- 1Global Alliance for Improved Nutrition (GAIN), Washington, DC, United States
- 2International Livestock Research Institute (ILRI), Nairobi, Kenya
- 3Natural Resources Institute, University of Greenwich, Greenwich, United Kingdom
Aflatoxin contamination of staple grains and legumes has been linked to hepatocellular carcinoma (HCC) and other adverse health outcomes, constituting a substantial public health concern globally. Low-resource food environments in sub-Saharan Africa are often under-regulated and are particularly vulnerable to adverse health and nutrition outcomes associated with aflatoxin exposure. This study identifies levels of HCC risk in the northern Nigerian adult population, leveraging a systematic review of available evidence on aflatoxin contamination in Nigerian maize, groundnut, rice, cowpea, and soybean. Estimated dietary intake (EDI) was computed using publicly available dietary consumption data and a probabilistic quantitative risk assessment was conducted to determine the relative risk of HCC associated with consumption of selected aflatoxin-contaminated commodities. In total, 41 eligible studies reporting aflatoxin contamination were used to model the distribution of aflatoxin concentrations in Nigerian commodities. EDIs for maize, groundnut, rice, and cowpea exceeded the provisional maximum tolerable daily intake (PMTDI) level of 1 kgbw-1 day-1, with maize yielding the highest mean EDI (36.7 kgbw-1 day-1). The quantitative risk assessment estimated that 1.77, 0.44, 0.43, 0.15, and 0.01 HCC cases per year/100,000 population were attributable to aflatoxin exposure through maize, groundnut, rice, cowpea, and soybean, respectively. Sensitivity analysis revealed that aflatoxin concentration, dietary consumption levels, consumption frequency, and other variables have differing relative contributions to HCC risk across commodities. These findings constitute a novel multi-study risk assessment approach in the Nigerian context and substantiate existing evidence suggesting that there is reason for public health concern regarding aflatoxin exposure in the Nigerian population.
1. Introduction
Traditional food markets—also known as informal markets and comprising “wet” or public markets, small shops, vendors and other non-modern retail—are critical for ensuring access to safe, nutritious diets in many low- and middle-income countries (LMIC) (Wertheim-Heck et al., 2019). Food safety regulatory systems in many LMIC markets, formal and informal, are often insufficient for preventing exposures to contaminants that can jeopardize community health and offset the nutritive value of food. However, most of the poor obtain food from traditional markets so these are of especial interest (Grace, 2015). One such contaminant, aflatoxin, is widespread in informal grain value chains in LMICs and constitutes a food safety concern of burgeoning importance both for public health and economic prosperity in traditional market settings. Aflatoxin is a potent carcinogen, implicated in hepatocellular carcinoma (HCC) in humans, and, in some contexts, a leading risk factor for HCC (Liu and Wu, 2010). In addition to its carcinogenic properties, aflatoxin is associated with growth impairment in children (Khlangwiset et al., 2011) and may be an important driver of environmental enteropathy, resulting in compromised gut integrity and limited nutrient adsorption (Smith et al., 2012), although evidence for contribution to stunting is less strong (Hoffmann et al., 2018). The risk of dietary aflatoxin exposure is particularly pronounced in many sub-Saharan African food systems, where obsolete monitoring infrastructure, poverty, and weak beaurocratic structures coincide with high reliance on aflatoxin-susceptible staples, such as maize and groundnuts.
In the context of Northern Nigeria, the breadth and magnitude of aflatoxin exposure risk associated with foods sold in traditional markets are poorly understood, particularly for the most vulnerable consumers, limiting the capacity to identify and mobilize mitigation opportunities. Foods acquired at traditional markets have distinct characteristics compared to those produced on farms and consumed locally. Foods sold at these markets are usually subjected to various layers of informal and formal quality control, such as sorting, pest management, or visual inspection by supply chain intermediaries, which can in some circumstances measurably reduce the aflatoxin burden in food; for example, it was found that the prevalence of mycotoxins in traders' maize was lower than at other nodes of the value chain, even after a period of storage (Liverpool-Tasie et al., 2019). In addition, the consumer has direct control of quantity when purchasing food, enabling them to purchase quantities that they know will not spoil before consumption. On the other hand, with respect to purchased foods, the consumer has little control over pre-market nodes of the value chain, such as production, storage, and transport, which can all be critical control points for aflatoxin management (Massomo, 2020). Given that aflatoxin contamination is often not visibly identifiable without specialized technology, even grain that appears safe in the marketplace may be contaminated.
Northern Nigeria's traditional food markets cater to diverse populations, and supply communities with numerous food commodities. As in the rest of the country, maize and rice—both susceptible to aflatoxin contamination—are important staple grains in the region. Maize is commonly ground to flour and consumed as a stiff porridge (tuwo masara) or similar preparations. Rice is consumed either cooked as-is or ground to flour and consumed as cakes (masa). High-protein legumes such as cowpea and soybean are an important part of the local diet, but there has historically been little evidence on aflatoxin contamination in this crop. A steamed cowpea pudding, moimoi, is commonly consumed in the region. Soybean is commonly consumed as a curdled soymilk “cheese” or tofu-like product (awara) in the region. Groundnut, a major source of dietary aflatoxin globally, is an important crop in the region with production centered in the northern states of Niger, Kano, Zamfara, Kebbi, and others, contributing measurably to the Nigerian economy (Usman et al., 2013). Apart from whole groundnuts as a snack food, groundnut press cake (kulikuli)—a by-product of traditional or mechanical oil extrusion—is often shaped into various forms and consumed as a fried snack. Many of these commodities are available as raw ingredients or as ready-to-eat prepared foods sold at the marketplace.
Despite a substantial body of survey evidence regarding aflatoxin contamination in Nigerian food commodities, including in marketplace environments, there has hitherto not been any comprehensive risk assessment that has focused on this context. The aim of this study, implemented as part of the United States Agency for International Development (USAID) Feed the Future Initiative program, EatSafe: Evidence and Action Toward Safe, Nutritious Food (EatSafe), is to elucidate the extent of aflatoxin exposure risk associated with commodities commonly purchased from traditional food markets in Nigeria. At the start, a systematic review was conducted of aflatoxin contamination in five aflatoxin-susceptible local commodities, namely maize, groundnut, rice, cowpea, and soybean, reported in surveys from Nigeria. We used these aflatoxin occurrence data, in conjunction with secondary data on local dietary consumption, to estimate exposure and risk of HCC using a probabilistic quantitative risk assessment methodology. Based on these findings, the relative risk associated with local consumption of each commodity was determined and key recommendations identified for aflatoxin management in northern Nigerian traditional food markets.
2. Materials and methods
2.1. Literature review of aflatoxin contamination and prevalence in Nigerian commodities
A systematic review of peer-reviewed literature was conducted in Web of Science to ascertain reported data on aflatoxin contamination in Nigerian maize, groundnut, cowpea, soybean, rice, and other food products derived therefrom. Each search included three keywords, (1) “aflatoxin,” (2) the commodity name, and (3) the geographic focus, “Nigeria.” Articles were first assessed for relevance based on a title and abstract screening, followed by full text review of relevant articles. Eligibility for inclusion in the study was assessed using the following inclusion criteria: (1) publication date 1980—present, (2) aflatoxin, either total aflatoxin or aflatoxin B1 (AFB1) concentration determined for raw commodity (not cooked or prepared), (3) samples are reasonably intended for human consumption (not for livestock feed, etc.), (4) fungal growth is naturally-occurring, not artificially inoculated, (5) aflatoxin is detected in at least one sample (studies with 0% detection retained for modeling prevalence, but excluded from the contamination model), (6) reported means reflect batches of several distinct samples, not replicates of the same pooled sample(s), (7) the number of total samples analyzed is reported, and (8) an estimate of batch variance (standard deviation, range, etc.) is reported. Additional studies were identified by the authors via non-systematic searches in Google Scholar using similar keywords.
Data extracted from eligible studies included: mean, standard deviation, median, and range of aflatoxin concentration (ng/g); study year; country; sub-national division; commodity type; sample source (household, market, or prepared food); metabolite (total aflatoxin or aflatoxin B1); total number of samples; number of positive samples or detection rate; limit of detection. Individual batch means reported within studies were extracted separately, when possible. Risk modeling was done based on the aflatoxin B1 (AFB1) metabolite, in accordance with the known carcinogenic potency associated with the hepato-carcinogenic effects of AFB1 in conjunction with hepatitis B infection (JECFA, 1999). Whenever available, reported estimates of AFB1 concentration were extracted from the included studies. To be as conservative as possible (i.e., to prevent under-estimation of exposure) in risk assessment when only total aflatoxin concentration was reported, the reported total aflatoxin levels were treated as AFB1 in the subsequent risk assessment. When only the concentration range (minimum and maximum) was reported, the midrange (e.g., min+max/2) was used for analysis in place of the mean, as previously reported (Andrade and Caldas, 2015).
The spatial distributions of available aflatoxin contamination data for each commodity were visualized using GIS mapping. Batch-wise estimates of mean aflatoxin concentration were grouped by state of sample origin. Studies wherein multiple (>2) states/regions were pooled, and therefore geographic origin indistinguishable, were omitted from GIS analysis. If a study's reported batches encompassed two states but state origins were not reported on a batchwise basis, the study was classified as the majority-sampled state for GIS mapping if >2/3 of batches were from that state; otherwise, the study was omitted from the maps. Maps were generated with the ‘ggspatial' package in R using open-access GIS datasets (Global Administrative Areas, 2022).
2.2. Risk modeling approach
A quantitative risk assessment model was developed to estimate exposure to AFB1 associated with consumption of staple foods in Nigeria, as well as the risk of HCC, a key adverse health outcome associated with aflatoxin dietary exposure. The model considers a “market to table” scenario to assess absolute and relative exposure associated with different food commodities and does not aim to include pre-harvest or supply chain risk factors upstream of retail. The model takes as key input the distribution of aflatoxin prevalence and concentration in the product at the point of purchase, and follows portions of each food through home handling, preparation, and consumption. Parameters included in the model and their distributions are summarized in Table 1 and described in the following sections. The model was programmed and executed in the R software environment (R Core Team, 2021). A second-order Monte-Carlo approach was used to account for both variability and uncertainty (using 1,000 iterations in each dimension) in input parameters and implemented using the “mc2d” package in R. The model included several key assumptions: (1) no fungal growth or additional toxin accumulation occurs during storage, before consumption; (2) aflatoxin concentration is stable at normal cooking temperatures, i.e., no concentration reduction occurs during cooking (Kabak and Var, 2008); (3) no detoxification procedure is implemented by the consumer, i.e., no “inactivation” of the toxin is accounted for.
The exposure model was based on Estimated Daily Intake (EDI) of aflatoxin attributable to per-capita consumption of each commodity. EDI was estimated for the study population using an equation adapted from that described previously (Udovicki et al., 2021):
Where C = concentration of aflatoxin per kg sample (ng/g), S = g consumed per person per day on a day the product is consumed, i = the frequency of contamination among portions, fcons = the frequency of maize consumption in the population, and BW = body weight (kg). An exposure model was constructed for each commodity using the probability distributions of C, S, i, fcons, and BW, following the EDI equation described above, and incorporated into a Monte-Carlo simulation model for risk assessment. Exposure model input parameters for each commodity are summarized in Table 1.
2.3. Probability distributions of input parameters
2.3.1. Aflatoxin prevalence in samples (i)
To account for the fact that not all samples (and, thus, not all portions consumed) are contaminated with detectable levels of aflatoxin, the probability of consuming a contaminated portion (i) was approximated by aflatoxin prevalence, or detection rate, in the model. The prevalence data reported by the literature considered were fit to a beta distribution and uncertainty around the parameter estimates was modeled using parametric bootstrapping (1,000 iterations) using the ‘bootdist' function in the ‘fitdistrplus' R package (Delignette-Muller and Dutang, 2015). In addition to its representation of the probability of consuming a contaminated portion, the ‘i” parameter was also used to correct for possible overestimation of exposure attributable to the tendency in the literature to report means only for contaminated samples (i.e., excluding non-detects). Due to the small number of studies reporting detection rate for soybean, it was not possible to fit a beta distribution to the data; instead, the mean detection rate reported in the available literature (7.3%; see reference in Table 1) was used in the exposure model.
2.3.2. Aflatoxin concentration in samples (C)
To select an appropriate probability distribution to describe the observed aflatoxin concentrations (C) reported by the included studies, for samples where aflatoxin was detected, the empirical distribution of study means was examined, and a series of goodness-of-fit tests were performed. Concentration estimate data were assumed to be derived using assays of the same sensitivity and precision; in other words, the initial mass of samples collected and analyzed was ignored. Given the expected skewedness of aflatoxin data, three probability distributions suitable for skewed data were considered: gamma, Weibull, and log-normal, in addition to the normal distribution. Q–Q plots, P–P plots, and cumulative distribution functions (CDFs) were examined for model fit. Three Goodness-of-Fit statistics (namely the Kolmogorov-Smirnov statistic, the Cramer-von Mises statistic, and the Anderson-Darling statistic) were estimated for each of the probability distributions and compared (Supplementary material S1). As a final test of Goodness-of-Fit across the candidate distributions, uncertainty in the parameters of the fitted distributions was estimated using parametric bootstrapping (1,000 iterations). To ensure adequate fit of the selected distributions, Q–Q plots and P–P plots were examined for the final distributions before proceeding with exposure assessment and risk analysis (see Supplementary material S2). For use in quantitative risk assessment, a distribution simulating variability conditional on the uncertainty bootstraps was generated using the ‘mcstoc' function in the ‘mc2d' R package. The upper bound used for truncation of the distribution was the maximum batch aflatoxin concentration reported for each commodity in the included studies.
Given that there were very few (n = 1) observations of detectable aflatoxin in soybean batches in the included studies, it was not possible to compute Goodness-of-Fit statistics for the candidate distributions, for this commodity. Instead, a log-normal distribution (truncated between 0 and the highest observed sample maximum) was assumed and fit to the available data. As the one included study reporting detectable aflatoxin in Nigeria reported a sample maximum of only 2 ng/g, a higher (i.e., more conservative) value of 4 ng/g, reported in a Rwandan study (Niyibituronsa et al., 2019) was used as the upper bound of the truncated distribution. Similarly, as there were scant records of aflatoxin contamination in Nigerian cowpea samples, one record from a neighboring country (Benin) that was identified in the systematic literature review was included in the dataset (Houssou et al., 2009).
2.3.3. Commodity consumption (fcons and S)
Two food consumption parameters were included as inputs in the exposure assessment model: (1) the frequency of consumption events (fcons), and (2) the quantity of product consumed per consumption event (S). For all commodities, fcons and S were derived from previously reported consumption data for key prepared food consumed in the study region. Local foods selected for the estimation included tuwo masara (maize), kulikuli (groundnut), cooked rice, moi-moi (cowpea), and awara (soybean), each of which is a common local preparation of the focus commodity. Empirical distributions were fit to fcons data (consumption events/week) for each commodity, based on reported values in the literature (Table 2). To account for the fact that aflatoxin data were reported for raw (unprepared) commodities, mass conversion rates (m) were applied to portion sizes to determine the equivalent of raw commodity consumed per portion (Table 2). Consumption amount S was assumed normally-distributed, and means (±SD) from prior studies were used to fit a probability distribution truncated at the lower bound of 0 (e.g., no negative consumption).
2.3.4. Adult body weight
Previously reported estimates of adult body weight (men, women, and the general population) for a Nigerian population (Akinpelu et al., 2015) were used to fit a probability distribution of this parameter for the exposure assessment. A mean (±SD) adult body weight of 63.17 (±10.28) kilograms (Akinpelu et al., 2015) was used for exposure assessment. Adult body weight was assumed to follow a normal distribution as per the approach previously described by Sirma et al. (2019).
2.3.5. Risk characterization: HCC
The risk of aflatoxin B1-associated hepatocellular carcinoma (HCC) attributable to consumption of each commodity was estimated based on probability distributions of exposure (i.e., EDI), the constituent aspects thereof, and the carcinogenic potency of aflatoxin. The carcinogenic potency of aflatoxin, defined as the probability of HCC (“r”) per 100,000-individual population was estimated using the equation (Udovicki et al., 2021):
It has been demonstrated that the probability of HCC attributable to aflatoxin exposure is greater for individuals with Hepatitis B surface antigen (HBsAG) positivity by a factor of 30. A HBsAG positivity rate of 13.2% has been reported for the Nigerian population (Garba et al., 2021). Thus, the carcinogenic potency was estimated as: (0.3 × 0.132) + [0.01 × (1–0.132)] = 0.04828. The risk of disease (HCC cases/year per 100,000 population) was estimated by multiplying the EDI by the carcinogenic potency (Udovicki et al., 2021), using the equation:
Sensitivity analyses were performed for each commodity-wise risk model in order to determine the relative contributions of the input parameters to the level of HCC risk. Spearman's rank-order correlation coefficient (ρ) was computed and tornado graphs generated for each model. This coefficient takes values between −1 and 1, where values nearer to 1 are more highly correlated with the outcome (HCC risk). Mean and median values of Spearman's ρ were computed and assessed together with 95% uncertainty intervals around the estimates.
3. Results
3.1. Occurrence of aflatoxin in Nigerian food products
Results of the systematic literature review are presented in Figure 1. The search strategy yielded 285 studies from the Web of Science search. An additional 14 studies were identified by the authors using Google Scholar and other sources, yielding a total of 300 studies, eligible for title and abstract screening. Among these records, 244 were found to be irrelevant and were excluded from full-text review. The remaining 55 records were subjected to full-text screening for eligibility against the inclusion and exclusion criteria. A total of 41 studies satisfied all criteria and were included in the analysis, including 18, 12, 12, 5, and 3 studies each for maize, groundnut, rice, cowpea, and soybean; five of the included studies reported on more than one of the target commodities (see Table 1).
The included studies reported 40, 26, 36, 5, and 3 estimates of batch-wise aflatoxin contamination in Nigerian maize, groundnuts, rice, cowpea, and soybean, respectively, including 4 batches in which aflatoxin was not detected. A batch is here defined as a mean representing several (>2) distinct samples, not replicate measures of the same pooled sample. Aflatoxin contamination data reported in the included studies are summarized in Table 2. Of the 41 studies included in the analysis, 16 (39%) reported only total aflatoxin, while the remaining studies included estimates of AFB1. Among the batches included in the study, AFB1 estimates were available for 62%, while the remaining 38% of batches yielded estimates of total aflatoxins only. Prevalence data (% positive samples reported within a given batch) were available for 81.8% of reported batches, where “positive” is here used interchangeably with “detected”. Prevalence was highest in groundnut (84.5% ± 17.8) and rice (74.3% ± 29.3) batches, and lowest in soybean (7.3% ± 12.7). However, very few observations available for soybeans and cowpea make results for these two commodities not generalizable.
Maize and groundnut were observed to have the highest mean aflatoxin contamination levels (106.4 ± 154.1 and 78.8 ± 219.2 ng/g, respectively) among the considered commodities. Mean AFB1 concentrations exceeded Nigerian regulatory legal limit of 2 ng/g AFB1 in maize and 20 ng/g total aflatoxin in groundnuts, respectively (Chilaka et al., 2022). Aflatoxin is presently not regulated in Nigeria for rice, cowpea, and soybean, but concentrations observed in rice and cowpea exceeded all limits for aflatoxins in regulated commodities. Groundnut yielded the highest batch-wise maximum aflatoxin concentration, with a concentration of 939 ng/g in the most contaminated batch. The mean values for all batches with at least one contaminated sample are shown in Figure 2.
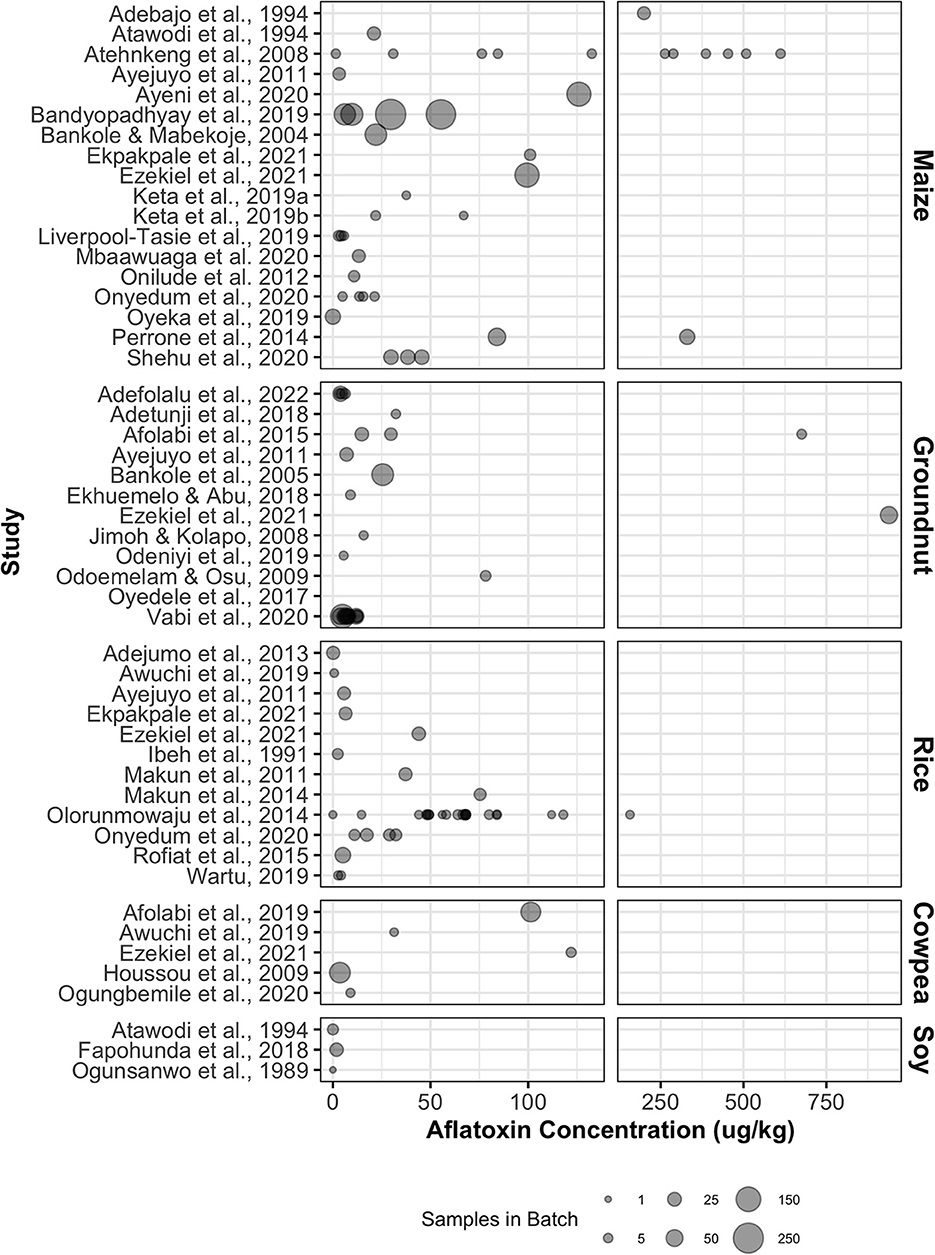
Figure 2. Summary of mean aflatoxin concentrations reported for batches of raw commodity samples in the included studies. Point size corresponds to the number of samples within each batch.
The aflatoxin data identified in the systematic review were not representative of the entire Nigerian context, but rather were concentrated in certain regions (Figure 3). Of the individual batches reported, the geographical origins were specified at State-level for 70%, while the remaining 30% of batches were geographically specified only at the regional level. By far, the greatest sampling effort at State-level was Kaduna State, which yielded 20% of the batches included in this study. Other states with relatively high sampling efforts included Niger State (8%), Kebbi State (7%), Oyo State (6%), and Lagos (5%). There was a conspicuous lack of available data reporting aflatoxin concentrations in the northeastern and southern regions of the country. The geographical breadth of sampling effort across commodities corresponded to the overall abundance of each commodity in the dataset; the geographical distributions of maize and groundnut batches (the most abundant reported commodities) were greater than those of the other commodities.
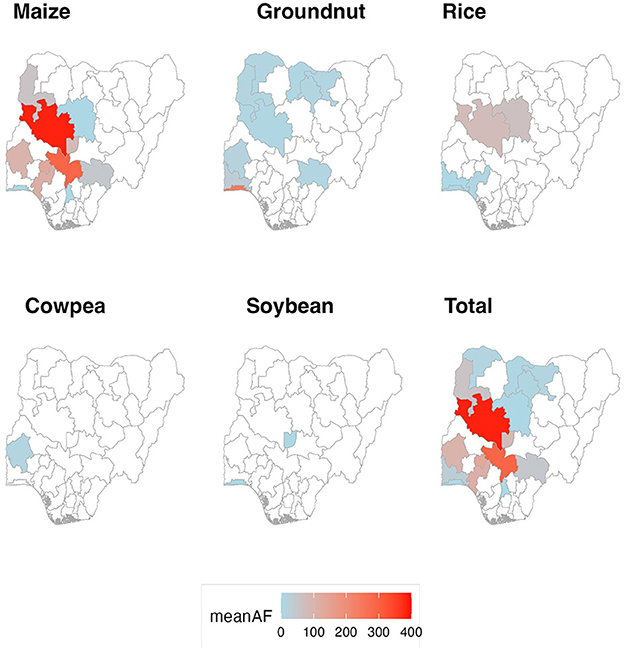
Figure 3. Geospatial overview of aflatoxin contamination reported in reviewed literature, reflecting sampling effort and detected levels.
3.2. Exposure levels and risk assessment
The probability distribution of the EDI was estimated for each commodity based on the distributions of aflatoxin concentrations, commodity consumption, and adult body weight. There is no tolerable daily intake (TDI) level for aflatoxin, because it has been classified as a Class 1 carcinogen, and thus exposure is considered unsafe at any dose (van de Perre et al., 2015; Saha Turna and Wu, 2022). However, a proposed provisional maximum tolerable daily intake level (PMTDI) of 1 ng/kg bw/day has been used as a reference value for judging risk associated with aflatoxin exposures (Kuiper-Goodman, 1998; Magrine et al., 2011). The commodity with the highest estimated EDI in the exposure model was maize, with a value of 36.7 (95% CI: [22.7–54.8]) ng/kg bw/day, substantially higher than the PMTDI level. The estimate of EDI in the groundnut, rice and cowpea models also exceeded the PMTDI level, but more modestly, with estimates of 9.12 (95% CI: [4.41–19.21]), 8.85 (95% CI: [6.91–11.09]), and 3.14 (95% CI: [1.25–7.22]) ng/kg bw/day, respectively. Dietary aflatoxin exposure attributable to soybean consumption in the study population is much less than the other commodities, with an EDI of 0.28 (95% CI: [0.24–0.32]) ng/kg bw/day, below the PMTDI level. The mean risk of HCC attributable to consumption of maize, groundnut, rice, cowpea, and soybean in the target population was estimated as 1.77 (95% CI: [1.10–2.65]), 0.44 (95% CI: [0.21–0.93]), 0.43 (95% CI: [0.33–0.54]), 0.15 (95% CI: [0.06–0.35]), and 0.01 (95% CI: [0.01–0.02]) HCC cases per year/100,000 population, respectively.
Sensitivity analysis of the relative impacts of variability in the model's input parameters on HCC risk indicated that variability in aflatoxin concentration (C) in a consumed portion has the greatest impact on HCC risk attributable to maize consumption (Spearman's ρ = 0.64), followed closely by variability in the frequency of maize consumption (ρ = 0.58). Variability in S, i, and BW had comparatively low impact on risk in the maize model. In the rice model, the frequency of rice consumption (fcons) had a very large impact on the level of HCC risk (ρ = 1.00), whereas estimates for the other parameters did not exceed 0.1. In both the cowpea and soybean models of HCC risk, the most impactful parameter on risk level was the frequency of contamination (i), for which Spearman's ρ was estimated at 0.97 and 1.00 for cowpea and soybean, respectively, indicating a near-perfect correlation. While this suggests that contamination frequency may be a contributory factor, it should be acknowledged that the estimated exposure attributable to these commodities was relatively low, and that the limited data available for these commodities may have obscured contributions from other factors in the sensitivity analysis.
4. Discussion
This study presents novel evidence that dietary consumption of several key commodities found in Nigerian traditional food marketplaces, particularly in the northern regions, may lead to aflatoxin exposures sufficient to cause adverse health outcomes. HCC incidence rate in Nigeria, from all causes, was previously estimated as 2.6 cases per 100,000 individuals (Kedar Mukthinuthalapati et al., 2021); thus, our findings suggest that a substantial proportion of total HCC incidence in the region may be attributable to dietary aflatoxin exposure via the consumption of the considered commodities, particularly maize and groundnut.
The estimates presented here are above the chosen PMTDI levels but below prior risk estimates for aflatoxin-associated HCC attributable to single-commodity consumption for infants and children in Nigeria (Adetunji et al., 2017). The relative ranking of these products observed in this study is consistent with existing evidence that cereals (maize and groundnuts in particular) are more susceptible to aflatoxin accumulation than pulses. For both maize and rice in the study area, the higher estimated risk levels are reflective of both high consumption levels and high contamination levels, whereas cowpea and soybean, despite moderate consumption levels in the population, harbor low concentrations of aflatoxin and therefore pose lower risk of aflatoxin-associated HCC. Despite having aflatoxin concentrations comparable to those of maize samples, the HCC risk associated with groundnut consumption is relatively lower, owing to low overall levels of groundnut consumption in the target population.
Moreover, while the present estimate of maize-associated HCC risk is very similar to what was estimated for aflatoxin exposure via groundnuts in a Nigerian population (1.38 HCC cases year−1 100,000 pop−1) (Adetunji et al., 2018), the estimate of risk associated with groundnut consumption in the present study was substantially lower. The discrepancy in risk associated with groundnut consumption between the present study and the study conducted by Adetunji et al. could be attributable to the fact that point estimates, rather than probability distributions, of groundnut consumption and aflatoxin concentration were used in their exposure assessment, and that detection rates were not accounted for. This illustrates the potential utility of an exposure modeling approach that accounts for population-specific probability distributions in exposure-related variables. Another Nigerian study (Garba et al., 2021) estimated AFB1-associated HCC risk levels much higher (3.06 cases per 100,000 population) than what was observed in this study; this illustrates the importance of taking a multi-commodity approach in risk assessment.
The risk estimates presented here are on par with other estimates in Nigeria, but they are higher than what was observed in a European population with lower maize consumption and contamination levels (Udovicki et al., 2021). This is consistent with the observation that our EDI estimates for maize and groundnut alone far exceed the average upper bound exposure level for adults reported by EFSA [EFSA Panel on Contaminants in the Food Chain (CONTAM), 2020]. In many sub-Saharan African food system contexts, optimal conditions for fungal growth and aflatoxin accumulation are often coupled with poor food monitoring and regulatory systems. In Nigeria, regulation and enforcement of food safety policy and standards is impeded by outdated infrastructure and insufficient expertise (Okoruwa and Onuigbo-Chatta, 2021).
While the present study offers important insights into the exposure and health risks associated with aflatoxin contamination of key foods in Nigerian traditional food marketplaces, several data gaps and limitations need to be considered. First, the models presented in this study were populated using secondary data sources, which necessitates some assumptions about their applicability to the target population. For instance, data from different Nigerian states were pooled together, unweighted—hence implicitly weighted by sampling effort in reported literature; estimates should therefore be considered representative of current evidence in the country, but not of specific regions or states or of the country as a whole. The systematic review methodology used to compile comprehensive regional aflatoxin contamination data was sufficient for commodities with well-established aflatoxin literature (i.e., maize, groundnut, and rice), but was much more limited for cowpea and soybean, which have scarcer published evidence on aflatoxin contamination levels, in all sub-Saharan Africa. Further risk assessments using primary data from more susceptible commodities, and applying context-specific sampling, would contribute invaluably to the knowledge base around aflatoxin exposure risk in specific regions, as the basis for targeted interventions.
Second, the heterogeneity in reporting formats for aflatoxin prevalence and concentrations hinders the characterization of concentration distributions in meta-analysis. An approach based on batch means was implemented here, capturing the variability across batches, while deprioritizing within-batch variability due to data limitations. In addition, some assumptions were made on consumption amounts and body weight, in the absence of more precise national consumption data. Overall, data were sufficient to support a sound preliminary assessment, but estimates are less accurate than assessments conducted using primary data. Following agreed-upon best reporting practices for aflatoxin detection rate, limits of detection, and concentrations would improve estimate accuracy. However, the level of detail included in this study—if applicable to the specific local context—is sufficient to inform intervention decisions and to highlight critical data gaps that prevent such decisions (e.g., here for cowpea or soybean and derived foods).
Third, this study focused specifically on risk of HCC, which is just one of several possible health outcomes of interest (Gong et al., 2016). HCC was selected based on its severity and its relatively well-defined dose-response relationship with aflatoxin. However, aflatoxin-associated malnutrition and growth impairment outcomes are increasingly a focus of the research community and are critically in need of comprehensive risk assessment as studies to date have not conclusively shown a causal linkage (Turner et al., 2007; Leroy et al., 2018) and the biological mechanisms have not been elucidated (Tessema et al., 2021). As these outcomes often arise as a result of several environmental factors in combination (Moore et al., 2001), it is challenging (and ethically dubious) to articulate dose-response relationships for these outcomes (Turner, 2013).
Our findings indicate substantial reason for concern regarding the risk of aflatoxin exposure in Nigeria, but this is set against a backdrop of promising management efforts in the country that have potential for further scalability and contextualization. For example, Nigeria has been a pioneer in Africa in the development and use of biocontrol agents to combat aflatoxin-producing fungi (Bandyopadhyay et al., 2019; Ortega-Beltran and Bandyopadhyay, 2021). Additionally, value chain approaches to mycotoxin management have been initiated in the country. For example, one recent study found that aflatoxin-safe labeling programs influence willingness to pay for actors across the value chain and may incentivize consumption of certified aflatoxin-safe products (Sanou et al., 2021). These efforts and others illustrate that there are practical control strategies in place that could leverage risk assessments to ensure that intervention is appropriately directed to populations with the greatest need.
Overall, this study provides a comprehensive quantitative synthesis of published evidence (as of 2022) on aflatoxin contamination in key staple commodities in Nigeria, national-level exposure and risk estimates based on such data, and a novel approach leveraging secondary data that can be adapted by other researchers to conduct similar assessments. Findings point to a non-negligible potential HCC risk associated with maize and groundnut, in alignment with previous estimates that highlight the specific contributions of maize and groundnut to HCC risk. Clear data gaps exist for cowpea and soybean, preventing a meaningful risk assessment; however, because of moderate consumption and less implication in aflatoxin exposure, cowpea and soybean are of less concern than maize and groundnut. The heterogeneity in aflatoxin levels, and hence risk estimates, across the country warrants attention if using these findings to inform localized intervention decisions; complementing country-scale findings with local primary data is encouraged.
Data availability statement
The original contributions presented in the study are included in the article/Supplementary material, further inquiries can be directed to the corresponding author.
Author contributions
AW and EL designed the study and developed the methodology. AW synthesized input data, coded, and run the model. EL oversaw all study steps and provided guidance. FM, LT, and DG provided input on study approach, input data, and result interpretation. AW led manuscript writing and graphics, with inputs and review from EL. All authors reviewed the final draft. All authors contributed to the article and approved the submitted version.
Funding
This document was made possible through support provided by Feed the Future through the U.S. Agency for International Development (USAID), under the terms of Agreement #7200AA19CA00010.
Acknowledgments
The authors are grateful for the review and feedback provided by Richard Pluke, David Atamewalen, and Abigail Reich of GAIN, and two reviewers. The opinions expressed herein are those of the Global Alliance for Improved Nutrition (GAIN) and do not necessarily reflect the views of USAID or the United States Government. This study is part of the EatSafe program, a collaboration between GAIN, USAID, the International Livestock Research Institute (ILRI), Pierce Mill Entertainment and Education, and the Busara Center for Behavioral Economics. For more details on EatSafe program activities, visit gainhealth.org/EatSafe or contact EatSafe@gainhealth.org.
Conflict of interest
The authors declare that the research was conducted in the absence of any commercial or financial relationships that could be construed as a potential conflict of interest.
Publisher's note
All claims expressed in this article are solely those of the authors and do not necessarily represent those of their affiliated organizations, or those of the publisher, the editors and the reviewers. Any product that may be evaluated in this article, or claim that may be made by its manufacturer, is not guaranteed or endorsed by the publisher.
Supplementary material
The Supplementary Material for this article can be found online at: https://www.frontiersin.org/articles/10.3389/fsufs.2023.1128540/full#supplementary-material
References
Adebajo, L. O., Idowu, A. A., and Adesanya, O. O. (1994). Mycoflora, and mycotoxins production in Nigerian corn and corn-based snacks. Mycopathologia 126, 183–192. doi: 10.1007/BF01103774
Adefolalu, F. S., Apeh, D. O., Salubuyi, S. B., Galadima, M., Agbo, A., Anthony, M. O., et al. (2022). Quantitative appraisal of total aflatoxin in ready-to-eat groundnut in north-central Nigeria. J. Chem. Health Risks 12, 25–31. doi: 10.22034/jchr.2021.1911495.1196
Adejumo, O., Atanda, O., Raiola, A., Somorin, Y., Bandyopadhyay, R., Ritieni, A., et al. (2013). Correlation between aflatoxin M1 content of breast milk, dietary exposure to aflatoxin B1 and socioeconomic status of lactating mothers in Ogun State, Nigeria. Food Chem. Toxicol. 56, 171–177. doi: 10.1016/j.fct.2013.02.027
Adetunji, M. C., Alika, O. P., Awa, N. P., Atanda, O. O., and Mwanza, M. (2018). Microbiological quality and risk assessment for aflatoxins in groundnuts and roasted cashew nuts meant for human consumption. J Toxicol. 2018, 1–11. doi: 10.1155/2018/1308748
Adetunji, M. C., Atanda, O. O., and Ezekiel, C. N. (2017). Risk Assessment of Mycotoxins in Stored Maize Grains Consumed by Infants and Young Children in Nigeria. Children 4, 58. doi: 10.3390/children4070058
Adewale, J. G. (2005). Socio-economic determinants of consumption of soybean products in Nigeria: a case study of Oyo State, Nigeria. Anthropologist 7, 57–60. doi: 10.1080/09720073.2005.11890884
Afolabi, C. G., Ezekiel, C. N., Kehinde, I. A., Olaolu, A. W., and Ogunsanya, O. M. (2015). Contamination of groundnut in South-Western Nigeria by Aflatoxigenic Fungi and Aflatoxins in relation to processing. J. Phytopathol. 163, 279–286. doi: 10.1111/jph.12317
Afolabi, C. G., Ezekiel, C. N., Ogunbiyi, A. E., Oluwadairo, O. J., Sulyok, M., Krska, R., et al. (2019). Fungi and mycotoxins in cowpea (Vigna unguiculata L.) on Nigerian markets. Food Addit. Contaminants Part B 13, 52–58. doi: 10.1080/19393210.2019.1690590
Akinpelu, A., Oyewole, O., and Adekanla, B. (2015). Body size perceptions and weight status of adults in a nigerian rural community. Ann. Med. Health Sci. Res. 5, 358. doi: 10.4103/2141-9248.165247
Akuso, O. M., and Kiin-Kabari, D. B. (2012). Protein quality and sensory evaluation of moin-moin prepared from cowpea/maize flour blends. African J. Food Sci. 6, 47–51. doi: 10.5897/AJFS11.125
Andrade, P. D., and Caldas, E. D. (2015). Aflatoxins in cereals: worldwide occurrence and dietary risk Assessment. World Mycotoxin J. 8, 415–431. doi: 10.3920/WMJ2014.1847
Atawodi, S. E., Atiku, A. A., and Lamorde, A. G. (1994). Aflatoxin contamination of Nigerian foods and feedingstuffs. Food Chem. Toxicol. 32, 61–63. doi: 10.1016/0278-6915(84)90038-3
Atehnkeng, J., Ojiambo, P. S., Ikotun, T., Sikora, R. A., Cotty, P. J., Bandyopadhyay, R., et al. (2008). Evaluation of atoxigenic isolates of Aspergillus flavus as potential biocontrol agents for aflatoxin in maize. Food Addit Contaminants Part A 25, 1264–1271. doi: 10.1080/02652030802112635
Awuchi, C. G., Owuamanam, I. C., Ogueke, C. C., and Hannington, T. (2020). The impacts of mycotoxins on the proximate composition and functional properties of grains. Eur Acad Res 8, 1024–1071.
Ayejuyo, O., Olowu, R., Agbaje, T., Atamenwan, M., and Osundiya, M. (2011). Enzyme - linked immunosorbent assay (Elisa) of aflatoxin b1 in groundnut and cereal grains in Lagos, Nigeria. Res. J. Chem. Sci. 1, 1–5.
Ayeni, K. I., Akinyemi, O. M., Kovac, T., and Ezekiel, C. N. (2020). Aflatoxin contamination of maize vended in Ondo state, Nigeria, and health risk assessment. Croatian J. Food Sci. Technol. 12, 123–129. doi: 10.17508/CJFST.2020.12.1.16
Bandyopadhyay, R., Atehnkeng, J., Ortega-Beltran, A., Akande, A., Falade, T. D. O., Cotty, P. J., et al. (2019). “Ground-truthing” efficacy of biological control for aflatoxin mitigation in farmers' fields in Nigeria: from field trials to commercial usage, a 10-year study. Front. Microbiol. 10, 2528. doi: 10.3389/fmicb.2019.02528
Bankole, S. A., and Mabekoje, O. O. (2007). Occurrence of aflatoxins and fumonisins in preharvest maize from South-Western Nigeria. Food Addit. Contam. 21, 251–255. doi: 10.1080/02652030310001639558
Bankole, S. A., Ogunsanwo, B. M., and Eseigbe, D. A. (2005). Aflatoxins in Nigerian dry-roasted groundnuts. Food Chem. 89, 503–506. doi: 10.1016/j.foodchem.2004.03.004
Chilaka, C. A., Obidiegwu, J. E., Chilaka, A. C., Atanda, O. O., and Mally, A. (2022). Mycotoxin regulatory status in Africa: a decade of weak institutional efforts. Toxins. 14, 442. doi: 10.3390/toxins14070442
Delignette-Muller, M. L., and Dutang, C. (2015). Fitdistrplus: an R package for fitting distributions. J. Stat. Softw. 64, 1–34. doi: 10.18637/jss.v064.i04
EFSA Panel on Contaminants in the Food Chain (CONTAM) Schrenk D, Bignami M, Bodin L, Chipman JK, del Mazo J. (2020). Risk assessment of aflatoxins in food. EFSA Journal 18, e06040. doi: 10.2903/j.efsa.2020.6040
Ekhuemelo, C., and Abu, S. O. (2019). Evaluation of the aflatoxin contamination and proximate composition of groundnut (Arachis hypogea L.) infected by Aspergillus spp. Niger J Biotechnol 35, 130–138. doi: 10.4314/njb.v35i2.16
Ekpakpale, D. O., Kraak, B., Meijer, M., Ayeni, K. I., Houbraken, J., Ezekiel, C. N., et al. (2021). Fungal diversity and aflatoxins in maize and rice grains and cassava-based flour (Pupuru) from Ondo state, Nigeria. J. Fungi 7, 635. doi: 10.3390/jof7080635
Ezekiel, C. N., Ayeni, K. I., Akinyemi, M. O., Sulyok, M., Oyedele, O. A., Babalola, D. A., et al. (2021). Dietary risk assessment and consumer awareness of mycotoxins among household consumers of cereals, nuts and legumes in north-central Nigeria. Toxins. 13, 635. doi: 10.3390/toxins13090635
Ezekiel, C. N., Sulyok, M., Babalola, D. A., Warth, B., Ezekiel, V. C., Krska, R., et al. (2013). Incidence and consumer awareness of toxigenic Aspergillus section Flavi and aflatoxin B1 in peanut cake from Nigeria. Food Control. 30, 596–601. doi: 10.1016/j.foodcont.2012.07.048
Fadupin, G. T. (2009). Food exchange lists of local foods in Nigeria. Afr J Diabetes Med. 419, 15–18.
Fapohunda, S. O., Anjorin, T. S., Sulyok, M., and Krska, R. (2018). Profile of major and emerging mycotoxins in sesame and soybean grains in the Federal Capital Territory, Abuja, Nigeria. Eur J Biol Res 8, 121–130. doi: 10.5281/zenodo.1307184
Garba, M., Makun, H., Jigam, A., Hadiza, L., AbdulRasheed-Adeleke, T., and Njobeh, P. (2021). Risk assessment on the aflatoxin-induced hepatocellular carcinoma in communities subsisting on sorghum products in the sudan Savannah Zone of Nigeria. Dutse J. Pure Appl. Sci. 7, 155–166.
Gong, Y. Y., Watson, S., and Routledge, M. N. (2016). Aflatoxin exposure and associated human health effects, a review of epidemiological studies. Food Safety 4, 14–27. doi: 10.14252/foodsafetyfscj.2015026
Grace, D. (2015). Food safety in low and middle income countries. Int. J. Environ. Res. Public Health 12, 10490–10507. doi: 10.3390/ijerph120910490
Hoffmann, V., Jones, K., and Leroy, J. L. (2018). The impact of reducing dietary aflatoxin exposure on child linear growth: a cluster randomised controlled trial in Kenya. BMJ Glob Health 3, e000983. doi: 10.1136/bmjgh-2018-000983
Houssou, P. A., Ahohuendo, B. C., Fandohan, P., Kpodo, K., Hounhouigan, D. J., Jakobsen, M., et al. (2009). Natural infection of cowpea (Vigna unguiculata (L.) Walp.) by toxigenic fungi and mycotoxin contamination in Benin, West Africa. J. Stored Prod. Res. 45, 40–44. doi: 10.1016/j.jspr.2008.07.002
Ibeh, I. N., Uraih, N., and Ogonor, J. I. (1991). Dietary exposure to aflatoxin in Benin City, Negeria: a possible public health concern. Int. J. Food Microbiol. 14, 171–174. doi: 10.1016/0168-1605(91)90104-W
JECFA (1999). Evaluation of certain food additives and contaminants : forty-ninth report of the Joint FAO/WHO Expert Committee on Food Additives. Available online at: https://apps.who.int/iris/handle/10665/42142 (accessed December 2, 2022).
Jimoh, K. O., and Kolapo, A. L. (2008). Mycoflora and aflatoxin production in market samples of some selected Nigerian foodstuffs. Res. J. Microbiol. 3, 169–174. doi: 10.3923/jm.2008.169.174
Kabak, B., and Var, I. (2008). Factors affecting the removal of aflatoxin M1 from food model by Lactobacillus and Bifidobacterium strains. J Environ Sci Health Part B. 43, 617–624. doi: 10.1080/03601230802234740
Kedar Mukthinuthalapati, V. V. P., Sewram, V., Ndlovu, N., Kimani, S., Abdelaziz, A. O., Chiao, E. Y., et al. (2021). Hepatocellular Carcinoma in Sub-Saharan Africa. JCO Glob Oncol 7, 756–766. doi: 10.1200/GO.20.00425
Keta, J., Aliero, A., and Suberu, H. (2019). Aflatoxin content of cereal grains in Kebbi State, Nigeria. J. Innovat. Res. Life Sci. 1, 62.
Keta, J. N., Aliero, A. A., Shehu, K., Suberu, H. A., Mohammed, N. K., Abdulkadir, B., et al. (2019). Incidence of Fungal flora and Aflatoxin content of millet and maize cereal grains sold in Guinea savanna zones of Kebbi State. Sci. World J. 14, 12–15.
Khlangwiset, P., Shephard, G. S., and Wu, F. (2011). Aflatoxins and growth impairment: a review. Crit. Rev. Toxicol. 41, 740–755. doi: 10.3109/10408444.2011.575766
Kuiper-Goodman, T. (1998). “Food safety: mycotoxins and phycotoxins in perspective,” in M. Miraglia, H. P. van Egmond, C. Brera, and J. Gilbert, eds Mycotoxins and Phycotoxins: Developments in Chemistry, Toxicology and Food Safety Mycotoxins and phycotoxins (Fort Collins: ALAKEN), p. 25–48.
Leroy, J. L., Sununtnasuk, C., García-Guerra, A., and Wang, J. S. (2018). Low level aflatoxin exposure associated with greater linear growth in southern Mexico: a longitudinal study. Matern. Child Nutr. 14, e12619. doi: 10.1111/mcn.12619
Liu, Y., and Wu, F. (2010). Global burden of aflatoxin-induced hepatocellular carcinoma: a risk assessment. Environ. Health Perspect. 118, 818–824. doi: 10.1289/ehp.0901388
Liverpool-Tasie, L. S. O., Turna, N. S., Ademola, O., Obadina, A., and Wu, F. (2019). The occurrence and co-occurrence of aflatoxin and fumonisin along the maize value chain in southwest Nigeria. Food Chem. Toxicol. 129, 458–465. doi: 10.1016/j.fct.2019.05.008
Magrine, I. C. O., Ferrari, S. S. C., Souza, G. F., Minamihara, L., Kemmelmeier, C., Bando, E., et al. (2011). Intake of aflatoxins through the consumption of peanut products in Brazil. Food Addit. Contaminants Part B 4, 99–105. doi: 10.1080/19393210.2011.561931
Makun, H., Ojochenemi, A. D., Adeyemi, H. R. Y., Nagago, T., Okeke, J. O., Mustapha, A. S., et al. (2014). Determination of aflatoxins in sesame, rice, millet and acha from Nigeria using HPLC. Chem. Sci. Trans. 3, 1516–1524. doi: 10.7598/cst2014.749
Makun, H. A., Dutton, M. F., Njobeh, P. B., Mwanza, M., and Kabiru, A. Y. (2011). Natural multi-occurrence of mycotoxins in rice from Niger State, Nigeria. Mycotoxin Res. 27, 97–104. doi: 10.1007/s12550-010-0080-5
Massomo, S. M. S. (2020). Aspergillus flavus and aflatoxin contamination in the maize value chain and what needs to be done in Tanzania. Sci Afr. 10, e00606. doi: 10.1016/j.sciaf.2020.e00606
Maziya-Dixon, B. (2004). Nigeria Food Consumption and Nutrition Survey 2001–2003: Summary. Ibadan, Nigeria: IITA.
Mbaawuaga, E. M., Nwabude, J. O., Shiriki, D., Mbaawuaga, E. M., Nwabude, J. O., Shiriki, D., et al. (2020). Effects of farmers' practices on Maize (Zea mays) contamination by potential Aflatoxigenic Fungi and Aflatoxin in Benue State, Nigeria. Agri. Sci. 11, 500–513. doi: 10.4236/as.2020.115031
Moore, S. E., Collinson, A. C., and Prentice, A. M. (2001). Immune function in rural Gambian children is not related to season of birth, birth size, or maternal supplementation status. Am. J. Clin. Nutr. 74, 840–847. doi: 10.1093/ajcn/74.6.840
Niyibituronsa, M., Onyango, A., Gaidashova, S., Imathiu, S., Uwizerwa, M., Wanjuki, I., et al. (2019). Evaluation of mycotoxin content in soybean (Glycine max l.) grown in Rwanda. Afr. J. Food Agric. Nutr. Develop. 18, 13808–13824.
Nkafamiya, I. I., Maina, H. M., Osemeahon, S. A., and Modibbo, U. U. (2010). Percentage oil yield and physiochemical properties of different groundnut species (Arachis hypogaea). African J. Food Sci. 4, 418–421.
Noh, E. J., Park, S. Y., Pak, J. I., Hong, S. T., and Yun, S. E. (2005). Coagulation of soymilk and quality of tofu as affected by freeze treatment of soybeans. Food Chem. 91, 715–721. doi: 10.1016/j.foodchem.2004.06.050
Odeniyi, O., Adebayo-Tayo, B., and Olasehinde, K. (2019). Mycological, toxigenic and nutritional characteristics of some vended groundnut and groundnut products from three Northern Nigerian ecological zones. African J. Biomed. Res. 22, 65–71. doi: 10.4314/ajbr.v22i1
Odoemelam, S. A., and Osu, C. I. (2009). Aflatoxin B1 contamination of some edible grains marketed in Nigeria. J. Chem. 6, 308–314. doi: 10.1155/2009/708160
Odogwu, B. A., Uzogara, M. O., Worlu, H., and Agbagwa, I. O. (2021). Factors affecting stakeholders' preferences for cowpea grains in selected parts of Nigeria. African J. Food Agric. Nutr. Develop. 21, 17669–17681. doi: 10.18697/ajfand.98.19890
Ogungbemile, O. A., Etaware, P. M., and Odebode, A. C. Aflatoxin detection quantification in stored cowpea seeds in Ibadan, Nigeria. J. Biotechnol. Biomed. (2020). 3, 10–17.
Ogunsanwo, B. M., Faboya, O. O., Ikotun, T., and Idowu, R. (1989). Fate of aflatoxins in soybeans during the preparation of “Soyogi”. Food Nahrung. 33, 485–487. doi: 10.1002/food.19890330530
Okoruwa, A., and Onuigbo-Chatta, N. (2021). Review of food safety policy in Nigeria. J. Law Policy Globaliz. 110, 57. doi: 10.7176/JLPG/110-07
Olayiwola, I. O., Oganah, B. C., Onabanjo, O. O., Oguntona, C. R. B., Popoola, A. R., Sanni, S. A., et al. (2012). Consumption pattern of maize-based dished in four agro-ecological zones of Nigeria. J. Agric. Sci. Environ. 12, 45–61.
Olorunmowaju, Y. B., Makun, H. A., Muhammad, H. L., Adeyemi, H. R. N., Ifeji, E., Muhammad, H. K., et al. (2014). Simultaneous occurrence of aflatoxin and ochratoxin a in rice from Kaduna State, Nigeria. Mycotoxicol. Soc. Nigeria Mycotoxicol. 1, 46–56.
Onilude, A. A., Wakil, S. M., Odeniyi, A. O., Fawole, A., Garuba, E. O., and Ja'afaru, I. M. (2012). Distribution of aflatoxin and aflatoxigenic, and other toxigenic fungi in maize samples marketed in Ibadan, Oyo State, Nigeria. Electronic J. Environ. Agric. Food Chem. 11, 148–155.
Onyedum, S. C., Adefolalu, F. S., Muhammad, H. L., Apeh, D. O., Agada, M. S., Imienwanrin, M. R., et al. (2020). Occurrence of major mycotoxins and their dietary exposure in North-Central Nigeria staples. Sci Afr 7, e00188. doi: 10.1016/j.sciaf.2019.e00188
Ortega-Beltran, A., and Bandyopadhyay, R. (2021). Contributions of integrated aflatoxin management strategies to achieve the sustainable development goals in various African countries. Glob. Food Sec. 30, 100559. doi: 10.1016/j.gfs.2021.100559
Oyedele, O. A., Ezekiel, C. N., Sulyok, M., Adetunji, M. C., Warth, B., Atanda, O. O., et al. (2017). Mycotoxin risk assessment for consumers of groundnut in domestic markets in Nigeria. Int. J. Food Microbiol. 251, 24–32. doi: 10.1016/j.ijfoodmicro.2017.03.020
Oyeka, C. A., Amasiani, R. N., and Ekwealor, C. C. (2019). Mycotoxins contamination of maize in Anambra State. Nigeria. 12, 280–288. doi: 10.1080/19393210.2019.1661528
Perrone, G., Haidukowski, M., Stea, G., Epifani, F., Bandyopadhyay, R., Leslie, J. F., et al. (2014). Population structure and Aflatoxin production by Aspergillus Sect. Flavi from maize in Nigeria and Ghana. Food Microbiol. 41, 52–59. doi: 10.1016/j.fm.2013.12.005
Rofiat, A. S., Fanelli, F., Atanda, O., Sulyok, M., Cozzi, G., Bavaro, S., et al. (2015). Fungal and bacterial metabolites associated with natural contamination of locally processed rice (Oryza sativa L.) in Nigeria. Food Additiv. Contaminants Part A 32, 950–959. doi: 10.1080/19440049.2015.1027880
Saha Turna, N., and Wu, F. (2022). Estimation of tolerable daily intake (TDI) for immunological effects of aflatoxin. Risk Anal. 42, 431–438. doi: 10.1111/risa.13770
Sanou, A., Liverpool-Tasie, L. S. O., Caputo, V., and Kerr, J. (2021). Introducing an aflatoxin-safe labeling program in complex food supply chains: evidence from a choice experiment in Nigeria. Food Policy 102, 102070. doi: 10.1016/j.foodpol.2021.102070
Sanusi, R., and Olurin, A. (2014). Portion and serving sizes of commonly consumed foods, in Ibadan, Southwestern Nigeria. African J. Biomed. Res. 15, 149–158. doi: 10.4314/ajbr.v15i3
Shehu, K., Salau, I. A., and Salisu, N. (2020). Fungal and Mycotoxin contamination of stored maize grains in Kebbi state, North-Western Nigeria. J. Adv. Botany Zool. 8, 4–8.
Sirma, A., Makita, K., Grace Randolph, D., Senerwa, D., and Lindahl, J. (2019). Aflatoxin exposure from milk in rural Kenya and the contribution to the Risk of Liver cancer. Toxins 11, 469. doi: 10.3390/toxins11080469
Smith, L. E., Stoltzfus, R. J., and Prendergast, A. (2012). Food chain mycotoxin exposure, gut health, and impaired growth: a conceptual framework. Adv Nutr. 3, 526–531. doi: 10.3945/an.112.002188
Tessema, M., Groote, d. e., Brouwer, H., de Boevre, I. D., Corominas, M., Stoecker, A. V., et al. (2021). Exposure to aflatoxins and fumonisins and linear growth of children in rural Ethiopia: a longitudinal study. Public Health Nutr. 24, 3662–3673. doi: 10.1017/S1368980021000422
Turner, P. C. (2013). The molecular epidemiology of chronic aflatoxin driven impaired child growth. Scientifica 2013, 1–21. doi: 10.1155/2013/152879
Turner, P. C., Collinson, A. C., Cheung, Y. B., Gong, Y., Hall, A. J., Prentice, A. M., et al. (2007). Aflatoxin exposure in utero causes growth faltering in Gambian infants. Int. J. Epidemiol. 36, 1119–1125. doi: 10.1093/ije/dym122
Udovicki, B., Tomic, N., Trifunovic, B. S., Despotovic, S., Jovanovic, J., Jacxsens, L., et al. (2021). Risk assessment of dietary exposure to aflatoxin B1 in Serbia. Food Chem. Toxicol. 151, 112116. doi: 10.1016/j.fct.2021.112116
Usman, I., Taiwo, A. B., Haratu, D., and Abubakar, M. A. (2013). Socio-economic factors affecting groundnut production in Sabongari Local Government of Kaduna State, Nigeria. Int. J. Food Agric. Econ. 1, 41–48. doi: 10.22004/AG.ECON.156141
Vabi, M. B., Eche, C. O., Ogara, M. I., Ajeigbe, H. A., and Kasim, A. A. (2020). Prevalence and distribution of aflatoxin (AfB1) in groundnut and groundnut-based products in Northwestern Nigeria. J. Toxicol. Environ. Health Sci. 12, 27–35. doi: 10.5897/JTEHS2020.0458
van de Perre, E., Jacxsens, L., Lachat, C., el Tahan, F., and de Meulenaer, B. (2015). Impact of maximum levels in European legislation on exposure of mycotoxins in dried products: case of aflatoxin B1 and ochratoxin A in nuts and dried fruits. Food Chem. Toxicol. 75, 112–117. doi: 10.1016/j.fct.2014.10.021
Wartu, J. R. (2021). Mycology and effects of dehauling local Oryza Sativa for human consumption on total aflatoxins within some parts of Kaduna metropolis. Sci. World J. 14, 89–92.
Keywords: aflatoxin, risk assessment, exposure, LMIC, mycotoxins, food safety, Nigeria, Africa
Citation: Wenndt A, Mutua F, Grace D, Thomas LF and Lambertini E (2023) Quantitative assessment of aflatoxin exposure and hepatocellular carcinoma (HCC) risk associated with consumption of select Nigerian staple foods. Front. Sustain. Food Syst. 7:1128540. doi: 10.3389/fsufs.2023.1128540
Received: 20 December 2022; Accepted: 25 January 2023;
Published: 01 March 2023.
Edited by:
Hani El-Nezami, The University of Hong Kong, Hong Kong SAR, ChinaReviewed by:
Luis Abrunhosa, University of Minho, PortugalWasiu Awoyale, Kwara State University, Nigeria
Copyright © 2023 Wenndt, Mutua, Grace, Thomas and Lambertini. This is an open-access article distributed under the terms of the Creative Commons Attribution License (CC BY). The use, distribution or reproduction in other forums is permitted, provided the original author(s) and the copyright owner(s) are credited and that the original publication in this journal is cited, in accordance with accepted academic practice. No use, distribution or reproduction is permitted which does not comply with these terms.
*Correspondence: Elisabetta Lambertini, elambertini@gainhealth.org