 5%. The genetic coefficients used for the calibration (Table 1) were used for evaluating the model. Phenology was well captured by the model simulating 46 days for days to flowering with RMSE (RRMSE) of 3 days (6.4%) and 89 days for days to maturity with RMSE (RRMSE) of 4 days (4.5%). Observed grain yields ranging from 1,163–3,188 kg ha−1 and biomass yields ranging between 4,345–13,397 kg ha−1 were adequately captured with Willmott’s d-values of 0.79 for grain and biomass yields and RRMSE less than 20% (Figure 4). However, at lower observed yields, the model systematically over-estimated and at higher observed yields, yields tend to be under-estimated.
Figure 4. Comparison of measured and simulated grain (A) and total biomass (B) yield of maize grown under different soil amendment options at Kade, Ghana a semi-humid region of Ghana.
The average yield of grain varied among the treatments with the control treatment consistently producing the lowest yield while the EFB + INOF produced the highest yield across most of the planting windows (Figure 5) in the major cropping season. In the minor season, average grain yields declined as planting was delayed. Except for the last planting window, the treatments with the INOFRecom and the EFB + INOF produced similar average grain yields. The inter-annual variability in grain yields within planting windows were generally higher in the minor season compared to the major season. For the control treatment for instance, the CV ranged between 7 and 15% in the major season compared to 12 and 34% in the minor season. Similar trends were observed with the use of the amendments. Variability in yields were also higher under the soil amendment treatments compared to the control (8 and 24% in major season compared with 17 and 72% in the minor season using the NPK fertilizer alone.
Figure 5. Simulated effect of amendments on maize grain yield over varied planting dates during two different seasons in the semi-deciduous forest agro-ecological zone of Ghana. INOFRecom, EFB + INOF and Comp+INOF are inorganic fertilizer alone, empty fruit bunch with urea and triple super phosphate, and EFB-based compost with urea and triple super phosphate, respectively. Each box represents the spread in yields response across 35 years.
Average grain yield varied across planting windows among soil amendments. Yields under the control treatment in the major season ranged from an average of 1,167 in the first planting window (March 15–28) to 1,409 kg ha−1 in the May 10–23 planting window. Stability in average grain yield was generally low with the earlier two planting windows recording higher yield variability (CV 14 and 15%). Applying inorganic fertilizer alone resulted in higher average grain yield of between 1969 and 3,143 kg ha−1 representing yield increases of between 41 and 167% over the control yields (Figure 6). Using EFB + INOF produced average grain yields of between 3,066 and 3,546 kg ha−1 which translates into yield increases of between 136 and 183%. While the use of Comp+INOF produced average grain yield increases of between 61 and 74%, the highest increase in average grain yield across sowing windows was obtained under the amendments with EFB + INOF while the treatment with Compost + INOF recorded the lowest average grain yield increase over the control yields. Variation in average increases in grain yield were higher with the use of inorganic fertilizer alone (27%) compared to those treatments with organic fertilizer integrated in them (18 and 12% for EFB + INOF and Compost + INOF respectively).
Figure 6. Variability in the simulated average grain yield increases relative to the control in response to various soil amendments across planting windows. INOFRecom, EFB + INOF and Comp+INOF are inorganic fertilizer alone, empty fruit bunch with urea and triple super phosphate, and EFB-based compost with urea and triple super phosphate, respectively. Each box represents the spread in yields across planting windows.
In the minor season, simulated average grain yield under the control ranged from 829 kg ha−1 in the fourth planting window (October 13–26) to 1,339 kg ha−1 in the first planting window (September 1–14). Thus, as in the major season where average grain yield varied (CV = 10%) across the various planting windows, a higher magnitude (CV = 22%) was obtained for the minor season. Applying inorganic fertilizer in the minor season produced average grain yields ranging between 1,284 and 3,452 kg ha−1 which represent yield increases of between 41 and 157% over the control yields. The average grain yield increases obtained with the use of the EFB + INOF across planting windows were similar to those obtained using INOFRecom. However, variation in average yields obtained across windows were slightly higher with the use of INOFRecom (CV = 41% for INOFRecom and 39% for EFB + INOF). Using Comp+ INOF resulted in much lower yield increases of between 30 and 64% across planting windows compared to the other two amendments (INOFRecom and EFB). Variability in grain yield across planting windows were generally higher in the minor season compared to the major season.
While the use of EFB + INOF produced the highest average yield increase over the control in the major season, yield increases in the minor season for the EFB + INOF and INOFRecom were similar (106 and 109% respectively).
Regression analysis between in-season total rainfall amount and grain yield indicated variation in the coefficient of determination among amendments and across planting windows in both cropping seasons. In the major season, the strength of the relationship between grain yield and in-season total rainfall in the earlier planting windows were generally higher under the control treatment and the treatment with Comp+INOF compared to the other two amendments. Additionally, the magnitude of the relationship generally declined with delayed planting windows except for the EFB treatment (Figure 7). For the control treatment, for instance, the magnitude of the relationships were 0.45 and 0.47 in the 1st and 2nd planting windows respectively, while the 5th and 6th windows were 0.06 and 0.11, respectively. Similar trends were observed for the other amendments. As in the major season, the magnitude of the relationship between grain yield and in-season total rainfall varied among amendments and across planting windows. The magnitude of the relationship was generally higher in the minor season compared to the major cropping season. Contrary to the trend in the major season, the magnitude of the relationship increased with delayed planting in the minor season.
Figure 7. Influence of amendments on the magnitude of the correlation between grain yield and total in-season rainfall across planting windows in the (A) major and (B) minor cropping seasons. INOFRecom, EFB + INOF, Comp+INOF are inorganic fertilizer alone, empty fruit bunch complemented with inorganic fertilizer and compost complemented with inorganic fertilizer, respectively. Pw1, Pw2, Pw3 Pw4, Pw5 and Pw6 represent planting window 1 to 6.
The UN sustainable Development Goal (SGD) 2 aspires to attain zero hunger and end malnutrition by 2030. To attain this goal in sub-Sahara Africa, a range of strategies are required to increase crop productivity and resource use efficiency. Effective nutrient management strategies will play a vital role in achieving increased crop productivity and efficiency of inputs in the farming systems given the soils are poor in fertility. While studies by MacCarthy et al. (2022) have reported increased productivity with the use of inorganic fertilizers, their usage remains low owing to among other factors, the high cost as well as low efficiency due to the reliance on rainfall which is becoming increasingly erratic. Our study has shown that combining locally available organic resources with reduced inorganic fertilizer can potentially increase maize yield and also reduce inter-annual variability. Integrated nutrient management has been reported to enhance crop productivity particularly on soils with low organic carbon. For instance, combined application of 9.6 t ha−1 FYM with inorganic fertilizer resulted in improved maize yield and N use efficiency in a legume-cereal crop sequence compared to inorganic fertilizer alone (Mamuye et al., 2021; Salama et al., 2021). Similarly, MacCarthy et al. (2020) also reported on improved yield of rice under combined use of inorganic fertilizer and biochar compared to the control condition and instances when only inorganic fertilizer was used. In this study, the combination of inorganic fertilizer with organic resources (EFB) produced grain yields similar to the recommended rate of inorganic fertilizer alone. The benefit of the combined application as explained by Anisuzzaman et al. (2021) is a result of accelerated microbial activity, the quick availability of the inorganic component and the gradual nutrient release from the organic constituents leading to improved soil fertility, higher fertilizer use efficiency and ultimately improved yields (Santhi and Selvakumari, 2000). This is an important finding as farmers can significantly reduce the use of inorganic fertilizer by using EFB which is currently considered a waste in the study area. The lower grain yield in response to the amendment combination with compost compared with that with EFB can be attributed to the higher amount of inorganic fertilizer in the EFB combination. Again, the differences in the nutrient release patterns of the two soil amendments made more nutrient readily available for plant uptake in the later. Additionally, due to the lower nutrient composition of EFB compared to the compost, larger quantities were used and, hence, resulted in higher soil water storage for plant growth. Kiboi et al. (2019) in their study on soil fertility inputs and tillage in the Central highlands of Kenya reported improved soil water holding capacity for treatments that received organic inputs such as crop residues. It is important to note that in this study, simulations did not consider the carry-over effect of amendments from the previous season which could potentially have benefited from residual effects. Thus, simulated yields could have been higher over time compared to what is currently reported. These results contribute to increasing productivity of maize in smallholder systems and, hence, contributes to achieving SDG 2. Strategies that reduce the amount of inorganic fertilizer required while ensuring comparable grain yield as illustrated in this study needs to be promoted for enhanced crop productivity. Even though mean yields under the integrated fertility management are above national average yield of 1.8 t ha−1, they still fall below the potential for the variety in question. Furthermore, it is important to state that, this study did not consider the effect of pest and diseases which contribute significantly to yield loss and hence, the magnitude of yields simulated in this study.
Understanding the effect of the variability in weather conditions on crop production is necessary to sustain or even enhance maize productivity and resource use efficiency in rain-fed farming systems. Variability in yields in response to amendments was higher in the minor rainy season compared to the major season mainly due to poorer rainfall distribution in the former. This study has also shown that combining local organic materials and inorganic fertilizer minimized inter-annual variability in yield. The uncertainty in crop productivity in the sub-region (due to the heavy reliance on rainfall) is usually a disincentive for farmers to invest in inorganic fertilizers. Thus, strategies that reduce the inter-annual variability in grain yields such as using EFB + INO has a high potential for adoption by smallholders.
The benefit of the combined application of organic and inorganic fertilizer depends on the feedstock, nutrient release patterns as well as its impact on soil moisture storage. The quick availability of nutrients in the inorganic component and the slow nutrient release of nutrients in the organic constituents leads to higher fertilizer use efficiency and ultimately improved yields (Vanlauwe et al., 2014). This study showed that irrespective of the sowing windows, the combinations of organic resources with inorganic fertilizers, were likely to increase maize yields.
Within-season variability in rainfall is common in the study area (Adiku et al., 1997; Baffour-Ata et al., 2021), resulting in dry spells at critical plant growth stages, and in turn alters soil moisture and nutrient bioavailability in the predominantly rain-fed cropping systems. The strong positive correlation between grain yield and cumulative rainfall amount over the growing season especially in the minor rainy season was expected given the cropping system was rain-fed. The higher impact of in-season rainfall amount on grain yield suggest rainfall as a major yield limiting factor particularly in the minor season. The reduction in yield has negative implication on the livelihood of rural subsistent farmers. However, the relatively lower impact of in-season rainfall on grain yield for the control treatment suggests other factors such as availability of nutrients was also important in determining grain yield in the study area. A number of studies investigated the relationship between crop yield and other factors such as farm management systems (Fischer, 1985; Affholder et al., 2013) and climate factors such as solar radiation (Yang et al., 2019, 2021) temperature (Ogunkanmi et al., 2021) and rainfall (Cudjoe et al., 2021). The results from Cudjoe et al. (2021) indicated a positive relationship between total rainfall amount and grain yield, emphasizing the importance of the in-season rainfall distribution rather than the total amount alone.
In the absence of meaningful technical adaptation options of nutrient and crop management, the sustainability of maize-based cropping systems is at risk. Adapting planting windows is currently suggested as a coping strategy to mitigate impacts of both uncertainty in rainfall and within season variability in rainfall (MacCarthy et al., 2021). To implement this adaptation strategy, farmers will require support from Meteorological agencies (e.g., Gmet) and other climate advisory institutions including NGOs that provide weather forecast such as onset of the season, and cessation of rains. Addressing other socio-economic constraints (such as access to credit and implements for land preparation) that influences farmers’ decision on when to plant will enable them to make the most of climate and planting date advisories. It is also important to note that seasonal forecasts are associated with a high degree of uncertainty (Taylor et al., 2015; Jackson-Blake et al., 2022), especially in the tropics. Hence, their values in guiding crop management decisions may be limited to some extent. The higher correlation between rainfall amounts and simulated grain yield in the minor cropping season, which is recurrently subject to considerable uncertainty, underscores the urgent need to further explore this coping option in the face of the changing climate, considering germplasm (with earlier maturing varieties) and the interactions between nutrients and water.
The enhanced yield stability reported under the improved nutrient management regime may potentially reduce grain yield fluctuations faced by vulnerable farming populations under rainfed conditions. These soil fertility management options could sustain resource use and enhance productivity in the study area, where sustainable crop nutrition practices are critically needed. The study has highlighted the potential for combining organic resources with inorganic fertilizer to obtain stable maize yields. A major challenge, however, is the large amount of the organic resource that are usually required which has contributed to poor adoption of the use of organic resources in many areas. Unlike other areas, the EFB is a readily available organic resources in the study area (considered as waste) known for large oil palm plantations (Rhebergen et al., 2020), however, transporting it to the fields where they are needed and the extra labor required for its application will come at an extra cost. As the demand for the EFB which hitherto is considered as a waste increases, the likelihood of it attracting a fee is inevitable. Hence, an economic analysis is required to determine its economic feasibility. Farmers will also require training from extension services on the proper handling and use of EFB.
This simulation study highlights the benefits of complementing the use of inorganic fertilizer with locally available organic resources. Variability in seasonal rainfall significantly affected maize grain yields particularly during the minor rainfall seasons. As a result, the correlations between grain yield and in-season rainfall were higher in the minor season and the strength of the correlation increased with delayed planting. Other factors were, however, more dominant in explaining the variation in grain yield during the major season. The results suggests that the combined use of locally available organic resources such as EFB with inorganic fertilizer has the potential to produce comparable maize grain yield as using only inorganic fertilizer. Additionally, this approach provides greater yield stability under variable climate. Integrating the use of locally available organic resources with inorganic fertilizer, therefore provides an option for farmers to increase yield and minimize yield uncertainties caused by variations in rainfall distribution. This approach also promotes sustainable agriculture by reducing the reliance on large amounts of inorganic fertilizers. For this study to be disseminated to farmers for adoption, an analysis of the economic feasibility of using the EFB and reduced inorganic fertilizer for maize is required. Furthermore, there is an urgent need for a long-term study that considers the buildup of soil organic carbon to fully understand its long-term potential in improving soil fertility, and moisture retention, as well as crop productivity under variable climate conditions.
The raw data supporting the conclusions of this article will be made available by the authors, without undue reservation.
DM, NA, GO-B, and AF: conceptualization, project administration, supervision, and funding acquisition. DM and BF: formal analysis and writing—original draft. DM, BF, and BF-M: methodology. DM, BF, and GO-B: data curation. DM, BF, and BF-M: validation. DM, BF, BF-M, NA, GO-B, and AF: writing—review, and editing. All authors contributed to the article and approved the submitted version.
Authors are grateful to the Swiss National Science Foundation (SNSF) and the Swiss Agency for Development and Cooperation (SDC) in the Swiss Program for Research on Global Issues for Development (r4d program), the Research Institute of Organic Agriculture (FiBL), for providing the financial support through farmer-driven organic resource management to build soil fertility (ORM4Soil Project Nr. 177582) project.
The authors declare that the research was conducted in the absence of any commercial or financial relationships that could be construed as a potential conflict of interest.
All claims expressed in this article are solely those of the authors and do not necessarily represent those of their affiliated organizations, or those of the publisher, the editors and the reviewers. Any product that may be evaluated in this article, or claim that may be made by its manufacturer, is not guaranteed or endorsed by the publisher.
Abu Bakar, R., Darus, S. Z., Kulaseharan, S., and Jamaluddin, N. (2011). Effects of ten year application of empty fruit bunches in an oil palm plantation on soil chemical properties. Nutr. Cycl. Agroecosyst. 89, 341–349. doi: 10.1007/s10705-010-9398-9
Adekayode, F., and Olojugba, M. (2010). The utilization of wood ash as manure to reduce the use of mineral fertilizer for improved performance of maize (Zea mays L.) as measured in the chlorophyll content and grain yield. J. soil science Environ. Manag. 1, 40–45.
Adetunji, I. (1997). Effect of time interval between pod set and harvesting on the maturity and seed quality of fluted pumpkin. Exp. Agric. 33, 449–457. doi: 10.1017/S0014479797004092
Adiku, S., Dayananda, P., Rose, C., and Dowuona, G. (1997). An analysis of the within-season rainfall characteristics and simulation of the daily rainfall in two savanna zones in Ghana. Agric. For. Meteorol. 86, 51–62. doi: 10.1016/S0168-1923(96)02414-8
Adjei-Nsiah, S. (2012). Evaluating sustainable cropping sequences with cassava and three grain legume crops: effects on soil fertility and maize yields in the semi-deciduous forest zone of Ghana. J. Soil Sci. Environ. Manag 3, 49–55.
Affholder, F., Poeydebat, C., Corbeels, M., Scopel, E., and Tittonell, P. (2013). The yield gap of major food crops in family agriculture in the tropics: assessment and analysis through field surveys and modelling. Field Crop Res. 143, 106–118. doi: 10.1016/j.fcr.2012.10.021
Ahn, P. M. (1961). Soils of the lower Tano basin. South western region of Ghana. Soil Land Use Surv. Div., Kumasi, Ghana, Mem. 2:266.
Anisuzzaman, M., Rafii, M. Y., Jaafar, N. M., Izan Ramlee, S., Ikbal, M. F., and Haque, M. A. (2021). Effect of organic and inorganic fertilizer on the growth and yield components of traditional and improved Rice (Oryza sativa L.) genotypes in Malaysia. Agronomy 11:1830. doi: 10.3390/agronomy11091830
Ayeni, L., Adetunji, M., Ojeniyi, S., Ewulo, B., and Adeyemo, A. (2008a). Comparative and cumulative effect of cocoa pod husk ash and poultry manure on soil and maize nutrient contents and yield. A-Eurasian J. Sustainable Agric. 2, 92–97.
Ayeni, L., Ayeni, O., Oso, O., and Ojeniyi, S. (2008b). Effect of sawdust and wood ash applications in improving soil chemical properties and growth of cocoa (Theobroma cacao) seedlings in the nurseries. Medwel Agric. J. 3, 323–326.
Badu-Apraku, B., and Fakorede, M. A. B. (2017). “Maize in sub-Saharan Africa: importance and production constraints,” in Advances in Genetic Enhancement of Early and Extra-Early Maize for Sub-Saharan Africa. eds. B. Badu-Apraku and M. A. B. Fakorede (Springer International Publishing: Basel, Switzerland), 3–10.
Baffour-Ata, F., Antwi-Agyei, P., Nkiaka, E., Dougill, A. J., Anning, A. K., and Kwakye, S. O. (2021). Effect of climate variability on yields of selected staple food crops in northern Ghana. J. Agriculture Food Res. Int. 6:100205. doi: 10.1016/j.jafr.2021.100205
Bationo, A., Fening, J.O., and Kwaw, A. (2018). "Assessment of soil fertility status and integrated soil fertility management in Ghana," in Improving the profitability, sustainability and efficiency of nutrients through site specific fertilizer recommendations in West Africa agro-ecosystems, eds. A. Bationo, D. Ngaradoum, S. Youl, F. Lompo, and J.O. Fening. Springer, Netherlands. 93–138.
Bationo, A., Waswa, B., Kihara, J., and Kimetu, J. (2007). Advances in integrated soil fertility management in sub Saharan Africa: challenges and opportunities. Nutr. Cycl. Agroecosyst. 79, 1–2. doi: 10.1007/s10705-007-9096-4
Begho, T., Glenk, K., Anik, A. R., and Eory, V. (2022). A systematic review of factors that influence farmers' adoption of sustainable crop farming practices: lessons for sustainable nitrogen management in South Asia. J. Sustainable Agric. Environ. Behav. 1, 149–160. doi: 10.1002/sae2.12016
Blake, G. R., and Hartge, K. (1986). “Particle density,” in Methods of soil analysis: Part 1 physical and mineralogical methods. ed. A. Klute, (John Wiley & Sons), 377–382.
Cudjoe, G. P., Antwi-Agyei, P., and Gyampoh, B. A. (2021). The effect of climate variability on maize production in the Ejura-sekyedumase municipality. Ghana. Climate 9:145. doi: 10.3390/cli9100145
Day, P. R. (1965). "Particle fractionation and particle-size analysis," in Methods of soil analysis: Part 1 physical mineralogical properties, including statistics of measurement sampling. ed. C. A. Black. (Wisconsin, USA: American Society of Agronomy, Inc., Publisher Madison), 545–567.
Fischer, R. (1985). Number of kernels in wheat crops and the influence of solar radiation and temperature. J. Agricultural Sci. Cambridge. 105, 447–461. doi: 10.1017/S0021859600056495
Holzworth, D. P., Huth, N. I., deVoil, P. G., Zurcher, E. J., Herrmann, N. I., McLean, G., et al. (2014). APSIM–evolution towards a new generation of agricultural systems simulation. Environ. Model. Software 62, 327–350. doi: 10.1016/j.envsoft.2014.07.009
Hue, N. (1992). Correcting soil acidity of a highly weathered Ultisol with chicken manure and sewage sludge. Commun. soil sci. plant analysis 23, 241–264. doi: 10.1080/00103629209368586
Jackson-Blake, L. A., Clayer, F., de Eyto, E., French, A. S., Frías, M. D., Mercado-Bettín, D., et al. (2022). Opportunities for seasonal forecasting to support water management outside the tropics. Hydrol. Earth Sys. Sci. 26, 1389–1406. doi: 10.5194/hess-26-1389-2022
Kavitha, B., Jothimani, P., and Rajannan, G. (2013). Empty fruit bunch-a potential organic manure for agriculture. Int. J. Sci, Environ. Technol. Health Care 2, 930–937.
Keating, B. A., Carberry, P. S., Hammer, G. L., Probert, M. E., Robertson, M. J., Holzworth, D., et al. (2003). An overview of APSIM, a model designed for farming systems simulation. Eur. J. Agron. 18, 267–288. doi: 10.1016/S1161-0301(02)00108-9
Khalid, H., Zakaria, Z. Z., and Anderson, J. (2000). Nutrient cycling in an oil palm plantation: the effects of residue management practices during replanting on dry matter and nutrient uptake of young palms. J. Oil Palm Res. 12, 29–37.
Kiboi, M. N., Ngetich, K., Fliessbach, A., Muriuki, A., and Mugendi, D. N. (2019). Soil fertility inputs and tillage influence on maize crop performance and soil water content in the central highlands of Kenya. Agric.l Water Manag. 217, 316–331. doi: 10.1016/j.agwat.2019.03.014
Lal, R. (2006). Enhancing crop yields in the developing countries through restoration of the soil organic carbon pool in agricultural lands. Land Degrad. Dev. 17, 197–209. doi: 10.1002/ldr.696
Lamers, J., Bruentrup, M., and Buerkert, A. (2015). Financial performance of fertilization strategies for sustainable soil fertility management in Sudano–Sahelian West Africa. 2: profitability of long-term capital investments in rockphosphate. Nutr. Cycl. Agroecosyst. 102, 149–165. doi: 10.1007/s10705-015-9671-z
Leitner, S., Pelster, D. E., Werner, C., Merbold, L., Baggs, E. M., Mapanda, F., et al. (2020). Closing maize yield gaps in sub-Saharan Africa will boost soil N2O emissions. Curr. Opin. Environ. Sustain. 47, 95–105. doi: 10.1016/j.cosust.2020.08.018
Lim, K., and Zaharah, A. (2000). Decomposition and N & K release by oil palm empty fruit bunches applied under mature palms. J. Oil Palm Research 12, 55–62.
MacCarthy, D. S., Adam, M., Freduah, B. S., Fosu-Mensah, B. Y., Ampim, P. A., Ly, M., et al. (2021). Climate change impact and variability on cereal productivity among smallholder farmers under future production systems in West Africa. Sustainability 13:5191. doi: 10.3390/su13095191
MacCarthy, D. S., Adiku, S. G., Freduah, B. S., Gbefo, F., and Kamara, A. Y. (2017). Using CERES-maize and ENSO as decision support tools to evaluate climate-sensitive farm management practices for maize production in the northern regions of Ghana. Front. Plant Sci. 8:31. doi: 10.3389/fpls.2017.00031
MacCarthy, D. S., Adiku, S. G., Kamara, A. Y., Freduah, B. S., and Kugbe, J. X. (2022). “The role of crop simulation modeling in managing fertilizer use in maize production systems in northern Ghana” in Enhancing agricultural research and precision Management for Subsistence Farming by integrating system models with experiments, first edition. T. J. Timlin and S. S. Anapalli (eds) (New Jersey: John Wiley & Sons, Inc), 48–68.
MacCarthy, D., Akponikpe, P., Narh, S., and Tegbe, R. (2015). Modeling the effect of seasonal climate variability on the efficiency of mineral fertilization on maize in the coastal savannah of Ghana. Nutr. Cycl. Agroecosyst. 102, 45–64. doi: 10.1007/s10705-015-9701-x
MacCarthy, D. S., Darko, E., Nartey, E. K., Adiku, S. G., and Tettey, A. (2020). Integrating biochar and inorganic fertilizer improves productivity and profitability of irrigated rice in Ghana. West Africa. Agronomy 10:904. doi: 10.3390/agronomy10060904
MacCarthy, D. S., Kihara, J., Masikati, P., and Adiku, S. G. (2018a). “Decision support tools for site-specific fertilizer recommendations and agricultural planning in selected countries in sub-Sahara Africa” in Improving the profitability, sustainability and efficiency of nutrients through site specific fertilizer recommendations in West Africa agro-ecosystems (Netherlands: Springer), 265–289.
MacCarthy, D. S., Zougmoré, R. B., Akponikpe, P. B. I., Koomson, E., Savadogo, P., and Adiku, S. G. K. (2018b). Assessment of greenhouse gas emissions from different land-use systems: A case study of CO2 in the Southern Zone of Ghana. Appl. Environ. Soil. Sci.
Mamuye, M., Nebiyu, A., Elias, E., and Berecha, G. (2021). Combined use of organic and inorganic nutrient sources improved maize productivity and soil fertility in southwestern Ethiopia. Int. J. Plant Production 15, 407–418. doi: 10.1007/s42106-021-00144-6
Naab, J., Mahama, G., Koo, J., Jones, J., and Boote, K. (2015). Nitrogen and phosphorus fertilization with crop residue retention enhances crop productivity, soil organic carbon, and total soil nitrogen concentrations in sandy-loam soils in Ghana. Nutr. Cycl. Agroecosyst. 102, 33–43. doi: 10.1007/s10705-015-9675-8
NASA Langley Research Center (2019). Available at: https://power.larc.nasa.gov/data-access-viewer/ [Accessed November 10 2019].
Ng, F. Y., Yew, F. K., Basiron, Y., and Sundram, K. (2012). A renewable future driven with Malaysian palm oil-based green technology. J. Oil Palm, Environment Health & Place 2, 1–7. doi: 10.5366/jope.2011.01
Noble, A., Zenneck, I., and Randall, P. (1996). Leaf litter ash alkalinity and neutralisation of soil acidity. Plant Soil 179, 293–302. doi: 10.1007/BF00009340
Ogunkanmi, L., MacCarthy, D. S., and Adiku, S. G. (2021). Impact of extreme temperature and soil water stress on the growth and yield of soybean (Glycine max (L.) Merrill). Agriculture 12:43. doi: 10.3390/agriculture12010043
Opoku, J., and Asante, F. (2008). “Palm oil production in Ghana.” in Final report on the status of the oil palm industry in Ghana. Accra: German Technical Co-operation (GTZ).
Osei-Amponsah, C., Visser, L., Adjei-Nsiah, S., Struik, P., Sakyi-Dawson, O., and Stomph, T. (2012). Processing practices of small-scale palm oil producers in the Kwaebibirem District, Ghana: a diagnostic study. NJAS-Wageningen J. Life Sci. 60-63, 49–56. doi: 10.1016/j.njas.2012.06.006
Probert, M., Dimes, J., Keating, B., Dalal, R., and Strong, W. (1998). APSIM's water and nitrogen modules and simulation of the dynamics of water and nitrogen in fallow systems. Agric. Syst. 56, 1–28. doi: 10.1016/S0308-521X(97)00028-0
Rhebergen, T., Zingore, S., Giller, K. E., Frimpong, C. A., Acheampong, K., Ohipeni, F. T., et al. (2020). Closing yield gaps in oil palm production systems in Ghana through best management practices. Eur. J. Agron. 115:126011. doi: 10.1016/j.eja.2020.126011
Rosenani, A., and Hoe, S. (1996). “Decomposition of oil palm empty fruit bunches in the field and mineralization of nitrogen,” in Progress in nitrogen cycling studies. eds. O. Van Cleemput, G. Hofman, and A. Vermomoesen. (Netherlands: Springer), 127–132.
Salama, H. S., Nawar, A. I., Khalil, H. E., and Shaalan, A. M. (2021). Improvement of maize productivity and N use efficiency in a no-tillage irrigated farming system: effect of cropping sequence and fertilization management. Plan. Theory 10:1459. doi: 10.3390/plants10071459
Santhi, R., and Selvakumari, G. (2000). Use of organic sources of nutrients in crop production. Theme papers on integrated nutrient management, Tamil Nadu Agricultural University Tamil Nadu Department of Agriculture. Tamil Nadu. 87–101.
Shiferaw, B., Tesfaye, K., Kassie, M., Abate, T., Prasanna, B. M., and Menkir, A. (2014). Managing vulnerability to drought and enhancing livelihood resilience in sub-Saharan Africa: technological, institutional and policy options. Weather and Climate extremes 3, 67–79. doi: 10.1016/j.wace.2014.04.004
Shindo, H., Hirahara, O., Yoshida, M., and Yamamoto, A. (2006). Effect of continuous compost application on humus composition and nitrogen fertility of soils in a field subjected to double cropping. Biol. Fertil. Soils 42, 437–442. doi: 10.1007/s00374-006-0088-3
Stewart, Z. P., Pierzynski, G. M., Middendorf, B. J., and Prasad, P. V. (2020). Approaches to improve soil fertility in sub-Saharan Africa. J. Experimental Botany 71, 632–641. doi: 10.1093/jxb/erz446
Taylor, A. L., Dessai, S., and De Bruin, W. B. (2015). Communicating uncertainty in seasonal and interannual climate forecasts in Europe. Philosophical Transactions of the Royal Society A: Mathematical, Physical Engineering Sciences 373:20140454. doi: 10.1098/rsta.2014.0454
Van Loon, M. P., Adjei-Nsiah, S., Descheemaeker, K., Akotsen-Mensah, C., van Dijk, M., Morley, T., et al. (2019). Can yield variability be explained? Integrated assessment of maize yield gaps across smallholders in Ghana. Field Crop Res. 236, 132–144. doi: 10.1016/j.fcr.2019.03.022
Vanlauwe, B., Coyne, D., Gockowski, J., Hauser, S., Huising, J., Masso, C., et al. (2014). Sustainable intensification and the African smallholder farmer. Current Opinion in Environ. Sustain. 8, 15–22. doi: 10.1016/j.cosust.2014.06.001
Walkley, A., and Black, A. (1934). Organic matter was determined by wet digestion: an examination of the Degtjareff method for determining soil organic matter, and a proposed modification of the chromic acid titration method. Soil Sci. 37, 29–38. doi: 10.1097/00010694-193401000-00003
Yang, Y., Guo, X.-X., Liu, H.-F., Liu, G.-Z., Liu, W.-M., Bo, M., et al. (2021). The effect of solar radiation change on the maize yield gap from the perspectives of dry matter accumulation and distribution. J. Integ. Agric. 20, 482–493. doi: 10.1016/S2095-3119(20)63581-X
Yang, Y., Xu, W., Hou, P., Liu, G., Liu, W., Wang, Y., et al. (2019). Improving maize grain yield by matching maize growth and solar radiation. Sci. Rep. 9, 1–11. doi: 10.1038/s41598-019-40081-z
Soil parameters used in model calibration simulations at SIREC, Kpong.
Keywords: local organic resources, yield uncertainty, integrated nutrient management, West Africa, resource use efficiency, climate variability
Citation: MacCarthy DS, Adamtey N, Freduah BS, Fosu-Mensah BY, Ofosu-Budu GK and Fliessbach A (2023) Modeling the effect of soil fertility management options on maize yield stability under variable climate in a sub-humid zone in Ghana. Front. Sustain. Food Syst. 7:1132732. doi: 10.3389/fsufs.2023.1132732
Received: 27 December 2022; Accepted: 11 August 2023;
Published: 29 August 2023.
Edited by:
Ole Mertz, University of Copenhagen, DenmarkReviewed by:
Dimitri Defrance, Independent researcher, Montpellier, FranceCopyright © 2023 MacCarthy, Adamtey, Freduah, Fosu-Mensah, Ofosu-Budu and Fliessbach. This is an open-access article distributed under the terms of the Creative Commons Attribution License (CC BY). The use, distribution or reproduction in other forums is permitted, provided the original author(s) and the copyright owner(s) are credited and that the original publication in this journal is cited, in accordance with accepted academic practice. No use, distribution or reproduction is permitted which does not comply with these terms.
*Correspondence: Dilys S. MacCarthy, ZG1hY2NhcnRoeUB1Zy5lZHUuZ2g=
Disclaimer: All claims expressed in this article are solely those of the authors and do not necessarily represent those of their affiliated organizations, or those of the publisher, the editors and the reviewers. Any product that may be evaluated in this article or claim that may be made by its manufacturer is not guaranteed or endorsed by the publisher.
Research integrity at Frontiers
Learn more about the work of our research integrity team to safeguard the quality of each article we publish.