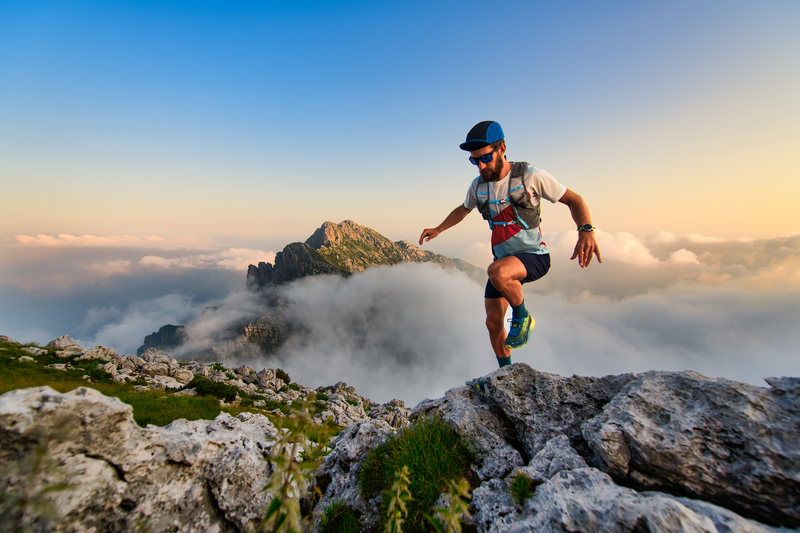
94% of researchers rate our articles as excellent or good
Learn more about the work of our research integrity team to safeguard the quality of each article we publish.
Find out more
ORIGINAL RESEARCH article
Front. Sustain. Food Syst. , 21 April 2023
Sec. Crop Biology and Sustainability
Volume 7 - 2023 | https://doi.org/10.3389/fsufs.2023.1130089
Maize is important to global food security, being one of the predominant cereals in human and domesticated livestock diets worldwide. Due to the increasing human population, it will be important to not only design cropping systems to increase maize yield and sustainability but also to improve the nutritional quality of maize edible tissues. To determine cropping system impacts on maize grain nutritional content, we sampled grain from conventional and organic maize varieties grown for three growing seasons using five cropping systems. We analyzed the grain using metabolic fingerprinting of methanol extracts with ultra-high performance liquid chromatography (UHPLC) coupled with mass spectrometry (MS), adopting both non-targeted and targeted approaches. The cropping systems are part of a long-term study at the Beltsville Agricultural Research Center in Beltsville, Maryland, and were a three-year conventional no-till rotation (NT), a three-year conventional chisel-till rotation (CT), a two-year organic rotation (Org2), a three-year organic rotation (Org3), and a six-year organic rotation (Org6). Each cropping system had been in place for at least 10 years, allowing specific cropping-system-induced alterations of soil edaphic and microbial properties. Non-targeted metabolic fingerprinting detected a total of 90 compounds, the majority of which were phenolics. Metabolic profiling was further targeted toward 15 phenolics, 1 phytohormone, 7 carbohydrates and 7 organic acids, which were quantified in the maize grain originating from the five cropping systems. Statistical analysis of this subset of quantitative data determined that cropping system can significantly influence levels of certain maize grain metabolites. However, natural impacts (growing year) were substantially greater than cropping system impacts, likely masking or over-riding some cropping system impacts. Additionally, maize cultivar genetics had greater impact than cropping system on the maize grain metabolome and was the greatest “managed” impact on the metabolite profiles. Results indicate that until natural environmental impacts on maize grain metabolite levels are understood and managed, the best approach to reliably increase maize grain nutritional quality is through development of maize cultivars with enhanced nutritional content that are robust to natural environmental influence.
Maize (Zea mays L.) is important to global food security, being one of the predominant cereals in human and domesticated animal diets worldwide. Maize grain contains energy-generating carbohydrates, lipids, and proteins as well as minerals and micronutrients such as essential fatty acids, vitamin A as provitamin A, several B complex vitamins (thiamine, niacin, riboflavin, pantothenic acid, pyridoxine, folic acid), and vitamins E and K (Nuss and Tanumihardjo, 2010; Sheng et al., 2018). These, and other, minerals and micronutrients are necessary as insufficient dietary doses can negatively impact human health, causing classical micronutrient deficiency diseases (Grusak and DellaPenna, 1999; Martin and Li, 2017). Maize grain also contains beneficial bioactive compounds such as phenolics, carotenoids, and phytosterols (Jiang and Wang, 2005; Lopez-Martinez et al., 2009; Nuss and Tanumihardjo, 2010; Sheng et al., 2018). Consumption of certain of these bioactive compounds has been correlated with decreased incidence of chronic diseases such as stroke, diabetes, heart disease, Alzheimer's, cataracts, and age-related functional decline (Grusak and DellaPenna, 1999; Liu, 2013; Martin and Li, 2017). Additionally, maize is important in production of raw materials (e.g., starch, oil) and fuel (Tanumihardjo et al., 2020).
There is increasing research on various cropping systems to produce maize and other crops in efforts to satisfy consumer demand, increase yield, and increase environmental sustainability (Roberts and Mattoo, 2018, 2019). One approach to meeting consumer demand is organic production [National Organic Program Standards; Organic Regulations|Agricultural Marketing Service (usda.gov)], which differs from conventional production in that several tools commonly used in conventional systems are prohibited, including genetically modified organisms, synthetic fertilizers, and many pesticides. Instead, organic production systems rely on leguminous cover crops to supply nitrogen, and animal manures and by-products to recycle crop nutrients, while pest management focuses on avoiding pest problems via crop rotation or using pest control chemicals that are usually expected to be less toxic than those used in conventional production. Within conventional production, cropping systems can vary by tillage intensity, varying from full inversion tillage, reduced tillage, and no-tillage. Aspirational cropping systems under development strive for high yield potential and low negative environmental impacts, drawing on the most sustainable management practices from organic and conventional crop production systems (Reganold and Wachter, 2016; Roberts and Mattoo, 2018). Going forward, it will be important to not only design cropping systems to increase maize yield and sustainability but also to improve the nutritional quality of edible tissues of maize to meet the nutritional/raw material requirements of the growing human and livestock populations (Roberts and Mattoo, 2019).
The quantity of nutrients and beneficial bioactive compounds in edible plant tissues is dependent on crop genetics but regulated by complex and overlapping mechanisms in response to developmental and environmental cues. Environmental cues are naturally occurring—temperature, light intensity, precipitation, and various stressors—or result from agricultural management practices—such as soil organic matter and nutrient levels (Zhu et al., 2007; Hirschi, 2009; Fatima et al., 2016; Vasconcelos et al., 2017; Roberts and Mattoo, 2019). Several studies have demonstrated the influence of genetics (crop varieties) and naturally occurring cues (year, drought, geographic location) on the biochemical composition of maize grain and other maize tissues (Reynolds et al., 2005; Röhlig et al., 2009; Anttonen et al., 2010; Röhlig and Engel, 2010; Frank et al., 2012; Baniasadi et al., 2014; Chen et al., 2016; Venkatesh et al., 2016; Zhang L. et al., 2020; Gaffney et al., 2021; Zhang et al., 2021). But there has been less work on maize grain biochemical composition and environmental cues resulting from agricultural management practices in replicated experiments. Nitrogen fertilizer rate and planting density (management cues) significantly impact maize protein and oil macronutrient contents (Genter et al., 1956; Jellum and Marion, 1966; Wang et al., 2008) while tillage impacts yield and macronutrient content in certain cereal crops (Malhi and Nyborg, 1990; Malhi et al., 2001; Gan et al., 2003; Wang et al., 2008). In studies with non-cereal crops, nitrogen (source, quantity), and certain environmental conditions influenced by cropping system, impacted micronutrients and beneficial bioactive compounds (Poiroux-Gonord et al., 2010; Fatima et al., 2016). There is also limited literature on the impact of biological components (cover crops, beneficial microbes) used in sustainable cropping systems on crop physiology or micronutrient quality (Mattoo and Teasdale, 2010; Roberts and Mattoo, 2019), but impacts can be substantial (Neelam et al., 2008; Mattoo and Teasdale, 2010; Fatima et al., 2012, 2016; Roberts and Mattoo, 2019). Clearly there is a need for further study to determine the importance of naturally occurring and cropping system impacts on the nutritional status of maize grain as information to date is piecemeal, being gathered from studies on a variety of crops and cultivars. Knowledge concerning these impacts may allow optimization of cropping systems to maximize maize nutritional content.
We report here impacts of naturally occurring cues and management cues on metabolite (nutrient) levels in the metabolome of maize grain grown under five cropping systems at the Farming Systems Project (FSP) in Beltsville, MD in three consecutive years (Table 1). The five cropping systems were a three-year conventional no-till rotation (NT), a three-year conventional chisel-till rotation (CT), a two-year organic rotation (Org2), a three-year organic rotation (Org3), and a six-year organic rotation (Org6) (Cavigelli et al., 2008; Spargo et al., 2011). These cropping systems, all of which include maize, represent a wide range of management practices used in the mid-Atlantic region of the US. They differ regarding use of legume cover crops and forage, tillage intensity, and fertility management, including materials used for nitrogen fertility. The five cropping systems have resulted in differences in crop yield and factors associated with soil health such as fertility, aggregate stability, organic carbon and nitrogen pools, and microbiome composition (Green et al., 2005; Cavigelli et al., 2008; Spargo et al., 2011; Teasdale and Cavigelli, 2017; Treonis et al., 2018; Maul et al., 2019; Kepler et al., 2020; White et al., 2021; Schmidt et al., 2022).
Table 1. Cropping systems and crop rotations (including cover crops) at the USDA-ARS Farming Systems Project (FSP).
Since soil fertility and soil health impact crop nutrient content, we hypothesized that cropping systems, which have been shown to affect soil properties, would also impact maize grain metabolite content. To test this hypothesis, we compared first order moments (means) of individual metabolites in the metabolome of grain from conventional and organic maize varieties grown under these five FSP cropping systems. We also compared second order moments (correlations) between metabolite pairs among cropping systems. Correlations, representing labile links among metabolite pathways, may constrain how metabolic responses can occur in plants grown under different cropping systems in field settings (Fukushima et al., 2011; Fatima et al., 2016).
The field site was the long-term FSP at the Beltsville Agricultural Research Center, Beltsville, MD, USA (39.0° N, 76.9° W). The climate is humid subtropical, with average annual precipitation of ~1,110 mm, and average temperature of 12.8°C. Dominant soil types include Christiana (fine, kaolinitic, mesic Typic Paleudults), Keyport (fine, mixed, semiactive, mesic Aquic Hapludults), Matapeake (fine-silty, mixed, semiactive, mesic Typic Hapludults), and Mattapex (fine-silty, mixed, active, mesic Aquic Hapludults) silt loams (Spargo et al., 2011). All soils are designated as Ultisols with variable amounts of clay in illuvial horizons, and variable average water table depths (Schmidt et al., 2022).
The FSP plots were established in 1996 in a randomized complete block split plot design with cropping system as the main plot, crop as the split, and with four replicates. Two of the cropping systems are managed using conventional and three using organic management practices (Cavigelli et al., 2008; Teasdale and Cavigelli, 2017; Table 1). All crops in a given rotation are present every year such that maize, for example, is present in each system each year. Management details are as previously reported (Cavigelli et al., 2008; White et al., 2019). Briefly, nutrients harvested in grain, straw, and forages were replaced with potassium fertilizer as K2SO4 in all systems, synthetic nitrogen (N; usually urea ammonium nitrate) and triple super phosphate fertilizers in the conventional systems (CT and NT), and poultry litter in the organic systems, with rates based on soil test results from the previous fall and Maryland Agricultural Nutrient Management Program recommendations (https://extension.umd.edu/anmp). Weed management practices included crop rotation in all systems, herbicides in the conventional systems, pre-plant tillage in CT and organic systems, and post-planting tillage (rotary hoeing and between row cultivation) in the organic systems.
Cropping systems were managed to mimic regional farmer practices so that research results are applicable to the mid-Atlantic region of the United States. Since most conventional farmers use genetically modified crop varieties, which are prohibited in certified organic systems, different crop varieties are used in the two conventional vs. the three organic systems. In 2011 and 2012 maize variety TA 65713 VP was used in conventional systems and in 2013 TA 647-22 DP was used. In organic systems, Blue River variety 53R57 was used in 2011 and 2012 while Blue River variety 51B57 was used in 2013. To assess whether differences in maize grain yield and nutrient content between conventional and organic systems (Cavigelli et al., 2008; Teasdale and Cavigelli, 2017) might be partly attributable to inherent differences between conventional and organic varieties, microplots were established in the 9.1 × 111 m main plots beginning in 2011 in which the conventional maize variety was grown in 3.0 × 27.7 m areas within the main organic plots that were otherwise planted to the organic variety. In 2012 and 2013, the same types of microplots were established in all maize plots, with the organic maize variety grown in microplots in the conventional systems and, as in 2011, the conventional maize variety grown in microplots in the organic systems. In 2013, the size of the microplots was reduced to 3.0 m x 18.5 m. Maize grain from microplots and adjacent, similarly sized areas of main plots was harvested using a small plot combine that harvested the two middle rows (Almaco Co., Nevada, IA). Subsamples were collected, dried, and stored in glass jars in an archive, which was housed in a climate-controlled building.
Soil samples were collected every fall or winter from the 0–15 or 0–20 cm depth increment. Eight cores (19 mm diameter) were taken per plot and combined to form one sample. Fresh soils were analyzed by A&L Great Lakes Labs in Fort Wayne, Indiana. Soil organic matter was analyzed by loss on ignition, pH using a glass electrode in a slurry formed from a 10 mL: 8.5 cm3 ratio of water:soil; and soil test P and K were extracted using the Mehlich 3 method and analyzed using ICP.
Maize grain samples of conventional and organic varieties grown side-by-side within each of the five FSP cropping systems were sub-sampled from the archive for this study. Thus, 40 samples (5 cropping systems x 2 varieties x 4 field replicates) from each of 2012 and 2013 were finely ground using a coffee grinder that was cleaned between samples. In 2011, 32 samples (3 organic cropping systems x 2 varieties x 4 field replicates + 2 conventional cropping systems (only one variety) x 4 field replicates) were processed in similar fashion.
Extraction of phenolics and sugars from finely ground maize grain samples was performed with 96% methanol (w:v = 1:10) and involved vortexing for 5 min followed by sonication in an ultrasonic bath for 10 min. After centrifugation for 10 min at 8,000 × g, supernatants were filtered through 0.2 μm cellulose filters (Agilent Technologies, Santa Clara, CA) and stored at 4°C until used (Banjanac et al., 2017). Extraction of organic acids was performed in 5% solution of phosphoric acid in water (w:v = 1:10), according to procedure previously published by Mišić et al. (2012), with some modification. Samples were vigorously mixed, vortexed for 10 s, sonicated in an ultrasonic bath for 10 min, and incubated overnight at 4°C. The following day, samples were vortexed for 10 s, centrifuged at 8,000 × g for 10 min at room temperature, the supernatants filtered through a 0.2 μm Econofilter (Agilent Technologies), and kept at 4°C until used. All extractions were performed in triplicate.
Chromatographic separations were performed using an Accela ultra-high performance liquid chromatography (UHPLC) system (ThermoFisher Scientific, Bremen, Germany) fitted with a Syncronis C18 100 × 2.1 mm analytical column with 1.7 μm particle size (ThermoFisher Scientific). The mobile phase consisted of (A) ultrapure water with 0.1% formic acid and (B) acetonitrile (MS grade) + 0.1% formic acid. The elution gradient program was as follows: 0.0 – 1.0 min, 5% B; 1.0 – 16.0 min, 5 – 95% B; 16.0 – 16.2 min, 95 – 5% B; 16.2 – 20.0 min, 5% B. The injection volume for all samples was 25 μL, and the flow rate was 0.3 mL/min.
The UHPLC system was coupled to a LTQ OrbiTrap mass spectrometer (ThermoFisher Scientific) equipped with a heated-electrospray ionization probe (HESI-II) operated in negative ionization mode. HESI-source parameters were the same as previously described (Banjanac et al., 2017). Mass spectra were acquired by full range acquisition covering 100–1,500 m/z. For the fragmentation study, a data-dependent scan was performed by deploying collision-induced dissociation (CID). The normalized collision energy of the CID cell was set to 35 eV.
Metabolites were identified and some of them quantified according to the corresponding spectral characteristics: mass spectra, accurate mass, characteristic fragmentation, and characteristic retention time. ThermoFisher Scientific Xcalibur software (version 2.1) was employed for instrument control, data acquisition, and data analysis. Fragmentation mechanism and characteristic MS2 and MS3 fragments were confirmed using ThermoFisher Scientific Mass Frontier software (version 6.0).
Separation of organic acids was performed on a Waters HPLC system (Waters Corp., Milford, MA, USA) consisting of 1525 binary pumps, thermostat, and 717+ autosampler connected to the Waters 2996 diode array detector (DAD) adjusted at 210 nm. The HPLC system was fitted with a Supelco C-610H (300 x 7.8 mm) anion exchange column (Sigma-Aldrich Co., Barcelona, Spain) connected to the appropriate guard column. Isocratic elution was carried out with 0.1% H3PO4 as the mobile phase at a flow rate of 0.5 ml/min and a holding column temperature of 40°C. Quantification of organic acids was based on developing calibration curves of pure compounds. Values are expressed as μg per g of dry weight (μg g−1 DW). Standards of tartaric, citric, isocitric, fumaric, malic, formic, and oxalic acids were purchased from Sigma Aldrich Co. (Steinheim, Germany).
Separation of sugars was performed on a Waters Breeze chromatographic system (Waters Corp.) connected to a Waters 2465 electrochemical detector (pulsed amperometric detection, PAD) with a 3 mm gold working electrode and hydrogen reference electrode. This HPLC system was fitted with a CarboPac PA1 (Dionex Corp., Sunnyvale, CA) 250 × 4 mm column equipped with the corresponding CarboPac PA1 guard column, held at 30°C, and used a flow rate of 1.0 ml/min. Sugars were eluted using 0.2 M sodium hydroxide (50% w/w, low carbonate; J.T. Baker Co., Deventer, Holland). Signals were detected in pulse mode using the following waveform: E1 = +0.15 V for 400 ms; E2 = +0.75 V for 200 ms; E3 = −0.80 V for 300 ms and within 150 ms of integration time. Filter timescale was 0.2 s and range set at 500 nA for the full mV scale. For both sugars and organic acids, data acquisition and spectral evaluation for peak confirmation were carried out using Waters Empower 2 Software (Waters Corp.). Quantification was performed using calibration curves of pure compounds. Standards of sucrose, fructose, glucose, sorbitol, and mannitol were purchased from Sigma Aldrich (Steinheim, Germany).
Metabolite variables were transformed as needed prior to statistical analyses due to skewed distributions. Sugars (sorbitol, mannitol, glucose, fructose, sucrose, monosaccharide, and disaccharide) were log transformed, as were gallic acid, p-hydroxyphenylacetic, and cis, trans abscisic acid. Organic acids (citric, tartaric, malic, isocitric, formic, and fumaric acids) were log (x + 1) transformed. Remaining metabolite variables were not transformed.
Linear mixed models, using lme4 (Bates et al., 2015), lmerTest (Kuznetsova et al., 2017), emmeans (Lenth, 2022), and pbkrtest (Halekoh and Højsgaard, 2014) packages in R (R Core Team, 2018), were used to determine the impact of fixed (cropping system, maize variety, soil properties, and their interactions) and random (year, interactions of year with fixed effects) effects on metabolite mean values (first moment effects), and for a stepwise variable selection on the full model (for both fixed and random effects). p-values on fixed effects following variable selection were adjusted using FDR (false discovery rate) since this is a repeated measures analysis. Variance decomposition was performed for each metabolite using the lme4 package to determine what percent of the variance could be attributed to each fixed or random effect. For variance decomposition, quantitative variables (e.g., soil pH) were transformed into qualitative ones by creating five bins capturing the range of values of that variable, with approximately equal numbers of observations in each bin (thus bin width was not fixed). The psych package in R (Revelle, 2018) was used to test if the correlation between metabolites significantly changed (second moment effects) among levels of some effects with a cutoff of α = 0.01 for significance. Due to sample size limitations, when testing for changes in correlation for levels in one effect, other effects were ignored.
Naturally occurring impacts on maize yield were clearly greater than impacts associated with cropping system as most of the variability (85.5%) in maize grain yield was attributable to the year random effect (Table 2). This is consistent with an earlier study where year effects were greatest and due mostly to amount of rainfall during critical periods (especially 9 to 13 weeks after planting for conventional maize and 6 to 11 weeks after planting for organic maize) (Teasdale and Cavigelli, 2017). The years 2011 and 2012 (Table 3) were relatively dry years (14.6–15.8 mm wk−1 during critical time periods) while 2013 had adequate rainfall for crop growth (20.3–23.4 mm wk−1 during critical time periods). Maize grain yields reflected these weather patterns during these years (Table 3).
Table 3. Stepwise selected model mean and standard error (SE) estimates of maize grain yields in 2011, 2012, and 2013 in FSP microplots (see text); multiple comparison letters (α = 0.01) following the SE apply across all 3 years. Data were not taken for some microplots in 2013 (NA).
Maize genetics also played a role in yield as maize grain yields in general were greater for the conventional than the organic variety regardless of year or cropping system (Table 3). The one exception was in year 2013 when yields were similar for the conventional and organic varieties when grown in the organic cropping system plots. Differences between conventional and organic varieties in the conventional cropping systems likely reflect differences in yield potential of the varieties while differences in yield between varieties within organic cropping systems likely reflect differences in yield potential and impacts of weed competition. Earlier research showed that impacts of weed competition on maize yields at FSP were greater in dry than wet years (Cavigelli et al., 2008; Teasdale and Cavigelli, 2010).
Soil test results (Table 4) show that soil organic matter was greater in organic than conventional plots in all years except for Org2 in 2011. There were few substantial differences in pH among systems. Soil test P was similar in the plots sampled in 2011 but substantially greater in organic than conventional systems in 2012 and 2013. Soil test K was always greater in organic than conventional systems. These differences are consistent with published reports showing that organic systems tend to have greater soil fertility, including soil organic matter, than conventional systems in FSP plots (Spargo et al., 2011; White et al., 2021; Boniface et al., 2022; Schmidt et al., 2022).
Table 4. Stepwise selected model mean and (SE) estimates of soil fertility parameters for FSP cropping systems collected to evaluate soil fertility requirements for maize in 2011, 2012, and 2013; multiple comparison letters (α = 0.01) following the SE are relevant only within a given column.
Metabolic fingerprinting of methanol extracts of maize grain using UHPLC coupled with mass spectrometry (MS) detection, which combines high-resolution mass spectrometry (HRMS) and multistage mass spectrometry (MS2 and MS3 fragmentation), identified a total of 90 compounds (Supplementary Table 1). The identity of 16 compounds (compounds 1, 5, 9, 12, 14, 21, 25, 26, 32, 66–68, 76, 87, 89, 90) were confirmed by direct comparison with appropriate standards, while the identity of the remaining 74 compounds were obtained after examination of MS data (exact mass and specific fragmentation), in consultation with the available literature on LC/MS and nuclear magnetic resonance (NMR) analysis of maize metabolites (Li et al., 2018; Zhou et al., 2019; Lux et al., 2020; Eleazu et al., 2021). Basic retention and MS data, as well as references confirming the presence and, in some cases, mass fragmentation of specific compounds in maize are listed in Supplementary Table 1. The structures of some compounds (compounds 10, 11, 15, 22, 27, 28, 33, 34, 38, 45, 70, 71) were proposed based only on MS data, because a search of available databases did not provide confirming information. These newly identified compounds are clearly labeled in Supplementary Table 1, as is the availability of very similar derivatives in the maize literature, which helped with their identification. Several compounds were identified in maize for the first time but previously detected in other species of the Poales order. These are compounds 29 (Lee et al., 2010), 42–44 (Shirota et al., 1996), and 72 and 73 (Kang et al., 2018). The chromatograms of all maize samples showed similar metabolic profiles. As an example, the base peak chromatogram of maize grain extract (Organic variety, Org6 cropping system) is shown in Supplementary Figure 1. The peak numbers in Supplementary Figure 1 correspond to the compound numbers in Supplementary Table 1. For easier understanding of Supplementary Table 1, the compounds were divided into subgroups based on structure: benzoic acid derivatives (12 compounds), cinnamic acid derivatives (33 compounds), hydroxycinnamic acid amides (20 compounds), flavonoids (3 compounds), lignans (5 compounds), plant hormones (11 compounds), and “other” compounds that didn't fit into this grouping (6 compounds).
Benzoic acid is the simplest aromatic carboxylic acid, with a carboxylic group bonded to the benzene ring. Benzoic acid derivatives were represented in the maize samples by simple acids (compounds 1, 5, 9, 12) and various glycosides (Supplementary Table 1). Mass spectra of all glycoside derivatives showed a similar fragmentation pathway characterized by loss of hexoside (162 Da), dihexoside (324 Da) or pentosyl hexoside (294 Da).
Cinnamic acid derivatives are polyphenolic compounds originating from the mavolanate-shikimate pathway that include simple phenolic compounds such as cinnamic acid, p-coumaric acid, ferulic acid, and caffeic acid. Monomers, dimers, and rarely trimers of hydroxycinnamic acids were detected in maize samples as sucrose acetyl esters (15 compounds) or bound to glycerol (5 compounds) (Supplementary Table 1). Consistent with the literature, there were numerous ferulic acid derivatives (dehydrodimers, glycosides, acyl glycosides, and soluble and insoluble amide derivatives) with a significant number of compounds containing two ferulic acids or one ferulic and one caffeic acid, sucrose, and several acetyl groups (Bento-Silva et al., 2018, 2020; Zhou et al., 2019). For example, compound 28, found at 763 m/z, showed a MS2 base peak at 721 m/z (loss of one acetyl group – 42 Da) and MS3 base peak at 545 m/z (further loss of feruloyl unit – 176 Da). All other MS2 and MS3 secondary peaks are depicted in Supplementary Figure 2. Based on all these findings, this compound was identified as feruloyl-caffeoyl-sucrose di-acetyl ester. The exact position of the two acetyl groups could not be determined. Supplementary Figure 2 presents the tentative structure and proposed fragmentation pathway of this compound.
Hydroxycinnamic acid amides are a diverse class of secondary metabolites that arise from condensation reactions between CoA esters of hydroxycinnamic acids with aliphatic/di/poly- or aromatic mono-amine groups. The combination of 96% methanol and ultrasound during extraction resulted in identification of 20 hydroxycinnamic acid amides in maize grain samples (Supplementary Table 1). Hydroxycinnamic acid amides, commonly present in maize, were detected as derivatives of putrescine (compounds 46, 55, 57, and 57–59), spermidine (compounds 47–54 and 61–63), and tryptamine (compounds 56, 64, 65) (Li et al., 2018; Bartolić et al., 2020; Bento-Silva et al., 2020), and one compound (60) was detected as a tyramine derivative (Marti et al., 2013). Only one glycosidic derivative (compound 47; 598 m/z) was detected, and the molecular formula, C31H41N3O9, was calculated by examining its exact mass. MS2 and MS3 spectra confirmed that this compound can be identified as a dicoumaroyl spermidine hexoside (Supplementary Figure 3), which was previously detected in maize seeds (Li et al., 2018).
Many different hydroxycinnamic acid amides consisting of 2 hydroxycinnamic acids linked through their carboxylic acid moiety by a chain of putrescine or spermidine, were also detected in the maize grain samples (Supplementary Table 1). Some of the feruloyl putrescines previously reported for maize are coumaroyl-feruloyl putrescine, Di feruloyl putrescine (Sen et al., 1994; Acosta-Estrada et al., 2015), dicoumaroyl putrescine, feruloyl putrescine, and p-coumaroyl putrescine (Sen et al., 1994). Most of these compounds were also present in maize grain samples analyzed in the present study. According to previous studies, the majority of the hydroxycinnamic acid amides are in the outer tissues of maize grain (Burt et al., 2019) and can be involved in defense against postharvest insect pests (García-Lara et al., 2010; García-Lara and Bergvinson, 2014) and serve as co-pigments in colored maize (Collison et al., 2015). This group of compounds was not quantified due to the lack of available standards.
Flavonoids are synthesized through the phenylpropanoid pathway, transforming phenylalanine into 4-coumaryoyl-CoA, which is transformed via chalcone synthase, the first enzyme in the flavonoid biosynthesis pathway, into chalcone scaffolds from which all flavonoids are derived. All three flavonoids detected, naringenin, apigenin, and hesperetin (compounds 66–68), were previously detected in maize, and their identity confirmed using appropriate standards (Supplementary Table 1).
Lignans share common biosynthetic pathways and consist of two propyl-benzene units coupled by a ß, ß'-bond. Of the 5 lignans identified in the methanol extracts of maize grain (Supplementary Table 1), none were previously detected in maize. Compound 69 (syringaresinol 4-O-hexoside) was not common in the Poales order, but its structure was confirmed in the literature (Li et al., 2018). Oryzativol A and B (compounds 72, 73), specific for rice (Kang et al., 2018), as well as two derivatives of oryzativol without the cinnamic acid residue (compounds 70 and 71) were detected in methanol extracts of the maize samples.
A total of 11 phytohormone compounds were identified in methanol extracts of maize grain (Supplementary Table 1). Zeanosides A and C (compounds 74, 75), named after maize, are indole-3-acetic acid (IAA)-related compounds (Tateishi et al., 1987). Three gibberellins (compounds 78, 79, 81) were detected: gibberellin A53, A12, and A53-aldehyde (Kobayashi et al., 1996). The stress hormone abscisic acid (compound 76) was also detected in maize grain.
Six compounds did not fit into the above grouping. Of these “other” compounds, the most interesting was DIMBOA-Glc (compound 85; Supplementary Table 1). DIMBOA-Glc is a glucoside derivative of DIMBOA, a powerful antibiotic naturally occurring in maize (Sauter et al., 2002).
Data presented here are consistent with previous studies that revealed that grain of various maize varieties contain several phenolic compounds, including phenolic acids (e.g., vanillic, p-hydroxybenzoic, caffeic, p-coumaric, ferulic, syringic, synaptic, protocatechuic) and flavonoids (Kandil et al., 2012; Guo and Beta, 2013; Das and Singh, 2015, 2016; Thakur et al., 2017) with ferulic acid and p-coumaric acid present in high concentrations (Mathew and Abraham, 2004; Vitaglione et al., 2008; Santiago and Malvar, 2010). Ferulic acid, p-coumaric acid, and vanillin were the predominant phenolic compounds in analyzed maize grain samples, in both conventional and organic varieties. Amounts of ferulic acid ranged between 0.39 and 3.68 mg kg−1 DW, while p-coumaric acid was present in amounts ranging between 0.99 and 7.62 mg kg−1 DW. Vanillin (0.41–3.64 mg kg−1 DW), vanillic acid (0.45–2.30 mg kg−1 DW), and p-hydroxybenzoic acid (0.24–1.37 mg kg−1 DW) were also abundant. All other phenolic compounds were present in significantly lower amounts. Among the flavonoids, the flavanones naringenin and hesperetin were detected in amounts up to 0.73 and 0.41 mg kg−1 DW, respectively. The flavone apigenin was detected at ≤ 0.30 mg kg−1 DW. Supplementary Table 2 contains concentrations of phenolics, sugars and organic acids by replicate, variety, cropping system, and year in maize grain for which quantitative data could be obtained.
Soluble sugars identified in maize grain samples (Supplementary Table 2) were the disaccharide sucrose, the monosaccharides glucose and fructose, and the sugar alcohols mannitol and sorbitol. Two more compounds, one monosaccharide and one disaccharide, were visible in HPLC-PAD chromatograms (Supplementary Figure 4); however, due to the lack of appropriate standards they were not unambiguously identified. The amounts of these unidentified saccharides in samples were calculated relatively, based on calibration curves for glucose and sucrose. The amount of sucrose in samples varied between 5.50 and 52.50 g kg−1 DW, depending on the maize variety, cropping system, and year. The second most abundant soluble sugar in maize grain was fructose with concentrations ranging between 0.04 and 2.00 g kg−1 DW, while glucose was detected between 0.04 and 1.41 g kg−1 DW. The sugar alcohols mannitol and sorbitol were detected at 0.31 and 0.55 mg kg−1 DW, respectively.
Organic acids identified and quantified in maize grain samples were oxalic, citric, tartaric, malic, iso-citric, formic, and fumaric acids (Supplementary Table 3). A representative HPLC-DAD chromatogram is shown in Supplementary Figure 5. The most abundant organic acid was tartaric acid, detected at 2.19 g kg−1 DW, followed by formic acid, detected at ≤ 1.68 g kg−1 DW, oxalic acid detected at ≤ 1.08 g kg−1 DW, and iso-citric acid detected at ≤ 1.01 g kg−1 DW. Malic, citric, and fumaric acids were present in significantly lower concentrations.
Quantitative data for 30 compounds detected in maize grain for which standards were available (15 phenolics, 1 phytohormone, 7 sugars, and 7 organic acids) were statistically analyzed. Variance decomposition showed that fixed effects (cropping system, maize variety, soil properties, and their interactions) explained little of the total variance in measured metabolites except for maize variety (Table 2). Importantly, cropping system was associated with very little of the variation in mean levels of any metabolites. Cropping system explained <7% of the variation among all 30 metabolites and 2% or less of the variation with 25 of the metabolites. Available soil fertility variables were not predictive (results not presented). Maize variety explained most of the variability associated for fixed effects with almost all metabolites. With certain metabolites (tartaric, isocitric, fumaric, formic, malic, unknown disaccharide, ferulic, caffeic) maize variety explained a sizable effect (>20%) of variation. In contrast to fixed effects, random effects, or naturally occurring impacts (year, interactions of year with fixed effects), explained a large percentage of the explainable variance. Of the 30 metabolites, 16 (including all sugars) had a sizable year effect (> 20% of the total variance). However, for most metabolites, the largest proportion of the total variance was unexplained (i.e., residual error), indicating that either there are other important and unmeasured factors influencing metabolite levels or that there was considerable biological variability in the system such as soil moisture and soil fertility factors that can vary substantially within fields.
In tests of significance, cropping system affected 9 of 30 metabolites and maize variety affected 11 of 30 metabolites (Table 2). Only one metabolite, unidentified disaccharide, was significantly affected by the cropping system × maize variety interaction. Tests of significance were also performed on soil fertility variables as cropping systems can affect soils. Soil organic matter impacted no metabolite while soil pH, P, and K impacted 4 to 6 metabolites (mannitol [K, pH], sucrose [pH], p-hydroxybenzoic [P], caffeic [P], vanillic [K, P], vanillin [P], ferulic [K, P], coniferyl aldehyde [P], oxalic [pH], citric [pH], malic [K], iso-citric [pH]; data not shown). Examining these results by metabolite, we found that two metabolites were affected by three fixed effect factors, eight metabolites were affected by two fixed effect factors, 11 by one fixed effect factor, and nine were unaffected by any of the fixed effect factors (Table 2). Thus, levels of 2/3 of the metabolites in maize grain tested responded to at least one potential cropping system management factor. However, while these changes in metabolite means were statistically significant, they accounted for very little of the total variance. Thus, most traditional crop production management practices represented among the five FSP cropping systems, such as tillage, crop rotation, fertility management, and pesticide use are poor candidates for manipulating metabolite levels in maize grain.
Separate quantitative data subsets for organic and conventional maize varieties were tested for the significance of cropping system impacts on the mean content of metabolites (Figure 1) as maize genetics (variety selection) can impact some metabolites substantially. Results indicate that cropping system is a significant factor determining the content of glucose, fructose and the unknown monosaccharide in the organic variety, and the unknown monosaccharide in the conventional variety. Among phenolics, the content of naringenin and luteolin in the conventional variety were significantly influenced by the cropping system. Other phenolic compounds, including the most abundant phenolic acids (ferulic acid, p-coumaric acid, and vanillin) and formic acid, were not influenced by the cropping system in either organic or conventional varieties.
Figure 1. Heat map giving contrast results for metabolite compounds (x-axis) for the two different maize varieties (organic, conventional) grown under various systems (y-axis). Blue squares are contrasts where the mean levels of the metabolite are significantly higher for the first system of the pair, at p < 0.01 for dark blue, and 0.01 < p < 0.05 for light blue. Red squares are contrasts where the mean levels of the metabolite are significantly higher for the second system of the pair, at p < 0.01 for dark red, and 0.01 < p < 0.05 for light red. O, Organic maize variety; C, Conventional maize variety; O2, Org2 or 2-year organic rotation; O3, Org3 or 3-year organic rotation; O6, Org6 or 6-year organic rotation; NT, 3-year conventional no-till rotation; CT, 3-year conventional tillage rotation. See materials and methods for more details.
The variance decomposition and results of F-tests for significance on the stepwise selected variables were largely consistent (i.e., fixed effects that explained a relatively large percent of the variance attributable to fixed effects were often significant). However, there were also instances where the two sets of results appeared contradictory. For example, there were four cases where the estimated percent contribution to the total variance was zero, yet that variable was deemed significant, and in several cases seemingly large percentages were not significant. Part of this, especially for low percentages, may have been due to the way the variables were constructed. For example, pH in the F-tests was a one degree of freedom linear effect; in the variance decomposition it was a four degree of freedom factor. However, for other effects, it appears that the parameters were simply poorly estimated within the model, i.e., with large standard errors. The variance decomposition does not include the accuracy of each estimated percent, and some were likely poorly estimated.
Metabolomic correlation analysis has been used to study impacts of genetic or environmental changes on metabolomes of plants and other organisms (Steuer, 2006; Szymanski et al., 2009; Fukushima et al., 2011; Fatima et al., 2016), and serves as a proxy indicating how metabolic pathways change under different conditions. Metabolomic correlation analysis was applied here to determine impacts of naturally occurring, cropping system, and crop variety impacts on the maize physiological state to complement the means analysis presented above. Metabolite interrelationships with significant changes in correlation (at α = 0.01) are given in Supplementary Table 2, except for six metabolites showing no changes in correlation and few significant changes among the various organic systems. The largest number of changes in metabolite correlations occurred among years, likely a response to strong environmental differences plants experienced with different years, such as rainfall. There were also a fair number of changes in correlations in the maize metabolome when conventional and organic varieties were compared. Changes in correlations among metabolites when organic systems were compared were small and few. When comparing Org2 to Org6 or Org3 to Org6, there were just two or three decreases in correlation. Between Org2 and Org3, there were two increases involving sugars and yield. More changes in correlation between metabolites occurred when comparing CT to the collective organic cropping systems (Org2, Org3, and Org6 collectively) or to the NT system. Importantly, correlation analysis showed that correlations between metabolites were not fixed, likely due to differences in regulation of biosynthetic pathways responsible for production of the evaluated compounds (e.g., see above the variety of biosynthetic pathways involved in production of phenolics detected with this present study) with different environmental conditions and crop varieties.
Metabolic fingerprinting of methanol extracts of maize grain using UHPLC coupled with MS detection identified a total of 90 compounds, the majority of which were phenolics. Phenolics from grain and other plant dietary sources are important phytochemicals that possess human health beneficial effects including antioxidant and anti-inflammatory activities that reduce certain chronic diseases (Liu, 2013; Zhang B. et al., 2020). Ferulic acid, p-coumaric acid, and vanillin were the predominant phenolic compounds detected, consistent with maize grain being considered an important source of ferulic acid and fiber in the human diet (Mathew and Abraham, 2004; Vitaglione et al., 2008). Other nutritional components of the maize grain metabolome, 7 carbohydrates and 7 organic acids, were also detected and analyzed. Macronutrients, minerals, vitamins, and essential amino acids and fatty acids important to human nutrition were not the subject of this investigation.
Means analysis on a subset of the metabolites we evaluated (15 phenolics, 1 phytohormone, 7 sugars, and 7 organic acids) indicated that the 5 cropping systems at FSP (two conventional and 3 organic systems) could significantly impact quantities of certain of these metabolites, and by extension other maize-grain nutrients important to human nutrition. However, cropping system effects explained very little of the total explainable variability, indicating that cropping system management tactics cannot be employed to consistently manipulate maize nutrient levels. By contrast, natural environmental influences (growing year) had a much greater impact on quantities of nutrients analyzed in maize grain. Correlation analysis of this same subset of compounds was consistent with the means analysis. Importantly, this is the first thorough analysis of the impact of cropping system on the maize metabolome where experiments were performed in well characterized soils where cropping systems had been in place long enough to alter soil edaphic and microbial properties (Green et al., 2005; Cavigelli et al., 2008; Spargo et al., 2011; Maul et al., 2019; Kepler et al., 2020; Schmidt et al., 2022). A second study comparing impacts of one organic and one conventional cropping system on a complementary set of maize grain metabolites (fatty acids, sugars, sugar alcohols, organic acids, amino acids and amines) support our findings as cropping systems impacts only led to minor changes in metabolite profiles and were subordinate to naturally occurring environmental impacts (Röhlig and Engel, 2010). However, the experiment was conducted only 1 year for this comparison. Our results are also consistent with the litany of studies finding no clear evidence for differences in nutritional quality between foods grown under conventional and organic production systems (Giampieri et al., 2022).
Large impacts of growing year on metabolite means and metabolite correlations were likely a response to strong environmental (non-management related) differences maize plants experienced during different years. This is consistent with findings from several metabolomic studies with maize looking at growing year impacts (Röhlig et al., 2009; Anttonen et al., 2010; Röhlig and Engel, 2010; Frank et al., 2012). Candidate factors in the present study that possibly varied yearly contributing to growing season impacts include rainfall, temperature, and light intensity. Substantial impacts have been found with geographically distant fields that differed in mean temperature, duration of day/night, average rainfall, and planting and harvesting date (Röhlig et al., 2009; Anttonen et al., 2010; Röhlig and Engel, 2010; Skogerson et al., 2010; Asiago et al., 2012; Frank et al., 2012; Baniasadi et al., 2014; Chen et al., 2016); which could influence the aforementioned factors.
Results indicate that until natural environmental impacts on maize grain metabolite levels are understood and can be managed, the best approach to reliably increase maize grain nutritional quality is through development of maize cultivars with enhanced nutritional content. Maize cultivar genetics had greater impact than cropping system on the maize grain metabolome and the greatest “managed” impact on the metabolite profile. Also, maize grain germplasm has moderate variability in metabolite concentrations to support a breeding approach and genetic manipulation to obtain improved maize grain nutritional content has been successful for high vitamin A and high lysine maize. However, new maize germplasm must be made that is robust to a variety of environmental conditions. It is possible that cropping system management of field-grown crops for improved nutritional content may never be obtained due to the variety and intricacy of regulation of biosynthesis of the nutrients, the difficulty in controlling environmental cues in production fields, and the interactions among environmental cues that influence the synthesis of these nutrients. Environmental factors that influence production of plant nutrients such as soil fertility, soil water availability, soil salinity, light intensity, ambient temperature, and pathogen infection are difficult to control and vary spatially and among production fields and temporally over growing seasons.
The original contributions presented in the study are included in the article/Supplementary material, further inquiries can be directed to the corresponding author.
This study was initiated by AM. AM, MC, DMi, UG, VM, MK, BK, DMa, JNŽ, and DR participated in planning, analysis of data, and writing the manuscript. All authors contributed to the article and approved the submitted version.
This study was supported by the United States Department of Agriculture. A part of this work was supported by the Ministry of the Education, Science and Technological Development of the Republic of Serbia, the contract nos. 451-03-68/2022-14/ 200007 and 451-03-9/2021-14/200053.
Mention of a trademark of a proprietary product does not constitute a guarantee, warranty or endorsement by the United States Department of Agriculture and does not imply its approval to the exclusion of other suitable products. This research is in part a contribution from the Long-Term Agroecosystem Research (LTAR) network. We thank Chris Rasmann, Anne Conklin, and Nhi Amy Tran for help in the field, lab and/or with data management.
The authors declare that the research was conducted in the absence of any commercial or financial relationships that could be construed as a potential conflict of interest.
All claims expressed in this article are solely those of the authors and do not necessarily represent those of their affiliated organizations, or those of the publisher, the editors and the reviewers. Any product that may be evaluated in this article, or claim that may be made by its manufacturer, is not guaranteed or endorsed by the publisher.
The Supplementary Material for this article can be found online at: https://www.frontiersin.org/articles/10.3389/fsufs.2023.1130089/full#supplementary-material
Acosta-Estrada, B. A., Serna-Saldívar, S. O., and Gutiérrez-Uribe, J. A. (2015). Chemopreventive effects of feruloyl putrescines from wastewater (Nejayote) of lime-cooked white maize (Zea mays). J. Cereal Sci. 64, 23–28. doi: 10.1016/j.jcs.2015.04.012
Anttonen, M. J., Lehesranta, S., Auriola, S., Röhlig, R. M., Engel, K-. H., Kärenlampi, S. O., et al. (2010). Genetic and environmental influence on maize kernel proteome. J. Proteome Res. 9, 6160–6168. doi: 10.1021/pr100251p
Asiago, V. M., Hazebroek, J., Harp, T., and Zhong, C. (2012). Effects of genetics and environment on the metabolome of commercial maize hybrids: a multisite study. J. Agric. Food Chem. 60, 11498–11508. doi: 10.1021/jf303873a
Baniasadi, H., Vlahakis, C., Hazeborek, J., Zhong, C., and Asiago, V. (2014). Effect of environment and genotype on commercial maize hybrids using LC/MS-based metabolomics. Agric. Food Chem. 62, 1412–1422. doi: 10.1021/jf404702g
Banjanac, T., Dragićević, M., Šiler, B., Gašić, U., Bohanec, B., Nestorović Živković, J., et al. (2017). Chemodiversity of two closely related tetraploid Centaurium species and their hexaploid hybrid: metabolomic search for high-resolution taxonomic classifiers. Phytochemistry 140, 27–44. doi: 10.1016/j.phytochem.2017.04.005
Bartolić, D., Maksimović, V., Maksimović, J. D., Stanković, M., Krstović, S., Baošić, R., et al. (2020). Variations in polyamine conjugates in maize (Zea mays L.) seeds contaminated with aflatoxin B1: a dose-response relationship. J. Sci. Food Agric. 100, 2905–2910. doi: 10.1002/jsfa.10317
Bates, D., Maechler, M., Bolker, B., and Walker, S. (2015). Fitting linear mixed-effects models using lme4. J. Statistical Software 67, 1–48. doi: 10.18637/jss.v067.i01
Bento-Silva, A., Duarte, N., Mecha, E., Belo, M., Vaz Patto, M. C., and do Rosário Bronze, M. (2020). Hydroxycinnamic acids and their derivatives in broa, a traditional ethnic maize bread. Foods 9, 1471. doi: 10.3390/foods9101471
Bento-Silva, A., Vaz Patto, M. C., and do Rosário Bronze, M. (2018). Relevance, structure and analysis of ferulic acid in maize cell walls. Food Chem. 246, 360–378. doi: 10.1016/j.foodchem.2017.11.012
Boniface, H., Tully, K., Mirsky, S. B., and Cavigelli, M. (2022). Evaluating the Impacts of Long-Term Management on Soil Carbon Dynamics in Mid-Atlantic US Grain Cropping Systems. ASA, CSSA, SSSA International Annual Meeting, Baltimore, MD.
Burt, A. J., Arnason, J. T., and García-Lara, S. (2019). Natural variation of hydroxycinnamic acid amides in maize landraces. J. Cereal Sci. 88, 145–149. doi: 10.1016/j.jcs.2019.06.002
Cavigelli, M. A., Teasdale, J. R., and Conklin, A. (2008). Long-term agronomic performance of organic and conventional field crops in the mid-Atlantic region. Agronomy J. 100, 785–794. doi: 10.2134/agronj2006.0373
Chen, M., Rao, R. S. P., Zhang, Y., Zhong, C., and Thelen, J. J. (2016). Metabolite variation in hybrid corn grain from a large-scale multisite study. Crop J. 4, 177–187. doi: 10.1016/j.cj.2016.03.004
Collison, A., Yang, L., Dykes, L., Murray, S., and Awika, J. M. (2015). Influence of genetic background on anthocyanin and copigment composition and behavior during thermoalkaline processing of maize. J. Agric. Food Chem. 63, 5528–5538. doi: 10.1021/acs.jafc.5b00798
Das, A. K., and Singh, V. (2015). Antioxidative free and bound phenolic constituents in pericarp, germ and endosperm of Indian dent (Zea mays var. Indentata) and flint (Zea mays var. Indurata) maize. J. Funct. Foods 13, 363–374. doi: 10.1016/j.jff.2015.01.012
Das, A. K., and Singh, V. (2016). Antioxidative free and bound phenolic constituents in botanical fractions of Indian specialty maize (Zea mays L.) genotypes. Food Chem. 201, 298–306. doi: 10.1016/j.foodchem.2016.01.099
Eleazu, C. O., Eleazu, F., Ukamaka, G., Adeolu, T., Ezeorah, V., Ezeorah, B., et al. (2021). Nutrient and antinutrient composition and heavy metal and phenolic profiles of Maize (Zea mays) as affected by different processing techniques. ACS Food Sci. Technol. 1, 113–123. doi: 10.1021/acsfoodscitech.0c00045
Fatima, T., Sobolev, A. P., Teasdale, J. R., Kramer, M., Bunce, J., Handa, A. K., et al. (2016). Fruit metabolite networks in engineered and non-engineered tomato genotypes reveal fluidity in a hormone and agroecosystem specific manner. Metabolomics 12, 103. doi: 10.1007/s11306-016-1037-2
Fatima, T., Teasdale, J. R., Bunce, J., and Mattoo, A. K. (2012). Tomato response to legume cover crop and nitrogen: differing enhancement patterns of fruit yield, photosynthesis and gene expression. Funct. Plant Biol. 39, 246–254. doi: 10.1071/FP11240
Frank, T., Röhlig, R. M., Davies, H. V., Barros, E., and Engel, K. H. (2012). Metabolite profiling of maize kernels: genetic modification vs. environmental influence. J. Agric. Food Chem. 60, 3005–3012. doi: 10.1021/jf204167t
Fukushima, A., Kusano, M., Redestig, H., Arita, M., and Saito, K. (2011). Metabolomic correlation-network modules in Arabidopsis based on a graph-clustering approach. Sys. Biol. 5, 1. doi: 10.1186/1752-0509-5-1
Gaffney, I., Sallach, J. B., Wilson, J., Bergström, E., and Thomas-Oates, J. (2021). (2021). Metabolomic approaches to studying the response to drought stress in corn (Zea mays) cobs. Metabolites 11, 438. doi: 10.3390/metabo11070438
Gan, Y. T., Miller, P. R., McConkey, B. G., Zentner, R. P., Stevenson, F. C., Mc Donald, C. L., et al. (2003). Influence of diverse cropping sequences on durum wheat yield and protein in the semiarid northern Great Plains. Agron. J. 95, 245–252. doi: 10.2134/agronj2003.2450
García-Lara, S., and Bergvinson, D. J. (2014). Phytochemical and nutraceutical changes during recurrent selection for storage pest resistance in tropical maize. Crop Sci. 54, 2423–2432. doi: 10.2135/cropsci2014.03.0223
García-Lara, S., Burt, A. J., Arnason, J. T., and Bergvinson, D. J. (2010). QTL mapping of tropical maize grain components associated with maize weevil resistance. Crop Sci. 50, 815–825. doi: 10.2135/cropsci2009.07.0415
Genter, C. F., Eheart, J. F., and Linkous, W. N. (1956). Effects of location, hybrid, fertilizer, and rate of planting on the oil and protein contents of corn grain. Agron. J. 48, 6–67. doi: 10.2134/agronj1956.00021962004800020005x
Giampieri, F., Mazzoni, L., Cianciosi, D., Alvarez-Suarez, J. M., Regolo, L., Sánchez-González, C., et al. (2022). Organic vs. conventional plant-based foods: a review. Food Chem. 383, 132352. doi: 10.1016/j.foodchem.2022.132352
Green, V. S., Cavigelli, M. A., Dao, T. H., and Flanagan, D. (2005). Soil physical properties and aggregate-associated C, N, and P distributions in organic and conventional cropping systems. Soil Sci. 170, 822–831. doi: 10.1097/01.ss.0000190509.18428.fe
Grusak, M. A., and DellaPenna, D. (1999). Improving the nutrient composition of plants to enhance human nutrition and health. Annu. Rev. Plant Physiol. Plant Mol. Biol. 50, 133–161. doi: 10.1146/annurev.arplant.50.1.133
Guo, W., and Beta, T. (2013). Phenolic acid composition and antioxidant potential of insoluble and soluble dietary fibre extracts derived from select whole-grain cereals. Food Res. Int'l. 51, 518–525. doi: 10.1016/j.foodres.2013.01.008
Halekoh, U., and Højsgaard, S. (2014). A Kenward-Roger approximation and parametric bootstrap methods for tests in linear mixed models—the R package pbkrtest. J. Stat. Software 59, 1–30. doi: 10.18637/jss.v059.i09
Hirschi, K. D. (2009). Nutrient biofortification of food crops. Annu. Rev. Nutr. 29, 401–429. doi: 10.1146/annurev-nutr-080508-141143
Jellum, M. D., and Marion, J. E. (1966). Factors affecting oil content and oil composition of corn (Zea mays L.) grain. Crop Sci. 6, 41–42. doi: 10.2135/cropsci1966.0011183X000600010012x
Jiang, Y. Z., and Wang, T. (2005). Phytosterols in cereal by-products. J. Amer. Oil Chem. Soc. 82, 439–444. doi: 10.1007/s11746-005-1090-5
Kandil, A., Li, J., Vasanthan, T., and Bressler, D. C. (2012). Phenolic acids in some cereal grains and their inhibitory effect on starch liquefaction and saccharification. J. Agric. Food Chem. 60, 8444–8449. doi: 10.1021/jf3000482
Kang, H. R., Yun, H. S., Lee, T. K., Lee, S., Kim, S-. H., Moon, E., et al. (2018). Chemical characterization of novel natural products from the roots of Asian rice (Oryza sativa) that control adipocyte and osteoblast differentiation. J. Agric. Food Chem. 66, 2677–2684. doi: 10.1021/acs.jafc.7b05030
Kepler, R. M., Epp Schmidt, D. J., Yarwood, S. A., Cavigelli, M. A., Reddy, K. N., Duke, S. O., et al. (2020). Soil microbial communities in diverse agroecosystems exposed to the herbicide glyphosate. Appl. Environ. Microbiol. 86, e01744–19. doi: 10.1128/AEM.01744-19
Kobayashi, M., Spray, C. R., Phinney, B. O., Gaskin, P., and MacMillan, J. (1996). Gibberellin metabolism in maize (the stepwise conversion of gibberellin A12-aldehyde to gibberellin A20. Plant Physiol. 110, 413–418. doi: 10.1104/pp.110.2.413
Kuznetsova, A., Brockhoff, P. B., and Christensen, R. H. B. (2017). lmerTest Package: tests in linear mixed effects models. J. Stat. Software 82, 1–26. doi: 10.18637/jss.v082.i13
Lee, S. Y., Choi, S. U., Lee, J. H., Lee, D. U., and Lee, K. R. (2010). A new phenylpropane glycoside from the rhizome of Sparganium stoloniferum. Arch. Pharm. Res. 33, 515–521. doi: 10.1007/s12272-010-0404-1
Lenth, R. V. (2022). Emmeans: Estimated Marginal Means, Aka Least-Squares Means. Available online at: https://github.com/rvlenth/emmeans (accessed March 08, 2023).
Li, Z., Zhao, C., Zhao, X., Xia, Y., Sun, X., Xie, W., et al. (2018). Deep annotation of hydroxycinnamic acid amides in plants based on ultra-high-performance liquid chromatography–high-resolution mass spectrometry and its in silico database. Anal. Chem. 90, 14321–14330. doi: 10.1021/acs.analchem.8b03654
Liu, R. H. (2013). Health-promoting components of fruits and vegetables in the diet. Adv. Nutr. 4384S-392S. doi: 10.3945/an.112.003517
Lopez-Martinez, L. X., Oliart-Ros, R. M., Valerio-Alfaro, G., Lee, C. H., Parkin, K. L., Garcia, H. S., et al. (2009). Antioxidant activity, phenolic compounds and anthocyanin content of eighteen strains of Mexican maize. LWT-Food Sci. Technol. 42, 1187–1192. doi: 10.1016/j.lwt.2008.10.010
Lux, P. E., Freiling, M., Stuetz, W., von Tucher, S., Carle, R., Steingass, C. B., et al. (2020). (Poly)phenols, carotenoids, and tocochromanols in corn (Zea mays L.) kernels as affected by phosphate fertilization and sowing time. J. Agric. Food Chem. 68, 612–622. doi: 10.1021/acs.jafc.9b07009
Malhi, S. S., Grant, G. A., Johnston, A. M., and Gill, K. S. (2001). Nitrogen fertilization management for no-till cereal production in Canadian Great Plan: a review. Soil Tillage Res. 60, 101–122. doi: 10.1016/S0167-1987(01)00176-3
Malhi, S. S., and Nyborg, M. (1990). Effect of tillage and straw on yield and N uptake of barley grown under different N fertility regimes. Soil Tillage Res. 17, 115–124. doi: 10.1016/0167-1987(90)90010-B
Marti, G., Erb, M., Boccard, J., Glauser, G., Doyen, G. R., Villard, N., et al. (2013). Metabolomics reveals herbivore-induced metabolites of resistance and susceptibility in maize leaves and roots. Plant Cell Environ. 36, 621–639. doi: 10.1111/pce.12002
Martin, C., and Li, J. (2017). Medicine is not health care, food is health care: plant metabolic engineering, diet and human health. New Phytol. 216, 699–719. doi: 10.1111/nph.14730
Mathew, S., and Abraham, T. E. (2004). Ferulic acid: an antioxidant found naturally in plant cell walls and feruloyl esterases involved in its release and their applications. Crit. Rev. Biotechnol. 24, 59–83. doi: 10.1080/07388550490491467
Mattoo, A. K., and Teasdale, J. R. (2010). Ecological and genetic systems underlying sustainable horticulture. Hort. Rev. 37, 331–362. doi: 10.1002/9780470543672.ch6
Maul, J. E., Cavigelli, M. A., Emche, S. E., Vinyard, B. T., and Buyer, J. S. (2019). Cropping system history and crop rotation phase drive the abundance of soil denitrification genes nirK, nirS and nosZ in conventional and organic grain agroecosystems. Agric. Ecosys. Environ. 273, 95–106. doi: 10.1016/j.agee.2018.11.022
Mišić, D., Šiler, B., Nestorović Živković, J., Simonović, A., Maksimović, V., Budimir, S. Nikolić. M., et al. (2012). Contribution of inorganic cations and organic compounds to osmotic adjustment in root cultures of two Centaurium species differing in tolerance to salt stress. Plant. Cell Tiss. Organ. Cult. 108, 389–400. doi: 10.1007/s11240-011-0050-4
Neelam, A., Cassol, T., Mehta, R. A., Abdul-Baki, A. A., Sobolev, A., Goyal, R. K., et al. (2008). A field-grown transgenic tomato line expressing higher levels of polyamines reveals legume cover crop mulch-specific perturbations in fruit phenotype at the levels of metabolite profiles, gene expression and agronomic characteristics. J. Exp. Bot. 59, 2337–2346. doi: 10.1093/jxb/ern100
Nuss, E. T., and Tanumihardjo, S. A. (2010). Maize: a paramount staple crop in the context of global nutrition. Comp. Rev. Food Sci. Food Safety. 9, 417–436. doi: 10.1111/j.1541-4337.2010.00117.x
Poiroux-Gonord, F., Bidel, L. P. R., Fanciullino, A-. L., Gautier, H., Lauri-Lopez, F., Urban, L., et al. (2010). Health benefits of vitamins and secondary metabolites of fruits and vegetables and prospects to increase their concentrations by agronomic approaches. J. Agric. Food Chem. 58, 12065–12082. doi: 10.1021/jf1037745
R Core Team (2018). R: A Language and Environment for Statistical Computing. R Foundation for Statistical Computing, Vienna, Austria.
Reganold, J. P., and Wachter, J. M. (2016). Organic agriculture in the twenty-first century. Nature Plants 2, 15221. doi: 10.1038/nplants.2015.221
Revelle, W. (2018). psych: Procedures for Personality and Psychological Research. Northwestern University, Evanston, Illinois, USA
Reynolds, T. L., Neeth, M. A., Glenn, K. C., Ridley, W. P., and Astwood, J. D. (2005). Natural variability of metabolites in maize grain: differences due to genetic background. J. Agric. Food Chem. 53, 10061–10067. doi: 10.1021/jf051635q
Roberts, D. P., and Mattoo, A. K. (2018). Sustainable agriculture—enhancing environmental benefits, food nutritional quality and building crop resilience to abiotic and biotic stresses. Agriculture 8, 8. doi: 10.3390/agriculture8010008
Roberts, D. P., and Mattoo, A. K. (2019). Sustainable crop production and human nutrition. Front. Sust. Food Sys. 3, 72. doi: 10.3389/fsufs.2019.00072
Röhlig, R. M., Eder, J., and Engel, K. (2009). Metabolite profiling of maize grain: Differentiation due to genetics and environment. Metabolomics 5, 459–477. doi: 10.1007/s11306-009-0171-5
Röhlig, R. M., and Engel, K. H. (2010). Influence of the input system (conventional vs. organic farming) on metabolite profiles of maize (Zea mays) kernels. J. Agric. Food Chem. 58, 3022–3030. doi: 10.1021/jf904101g
Santiago, R., and Malvar, R. A. (2010). Role of dehydrodiferulates in maize resistance to pests and diseases. Int'l. J. Molec. Sci. 11, 691–703. doi: 10.3390/ijms11020691
Sauter, A., Abrams, R. R., and Hartung, W. (2002). Structural requirements of abscisic acid (ABA) and its impact on water flow during radial transport of ABA analogues through maize roots. J. Plant Growth Reg. 21, 50–59. doi: 10.1007/s003440010040
Schmidt, D. E., Dlott, G., Cavigelli, M. A., Yarwood, S., and Maul, J. E. (2022). Soil microbes in three farming systems more affected by depth than farming systems. Appl. Soil Ecol. 173, 104396. doi: 10.1016/j.apsoil.2022.104396
Sen, A., Bergvinson, D., Miller, S. S., Atkinson, J., Fulcher, R. G., Arnason, J. T., et al. (1994). Distribution and microchemical detection of phenolic acids, flavonoids, and phenolic acid amides in maize kernels. J. Agric. Food Chem. 42, 1879–1883. doi: 10.1021/jf00045a009
Sheng, S., Li, T., and Lium, R. (2018). Corn phytochemicals and their health benefits. Food Sci. Human Wellness. 7, 185–195. doi: 10.1016/j.fshw.2018.09.003
Shirota, O., Sekita, S., Satake, M., Ni, Y., and Weiyi, H. (1996). Chemical constituents of Chinese folk medicine “Sân Léng”, Sparganium stoloniferum. J. Nat. Prod. 59, 242–245. doi: 10.1021/np960160h
Skogerson, K., Harrigan, G. G., Reynolds, T. L., Halls, S. C., Ruebelt, M., Iandolino, A., et al. (2010). Impact of genetics and environment on the metabolite composition of maize grain. J. Agric. Food Chem. 58, 3600–3610. doi: 10.1021/jf903705y
Spargo, J. T., Cavigelli, M. A., Mirsky, S. B., Maul, J. E., and Meisinger, J. J. (2011). Mineralizable soil nitrogen and labile soil organic matter in diverse long-term cropping systems. Nutr. Cycl. Agroecosyst. 90, 253–266. doi: 10.1007/s10705-011-9426-4
Steuer, R. (2006). On the analysis and interpretation of correlations in metabolomic data. Briefings Bioinform. 7, 151–158. doi: 10.1093/bib/bbl009
Szymanski, J., Jozefczuk, S., Nikoloski, Z., Selbig, J., Nikiforova, V., Catchpole, G., et al. (2009). Stability of metabolic correlations under changing environmental conditions in Escherichia coli—A systems approach. PLoS ONE 4, e7441. doi: 10.1371/journal.pone.0007441
Tanumihardjo, S. A. McCulley, L., Roh, R., Lopez-Ridaura, S., Palacios-Rojas, N., and Gunaratna, N. S. (2020). Maize agro-food systems to ensure food and nutrition security in reference to the sustainable development goals. Global Food Sec. 25, 100327. doi: 10.1016/j.gfs.2019.100327
Tateishi, K., Shibata, H., Matsushima, Y., and Iijima, T. (1987). Isolation of two new IAA conjugates, 7'O-β-d- glucopyranoside of 3,7-dihydroxy-2-indolinone-3-acetic acid and 8'-O-d-glucopyranoside of 8-hydroxy-2-quinolone-4-carboxylic acid, from immature sweet corn kernels (Zea mays L). Agric. Biol. Chem. 51, 3445–3447. doi: 10.1271/bbb1961.51.3445
Teasdale, J. R., and Cavigelli, M. A. (2010). Subplots facilitate assessment of corn yield losses from weed competition in a long-term cropping systems experiment. Agron. Sustain. Devel. 30, 445–453. doi: 10.1051/agro/2009048
Teasdale, J. R., and Cavigelli, M. A. (2017). Meteorological fluctuations define long-term crop yield patterns in conventional and organic production systems. Sci. Rep. 7, 688. doi: 10.1038/s41598-017-00775-8
Thakur, S., Singh, N., Kaur, A., and Singh, B. (2017). Effect of extrusion on physicochemical properties, digestibility, and phenolic profiles of grit fractions obtained from dry milling of normal and waxy corn. J. Food Sci. 82, 1101–9. doi: 10.1111/1750-3841.13692
Treonis, A. M., Unangst, S., Kepler, R., Emche, S., Buyer, J., Cavigelli, M. A., et al. (2018). Characterization of soil nematode communities among three cropping systems through morphological and DNA metabarcoding approaches. Sci. Rep. 8, 2004. doi: 10.1038/s41598-018-20366-5
Vasconcelos, M. W., Gruissem, W., and Bhullar, N. K. (2017). Iron biofortification in the 21st century: setting realistic targets, overcoming obstacles, and new strategies for healthy nutrition. Curr. Opin. Biotechnol. 44, 8–15. doi: 10.1016/j.copbio.2016.10.001
Venkatesh, T. V., Chassy, A. W., Fiehn, O., Flint-Garcia, S., Zeng, Q., Skogerson, K., et al. (2016). Metabolomic assessment of key maize resources: GC-MS and NMR profiling of grain from B73 hybrids of the nested association mapping (NAM) founders and of geographically diverse landraces. J. Agric. Food Chem. 64, 2162–2172. doi: 10.1021/acs.jafc.5b04901
Vitaglione, P., Napolitano, A., and Fogliano, V. (2008). Cereal dietary fibre: a natural functional ingredient to deliver phenolic compounds into the gut. Trends Food Sci. Technol. 19, 451–463. doi: 10.1016/j.tifs.2008.02.005
Wang, Z-. H., Li, S-. X., and Malhi, S. (2008). Effects of fertilization and other agronomic measures on nutritional quality of crops. J. Sci. Food Agric. 88, 7–23. doi: 10.1002/jsfa.3084
White, K. E., Cavigelli, M. A., and Bagley, G. (2021). Legumes and nutrient management improve phosphorus and potassium balances in long-term crop rotations. Agronomy J. 113, 2681–2697. doi: 10.1002/agj2.20651
White, K. E., Cavigelli, M. A., Conklin, A. E., and Rasmann, C. (2019). Economic performance of long-term organic and conventional crop rotations in the Mid-Atlantic. Agron. J. 111, 1358–1370. doi: 10.2134/agronj2018.09.0604
Zhang, B., Zhang, Y., Li, H., Deng, Z., and Tsao, R. (2020). A review on insoluble-bound phenolics in plant-based food matrix and their contribution to human health with future perspectives. Trends. Food Sci. Technol. 105, 347–362. doi: 10.1016/j.tifs.2020.09.029
Zhang, F., Wu, J., Sade, N., Wu, S., Egbaria, A., Fernie, A. R., et al. (2021). Genomic basis underlying the metabolome-mediated drought adaptation of maize. Genome Biol. 22, 260. doi: 10.1186/s13059-021-02481-1
Zhang, L., Yu, Y., and Yu, R. (2020). Analysis of metabolites and metabolic pathways in three maize (Zea mays L.) varieties from the same origin using GC-MS. Sci. Rep. 10, 17990. doi: 10.1038/s41598-020-73041-z
Zhou, S., Zhang, Y. K., Kremling, K. A., Ding, Y., Bennett, J. S., Bae, J. S., et al. (2019). Ethylene signaling regulates natural variation in the abundance of antifungal acetylated diferuloylsucroses and Fusarium graminearum resistance in maize seedling roots. New Phytol. 221, 2096–2111. doi: 10.1111/nph.15520
Keywords: cropping system, environment, maize grain, metabolomics, phenolics
Citation: Mattoo AK, Cavigelli MA, Mišić DM, Gašić U, Maksimović VM, Kramer M, Kaur B, Matekalo D, Nestorović Živković J and Roberts DP (2023) Maize metabolomics in relation to cropping system and growing year. Front. Sustain. Food Syst. 7:1130089. doi: 10.3389/fsufs.2023.1130089
Received: 22 December 2022; Accepted: 27 March 2023;
Published: 21 April 2023.
Edited by:
Raju Datla, Global Institute for Food Security (GIFS), CanadaReviewed by:
Lena Gálvez Ranilla, Catholic University of Santa María, PeruCopyright © 2023 Mattoo, Cavigelli, Mišić, Gašić, Maksimović, Kramer, Kaur, Matekalo, Nestorović Živković and Roberts. This is an open-access article distributed under the terms of the Creative Commons Attribution License (CC BY). The use, distribution or reproduction in other forums is permitted, provided the original author(s) and the copyright owner(s) are credited and that the original publication in this journal is cited, in accordance with accepted academic practice. No use, distribution or reproduction is permitted which does not comply with these terms.
*Correspondence: Autar K. Mattoo, YXV0YXIubWF0dG9vQHVzZGEuZ292
Disclaimer: All claims expressed in this article are solely those of the authors and do not necessarily represent those of their affiliated organizations, or those of the publisher, the editors and the reviewers. Any product that may be evaluated in this article or claim that may be made by its manufacturer is not guaranteed or endorsed by the publisher.
Research integrity at Frontiers
Learn more about the work of our research integrity team to safeguard the quality of each article we publish.